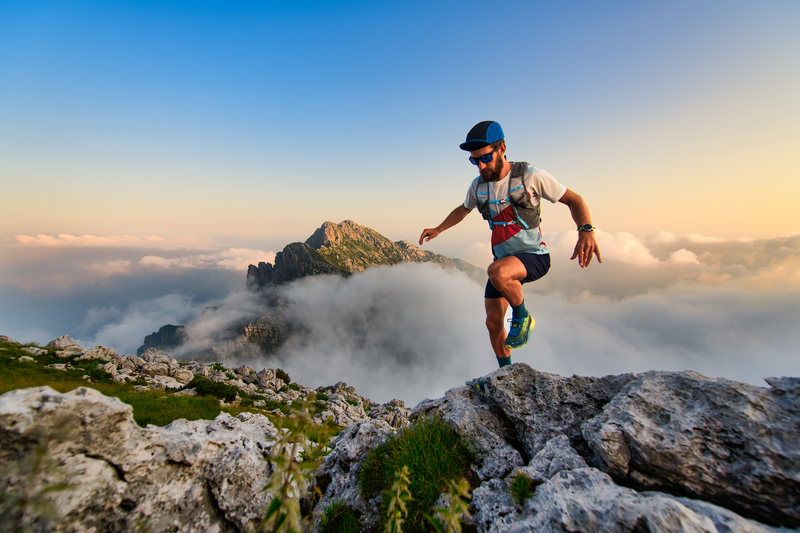
94% of researchers rate our articles as excellent or good
Learn more about the work of our research integrity team to safeguard the quality of each article we publish.
Find out more
ORIGINAL RESEARCH article
Front. Neurosci. , 11 March 2020
Sec. Autonomic Neuroscience
Volume 14 - 2020 | https://doi.org/10.3389/fnins.2020.00178
Pupillary unrest is an established indicator of drowsiness or sleepiness. How sympathetic and parasympathetic activity contribute to pupillary unrest is not entirely unclear. In this study, we investigated 83 young healthy volunteers to assess the relationship of pupillary unrest to other markers of the autonomic nervous system. Sample entropy (SE) and the established pupillary unrest index (PUI) were calculated to characterize pupil size variability. Autonomic indices were derived from heart rate, blood pressure, respiration, and skin conductance. Additionally, we assessed individual levels of calmness, vigilance, and mood. In an independent sample of 26 healthy participants, we stimulated the cardiovagal system by a deep breathing test. PUI was related to parasympathetic cardiac indices and sleepiness. A linear combination of vagal heart rate variability [root mean square of heart beat interval differences (RMSSD)] and skin conductance fluctuations (SCFs) was suited best to explain interindividual variance of PUI. Complexity of pupil diameter (PD) variations correlated to indices of sympathetic skin conductance. Furthermore, we found that spontaneous fluctuations of skin conductance are accompanied by increases of pupil size. In an independent sample, we were able to corroborate the relation of PUI with RMSSD and skin conductance. A slow breathing test enhanced RMSSD and PUI proportionally to each other, while complexity of PD dynamics decreased. Our data suggest that the slow PD oscillations (f < 0.15 Hz) quantified by PUI are related to the parasympathetic modulation. Sympathetic arousal as detected by SCFs is associated to transient pupil size increases that increase non-linear pupillary dynamics.
The pupil seems to offer unique insights into the human mind. For centuries, the pupillary system has been in the focus of psychophysiological research (Sirois and Brisson, 2014). Charles Darwin discovered pupillary reactions to fear and other emotions in animals (Darwin, 1872). Hess and colleagues reported that human pupils dilate in response to appealing images and statements (Hess and Polt, 1960; Hess, 1965; Hess et al., 1965). Since then, pupillometric investigations have been used in numerous studies to investigate emotional responses to visual (Bradley et al., 2008) or auditory presentation (Partala and Surakka, 2003; Gingras et al., 2015). Dilation of the pupils can also indicate cognitive load (Steinhauer et al., 2004; Eckstein et al., 2017). Pupil size has been shown to react to various types of cognitive demands, like memory load (Beatty, 1982), arithmetic tests (Võ et al., 2008), or speech processing (Stanners et al., 1979; Zekveld et al., 2010).
Moreover, the pupils are of great importance in clinical routine to evaluate autonomic function. The pupil’s reaction to light is part of every clinical examination (Cross, 1993). In contrary, little is known about pupillary fluctuations at rest. Lowenstein and Loewenfeld (1952) described spontaneous pupil diameter (PD) oscillations with high amplitude associated with sleepiness, referred to as “fatigue waves” (Lowenstein et al., 1963). The characteristic pattern of pupil size fluctuations can be quantified by the pupillary unrest index (PUI) which estimates the deviation of the PD at low frequencies (Lüdtke et al., 1998). In order to standardize the assessment of pupillary unrest, the pupillary sleepiness test was introduced (Wilhelm et al., 1998; Eggert et al., 2012). PUI is usually recorded over 11 min in a dark and quiet environment.
Borgdorff (1975) discovered slow changes of pupil size in phase with breathing. In several publications, the respiratory influence on pupil size fluctuations was investigated (Daum and Fry, 1981; Ohtsuka et al., 1988; Yoshida et al., 1994; Calcagnini and Lino, 1997; Onorati et al., 2013). Generally, sino-aortic baroreceptors are deemed responsible mediating respiratory changes of blood pressure that can be discovered in pupil size variation (Borgdorff, 1975; Häbler et al., 1994). Calcagnini et al. (2000, 2001) confirmed this explanation by stimulation of baroreceptors.
Furthermore, non-linear fluctuations seem to contribute to pupillary unrest (Mesin et al., 2013; Onorati et al., 2015; Schumann et al., 2015; Villalobos-Castaldi et al., 2016). The variety of influencing factors make spontaneous pupil size variations at rest complicated to quantify and to interpret (Usui and Stark, 1982; Mesin et al., 2013; Schumann et al., 2017b).
