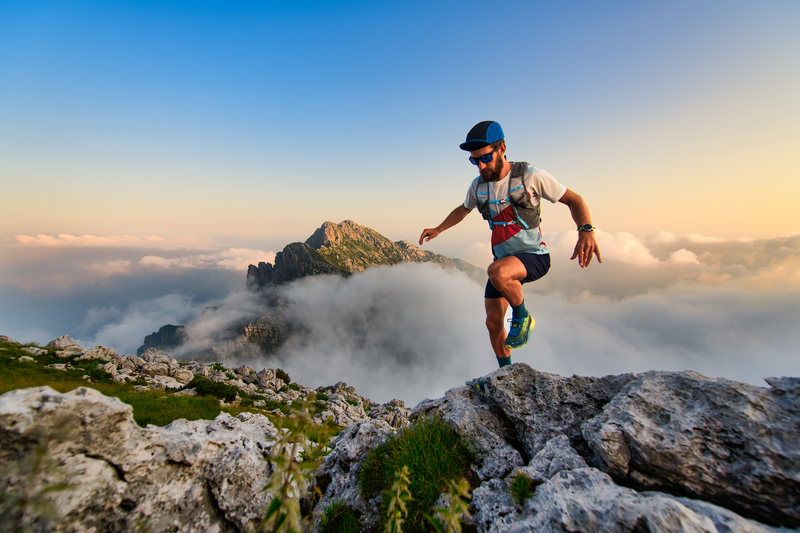
94% of researchers rate our articles as excellent or good
Learn more about the work of our research integrity team to safeguard the quality of each article we publish.
Find out more
REVIEW article
Front. Neurosci. , 23 June 2020
Sec. Neuroprosthetics
Volume 14 - 2020 | https://doi.org/10.3389/fnins.2020.00528
Neurofeedback (NF) and brain-computer interface (BCI) applications rely on the registration and real-time feedback of individual patterns of brain activity with the aim of achieving self-regulation of specific neural substrates or control of external devices. These approaches have historically employed visual stimuli. However, in some cases vision is unsuitable or inadequately engaging. Other sensory modalities, such as auditory or haptic feedback have been explored, and multisensory stimulation is expected to improve the quality of the interaction loop. Moreover, for motor imagery tasks, closing the sensorimotor loop through haptic feedback may be relevant for motor rehabilitation applications, as it can promote plasticity mechanisms. This survey reviews the various haptic technologies and describes their application to BCIs and NF. We identify major trends in the use of haptic interfaces for BCI and NF systems and discuss crucial aspects that could motivate further studies.
Over the past decade, advances in brain science and computer technology have led to a growth in the development of neurofeedback (NF) and brain-computer interface (BCI) applications. Recent technological advances, such as machine learning analyses, wireless applications, and real-time neural recordings, have increased interest in NF and BCI approaches, especially EEG-based NF/BCIs. One of the cornerstones of NF and BCIs is the feedback given to the subject, which the subject uses to learn and improve their mental strategy. Traditionally, BCI/NF applications have mostly employed visual feedback, but its use may be questionable in some cases. For example, visual feedback is not always suitable for individuals with an impaired visual system or during a mental motor imagery task, which requires great abstraction from the subject. In such situations, tactile feedback would seem more appropriate and more intuitive than visual feedback (Cincotti et al., 2007b). However, haptic feedback is more often used in conjunction with visual feedback to provide enriched information to the user.
Visual feedback has been shown to be the type of sensory input that produces the best learning processes (Hinterberger et al., 2004). However, up until now, other feedback modalities have been explored less, even though specific circumstances may require differential feedback due to the particular pathology or requirements of the rehabilitation process, e.g., for locked-in patients (Sollfrank et al., 2016). Moreover, it has been suggested that providing haptic feedback could improve the subject's sense of agency, a technology acceptance-related factor, in motor imagery (MI) BCIs (Thurlings et al., 2012). Preliminary studies have shown that BCI performance is not affected by the specific type of feedback (Brouwer and van Erp, 2010), whether visual, auditory, or haptic. Nevertheless, a combination of multiple types of feedback, referred to as multisensory feedback, is expected to provide enriched information (Gürkök and Nijholt, 2012). However, to be efficient, feedback should not be too complex and should be provided in manageable pieces (Lotte et al., 2013).
Haptic feedback is still scarcely used in the BCI/NF community, although the haptic sense is the only one that allows us to interact with the world around us and, at the same time, perceive these interactions (Minogue and Jones, 2006). However, applications related to haptic-based BCIs are myriad, such as in rehabilitation and entertainment. For example, the majority of the clinical papers referenced in this survey focus on stroke patients, because haptic-based BCI/NF seems to be a promising approach to rehabilitation, as such non-invasive techniques may contribute to closing the loop between brain and effect (Gomez-Rodriguez et al., 2011). Haptic-based BCIs could also be used as communication applications to enable patients to perform daily activities independently, e.g., using a wheelchair-driving system (Kaufmann et al., 2013b; Herweg et al., 2016). Given that haptic feedback has evolved over the past decades and haptic displays are becoming increasingly sophisticated, haptic-based BCIs have become unobtrusive and thus more effective and more acceptable to users. In this paper, the term “haptic feedback” encompasses two different types of feedback: tactile and kinesthetic (Figure 4). Tactile feedback refers to the sense of touch, which allows one to recognize texture, pressure, and vibrational stimuli; kinesthetic feedback includes proprioception, which allows one to perceive forces/torques in contact with the body as well as to know the body's position in space, even with eyes closed (Roll et al., 1988).
Haptic interfaces also have different purposes in BCI and NF applications. Historically, NF is used to develop internal control, while BCIs are primarily intended to instruct the control of external objects (an orthosis, a computer, etc.); also, by definition NF is biofeedback from brain areas (Sitaram et al., 2017) with the purpose of self-modulation of brain activity, i.e., for personal control rather than redirection of an object. Following the definitions of NF and BCI, this survey will distinguish the concepts of NF and BCI on the basis of the rationale of their implementation. For example, when a stroke patient uses an exoskeleton for feedback, the goal is not to control that skeleton for the sake of controlling it, but rather to work the perilesional areas in order to activate the plasticity systems. In this case, as the purpose is to enhance neuronal activity, the term NF is appropriate. However, if the paradigm is to control the orthosis, then we will speak of BCI.
In their recent paper, Van Erp and Brouwer (2014) provide an extensive state-of-the-art touch-based BCI. Our survey aims to complement their work with an extension to all haptic modalities/cues and to both BCI and NF applications. Our objective is to better understand the current possibilities of haptic feedback and further improve the design of future studies.
The remainder of this paper is organized as follows. First, we provide an overview of existing haptic technology in section 2. Second, in section 3 we survey recent studies on exploiting haptic feedback in BCI/NF applications, showing the experimental and technical challenges. These works are then discussed in section 4, where we also propose guidelines on the use of haptic technology and identify some remaining challenges. Finally, a conclusion is given section 5.
The study of haptic interfaces is an expanding research area focusing on human touch and interaction with the environment through touch. Its integration within BCI experiments is rather recent (since 2007) and was pioneered by Chatterjee et al. (2007) and Cincotti et al. (2007b). The term haptic can be defined as “sensory and/or motor activity of the skin, muscles, joints, and tendons” (ISO, 2011 244: 1). Information delivered through a haptic device is very different from that provided by a visual display. The design of haptic feedback depends on in-depth knowledge of the human haptic sense, either the tactile sense or the kinesthetic sense.
The purpose of feedback in a standard BCI/NF protocol is to give cues for a specific type of brain activity in order to have a beneficial impact on the learning of a BCI/NF task (Jeunet et al., 2015). Thus, the effect of the feedback is dependent not only on its content but also on the way it is presented to the subject (Pillette, 2019). For this reason, gaining knowledge of the human haptic sense is a fundamental step in the development of a haptic interface for BCI/NF systems. Haptic interfaces have possible interactions with many parts of the body, which implies that the sense of touch has the potential to become a very useful tool for digital interaction. The human skin is capable of detecting mechanical stimulation, heat, and pain (Aoyagi et al., 2006). When a haptic event occurs, a sequence of voltage pulses is generated and transmitted by the nerves directly to the brain, where the information is processed. For example, picking up an object and sensing its properties (shape, texture, weight, etc.) requires integrating information from the tactile and kinesthetic senses. The primary motor cortex is the physiological location where haptic information is processed. A visualization of a schematic coronal cut showing the distribution of various parts of the body in the primary motor cortex (Figure 1) demonstrates that a considerable proportion is accounted for by the hands and the fingers.
Figure 1. Functional brain areas in the motor cortex (Faller et al., 2012).
The tactile sense is associated with receptors distributed under the surface of the skin. This sense is commonly called the “sense of touch,” as tactile receptors (high-frequency sensors) discriminate very fine surface properties, such as small shapes and fine textures and have a particularly high density under the palm and the fingers (Olausson et al., 2000). On the hands, four types of physiological receptors can be found, as described in Li et al. (2017): “our fingertips can sense a wide range of tactile stimuli, such as temperature, pressure, pain, or vibration.”
The kinesthetic sense, or proprioception, is associated with receptors in muscles, tendons, and joints and provides information about the movement, position, and torque of limbs (Antona and Stephanidis, 2015). The term “proprioception” is often used for properties relating to the whole body, whereas “kinesthetic” tends to refer to the perception of properties of limbs; however, this distinction will be neglected in the present survey.
This section presents the wide spectrum of existing haptic technologies. Haptic feedback can take different forms, but two main categories can be distinguished: tactile feedback and force feedback. Before describing them in more detail, several important properties of haptic interfaces will be provided.
