- 1Department of Radiology, Maastricht University Medical Center, Maastricht, Netherlands
- 2School for Mental Health and Neuroscience, Maastricht University Medical Center, Maastricht, Netherlands
- 3Department of Neurology, Maastricht University Medical Center, Maastricht, Netherlands
- 4Cardiovascular Research Institute Maastricht, Maastricht University Medical Center, Maastricht, Netherlands
- 5Department of Psychiatry and Neuropsychology, Maastricht University Medical Center, Maastricht, Netherlands
- 6Department of Internal Medicine, Maastricht University Medical Center, Maastricht, Netherlands
Type 2 diabetes mellitus is associated with accelerated cognitive decline and various cerebral abnormalities visible on MRI. The exact pathophysiological mechanisms underlying cognitive decline in diabetes still remain to be elucidated. In addition to conventional images, MRI offers a versatile set of novel contrasts, including blood perfusion, neuronal function, white matter microstructure, and metabolic function. These more-advanced multiparametric MRI contrasts and the pertaining parameters are able to reveal abnormalities in type 2 diabetes, which may be related to cognitive decline. To further elucidate the nature of the link between diabetes, cognitive decline, and brain abnormalities, and changes over time thereof, biomarkers are needed which can be provided by advanced MRI techniques. This review summarizes to what extent MRI, especially advanced multiparametric techniques, can elucidate the underlying neuronal substrate that reflects the cognitive decline in type 2 diabetes.
Introduction
Type 2 diabetes mellitus is a common metabolic disorder, characterized by chronic hyperglycemia, in a context of insulin resistance and relative insulin deficiency (Gispen and Biessels, 2000). Type 2 diabetes has commonly been considered a disease of elderly populations. However, with today's unhealthy lifestyle, also an increasing number of younger (that is, middle-age) people are developing diabetes.
Type 2 diabetes has a broad range of serious clinical complications, including nephropathy, retinopathy, and cardiovascular disease, and is often accompanied by cardiovascular risk factors such as hypertension and dyslipidemia. Hyperglycemia damages a selection of cell types, including neurons, which are unable to reduce the transport of glucose inside the cell, leading to high glucose (Brownlee, 2005). Type 2 diabetes is also associated with cognitive deficits, accelerated cognitive decline, an increased risk of dementia, and Alzheimer disease (AD) (Biessels et al., 2006). In type 2 diabetes, cognitive changes mainly affect learning, memory and information processing speed (Cheng et al., 2012). For recent reviews on cognition and type 2 diabetes, the reader is referred to specific recent reviews by Koekkoek et al. (2014) and Geijselaers et al. (2015).
In recent years, numerous studies have highlighted the adverse effects of diabetes on brain physiology and cognitive function to assess contributing pathophysiological mechanisms (Biessels and Reijmer, 2014; Brundel et al., 2014a). Most studies have applied conventional MRI with multiple contrasts to detect macrostructural cerebral changes. However, macrostructural abnormalities on MRI reflect end-stage effects of impaired tissue, and conventional MRI is probably not sensitive enough to detect the earliest cerebral changes, expectedly more closely reflecting mechanisms, associated with cognitive decline (Tofts, 2003). For this purpose, potentially more-sensitive MRI techniques, such as functional MRI (fMRI) and diffusion MRI (dMRI), can be used, which could lead to a better insight into the mechanisms that precede macrostructural (end-stage) abnormalities.
The present narrative review summarizes recent literature and provides an overview of the various brain abnormalities associated with type 2 diabetes in combination with cognitive decrements. The aim is to provide the available evidence for neuronal substrates of cognitive impairment in type 2 diabetes. It will explore the appropriate MRI techniques to study associations with cognitive performance in patients with type 2 diabetes (for an overview of typical abnormalities and the corresponding techniques, see Figure 1), and will make recommendations for future research. This review is structured according to the various types of cerebral abnormalities and the appropriate MRI techniques available to study pathophysiology, in the range from routine clinical application to explorative research.
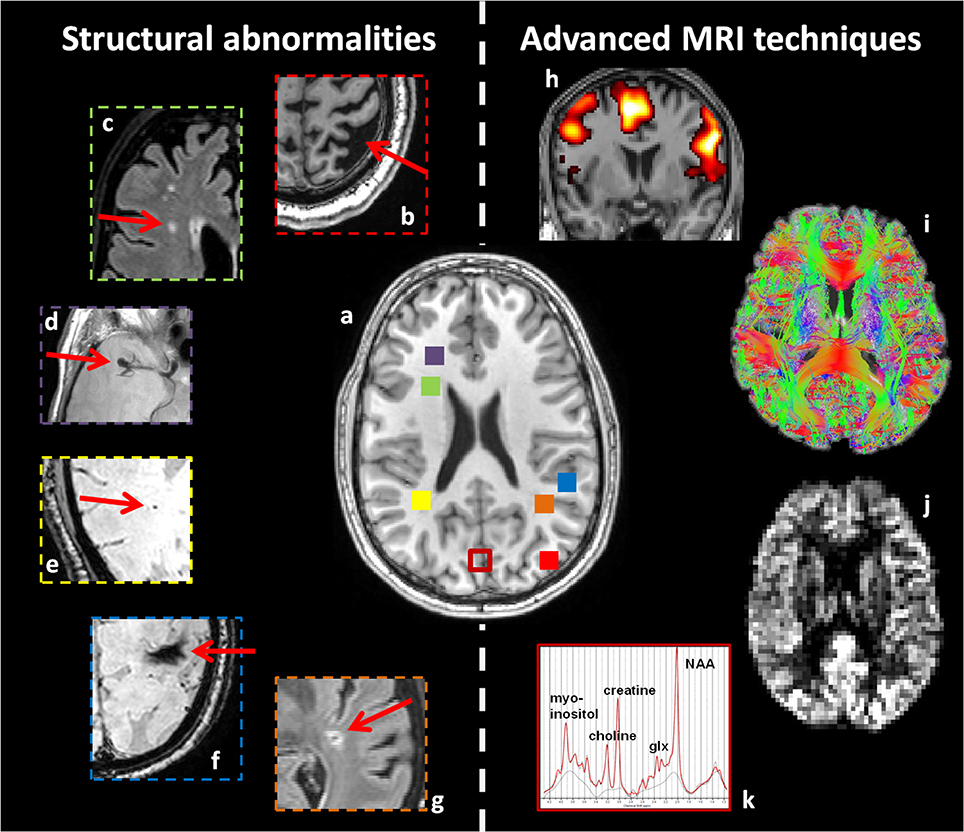
Figure 1. Overview of structural abnormalities which may be found in patients with type 2 diabetes (b–g), and advanced MRI techniques sensitive to more subtle cerebral alterations (h–k). This figure is an illustration from the authors' clinic. (a) T1-weighted image of a healthy young brain. Structural abnormalities in patients with type 2 diabetes, as highlighted with red arrows. (b) Atrophy (T1WI), (c) white matter lesions (FLAIR), (d) aneurysm (T2WI), (e) microbleeding (T2*-weighted), (f) macrobleeding (T2*-weighted), and (g) lacunar infarct (FLAIR). Advanced MRI techniques: (h) fMRI, (i) dMRI, (j) arterial spin labeling, and (k) MRS. Corresponding colored squares in (a) represent the approximate location where the structural abnormalities were found and where the single voxel for spectroscopy was located, respectively.
Atrophy
Cerebral atrophy can generally be defined as the shrinkage of brain tissue, which is a result of neurodegenerative processes, such as the loss of neurons and their interconnections (Jobst et al., 1994). Many studies on type 2 diabetes, using various structural MRI techniques, report on atrophy (den Heijer et al., 2003; de Bresser et al., 2010; van Elderen et al., 2010). Associations have been found between brain atrophy and decreased performance in various cognitive domains (Tiehuis et al., 2009; Hayashi et al., 2011; Moran et al., 2013; Zhang Y. et al., 2014), including memory, attention and executive function, as well as processing speed, motor speed, and sensory speed. Also the progression of atrophy was found related to cognitive decrements in type 2 diabetes (van Elderen et al., 2010; Reijmer et al., 2011).
