- 1Department of Psychiatry, University of North Carolina at Chapel Hill, Chapel Hill, NC, USA
- 2Department of Computer Science, University of North Carolina at Chapel Hill, Chapel Hill, NC, USA
- 3Kitware, Inc., Carrboro, NC, USA
- 4Harlow Center for Biological Psychology, University of Wisconsin-Madison, Madison, WI, USA
- 5Waisman Laboratory for Brain Imaging and Behavior, University of Wisconsin-Madison, Madison, WI, USA
Rhesus macaques are commonly used as a translational animal model in neuroimaging and neurodevelopmental research. In this report, we present longitudinal data from both structural and diffusion MRI images generated on a cohort of 34 typically developing monkeys from 2 weeks to 36 months of age. All images have been manually skull stripped and are being made freely available via an online repository for use by the research community.
Introduction
Brain maturation is a complex process driven by cell proliferation and differentiation, myelination, and the growth of neurons and their connections during the first years of life. The initial increase in brain connections is followed by a process of dendritic pruning and loss of synaptic contacts, presumably shaping and sculpting a more efficient network of connections that are continuously remodeled throughout life (Engert and Bonhoeffer, 1999; Stepanyants et al., 2002; Lebel et al., 2008). Although neurobehavioral maturation has been studied extensively, both at a functional level (behavior) and at a structural and mediating level (cellular anatomy and physiology), information at the level of neuroanatomical connectivity and about maturational changes during the peripubertal years is not as comprehensive. This information is of special interest because it provides insight into a different aspect of neural malleability and also captures a second period of major change leading up to the adult phenotype. Furthermore, understanding developmental changes in the brain will ultimately help us to better understand the etiology of psychiatric conditions that first present clearly in adolescence, such as depression, and to devise targeted therapies for neurodegenerative brain disorders with an onset later in adulthood.
Non-human primate models are widely used to provide comparative information associated with human neuropathology (Glatzel et al., 2002; Grant and Bennett, 2003; Machado and Bachevalier, 2003; Lebherz et al., 2005; Sullivan et al., 2005; Barr and Goldman, 2006; Lubach and Coe, 2006; Segerstrom et al., 2006; Bennett, 2008; Williams et al., 2008). Advantages include the biological similarity of monkeys and humans, such as the gestation of a single offspring, a prolonged in utero development, and the maturational stage of the neonatal brain at birth. Among non-human primate models, the rhesus macaque (Macaca mulatta) has been the most widely used monkey to investigate the neural substrates of human behavior due to its phylogenetic closeness to humans (Lacreuse and Herndon, 2009) and the potential to examine more complex cognitive functions and social behavior associated with encephalization (Price and Coe, 2000). Furthermore, primate models allow for brain imaging at the very early, critical stages of neurodevelopment when it is challenging to recruit and image young human participants under rigorous experimental control. The use of monkeys in a laboratory setting also facilitates environmentally controlled studies with all infants raised similarly. Additionally, rhesus macaques show hemispheric asymmetry and sex differences in brain size and maturational rates during adolescence similar to humans. For more than 40 years, this species has also been used to evaluate how disturbances of the early rearing environment can induce behavioral abnormalities and influence brain development (Harlow et al., 1971).
MRI studies have significantly expanded our knowledge of human brain development during childhood and adolescence through several large-scale cross-sectional (Pfefferbaum et al., 1994; Caviness et al., 1996; Reiss et al., 1996; Courchesne et al., 2000; De Bellis et al., 2001) and longitudinal studies (Giedd et al., 1999; Sowell et al., 2004; Lenroot and Giedd, 2006). Critical periods in human brain development have been identified. In contrast, there are still some lacunae in our knowledge of non-human primate development, especially with regard to the maturational changes during the peripubertal years. While there are detailed neuroanatomical descriptions of early brain maturation in the monkey (Rakic and Goldman-Rakic, 1982), less information is available on the normal postnatal maturation of the monkey brain, with the exception of certain brain regions, such as the visual cortex. The prevailing view from studies in humans is that the total brain volume undergoes a rapid non-linear increase during childhood and reaches a maximum size around puberty. Gray matter and white matter follow distinct structure-specific developmental trajectories (Jernigan and Tallal, 1990; Schaefer et al., 1990; Reiss et al., 1996; Giedd et al., 1999; Gilmore et al., 2007a; Knickmeyer et al., 2008). While human studies suggest that postnatal cortical development is very heterochronous, postmortem studies suggest that cortical development in monkeys is more synchronous (Rakic et al., 1986; Bourgeois and Rakic, 1993).
