- 1Kansei Fukushi Research Institute, Tohoku Fukushi University, Sendai, Japan
- 2Neuroscience Research Institute, Gachon University, Incheon, South Korea
Many human characteristics must be evaluated to comprehensively understand an individual, and measurements of the corresponding cognition/behavior are required. Brain imaging by functional MRI (fMRI) has been widely used to examine brain function related to human cognition/behavior. However, few aspects of cognition/behavior of individuals or experimental groups can be examined through task-based fMRI. Recently, resting state fMRI (rs-fMRI) signals have been shown to represent functional infrastructure in the brain that is highly involved in processing information related to cognition/behavior. Using rs-fMRI may allow diverse information about the brain through a single MRI scan to be obtained, as rs-fMRI does not require stimulus tasks. In this study, we attempted to identify a set of functional networks representing cognition/behavior that are related to a wide variety of human characteristics and to evaluate these characteristics using rs-fMRI data. If possible, these findings would support the potential of rs-fMRI to provide diverse information about the brain. We used resting-state fMRI and a set of 130 psychometric parameters that cover most human characteristics, including those related to intelligence and emotional quotients and social ability/skill. We identified 163 brain regions by VBM analysis using regression analysis with 130 psychometric parameters. Next, using a 163 × 163 correlation matrix, we identified functional networks related to 111 of the 130 psychometric parameters. Finally, we made an 8-class support vector machine classifiers corresponding to these 111 functional networks. Our results demonstrate that rs-fMRI signals contain intrinsic information about brain function related to cognition/behaviors and that this set of 111 networks/classifiers can be used to comprehensively evaluate human characteristics.
Introduction
Humans exhibit diverse characteristics of emotion, cognition, and behavior that describe individuals. Many different psychometric parameters from questionnaires or behavioral tasks have been developed to evaluate human characteristics of cognition/behavior (Diener et al., 1985; Raine, 1991; Carver and White, 1994; Yamauchi et al., 2009). Brain function underlies cognition/behaviors, and thus, it is possible that the characteristics of an individual can be evaluated by measuring brain function.
Functional MRI (fMRI) is the most widely used non-invasive method of measuring human brain function (Ogawa et al., 1992; Kim and Ugurbil, 1997). Measurements of human cognition/behavior by fMRI require psychometric parameters describing cognition/behavior that are embodied as tasks to induce neuronal processing in the brain (brain activation). Some psychometric parameters are easy to formalize, whereas others are not (Rupp and Zumbo, 2006). For the former type of parameters, a task can be established to evoke brain activation, and the corresponding fMRI signals of brain activation can be detected from the relevant brain areas.
Brain imaging by task-based fMRI (tb-fMRI) has provided information about the brain areas and brain networks that represent given tasks (Poldrack et al., 2013). However, most brain imaging studies have focused on specific psychometric parameters (Gauthier et al., 2000; Grill-Spector et al., 2004; Fernández-Alcántara et al., 2016; Krendl and Kensinger, 2016; Kogler et al., 2017) rather than considering a comprehensive set of psychometric parameters that can describe the diverse human characteristics of cognition/behavior. This is because it is very difficult to identify brain areas/networks responsible for the many diverse human characteristics due to limitations in task designs and time to perform tests.
In contrast to tb-fMRI, resting-state fMRI (rs-fMRI) signals come from intrinsic brain activities not designated to explicit tasks (Fransson, 2005; Fox and Raichle, 2007), and thus, there is no information to link a measured rs-fMRI signal with a specific brain function. However, functional networks produced from the correlation of rs-fMRI signals with certain brain areas, such as the default mode network, are known to represent various aspects of brain function (Greicius et al., 2004; Mason et al., 2007). Recent studies have further shown that task performance can be estimated by brain networks identified by rs-fMRI (Tavor et al., 2016; Craig et al., 2017; Song et al., 2017). In addition, some studies have proven that brain areas or functional connectivity in a rs-fMRI network are correlated with the scores of an explicit task used to identify functional areas by tb-fMRI (Finn et al., 2015; Meskaldji et al., 2016). These previous studies suggested that rs-fMRI signals can be used to identify brain networks that represent sensory and higher-order cognitive function or higher-order social function (Finn et al., 2015; Lei et al., 2017; Yang et al., 2017). That is, rs-fMRI signals may represent functional infrastructures for processing information in the brain that is highly involved in the brain function related to cognition/behavior. These characteristics of rs-fMRI may allow diverse information about the brain to be obtained from rs-fMRI signals acquired during a single MRI scan if appropriate supporting information is provided that can explain the characteristics of rs-fMRI signals or the correlation between brain areas based on the signals.
Using behavioral data about psychometric parameters as the supporting information, we attempted to test whether functional networks of the brain could be identified by rs-fMRI signals and psychological parameters (Figure 1). Recently some studies have shown that it is possible to decode brain states from fMRI responses using machine learning algorithms, such as a support vector machine (Guo et al., 2015; Altmann et al., 2016; Hu et al., 2017; Zafar et al., 2017). To verify that the functional networks identified represent the corresponding psychological parameters, and also to evaluate human characteristics from the rs-fMRI signals, we used a support vector machine (SVM).
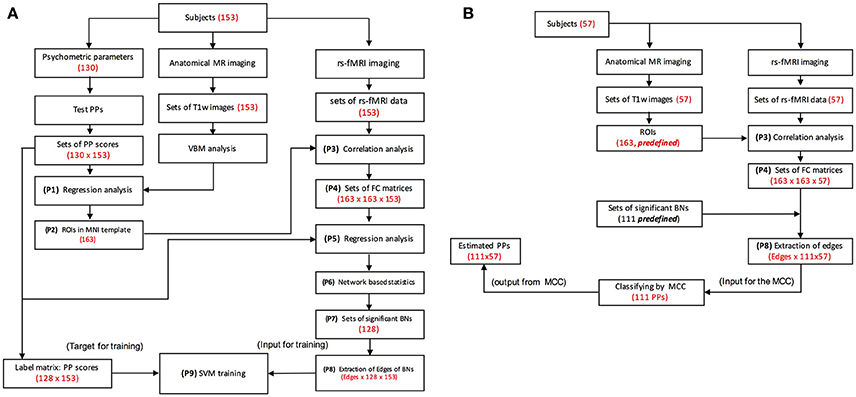
Figure 1. Procedure for MRI data processing. P1, P2, etc., stand for processing steps. (A) Diagrams of data flow for the primary experiment. Brain areas are identified by psychometric parameters and MRI data by regression analysis (P1, P2). fMRI signals are extracted from the ROIs, and the correlation matrix is constructed (P3, P4). Regression analysis and network based statistics (NBS) analysis were performed for the correlation matrices of 153 subjects (134 for IQ and 123 for EQ), and significant brain networks are identified (P5, P6, P8). Inputs to the SVM classifiers are determined from the edges of the identified functional networks (P7, P8). Scores of psychometric parameters are estimated by classifiers and compared with psychologically measured scores to calculate accuracy. Numbers and letters in the parenthesis stand for specific information obtained after the processing at each step. PP, psychometric parameter; SVM, support vector machine; rs-Fmri, resting-state functional MRI; FC, functional connectivity; BN, brain network; MCC, multiple class classifiers; T1w, T1 weighted. (B) Diagrams of data flow for the supplementary experiment. Bypassing the regression analyses used during training, fMRI signals are extracted from the ROIs, and the edges of functional networks are calculated (P3, P4, P8) for input into the SVM classifiers (P9). Scores of psychometric parameters are estimated by the classifiers.