Both the sympathetic and parasympathetic outflow impact on pupillary muscles and modulate the size of the pupil. How pupillary unrest is affected by sympathetic activation is still illusive. Similar to pupillary reactions, skin conductance responds to emotional stimulation and cognitive load (Kohlisch and Schaefer, 1996; Najström and Jansson, 2007; Reimer and Mehler, 2011). In contrast, the electrodermal system is innervated by sympathetic sudomotor nerves only. Skin conductance reactions recorded at the hand have a latency of about 2–2.5 s due to the slow signal transmission via unmyelinated fibers (Lim et al., 2003; Bradley et al., 2008). Without a given external stimulus, spontaneous skin conductance fluctuations (SCFs) indicate sympathetic tone in face of stress or anxiety etc. (see review by Kreibig, 2010).
Since changes of the pupillary motility have been discovered in several diseases (Bär et al., 2008; Graur and Siegle, 2013), the assessment of pupillary fluctuations promises some clinical value. Recently, we demonstrated differences of pupillary unrest in patients suffering from major depression (Schumann et al., 2017a). Heart rate, skin conductance, and PUI were shown to accurately classify patients and controls (accuracy of 88.5%). In healthy subjects, PUI correlated mainly with vagal cardiovascular measures. However, the sample was rather small and somewhat heterogeneous with respect to age and gender.
Here, we investigated a larger group of healthy volunteers. We aimed to replicate and further investigate the association of pupillary unrest with other autonomic indices. An additional measure complexity of pupil size variations was estimated to quantify non-linear pupillary dynamics. Furthermore, a deep breathing test was applied in order to modulate cardiovagal function to gain insights on the dependency of pupillary dynamics on autonomic cardiac status.
We assessed physiological data at rest in 83 healthy volunteers [59 females, age: 23 ± 2 years, body mass index (BMI): 21.9 ± 2.6]. The multidimensional test for mental states (MDBF) was used to evaluate subjective ratings of mood, calmness, and vigilance (Steyer et al., 1994). Demographic data and individual ratings of the three dimensions of the mental state are depicted in Table 1.
Healthy subjects had no present or past history of psychiatric, neurological, or other clinically significant disorders. This was excluded by taking the medical history and by a full clinical examination of all subjects. All participants gave their informed written consent in accordance with the protocol approved by the Ethics Committee of Jena.
The examination room was quiet and fully shaded with a low intensity ambient light source. Additionally, participants wore headphones to be isolated from a potential surrounding noise. Via a monitor fixed over the couch a dark gray ellipse was displayed on light gray background as fixation anchor. Room temperature was controlled to 22°C. Resting recordings were conducted in supine position for 15 min. The first 5 min were not analyzed, to exclude the adjustment period to the environment.
In 26 subjects (see Table 2), resting recordings were followed by a deep breathing test. Subjects were instructed to breathe at a fixed rate of 6 breaths/min for 5 min (Low et al., 2013). An audio track of deep ventilatory noises was given via headphones to indicate the aspired respiration rate without any change of visual presentation.
We used the MP150 system (BIOPAC Systems Inc., Goleta, CA, United States) to record multiple physiological signals simultaneously at 1000 Hz sampling rate (Schumann et al., 2017c). ECG was acquired by three electrodes on the chest according to an adjusted Einthoven triangle. The non-invasive blood pressure was measured continuously by the vascular unloading technique. ECG and blood pressure were band-pass filtered between 0.05 and 35 Hz. Abdominal and thoracic respiratory movement were recorded by two individual strain gauge transducers and low pass filtered at 10 Hz. Skin conductance was measured by constant voltage technique at the left hand’s palm with electrodes placed on the thenar and hypothenar eminence.
Heart beats were extracted automatically and checked manually offline. Artifacts and ectopic beats were detected and interpolated using an adaptive filtering technique (Wessel et al., 2000). Maximum and minimum blood pressure values embedded in one cardiac cycle were transferred to systolic and diastolic blood pressure (DBP) time series.
The pupil size was assessed every 4 ms by the infrared camera system RED 250 (SensoMotoric Inc., Boston, MA, United States). Blinks distorting PD recordings as sudden drops with duration typically less than half a second (Schleicher et al., 2008) were eliminated using a median filter with 1000 ms time window followed by temporal smoothing (400 ms). This procedure removes blinks reliably without considerable influence on the underlying PD signal.
The heart rate HR and its short-term variability root mean square of heart beat interval differences (RMSSD) were estimated according to the established standard procedures (TaskForce, 1996). Additionally, respiratory sinus arrhythmia was calculated using the peak-valley-approach. Mean values of systolic blood pressure (SBP) and DBP, and breathing rate (BR) were assessed. Variability of blood pressure and respiratory cycles were quantified using the standard deviation (sdRC, sdSBP, sdDBP). Baroreflex sensitivity was estimated by the sequence method (Wessel et al., 2000). We analyzed bradycardic baroreflex sequences, i.e., the over three consecutive increases of beat-to-beat intervals accompanied by three increasing SBP values.
Spontaneous fluctuations of skin conductance (SCF, non-specific responses) were extracted from the raw signal, exploiting their typical shape (Lim et al., 1997). Skin conductance level (SCL) was estimated by averaging the whole signal. SCL and SCF were log-transformed.
Rapid regulation of heart rate as measured by RMSSD and BRS are supposed to indicate parasympathetic cardiac function (Hayano et al., 1991; Malik et al., 1996). As skin conductance is determined by sympathetic sudomotor activity, SCL and SCF are used to quantify sympathetic arousal (Bach et al., 2010).