Small Vessel Disease
Cerebral small vessel disease (cSVD) can be generally defined as pathological processes with various etiologies that affect the small arteries, arterioles, venules, and capillaries of the brain (Wardlaw et al., 2013). Signs of cSVD are white matter lesions, microbleeds, silent brain infarcts and lacunar abnormalities, which are also indicative for cognitive decline (Imamine et al., 2011).
White Matter Lesions
White matter lesions (WMLs) are typically observed as regions of bright, high-signal intensity in the white matter (i.e., white matter hyperintensities) depicted on T2-weighted and, especially, FLAIR images (Wardlaw et al., 2013). The underlying pathophysiology of WMLs is still poorly understood and is assumed to include multiple factors of vascular (through ischemia or arteriosclerosis) or inflammatory (through transudation of CSF) origin (Fazekas et al., 1998).
WMLs are often divided in periventricular WMLs, which are located close to the ventricles, and deep WMLs, which are located in subcortical gray matter (Wardlaw et al., 2013). It was shown that periventricular, but not subcortical, WMLs are associated with the rate of cognitive decline in elderly non-demented individuals (De Groot et al., 2002).
Numerous studies report on WMLs in patients with type 2 diabetes (Manschot et al., 2006; Jongen et al., 2007; van Harten et al., 2007; Imamine et al., 2011). More specific, deep (subcortical) WMLs, periventricular WMLs, and WMLs in general are found in patients with type 2 diabetes. WMLs are also related with impaired cognition in type 2 diabetes (Manschot et al., 2006; Jongen et al., 2007; van Harten et al., 2007; Imamine et al., 2011), especially in the domains of processing speed, memory, attention and executive functioning, and motor speed.
Microbleeds
Cerebral microbleeds result from focal leakages of small blood vessels (Wardlaw et al., 2013). They are thought to contain iron deposits (Wardlaw et al., 2013). Typically, microbleeds are found only incidentally on MRI, but are thought to play an important role in cognitive decline (Wardlaw et al., 2013). The reported prevalence of microbleeds increases with age (Imamine et al., 2011). Microbleeds do not seem to be associated with type 2 diabetic patients with cognitive impairment (Moran et al., 2013), which is also confirmed at high field (7T) (Brundel et al., 2014b).
Silent Brain Infarcts
Silent brain infarcts (SBIs) are clinically asymptomatic (i.e., they lack stroke-like symptoms), but visible (generally 2–5 mm in diameter) as focal lesions on MRI, and are associated with cognitive deficits that commonly remain unnoticed (vermeer et al., 2007).
Patients with type 2 diabetes often display SBIs, which are also related to impaired cognitive performance (Manschot et al., 2006; Imamine et al., 2011). The number of SBIs and/or progression of SBIs are especially linked to decrements in motor speed, attention and executive functioning (Imamine et al., 2011; Umegaki et al., 2011).
Lacunar Abnormalities
Lacunes are pathologically defined as small areas (3–15 mm in diameter) of infarction, which is a result from an occlusion of one of the small penetrating branches of large cerebral arteries (Wardlaw et al., 2013) and are associated with cognitive impairment (Schneider et al., 2003). In type 2 diabetes, lacunar infarcts often progress (van Harten et al., 2006; Umegaki, 2010), likely caused by ischemia (Imamine et al., 2011).
Cerebral infarcts (i.e., lacunar, cortical, subcortical infarcts, or infarcts in general) have been observed in patients with type 2 diabetes (Manschot et al., 2006; Moran et al., 2013). Most studies report a relationship between cerebral infarcts and decreased performance in various cognitive domains, including processing speed, sensory speed, memory, executive function, and global cognition.
For the detection of cerebral atrophy or cSVD, various structural MRI techniques have been used. However, these techniques cannot unravel more subtle details of tissue alterations that underlie or precede the atrophy or cSVD. For this, more-advanced MRI techniques can be used, which will be discussed below.
Impaired Cerebral Perfusion
Cerebral perfusion is defined as the amount of blood flowing through a definite volume of tissue in a given time (Filippi et al., 2010) and can be estimated using Arterial Spin Labeling (ASL) and Intravoxel Incoherent Motion (IVIM) imaging, or measured globally using a velocity-sensitive, phase-contrast MRI technique (Tiehuis et al., 2008; Brundel et al., 2012b; Novak et al., 2014; Rusinek et al., 2015; van Bussel et al., 2015; Jansen et al., 2016). The ASL technique is based on magnetic labeling of arterial blood (e.g., blood in the common carotid artery), which is used as an temporary endogenous tracer in the brain. The IVIM technique enables assessment of both the parenchyma and microvasculature and is based on the diffusion of water molecules in parenchyma and incoherent motion of water molecules in the microvasculature. The velocity-sensitive, phase-contrast MRI technique is based on differences in phase of the magnetic spins. An advantage of using ASL or IVIM is that these techniques make it possible to investigate regional differences related to disease pathology instead of only a gross measurement of total brain perfusion with phase-contrast MRI (Le Bihan et al., 1986; Ryan et al., 2014).
A fair comparison between ASL and IVIM is not trivial due to the different complex physical mechanisms that contribute to the detected signal. However, the former is a truly quantitative method, which has been validated with PET (positron emission tomography) perfusion measurements (van Golen et al., 2014), whereas the latter is yet more experimental, though it can provide a higher signal-to-noise ratio (SNR), and the possibility for an increased spatial resolution.
Thus far, some studies, one using phase contrast MRI (1.5T) (Tiehuis et al., 2008) and one using ASL (3T) (Rusinek et al., 2015), did not find any differences in global perfusion between patients with type 2 diabetes and controls, while other studies (ASL, Novak et al., 2014; Xia et al., 2015a and IVIM, van Bussel et al., 2015, all at 3T) observed regional differences in perfusion. Possibly, differences in MRI methodology could explain these conflicting results. Atrophy can be a big confounder when assessing hypoperfusion using ASL, indeed most results disappear after correction for atrophy (Jansen et al., 2016). A recent ASL study applied a new analysis approach tallying the “distributed deviating voxels,” and hypoperfusion was found in patients with type 2 diabetes, which remained significant after correction for atrophy in the subcortical gray matter (Jansen et al., 2016).
One phase contrast MRI study observed a positive association between perfusion and cognition, but this study was not able to explain the link of diabetes with cognitive performance (Tiehuis et al., 2008). Some studies did find a relationship between perfusion and impaired cognition in patients with type 2 diabetes (Brundel et al., 2012b; Xia et al., 2015a), although another study did not find this relationship (Jansen et al., 2016). Promising results regarding reduced cerebral perfusion in the insula cortex and cognitive performance were shown in a pilot ASL study (Novak et al., 2014). After insulin administration, memory and verbal fluency improved, and perfusion was elevated in the insula cortex of participants with diabetes, suggesting the involvement of an insulin mechanism. In type 2 diabetes, perfusion of the global gray matter was positively associated with verbal fluency (Rusinek et al., 2015), although local hippocampal perfusion (as measured using IVIM) had a negative association with memory performance (van Bussel et al., 2015). These results suggest the involvement of a vascular mechanism, and that the association might be dependent on the brain region.
Taken together, all perfusion techniques observed a relation with cognitive performance, which highlights the link between a vascular mechanism and cognitive decline. However, to observe regional differences in perfusion, the more-advanced MRI techniques (i.e., ASL and IVIM) appear more sensitive to contribute to the understanding of cognitive decline in patients with type 2 diabetes.