Studies in humans indicate that there is marked sexual dimorphism in the central nervous system during childhood and adulthood. The most consistent findings include: larger volume of the cerebrum in males, higher proportion of gray matter to white matter in females, relatively greater volume of the amygdala in males, and relatively greater volume of the caudate and hippocampus in females (Dekaban, 1978; Filipek et al., 1994; Caviness et al., 1996; Giedd et al., 1996, 1997; Reiss et al., 1996; Nopoulos et al., 1997; Filipek, 1999; Gur et al., 1999; Goldstein et al., 2001; Good et al., 2001; De Bellis et al., 2001; Allen et al., 2003; Gilmore et al., 2007b; Knickmeyer et al., 2008). Studies in macaques have shown similar developmental trajectories (Knickmeyer et al., 2010; Hunsaker et al., 2014). However, it is important to note that there have been some conflicting findings in the macaque with Payne et al. finding no sex differences in the hippocampus or amygdala, although hemispheric asymmetries were present (Payne et al., 2010). Additionally, in humans, males and females also differ in specific developmental growth trajectories. Total cerebral volume, caudate volume, and gray matter volume in the frontal and parietal lobes peak earlier in girls than in boys (ages vary depending on region), a pattern which may relate to sex differences in the timing of puberty. In adolescence, white matter increases more rapidly in males than females (Lenroot et al., 2007). These sex differences in neurobiology may be relevant to certain neurodevelopmental pathologies, which frequently indicate that there are marked sex differences in risk, phenotypic expression, and treatment response (Szatmari et al., 1989; Moffitt, 1990; Gur et al., 1996; Häfner et al., 1998; Chakrabarti and Fombonne, 2001; Moffitt and Caspi, 2001; Baird et al., 2006; Kulkarni et al., 2008). Research on monkeys can be particularly informative about the role of pubertal onset in determining these brain changes because females reach puberty1–3 years before males. In addition, adult rhesus monkeys also display large sex differences in brain size (Falk et al., 1999). This dimorphism occurs in part because the male brain continues to grow significantly after puberty (Franklin et al., 2000). However, some areas, such as the amygdala, continue to be of similar size in male and female monkeys.
As portrayed in Figure 1 below, white matter changes are observed throughout the first two decades of life and beyond in humans (Lebel et al., 2008) and can be measured by DTI. Few developmental studies using DTI have been published for non-human primates. Makris et al. (2007) investigated changes in white matter fiber bundles with aging, but this study was done in elderly macaques. They reported reductions in fractional anisotropy (FA) in cortico-cortical association fibers with age and general agreement with observations in humans. Styner et al. (2008) explored changes in the developing macaque brain using an atlas-driven brain parcellation and showed increases in FA, particularly in the corpus callosum, as well as a decrease in mean diffusivity. Combining structural MRI and DTI is a particularly useful approach to investigate brain development over the full course of neurodevelopment. While DTI provides sufficient white matter contrast at the earliest stages of brain development, it is more difficult to analyze the fetal and young infant brain with structural MRI due to weak intensity contrast and contrast inversion.
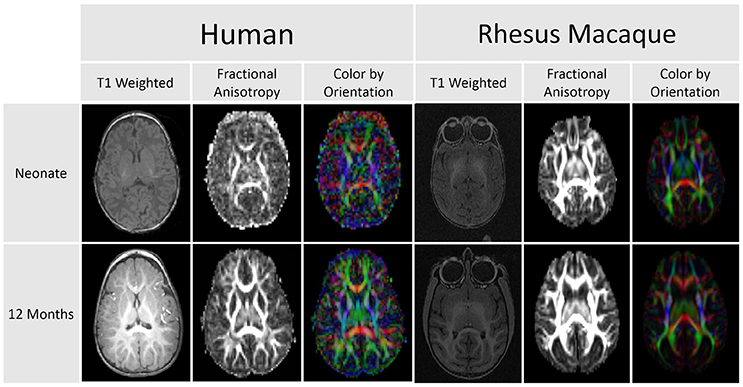
Figure 1. This comparison of DTI scans for humans and rhesus macaques demonstrates that diffusion anisotropy is observable before a clear distinction between the white and gray matter is visible in the T1 weighted image. This difference in clarity and delineation is apparent in both the human and macaque images.
Scott et al. also investigated typical brain development in the rhesus macaque (Scott et al., 2016). However, DTI data were not presented and those data have not been made publically available at this time. A DTI atlas characterizing typical brain development in the rhesus macaque was made publically available, however, it had a relatively low resolution and few gradient directions (Zakszewski et al., 2014). Additionally, this study only made available the final atlas and not any of the individual scans.
The current paper marks the major release of new data to our publicly available MRI database characterizing macaque brain development. It is hoped that this freely available resource will be of great utility to others in the neuroimaging field and enable more effective use of monkeys as translational models in both basic neuroscience and clinical research. Non-human primates, such as the rhesus macaque, provide a unique opportunity to study normal brain maturation and behavioral development in a comparative manner, especially in early infancy, where crucial information is still missing. Additionally, this database will continue to be updated as we process the data, create atlases, and perform fiber tracking on the DTI data.
Methods
Subjects
The subjects for these analyses were 34 rhesus monkeys (Macaca mulatta) reared and housed at the Harlow Primate Laboratory (HPL) at the University of Wisconsin-Madison. The HPL maintains a 500+ monkey colony dedicated to producing infants, with a capacity for generating approximately 70–100 infants per year. Complete rearing and health histories, as well as pedigrees, are known for all animals. Adults were mated with single sires to ensure the required number of subjects was met. Only healthy infants and juveniles were assigned to this project.
The housing consisted of stainless steel caging (each 0.9 × 0.9 × 0.9 m), where each female monkey lived with her infant, either individually or as a pair with another adult female in double cages. The housing arrangement is conducive to rigorous experimental control over pregnancy conditions, food consumption, and other environmental variables. Animals were fed a standardized diet of commercial biscuits and fruit supplements and foraging devices for enrichment. Water was available ad libitum, the temperature was controlled at 21.5°C, and the light/dark cycle was maintained at 14:10 with lights on at 06:00.