This categorization is based on whether the haptic interfaces are mobile or anchored to the environment. The design of haptic interfaces recently started to take into account portability as a crucial parameter (Pacchierotti et al., 2017). Furthermore, wearable devices should not limit the user's motion and should enable the stimulation of grasping-related sensations, whereas grounded devices restrain the user's motion and have the ability to stop and block the user. Ground-based interfaces are haptic interfaces anchored in the environment. They can generally be classified as link type, magnetic-levitation type, or tension-based type (Kim et al., 2000). The PHANToM, a force-feedback pen with six degrees of freedom (DOF) that provides a force-reflecting interface between a human user and a computer, is an example of a performing link-type haptic interface (Massie and Salisbury, 1994). Wearable haptic interfaces are attached to the body of the user. Wearable devices are not limited to a constrained workspace, so they allow users to move freely and perceive haptic feedback in a much larger range. On the other hand, wearability introduces power limitations. Devices must be built with miniature technology, and actuation is limited by weight and power consumption. Pacchierotti et al. (2017) provide a list of guidelines for the design of wearable tactile devices that considers multiple factors, such as the form factor, weight, impairment, and comfort (Figure 2).
Figure 2. Wearability in haptic devices: from grounded haptic interfaces to more wearable and portable designs. (A) ENTROPiA, a cylindrical spinning prop attached to a robot to provide haptic virtual texture (Mercado et al., 2019); (B) a hand exoskeleton for natural pitching (Lucas et al., 2004); (C) a cutaneous display providing normal and shear stimuli (Pacchierotti et al., 2016).
Haptic feedback can be divided into two categories: active and passive. Usually active touch refers to the act of touching, while passive touch refers to being touched (Gibson, 1962). For active touch the sensation occurs in the perceiver, and for passive touch it arises in an external device. Hence, passive haptics refer to the haptic properties of physical objects, such as a keyboard or a cup of coffee, and active haptics refer to the haptic properties that are actively generated by the device based on haptic actuators and software. In the haptic field most interfaces are active, but this is not the case for haptic-based BCI/NF systems. Indeed, haptic-based BCI/NF interfaces use calculated feedback from the brain activity and not feedback from the sense of touch. Passive touch is associated with haptic feedback that is not calculated according to the user. For example, a standard vibrotactile alert from a mobile phone can be considered passive feedback.
In the design of a haptic interface, the nature of the contact between the user and the interface can be of three types. Direct contact interfaces are attached haptic interfaces such that the user is always in contact with the device. With intermittent contact interfaces, user contact with the device is limited and provided only when required. For example, Frisoli et al. (2008) developed a grounded fingertip haptic interface whereby a plate comes into contact with the user whenever their finger touches a virtual surface (Figure 3). Indirect/mid-air interfaces produce haptic feedback without having any contact with the user and are therefore not constrained by requirements of the user to wear gloves or hold a device (Bermejo and Hui, 2017). UltraHaptics (Carter et al., 2013), a grounded ultrasonic device, is an example of a mid-air device that provides multi-point haptic feedback to the user's skin. A state-of-the-art review of mid-air devices can be found in Bermejo and Hui (2017).
Figure 3. Conceptual schematic of an intermittent contact interface: a tangible object comes into contact with the hand when the finger grabs a virtual ball (de Tinguy et al., 2020).
Tactile feedback stimulates the surface of the skin through direct contact. Tactile interfaces can be classified according to the sensations they provide: vibration, contact, pressure, temperature, curvature, texture, softness/hardness, and friction (Klatzky and Lederman, 2003). Generally, tactile devices must be lightweight and small, and if the tactile display is to be worn by mobile users, its power consumption must be minimized (Jones and Sarter, 2008). This review will focus on vibration, contact, and pressure interfaces, as these are the most common tactile interfaces in the BCI/NF field. Only feedback related to vibration, contact, pressure, temperature, and electrotactile stimuli will be described in this section; these are the most commonly used types of feedback in the BCI/NF field today.
Vibrotactile feedback is generated by mechanical vibration normal or transverse to the skin surface. Mechanical vibration conveys tactile information modulating vibration frequency, amplitude, duration, timber, or spatial location. Vibrotactile feedback uses the same principle as audio headphones, i.e., it converts electrical signals to sound waves. The quality of vibrotactile stimulus perception is influenced by the frequency of the vibration (~50–300 Hz, which corresponds to the bandwidth of the human tactile sense), the body position, and the underlying tissues. The use of oscillating pressure (sinusoidal or square wave and amplitude modulations) also adds new DOF to the design of vibrotactile stimuli, such as waveform and amplitude modulations at different modulation frequencies of the carrier frequency (Klatzky and Lederman, 2003). Vibrotactile devices delivering variable pressure on the skin have been employed, for instance, as alternative sensory channels for blind or deaf individuals (Richardson and Symmons, 1995). The sensitivity of vibrotactile stimulation depends on the body position and age of the subject (Cholewiak and Collins, 2003).
Contact or pressure feedback can be used to simulate encounters with virtual object surfaces. The effects of such encounters can be simulated with pneumatic systems or surface encounter devices that follow and anticipate the operator's movements (Gabardi et al., 2016). For example, Frisoli et al. (2008) proposed a grounded fingertip haptic interface such that a plate comes into contact with the user when their finger touches a virtual surface.
Thermal interfaces provide thermal cues to the user that are usually experienced during interactions with objects. Following this principle, Guiatni et al. (2012) created a haptic interface that provides thermal and force feedback for surgical operations (Figure 4). The thermal feedback is matched to the thermophysical properties and temperatures of living organs to aid the surgeon's perception. It has also been proposed to use thermal feedback to make a thermal sensing system for prostheses (Cho et al., 2007). For prosthesis users, thermal stimulation improves their interaction with the surrounding environment and provides them with useful information for everyday activities, such as material discrimination and pain avoidance, as well as psychological comfort. A state-of-the-art survey on thermal displays can be found in Jones and Ho (2008).
Figure 4. Haptic interface classification. On the left are two representative examples of tactile stimulation interfaces: vibrotactile actuators [C2-tactors (Cincotti et al., 2007a)]; pressure and contact interfaces (Chinello et al., 2012). On the right are two representative examples of kinesthetic stimulation interfaces: Orthosis developed by Ramos-Murguialday et al. (2012); grounded force interface ENTROPiA (Mercado et al., 2019).
Light electrical stimulation, also known as electrotactile stimulation, can raise the user's awareness and can be used for tactile feedback. Several electrotactile displays have been developed as sensory aids for hearing (Summers et al., 1994) and vision (Kaczmarek and Haase, 2003) and can also be used to create perceptual illusions of surface changes (Wolf and Bäder, 2015). Variations in the intensity and temporal parameters of the stimulation and in the spatial sequence of the electrodes activated can be used to convey information (Jones and Sarter, 2008). However, both the absolute threshold and the subjective magnitude of electrotactile stimulation increase rapidly with changes in the current amplitude (Rollman, 1974). The stimulation current must be controlled carefully to avoid painful sensations in the user. The level of intensity is usually established during a practice session before the recordings. Electrotactile stimulation can also be used for a tongue display unit (Figure 4), which consists of a signal generator that controls the voltage output, a flexible connector cable, and the electrode array. A survey on electrical feedback can be found in Pfeiffer and Rohs (2017).
In contrast to tactile feedback, force feedback relates to the kinesthetic sense, involving positions, velocities, forces, and constraints sensed through muscles and tendons. Kinesthetic feedback can provide information about limb position and strength applied. Devices that use kinesthetic feedback are usually grounded, since the display of the force or motion is delivered through a tool, such as PHANToM (Massie and Salisbury, 1994) or Omega. However, grasping haptic devices and exoskeletons include wearable devices (e.g., haptic gloves). Haptic clinical devices, such as orthoses or robotic systems have notably been used to guide the movements of paralyzed limbs of patients (López-Larraz et al., 2018).
Force-feedback devices usually serve two main purposes: to measure the positions of and contact forces on the user's hand (or other body parts), and to display contact forces and positions to the user. These haptic interfaces are usually composed of rotating joints that connect rigid links (Massie and Salisbury, 1994). Force-feedback devices can be categorized according to the DOF provided by each device, from a simple 1-DOF device to a complex 7-DOF device. Other designs, such as cable systems or stringed haptic interfaces are also grounded force-feedback devices, as they are tension-based systems (Figure 4). Cables are fixed around the corners of a structure, such as a cube. Each cable includes a torque motor, a cable, a tensioning mechanism, and a force sensor. Tension-based haptic interfaces (Sato, 1991) have the advantages of fast reaction speed, simple structure, smooth manipulation, and scalable workspace (Williams, 1998).
An exoskeleton can be used to provide forces on the body with natural degrees of freedom. For example, the orthosis in Figure 4 has to fit the hand naturally without impairing it or interfering with its actions. Heavier exoskeletons can decrease the comfort of the user (Leonardis et al., 2015). The terms orthosis and exoskeleton are used generally to indicate the system effectors, often in an ambivalent way. This review will use the definition from Herr (2009), which stipulates that “generally exoskeleton augments the performance of an able-bodied wearer, whereas orthosis are used to assist a person with a limb pathology and help correct, rehabilitate or support parts of the body.” A review of wearable kinesthetic interfaces can be found in Pacchierotti et al. (2017).
Functional electrical stimulation (FES), also known as electrical muscle stimulation, is a more intensive type of stimulation [up to 150 V (Pfurtscheller et al., 2003)] than electrotactile stimulation (Li et al., 2017). This kind of electrical stimulation actuates muscle contraction and thus provides a kinesthetic sensation. FES has been used efficiently for motor rehabilitation in stroke patients (Kim et al., 2016; Leeb et al., 2016; Mrachacz-Kersting et al., 2016; Frolov et al., 2017) (Figure 4), showing promising results for motor recovery. A survey on FES can be found in Pfeiffer and Rohs (2017).
This section describes state-of-the art haptic applications to different BCI and NF paradigms. To date, the MI paradigm is the most used paradigm for haptic feedback, as it offers the possibility of closing the sensorimotor loop: the user imagines a movement and the modulated signal can be employed to control haptic interfaces that in turn give the subject a sensorimotor stimulus. Other paradigms requiring less training, such as P300 and steady-state somato-sensory evoked potential (SSSEP), have also been used in association with haptic interfaces. These haptic sensors are used to stimulate various parts of the body (at different frequencies), and the elicited electroencephalogram (EEG) signals are processed to generate control commands. Haptic displays therefore have different purposes in these two kinds of BCIs: in sensorimotor paradigms they provide haptic feedback from the brain activity of the subject, whereas for P300 and SSSEP haptic interfaces they are used as stimulation, and the evoked brain activity is further decoded to produce a command (Figure 5).