Neuronal Dysfunction
Neuronal dysfunction refers to all impairments of the neuronal system, including reduced functional activity of certain brain regions and connectivity between different regions (Zhou et al., 2010). Functional MRI (fMRI) offers the opportunity to investigate to which extent neuronal regions are active, in terms of blood oxygenation changes. The underlying principle is that neuronal activity leads to locally increased blood flow and oxygenation. Previous studies using the amplitude of low frequency fluctuations (ALFF), a measure of spontaneous neuronal activity, regional homogeneity, a measure of the neural regional synchronization, and functional connectivity, assessed by correlating time signals from distinct brain regions, reported on abnormal brain activity in patients with type 2 diabetes (Zhou et al., 2010; Musen et al., 2012; Xia et al., 2013; Cui et al., 2014).
Functional Connectivity
Reduced functional connectivity in the default mode network (DMN), i.e., the network of active brain regions when the brain is at rest and the participant is not focusing on anything particular, has been observed in patients with type 2 diabetes (Zhou et al., 2010; Musen et al., 2012; Chen et al., 2014, 2015, 2016; Hoogenboom et al., 2014; Cui et al., 2015; Xia et al., 2015b; Zhang H. et al., 2015). Moreover, reduced functional connectivity between the hippocampus and widespread regions in the DMN (Zhou et al., 2010), including the medial frontal cortex (Zhang H. et al., 2015) has been reported, in addition to reduced functional connectivity between the posterior cingulate and the medial frontal gyri and other regions in the DMN (Musen et al., 2012; Hoogenboom et al., 2014). Furthermore, reduced connectivity within the attention networks has been described (Xia et al., 2015b), which was associated with neuropsychological scores and glycated hemoglobin. Reduced connectivity of the DMN was related to impaired memory (Zhou et al., 2010; Zhang H. et al., 2015), executive function (Zhou et al., 2010), verbal fluency (Zhang H. et al., 2015), and lower global cognition (Zhang H. et al., 2015). The disrupted functional connectivity in the DMN has been shown to be inversely correlated with insulin resistance (Musen et al., 2012) in type 2 diabetes, hinting at an underlying insulin-related mechanism. This thought is enhanced by the observation of acutely increased functional connectivity between the hippocampus and multiple regions in the DMN after intranasal insulin administration (Zhang H. et al., 2015).
Interestingly, it was recently shown that participants with type 2 diabetes displayed altered fMRI network measures, characterized by a higher efficiency, compared with control participants (van Bussel et al., 2016c). Also subjects with pre-diabetes were studied, whose network measures fell between those with diabetes and control participants. The authors suggested that functional reorganization of the cerebral networks might act as a compensatory mechanism for cognitive decrements (van Bussel et al., 2016c).
Signal Fluctuations
ALFF and regional homogeneity alterations have been reported in a variety of DMN brain regions (including temporal lobe and frontal lobes) in patients with type 2 diabetes (Xia et al., 2013; Cui et al., 2014; Zhou et al., 2014). The altered ALFF and regional homogeneity values were related to impaired cognition, especially in the domains of attention and executive function (Xia et al., 2013; Cui et al., 2014; Zhou et al., 2014), speed (Xia et al., 2013; Cui et al., 2014), memory (Cui et al., 2014), and global cognition (Zhou et al., 2014). Moreover, ALFF values in the middle temporal gyrus were also inversely related to glycated hemoglobin (Xia et al., 2013) and insulin resistance in the diabetic group was negatively correlated with altered neuronal activity (Cui et al., 2014).
Brain Activation
Altered brain activation has also been found in patients with type 2 diabetes during a memory task, especially in task-related regions of the DMN (Marder et al., 2014), frontal cortex (Chen et al., 2014; Marder et al., 2014; He et al., 2015), parietal cortex (He et al., 2015) and the fronto-parietal network (Zhang Y. et al., 2016). Moreover, functional activation or connectivity is not only associated with memory performance (Zhang Y. et al., 2016), but also insulin resistance (Marder et al., 2014; Xia et al., 2015c), glycated hemoglobin (Marder et al., 2014; He et al., 2015), plasma glucose (Marder et al., 2014), and cholesterol (Xia et al., 2015d), suggesting a major role of glucose and lipid metabolism.
Overall, all functional MRI studies consistently show evidence of altered neuronal activity or functional connectivity in patients with type 2 diabetes and cognitive decrements.
White Matter Tract Abnormalities
White matter tract abnormalities refer to impaired integrity or altered organization of axonal bundles and can be investigated using diffusion MRI (dMRI). This technique is based on diffusion of water molecules, and during the dMRI acquisition, tissue is sensitized with the local characteristics of molecular diffusion. The measures most often analyzed by dMRI are fractional anisotropy (FA) and apparent diffusion coefficient (ADC). FA is a measure of tract directionality and ADC is a measure of water diffusivity. Clinically, an increase in ADC has been associated with reduced (neuronal) cell packing and increased extracellular space, possibly due to failure of neurogenesis or cell loss (Eriksson et al., 2001). Recently, analysis methods have become available that allow the assessment of the integrity and efficiency of structural networks, using graph theoretical analysis on dMRI data (Reijmer et al., 2013b).
Local Alterations
Microstructural abnormalities have been published for various brain regions in type 2 diabetes (Yau et al., 2009, 2010; Falvey et al., 2013; Zhang J. et al., 2014, 2016; van Bussel et al., 2016b; Xiong et al., 2016). Reduced FA has been observed in the white matter (Yau et al., 2010; Falvey et al., 2013) mostly concentrated in frontal and temporal regions (Yau et al., 2009), while elevated ADC values were found in a number of brain regions, including the hippocampus (Falvey et al., 2013) and multiple gray matter regions (Yau et al., 2010). Temporal lobe abnormalities were associated with impaired memory (Yau et al., 2009; van Bussel et al., 2015).
Network Alterations
Altered network and structural connectivity in type 2 diabetes have been shown using tractography (Reijmer et al., 2013a,b; Hoogenboom et al., 2014; van Bussel et al., 2016b; Yang et al., 2016; Zhang J. et al., 2016). Local and global network properties (i.e., cluster coefficient, global efficiency, path length) were altered and associated with impaired processing speed (Reijmer et al., 2013b). Elevated ADC and reduced FA were found in different tracts, including the superior longitudinal fasciculus (Reijmer et al., 2013a), uncinate fasciculus (Reijmer et al., 2013a; Hoogenboom et al., 2014), inferior longitudinal fasciculus (Reijmer et al., 2013a), corpus callosum (Reijmer et al., 2013a), and cingulum bundle (Hoogenboom et al., 2014). These tract abnormalities were associated with impaired processing speed and memory (Reijmer et al., 2013a; Hoogenboom et al., 2014) and highlight an underlying glucose-mediated mechanism as glycated hemoglobin and fasting blood glucose were also related to these tract abnormalities (Hoogenboom et al., 2014). Moreover, altered hippocampal white matter connectivity appear to be associated with memory decrements and type 2 diabetes (van Bussel et al., 2016b).
Diffusion MRI studies implicate that patients with type 2 diabetes show evidence of white matter microstructure, tract, and network abnormalities.
Metabolic Dysfunction
Proton magnetic resonance spectroscopy (1H-MRS) enables the assessment of metabolic changes through the identification and quantification of spectral peaks associated with tissue metabolites (Jansen et al., 2006). 1H-MRS is often used to investigate N-acetylaspartate (NAA), Choline (Cho), Creatine (Cr), myo-inositol (mIns), γ-aminobutyric acid (GABA), and glutamate. NAA is a measure of neuronal integrity and a surrogate marker of normal functioning neurons. Cho is an indirect marker of myelination and cell membrane metabolism. Cr is a measure of energy metabolism, and mIns has been proposed as a glial marker and as an end-product of persistent hyperglycaemia (Jansen et al., 2006). GABA and glutamate are major inhibitory and exhibitory neurotransmitters, respectively. However, in vivo detection and quantification of these neurotransmitter concentrations at low field strengths (<3T) are complicated due to spectral overlap with other metabolites. Another relevant metabolite in the context of diabetes is glucose, which typically requires high field strengths (>3T) for reliably detection with 1H-MRS (Gruetter et al., 1996). An alternative method to study brain glucose levels using MR spectroscopy is 13C-MR spectroscopy (van De Ven et al., 2012).