We attempted to derive a specific SVM classifier related to each identified functional network. Second, we aimed to identify a set of functional networks of the brain to comprehensively evaluate human characteristics (Figure 1). For this purpose, we prepared a set of 130 psychometric parameters (Table 1; Supplementary References), including those related to intelligence quotient (IQ), emotional quotient (EQ), and social ability/skill that can explain the most conceivable aspects of human cognition/behavior by which an individual can be described.
Materials and Methods
The experiments in this study comprised a primary experiment and a supplementary experiment. The primary experiment was designed to identify functional networks and to design the SVM classifiers. The supplementary experiment was performed to test the SVM classifiers developed in the primary experiment.
Measurements of Psychometric Parameters
In the primary experiment, psychometric parameters were measured on three separate days: one day for the Wechsler Adult Intelligence Scale (WAIS; a measure of IQ), one day for the Emotional Quotient Scale (EQS) (Uchiyama et al., 2001), and one day for the other psychometric parameters including those related to social ability/skill. The psychometric parameters related to IQ consisted of 11 subset scores, 5 IQ scores, and 2 sub-indices derived from the subset scores. The psychometric parameters related to EQS consisted of 21 sub-factors, 12 major factors derived from the sub-factors, and a total score. The other psychometric parameters consisted of 59 sub-factors and 19 major factors, some of which were derived from the sub-factors. The parameters are listed in Table 1. Major scores and factors appear in bold type. The total number of parameters including major parameters and sub-parameters was 130. To the best of our knowledge, the number of parameters in this study is greater than in any previous psychological study. This set of 130 parameters covers most conceivable human characteristics and is considered to provide sufficient information to complete a comprehensive evaluation of human characteristics, therefore, all 130 parameters were used for the identification of functional networks.
MRI Measurements
For both the primary experiment and the supplementary experiment, each subject underwent an MRI using a 3-Tesla MRI scanner (Skyra-fit; Siemens Co., Erlangen, Germany). All subjects were scanned in two sessions corresponding to a structural image (T1) measurement for VBM and a functional image measurement (resting-state fMRI). Structural images were acquired using the following parameters: repetition time = 1,900 ms, echo time = 2.52 ms, matrix size = 256 × 256, in-plane resolution = 1 × 1 mm2, slice thickness = 1 mm, and number of slices = 192. The imaging orientation was sagittal. Resting-state fMRI data were acquired using the following parameters: repetition time = 1,000 ms, echo time = 24 ms, matrix size = 64 × 64, in-plane resolution = 3.4 × 3.4 mm2, slice thickness = 3.4 mm, and number of volumes = 480. In the resting-state fMRI session, subjects were asked to lie on the bed and not to wander their mind with their eyes open and to gently focus their eyes on the center of the visual field. The lights in the room were turned off during all MRI scans.
Participants
Primary Experiment
A group of 162 participants consisting of university freshmen and sophomores [55 males, 107 females; mean age and standard deviation (SD) 20.10 ± 0.86 years; 26 left hand, 136 right hand] participated in both psychometric measurements and underwent MRI as part of the primary experiment. The aim of the primary experiment was to identify functional networks and to derive the classifiers.
Supplementary Experiment
In the supplementary experiment to test the networks and classifiers obtained in the primary experiment, a group of 57 participants (10 males, 47 females; mean age and SD 21.97 ± 1.47 years) participated in the psychometric measurements (parameters related to social ability/skill; refer to Measurements of Psychometric Parameters) and MRI measurements.
None of the participants had a history of neurological disease or any medical condition (i.e., pregnancy, use of a cardiac pacemaker, or claustrophobia). After the participants had been provided a complete description of the study, written informed consent was obtained in accordance with the Declaration of Helsinki. This study was approved by the Institutional Review Board Tohoku Fukushi University (Japan), and all experiments were performed with the relevant guidelines and regulations of the Institutional Review Board, which approved all the experimental protocols.
MRI Data Analysis: Primary Experiment
The analysis of the primary experiment was performed through several processing steps for 153 participants after the removal of participants with motion artifacts (n = 9) during MRI acquisition (Figure 1A).
VBM Analysis
For identification of functional networks anatomical brain templates such as automated anatomical labeling (AAL) atlas (Yahata et al., 2016) have been used. To avoid partial volume effect and improve sensitivity, in this study, we tried to identify brain areas specific to psychometric parameters in each of gyri in AAL atlas. MRI data were analyzed using VBM8 (http://dbm.neuro.uni-jena.de/vbm/) implemented in the Statistical Parametric Mapping software (SPM 12; Wellcome Department of Imaging Neuroscience, London, United Kingdom), which was implemented via Matlab 2015a (MathWorks, Sherborn, MA, USA). The pre-processing by VBM8, with the default parameters used in each step, included segmentation with a bias-corrected option and spatial normalization with diffeomorphic anatomical registration using exponentiated Lie algebra (DARTEL) (Ashburner, 2007), normalization to the Montreal Neurological Institute and Hospital (MNI) coordinate system with smoothing with an 8-mm full-width at half-maximum (FWHM) filter, and modulation by Jacobian determinants, which provided segmented, normalized, and modulated gray matter images. After preprocessing, a statistical test (simple regression analysis) was used to identify brain areas correlated with each psychometric parameter (P1 and P2 of Figure 1A). In the regression analysis, total brain value (TBV) was used as a covariate. Our purpose was to find specific brain areas in AAL gyri we performed a small volume correction in each gyrus based on the AAL atlas to correct for multiple comparisons (Gur et al., 2000; Singh et al., 2014). The statistical threshold was set at an uncorrected p = 0.001 and at p = 0.05 for family-wise error (FWE) correction at the voxel level.
rs-fMRI Analysis
For functional MRI data, we used the data processing assistant for resting-state fMRI software (DPABI) (Chao-Gan and Yu-Feng, 2010; Yan et al., 2016) to pre-process the data of all 153 subjects. The pre-processing included slice-scan time correction, 3D motion correction (maximum head motion: 151 subjects passed the maximum threshold of 1.5 mm and 1.5°, and other two subjects passed the maximum threshold of 3.0 mm and 3°), removal of head motion effects by the Friston 24-parameter model, bandpass temporal filtering (between 0.01 and 0.1 Hz), and artifact rejection based on the CSF signal. These functional images were smoothed with a 6-mm FWHM filter and co-registered with each corresponding structural image. All resting-state functional images were analyzed by ROI-based correlation analysis for the predefined 163 ROIs identified by VBM (P3 of Figure 1A). In the analysis, for the time courses of the 163 ROIs, correlations were calculated, and correlation coefficient matrices (163 × 163 × 153) were created (P4 of Figure 1A). A simple regression of the correlation coefficient matrices was performed with psychologically measured scores of the psychometric parameters, and then network-based statistics (NBS) were performed to identify sets of significant brain networks (i.e., a brain network for each psychometric parameter). Zalesky et al. (2010) (P6 of Figure 1A) to identify sets of significant brain networks (mostly a brain networks for each psychometric parameter; P7 of Figure 1A). In this step, randomly shuffled correlation matrices were generated for a permutation test. Then, correlational analyses were performed on the true correlation matrix with psychometric parameters, and a non-parametric permutation test was performed on random correlation matrices with 5,000 iterations. Correction for multiple comparisons based on NBS statistics was performed at an initial threshold of p < 0.005, and the number of edges in the network was limited to as close to 15 as possible. Previous research has shown that a small number of brain connections can predict a psychiatric disorder (Yahata et al., 2016).