All infants were reared normally by their mothers until weaning occurred at 6–7 months of age. Afterwards, the older juveniles were housed in small social groups or as a pair to provide companionship. This rearing and housing strategy was designed to facilitate their normal socialization and to ensure standardized rearing conditions.
The research protocol was approved by the Institutional Animal Care and Use Committee (IACUC). Care and treatment of the animals at HPL are designed to meet and exceed the guidelines promulgated by the National Institutes of Health Guide for the Care and Use of Laboratory Animals. The quality of the research findings is predicated on the high quality of care.
Subject Pedigree
Of the 34 macaques included in this study, three of the subjects were maternal half siblings, while eighteen were paternal half siblings (same father). Six of the eighteen paternal half siblings had two paternally related half siblings. No subject had more than two half siblings or both a paternal and maternal half sibling. Additionally, none of the subjects were full siblings. See Table 1 for full pedigree information.
Inclusion Criteria for Dams
The breeding females chosen for this study were adults between 5 and 15 years of age and multiparous. The females were healthy, did not have a prior history of a Caesarean section, and had successfully reared at least one infant.
Gestational and Infant Growth Parameters
Female macaques were mated with a designated sire to ensure knowledge of paternity. Maternal and infant weight data were obtained at periodic intervals to document normal growth. All monkeys scanned were healthy, and any illness would have been evaluated by the attending veterinarian.
Scanning Schedule
Thirty-six subjects were initially assigned to this study which involved a series of up to five scans at scheduled intervals. Two subjects were ultimately excluded during the course of the study: one because of an adverse reaction to the anesthetic used for scanning, and a second because of illness during early postnatal development prior to first scan. All subjects retained in the final analyses were scanned five times, except for one subject scanned only four times due to a scheduling issue. There were additionally two subjects scanned only once and then subsequently dropped from the study due to adverse reactions to the anesthetic. However, these single scans were included in this analysis because they provided information on the critical early infancy period (<3 months of age).
The original scanning schedule was as follows: for subjects with their first scan when younger than 4 months, a 3 month scan interval was used. For subjects with their first scan at 4 months or older, a 4 month scan interval was used. Two animals were scanned at each time point, one male and one female. Four animal pairs were scanned beginning at 2 weeks of age, two pairs beginning at 1 and 2 months of age, and one pair scanned beginning at 3, 4, 5, 6, 8, 12, 16, and 20 months of age. During the course of this project, a small adjustment was made in the schedule (see Figure 2). The scanning schedule was amended such that two pairs of subjects were first scanned when younger than 5 months in order to increase the sample size for early infancy.
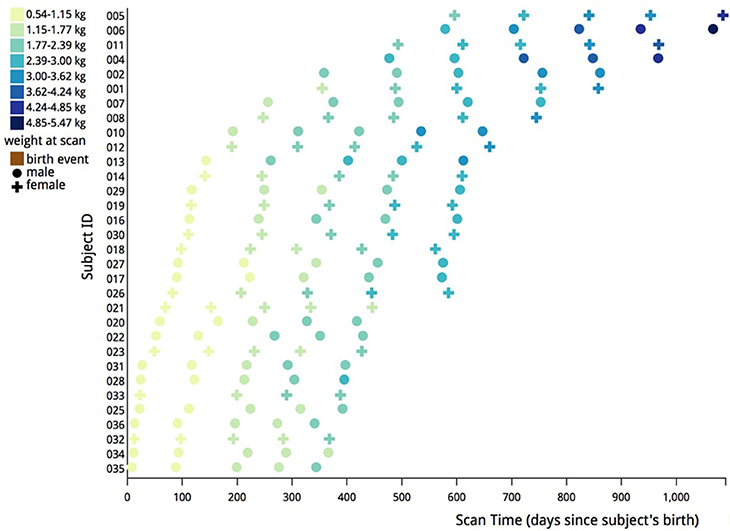
Figure 2. Illustration of actual scanning schedule used in this analysis. Each data point represents a scan of either a male (circle) or female (cross) subject. The data points are color coded based on the weight in kilograms of each subject at the time of the scan. Most subjects were scanned at five different times to create the data for this study.
This staggered scheme also allowed for testing any possible influence of the sedation or handling procedures. Another advantage of this experimental design is the relatively large sample size, with the repeated scanning enabling us to conduct both longitudinal and cross-sectional analyses.
MRI Acquisition
Scans were performed on a GE MR750 3.0T scanner (General Electric Medical, Milwaukee WI) using the human 8-channel brain array coil at the Waisman Laboratory for Brain Imaging and Behavior at the University of Wisconsin-Madison. In order to ensure a safe plane of anesthesia and recovery, the scanning protocol for animals younger than 6 months lasted approximately 30 min. For subjects older than 6 months of age, the scanning procedure was extended to slightly <1 h to improve the signal-to-noise ratio on the diffusion weighted image (DWI) scan.
Anesthesia
Subjects were given a pre-anesthetic (ketamine hydrochloride 10 mg/kg I.M.) for transport to the MRI facility. For infants younger than 6 months of age, immobilization during the scan was achieved with inhalant isoflurane. Older subjects were immobilized throughout the scanning procedure by an initial administration of ketamine hydrochloride (10 mg/kg I.M.) followed by dexdomitor (0.01 mg/kg I.M.). The effects were reversed at the end of the session by administering atipamezole (0.15 mg/kg I.V.). The plane of anesthesia was monitored with a pulse oximeter to track heart rate and oxygen saturation in both younger and older subjects.