MR spectroscopy studies on type 2 diabetes in relationship with cognition have thus far been proven to be challenging, and often no associations between metabolic alterations and cognitive performance were found (Haroon et al., 2009; Tiehuis et al., 2010). However, a recent study found higher GABA+ levels in participants with type 2 diabetes, and higher GABA+ levels in participants with both high HbA1c levels and less cognitive performance (van Bussel et al., 2016a). The authors concluded that participants with type 2 diabetes have alterations in the GABAergic neurotransmitter system, which are related to lower cognitive functioning, which hints at the involvement of an underlying metabolic mechanism.
Interpretation
Table 1 provides an overview of all studies on type 2 diabetes, in which cognitive performance is related to diverse cerebral MRI contrasts. From this it can be appreciated that neuroradiologically visible MRI biomarkers (atrophy, WMLs, and lacunar abnormalities) and more subtle abnormalities (impaired cerebral perfusion, neuronal dysfunction, and white matter tract abnormalities) are related to cognitive decline, with a striking agreement between studies. For the other abnormalities (including microbleeds, SBIs, and metabolic dysfunction) the evidence of relationships with cognition is less convincing.
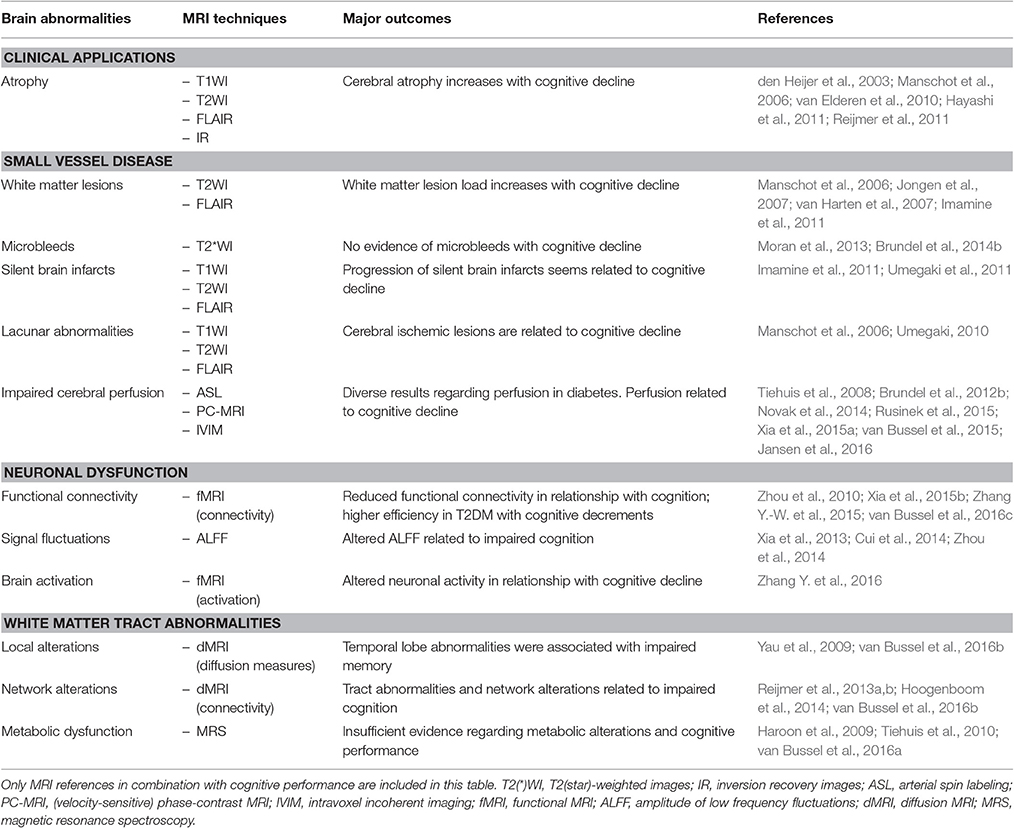
Table 1. Overview of neuroimaging abnormalities associated with cognitive performance in type 2 diabetes mellitus.
Most studies are associated with various methodological limitations. Most notably, often only a limited number of subjects is included. Furthermore, the studies show a pronounced diversity regarding subject selection, matching of subjects, diagnosis and classification of diabetes, adjustment for risk factors, and data analysis methods. Due to the different designs and limited number of available studies, it is difficult for studies reporting negative results to assess whether the applied techniques (or study methods) are not sensitive enough to pick up cognitive performance-related alterations, or whether these alterations are not present at all. Interestingly, in those studies where cerebral changes were found, these were most often located in the frontal and/or temporal lobe (den Heijer et al., 2003; Zhou et al., 2010, 2014; Musen et al., 2012; He et al., 2015; van Bussel et al., 2015), which is in close agreement with the type of cognitive decline typically experienced in type 2 diabetes (Gold et al., 2007).
Type 2 diabetes is also known to increase the risk of developing AD (Steen et al., 2005; Cheng et al., 2012). MRI studies show that gray matter loss, insulin resistance, and medial temporal lobe atrophy are associated with AD (Thompson et al., 2003; Biessels et al., 2006), traits also present in patients with type 2 diabetes (den Heijer et al., 2003). These results suggest that diabetes might to some extent be linked to Alzheimer's Disease (AD) and that diabetes and AD might share similar mechanisms underlying cognitive decline (Ryan et al., 2014).
Future Outlook
As the neuronal mechanisms underlying cognitive decline associated with type 2 diabetes still remain to be elucidated, and studies using more-advanced and potentially more-sensitive MRI techniques are scarce, intensified research is needed to investigate the underlying mechanisms of brain damage (Jouvent et al., 2010). It will also be interesting to investigate cognitive decline in pre-diabetic stages such as the metabolic syndrome or impaired glucose mechanism (Grundy, 2006; van Bussel et al., 2016c).
In addition to the imaging techniques discussed in this review, other novel MRI approaches might also yield interesting new biomarkers, such as Dynamic Contrast Enhanced MR Imaging, which is an MRI technique where T1-weighted scans are acquired dynamically after injection of a contrast agent, and pharmacokinetic modeling of the enhancing tissue signal can provide information about physiological tissue characteristics, including BBB integrity in terms of leakage of contrast medium (Taheri et al., 2011). It could be relevant to study the role of BBB in diabetes, because disruption of the BBB is also considered to be a result of cSVD.
Furthermore, metabolites that are relatively difficult to detect, such as GABA, dedicated MRS spectral editing sequences exist to identify and quantify these metabolite concentrations (Puts and Edden, 2012). The use of a specifically designed MRS acquisition scheme allows for the selective recording of signals only from the desired metabolite, while other metabolites are eliminated.
Another important direction is the application of high field MRI (Brundel et al., 2012a), as most studies in this review were performed at 1.5T. High field MRI (≥3T) has several benefits as it provides higher spatial resolution and improved SNR, although it is more susceptible for artifacts. Additionally, future studies should incorporate a multiparametric approach, to provide a more complete picture of the locations and nature of affected cerebral regions. Also, analysis approaches for fMRI and dMRI should focus on cerebral networks, as cognitive functions affected by diabetes correspond to networks, rather than localized brain regions.