To test whether non-specific fluctuations of skin conductance (SCF) are accompanied by pupillary responses, we extracted and averaged segments of the pupillary signal based on SCF occurrence. Phase-rectified signal averaging (PRSA) is a framework to detect and analyze recurrent patterns in non-stationary signals (Bauer et al., 2006). In the bivariate approach, a trigger signal is used to define anchor points that indicate the occurrence of a certain pattern in another signal. We used the onsets of SCF as anchors to assess the concurrent change of pupil size (blue circles in step 1, Figure 1, see Schumann et al., 2019).
Figure 1. Schematic illustration of the bivariate phase rectified signal averaging (PRSA) procedure. Step 1: Onsets of skin conductance fluctuations (SCF) served as anchor points (blue circles). Randomly defined anchor points were used as reference condition (black circles). Step 2: Anchor points were transferred to the pupil diameter signal (PD) with a temporal delay of 2.5 s as the mean cross correlation function of skin conductance and PD changes reached its maximum at this time lag. Step 3: Delay-compensated anchor points were used to extract segments of pupil diameter responses (PDR). Step 4: Segments were phase-rectified and normalized. Step 5: PDR segments were averaged and analyzed.
We accounted for the significantly higher reaction delay of the electrodermal system (about 2–2.5 s, Lim et al., 1999; Lim et al., 2003; Rachow et al., 2011) when compared to the pupillomotor system (about 0.2 s, Korn and Bach, 2016). Therefore, the cross-correlation function of the temporal derivatives of both signals were estimated, normalized (divided by maximum), and averaged over all subjects. A representative time lag was extracted when skin conductance and pupil size changes are synchronized (maximum average cross-correlation). This time span was used to delay the anchor time points in the pupillary signal (step 2, Figure 1).
Pupil diameter responses (PDRs) over 7 s were extracted and normalized to the average pupil size in an interval 1 s prior to the anchor time point (baseline) (step 3 and 4 in Figure 1). PDRs were averaged per subject and characterized by the maximum pupil dilation and area under the curve (AUC) (step 5 in Figure 1).
To evaluate specificity of the concurrent change of pupil size, a control condition was designed by using non-SCF time points for bivariate PRSA (black circles in step 1 in Figure 1). The same number of time points as SCF actually detected in the respective subject was randomly chosen (at least 1 s distance to an SCF) as anchor points using a self-written MATLAB script based on the built-in function randperm.m (R2012a, The MathWorks, Natick, MA, United States). Changes of pupil size and skin conductance were extracted, averaged, and quantified the same way as before.
Sample entropy (SE) quantifies complexity of a time series by estimating the probability that similar subseries also match at the next point of comparison. It is defined as the negative natural logarithm of a conditional probability estimate that series of length m match within tolerance r at the current and the next point (Richman and Moorman, 2000). The time series of PD sampled at 100 ms was analyzed using m = 5 and r = 0.2∗sdPD, i.e., standard deviation of the time series.
The PUI was introduced to evaluate sleepiness by quantifying spontaneous fluctuation (Lüdtke et al., 1998). According to the standard calculation of PUI, mean diameter values in non-overlapping segments lasting L = 640 ms were extracted. This is a procedure similar to low-pass filtering in order to exclude high frequency noise form PUI computation. Therefore, PUI primarily quantifies slow pupil size oscillations below 1.56 Hz. Absolute differences of these values were summed up and averaged per minute (Lüdtke et al., 1998).
Bivariate linear dependencies between pupillary unrest to behavioral measures and autonomic indices were assessed by Pearson’s correlation coefficient r and respective p-value. In correlative analyses, statistical thresholds were corrected for multiple comparisons using false discovery rate (Benjamini and Hochberg, 1995). Statistical significance with 5% type-I error was assumed at p < 0.006. A multiple regression model was used to assess to which extent interindividual variance of PUI can be explained by other observed autonomic parameters. Correlational analyses were performed in the replication sample of 26 subjects at rest and during deep breathing. Additionally, autonomic and pupillometric indices were compared in the two conditions using a two-sample t-test (rest vs. deep breathing).
Estimates of pupillary unrest, heart rate, blood pressure, respiration, and skin conductance are listed in Table 3. Subjects in this sample had a mean heart rate of HR = 67/min with a variability of RMSSD = 55.7 ms and relatively low blood pressure values of 109/67 mmHg (systolic/diastolic) on average. There were no significant differences between female and male participants (Table 3).
The standard PUI and SE of PD was estimated to quantify slow variations and complexity of pupil size.
Sample entropy of PD was proportional to skin conductance parameters (SCL, SCF, see Table 4). PUI correlated with vagal cardiovascular indices (RMSSD, BRS). With regard to the mental state, a significant correlation of PUI and vigilance was found (r = −0.354, p < 0.006). A linear regression model revealed that the variance of PUI can be explained by RMSSD (b = 0.476) and SCF (b = −0.269) to an extent of R2 = 0.30 (p < 0.001).
The cross-correlation function of temporal derivatives of skin conductance and pupil signals revealed a maximum correlation at a delay of 2501 ms (see Figure 1). The averaged change of PD is depicted together with the mean time course of spontaneous SCFs (SC) in Figure 2A. In gray, the segments extracted from non-SCF (randomly defined anchor points) are illustrated that served as control condition. SCFs are accompanied by PD increases of 9.1 ± 9.4% on average. The one sample t-test indicated a positive area under the PDR curve (70 ± 110 n.u., p < 0.001). Both measures were significantly higher when PDR extraction was triggered by SCF when compared to the control condition with randomly defined anchor points (both p < 0.001, Figure 2B). Maximum PD change was correlated to PUI (r = 0.545, p < 0.001).