SVM Analysis
Classifier Design
SVMs are typically used to identify a hyperplane that distinguishes between 2 groups. But in this study we attempted to design 8-class SVM classifiers. Behavioral scores are measured on a scale of 1–100. Our purpose is ideally to measure psychometric parameters by MRI at the same scale. However, there are several limitations such as sensitivity and number of samples. So, we reduced it to a scale of 1–10, 10 levels. But in classification study such as the neural network, the number of levels should be a power of 2. Therefore we decided to use 8 (i.e., 23) classes. The 8-class SVM classifier can estimate the score of a psychometric parameter from rs-fMRI data into 8 levels. Although psychometric parameter scores are linear and some regression analyses such as support vector regression can be applied, we attempted to design the SVM classifiers because a discretization is generally stable to variations and some variations would exist in fMRI and psychometric measurements.
Multiple class classification with the one-against-all (OAA) method (Bishop, 2007) was performed using LIBSVM (Chang and Lin, 2011), which was implemented in Matlab 2015a (MathWorks, Sherborn, MA, USA). The OAA method involved two steps: training a single classifier per class with one positive sample of each class and all other negative samples of the remaining classes and repeating the procedure for the total number of classes. The classifier with the highest classification score was selected as a final multi-class classifier.
Data Preparation for Training—Classification
Data sets for the SVM classifier design were prepared from the results of the brain network analysis and the psychologically measured data. The input dataset consisted of data matrices of features (P8 of Figure 1A) and labels (P9 of Figure 1A). A feature data matrix consisted of edges of a network (correlation coefficients of networks at P8 of Figure 1A) and a label matrix consisted of psychologically measured data (P9 of Figure 1A). For classification label data (the label matrix), the scores of psychologically measured psychometric parameters were normalized and divided into eight classes by the equation {(x-min)/(max-min)} * 8, where × is the score of the psychologically measured psychometric parameter. In the calculation results, the number after the decimal point was rounded up. The dimensions of a feature matrix were the number of edges by the number of subjects, and the number of feature matrices was the number of functional networks. Therefore, the dimensions of the input data set for the SVM were the number of edges by the number of functional networks by the number of subjects (the number of edges were omitted in Figure 1B for simplification). The dimensions of the label matrices were the number of functional networks (scores of psychologically measured psychometric parameters rated into one of eight classes) by the number of subjects.
Training of Classifiers
With the data sets of all psychometric parameters, a leave-one-out cross validation was run using a radial basis function (RBF) kernel defined as K (x, y) = exp (−γ ∥ x − y ∥ 2) for optimizing the C and gamma (γ) values. In parameter optimization, the grid-search method was performed with various ranges of C and γ values (C = 2−5, 2−4, …, 215, γ = 2−15, 2−14, …, 215). After cross validation, the multiple class classifiers of the psychometric parameters with the best C and γ values were varied and deemed significant (P7 of Figure 1A).
Procedure for MRI Data: Supplementary Experiment
Test of Classifiers
In the supplementary experiment, the analyses were performed on 57 participants to test the classifiers. MRI data were preprocessed via the same procedures as in the primary experiment but bypassing the regression analyses in the training procedure. fMRI signals were extracted from the ROIs, the edges of the functional networks were calculated (P3, P4, P8), and inputs for the SVM classifiers were determined from the edges (P8). Scores of psychometric parameters were estimated by the trained classifiers (Figure 1B). The estimated scores were compared with the psychologically measured scores to calculate the accuracy.
Summary of the Data Processing Procedures
Training Procedure Figure 1A
Brain areas were identified by the psychometric parameters and the MRI data via a regressing analysis (P1, P2). From the ROIs, fMRI signals were extracted, and raw correlation matrices were constructed (P3, P4). A regression was performed and then a network-based statistics (NBS) analysis was performed, to identify significant brain networks (P5, P6, P7). The inputs for the SVM classifiers were from edges of the identified functional networks (P8). Scores for the psychometric parameters were estimated using a scale from 1 to 8 by the SVM classifiers and compared with psychologically measured scores, which were also rescaled to 8 levels, to calculate accuracy.
Test Procedure Figure 1B
Bypassing the regression analyses in the training, fMRI signals were extracted from the ROIs, and then the edges of functional networks were calculated (P3, P4, P7, P8). The extracted edges were used for the inputs for the SVM classifiers and the scores of psychometric parameters were estimated by the classifiers.
Results
Psychological Data
Scores for the psychometric parameters were acquired for all 153 participants (153 for social ability, 134 for IQ, and 123 for EQ; as data were acquired over several days, IQ and EQ data could not be acquired for some participants) included in the primary experiment (see the Materials and Methods section) and are listed in the order of the MRI measurements performed. We used all 130 parameters for subsequent MRI processing to map each parameter to the brain, such that each functional network to be identified would have sufficient information to evaluate the psychometric parameters. The behavioral scores for all 130 parameters are listed in Table 2 for the participants in the primary experiment.
Voxel-Based Morphometry
Brain areas that were significantly correlated with each psychometric parameter, reflecting a specific function of cognition/behavior related to the parameter, were identified by correlation analysis of the VBM data with the score of each psychometric parameter (P1 of Figure 1A). Brain areas for 96 parameters among 130 total psychometric parameters were identified by the analysis (p = 0.05, corrected; see section Materials and Methods for more details), which corresponded to a total of 187 brain areas, as some parameters were correlated with multiple brain areas. An ROI was defined as a 5-mm-diameter sphere around the voxel corresponding to the peak t-value in each of the 187 brain areas. Among the 187 ROIs, some ROIs overlapped with others. Therefore, the overlapping ROIs, except the first overlapping ROI in the parameter list, were removed, leaving a total of 163 remaining ROIs (P2 of Figure 1A). The locations of the ROIs in the brain are shown in Figure 2, and their coordinates are given in Table 3. The ROIs were distributed over the frontal, parietal, temporal, occipital, and cerebellar cortices and sub-cortices of the brain.