Additionally, future, preferably large multicenter studies, are required to validate current findings, or provide a more definitive answer regarding issues for which currently contradictory findings have been reported in different studies (such as the inconsistencies reported regarding type 2 diabetes and global perfusion). For this, quantitative measures are essential, regarding both quantitative MRI as neuropsychological tests to characterize and define in more detail the cognitive status of the population under investigation.
Clinical Relevance
The application of neuroimaging techniques to study diabetes associated accelerated cognitive decline is relevant as we expect to obtain new insights regarding affected brain regions, networks, and tissue abnormalities. Furthermore, MRI measures might provide early biomarkers for cognitive decline (see Table 1 for an overview), and could potentially be used to identify patients at risk. Follow-up studies can be performed to confirm that subjects with sufficient cerebral MRI alterations eventually develop cognitive problems, and one could consider an interventional study with a combination of diet, exercise or medication (Zhang H. et al., 2015) to explore whether cerebral MRI alterations also delay, or even improve, after intervention (Raji et al., 2015). Hence, by performing advanced neuroimaging, a more complete picture can be obtained of the effect of diabetes on the brain, it might provide a better timing of (preventive) therapy, and it could shed some light on the course and efficacy of the therapy to prevent or halt cognitive decline.
Conclusions
Cognitive decline in type 2 diabetes is associated with brain alterations, which can be detected using neuroimaging. The battery of MRI techniques available to study this topic is highly versatile, and several aspects of brain function and integrity can be studied noninvasively. Advanced, novel MRI techniques are expected to reveal more subtle brain alterations compared with only structural MRI. Therefore, more-advanced multiparametric MRI techniques should be implemented in future studies to investigate the role of diabetes on cognitive performance, and the underlying pathophysiological mechanisms.
Literature Search
We searched PubMed for articles published until September 19, 2016, with the following terms and combinations of these terms: “arterial disease,” “arterial spin labeling,” “atrophy,” “axon damage,” “brain,” “cerebral,” “cogniti*,” “connectivity,” “diabet*,” “diffusion tensor imaging,” “DTI,” “fMRI,” “functional MRI,” “imaging,” “lacun*,” “lacunar infarct,” “microbleeds,” “microstructural abnormalit*,” “MRI,” “MRS,” “MR spectroscopy,” “neuronal dysfunction,” “neuronal function,” “neuropathy,” “perfusion,” “syndrome,” “type 2,” “vessel disease,” “white matter lesion.”
We included articles identified from these searches and relevant references cited in the articles.
The neuropsychological terminology is subdivided in (1) (verbal) memory, (2a) (information) processing speed, (2b) sensory speed, (2c) motor speed, (3) IQ, (4) global cognition, (5) attention functions, (6) executive functions, (7) psychomotor functions, (8) visuoconstruction, and (9) fluency, according to Hebben and Milberg (2009). Speed is subdivided into three components: (1) processing speed (central part/brain), (2) sensory speed (visual aspects) and (3) motor speed (conducting part of a test/trail).
Animal studies, studies on patients with type 1 diabetes mellitus, and studies in which MRI results were presented without addressing correlations with cognitive performance were not included. Only articles written in English were included.
Author Contributions
FCGvB searched for published reports and wrote the first draft of the Review. WB and JJ helped to improve the first draft with addition of relevant reports, suggestions for structure of the Review, and the idea for a schematic table. PH, Rv, Mv, FRJV, HS, MS, CS, and JW read the Review critically and made suggestions for improvements.
Funding
JJ was funded by VENI research grant 916.11.059 from The Netherlands Organization for Scientific Research (NWO) and The Netherlands Organization for Health Research and Development (ZonMw). Additionally, this work was supported by “Stichting de Weijerhorst” foundation.
Conflict of Interest Statement
The authors declare that the research was conducted in the absence of any commercial or financial relationships that could be construed as a potential conflict of interest.
References
Biessels, G. J., and Reijmer, Y. D. (2014). Brain changes underlying cognitive dysfunction in diabetes: what can we learn from MRI? Diabetes 63, 2244–2252. doi: 10.2337/db14-0348
Biessels, G. J., Staekenborg, S., Brunner, E., Brayne, C., and Scheltens, P. (2006). Risk of dementia in diabetes mellitus: a systematic review. Lancet Neurol. 5, 64–74. doi: 10.1016/S1474-4422(05)70284-2
Brownlee, M. (2005). The pathobiology of diabetic complications: a unifying mechanism. Diabetes 54, 1615–1625. doi: 10.2337/diabetes.54.6.1615
Brundel, M., Heringa, S. M., De Bresser, J., Koek, H. L., Zwanenburg, J. J., Jaap Kappelle, L., et al. (2012a). High prevalence of cerebral microbleeds at 7Tesla MRI in patients with early Alzheimer's disease. J. Alzheimers. Dis. 31, 259–263. doi: 10.3233/JAD-2012-120364
Brundel, M., Kappelle, L. J., and Biessels, G. J. (2014a). Brain imaging in type 2 diabetes. Eur. Neuropsychopharmacol. 24, 1967–1981. doi: 10.1016/j.euroneuro.2014.01.023
Brundel, M., Reijmer, Y. D., Van Veluw, S. J., Kuijf, H. J., Luijten, P. R., Kappelle, L. J., et al. (2014b). Cerebral microvascular lesions on high-resolution 7-Tesla MRI in patients with type 2 diabetes. Diabetes 63, 3523–3529. doi: 10.2337/db14-0122
Brundel, M., Van Den Berg, E., Reijmer, Y. D., De Bresser, J., Kappelle, L. J., Biessels, G. J., et al. (2012b). Cerebral haemodynamics, cognition and brain volumes in patients with type 2 diabetes. J. Diabetes Complicat. 26, 205–209. doi: 10.1016/j.jdiacomp.2012.03.021
Chen, Y., Liu, Z., Wang, A., Zhang, J., Zhang, S., Qi, D., et al. (2016). Dysfunctional organization of default mode network before memory impairments in type 2 diabetes. Psychoneuroendocrinology 74, 141–148. doi: 10.1016/j.psyneuen.2016.08.012
Chen, Y., Liu, Z., Zhang, J., Tian, G., Li, L., Zhang, S., et al. (2015). Selectively disrupted functional connectivity networks in type 2 diabetes mellitus. Front. Aging Neurosci. 7:233. doi: 10.3389/fnagi.2015.00233
Chen, Y., Liu, Z., Zhang, J., Xu, K., Zhang, S., Wei, D., et al. (2014). Altered brain activation patterns under different working memory loads in patients with type 2 diabetes. Diabetes Care 37, 3157–3163. doi: 10.2337/dc14-1683
Cheng, G., Huang, C., Deng, H., and Wang, H. (2012). Diabetes as a risk factor for dementia and mild cognitive impairment: a meta-analysis of longitudinal studies. Intern. Med. J. 42, 484–491. doi: 10.1111/j.1445-5994.2012.02758.x
Cui, Y., Jiao, Y., Chen, H. J., Ding, J., Luo, B., Peng, C. Y., et al. (2015). Aberrant functional connectivity of default-mode network in type 2 diabetes patients. Eur. Radiol. 25, 3238–3246. doi: 10.1007/s00330-015-3746-8
Cui, Y., Jiao, Y., Chen, Y. C., Wang, K., Gao, B., Wen, S., et al. (2014). Altered spontaneous brain activity in type 2 diabetes: a resting-state functional MRI study. Diabetes 63, 749–760. doi: 10.2337/db13-0519
de Bresser, J., Tiehuis, A. M., Van Den Berg, E., Reijmer, Y. D., Jongen, C., Kappelle, L. J., et al. (2010). Progression of cerebral atrophy and white matter hyperintensities in patients with type 2 diabetes. Diabetes Care 33, 1309–1314. doi: 10.2337/dc09-1923
De Groot, J. C., De Leeuw, F. E., Oudkerk, M., Van Gijn, J., Hofman, A., Jolles, J., et al. (2002). Periventricular cerebral white matter lesions predict rate of cognitive decline. Ann. Neurol. 52, 335–341. doi: 10.1002/ana.10294
den Heijer, T., Vermeer, S. E., Van Dijk, E. J., Prins, N. D., Koudstaal, P. J., Hofman, A., et al. (2003). Type 2 diabetes and atrophy of medial temporal lobe structures on brain MRI. Diabetologia 46, 1604–1610. doi: 10.1007/s00125-003-1235-0
Eriksson, S. H., Rugg-Gunn, F. J., Symms, M. R., Barker, G. J., and Duncan, J. S. (2001). Diffusion tensor imaging in patients with epilepsy and malformations of cortical development. Brain 124, 617–626. doi: 10.1093/brain/124.3.617
Falvey, C. M., Rosano, C., Simonsick, E. M., Harris, T., Strotmeyer, E. S., Satterfield, S., et al. (2013). Macro- and microstructural magnetic resonance imaging indices associated with diabetes among community-dwelling older adults. Diabetes Care 36, 677–682. doi: 10.2337/dc12-0814
Fazekas, F., Schmidt, R., and Scheltens, P. (1998). Pathophysiologic mechanisms in the development of age-related white matter changes of the brain. Dement. Geriatr. Cogn. Disord. 9(Suppl. 1), 2–5. doi: 10.1159/000051182
Filippi, M., Inglese, M., Rovaris, M., and Rocca, M. A. (2010). “Diffusion and perfusion MRI in inflammation and demyelination,” in Clinical MR Neuroimaging: Physiological and Functional Techniques, 2nd Edn., eds J. H. Gillard, A. D. Waldman, and P. B. Barker (New York, NY: Cambridge University Press), 488–500.