Structural Imaging
High-resolution 3D T1-weighted imaging was performed using an axial Inversion Recovery (IR) prepared fast gradient echo (fGRE) sequence (GE BRAVO) (TI = 450 ms, TR = 8.684 ms, TE = 3.652 ms, FOV = 140 × 140 mm, flip angle = 12°, matrix = 256 × 256, thickness = 0.8 mm, gap = −0.4 mm, 80 percent field-of-view in phase encoding direction, bandwidth = 31.25 kHz, 2 averages, total time = 10:46 min) provided an effective voxel resolution of 0.55 × 0.55 × 0.8 mm across the entire cranium. The T2-weighted scan was performed using a sagittal 3D CUBE FSE sequence (TR = 2500 ms, TE = 87 ms, FOV = 154 × 154 mm, flip angle = 90°, matrix = 256 × 256, 90 percent field of view in the phase encoding direction, slice thickness = 0.6 mm, gap = 0 mm, bandwidth = 62.5 kHz, ARC parallel imaging with a factor of 2 acceleration in both phase encoding and slice encoding directions, total time = 6:36 min) across the cranium was acquired with a voxel resolution of 0.6 × 0.6 × 0.6 mm.
Diffusion Imaging
The following DWI protocol was used in order to obtain the highest possible resolution for the DTI: TR = 8000 ms, TE = 65.7 ms, FOV = 16.7 mm, matrix = 128 × 128, 2.6 mm slice thickness with 1.3 mm slice overlap (resolution 1.3 × 1.3 × 2.6 mm3), upsampled to an voxel dimension on the scanner of 0.65 × 0.65 × 1.3 mm3, ASSET parallel imaging with an acceleration factor of 2, and a coronal slice orientation. The overlapping slices provide finer spatial sampling than the original resolution (similar to the Fourier interpolation in-plane), and also provide higher SNR. There were 120 unique gradient directions acquired with b = 1000 s/mm2 and ten baseline images with b = 0 s/mm2. A scan time of approximately 17.5 min was required to obtain these images.
Data Transfer
All MRI data were transferred electronically via an existing web-based upload center from the Waisman Imaging Center to the UNC NIRAL, where they were integrated into study data and automatically backed up on local servers.
Image Analysis
All of the images were segmented automatically using an existing image analysis pipeline. However, many of the automatically generated segmentations were inaccurate and all segmentations were examined manually and corrected. Subjects younger than 6 months of age required the most significant manual corrections. Manual correction of the segmentations was performed using the ITK-SNAP1 tool. An overview of the pipeline is described below and can be seen visually in Figure 3.
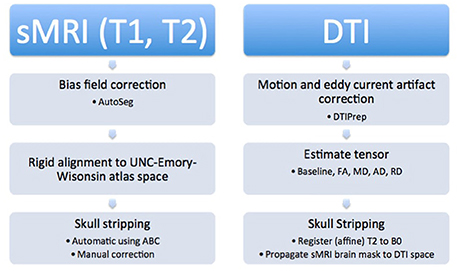
Figure 3. Structural images were processed first by performing bias field correction, followed by rigid alignment to the atlas. Additionally, automatic and manual skull stripping was done. The DTI images were corrected for motion and eddy current artifacts, tensors were calculated, and finally, the T2 weighted image was registered to the baseline image and the brain mask was applied to the diffusion data to complete skull stripping.
Structural MRI Pipeline
AutoSeg2 was used to perform the processing of the structural images. First, a bias field correction for the T1 and T2 images, respectively, was applied using the N4 method (Tustison et al., 2010). T1 images were aligned to an external T1 atlas (Emory-UNC atlas at 12 months) with rigid body registration (normalized mutual information based registration with BRAINSfit3). Then, the T2 images were aligned to their atlas-registered T1 images. As part of this realignment, the T1 and T2 images were re-sampled in atlas space to 0.2375 × 0.2375 × 0.2375 mm resolution. Atlas Based Classification4 (ABC) was applied to the registered images for expectation maximization based tissue classification (white matter, gray matter, cerebrospinal fluid, background) (Van Leemput et al., 1999). Tissue segmentation results were used to generate binary brain masks. Finally, all automatically generated brain masks were corrected manually by a human expert (JY).
Diffusion Imaging Pipeline
The DWI arrived at UNC NIRAL in the DICOM file format. These files were converted to the NRRD format using a tool known as DWIConverter5 that is a built-in module of 3D Slicer.6 3D Slicer is an open source software package for image visualization and analysis. The converter tool within 3D Slicer computes DWI based on the b-matrix information in the DICOM header.
Diffusion MRI quality control and motion and distortion correction was performed using our quality control (QC) tool, DTIPrep,7 that is also available as a built-in module of 3D Slicer (Liu et al., 2010; Oguz et al., 2014). This tool allows for image viewing and cropping, checking b-values and gradient vectors, noise filtering, evaluating correlation intensities to check for brightness and venetian blind artifacts, detection of vibration artifacts, and motion and eddy current artifact correction (Farzinfar et al., 2013). Motion and eddy current correction is done first for baseline (b = 0) images via a rigid transform based iterative average image computation over all baseline images, followed by affine registration of the diffusion weighted images to the final baseline average image. This QC step is done automatically using a protocol file containing all parameter settings for DTIPrep (Oguz et al., 2014; Verde et al., 2014). The images were examined visually after the automatic QC to exclude additional gradient directions that contained motion or artifacts. The average remaining gradient directions was 116 ± 11. There were no associations in gradient direction exclusion with respect to age or gender. Three subjects had partial acquisitions but were still included in the database for the interested parties. Once the visual QC was completed the DTI images were automatically generated from the corrected DWI data via weighted least square estimation.