Figure 2. Mean changes of pupil diameter (PD) in phase with fluctuations of skin conductance (SC). (A) Averaged change of pupil diameter (PD) normalized to a baseline 500 ms before the reference time point t = 0. (B) Averaged fluctuations of skin conductance (SCF) used as events to analyze PD reactions. Reference time point t = 0 was extracted 2500 ms prior to each SCF onset. SC changes were normalized to a baseline 500 ms before the reference time point. ∗∗∗p < 0.001.
In a group of 26 subjects, a deep breathing test was performed after the resting state measurement. The test had a significant impact on autonomic indices as well as pupillary unrest (see Table 5). Respiratory measures indicated that subjects succeeded in breathing steadily at a rate of 6 breaths/min. As we expected, cardiovagal parameters such as RMSSD and BRS were enhanced during deep breathing. The test had significant effects on mean blood pressure values (SBP, DBP) but not on average heart rate. While, pupil size and PUI increased during the test, complexity of PD in terms of SE decreased.
Correlation coefficients of PD variability and autonomic indices in both conditions are depicted in Table 6. A significant correlation of PUI to RMSSD and skin conductance parameters (SCF and SCL) remained during deep breathing. Most interestingly, the increase of RMSSD during the breathing maneuver was proportional to the increase of PUI (r = 0.47, p < 0.01; see Figure 3).
Table 6. Correlation analysis of pupillary dynamics and autonomic indices at rest and deep breathing.
Figure 3. Effect of deep breathing on short-term heart rate variability (RMSSD) and pupillary unrest index (PUI). Increases of both parameters during breathing test were correlated (r = 0.47, p < 0.01). ∗ p < 0.05, ∗∗ p < 0.01.
In this study, the relation of pupillary unrest to other autonomic markers was assessed in young healthy volunteers. The classical PUI was related to parasympathetic cardiac indices and sleepiness. We found that a linear combination of vagal heart rate variability (RMSSD) and skin conductance fluctuations (SCFs) was suited best to explain interindividual variance of PUI. Complexity of PD variations correlated to indices of sympathetic skin conductance. The association of skin conductance and pupillary dynamics might be due to increases of pupil size concurrent to spontaneous SCF. In an independent sample, we were able to corroborate the correlation of pupillary unrest and heart rate variability and skin conductance. A slow breathing test enhanced cardiovagal function in this sample. While pupillary unrest was also increased, complexity of PD decreased.
The correlation of PUI with baroreflex sensitivity and heart rate variability (RMSSD) validated a link between pupillary unrest and parasympathetic cardiac modulation as reported previously (Schumann et al., 2015, 2017a). A significant correlation between PUI and individual levels of vigilance was found as well. This is in line with the idea that sleepiness induces slow rhythmic changes of pupil size that are also referred to as pupillary hippus or fatigue waves (Lowenstein and Loewenfeld, 1952, 1962; Hornung, 1968; Bouma and Baghuis, 1971). Recent research demonstrated that these pupillary oscillations are associated primarily by parasympathetic influence (Turnbull et al., 2017).
In a deep breathing test, we aimed at stimulating cardiovagal function. It has been suggested that deep breathing modulates rhythmic discharge of vagal efferent fibers without enhancing the overall level of vagal outflow (Brown et al., 1993). This leads to an increase of HRV but does not affect average HR—as we have found in our study. Since RMSSD and PUI increased proportionally to each other, we gained further evidence indicating that pupillary unrest is related to vagal modulation of cardiac function. Activity of neurons in important regulatory brainstem regions, such as the nucleus ambiguous, is modulated by respiration (see Garcia et al., 2013). At this level, systems controlling oculomotor and cardiovagal function seem to be interconnected (Borgdorff, 1975; Calcagnini et al., 2001).
While oscillatory variations of pupil size increased, non-linear complexity of pupillary dynamics decreased during deep breathing. Both during rest and breathing maneuver, SE of PD was positively correlated to skin conductance indices indicating that sympathetic arousal seems to relate to non-linear pupillary dynamics. Since an impressive co-variation of neuronal firing rate in the locus coeruleus (LC) and pupil dilation was reported (Aston-Jones and Cohen, 2005; Costa and Rudebeck, 2016), pupillary responses have been considered as a proxy of noradrenergic activity in humans (Sirois and Brisson, 2014; Eckstein et al., 2017; Schumann et al., 2018). LC activity can be decomposed into a tonic and phasic component. Tonic activity represents a kind of baseline arousal and is low during certain automatic behavior and drowsiness (Rajkowski et al., 1994; Aston-Jones et al., 1999). LC neurons become phasically activated by salient external or internal stimuli (Corbetta et al., 2008; Köhler et al., 2016). Analogously, skin conductance was divided into a tonic and phasic constituent and is most probably also influenced by the LC-noradrenergic system (Yamamoto et al., 1990). Our results indicate that phasic fluctuations of sympathetic skin conductance are synchronized with PD increases. The amplitude of pupillary responses is related to PUI, whereas the occurrence of SCF was linked to non-linear nature of PD. Using functional magnetic resonance imaging, Breeden et al. (2017) highlighted that neural correlates to fluctuations of pupil size and skin conductance have a strong overlap. In accordance to this finding, other studies suggest that changes of pupil size are mainly regulated by the salience network that contains structures related to sympathetic control (Beissner et al., 2013; Schneider et al., 2016; Breeden et al., 2017). This functional brain network is supposed to process sensory information in order to prepare executive action when salient stimuli are detected (Seeley et al., 2007). In the absence of external stimulation, thoughts or ideas during mind-wandering might elicit changes of pupil size (Smallwood et al., 2011; Grandchamp et al., 2014; Urai et al., 2017).
In a previous study, we found increased PUI values in patients with major depression that were not related to vagal HRV as in healthy controls (Schumann et al., 2017a). Furthermore, the SCF occurrence was elevated in patients. Considering the results of the present study, pupillary fluctuations associated to sympathetic arousal might have had stronger impact on PUI in patients. Most probably, internal stimuli with negative value are more predominant in patients as rumination, negative thoughts and worries are consistent symptoms related to depression (Nolen-Hoeksema et al., 2008). Therefore, elevated PUI in patients with major depression might be a consequence of a predominantly sympathetic influence on the pupil.