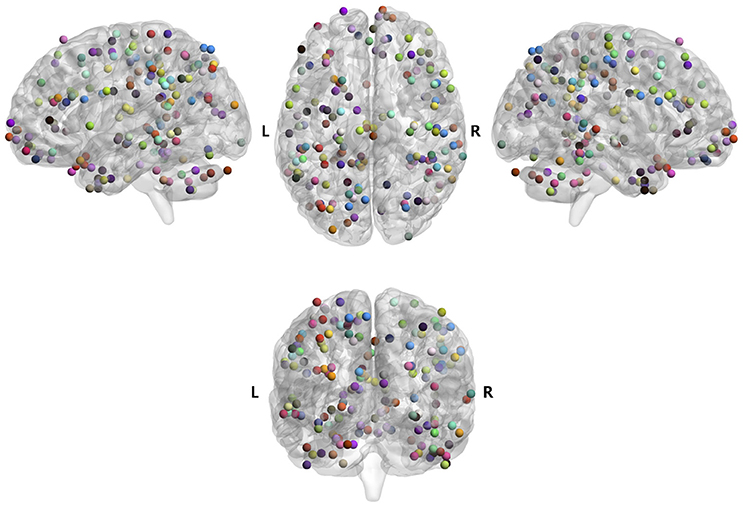
Figure 2. Brain maps of 163 regions of interest (ROIs) for 96 psychometric parameters. Those ROIs were identified by the regression analysis (P1 and P2 of Figure 1A). The psychometric parameters corresponding to the ROIs are shown in Table S1.

Table 3. Description of 163 regions of interest and the coordinates of the regions in MNI coordinates.
rs-fMRI
Functional networks were identified from the rs-fMRI time series. The rs-fMRI time series from the 163 ROIs were used to construct correlation matrices by correlation analysis (P3 of Figure 1A). After constructing of correlation matrices between ROIs (P4 of Figure 1A), a regression analysis was performed between the set of correlation matrices and the scores for the psychometric parameters to estimate statistically significant networks. Next, network-based statistics (NBS) were applied for network-based correction (P6 of Figure 1A). Networks were identified for a fixed significance level of p = 0.001 (corrected), which had different numbers of edges ranging from a few edges to more than 50. To obtain a similar number of edges for every identified network, two thresholds were used: one was at a significance level of p = 0.005 (corrected; see the Materials and Methods section for more details) and the other was the number of edges, namely, 15 per network (Termenon et al., 2016). Therefore, the number of edges in the identified networks were limited to as close to 15 as possible, except for those that had fewer than 15 edges even at the threshold of p = 0.005. For 116 of the 130 psychometric parameters, 128 significant functional networks were identified, of which 82 corresponded to social ability/skill parameters, 17 to IQ parameters and 29 to emotion quotient scale (EQS) parameters (P7 of Figure 1A). The functional networks corresponded to 116 psychometric parameters (74 for social ability/skill parameters, 15 for IQ parameters and 27 for EQS parameters), as 12 psychometric parameters corresponded to more than one functional network (8 for social ability/skill parameters, 2 for IQ parameters, and 2 for EQS parameters). The average number of edges of the 128 networks was 15.01 (mean) ± 4.72 (standard deviation). The set of all functional networks is shown in Figure S1 and Table S1. Each network identified through these processes can be considered to be involved in a brain function responsible for the corresponding psychometric parameter. Ultimately, we identified 128 functional networks representing 116 psychometric parameters, from which we can evaluate a wide variety of human characteristics that the 116 psychometric parameters reflect.
As an example network, we show the network corresponding to the psychometric parameter “verbal intelligence quotient (VIQ)” (Figure 3) (Barona et al., 1984), as IQs are important in understanding human behavior and reflect diverse brain neurological differences (Deary et al., 2010). Among IQs, the VIQ reflects language ability, which is tightly associated with social function and is a basis of diverse human activities.
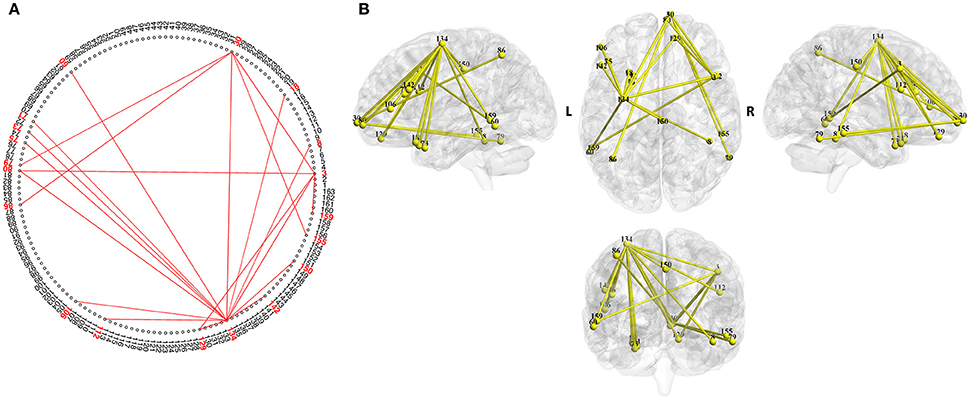
Figure 3. Brain map of the functional network for the psychometric parameter “verbal intelligence quotient (VIQ).” The width represents the strength of the correlation. The network was identified by NBS analysis (P5 of Figure 1A). This network reflects brain function related to “VIQ” and can be used to estimate VIQ. Similar assessments are possible for the other functional networks, and thus, comprehensive human characteristics can be evaluated. (A) Two-dimensional view of the network. (B) Three-dimensional view of the network.
The ROIs constituting the functional network are shown in Table 4. The ROIs included in the brain areas of Brodmann area (BA) 47 and BA 22 are known to be associated with language-related function (Shaywitz et al., 1998).
SVM
To verify that the functional networks identified from the rs-fMRI signals and psychometric parameters can significantly represent cognition/behavior, we derived a multiclass SVM classifier for each functional network. A binary classifier was considered to be sufficient for verification, but we attempted to derive multiclass SVM classifiers because our future aim is to estimate scores of psychometric parameters using only rs-fMRI data. To determine if this was possible, an eight-class SVM classifier was chosen, although a multiclass SVM with more classes would have been ideal for estimating the scores. A multiclass (eight-class) SVM classifier for each psychometric parameter could be derived by using the edges of each network as the input (P8 of Figure 1A). All the classifiers were revealed to have significant accuracy upon cross-validation (one-sample t-test, p = 0.05) above the chance level, although the accuracy is low, except for five classifiers corresponding to five psychometric parameters (P9). That is, significant classifiers were derived for 123 of the 128 functional networks (78 for social ability/skill parameters, 17 for IQ parameters and 28 for EQS parameters). These 123 SVM classifiers were related to 111 psychometric parameters (70 for social ability/skill parameters, 15 for IQ parameters, and 26 for EQS parameters), as 12 psychometric parameters corresponded to more than one classifier (Figure S2, Table S1).
Testing the SVM Classifiers in Different Populations
To test the performances of the SVM classifiers, we estimated the scores of the psychometric parameters from level 1 to 8 (related to social ability/skill) from MRI data using the 70 SVM classifiers (only one classifier was chosen for each psychometric parameter that corresponded to multiple classifiers) that corresponded to the 70 psychometric parameters related to social ability/skill. We compared the estimated scores with psychologically measured scores of the 70 psychometric parameters for 57 participants in the supplementary experiment. All 70 SVM classifiers exhibited an accuracy above chance level with low sensitivity of 14.1 ± 3.7% but high specificity of 87.8 ± 0.8% (Table 5), and the accuracy results found for this supplementary experiment were similar to those observed in the primary experiment (Figure 4).