Geijselaers, S. L., Sep, S. J., Stehouwer, C. D., and Biessels, G. J. (2015). Glucose regulation, cognition, and brain MRI in type 2 diabetes: a systematic review. Lancet Diabetes Endocrinol 3, 75–89. doi: 10.1016/S2213-8587(14)70148-2
Gispen, W. H., and Biessels, G. J. (2000). Cognition and synaptic plasticity in diabetes mellitus. Trends Neurosci. 23, 542–549. doi: 10.1016/S0166-2236(00)01656-8
Gold, S. M., Dziobek, I., Sweat, V., Tirsi, A., Rogers, K., Bruehl, H., et al. (2007). Hippocampal damage and memory impairments as possible early brain complications of type 2 diabetes. Diabetologia 50, 711–719. doi: 10.1007/s00125-007-0602-7
Gruetter, R., Garwood, M., Ugurbil, K., and Seaquist, E. R. (1996). Observation of resolved glucose signals in 1H NMR spectra of the human brain at 4 Tesla. Magn. Reson. Med. 36, 1–6. doi: 10.1002/mrm.1910360102
Grundy, S. M. (2006). Metabolic syndrome: connecting and reconciling cardiovascular and diabetes worlds. J. Am. Coll. Cardiol. 47, 1093–1100. doi: 10.1016/j.jacc.2005.11.046
Haroon, E., Watari, K., Thomas, A., Ajilore, O., Mintz, J., Elderkin-Thompson, V., et al. (2009). Prefrontal myo-inositol concentration and visuospatial functioning among diabetic depressed patients. Psychiatry Res. 171, 10–19. doi: 10.1016/j.pscychresns.2008.03.006
Hayashi, K., Kurioka, S., Yamaguchi, T., Morita, M., Kanazawa, I., Takase, H., et al. (2011). Association of cognitive dysfunction with hippocampal atrophy in elderly Japanese people with type 2 diabetes. Diabetes Res. Clin. Pract. 94, 180–185. doi: 10.1016/j.diabres.2011.07.002
He, X. S., Wang, Z. X., Zhu, Y. Z., Wang, N., Hu, X., Zhang, D. R., et al. (2015). Hyperactivation of working memory-related brain circuits in newly diagnosed middle-aged type 2 diabetics. Acta Diabetol. 52, 133–142. doi: 10.1007/s00592-014-0618-7
Hebben, N., and Milberg, W. (2009). Essentials of Neuropsychological Assessment. Hoboken, NJ: John Wiley & Sons Ltd.
Hoogenboom, W. S., Marder, T. J., Flores, V. L., Huisman, S., Eaton, H. P., Schneiderman, J. S., et al. (2014). Cerebral white matter integrity and resting-state functional connectivity in middle-aged patients with type 2 diabetes. Diabetes 63, 728–738. doi: 10.2337/db13-1219
Imamine, R., Kawamura, T., Umemura, T., Umegaki, H., Kawano, N., Hotta, M., et al. (2011). Does cerebral small vessel disease predict future decline of cognitive function in elderly people with type 2 diabetes? Diabetes Res. Clin. Pract. 94, 91–99. doi: 10.1016/j.diabres.2011.06.014
Jansen, J. F., Backes, W. H., Nicolay, K., and Kooi, M. E. (2006). 1H MR spectroscopy of the brain: absolute quantification of metabolites. Radiology 240, 318–332. doi: 10.1148/radiol.2402050314
Jansen, J. F., Van Bussel, F. C., Van De Haar, H. J., Van Osch, M. J., Hofman, P., Van Boxtel, M. P., et al. (2016). Cerebral blood flow, blood supply, and cognition in Type 2 Diabetes Mellitus. Sci. Rep. 6:10. doi: 10.1038/s41598-016-0003-6
Jobst, K. A., Smith, A. D., Szatmari, M., Esiri, M. M., Jaskowski, A., Hindley, N., et al. (1994). Rapidly progressing atrophy of medial temporal lobe in Alzheimer's disease. Lancet 343, 829–830. doi: 10.1016/S0140-6736(94)92028-1
Jongen, C., Van Der Grond, J., Kappelle, L. J., Biessels, G. J., Viergever, M. A., Pluim, J. P., et al. (2007). Automated measurement of brain and white matter lesion volume in type 2 diabetes mellitus. Diabetologia 50, 1509–1516. doi: 10.1007/s00125-007-0688-y
Jouvent, E., Viswanathan, A., and Chabriat, H. (2010). Cerebral atrophy in cerebrovascular disorders. J. Neuroimaging 20, 213–218. doi: 10.1111/j.1552-6569.2009.00370.x
Koekkoek, P. S., Rutten, G. E., and Biessels, G. J. (2014). Cognitive disorders in diabetic patients. Handb. Clin. Neurol. 126, 145–166. doi: 10.1016/B978-0-444-53480-4.00011-4
Le Bihan, D., Breton, E., Lallemand, D., Grenier, P., Cabanis, E., and Laval-Jeantet, M. (1986). MR imaging of intravoxel incoherent motions: application to diffusion and perfusion in neurologic disorders. Radiology 161, 401–407. doi: 10.1148/radiology.161.2.3763909
Manschot, S. M., Brands, A. M., Van Der Grond, J., Kessels, R. P., Algra, A., Kappelle, L. J., et al. (2006). Brain magnetic resonance imaging correlates of impaired cognition in patients with type 2 diabetes. Diabetes 55, 1106–1113. doi: 10.2337/diabetes.55.04.06.db05-1323
Marder, T. J., Flores, V. L., Bolo, N. R., Hoogenboom, W. S., Simonson, D. C., Jacobson, A. M., et al. (2014). Task-induced brain activity patterns in type 2 diabetes: a potential biomarker for cognitive decline. Diabetes 63, 3112–3119. doi: 10.2337/db13-1783
Moran, C., Phan, T. G., Chen, J., Blizzard, L., Beare, R., Venn, A., et al. (2013). Brain atrophy in type 2 diabetes: regional distribution and influence on cognition. Diabetes Care 36, 4036–4042. doi: 10.2337/dc13-0143
Musen, G., Jacobson, A. M., Bolo, N. R., Simonson, D. C., Shenton, M. E., McCartney, R. L., et al. (2012). Resting-state brain functional connectivity is altered in type 2 diabetes. Diabetes 61, 2375–2379. doi: 10.2337/db11-1669
Novak, V., Milberg, W., Hao, Y., Munshi, M., Novak, P., Galica, A., et al. (2014). Enhancement of vasoreactivity and cognition by intranasal insulin in type 2 diabetes. Diabetes Care 37, 751–759. doi: 10.2337/dc13-1672
Puts, N. A., and Edden, R. A. (2012). In vivo magnetic resonance spectroscopy of GABA: a methodological review. Prog. Nucl. Magn. Reson. Spectrosc. 60, 29–41. doi: 10.1016/j.pnmrs.2011.06.001
Raji, C. A., Eyre, H., Wei, S. H., Bredesen, D. E., Moylan, S., Law, M., et al. (2015). Hot topics in research: preventive neuroradiology in brain aging and cognitive decline. AJNR Am. J. Neuroradiol. 36, 1803–1809. doi: 10.3174/ajnr.A4409