The reader has to keep in mind that we did not assess autonomic activity invasively. As parasympathetic and sympathetic activation are not mutually independent both branches influence each other at multiple levels of autonomic control. For instance, the activity of the sinoatrial node is modulated by parasympathetic and sympathetic impact in a widely antagonistic fashion. In contrast, skin conductance is exclusively modulated by sudomotor activity and indicates sympathetic arousal (Bach et al., 2010). Another limitation of the study is the age difference between the larger sample and the test sample that performed the deep breathing maneuver. In spite this heterogeneity, we were able to replicate a relation of pupillary unrest with heart rate variability and skin conductance.
In summary, we demonstrated that pupillary unrest is related to both sympathetic and parasympathetic markers of autonomic control. Slow PD oscillations (f < 0.15 Hz) quantified by PUI were related to cardiovagal function. Sympathetic arousal as detected by SCFs was associated to transient pupil size increases that increase non-linear complexity of pupil size variations. The investigation of the reported relationships during different autonomic challenges might reveal further insights on the modulation of pupillary unrest.
The datasets generated for this study are available on request to the corresponding author.
The studies involving human participants were reviewed and approved by the Ethikkommission der Medizinischen Fakultät der Friedrich-Schiller-Universität Jena. The patients/participants provided their written informed consent to participate in this study.
AS contributed to analysis and interpretation of the data and preparing the manuscript. SK contributed to acquisition of the data. JE contributed to quality control and preprocessing of the data. KB contributed to study conception, preparing the manuscript, and critical revision.
The authors declare that the research was conducted in the absence of any commercial or financial relationships that could be construed as a potential conflict of interest.
Aston-Jones, G., and Cohen, J. D. (2005). An integrative theory of locus coeruleus-norepinephrine function: adaptive gain and optimal performance. Annu. Rev. Neurosci. 28, 403–450. doi: 10.1146/annurev.neuro.28.061604.135709
Aston-Jones, G., Rajkowski, J., and Cohen, J. (1999). Role of locus coeruleus in attention and behavioral flexibility. Biol. Psychiatry 46, 1309–1320. doi: 10.1016/S0006-3223(99)00140-7
Bach, D. R., Friston, K. J., and Dolan, R. J. (2010). Analytic measures for quantification of arousal from spontaneous skin conductance fluctuations. Int. J. Psychophysiol. 76, 52–55. doi: 10.1016/j.ijpsycho.2010.01.011
Bär, K.-J., Boettger, M. K., Schulz, S., Harzendorf, C., Agelink, M. W., Yeragani, V. K., et al. (2008). The interaction between pupil function and cardiovascular regulation in patients with acute schizophrenia. Clin. Neurophysiol. 119, 2209–2213. doi: 10.1016/j.clinph.2008.06.012
Bauer, A., Kantelhardt, J., Bunde, A., Barthel, P., Schneider, R., Malik, M., et al. (2006). Phase-rectified signal averaging detects quasi-periodicities in non-stationary data. Phys. A Stat. Mech. Appl. 364, 423–434. doi: 10.1016/j.physa.2005.08.080
Beatty, J. (1982). Task evoked pupillary responses, processing load, and the structure of resources. Psychol. Bull. 91, 276–292.
Beissner, F., Meissner, K., Bär, K.-J., and Napadow, V. (2013). The autonomic brain: an activation likelihood estimation meta-analysis for central processing of autonomic function. J. Neurosci. 33, 10503–10511. doi: 10.1523/JNEUROSCI.1103-13.2013
Benjamini, Y., and Hochberg, Y. (1995). Controlling the false discovery rate: a practical and powerful approach to multiple testing. J. R. Stat. Soc. 57, 289–300. doi: 10.2307/2346101
Bouma, H., and Baghuis, L. C. (1971). Hippus of the pupil: periods of slow oscillations of unknown origin. Vision Res. 11, 1345–1351. doi: 10.1016/0042-6989(71)90016-2
Bradley, M. B., Miccoli, L. M., Escrig, M. A., and Lang, P. J. (2008). The pupil as a measure of emotional arousal and automatic activation. Psychophysiology 45, 602–607. doi: 10.1111/j.1469-8986.2008.00654.x.The
Breeden, A. L., Siegle, G. J., Norr, M. E., Gordon, E. M., and Vaidya, C. J. (2017). Coupling between spontaneous pupillary fluctuations and brain activity relates to inattentiveness. Eur. J. Neurosci. 45, 260–266. doi: 10.1111/ejn.13424
Brown, T. E., Beightol, L. A., Koh, J., and Eckberg, D. L. (1993). Important influence of respiration on human R-R interval power spectra is largely ignored. J. Appl. Physiol. 75, 2310–2317. doi: 10.1152/jappl.1993.75.5.2310
Calcagnini, G., Censi, F., Lino, S., and Cerutti, S. (2000). “Pupil diameter variability in humans,” in Proceedings of the 22nd Annual International Conference of the IEEE, Vol. 3, (Piscataway, NJ: IEEE), 2298–2301. doi: 10.1109/IEMBS.2000.900601
Calcagnini, G., Giovannelli, P., Censi, F., Bartolini, P., and Barbaro, V. (2001). “Baroreceptor-sensitive fluctuations of heart rate and pupil diameter,” in Proceedings of the Annual International Conference of the IEEE Engineering in Medicine and Biology Society, Vol. 1, (Piscataway, NJ: IEEE), 600–603. doi: 10.1109/IEMBS.2001.1019006
Calcagnini, G., and Lino, S. (1997). Cardiovascular autonomic rhythms in spontaneous pupil fluctuations. Comput. Cardiol. 24, 133–136.