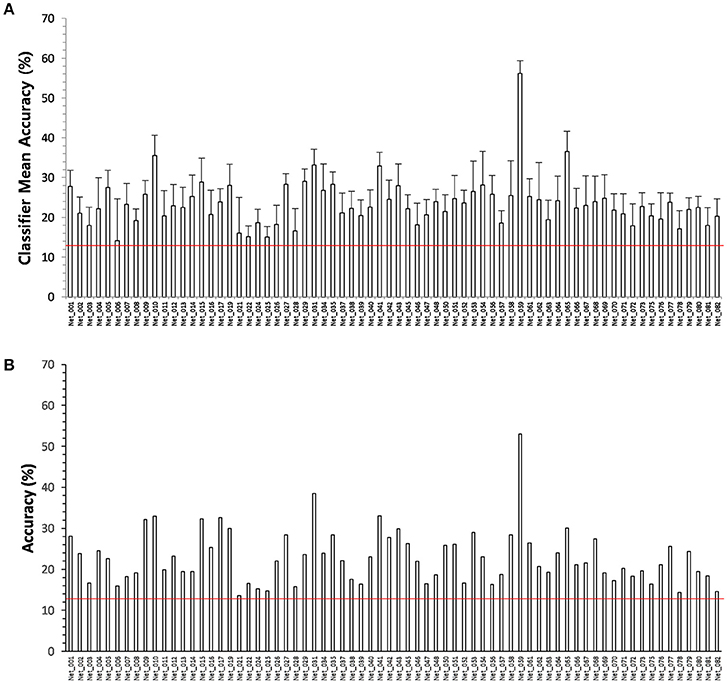
Figure 4. (A) Accuracies of 70 SVM classifiers corresponding to the psychometric parameters related to social ability/skill in 153 participants. The error bar denotes the standard deviation. All classifiers are significant at p = 0.05. (B) Accuracies of same 70 SVM classifiers in another 57 participants. The accuracy results of test are similar to those observed during the training. This shows that the derived classifiers work properly. The redlines on the figures indicate the chance level 12.5%.
Discussion
The primary aims of this study were to prove that functional networks identified by rs-fMRI signals and psychometric parameters represent brain functions to which corresponding cognition/behavior are related and to determine whether the functional networks can be interpreted in a similar way to functional areas identified by tb-fMRI signals upon task stimulation. Another aim was to identify a set of functional networks for comprehensively evaluating human characteristics.
We found functional networks corresponding to 111 out of 130 psychometric parameters and derived a multiclass SVM classifier for each psychological parameter. These findings demonstrate that each rs-fMRI functional network can represent a corresponding cognition/behavior and that the set of functional networks reported here, which correspond to 111 psychometric parameters, can be used to comprehensively evaluate human characteristics.
Previous studies have attempted to identify functional networks from rs-fMRI signals. Some have identified functional networks by correlation between rs-fMRI signals and known ROIs (Wang et al., 2009; Tian et al., 2011), and others have derived functional networks by correlation between a known seed area or image voxel of the brain and other brain areas/voxels (Greicius et al., 2003; Fox et al., 2009) or by independent component analysis (ICA) in which a group of brain areas sharing a common component of an independent signal are identified to constitute a functional network (Damoiseaux et al., 2006; De Luca et al., 2006). However, the functional roles of the networks were interpreted based on various cognition/behavior related to the tasks in tb-fMRI (van den Heuvel and Hulshoff Pol, 2010), as a combination of functional areas previously identified by tb-fMRI and the tasks performed during tb-fMRI were used in the identification procedure of the functional networks. Therefore, it remains unclear whether functional networks can be identified by psychological indices (psychometric parameters consisting of self-reported questionnaire scores) and whether rs-fMRI signals can represent the corresponding cognition/behavior. By identifying brain networks related to psychometric parameters and the deriving multiclass SVM classifiers corresponding to those psychological parameters, our results demonstrate that rs-fMRI functional networks can represent cognition/behavior. In tb-fMRI, SVM classifiers have been used to prove functional specificity of a brain area by testing whether the brain area can discriminate the related stimulus exemplar from other stimuli (MacEvoy and Epstein, 2009). Similarly, each of our identified classifiers could significantly discriminate the scores of the corresponding psychological parameter, showing that each functional network represented the corresponding cognition/behavior. The results indicate that the identified functional networks can be used in a similar way to the functional areas identified by tb-fMRI for brain imaging. Recent studies on rs-fMRI support our results that the functional brain networks identified by rs-fMRI signals contain intrinsic information of the brain system and have shown that similar brain maps obtained by tb-fMRI can also be acquired by rs-fMRI (Finn et al., 2015; Tavor et al., 2016).
The identification of 111 functional networks and the derivation of SVM classifiers for each of the 111 functional networks suggest that the variance of rs-fMRI signals between subjects reflects differences in cognition/behavior, which also indicates that variations in neural systems develop differently in each individual. Therefore, it is inferred that brain plasticity with different genetic and environmental conditions (Bouchard, 2004) varies among participants and that such variation in plasticity could appear in the functional network representation.
Several studies of brain plasticity at the systems level have been performed. Long-term plasticity has been shown in primary sensory areas, such as in visual areas of blind subjects and auditory areas of deaf subjects (Karni and Sagi, 1991; Gaser and Schlaug, 2003), which could be considered as modifications to brain “hardware.” Mid-term plasticity was also shown in high-level areas like the hippocampus among people who have extensively trained for many years, such as in taxi drivers (Maguire et al., 2000). In addition, short-term plasticity was shown in high-level areas such as the hippocampus in those undergoing intense cognitive function training, such as in those studying for a difficult medical exam for a few months or during physical juggling training for a few weeks (Draganski et al., 2006). Such plastic changes have been observed by rs-fMRI and VBM. Considering the brain plasticity observed by rs-fMRI or VBM, we can infer that the 163 functional areas and 111 functional networks identified by the same modalities used in the previous studies also reflect brain plasticity.
We performed the leave-one-out cross validation in this study because we thought it gives less biased predictions. Recently a study has suggested conservative evaluation for reliability of cross-validation methods in applying machine learning algorithms for small sample sizes (Varoquaux, 2017). In this study, we completely separated data sets for the training and the test and could acquire similar results for the training and the test. In addition, the average accuracy 23.6% of the test data is still above a corrected chance level (about 17% for 150 samples), corrected by the number of samples, which was proposed by a previous study (Combrisson and Jerbi, 2015). These support that our classifiers are reliable.
In terms of the general use of classifiers, accuracy is an important factor. The accuracy of the SVM classifiers is low, although it is above the chance level. One reason is that the number of classes - 8 - could be too high. For 3-class SVM classifiers we could get about 53% accuracy; similarly, we can expect about 80% accuracy for a binary classifier. In this study, we used the same number of classes for all psychometric parameters when designing SVM classifiers, although there was an optimal number of clusters. We used the same algorithm and kernel for all psychometric parameters. However, other classification algorithms or kernels may be better for some psychometric parameters depending on their features and data structures. In future studies, it will be necessary to optimize the classification algorithm to each parameter and to optimize the number of classes to suit the evaluation of human characteristics, especially transient changes in characteristics by brain plasticity resulting from education, training, or diseases. However, even with the 8-class SVM classifier we derived, we can significantly evaluate human characteristics. For example, in a supplementary experiment (not published), we obtained rs-fMRI data from one participant 8 times across 2 weeks. We found that 66 out of 70 SVM classifiers gave the same score (level) more than 4 times, which means that repeated measurements enable our 8-class SVM classifiers to be applied to estimate a wide variety of human characteristics with high specificity (more than 87%; Table 5).