3D Slicer was used to perform a visual QC on the corrected DTI images. This was done to verify the correct directional encoding, ensure an adequate signal-to-noise ratio (SNR), and perform fiducial tractography to verify the presence of all major tracts as described in Verde et al. (2014).
Skull stripping was performed by applying the brain mask created in the structural processing pipeline to the diffusion data. For each subject, its T2 weighted image was registered to the corresponding baseline image via standard normalized mutual information based affine registration, and the previously manually edited structural brain mask was propagated with this transformation to generate the DTI brain mask. This DTI brain mask was employed during the computation of the masked diffusion tensor data using weighted least squares fitting (3D Slicer external DTIProcess module).8 Eigenvalue maps (λ1, λ2, λ3) as well as diffusion property maps of fractional anisotropy (), mean diffusivity (), axial diffusivity (AD = λ1) and radial diffusivity () were computed from the masked DTI data.
Results
Using the methods outlined above, we were able to successfully obtain and process the serial images of brain development in rhesus monkeys. Both the structural and DTI data have been made freely available via the Girder repository.9 A representative example of the processed structural MRI data is shown below in Figure 4. All of the raw, unprocessed, structural and DWI data are also now available in this repository. The data can be downloaded in whole or in part using a standard secure FTP client, such as Transmit, Cyberduck, Filezilla, or the Unix or Mac command line utility.
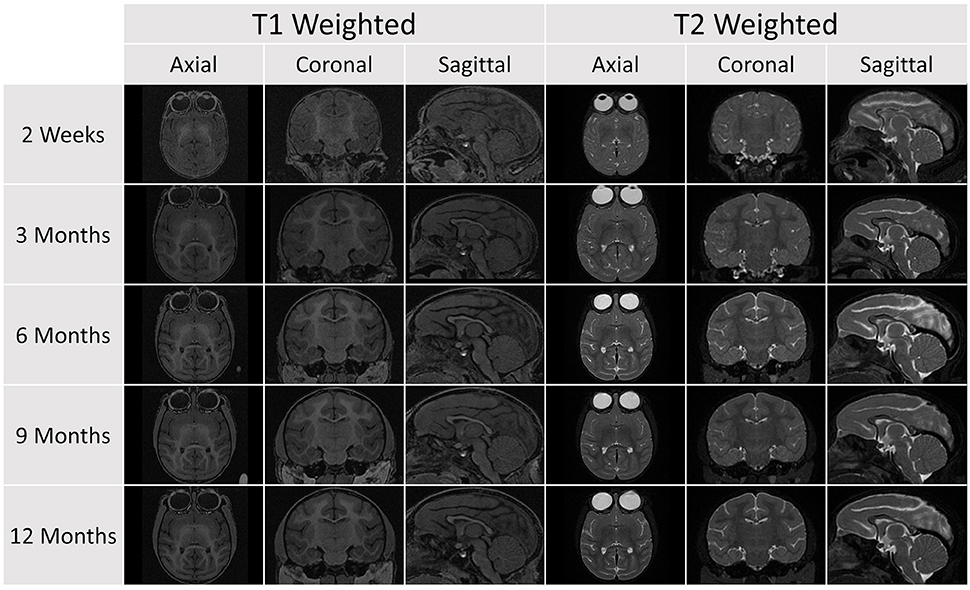
Figure 4. A representative sample of the serial images acquired for a single subject in the database (Subject 032). Each subject was scanned at five age points. At each time point, a T1 and T2 scan was obtained and the image above shows axial, coronal, and sagittal views for each scan sequence.
The structural scans required significant manual corrections after the automatic segmentation was applied. These manual corrections were performed by an expert rater (JY). A representative case of these manual corrections can be seen in Figure 5. Due to the rapid growth of the brain during the early stages of development there is a high degree of variation with regard to brain structure between subjects. Because of this population variance, automatic segmentation is less effective in younger subjects and all images of subjects younger than 6 months required significant manual corrections. For the subjects older than 6 months only minimal manual corrections were needed.
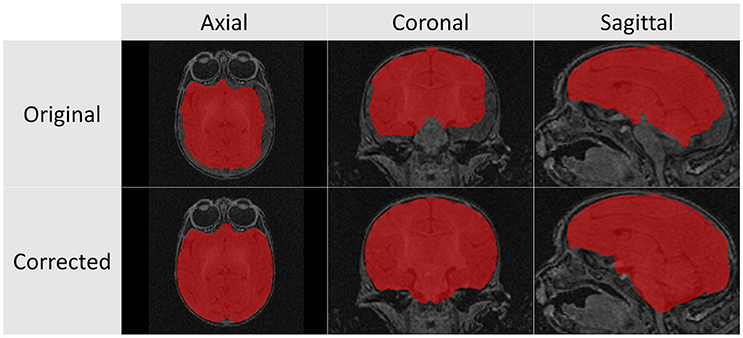
Figure 5. These T1 images illustrate the difference between the automatic segmentation (top) and the manual corrected results (bottom) when performed on scans of a 2 week infant monkey. Automatic segmentations on younger subjects required significantly more manual corrections in part due to poor tissue contrast.