Corbetta, M., Patel, G., and Shulman, G. L. (2008). The reorienting system of the human brain: from environment to theory of mind. Neuron 58, 306–324. doi: 10.1016/j.neuron.2008.04.017
Costa, V. D., and Rudebeck, P. H. (2016). More than meets the eye: the relationship between pupil size and locus coeruleus activity. Neuron 89, 8–10. doi: 10.1016/j.neuron.2015.12.031
Cross, S. A. (1993). “Autonomic disorders of the pupil, ciliar body, and lacrimal apparatus,” in Clinical Autonomic Disorders: Evaluation and Management. Library of Congress, ed. P. A. Low, (Boston, MA: Little Brown and Company), 263–277.
Darwin, C. (1872). The Expression of the Emotions in Man and Animals. London: John Marry, 374. doi: 10.1037/h0076058
Daum, K. M., and Fry, G. A. (1981). The component of physiological pupillary unrest correlated with respiration. Am. J. Optom. Physiol. Opt. 58, 831–840.
Eckstein, M. K., Guerra-Carrillo, B., Miller Singley, A. T., and Bunge, S. A. (2017). Beyond eye gaze: What else can eyetracking reveal about cognition and cognitive development? Dev. Cogn. Neurosci. 25, 69–91. doi: 10.1016/j.dcn.2016.11.001
Eggert, T., Sauter, C., Popp, R., Zeitlhofer, J., and Danker-Hopfe, H. (2012). The pupillographic sleepiness test in adults: effect of age, gender, and time of day on pupillometric variables. Am. J. Hum. Biol. 24, 820–828. doi: 10.1002/ajhb.22326
Garcia, A. J., Koschnitzky, J. E., Dashevskiy, T., and Ramirez, J. M. (2013). Cardiorespiratory coupling in health and disease. Auton. Neurosci. Basic Clin. 175, 26–37. doi: 10.1016/j.autneu.2013.02.006
Gingras, B., Marin, M. M., Puig-Waldmüller, E., and Fitch, W. T. (2015). The Eye is listening: music-induced arousal and individual differences predict pupillary responses. Front. Hum. Neurosci. 9:619. doi: 10.3389/fnhum.2015.00619
Grandchamp, R., Braboszcz, C., and Delorme, A. (2014). Oculometric variations during mind wandering. Front. Psychol. 5:31. doi: 10.3389/fpsyg.2014.00031
Graur, S., and Siegle, G. (2013). Pupillary motility: bringing neuroscience to the psychiatry clinic of the future. Curr. Neurol. Neurosci. Rep. 13, 1–13. doi: 10.1016/j.biotechadv.2011.08.021.Secreted
Häbler, H.-J., Jänig, W., and Michaelis, M. (1994). Respiratory modulation in the activity of sympathetic neurones. Prog. Neurobiol. 43, 567–606.
Hayano, J., Sakakibara, Y., Yamada, A., Yamada, M., Mukai, S., Fujinami, T., et al. (1991). Accuracy of assessment of cardiac vagal tone by heart rate variability in normal subjects. Am. J. Cardiol. 67, 199–204.
Hess, E. H. (1965). Attitude and pupil size. Sci. Am. 212, 46–54. doi: 10.1038/scientificamerican0465-46
Hess, E. H., and Polt, J. M. (1960). Pupil size as related to interest value of visual stimuli. Science 132, 349–350. doi: 10.1126/science.132.3423.349
Hess, E. H., Seltzer, A. L., and Shlien, J. M. (1965). Pupil response of hetero- and homosexual males to pictures of men and women: a pilot study. J. Abnorm. Psychol. 70, 165–168. doi: 10.1037/h0021978
Hornung, J. (1968). Über das Frequenzverhalten der menschlichen Pupille. Doc. Ophthalmol. 25, 21–42. doi: 10.1007/BF02550817
Köhler, S., Bär, K. J., and Wagner, G. (2016). Differential involvement of brainstem noradrenergic and midbrain dopaminergic nuclei in cognitive control. Hum. Brain Mapp. 37, 2305–2318. doi: 10.1002/hbm.23173
Kohlisch, O., and Schaefer, F. (1996). Physiological changes during computer tasks: responses to mental load or to motor demands? Ergonomics 39, 213–224. doi: 10.1080/00140139608964452
Korn, C. W., and Bach, D. R. (2016). A solid frame for the window on cognition: modeling event-related pupil responses. J. Vis. 16:28. doi: 10.1167/16.3.28
Kreibig, S. D. (2010). Autonomic nervous system activity in emotion: a review. Biol. Psychol. 84, 394–421. doi: 10.1016/j.biopsycho.2010.03.010
Lim, C., Seto-Poon, M., Clouston, P., and Morris, J. G. (2003). Sudomotor nerve conduction velocity and central processing time of the skin conductance response. Clin. Neurophysiol. 114, 2172–2180. doi: 10.1016/S1388-2457(03)00204-9
Lim, C. L., Gordon, E., Rennie, C., Wright, J. J., Bahramali, H., Li, W. M., et al. (1999). Dynamics of SCR, EEG, and ERP activity in an oddball paradigm with short interstimulus intervals. Psychophysiology 36, 543–551. doi: 10.1017/S0048577299971226
Lim, C. L., Rennie, C., Barry, R. J., Bahramali, H., Lazzaro, I., Manor, B., et al. (1997). Decomposing skin conductance into tonic and phasic components. Int. J. Psychophysiol. 25, 97–109.
Low, P. A., Tomalia, V. A., and Park, K.-J. (2013). Autonomic function tests: some clinical applications. J. Clin. Neurol. 9, 1–8. doi: 10.3988/jcn.2013.9.1.1
Lowenstein, O., Feinberg, R., and Loewenfeld, I. I. E. (1963). Pupillary movements during acute and chronic fatigue a new test for the objective evaluation of tiredness. Investig. Ophthalmol. 2, 138–158.