For training, the SVM classifier that we used here only has information regarding the edges of the functional networks with which the correlations of the psychometric scores were comparatively low, 0.51 ± 0.07 (mean ± SD), as shown in Figure S2, which might be a limitation of the performance of the SVM classifiers. But other factors related to the topology of a functional network as a graph, such as centrality and mean path, can be used to improve the accuracy. Additional data from other modalities, such as fractional anisotropy or mean diffusivity from diffusion-weighted imaging, may also be useful for further improving the accuracy. In the future, we plan to use these types of data to further improve the accuracy for generalizing our classifiers.
The tb-fMRI signal is known to be induced by stimulation through neuro-vascular coupling, and the site at which an fMRI signal is measured is known to contain neurons that are processing the information related to a given stimulus. Therefore, tb-fMRI is known to be a direct method to identify functional areas. However, resting-state fMRI is considered to be indirect because additional supporting information is needed to characterize the connectivity, such as psychological parameters or behavioral information, and it remains unclear whether neurons in brain areas of a rs-fMRI functional network are directly related to the processing of the information required for the expected cognitive or behavioral brain function. Therefore, even in the case in which the data acquired by the two modalities can yield the same results in evaluating cognition/behavior, the functional mechanism represented by brain areas or networks may be different. This may explain why ROIs for a psychometric parameter were not identified but a functional network was identified. This type of problem related to intrinsic functional characteristics should be further examined to elucidate the mechanism of brain function.
To understand the details of the functional role of the functional network of each psychological parameter, tb-fMRI may be needed. However, in this study, the aim was to prove that functional networks can be identified from rs-fMRI signals and psychometric parameters and to identify biomarkers of cognition/behavior to evaluate a wide variety of human characteristics that can be used to describe individuals. Therefore, although the details of identified functional networks should be investigated further, we believe that our aim of identifying functional networks/classifiers that characterize most of the intended psychometric parameters has been achieved.
Regarding the size of the subject population for significantly obtaining brain information from rs-fMRI and psychological parameters, the population size of 153 subjects for this study is considered to be appropriate because a previous study suggested that more than 100 subjects can provide reliable variation for estimating brain plasticity (Termenon et al., 2016).
To the best of our knowledge, this study derived the largest number of functional networks/classifiers (or identified functional brain networks) reflecting cognition/behavior among fMRI studies performed to date. Although there are several large databases around the world that are represented by the “human connectome project” (www.humanconnectome.org), none of the databases include as many psychometric parameters as included in this study. This is the first study of its kind in the field of brain imaging that reveals the possibility to describe an individual based on a comprehensive set of diverse human characteristics. However, other psychometric parameters may need to be added to obtain more classifiers so that an individual can be described as completely as possible. In the present study, 19 psychological parameters were not significantly associated with a functional network, for which we may need to devise new tasks and use tb-fMRI to elucidate the corresponding functional networks. In addition, it may be possible that abilities or emotions related to the psychometric parameters are not reflected in resting state fMRI signals, which may be an interesting topic to investigate in a future study.
Taken together, our results demonstrate that; (i) rs-fMRI signals include intrinsic information of brain function related to cognition/behavior, (ii) functional networks identified by psychometric parameters can represent corresponding cognition/behavior, and (iii) the set of functional networks/classifiers identified here can be used to comprehensively evaluate human characteristics.
Conclusion
We identified a set of 128 functional networks of cognition/behavior by rs-fMRI that span a variety of human characteristics and psychometric parameters, and we derived 123 multiple-class SVM classifiers corresponding to 111 psychometric parameters. This demonstrates that we can identify functional areas or networks of the brain not only by tb-fMRI but also by rs-fMRI. It also demonstrates that we can evaluate cognition/behavior and develop biomarkers for a wide variety of human characteristics using the 111 dimensions of the data obtained from a single rs-fMRI scan. The data and classifiers may also be applied to longitudinal studies or studies evaluating educational, training, or career development programs.
Author Contributions
Y-WS, YK, and SO designed this study. The psychological data for this study were acquired by YK, CA, and YO. The anatomical and functional magnetic resonance images (MRI) data were acquired by Y-WS and DK. The VBM analysis of anatomical data was conducted by Y-WS and DK. Brain network analysis and SVM analysis of functional data was conducted by U-SC. Y-WS wrote the first draft of the manuscript with SO, YK, and U-SC contributed to revise this manuscript. All authors reviewed this manuscript.
Conflict of Interest Statement
The authors declare that the research was conducted in the absence of any commercial or financial relationships that could be construed as a potential conflict of interest.
Acknowledgments
This study was supported by the MEXT-Supported program for the Strategic Research Foundation at Private Universities, 2014–2018.
Supplementary Material
The Supplementary Material for this article can be found online at: https://www.frontiersin.org/articles/10.3389/fnins.2018.00149/full#supplementary-material
Figure S1. Set of all functional networks.
Figure S2. Correlation between each functional network and its psychometric score for 70 functional networks with 153 subject data by a linear multiple regression based on the least square analysis. The average correlation value R is 0.51 (S.D 0.07) and R2 is 0.27 (S.D 0.07), p < 0.0001.
Table S1. Description of all functional networks.
Supplementary Datasheet 1. Supplementary references.
References
Altmann, A., Schröter, M. S., Spoormaker, V. I., Kiem, S. A., Jordan, D., Ilg, R., et al. (2016). Validation of non-REM sleep stage decoding from resting state fMRI using linear support vector machines. NeuroImage 125, 544–555. doi: 10.1016/j.neuroimage.2015.09.072
Ashburner, J. (2007). A fast diffeomorphic image registration algorithm. NeuroImage 38, 95–113. doi: 10.1016/j.neuroimage.2007.07.007
Barona, A., Reynolds, C. R., and Chastain, R. (1984). A demographically based index of premorbid intelligence for the WAIS—R. J. Consult. Clin. Psychol. 52, 885–887. doi: 10.1037/0022-006X.52.5.885
Bishop, C. (2007). Pattern Recognition and Machine Learning (Information Science and Statistics), 1st Edn. 2006. Corr. 2nd Printing Edn. New York, NY: Springer.