After the diffusion scans were processed, diffusion property maps were generated for each subject. These maps included AD, RD, FA, and color by orientation. Figure 6 illustrates the property maps that were computed.
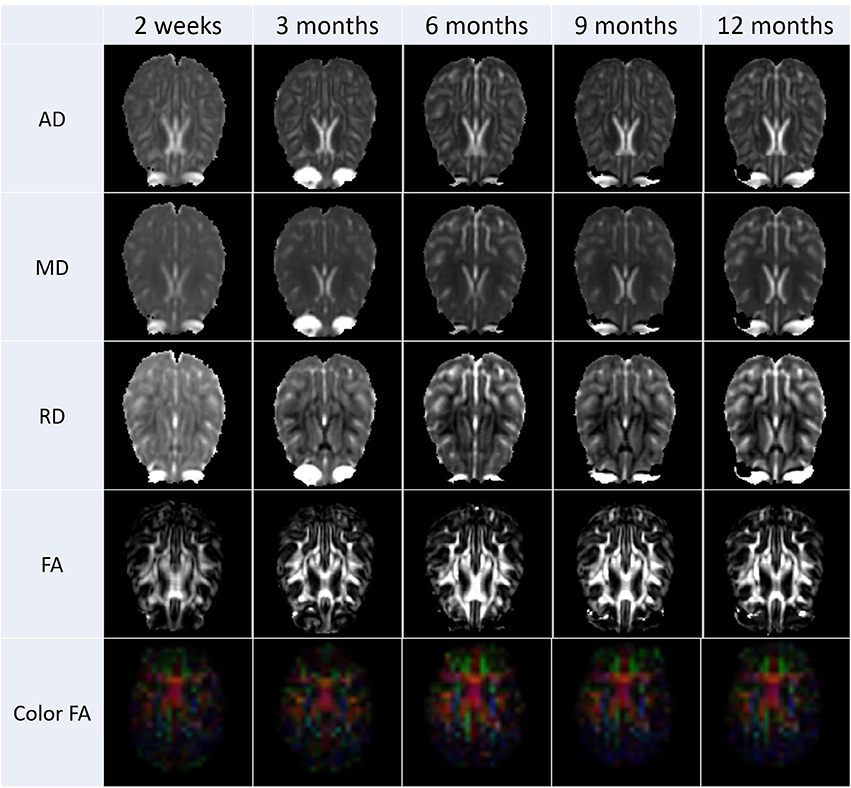
Figure 6. These images are an illustrative example the DTI results calculated from scans from all subjects. Property maps were generated at each scanning time point.
Discussion
This paper coincides with the public release of the processed data online. The data are available on Girder, an online data repository developed by Kitware (Figure 7). Prior to this release, the raw, preprocessed data were already available on Girder. These raw data have been downloaded 285 times since they were made available in April 2015. Further refinements to the UNC-Wisconsin Neurodevelopment Rhesus Database are still ongoing. In the future, age-specific brain atlases will be constructed at each time point using other derived data obtained from this study. These atlases will also be publicly disseminated for use by others. We also plan to perform full brain tractography and will release that data after validation and post-processing of the tracts.
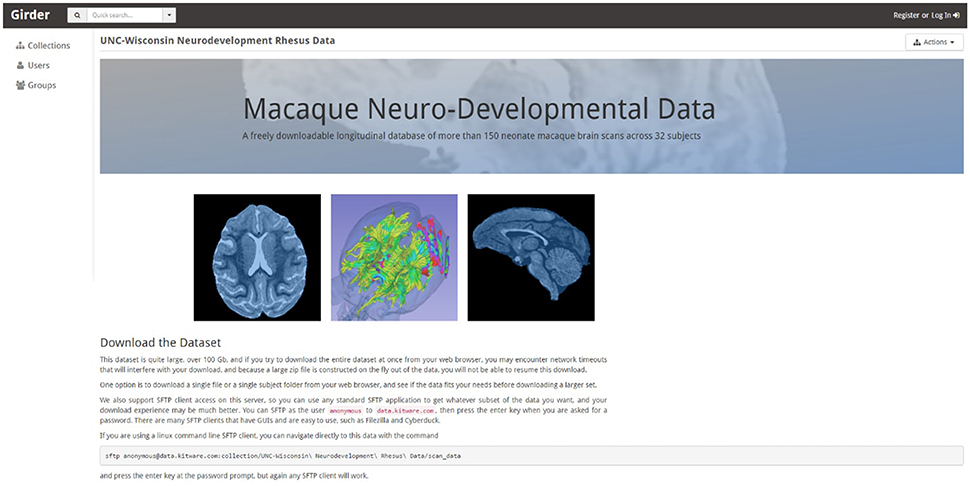
Figure 7. All data from this study is being made publically available and can be accessed from the homepage shown above. The data can be downloaded in whole or in part using a standard SFTP client. This repository will continue to be updated as we process the data.