Figure 5. Implementation of haptic feedback in active BCIs (aBCIs) and reactive BCIs (rBCIs). In aBCIs haptic interfaces provide feedback from the user's neural activity, whereas in rBCIs haptic interfaces provide stimulation, and the elicited brain activity is further decoded and transmitted as a command. The aBCI loop is shown as a black solid closed curve and the rBCI loop is represented by the dotted closed curve.
BCIs can be divided into three classes: active, reactive, and passive (Van Erp and Brouwer, 2014). This review considers only BCI applications based on brain patterns and which are actively or reactively generated by the user: active BCIs (aBCIs) and reactive BCIs (rBCIs). An aBCI provides non-muscular communication between the brain and the external environment without external stimuli, for instance in sensorimotor rhythm (SMR) paradigms (Pfurtscheller and Neuper, 1997, 2001; Baker, 2007; Yuan and He, 2014). An rBCI uses external stimuli to provide information to the subject, for example in somato-sensory evoked potential (SSEP) or P300 paradigms. Passive BCIs (pBCIs), which measure the cognitive or emotional state of the subject from brain patterns without any need for specific user activity (George et al., 2012), will be disregarded in the present work.
For an interactive system, the sense of touch is ideal because of its nature. For example, our haptic sense is bidirectional because humans can perceive and actuate via touch (Klatzky and Lederman, 2003). In terms of interface design, this means that touch can be used as both an input and an output tool.
SMR remains the most popular MI paradigm in haptic-based BCI/NF applications. SMR refers to desynchronization of localized brain rhythms in the upper alpha band (10–12 Hz), usually accompanied by changes in synchronization in the beta band (13–25 Hz) (Pfurtscheller and Neuper, 2001) that occur when performing a real or imagined motor task. This paradigm seems well-adapted to haptic-based BCIs, where tactile and kinesthetic feedback can potentially mimic the natural representation of limb state variables (London et al., 2011). Most SMR-based haptic systems use kinesthetic sensations as feedback from MI performance. The first SMR-based orthosis (hand orthosis, one finger) was designed by Pfurtscheller et al. (2000) for a tetraplegic patient; it was shown that after a period of training (5 months) the patient was able to efficiently control the orthosis with foot or hand MI. Kinesthetic systems differ in their design, which can for instance involve the whole hand or just a few fingers. In most of the studies examined (see Table 1), since the input signal was uni-dimensional, the systems used only one DOF, even if they can deliver more (e.g., the 7-DOF arm orthosis from Gomez-Rodriguez et al., 2011). Different types of movements, such as grasping or opening the hand, can then be transmitted. Grounded systems are typically used for kinesthetic feedback because orthoses are heavy (Buch et al., 2008; Ang et al., 2010, 2014; Ramos-Murguialday et al., 2012; Ono et al., 2014; Darvishi et al., 2017; Chowdhury et al., 2018). However, portability is an important factor for haptic interfaces, which should not limit the motion of the user. Based on this consideration, some studies have investigated portable kinesthetic feedback (Chen et al., 2009; Bundy et al., 2017; Frolov et al., 2017).
Haptic feedback can be delivered both continuously (where the feedback is given during execution of the mental task and directly reports the neural activity) and discretely (where the feedback is given after reaching a threshold). For example, Murguialday et al. (2011) proposed a system composed of a mechanical hand orthosis attached to the upper limb that can extend and close all fingers in order to investigate the effect of proprioception on BCI control. They showed that in healthy subjects, SMR-based BCI/NF training with contingent haptic feedback improves BCI performance and motor learning, enhancing SMR desynchronization during MI. These results were also found by Soekadar et al. (2011), who showed that graded haptic feedback outperforms binary feedback, leading to faster BCI learning and more accurate SMR-ERD modulation (where ERD stands for event-related desynchronization).
The use of tactile feedback for SMR-based BCI/NF systems has also been developed, because of its greater portability, comfort, and affordability relative to kinesthetic interfaces. Tactile interfaces have been used to unload the visual channel (Cincotti et al., 2007b; Leeb et al., 2013; Liburkina et al., 2018) for individuals with impaired vision (Wilson et al., 2012) and patients with spinal cord injury (Cincotti et al., 2007b). Chatterjee et al. (2007) demonstrated that users can control a BCI system using only tactile feedback with vibrotactile stimulators placed on the right or left upper arm. They found that vibrotactile feedback helped the subject regulate contralateral imaginary tasks. In a lingual electrotactile study, Wilson et al. (2012) demonstrated that task performance with tactile feedback was comparable to that with visual feedback. In an extended experiment involving 30 healthy participants and three subjects with spinal cord injury, Cincotti et al. (2007b) showed that the vibrotactile channel can function as a valuable feedback modality, especially when the visual channel is loaded by a secondary task.
Even though the first study of haptic feedback in clinical applications was a case report on a tetraplegic patient (Pfurtscheller et al., 2000), a large proportion of these studies focus on stroke rehabilitation (Buch et al., 2008; Ang et al., 2010; Gomez-Rodriguez et al., 2011; Soekadar et al., 2011; Ramos-Murguialday et al., 2013; Ono et al., 2014; Frolov et al., 2017; Chowdhury et al., 2018). Haptic-based MI BCIs are promising for functional rehabilitation of stroke patients, as such training can also be used with patients having no residual movement. The aim of such BCI/NF systems is to stimulate neural plasticity in perilesional brain motor areas and support upper limb functional improvement (Lioi et al., 2018, 2020). Since haptic BCI/NF-based SMR techniques achieve motor imagery with concurrent motor learning via kinesthetic feedback, it is natural to think of rehabilitation of stroke patients even in a chronic condition. In these applications the question of the cortical target is still open. Usually control of the orthosis is modulated by the ipsilesional side of the brain (Pichiorri et al., 2015), contralateral to the affected hand; however, the ability to modulate perilesional activity decreases with increased cortical damage (Buch et al., 2012). For example, Bundy et al. (2017) studied the contralesional motor area for control of a portable exoskeleton, the assumption being that recovery would be optimal on the contralesional side and that functional improvements may be elicited (Ward et al., 2003). In 2008, Buch et al. (2008) demonstrated that chronic stroke patients with upper limb hemiplegia were able to control a magnetoencephalography (MEG) BCI by voluntarily modulating the ipsilesional SMR amplitude while receiving contingent haptic feedback with a hand orthosis. The haptic system used was a grounded mechanical orthosis attached to the plegic hand, with one attachment on each finger except the thumb. The feedback was given in a passive way, with a movement of the orthosis elicited only if the modulation had reached a certain threshold at the end of the trial. Kinesthetic feedback is mostly employed for stroke rehabilitation, in agreement with the finding that rehabilitation of motor functions is more efficient with proprioceptive feedback. Most studies of rehabilitation involve kinesthetic feedback, but FES-based MI has also been performed for patients. In an early study by Pfurtscheller et al. (2003), they reported applying non-invasive techniques to restore grasp functionality in a tetraplegic patient through FES. This same method was used with chronic stroke patients in Leeb et al. (2013). The interested reader can find more information about BCI applications for stroke rehabilitation in the 2018 review article by López-Larraz et al. (2018).
Brain signals can be elicited by using external stimulation. Frequently used paradigms include SSEP and event-related potentials (ERPs). Most BCIs using ERPs can be employed without any prior training and do not exhibit the so-called “BCI illiteracy” problem (where the BCI system fails to correctly detect the mental state of its user). The following paragraph will deal with external paradigms (P300 and SSSEP) and their relationship with the haptic modality. To the best of our knowledge, in contrast to SMR-based BCI/NF systems where haptic technologies are used to provide the feedback, in external stimulation paradigms haptic interfaces are mostly employed as a stimulus.
P300 is a positive deflection of the EEG signal occurring around 300 ms after presentation of a given stimulus (visual, haptic, or auditory). A major strength of the P300 paradigm is its reproducibility and stability as a feature of rBCIs (Donchin et al., 2000). The majority of P300-based BCI studies use the visual channel for stimulation (Table 2): one motivation for using haptics for P300-based BCIs is in fact to reduce the dependence of the gaze in rBCIs. The interest here is not so much to imitate a kinesthetic or tactile sensation but rather to give the haptic stimulation in the most efficient way. Indeed, most haptic-based P300 studies use tactile rather than kinesthetic sensation as stimulation. The first to adopt this paradigm in a haptic-based BCI study were (Aloise et al., 2007), who investigated the influence of a tactile stimulus on classification performance in eight subjects. The tactile stimulus was provided with eight vibrotactile stimulators placed at different positions on the hands and wrists. The authors reported that the tactile stimulus increased the latency of the principal P300 component (a 600 ms peak after the haptic stimulus against 400 ms for the visual stimulus) and that online classification performance was weaker than with a visual stimulus (68 against 93%). Other studies using vibrotactile tactors in P300-based BCIs followed, differing in where the vibrators were located: on the wrist, on the arm, on the palm (Rutkowski and Mori, 2015), on the neck, or even on the head (Mori et al., 2013).
The use of other forms of haptic interfaces in P300-based BCIs is still marginal, and further studies are required to assess if they have the potential to enhance BCI efficiency. Kinesthetic stimulation with force feedback was investigated in Kono et al. (2013), where the kinesthetic sensation was delivered through a joystick to the subject's dominant hand and provided four different movements corresponding to the four main directions. Hamada et al. (2014) tested the first non-contact method of producing tactile sensations for BCIs (mid-air haptics), and in Shimizu et al. (2014) tactile pressure sensation was tested.