Bouchard, T. J. (2004). Genetic influence on human psychological traits a survey. Curr. Dir. Psychol. Sci. 13, 148–151. doi: 10.1111/j.0963-7214.2004.00295.x
Carver, C. S., and White, T. L. (1994). Behavioral inhibition, behavioral activation, and affective responses to impending reward and punishment: the BIS/BAS Scales. J. Pers. Soc. Psychol. 67, 319–333. doi: 10.1037/0022-3514.67.2.319
Chang, C.-C., and Lin, C.-J. (2011). LIBSVM: a library for support vector machines. ACM Trans. Intell. Syst. Technol. 2, 27:1–27:27. doi: 10.1145/1961189.1961199
Chao-Gan, Y., and Yu-Feng, Z. (2010). DPARSF: a MATLAB toolbox for “Pipeline” data analysis of resting-state fMRI. Front. Syst. Neurosci. 4:13. doi: 10.3389/fnsys.2010.00013
Combrisson, E., and Jerbi, K. (2015). Exceeding chance level by chance: the caveat of theoretical chance levels in brain signal classification and statistical assessment of decoding accuracy. J. Neurosci. Methods 250, 126–136. doi: 10.1016/j.jneumeth.2015.01.010
Craig, M. M., Manktelow, A. E., Sahakian, B. J., Menon, D. K., and Stamatakis, E. A. (2017). Spectral diversity in default mode network connectivity reflects behavioral state. J. Cogn. Neurosci. 30, 526–539. doi: 10.1162/jocn_a_01213
Damoiseaux, J. S., Rombouts, S. A., Barkhof, F., Scheltens, P., Stam, C. J., Smith, S. M., et al. (2006). Consistent resting-state networks across healthy subjects. Proc. Natl. Acad. Sci. U.S.A. 103, 13848–13853. doi: 10.1073/pnas.0601417103
Deary, I. J., Penke, L., and Johnson, W. (2010). The neuroscience of human intelligence differences. Nat. Rev. Neurosci. 11, 201–211. doi: 10.1038/nrn2793
De Luca, M., Beckmann, C. F., De Stefano, N., Matthews, P. M., and Smith, S. M. (2006). fMRI resting state networks define distinct modes of long-distance interactions in the human brain. NeuroImage 29, 1359–1367. doi: 10.1016/j.neuroimage.2005.08.035
Diener, E., Emmons, R. A., Larsen, R. J., and Griffin, S. (1985). The satisfaction with life scale. J. Pers. Assess. 49, 71–75. doi: 10.1207/s15327752jpa4901_13
Draganski, B., Gaser, C., Kempermann, G., Kuhn, H. G., Winkler, J., Büchel, C., et al. (2006). Temporal and spatial dynamics of brain structure changes during extensive learning. J. Neurosci. Off. J. Soc. Neurosci. 26, 6314–6317. doi: 10.1523/JNEUROSCI.4628-05.2006
Fernández-Alcántara, M., Cruz-Quintana, F., Pérez-Marfil, M. N., Catena-Martínez, A., Pérez-García, M., and Turnbull, O. H. (2016). Assessment of emotional experience and emotional recognition in complicated grief. Front. Psychol. 7:126. doi: 10.3389/fpsyg.2016.00126
Finn, E. S., Shen, X., Scheinost, D., Rosenberg, M. D., Huang, J., Chun, M. M., et al. (2015). Functional connectome fingerprinting: identifying individuals using patterns of brain connectivity. Nat. Neurosci. 18, 1664–1671. doi: 10.1038/nn.4135
Fox, M. D., and Raichle, M. E. (2007). Spontaneous fluctuations in brain activity observed with functional magnetic resonance imaging. Nat. Rev. Neurosci. 8, 700–711. doi: 10.1038/nrn2201
Fox, M. D., Zhang, D., Snyder, A. Z., and Raichle, M. E. (2009). The global signal and observed anticorrelated resting state brain networks. J. Neurophysiol. 101, 3270–3283. doi: 10.1152/jn.90777.2008
Fransson, P. (2005). Spontaneous low-frequency BOLD signal fluctuations: an fMRI investigation of the resting-state default mode of brain function hypothesis. Hum. Brain Mapp. 26, 15–29. doi: 10.1002/hbm.20113
Gaser, C., and Schlaug, G. (2003). Brain structures differ between musicians and non-musicians. J. Neurosci. 23, 9240–9245.
Gauthier, I., Skudlarski, P., Gore, J. C., and Anderson, A. W. (2000). Expertise for cars and birds recruits brain areas involved in face recognition. Nat. Neurosci. 3, 191–197. doi: 10.1038/72140
Greicius, M. D., Krasnow, B., Reiss, A. L., and Menon, V. (2003). Functional connectivity in the resting brain: a network analysis of the default mode hypothesis. Proc. Natl. Acad. Sci. U.S.A. 100, 253–258. doi: 10.1073/pnas.0135058100
Greicius, M. D., Srivastava, G., Reiss, A. L., and Menon, V. (2004). Default-mode network activity distinguishes Alzheimer's disease from healthy aging: evidence from functional MRI. Proc. Natl. Acad. Sci. U.S.A. 101, 4637–4642. doi: 10.1073/pnas.0308627101
Grill-Spector, K., Knouf, N., and Kanwisher, N. (2004). The fusiform face area subserves face perception, not generic within-category identification. Nat. Neurosci. 7, 555–562. doi: 10.1038/nn1224
Guo, B., Zheng, X., Lu, Z. G., Wang, X., Yin, Z., Hou, W. S., et al. (2015). Decoding brain responses to pixelized images in the primary visual cortex: implications for visual cortical prostheses. Neural Regen. Res. 10, 1622–1627. doi: 10.4103/1673-5374.167761
Gur, R. C., Alsop, D., Glahn, D., Petty, R., Swanson, C. L., Maldjian, J. A., et al. (2000). An fMRI study of sex differences in regional activation to a verbal and a spatial task. Brain Lang. 74, 157–170. doi: 10.1006/brln.2000.2325
Hu, X., Guo, L., Han, J., and Liu, T. (2017). Decoding power-spectral profiles from FMRI brain activities during naturalistic auditory experience. Brain Imaging Behav. 11, 253–263. doi: 10.1007/s11682-016-9515-8
Karni, A., and Sagi, D. (1991). Where practice makes perfect in texture discrimination: evidence for primary visual cortex plasticity. Proc. Natl. Acad. Sci. U.S.A. 88, 4966–4970. doi: 10.1073/pnas.88.11.4966
Kim, S. G., and Ugurbil, K. (1997). Functional magnetic resonance imaging of the human brain. J. Neurosci. Methods 74, 229–243. doi: 10.1016/S0165-0270(97)02252-8
Kogler, L., Seidel, E. M., Metzler, H., Thaler, H., Boubela, R. N., Pruessner, J. C., et al. (2017). Impact of self-esteem and sex on stress reactions. Sci. Rep. 7:17210. doi: 10.1038/s41598-017-17485-w
Krendl, A. C., and Kensinger, E. A. (2016). Does older adults' cognitive function disrupt the malleability of their attitudes toward outgroup members? an fMRI investigation. PLoS ONE 11:e0152698. doi: 10.1371/journal.pone.0152698
Lei, X., Zhong, M., Liu, Y., Jin, X., Zhou, Q., Xi, C., et al. (2017). A resting-state fMRI study in borderline personality disorder combining amplitude of low frequency fluctuation, regional homogeneity and seed based functional connectivity. J. Affect. Disord. 218, 299–305. doi: 10.1016/j.jad.2017.04.067