Reijmer, Y. D., Brundel, M., De Bresser, J., Kappelle, L. J., Leemans, A., Biessels, G. J., et al. (2013a). Microstructural white matter abnormalities and cognitive functioning in type 2 diabetes: a diffusion tensor imaging study. Diabetes Care 36, 137–144. doi: 10.2337/dc12-0493
Reijmer, Y. D., Leemans, A., Brundel, M., Kappelle, L. J., and Biessels, G. J. Utrecht Vascular Cognitive Impairment Study Group (2013b). Disruption of the cerebral white matter network is related to slowing of information processing speed in patients with type 2 diabetes. Diabetes 62, 2112–2115. doi: 10.2337/db12-1644
Reijmer, Y. D., Van Den Berg, E., De Bresser, J., Kessels, R. P., Kappelle, L. J., Algra, A., et al. (2011). Accelerated cognitive decline in patients with type 2 diabetes: MRI correlates and risk factors. Diabetes Metab. Res. Rev. 27, 195–202. doi: 10.1002/dmrr.1163
Rusinek, H., Ha, J., Yau, P. L., Storey, P., Tirsi, A., Tsui, W. H., et al. (2015). Cerebral perfusion in insulin resistance and type 2 diabetes. J. Cereb. Blood Flow Metab. 35, 95–102. doi: 10.1038/jcbfm.2014.173
Ryan, J. P., Fine, D. F., and Rosano, C. (2014). Type 2 diabetes and cognitive impairment: contributions from neuroimaging. J. Geriatr. Psychiatry Neurol. 27, 47–55. doi: 10.1177/0891988713516543
Schneider, J. A., Wilson, R. S., Cochran, E. J., Bienias, J. L., Arnold, S. E., Evans, D. A., et al. (2003). Relation of cerebral infarctions to dementia and cognitive function in older persons. Neurology 60, 1082–1088. doi: 10.1212/01.WNL.0000055863.87435.B2
Steen, E., Terry, B. M., Rivera, E. J., Cannon, J. L., Neely, T. R., Tavares, R., et al. (2005). Impaired insulin and insulin-like growth factor expression and signaling mechanisms in Alzheimer's disease–is this type 3 diabetes? J. Alzheimers. Dis. 7, 63–80.
Taheri, S., Gasparovic, C., Shah, N. J., and Rosenberg, G. A. (2011). Quantitative measurement of blood-brain barrier permeability in human using dynamic contrast-enhanced MRI with fast T1 mapping. Magn. Reson. Med. 65, 1036–1042. doi: 10.1002/mrm.22686
Thompson, P. M., Hayashi, K. M., De Zubicaray, G., Janke, A. L., Rose, S. E., Semple, J., et al. (2003). Dynamics of gray matter loss in Alzheimer's disease. J. Neurosci. 23, 994–1005.
Tiehuis, A. M., Mali, W. P., Van Raamt, A. F., Visseren, F. L., Biessels, G. J., Van Zandvoort, M. J., et al. (2009). Cognitive dysfunction and its clinical and radiological determinants in patients with symptomatic arterial disease and diabetes. J. Neurol. Sci. 283, 170–174. doi: 10.1016/j.jns.2009.02.337
Tiehuis, A. M., Vincken, K. L., Van Den Berg, E., Hendrikse, J., Manschot, S. M., Mali, W. P., et al. (2008). Cerebral perfusion in relation to cognitive function and type 2 diabetes. Diabetologia 51, 1321–1326. doi: 10.1007/s00125-008-1041-9
Tiehuis, A., Van Der Meer, F., Mali, W., Pleizier, M., Biessels, G. J., Kappelle, J., et al. (2010). MR spectroscopy of cerebral white matter in type 2 diabetes; no association with clinical variables and cognitive performance. Neuroradiology 52, 155–161. doi: 10.1007/s00234-009-0598-4
Tofts, P. (2003). Quantitative MRI of the Brain Measuring Changes Caused by Disease. Chichester; Hoboken: John Wiley & Sons Ltd.
Umegaki, H. (2010). Pathophysiology of cognitive dysfunction in older people with type 2 diabetes: vascular changes or neurodegeneration? Age Ageing 39, 8–10. doi: 10.1093/ageing/afp211
Umegaki, H., Kawamura, T., Kawano, N., Umemura, T., Kanai, A., and Sano, T. (2011). Factors associated with cognitive decline in elderly diabetics. Dement. Geriatr. Cogn. Dis. Extra 1, 1–9. doi: 10.1159/000323188
van Bussel, F. C., Backes, W. H., Hofman, P. A., Puts, N. A., Edden, R. A., Van Boxtel, M. P., et al. (2016a). Increased GABA concentrations in type 2 diabetes mellitus are related to lower cognitive functioning. Medicine (Baltimore). 95:e4803. doi: 10.1097/MD.0000000000004803
van Bussel, F. C., Backes, W. H., Hofman, P. A., Van Boxtel, M. P., Schram, M. T., Stehouwer, C. D., et al. (2016b). Altered hippocampal white matter connectivity in Type 2 Diabetes Mellitus and memory decrements. J. Neuroendocrinol. 28, 12366. doi: 10.1111/jne.12366
van Bussel, F. C., Backes, W. H., Hofman, P. A., Van Oostenbrugge, R. J., Kessels, A. G., Van Boxtel, M. P., et al. (2015). On the interplay of microvasculature, parenchyma, and memory in type 2 diabetes. Diabetes Care 38, 876–882. doi: 10.2337/dc14-2043
van Bussel, F. C., Backes, W. H., Van Veenendaal, T. M., Hofman, P. A., Van Boxtel, M. P., Schram, M. T., et al. (2016c). Functional brain networks are altered in type 2 diabetes and prediabetes: signs for compensation of cognitive decrements? the maastricht study. Diabetes 65, 2404–2413. doi: 10.2337/db16-0128
van De Ven, K. C., Van Der Graaf, M., Tack, C. J., Heerschap, A., and De Galan, B. E. (2012). Steady-state brain glucose concentrations during hypoglycemia in healthy humans and patients with type 1 diabetes. Diabetes 61, 1974–1977. doi: 10.2337/db11-1778
van Elderen, S. G., De Roos, A., De Craen, A. J., Westendorp, R. G., Blauw, G. J., Jukema, J. W., et al. (2010). Progression of brain atrophy and cognitive decline in diabetes mellitus: a 3-year follow-up. Neurology 75, 997–1002. doi: 10.1212/WNL.0b013e3181f25f06
van Golen, L. W., Kuijer, J. P., Huisman, M. C., Rg, I. J., Barkhof, F., Diamant, M., et al. (2014). Quantification of cerebral blood flow in healthy volunteers and type 1 diabetic patients: comparison of MRI arterial spin labeling and [15O]H2O positron emission tomography (PET). J. Magn. Reson. Imaging 40, 1300–1309. doi: 10.1002/jmri.24484
van Harten, B., De Leeuw, F. E., Weinstein, H. C., Scheltens, P., and Biessels, G. J. (2006). Brain imaging in patients with diabetes: a systematic review. Diabetes Care 29, 2539–2548. doi: 10.2337/dc06-1637
van Harten, B., Oosterman, J., Muslimovic, D., Van Loon, B. J., Scheltens, P., and Weinstein, H. C. (2007). Cognitive impairment and MRI correlates in the elderly patients with type 2 diabetes mellitus. Age Ageing 36, 164–170. doi: 10.1093/ageing/afl180