The P300 paradigm requires less training and may achieve higher accuracy than the MI paradigm (Allison and Neuper, 2010), as well as having the potential to be used in the control of communication systems for patients with locked-in syndrome (LIS) or completely locked-in syndrome (CLIS). LIS or CLIS are conditions where the patient cannot communicate or does not have control over their motor functions except for vertical eye movements and blinking (Duffy, 2012). BCIs may offer a new communication solution for such patients who have sufficiently intact cognitive abilities (Wolpaw et al., 2002; Karim et al., 2006). It is from this perspective that Guger et al. (2017) compared the two paradigms to assess whether vibrotactile P300 could outperform MI in a communication system for LIS patients. The use of haptic-based P300 for control of an object in the everyday environment has also been studied, in particular in the context of wheelchair control, because visual feedback limits the user's interaction with the external environment (Herweg et al., 2016). Recently, a spelling application involving the use of tactile stimulation on the fingertips was developed by van der Waal et al. (2012), with resulting spelling rates similar to those of visual spellers. Kaufmann et al. (2013a) described an experiment in which they tested healthy users steering a virtual wheelchair in a building. The four navigational directions were associated with different tactor locations on the body. Out of the 15 participants, 11 successfully navigated a route along four waypoints, supporting the view that the haptic P300 paradigm has potential for medical applications.
SSSEPs constitute a steady-state component of brain signals evoked by sustained repetitive vibrotactile stimulation within the frequency range of 17–35 Hz (Breitwieser et al., 2012). The idea behind the method is to increase the information transfer rate (ITR) (which is slower with SMR-based paradigms because it requires some time to establish ERD patterns) without loading the eye gaze (Müller-Putz et al., 2006). SSSEPs also represent an alternative to visual-based P300 or steady-state visual evoked potential (SSVEP) systems. Because the stimulation paradigm is based on vibrations, most studies employ tactile interfaces, using a vibrator to deliver the stimulus (Table 3). The first appearance of SSSEP within a haptic-based BCI environment is in the study by Müller-Putz et al. (2006), in which the authors investigated whether SSSEP is as efficient as the BCI paradigm. Tactile stimulation was provided by vibrotactile stimulators placed on both index fingers, and the user had to concentrate on one stimulus (right or left) at a time. Müller-Putz et al. reported that of four healthy subjects, only half attained a classification accuracy of 70%. The placement of the vibrotactile stimulators in SSSEP-based BCIs differs between studies, though in most cases the stimulators are concentrated on the user's hands (fingers, wrist) or feet (Kim and Lee, 2017), as the discrimination of different vibration frequencies is higher when the tactors are placed in these locations. Comparison of different paradigms has also been done; for example, Severens et al. (2013) studied the difference between the performance of SSSEP and that of P300, reporting that P300 outperformed SSSEP and that the combination of the two did not result in better performance than with P300 alone. These results show the limitations of the SSSEP paradigm: the comfort of subjects is low (they have to concentrate on one of two or more tactile stimuli) (Ahn et al., 2016), which is not the case with SSVEP, where eye position primarily determines the target (Müller-Putz et al., 2006). Combining SSSEP with other paradigms could be a more promising approach. Ahn and Jun (2012) combined SSSEP (left and right fingers) with an imagined-movement BCI paradigm. Kim and Lee (2017) designed a wheelchair-driving system that uses three vibrotactile stimulators to control different directions, indicating that this system has the potential to help amyotrophic lateral sclerosis (ALS) patients or other patients with LIS gain independence in their daily activities.
Haptic-based BCI/NF applications have gained increasing interest in recent years. Researchers using haptics to provide feedback or stimulation have focused on three different paradigms: (1) haptic-based SMR, which mostly employs kinesthetic feedback and is used for stroke rehabilitation; (2) haptic-based P300, where tactile stimuli are generally used to elicit a brain response for the control of an object; and (3) haptic-based SSSEP, in which vibrotactile stimuli are employed. In each of the paradigms discussed in this review, clinical applications have been tested with promising results. Nevertheless, there are limitations and challenges that must be addressed by the haptic-based BCI community. In this section, we discuss some points regarding the design of haptic systems adapted to BCIs, the utility and interest of haptic feedback for BCI and NF applications (compared with other modalities), and limitations of the current solutions.
Most BCI studies involving haptics have used the MI paradigm, often in conjunction with visual feedback. This general trend is mostly explained by the fact that in an MI task, closing the sensorimotor loop has the potential to improve the quality and pertinence of the feedback provided, thus enhancing user engagement and NF performance. On the other hand, for the SSSEP and P300 paradigms, haptic feedback is seen more as an alternative to the visual channel. Concerning the applications of haptic BCI/NF, this review indicates that there is a major tendency toward using these systems for rehabilitation, especially for stroke patients, and that the vast majority of studies used kinesthetic feedback, with the goal of reproducing a real and complex movement. On the other hand, tactile feedback is mainly used with the aim of restoring comfort for patients with LIS or visual impairments, rather than for rehabilitation purposes. The majority of kinesthetic feedback involves the upper limbs, with orthoses placed on either the hand or the arm; this is not necessarily the case for tactile feedback, which can be placed on different parts of the body. The visual modality is also commonly employed in these studies, either to provide a visual clue (i.e., to know if the user has to imagine a right or left movement) or as feedback complementary to the haptic feedback. The visual feedback either takes a classic form (e.g., a bar or thermometer) or is a more realistic kind of proprioceptive feedback representing, for instance, a hand. The gain of the haptic modality with respect to the visual modality in different paradigms still needs to be more accurately assessed, although several studies have paved the way and seem to converge on the finding that haptic feedback is either equivalent to or more effective than visual feedback in certain applications.
Haptic-based P300 mostly uses tactile vibration as a stimulus, and rarely other modalities. Visual stimuli are used together with haptic stimulation in the P300 paradigm mainly to assess the gain of a haptic stimulus. There is still no consensus around this gain, as some studies show an equivalent effect on the classification performance while in others haptic-based paradigms have reduced performance relative to visual ones. The use of a haptic stimulus is often motivated by the fact that haptic stimulation is the only possible communication channel for some patients (e.g., those with LIS or CLIS), for whom use of the visual channel is not always possible. In contrast to haptic-based SMR paradigms, for P300 applications there is a richer literature dealing specifically with the design of the haptic interface.
Applications based on haptic SSSEP are very similar to P300 paradigms, but limited research has been done on the design of such systems. Since P300 and SSSEP share similar objectives, it would be interesting to compare these two paradigms in future studies.
The integration of haptics in BCI/NF environments can be complex and entails some challenges at different levels. This is also because haptic-based BCI/NF studies are usually designed by imitating visual feedback protocols, even if the design may be sub-optimal for the haptic modality. In this section, we discuss some issues that should be addressed in the design of haptic BCI/NF protocols adapted to specific applications.
The basis of human-computer interaction is the use of feedback, which underlies the interactions occurring between the user and the system (Hewett et al., 1992). A recurring question in the BCI community is the frequency at which the feedback should be provided. The feedback can be given in two different ways: continuously or discretely. It would seem more natural in a BCI environment to give the feedback at the end of a successful trial rather than continuously. By contrast, in an NF paradigm, the feedback is an indicator to the user of their own cerebral activity, so in this case it would seem more appropriate to give haptic feedback in real time. A recent study (Shu et al., 2017) indicates that improvement in an MI task could be achieved if vibrotactile stimulation of the non-dominant or paretic hand of the patient is performed during MI, thus highlighting the importance of defining the feedback delivery modality depending on the desired application.
In haptic BCI/NF applications, various artifacts can contaminate the signal; these can be generated by the devices controlled with the haptic feedback [e.g., noise generated by actuators based on electric/magnetic neurostimulation or by robotic devices (Leeb et al., 2013; Insausti-Delgado et al., 2017)] or have a physiological origin (e.g., compensatory movements, cranial and neck muscle activity, eye movements, swallowing, etc.). The question of whether the haptic feedback introduces additional artifacts that influence BCI performance is still debated, and the answer depends greatly on which haptic system has been tested. For tactile feedback, some studies have shown no interference with the electric signal (Kauhanen et al., 2006; Chatterjee et al., 2007). For example, Leeb et al. (2013) demonstrated no significant difference during the rest and the stimulation with vibrotactile feedback. However, Hommelsen et al. (2017) showed FES feedback to be a considerable source of false positives when the mu rhythm was used for the detection of efferent commands. We suggest that a thorough study of the influence of haptic feedback, whether tactile or kinesthetic, be conducted to determine what kinds of artifacts are induced by vibratory feedback and feedback with an orthosis.
According to recent findings of Bashashati et al. (2015), the choice of the classifier for a BCI system depends on the feature extraction method used. We also recommend that the choice of classifier and the choice of feature take into account the specific feedback modality employed; for example, an optimal classifier for haptic feedback may not be efficient for visual feedback. The majority of EEG classification algorithms were developed for vision-based BCI/NF, while neurophysiological responses to tactile stimuli may differ; a research effort to define a methodological framework specific to the analysis of haptic features is therefore needed.
To date, the BCI community has been using haptic interfaces for sensory feedback or as stimulation systems that are generally simple and sometimes dated. The design of haptic interfaces has progressed hugely in recent years, and it would be interesting to integrate the technological advances into BCI/NF studies. If we consider, for instance, the DOF of haptic devices, at present the majority of studies involving a kinesthetic system are limited to only one DOF, even if the device can provide more. Using more DOF may facilitate motor learning (Scheidt et al., 2000) and should be investigated for rehabilitation of stroke patients. For stroke rehabilitation, tactile or kinesthetic devices already exist but not in a BCI environment; for example, Lin et al. (2016) developed a haptic glove equipped with vibrotactile stimulators that interact with a virtual-reality environment. Other studies have focused more on ergonomics for the user by designing exoskeletons with multiple DOF (Wege and Hommel, 2005; Godfrey et al., 2013). However, wearability is often not a priority, though it must be taken into account to enable the user to optimally perceive and interact with the environment. For example, In et al. (2011) developed wearable hand interfaces and proposed a jointless hand exoskeleton weighing only 80 g. We suggest that portability of haptic feedback should become more central to the study of haptics design in the future.
Visual feedback has historically dominated the field of BCI/NF, and only in recent years have other modalities (auditory or haptic) for delivering information been explored (Lukoyanov et al., 2018).