This normative database provides a significant resource for the neuroimaging field and creates a foundation for investigations of abnormal development that may occur in the context of drug or teratogen exposure or following atypical rearing or nutrition conditions. The scan data from this project span two transitional periods likely to be critical in the etiology of mental illnesses. First, there is the period of rapid synaptic elaboration during infancy, the non-human primate equivalent of early childhood when neurodevelopmental conditions, such as autism spectrum disorder first present. Second, the scans of older juveniles and sub-adult monkeys in the 2–3 year old age range were obtained during the dynamic peripubertal period, when dendritic pruning occurs. Our data clearly demonstrate the emergence of significant sex differences in brain volumes and rate of maturation at this time. In humans, this stage is when gender differences in depression and eating disorders emerge, as well as when other psychopathologies like schizophrenia become more manifest. Our database provides both longitudinal and cross-sectional reference points against which to calibrate abnormal maturation during these vital points in neural development. It would be challenging to schedule an equivalent series of scans for children and adolescents, as well as to control all aspects of their diet and living conditions that can influence the maturing brain. These studies in humans are only now beginning, such as the baby connectome project which has just received funding. In addition, we were able to ensure the absence of prenatal and delivery complications, and exclude any monkey with significant clinical illness during the course of the study. We anticipate that this database will contribute to novel discoveries and look forward to seeing these data being productively incorporated into other analyses in the future.
Author Contributions
JY, YS performed the analysis and interpretation of the data. MN, RK contributed to the conception of the study. MG, FB, and BD contributed to the analysis of the data. CC, GL, and AA contributed to the conception of the study and the acquisition of the data. MS contributed to the conception of the study, analysis, and interpretation of the data. All authors contributed to the drafting and revising of the manuscript, as well as giving final approval for publication.
Conflict of Interest Statement
The authors declare that the research was conducted in the absence of any commercial or financial relationships that could be construed as a potential conflict of interest.
Acknowledgments
This research was supported by grants from the NIMH (MH901645, MH091645-S1, and MH100031) and the NICHD (HD003352, HD003110, and HD079124).
Footnotes
2. ^https://www.nitrc.org/projects/autoseg/
3. ^https://www.nitrc.org/projects/multimodereg/
4. ^https://www.nitrc.org/projects/abc
5. ^http://wiki.slicer.org/slicerWiki/index.php/Documentation/4.4/Modules/DWIConverter
7. ^http://www.nitrc.org/projects/dtiprep
References
Williams, R., Bokhari, S., Silverstein, P., Pinson, D., Kumar, A., and Buch, S. (2008). Non-human primate models of NeuroAIDS. J. Neurovirol. 14, 292–300. doi: 10.1080/13550280802074539
Allen, J. S., Damasio, H., Grabowski, T. J., Bruss, J., and Zhang, W. (2003). Sexual dimorphism and asymmetries in the gray-white composition of the human cerebrum. Neuroimage 18, 880–894. doi: 10.1016/S1053-8119(03)00034-X
Baird, G., Simonoff, E., Pickles, A., Chandler, S., Loucas, T., Meldrum, D., et al. (2006). Prevalence of disorders of the autism spectrum in a population cohort of children in South Thames: the Special Needs and Autism Project (SNAP). Lancet 368, 210–215. doi: 10.1016/S0140-6736(06)69041-7
Barr, C. S., and Goldman, D. (2006). Non-human primate models of inheritance vulnerability to alcohol use disorders. Addict. Biol. 11, 374–385. doi: 10.1111/j.1369-1600.2005.00034.x
Bennett, A. (2008). Gene environment interplay: non-human primate models in the study of resilience and vulnerability. Dev Psychobiol. 50, 48–59. doi: 10.1002/dev.20263
Bourgeois, J. P., and Rakic, P. (1993). Changes of synaptic density in the primary visual cortex of the macaque monkey from fetal to adult stage. J. Neurosci. 13, 2801–2820.
Caviness, V. S. Jr., Kennedy, D. N., Richelme, C., Rademacher, J., and Filipek, P. A. (1996). The human brain age 7-11 years: A volumetric analysis based on magnetic resonance images. Cereb. Cortex 6, 726–736. doi: 10.1093/cercor/6.5.726
Chakrabarti, S., and Fombonne, E. (2001). Pervasive developmental disorders in preschool children. JAMA 285, 3093–3099. doi: 10.1001/jama.285.24.3093
Courchesne, E., Chisum, H. J., Townsend, J., Cowles, A., Covington, J., Egaas, B., et al. (2000). Normal brain development and aging: quantitative analysis at in vivo MR imaging in healthy volunteers. Radiology 216, 672–682. doi: 10.1148/radiology.216.3.r00au37672
De Bellis, M. D., Keshavan, M. S., Beers, S. R., Hall, J., Frustaci, K., Masalehdan, A., et al. (2001). Sex differences in brain maturation during childhood and adolescence. Cereb. Cortex 11, 552–557. doi: 10.1093/cercor/11.6.552