vermeer, S. E., Longstreth, W. T. Jr., and Koudstaal, P. J. (2007). Silent brain infarcts: a systematic review. Lancet Neurol. 6, 611–619. doi: 10.1016/S1474-4422(07)70170-9
Wardlaw, J. M., Smith, E. E., Biessels, G. J., Cordonnier, C., Fazekas, F., Frayne, R., et al. (2013). Neuroimaging standards for research into small vessel disease and its contribution to ageing and neurodegeneration. Lancet Neurol. 12, 822–838. doi: 10.1016/S1474-4422(13)70124-8
Xia, W., Rao, H., Spaeth, A. M., Huang, R., Tian, S., Cai, R., et al. (2015a). Blood pressure is associated with cerebral blood flow alterations in patients with T2DM as revealed by perfusion functional MRI. Medicine (Baltimore). 94:e2231. doi: 10.1097/MD.0000000000002231
Xia, W., Wang, S., Rao, H., Spaeth, A. M., Wang, P., Yang, Y., et al. (2015b). Disrupted resting-state attentional networks in T2DM patients. Sci. Rep. 5:11148. doi: 10.1038/srep11148
Xia, W., Wang, S., Spaeth, A. M., Rao, H., Wang, P., Yang, Y., et al. (2015c). Insulin resistance-associated interhemispheric functional connectivity alterations in T2DM: A resting-state fMRI study. Biomed Res. Int. 2015:719076. doi: 10.1155/2015/719076
Xia, W., Wang, S., Sun, Z., Bai, F., Zhou, Y., Yang, Y., et al. (2013). Altered baseline brain activity in type 2 diabetes: a resting-state fMRI study. Psychoneuroendocrinology 38, 2493–2501. doi: 10.1016/j.psyneuen.2013.05.012
Xia, W., Zhang, B., Yang, Y., Wang, P., Yang, Y., and Wang, S. (2015d). Poorly controlled cholesterol is associated with cognitive impairment in T2DM: a resting-state fMRI study. Lipids Health Dis. 14:47. doi: 10.1186/s12944-015-0046-x
Xiong, Y., Sui, Y., Xu, Z., Zhang, Q., Karaman, M. M., Cai, K., et al. (2016). A diffusion tensor imaging study on white matter abnormalities in patients with type 2 diabetes using tract-based spatial statistics. AJNR Am. J. Neuroradiol. 37, 1462–1469. doi: 10.3174/ajnr.A4740
Yang, S. Q., Xu, Z. P., Xiong, Y., Zhan, Y. F., Guo, L. Y., Zhang, S., et al. (2016). Altered Intranetwork and internetwork functional connectivity in type 2 diabetes mellitus with and without cognitive impairment. Sci. Rep. 6:32980. doi: 10.1038/srep32980
Yau, P. L., Javier, D. C., Ryan, C. M., Tsui, W. H., Ardekani, B. A., Ten, S., et al. (2010). Preliminary evidence for brain complications in obese adolescents with type 2 diabetes mellitus. Diabetologia 53, 2298–2306. doi: 10.1007/s00125-010-1857-y
Yau, P. L., Javier, D., Tsui, W., Sweat, V., Bruehl, H., Borod, J. C., et al. (2009). Emotional and neutral declarative memory impairments and associated white matter microstructural abnormalities in adults with type 2 diabetes. Psychiatry Res. 174, 223–230. doi: 10.1016/j.pscychresns.2009.04.016
Zhang, H., Hao, Y., Manor, B., Novak, P., Milberg, W., Zhang, J., et al. (2015). Intranasal insulin enhanced resting-state functional connectivity of hippocampal regions in type 2 diabetes. Diabetes 64, 1025–1034. doi: 10.2337/db14-1000
Zhang, J., Liu, Z., Li, Z., Wang, Y., Chen, Y., Li, X., et al. (2016). Disrupted white matter network and cognitive decline in type 2 diabetes patients. J. Alzheimers. Dis. 53, 185–195. doi: 10.3233/JAD-160111
Zhang, J., Wang, Y., Wang, J., Zhou, X., Shu, N., Wang, Y., et al. (2014). White matter integrity disruptions associated with cognitive impairments in type 2 diabetic patients. Diabetes 63, 3596–3605. doi: 10.2337/db14-0342
Zhang, Y., Lu, S., Liu, C., Zhang, H., Zhou, X., Ni, C., et al. (2016). Altered brain activation and functional connectivity in working memory related networks in patients with type 2 diabetes: An ICA-based analysis. Sci. Rep. 6:23767. doi: 10.1038/srep23767
Zhang, Y.-W., Zhang, J. Q., Liu, C., Wei, P., Zhang, X., Yuan, Q. Y., et al. (2015). Memory dysfunction in type 2 diabetes mellitus correlates with reduced hippocampal CA1 and subiculum volumes. Chin. Med. J. 128, 465–471. doi: 10.4103/0366-6999.151082
Zhang, Y., Zhang, X., Zhang, J., Liu, C., Yuan, Q., Yin, X., et al. (2014). Gray matter volume abnormalities in type 2 diabetes mellitus with and without mild cognitive impairment. Neurosci. Lett. 562, 1–6. doi: 10.1016/j.neulet.2014.01.006
Zhou, H., Lu, W., Shi, Y., Bai, F., Chang, J., Yuan, Y., et al. (2010). Impairments in cognition and resting-state connectivity of the hippocampus in elderly subjects with type 2 diabetes. Neurosci. Lett. 473, 5–10. doi: 10.1016/j.neulet.2009.12.057
Keywords: type 2 diabetes mellitus, magnetic resonance imaging, cognition, functional MRI, multiparametric MRI
Citation: van Bussel FCG, Backes WH, Hofman PAM, van Oostenbrugge RJ, van Boxtel MPJ, Verhey FRJ, Steinbusch HWM, Schram MT, Stehouwer CDA, Wildberger JE and Jansen JFA (2017) Cerebral Pathology and Cognition in Diabetes: The Merits of Multiparametric Neuroimaging. Front. Neurosci. 11:188. doi: 10.3389/fnins.2017.00188
Received: 19 September 2016; Accepted: 21 March 2017;
Published: 05 April 2017.
Edited by:
Gaetano Santulli, Columbia University, USAReviewed by:
Quan Zhang, Tianjin Medical University General Hospital, ChinaAngela Lombardi, Albert Einstein College of Medicine, USA
Copyright © 2017 van Bussel, Backes, Hofman, van Oostenbrugge, van Boxtel, Verhey, Steinbusch, Schram, Stehouwer, Wildberger and Jansen. This is an open-access article distributed under the terms of the Creative Commons Attribution License (CC BY). The use, distribution or reproduction in other forums is permitted, provided the original author(s) or licensor are credited and that the original publication in this journal is cited, in accordance with accepted academic practice. No use, distribution or reproduction is permitted which does not comply with these terms.
*Correspondence: Jacobus F. A. Jansen, jacobus.jansen@mumc.nl