The gain of the haptic modality over other modalities can be assessed looking at different parameters, such as BCI/NF performance, comfort of the subject, or adaptation in an everyday environment. For example, haptic feedback could enhance MI (Shu et al., 2017) by bypassing BCI illiteracy. BCI illiteracy represents a big challenge in BCI research (Vidaurre and Blankertz, 2010), and currently available SMR-based BCI/NF systems may have reached the limits of their performance, as ~30% of healthy subjects (Blankertz et al., 2010) and 40% of stroke patients (Ang and Guan, 2015) cannot attain the critical BCI accuracy of 70%. Recent work of Lukoyanov et al. (2018) suggests that after some training, the type of feedback (visual or tactile) does not affect the classification accuracy. It does, however, affect the comfort of subjects, who describe tactile feedback as being more natural. Moreover, there are still few studies that compare different modalities: for SMR paradigms it seems that the visual and haptic modalities are comparable in terms of BCI performance; however, for P300-based studies this is still not clear. The gain of the haptic modality must also be assessed with respect to the decrease in visual workload, since the feedback no longer occupies the visual channel.
In current approaches haptic feedback is delivered in a uni-dimensional way; for example, the task performed by the user is usually binary, such as open/close or open/grasp. For stroke rehabilitation this could be a limitation, since the mental task is often more complex in reality. Future studies should explore the possibility of including more than one task in order to provide more complex training (bearing in mind that this would also increase the training time). We recommend that further research be done on the design of more realistic haptic training.
In our daily environment we encounter many simultaneous and multimodal stimuli. It is therefore of interest to test a multisensory feedback approach in a BCI context. One might hypothesize that multimodal feedback, such as visuohaptic or audiovisual feedback would be more effective than simple unimodal feedback (Sigrist et al., 2013). In a clinical context this might also be interesting to investigate; for example, vision may be compromised in LIS, CLIS, or ALS patients, and additional sensory feedback may provide a good alternative to uni-dimensional feedback. Several studies have tested the impact of multimodal visuo-auditory feedback for BCI-based SMR; overall the multimodal feedback was found to either have a similar effect to unimodal visual feedback (Schreuder et al., 2010) or yield better results in the first session (Sollfrank et al., 2016). In some cases multimodal feedback increased performance in some naive subjects (Gargiulo et al., 2012). For the visuohaptic modality, an investigation of Brouwer and van Erp (2010) showed that visual-tactile feedback has better performance than uni-sensory stimulation. It has also been suggested that the feedback given to the subject could be either equally shared between different channels, replicated on each channel (Cincotti et al., 2007b), or even dynamically distributed between channels. Although the use of visual feedback in addition to haptic feedback is often systematic, it is not always justified. This suggests that further work is needed to shed light on the use of multimodal feedback and to assess the efficacy of visuohaptic feedback compared to unimodal feedback, whether visual or haptic.
Haptic interfaces are undergoing major technological progress, and the BCI/NF community is looking at the haptic modality with increasing interest. In this review we have summarized and discussed the state-of-the-art research on haptic-based BCI/NF applications. We have outlined different paradigms using haptic interfaces, such as SMR, P300, and SSSEP, as well as methodologies for the design of pertinent haptic applications. We have identified major trends in the use of haptics in BCIs and NF and discussed the limitations of current solutions. To date there is no consensus on the effectiveness of haptic feedback for BCI and NF systems. This review shows that haptic interfaces have the potential to enhance performance and increase the pertinence of the feedback provided, in particular for the SMR paradigm, which is used in the context of motor rehabilitation. Further studies are, however, needed to test the use of innovative haptic technologies for BCI and NF and to assess the utility of the haptic modality, used either alone or in combination with other modalities.
MF, GL, CB, and AL contributed the conception and design of the review. MF wrote the first draft of the manuscript, wrote the sections of the manuscript, and organized the database. GL wrote the sections of the manuscript. All authors contributed to the article and approved the submitted version.
The authors declare that the research was conducted in the absence of any commercial or financial relationships that could be construed as a potential conflict of interest.
Ahn, S., Ahn, M., Cho, H., and Chan Jun, S. (2014). Achieving a hybrid brain-computer interface with tactile selective attention and motor imagery. J. Neural Eng. 11:066004. doi: 10.1088/1741-2560/11/6/066004
Ahn, S., and Jun, S. C. (2012). “Feasibility of hybrid BCI using ERD- and SSSEP-BCI,” in 2012 12th International Conference on Control, Automation and Systems, 2053–2056.
Ahn, S., Kim, K., and Jun, S. C. (2016). Steady-state somatosensory evoked potential for brain-computer interface—present and future. Front. Hum. Neurosci. 9:716. doi: 10.3389/fnhum.2015.00716
Allison, B., and Neuper, C. (2010). Could Anyone Use a BCI?(B + H) CI: Brain-Computer Interfaces (Human-Computer Interaction Series). Berlin: Springer.
Aloise, F., Lasorsa, I., Schettini, F., Brouwer, A., Mattila, D., Babiloni, F., et al. (2007). Multimodal stimulation for a p300-based BCI. Int. J. Bioelectromagn 9, 128–130.
Ang, H. K., Guan, C., Chua, K. S. G., Ang, B. T., Kuah, C., Wang, C., et al. (2010). “Clinical study of neurorehabilitation in stroke using EEG-based motor imagery brain-computer interface with robotic feedback,” in 2010 Annual International Conference of the IEEE Engineering in Medicine and Biology (IEEE), 5549–5552. doi: 10.1109/IEMBS.2010.5626782
Ang, K. K., and Guan, C. (2015). Brain–computer interface for neurorehabilitation of upper limb after stroke. Proc. IEEE 103, 944–953. doi: 10.1109/JPROC.2015.2415800
Ang, K. K., Guan, C., Phua, K. S., Wang, C., Zhou, L., Tang, K. Y., et al. (2014). Brain-computer interface-based robotic end effector system for wrist and hand rehabilitation: results of a three-armed randomized controlled trial for chronic stroke. Front. Neuroeng. 7:30. doi: 10.3389/fneng.2014.00030
Antona, M., and Stephanidis, C. (2015). “Universal access in human-computer interaction,” in Access to the Human Environment and Culture: 9th International Conference, UAHCI 2015, Held as Part of HCI International 2015, August 2–7, 2015, Proceedings, Vol. 9178 (Los Angeles, CA: Springer).
Aoyagi, S., Tanaka, T., and Minami, M. (2006). “Recognition of contact state of four layers arrayed type tactile sensor by using neural network,” in Proceedings of IEEE ICIA 2006–2006 IEEE International Conference on Information Acquisition, 393–397. doi: 10.1109/ICIA.2006.305744
Baker, S. N. (2007). Oscillatory interactions between sensorimotor cortex and the periphery. Curr. Opin. Neurobiol. 17, 649–655. doi: 10.1016/j.conb.2008.01.007
Bashashati, H., Ward, R. K., Birch, G. E., and Bashashati, A. (2015). Comparing different classifiers in sensory motor brain computer interfaces. PLoS ONE 10:e0129435. doi: 10.1371/journal.pone.0129435
Bermejo, C., and Hui, P. (2017). A survey on haptic technologies for mobile augmented reality. arXiv 1709.00698.
Blankertz, B., Sannelli, C., Halder, S., Hammer, E. M., Kübler, A., Müller, K.-R., et al. (2010). Neurophysiological predictor of SMR-based BCI performance. Neuroimage 51, 1303–1309. doi: 10.1016/j.neuroimage.2010.03.022
Breitwieser, C., Kaiser, V., Neuper, C., and Müller-Putz, G. R. (2012). Stability and distribution of steady-state somatosensory evoked potentials elicited by vibro-tactile stimulation. Med. Biol. Eng. Comput. 50, 347–357. doi: 10.1007/s11517-012-0877-9
Breitwieser, C., Pokorny, C., Neuper, C., and Muller-Putz, G. R. (2011). “Somatosensory evoked potentials elicited by stimulating two fingers from one hand—usable for BCI?” in 2011 Annual International Conference of the IEEE Engineering in Medicine and Biology Society (IEEE), 6373–6376. doi: 10.1109/IEMBS.2011.6091573
Brouwer, A.-M., and van Erp, J. (2010). A tactile P300 brain-computer interface. Front. Neurosci. 4:19. doi: 10.3389/fnins.2010.00019
Buch, E., Weber, C., Cohen, L. G., Braun, C., Dimyan, M. A., Ard, T., et al. (2008). Think to move: a neuromagnetic brain-computer interface (BCI) system for chronic stroke. Stroke 39, 910–917. doi: 10.1161/STROKEAHA.107.505313
Buch, E. R., Modir Shanechi, A., Fourkas, A. D., Weber, C., Birbaumer, N., and Cohen, L. G. (2012). Parietofrontal integrity determines neural modulation associated with grasping imagery after stroke. Brain 135, 596–614. doi: 10.1093/brain/awr331
Bundy, D. T., Souders, L., Baranyai, K., Leonard, L., Schalk, G., Coker, R., et al. (2017). Contralesional brain–computer interface control of a powered exoskeleton for motor recovery in chronic stroke survivors. Stroke 48, 1908–1915. doi: 10.1161/STROKEAHA.116.016304
Carter, T., Seah, S. A., Long, B., Drinkwater, B., and Subramanian, S. (2013). “Ultrahaptics: multi-point mid-air haptic feedback for touch surfaces,” in Proceedings of the 26th Annual ACM Symposium on User Interface Software and Technology (ACM), 505–514. doi: 10.1145/2501988.2502018
Chatterjee, A., Aggarwal, V., Ramos, A., Acharya, S., and Thakor, N. V. (2007). A brain-computer interface with vibrotactile biofeedback for haptic information. J. Neuroeng. Rehabil. 4:40. doi: 10.1186/1743-0003-4-40
Chen, C.-W., Lin, C.-C. K., and Ju, M.-S. (2009). Hand orthosis controlled using brain-computer interface. J. Med. Biol. Eng. 29, 234–241.