MacEvoy, S. P., and Epstein, R. A. (2009). Decoding the representation of multiple simultaneous objects in human occipitotemporal cortex. Curr. Biol. 19, 943–947. doi: 10.1016/j.cub.2009.04.020
Maguire, E. A., Gadian, D. G., Johnsrude, I. S., Good, C. D., Ashburner, J., Frackowiak, R. S., et al. (2000). Navigation-related structural change in the hippocampi of taxi drivers. Proc. Natl. Acad. Sci. U.S.A. 97, 4398–4403. doi: 10.1073/pnas.070039597
Mason, M. F., Norton, M. I., Van Horn, J. D., Wegner, D. M., Grafton, S. T., and Macrae, C. N. (2007). Wandering minds: the default network and stimulus-independent thought. Science 315, 393–395. doi: 10.1126/science.1131295
Meskaldji, D. E., Preti, M. G., Bolton, T. A., Montandon, M. L., Rodriguez, C., Morgenthaler, S., et al. (2016). Prediction of long-term memory scores in MCI based on resting-state fMRI. NeuroImage Clin. 12, 785–795. doi: 10.1016/j.nicl.2016.10.004
Ogawa, S., Tank, D. W., Menon, R., Ellermann, J. M., Kim, S. G., Merkle, H., et al. (1992). Intrinsic signal changes accompanying sensory stimulation: functional brain mapping with magnetic resonance imaging. Proc. Natl. Acad. Sci. U.S.A. 89, 5951–5955. doi: 10.1073/pnas.89.13.5951
Poldrack, R. A., Barch, D. M., Mitchell, J. P., Wager, T. D., Wagner, A. D., Devlin, J. T., et al. (2013). Toward open sharing of task-based fMRI data: the OpenfMRI project. Front. Neuroinformatics 7:12. doi: 10.3389/fninf.2013.00012
Raine, A. (1991). The SPQ: a scale for the assessment of schizotypal personality based on DSM-III-R criteria. Schizophr. Bull. 17, 555–564. doi: 10.1093/schbul/17.4.555
Rupp, A. A., and Zumbo, B. D. (2006). Understanding parameter invariance in unidimensional IRT models. Educ. Psychol. Meas. 66, 63–84. doi: 10.1177/0013164404273942
Shaywitz, S. E., Shaywitz, B. A., Pugh, K. R., Fulbright, R. K., Constable, R. T., Mencl, W. E., et al. (1998). Functional disruption in the organization of the brain for reading in dyslexia. Proc. Natl. Acad. Sci. U.S.A. 95, 2636–2641. doi: 10.1073/pnas.95.5.2636
Singh, M. K., Chang, K. D., Kelley, R. G., Saggar, M., Reiss, A. L., and Gotlib, I. H. (2014). Early signs of anomalous neural functional connectivity in healthy offspring of parents with bipolar disorder. Bipolar Disord. 16, 678–689. doi: 10.1111/bdi.12221
Song, X., Qian, S., Liu, K., Zhou, S., Zhu, H., Zou, Q., et al. (2017). Resting-state BOLD oscillation frequency predicts vigilance task performance at both normal and high environmental temperatures. Brain Struct. Funct. 222, 4065–4077. doi: 10.1007/s00429-017-1449-4
Tavor, I., Parker Jones, O., Mars, R. B., Smith, S. M., Behrens, T. E., and Jbabdi, S. (2016). Task-free MRI predicts individual differences in brain activity during task performance. Science 352, 216–220. doi: 10.1126/science.aad8127
Termenon, M., Jaillard, A., Delon-Martin, C., and Achard, S. (2016). Reliability of graph analysis of resting state fMRI using test-retest dataset from the Human Connectome Project. NeuroImage 142, 172–187. doi: 10.1016/j.neuroimage.2016.05.062
Tian, L., Wang, J., Yan, C., and He, Y. (2011). Hemisphere- and gender-related differences in small-world brain networks: a resting-state functional MRI study. NeuroImage 54, 191–202. doi: 10.1016/j.neuroimage.2010.07.066
Uchiyama, K., Shimai, T., Utsuki, N., and Otake, K. (2001). EQS Manual. Tokyo: Jitsumukyoiku Syuppan, Practical Education Press.
van den Heuvel, M. P., and Hulshoff Pol, H. E. (2010). Exploring the brain network: a review on resting-state fMRI functional connectivity. Eur. Neuropsychopharmacol. 20, 519–534. doi: 10.1016/j.euroneuro.2010.03.008
Varoquaux, G. (2017). Cross-validation failure: small sample sizes lead to large error bars. Neuroimage. doi: 10.1016/j.neuroimage.2017.06.061. [Epub ahead of print].
Wang, J., Wang, L., Zang, Y., Yang, H., Tang, H., Gong, Q., et al. (2009). Parcellation-dependent small-world brain functional networks: a resting-state fMRI study. Hum. Brain Mapp. 30, 1511–1523. doi: 10.1002/hbm.20623
Yahata, N., Morimoto, J., Hashimoto, R., Lisi, G., Shibata, K., Kawakubo, Y., et al. (2016). A small number of abnormal brain connections predicts adult autism spectrum disorder. Nat. Commun. 7:11254. doi: 10.1038/ncomms11254
Yamauchi, T., Sudo, A., and Tanno, Y. (2009). [Reliability and validity of the Japanese version of the brief core schema scales]. Shinrigaku Kenkyu 79, 498–505. doi: 10.4992/jjpsy.79.498
Yan, C. G., Wang, X. D., Zuo, X. N., and Zang, Y. F. (2016). DPABI: data processing & analysis for (resting-state) brain imaging. Neuroinformatics 14, 339–351. doi: 10.1007/s12021-016-9299-4
Yang, X., Liu, J., Meng, Y., Xia, M., Cui, Z., Wu, X., et al. (2017). Network analysis reveals disrupted functional brain circuitry in drug-naive social anxiety disorder. Neuroimage. doi: 10.1016/j.neuroimage.2017.12.011. [Epub ahead of print].
Zafar, R., Kamel, N., Naufal, M., Malik, A. S., Dass, S. C., Ahmad, R. F., et al. (2017). Decoding of visual activity patterns from fMRI responses using multivariate pattern analyses and convolutional neural network. J. Integr. Neurosci. 16, 275–289. doi: 10.3233/JIN-170016
Keywords: resting-state fMRI, functional network, neuronal plasticity, human characteristics, psychometric parameters
Citation: Sung Y-W, Kawachi Y, Choi U-S, Kang D, Abe C, Otomo Y and Ogawa S (2018) A Set of Functional Brain Networks for the Comprehensive Evaluation of Human Characteristics. Front. Neurosci. 12:149. doi: 10.3389/fnins.2018.00149
Received: 04 September 2017; Accepted: 26 February 2018;
Published: 14 March 2018.
Edited by:
Bertrand Thirion, Institut National de Recherche en Informatique et en Automatique (INRIA), FranceReviewed by:
Kaiming Li, Sichuan University, ChinaDarya Chyzhyk, Inria Saclay - Île-de-France Research Centre, France
Copyright © 2018 Sung, Kawachi, Choi, Kang, Abe, Otomo and Ogawa. This is an open-access article distributed under the terms of the Creative Commons Attribution License (CC BY). The use, distribution or reproduction in other forums is permitted, provided the original author(s) and the copyright owner are credited and that the original publication in this journal is cited, in accordance with accepted academic practice. No use, distribution or reproduction is permitted which does not comply with these terms.
*Correspondence: Yul-Wan Sung, c3VuZ0B0ZnUtbWFpbC50ZnUuYWMuanA=; c3VuZ3N0b25lQGdtYWlsLmNvbQ==