Lowenstein, O., and Loewenfeld, I. (1952). Disintegration of central autonomic regulation during fatigue and its reintegration by psychosensory controlling mechanisms. II. Reintegration; pupillographic studies. J. Nerv. Ment. Dis. 115, 121–145.
Lowenstein, O., and Loewenfeld, I. E. (1962). “The Pupil,” in The Eye, Vol. 3, ed. H. Davson, (New York, NY: Academic Press), 256–329.
Lüdtke, H., Wilhelm, B., Adler, M., Schaeffel, F., and Wilhelm, H. (1998). Mathematical procedures in data recording and processing of pupillary fatigue waves. Vision Res. 38, 2889–2896.
Malik, M., Bigger, J., Camm, A., and Kleiger, R. (1996). Heart rate variability. standards of measurement, physiological interpretation, and clinical use. task force of the european society of cardiology and the north American society of pacing and electrophysiology. Eur. Heart J. 17, 354–381.
Mesin, L., Monaco, A., and Cattaneo, R. (2013). Investigation of nonlinear pupil dynamics by recurrence quantification analysis. Biomed Res. Int. 2013:420509. doi: 10.1155/2013/420509
Najström, M., and Jansson, B. (2007). Skin conductance responses as predictor of emotional responses to stressful life events. Behav. Res. Ther. 45, 2456–2463. doi: 10.1016/j.brat.2007.03.001
Nolen-Hoeksema, S., Wisco, B. E., and Lyubomirsky, S. (2008). Rethinking rumination. Perspect. Psychol. Sci. 3, 1301–1307.
Ohtsuka, K., Asakura, K., Kawasaki, H., and Sawa, M. (1988). Respiratory fluctuations of the human pupil. Exp. Brain Res. 71, 215–217. doi: 10.1007/BF00247537
Onorati, F., Barbieri, R., Mauri, M., Russo, V., and Mainardi, L. (2013). Characterization of affective states by pupillary dynamics and autonomic correlates. Front. Neuroeng. 6:9. doi: 10.3389/fneng.2013.00009
Onorati, F., Mainardi, L. T., Sirca, F., Russo, V., and Barbieri, R. (2015). Nonlinear analysis of pupillary dynamics. Biomed. Tech. 61, 95–106. doi: 10.1515/bmt-2015-0027
Partala, T., and Surakka, V. (2003). Pupil size variation as an indication of affective processing. Int. J. Hum. Comput. Stud. 59, 185–198. doi: 10.1016/S1071-5819(03)00017-X
Rachow, T., Berger, S., Boettger, M. K., Schulz, S., Guinjoan, S., Yeragani, V. K., et al. (2011). Nonlinear relationship between electrodermal activity and heart rate variability in patients with acute schizophrenia. Psychophysiology 48, 1323–1332. doi: 10.1111/j.1469-8986.2011.01210.x
Rajkowski, J., Kubiak, P., and Aston-Jones, G. (1994). Locus coeruleus activity in monkey: phasic and tonic changes are associated with altered vigilance. Brain Res. Bull. 35, 607–616.
Reimer, B., and Mehler, B. (2011). The impact of cognitive workload on physiological arousal in young adult drivers: a field study and simulation validation. Ergonomics 54, 932–942. doi: 10.1080/00140139.2011.604431
Richman, J. S., and Moorman, J. R. (2000). Physiological time-series analysis using approximate entropy and sample entropy maturity in premature infants Physiological time-series analysis using approximate entropy and sample entropy. Am. J. Physiol. Hear. Circ. Physiol. 278, H2039–H2049.
Schleicher, R., Galley, N., Briest, S., and Galley, L. (2008). Blinks and saccades as indicators of fatigue in sleepiness warnings: Looking tired? Ergonomics 51, 982–1010. doi: 10.1080/00140130701817062
Schneider, M., Hathway, P., Leuchs, L., Sämann, P. G., Czisch, M., and Spoormaker, V. I. (2016). Spontaneous pupil dilations during the resting state are associated with activation of the salience network. Neuroimage 139, 189–201. doi: 10.1016/j.neuroimage.2016.06.011
Schumann, A., Andrack, C., and Bär, K. J. (2017a). Differences of sympathetic and parasympathetic modulation in major depression. Prog. Neuropsychopharmacol. Biol. Psychiatry 79, 324–331. doi: 10.1016/j.pnpbp.2017.07.009
Schumann, A., Ebel, J., and Bär, K. J. (2017b). Forecasting transient sleep episodes by pupil size variability. Curr. Dir. Biomed. Eng. 3, 583–586. doi: 10.1515/cdbme-2017-0121
Schumann, A., Kietzer, S., Ebel, J., and Bär, K. J. (2019). “The relation of skin conductance and pupillary fluctuations assessed by phase-rectified signal averaging,” in Proceedings of the Annual International Conference of the IEEE Engineering in Medicine and Biology Society EMBS, (Piscataway, NJ: IEEE). doi: 10.1109/EMBC.2019.8857713
Schumann, A., Köhler, S., De La Cruz, F., Güllmar, D., Reichenbach, J. R., Wagner, G., et al. (2018). The use of physiological signals in brainstem/midbrain fMRI. Front. Neurosci. 12:718. doi: 10.3389/fnins.2018.00718
Schumann, A., Kralisch, C., and Bär, K. J. (2015). “Spectral decomposition of pupillary unrest using wavelet entropy,” in Proceedings of the Annual International Conference of the IEEE Engineering in Medicine and Biology Society EMBS, (Piscataway, NJ: IEEE), 6154–6157. doi: 10.1109/EMBC.2015.7319797
Schumann, A., Schulz, S., Voss, A., Scharbrodt, S., Baumert, M., and Bär, K. J. (2017c). Baroreflex coupling assessed by cross-compression entropy. Front. Physiol. 8:282. doi: 10.3389/fphys.2017.00282