Chinello, F., Malvezzi, M., Pacchierotti, C., and Prattichizzo, D. (2012). “A three DoFs wearable tactile display for exploration and manipulation of virtual objects,” in 2012 IEEE Haptics Symposium (HAPTICS) (IEEE), 71–76. doi: 10.1109/HAPTIC.2012.6183772
Cho, Y., Liang, K., Folowosele, F., Miller, B., and Thakor, N. V. (2007). “Wireless temperature sensing cosmesis for prosthesis,” in 2007 IEEE 10th International Conference on Rehabilitation Robotics (IEEE), 672–677. doi: 10.1109/ICORR.2007.4428497
Cholewiak, R. W., and Collins, A. A. (2003). Vibrotactile localization on the arm: effects of place, space, and age. Percept. Psychophys. 65, 1058–1077. doi: 10.3758/BF03194834
Chowdhury, A. (2018). Active physical practice followed by mental practice using BCI-driven hand exoskeleton: a pilot trial for clinical effectiveness and usability. IEEE J. Biomed. Health Inform. 22, 1786–1795. doi: 10.1109/JBHI.2018.2863212
Cincotti, F., Kauhanen, L., Aloise, F., Palomaki, T., Caporusso, N., Jylänki, P., et al. (2007a). Preliminary experimentation on vibrotactile feedback in the context of mu-rhythm based BCI. Conf. Proc. 2007, 4739–4742. doi: 10.1109/IEMBS.2007.4353398
Cincotti, F., Kauhanen, L., Aloise, F., Palomäki, T., Caporusso, N., Jylänki, P., et al. (2007b). Vibrotactile feedback for brain-computer interface operation. Comput. Intell. Neurosci. 2007:48937. doi: 10.1155/2007/48937
Darvishi, S., Gharabaghi, A., Boulay, C. B., Ridding, M. C., Abbott, D., and Baumert, M. (2017). Proprioceptive feedback facilitates motor imagery-related operant learning of sensorimotor β-band modulation. Front. Neurosci. 11:60. doi: 10.3389/fnins.2017.00060
de Tinguy, X., Howard, T., Pacchierotti, C., Marchal, M., and Lécuyer, A. (2020). “Weatavix: wearable actuated tangibles for virtual reality experiences,” in EUROHAPTICS 2020.
Donchin, E., Spencer, K. M., and Wijesinghe, R. (2000). The mental prosthesis: assessing the speed of a p300-based brain-computer interface. IEEE Trans. Rehabil. Eng. 8, 174–179. doi: 10.1109/86.847808
Duffy, J. R. (2012). Motor Speech Disorders: Substrates, Differential Diagnosis, and Management. Elsevier Health Sciences.
Faller, A., Schünke, M., and Schünke, G. (2012). Der Korper des Menschen: Einf¨uhrung in Bau und Funktion¨. Stuttgart: Thieme.
Frisoli, A., Solazzi, M., Salsedo, F., and Bergamasco, M. (2008). A fingertip haptic display for improving curvature discrimination. Presence Teleoper. Virt. Environ. 17, 550–561. doi: 10.1162/pres.17.6.550
Frolov, A. A., Mokienko, O., Lyukmanov, R., Biryukova, E., Kotov, S., Turbina, L., et al. (2017). Post-stroke rehabilitation training with a motor-imagery-based brain-computer interface (BCI)-controlled hand exoskeleton: a randomized controlled multicenter trial. Front. Neurosci. 11:400. doi: 10.3389/fnins.2017.00400
Gabardi, M., Solazzi, M., Leonardis, D., and Frisoli, A. (2016). “A new wearable fingertip haptic interface for the rendering of virtual shapes and surface features,” in 2016 IEEE Haptics Symposium (HAPTICS) (IEEE), 140–146. doi: 10.1109/HAPTICS.2016.7463168
Gargiulo, G., Mohamed, A., McEwan, A. L., Bifulco, P., Cesarelli, M., Jin, C. T., et al. (2012). Investigating the role of combined acoustic-visual feedback in one-dimensional synchronous brain computer interfaces, a preliminary study. Med. Dev. Evid. Res. 5:81. doi: 10.2147/MDER.S36691
George, L., Marchal, M., Glondu, L., and Lécuyer, A. (2012). Combining Brain-Computer Interfaces and Haptics: Detecting Mental Workload to Adapt Haptic Assistance. Berlin; Heidelberg: Springer.
Gibson, J. J. (1962). Psychological review observations on active touch. Psychol. Rev. 69, 477–491. doi: 10.1037/h0046962
Godfrey, S. B., Holley, R. J., and Lum, P. S. (2013). Clinical effects of using hexorr (hand exoskeleton rehabilitation robot) for movement therapy in stroke rehabilitation. Am. J. Phys. Med. Rehabil. 92, 947–958. doi: 10.1097/PHM.0b013e31829e7a07
Gomez-Rodriguez, M., Peters, J., Hill, J., Schölkopf, B., Gharabaghi, A., and Grosse-Wentrup, M. (2011). Closing the sensorimotor loop: haptic feedback facilitates decoding of motor imagery. J. Neural Eng. 8:036005. doi: 10.1088/1741-2560/8/3/036005
Guger, C., Spataro, R., Allison, B. Z., Heilinger, A., Ortner, R., Cho, W., et al. (2017). Complete locked-in and locked-in patients: command following assessment and communication with vibro-tactile P300 and motor imagery brain-computer interface tools. Front. Neurosci. 11:251. doi: 10.3389/fnins.2017.00251
Guiatni, M., Riboulet, V., Duriez, C., Kheddar, A., and Cotin, S. (2012). A combined force and thermal feedback interface for minimally invasive procedures simulation. IEEE/ASME Trans. Mechatron. 18, 1170–1181. doi: 10.1109/TMECH.2012.2197862
Gürkök, H., and Nijholt, A. (2012). Brain–computer interfaces for multimodal interaction: a survey and principles. Int. J. Hum. Comput. Interact. 28, 292–307. doi: 10.1080/10447318.2011.582022
Hamada, K., Mori, H., Shinoda, H., and Rutkowski, T. M. (2014). Airborne ultrasonic tactile display brain-computer interface paradigm. arXiv 1404.4184. doi: 10.1007/978-3-319-25190-5_6
Herr, H. (2009). Exoskeletons and orthoses: classification, design challenges and future directions. J. Neuroeng. Rehabil. 6:21. doi: 10.1186/1743-0003-6-21
Herweg, A., Gutzeit, J., Kleih, S., and Kübler, A. (2016). Wheelchair control by elderly participants in a virtual environment with a brain-computer interface (BCI) and tactile stimulation. Biol. Psychol. 121, 117–124. doi: 10.1016/j.biopsycho.2016.10.006
Hewett, T. T., Baecker, R., Card, S., Carey, T., Gasen, J., Mantei, M., et al. (1992). ACM SIGCHI Curricula for Human-Computer Interaction. ACM.
Hinterberger, T., Neumann, N., Pham, M., Kübler, A., Grether, A., Hofmayer, N., et al. (2004). A multimodal brain-based feedback and communication system. Exp. Brain Res. 154, 521–526. doi: 10.1007/s00221-003-1690-3
Hommelsen, M., Schneiders, M., Schuld, C., Keyl, P., and Rupp, R. (2017). Sensory feedback interferes with mu rhythm based detection of motor commands from electroencephalographic signals. Front. Hum. Neurosci. 11:523. doi: 10.3389/fnhum.2017.00523
In, H. K., Cho, K. J., Kim, K. R., and Lee, B. S. (2011). “Jointless structure and under-actuation mechanism for compact hand exoskeleton,” in 2011 IEEE International Conference on Rehabilitation Robotics (IEEE), 1–6. doi: 10.1109/ICORR.2011.5975394
Insausti-Delgado, A., López-Larraz, E., Bibián, C., Nishimura, Y., Birbaumer, N., and Ramos-Murguialday, A. (2017). “Influence of trans-spinal magnetic stimulation in electrophysiological recordings for closed-loop rehabilitative systems,” in 2017 39th Annual International Conference of the IEEE Engineering in Medicine and Biology Society (EMBC) (IEEE), 2518–2521. doi: 10.1109/EMBC.2017.8037369
Jeunet, C., Vi, C., Spelmezan, D., N'Kaoua, B., Lotte, F., and Subramanian, S. (2015). Continuous Tactile Feedback for Motor-Imagery Based Brain-Computer Interaction in a Multitasking Context. Springer.
Jones, L. A., and Ho, H.-N. (2008). Warm or cool, large or small? The challenge of thermal displays. IEEE Trans. Haptics 1, 53–70. doi: 10.1109/TOH.2008.2
Jones, L. A., and Sarter, N. B. (2008). Tactile displays: guidance for their design and application. Hum. Fact. 50, 90–111. doi: 10.1518/001872008X250638
Kaczmarek, K. A., and Haase, S. J. (2003). Pattern identification as a function of stimulation on a fingertip-scanned electrotactile display. IEEE Trans. Neural Syst. Rehabil. Eng. 11, 269–275. doi: 10.1109/TNSRE.2003.816874
Karim, A. A., Hinterberger, T., Richter, J., Mellinger, J., Neumann, N., Flor, H., et al. (2006). Neural internet: web surfing with brain potentials for the completely paralyzed. Neurorehabil. Neural Repair 20, 508–515. doi: 10.1177/1545968306290661
Kaufmann, T., Herweg, A., and Kübler, A. (2013a). “Tactually-evoked event-related potentials for BCI-based wheelchair control in a virtual environment,” in Proceedings of the Fifth International Brain Computer Interface Meeting.
Kaufmann, T., Holz, E. M., and Kübler, A. (2013b). Comparison of tactile, auditory, and visual modality for brain-computer interface use: a case study with a patient in the locked-in state. Front. Neurosci. 7:129. doi: 10.3389/fnins.2013.00129
Kauhanen, L., Palomäki, T., Jylänki, P., Aloise, F., Nuttin, M., Millan, J. R., et al. (2006). “Haptic feedback compared with visual feedback for BCI,” in Proceedings of the 3rd International Brain-Computer Interface Workshop & Training Course (Graz).
Kim, K.-T., and Lee, S.-W. (2017). “Towards an EEG-based intelligent wheelchair driving system with vibro-tactile stimuli,” in 2016 IEEE International Conference on Systems, Man, and Cybernetics, SMC 2016–Conference Proceedings, 2382–2385. doi: 10.1109/SMC.2016.7844595
Kim, S., Ishii, M., Koike, Y., and Sato, M. (2000). “Haptic interface with 7 DOF using 8 strings: SPIDAR-G,” in Proceedings of the 10th International Conference on Artificial Reality and Tele-existence (ICAT '00).
Kim, T., Kim, S., and Lee, B. (2016). Effects of action observational training plus brain-computer interface-based functional electrical stimulation on paretic arm motor recovery in patient with stroke: a randomized controlled trial. Occup. Ther. Int. 23, 39–47. doi: 10.1002/oti.1403
Klatzky, R. L., and Lederman, S. J. (2003). “Touch,” in Handbook of Psychology (Wiley Online Library), 147–176. doi: 10.1002/0471264385.wei0406
Kono, S., Aminaka, D., Makino, S., and Rutkowski, T. (2013). “EEG signal processing and classification for the novel tactile-force brain-computer interface paradigm,” in Proceedings–2013 International Conference on Signal-Image Technology and Internet-Based Systems, SITIS 2013, 812–817. doi: 10.1109/SITIS.2013.132
Leeb, R., Biasiucci, A., Schmidlin, T., Corbet, T., Vuadens, P., Millán, J., et al. (2016). “BCI controlled neuromuscular electrical stimulation enables sustained motor recovery in chronic stroke victims,” in Proceedings of the 6th International Brain-Computer Interface Meeting, Number CONF.
Leeb, R., Gwak, K., Kim, D. S., and Millan, J. del R. (2013). “Freeing the visual channel by exploiting vibrotactile BCI feedback,” in 2013 35th Annual International Conference of the IEEE Engineering in Medicine and Biology Society (EMBC) (IEEE), 3093–3096. doi: 10.1109/EMBC.2013.6610195
Leonardis, D., Barsotti, M., Loconsole, C., Solazzi, M., Troncossi, M., Mazzotti, C., et al. (2015). An EMG-controlled robotic hand exoskeleton for bilateral rehabilitation. IEEE Trans. Haptics 8, 140–151. doi: 10.1109/TOH.2015.2417570
Li, K., Fang, Y., Zhou, Y., and Liu, H. (2017). Non-invasive stimulation-based tactile sensation for upper-extremity prosthesis: a review. IEEE Sens. J. 17, 2625–2635. doi: 10.1109/JSEN.2017.2674965
Liburkina, S. P., Vasilyev, A. N., Yakovlev, L. V., Gordleeva, S. Y., and Kaplan, A. Y. (2018). A motor imagery-based brain–computer interface with vibrotactile stimuli. Neurosci. Behav. Physiol. 48, 1067–1077. doi: 10.1007/s11055-018-0669-2
Lin, C.-Y., Tsai, C.-M., Shih, P.-C., and Wu, H.-C. (2016). Development of a novel haptic glove for improving finger dexterity in poststroke rehabilitation. Technol. Health Care 24, S97–S103. doi: 10.3233/THC-151056
Lioi, G., Butet, S., Fleury, M., Bannier, E., Lécuyer, A., Bonan, I., et al. (2020). A multi-target motor imagery training using bimodal EEG-fMRI neurofeedback: a pilot study in chronic stroke patients. Front. Hum. Neurosci. 14:37. doi: 10.3389/fnhum.2020.00037
Lioi, G., Fleury, M., Butet, S., Lécuyer, A., Barillot, C., and Bonan, I. (2018). Bimodal eeg-fmri neurofeedback for stroke rehabilitation: a case report. Ann. Phys. Rehabil. Med. 61, e482–e483. doi: 10.1016/j.rehab.2018.05.1127
London, B. M., Torres, R. R., Slutzky, M. W., and Miller, L. E. (2011). “Designing stimulation patterns for an afferent BMI: representation of kinetics in somatosensory cortex,” in 2011 Annual International Conference of the IEEE Engineering in Medicine and Biology Society (IEEE), 7521–7524. doi: 10.1109/IEMBS.2011.6091854
López-Larraz, E., Sarasola-Sanz, A., Irastorza-Landa, N., Birbaumer, N., and Ramos-Murguialday, A. (2018). Brain-machine interfaces for rehabilitation in stroke: a review. Neurorehabilitation 43, 77–97. doi: 10.3233/NRE-172394
Lotte, F., Larrue, F., and Mühl, C. (2013). Flaws in current human training protocols for spontaneous brain-computer interfaces: lessons learned from instructional design. Front. Hum. Neurosci. 7:568. doi: 10.3389/fnhum.2013.00568
Lucas, L., DiCicco, M., and Matsuoka, Y. (2004). An emg-controlled hand exoskeleton for natural pinching. J. Robot. Mechatron. 16, 482–488. doi: 10.20965/jrm.2004.p0482
Lugo, Z. R., Rodriguez, J., Lechner, A., Ortner, R., Gantner, I. S., Laureys, S., et al. (2014). A vibrotactile P300-based brain–computer interface for consciousness detection and communication. Clin. EEG Neurosci. 45, 14–21. doi: 10.1177/1550059413505533
Lukoyanov, M. V., Gordleeva, S. Y., Pimashkin, A. S., Grigor'ev, N. A., Savosenkov, A. V., Motailo, A., et al. (2018). The efficiency of the brain-computer interfaces based on motor imagery with tactile and visual feedback. Hum. Physiol. 44, 280–288. doi: 10.1134/S0362119718030088
Massie, T. H., and Salisbury, J. K. (1994). “The phantom haptic interface: a device for probing virtual objects,” in Proceedings of the ASME Winter Annual Meeting, Symposium on Haptic Interfaces for Virtual Environment and Teleoperator Systems, Vol. 55 (Citeseer),295–300.
Mercado, V. R., Marchal, M., and Lécuyer, A. (2019). Entropia: towards infinite surface haptic displays in virtual reality using encountered-type rotating props. IEEE Trans. Vis. Comput. Graph. doi: 10.1109/TVCG.2019.2963190
Minogue, J., and Jones, M. G. (2006). Haptics in education: exploring an untapped sensory modality. Rev. Educ. Res. 76, 317–348. doi: 10.3102/00346543076003317
Mori, H., Matsumoto, Y., Struzik, Z. R., Mori, K., Makino, S., Mandic, D., et al. (2013). Multi-command tactile and auditory brain computer interface based on head position stimulation. arXiv 1301.6357.
Mrachacz-Kersting, N., Jiang, N., Stevenson, A. J. T., Niazi, I. K., Kostic, V., Pavlovic, A., et al. (2016). Efficient neuroplasticity induction in chronic stroke patients by an associative brain-computer interface. J. Neurophysiol. 115, 1410–1421. doi: 10.1152/jn.00918.2015
Müller-Putz, G., Scherer, R., Neuper, C., and Pfurtscheller, G. (2006). Steady-state somatosensory evoked potentials: suitable brain signals for brain-computer interfaces? IEEE Trans. Neural Syst. Rehabil. Eng. 14, 30–37. doi: 10.1109/TNSRE.2005.863842
Murguialday, A., Hill, J., Bensch, M., Martens, S., Halder, S., Nijboer, F., et al. (2011). Transition from the locked in to the completely locked-in state: a physiological analysis. Clin. Neurophysiol. 122, 925–933. doi: 10.1016/j.clinph.2010.08.019
Olausson, H., Wessberg, J., and Kakuda, N. (2000). Tactile directional sensibility: peripheral neural mechanisms in man. Brain Res. 866, 178–187. doi: 10.1016/S0006-8993(00)02278-2
Ono, T., Shindo, K., Kawashima, K., Ota, N., Ito, M., Ota, T., et al. (2014). Brain-computer interface with somatosensory feedback improves functional recovery from severe hemiplegia due to chronic stroke. Front. Neuroeng. 7:19. doi: 10.3389/fneng.2014.00019
Pacchierotti, C., Salvietti, G., Hussain, I., Meli, L., and Prattichizzo, D. (2016). “The hring: a wearable haptic device to avoid occlusions in hand tracking,” in 2016 IEEE Haptics Symposium (HAPTICS) (IEEE), 134–139. doi: 10.1109/HAPTICS.2016.7463167
Pacchierotti, C., Sinclair, S., Solazzi, M., Frisoli, A., Hayward, V., and Prattichizzo, D. (2017). Wearable haptic systems for the fingertip and the hand: taxonomy, review, and perspectives. IEEE Trans. Haptics 10, 580–600. doi: 10.1109/TOH.2017.2689006
Pfeiffer, M., and Rohs, M. (2017). “Haptic feedback for wearables and textiles based on electrical muscle stimulation,” in Smart Textiles (Springer), 103–137. doi: 10.1007/978-3-319-50124-6_6
Pfurtscheller, G., Guger, C., Müller, G., Krausz, G., and Neuper, C. (2000). Brain oscillations control hand orthosis in a tetraplegic. Neurosci. Lett. 292, 211–214. doi: 10.1016/S0304-3940(00)01471-3
Pfurtscheller, G., Müller, G. R., Pfurtscheller, J., Gerner, H. J., and Rupp, R. (2003). “Thought”-control of functional electrical stimulation to restore hand grasp in a patient with tetraplegia. Neurosci. Lett. 351, 33–36. doi: 10.1016/S0304-3940(03)00947-9
Pfurtscheller, G., and Neuper, C. (1997). Motor imagery activates primary sensorimotor area in humans. Neurosci. Lett. 239, 65–68. doi: 10.1016/S0304-3940(97)00889-6
Pfurtscheller, G., and Neuper, C. (2001). Motor imagery and direct brain-computer communication. Proc. IEEE 89, 1123–1134. doi: 10.1109/5.939829
Pichiorri, F., Morone, G., Petti, M., Toppi, J., Pisotta, I., Molinari, M., et al. (2015). Brain-computer interface boosts motor imagery practice during stroke recovery. Ann. Neurol. 77, 851–865. doi: 10.1002/ana.24390
Pillette, L. (2019). Redefining and adapting feedback for mental-imagery based brain-computer interface user training to the learners' traits and states (Ph.D. thesis), Université de Bordeaux, France.