Seeley, W. W., Menon, V., Schatzberg, A. F., Keller, J., Gary, H., Kenna, H., et al. (2007). Dissociable intrinsic connectivity networks for salience processing and executive control. J. Neurosci. 27, 2349–2356. doi: 10.1523/JNEUROSCI.5587-06.2007.Dissociable
Sirois, S., and Brisson, J. (2014). Pupillometry. Wiley Interdiscip. Rev. Cogn. 5, 115–121. doi: 10.1002/wcs.1323
Smallwood, J., Brown, K. S., Tipper, C., Giesbrecht, B., Franklin, M. S., Mrazek, M. D., et al. (2011). Pupillometric evidence for the decoupling of attention from perceptual input during offline thought. PLoS One 6:e18298. doi: 10.1371/journal.pone.0018298
Stanners, R. F., Coulter, M., Sweet, A. W., and Murphy, P. (1979). The pupillary response as an indicator of arousal and cognition. Motiv. Emot. 3, 319–340. doi: 10.1007/BF00994048
Steinhauer, S. R., Siegle, G. J., Condray, R., and Pless, M. (2004). Sympathetic and parasympathetic innervation of pupillary dilation during sustained processing. Int. J. Psychophysiol. 52, 77–86. doi: 10.1016/j.ijpsycho.2003.12.005
Steyer, R., Schwenkmezger, P., Notz, P., and Eid, M. (1994). Theoretical analysis of a multidimensional mood questionnaire (MDBF). Diagnostica 40, 320–328.
TaskForce, (1996). Heart rate variability: standards of measurement, physiological interpretation, and clinical use. Task Force of the European Society of Cardiology and the North American Society of Pacing and Electrophysiology. Eur. Heart J. 17, 354–381.
Turnbull, P. R. K., Irani, N., Lim, N., and Phillips, J. R. (2017). Origins of pupillary hippus in the autonomic nervous system. Invest. Ophthalmol. Vis. Sci. 58, 197–203. doi: 10.1167/iovs.16-20785
Urai, A. E., Braun, A., and Donner, T. H. (2017). Pupil-linked arousal is driven by decision uncertainty and alters serial choice bias. Nat. Commun. 8:14637. doi: 10.1038/ncomms14637
Usui, S., and Stark, L. (1982). A model for nonlinear stochastic behavior of the pupil. Biol. Cybern. 45, 13–21. doi: 10.1007/BF00387209
Villalobos-Castaldi, F. M., Ruiz-Pinales, J., Valverde, N. C. K., and Flores, M. (2016). Time-frequency analysis of spontaneous pupillary oscillation signals using the Hilbert-Huang transform. Biomed. Signal Process. Control 30, 106–116. doi: 10.1016/j.bspc.2016.06.002
Võ, M. L. H., Jacobs, A. M., Kuchinke, L., Hofmann, M., Conrad, M., Schacht, A., et al. (2008). The coupling of emotion and cognition in the eye: introducing the pupil old/new effect. Psychophysiology 45, 130–140. doi: 10.1111/j.1469-8986.2007.00606.x
Wessel, N., Voss, A., Malberg, H., Ziehmann, C., Voss, H. U., Schirdewan, A., et al. (2000). Nonlinear analysis of complex phenomena in cardiological data. Herzschrittmacherther. Elektrophysiol. 11, 159–173. doi: 10.1007/s003990070035
Wilhelm, H., Lüdtke, H., and Wilhelm, B. (1998). Pupillographic sleepiness testing in hypersomniacs and normals. Graefes Arch. Clin. Exp. Ophthalmol. 236, 725–729. doi: 10.1007/s004170050149
Yamamoto, K., Arai, H., and Nakayama, S. (1990). Skin conductance response after 6-hydroxydopamine lesion of central noradrenaline system in cats. Biol. Psychiatry 28, 151–160. doi: 10.1016/0006-3223(90)90632-C
Yoshida, H., Yana, K., Okuyama, F., and Tokoro, T. (1994). Time-varying properties of respiratory fluctuations in pupil diameter of human eyes. Methods Inform. Med. 33, 46–48.
Keywords: pupillometry, heart rate variability, blood pressure, skin conductance, respiration
Citation: Schumann A, Kietzer S, Ebel J and Bär KJ (2020) Sympathetic and Parasympathetic Modulation of Pupillary Unrest. Front. Neurosci. 14:178. doi: 10.3389/fnins.2020.00178
Received: 09 July 2019; Accepted: 17 February 2020;
Published: 11 March 2020.
Edited by:
Alberto Porta, University of Milan, ItalyReviewed by:
Karsten Heusser, German Aerospace Center (DLR), GermanyCopyright © 2020 Schumann, Kietzer, Ebel and Bär. This is an open-access article distributed under the terms of the Creative Commons Attribution License (CC BY). The use, distribution or reproduction in other forums is permitted, provided the original author(s) and the copyright owner(s) are credited and that the original publication in this journal is cited, in accordance with accepted academic practice. No use, distribution or reproduction is permitted which does not comply with these terms.
*Correspondence: Karl Jürgen Bär, S2FybC1KdWVyZ2VuLkJhZXJAbWVkLnVuaS1qZW5hLmRl
Disclaimer: All claims expressed in this article are solely those of the authors and do not necessarily represent those of their affiliated organizations, or those of the publisher, the editors and the reviewers. Any product that may be evaluated in this article or claim that may be made by its manufacturer is not guaranteed or endorsed by the publisher.
Research integrity at Frontiers
Learn more about the work of our research integrity team to safeguard the quality of each article we publish.