Qiu, Z., Jin, J., Zhang, Y., Sun, H., and Wang, X. (2017). “Comparisons of three BCIs which do not rely on the visual modality,” in 2016 3rd International Conference on Systems and Informatics, ICSAI 2016, 82–86. doi: 10.1109/ICSAI.2016.7810934
Ramos-Murguialday, A., Broetz, D., Rea, M., Läer, L., Yilmaz, Ö., Brasil, F. L., et al. (2013). Brain-machine interface in chronic stroke rehabilitation: a controlled study. Ann. Neurol. 74, 100–108. doi: 10.1002/ana.23879
Ramos-Murguialday, A., Schürholz, M., Caggiano, V., Wildgruber, M., Caria, A., Hammer, E. M., et al. (2012). Proprioceptive feedback and brain computer interface (BCI) based neuroprostheses. PLoS ONE 7:e47048. doi: 10.1371/journal.pone.0047048
Richardson, B., and Symmons, M. (1995). Vibrotactile devices for the deaf: are they out of touch? Ann. Otol. Rhinol. Laryngol. Suppl. 166, 458–461.
Roll, J., Roll, R., Amblard, B., Berthoz, A., and Clarac, F. (1988). Posture and Gait: Development, Adaptation and Modulation. Excerpta Medica.
Rutkowski, T. M., and Mori, H. (2015). Tactile and bone-conduction auditory brain computer interface for vision and hearing impaired users. J. Neurosci. Methods 244, 45–51. doi: 10.1016/j.jneumeth.2014.04.010
Sato, M. (1991). Space interface device for artificial reality-spidar. The transactions of the Institute of Electronics. Inform. Commun. Eng. 74, 887–894.
Scheidt, R. A., Reinkensmeyer, D. J., Conditt, M. A., Rymer, W. Z., and Mussa-Ivaldi, F. A. (2000). Persistence of motor adaptation during constrained, multi-joint, arm movements. J. Neurophysiol. 84, 853–862. doi: 10.1152/jn.2000.84.2.853
Schreuder, M., Blankertz, B., and Tangermann, M. (2010). A new auditory multi-class brain-computer interface paradigm: spatial hearing as an informative cue. PLoS ONE 5:e9813. doi: 10.1371/journal.pone.0009813
Severens, M., Farquhar, J., Duysens, J., and Desain, P. (2013). A Multi-Signature Brain–Computer Interface: Use of Transient and Steady-State Responses. IOP Publishing.
Severens, M., Van der Waal, M., Farquhar, J., and Desain, P. (2014). Comparing tactile and visual gaze-independent brain-computer interfaces in patients with amyotrophic lateral sclerosis and healthy users. Clin. Neurophysiol. 125, 2297–2304. doi: 10.1016/j.clinph.2014.03.005
Shimizu, K., Mori, H., Makino, S., and Rutkowski, T. M. (2014). “Tactile pressure brain-computer interface using point matrix pattern paradigm,” in 2014 Joint 7th International Conference on Soft Computing and Intelligent Systems (SCIS) and 15th International Symposium on Advanced Intelligent Systems (ISIS) (IEEE), 473–477. doi: 10.1109/SCIS-ISIS.2014.7044756
Shu, X., Yao, L., Sheng, X., Zhang, D., and Zhu, X. (2017). Enhanced motor imagery-based BCI performance via tactile stimulation on unilateral hand. Front. Hum. Neurosci. 11:585. doi: 10.3389/fnhum.2017.00585
Sigrist, R., Rauter, G., Riener, R., and Wolf, P. (2013). Augmented visual, auditory, haptic, and multimodal feedback in motor learning: a review. Psychon. Bull. Rev. 20, 21–53. doi: 10.3758/s13423-012-0333-8
Sitaram, R., Ros, T., Stoeckel, L., Haller, S., Scharnowski, F., Lewis-Peacock, J., et al. (2017). Closed-loop brain training: the science of neurofeedback. Nat. Rev. Neurosci. 18, 86–100. doi: 10.1038/nrn.2016.164
Soekadar, S., Witkowski, M., Gómez, C., Opisso, E., Medina, J., Cortese, M., et al. (2016). Hybrid eeg/eog-based brain/neural hand exoskeleton restores fully independent daily living activities after quadriplegia. Sci. Robot. 1:eaag3296. doi: 10.1126/scirobotics.aag3296
Soekadar, S. R., Witkowski, M., Birbaumer, N., and Cohen, L. G. (2015). Enhancing hebbian learning to control brain oscillatory activity. Cereb. Cortex 25, 2409–2415. doi: 10.1093/cercor/bhu043
Soekadar, S. R., Witkowski, M., Mellinger, J., Ramos, A., Birbaumer, N., and Cohen, L. G. (2011). Erd-based online brain-machine interfaces (BMI) in the context of neurorehabilitation: optimizing BMI learning and performance. IEEE Trans. Neural Syst. Rehabil. Eng. 19, 542–549. doi: 10.1109/TNSRE.2011.2166809
Sollfrank, T., Ramsay, A., Perdikis, S., Williamson, J., Murray-Smith, R., Leeb, R., et al. (2016). The effect of multimodal and enriched feedback on SMR-BCI performance. Clin. Neurophysiol. 127, 490–498. doi: 10.1016/j.clinph.2015.06.004
Summers, I. R., Dixon, P. R., Cooper, P. G., Gratton, D. A., Brown, B. H., and Stevens, J. C. (1994). Vibrotactile and electrotactile perception of time-varying pulse trains. J. Acoust. Soc. Am. 95, 1548–1558. doi: 10.1121/1.408542
Thurlings, M. E., Brouwer, A.-M., Van Erp, J. B. F., Blankertz, B., and Werkhoven, P. J. (2012). Does bimodal stimulus presentation increase ERP components usable in BCIs? J. Neural Eng. 9:045005. doi: 10.1088/1741-2560/9/4/045005
van der Waal, M., Severens, M., Geuze, J., and Desain, P. (2012). Introducing the tactile speller: an ERP-based brain-computer interface for communication. J. Neural Eng. 9:045002. doi: 10.1088/1741-2560/9/4/045002
Van Erp, J., and Brouwer, A.-M. (2014). “Touch-based brain computer interfaces: state of the art,” in IEEE Haptics Symposium, HAPTICS, 397–401. doi: 10.1109/HAPTICS.2014.6775488
Vidaurre, C., and Blankertz, B. (2010). Towards a cure for BCI illiteracy. Brain Topogr. 23, 194–198. doi: 10.1007/s10548-009-0121-6
Ward, N., Brown, M., Thompson, A., and Frackowiak, R. (2003). Neural correlates of motor recovery after stroke: a longitudinal fMRI study. Brain 126, 2476–2496. doi: 10.1093/brain/awg245
Wege, A., and Hommel, G. (2005). “Development and control of a hand exoskeleton for rehabilitation of hand injuries,” in 2005 IEEE/RSJ International Conference on Intelligent Robots and Systems (IEEE), 3046–3051. doi: 10.1109/IROS.2005.1545506
Williams, R. L. II. (1998). Cable-suspended haptic interface. Int. J. Virtual Reality 3, 13–20. doi: 10.20870/IJVR.1998.3.3.2627
Wilson, A. J., Walton, M., Tyler, M., and Williams, J. (2012). Lingual electrotactile stimulation as an alternative sensory feedback pathway for brain–computer interface applications. J. Neural Eng. 9:045007. doi: 10.1088/1741-2560/9/4/045007
Wolf, K., and Bäder, T. (2015). “Illusion of surface changes induced by tactile and visual touch feedback,” in Proceedings of the 33rd Annual ACM Conference Extended Abstracts on Human Factors in Computing Systems, 1355–1360. doi: 10.1145/2702613.2732703
Wolpaw, J. R., Birbaumer, N., McFarland, D. J., Pfurtscheller, G., and Vaughan, T. M. (2002). Brain–computer interfaces for communication and control. Clin. Neurophysiol. 113, 767–791. doi: 10.1016/S1388-2457(02)00057-3
Yao, L., Sheng, X., Zhang, D., Jiang, N., Farina, D., and Zhu, X. (2017). A BCI system based on somatosensory attentional orientation. IEEE Trans. Neural Syst. Rehabil. Eng. 25, 78–87. doi: 10.1109/TNSRE.2016.2572226
Keywords: neurofeedback, BCI, haptic feedback, EEG, fMRI, multisensory, BMI, touch
Citation: Fleury M, Lioi G, Barillot C and Lécuyer A (2020) A Survey on the Use of Haptic Feedback for Brain-Computer Interfaces and Neurofeedback. Front. Neurosci. 14:528. doi: 10.3389/fnins.2020.00528
Received: 07 January 2020; Accepted: 28 April 2020;
Published: 23 June 2020.
Edited by:
Jose Luis Contreras-Vidal, University of Houston, United StatesReviewed by:
Surjo R. Soekadar, Charité–Universitätsmedizin Berlin, GermanyCopyright © 2020 Fleury, Lioi, Barillot and Lécuyer. This is an open-access article distributed under the terms of the Creative Commons Attribution License (CC BY). The use, distribution or reproduction in other forums is permitted, provided the original author(s) and the copyright owner(s) are credited and that the original publication in this journal is cited, in accordance with accepted academic practice. No use, distribution or reproduction is permitted which does not comply with these terms.
*Correspondence: Mathis Fleury, bWF0aGlzLmZsZXVyeUBpbnJpYS5mcg==
Disclaimer: All claims expressed in this article are solely those of the authors and do not necessarily represent those of their affiliated organizations, or those of the publisher, the editors and the reviewers. Any product that may be evaluated in this article or claim that may be made by its manufacturer is not guaranteed or endorsed by the publisher.
Research integrity at Frontiers
Learn more about the work of our research integrity team to safeguard the quality of each article we publish.