Dekaban, A. S. (1978). Changes in brain weights during the span of human life: relation of brain weights to body heights and body weights. Ann. Neurol. 4, 345–356. doi: 10.1002/ana.410040410
Engert, F., and Bonhoeffer, T. (1999). Dendritic spine changes associated with hippocampal long-term synaptic plasticity. Nature 399, 66–70. doi: 10.1038/19978
Falk, D., Froese, N., Sade, D. S., and Dudek, B. C. (1999). Sex differences in brain/body relationships of Rhesus monkeys and humans. J. Hum. Evol. 36, 233–238. doi: 10.1006/jhev.1998.0273
Farzinfar, M., Oguz, I., Smith, R. G., Verde, A. R., Dietrich, C., Gupta, A., et al. (2013). Diffusion imaging quality control via entropy of principal direction distribution. Neuroimage 82, 1–12. doi: 10.1016/j.neuroimage.2013.05.022
Filipek, P. A. (1999). Neuroimaging in the developmental disorders: the state of the science. J. Child Psychol. Psychiatry 40, 113–128. doi: 10.1111/1469-7610.00426
Filipek, P. A., Richelme, C., Kennedy, D. N., and Caviness, V. S. Jr. (1994). The young adult human brain: An MRI-based morphometric analysis. Cereb. Cortex 4, 344–360. doi: 10.1093/cercor/4.4.344
Franklin, M. S., Kraemer, G. W., Shelton, S. E., Baker, E., Kalin, N. H., and Uno, H. (2000). Gender differences in brain volume and size of corpus callosum and amygdala of rhesus monkey measured from MRI images. Brain Res. 852, 263–267. doi: 10.1016/S0006-8993(99)02093-4
Giedd, J. N., Blumenthal, J., Jeffries, N. O., Castellanos, F. X., Liu, H., Zijdenbos, A., et al. (1999). Brain development during childhood and adolescence: a longitudinal MRI study. Nat. Neurosci. 2, 861–863. doi: 10.1038/13158
Giedd, J. N., Castellanos, F. X., Rajapakse, J. C., Vaituzis, A. C., and Rapoport, J. L. (1997). Sexual dimorphism of the developing human brain. Prog. Neuropsychopharmacol. Biol. Psychiatry 21, 1185–1201. doi: 10.1016/s0278-5846(97)00158-9
Giedd, J. N., Snell, J. W., Lange, N., Rajapakse, J. C., Casey, B. J., Kozuch, P. L., et al. (1996). Quantitative magnetic resonance imaging of human brain development: ages 4-18. Cereb. Cortex 6, 551–560. doi: 10.1093/cercor/6.4.551
Gilmore, J. H., Lin, W., Corouge, I., Vetsa, Y. S. K., Smith, J. K., Kang, C., et al. (2007a). Early postnatal development of corpus callosum and corticospinal white matter assessed with quantitative tractography. Am. J. Neuroradiol. 28, 1789–1795. doi: 10.3174/ajnr.A0751
Gilmore, J. H., Lin, W., Prastawa, M. W., Looney, C. B., Vetsa, Y. S. K., Knickmeyer, R. C., et al. (2007b). Regional gray matter growth, sexual dimorphism, and cerebral asymmetry in the neonatal brain. J. Neurosci. 27, 1255–1260. doi: 10.1523/JNEUROSCI.3339-06.2007
Glatzel, M., Pekarik, V., Lührs, T., Dittami, J., and Aguzzi, A. (2002). Analysis of the prion protein in primates reveals a new polymorphism in codon 226 (Y226F). Biol. Chem. 383, 1021–1025. doi: 10.1515/BC.2002.109
Goldstein, J. M., Seidman, L. J., Horton, N. J., Makris, N., Kennedy, D. N., Caviness, V. S. Jr., et al. (2001). Normal sexual dimorphism of the adult human brain assessed by in vivo magnetic resonance imaging. Cereb. Cortex 11, 490–497. doi: 10.1093/cercor/11.6.490
Good, C. D., Johnsrude, I., Ashburner, J., Henson, R. N., Friston, K. J., and Frackowiak, R. S. (2001). Cerebral asymmetry and the effects of sex and handedness on brain structure: a voxel-based morphometric analysis of 465 normal adult human brains. Neuroimage 14, 685–700. doi: 10.1006/nimg.2001.0857
Grant, K. A., and Bennett, A. J. (2003). Advances in non-human primate alcohol abuse and alcoholism research. Pharmacol. Ther. 100, 235–255. doi: 10.1016/j.pharmthera.2003.08.004
Gur, R. C., Turetsky, B. I., Matsui, M., Yan, M., Bilker, W., Hughett, P., et al. (1999). Sex differences in brain gray and white matter in healthy young adults: correlations with cognitive performance. J. Neurosci. 19, 4065–4072.
Gur, R. E., Petty, R. G., Turetsky, B. I., and Gur, R. C. (1996). Schizophrenia throughout life: Sex differences in severity and profile of symptoms. Schizophr. Res. 21, 1–12. doi: 10.1016/0920-9964(96)00023-0
Häfner, H., Maurer, K., Löffler, W., An Der Heiden, W., Munk-Jørgensen, P., Hambrecht, M., et al. (1998). The ABC Schizophrenia Study: a preliminary overview of the results. Soc. Psychiatry psychiatric Epidemiol. 33, 380–386.
Harlow, H. F., Harlow, M. K., and Suomi, S. J. (1971). From thought to therapy: lessons from a primate laboratory. Am. Sci. 59, 538–549.
Hunsaker, M. R., Scott, J. A., Bauman, M. D., Schumann, C. M., and Amaral, D. G. (2014). Postnatal development of the hippocampus in the Rhesus macaque (Macaca mulatta): a longitudinal magnetic resonance imaging study. Hippocampus 24, 794–807. doi: 10.1002/hipo.22271
Jernigan, T. L., and Tallal, P. (1990). Late childhood changes in brain morphology observable with MRI. Dev. Med. Child Neurol. 32, 379–385. doi: 10.1111/j.1469-8749.1990.tb16956.x
Knickmeyer, R. C., Gouttard, S., Kang, C., Evans, D., Wilber, K., Smith, J. K., et al. (2008). A structural MRI study of human brain development from birth to 2 years. J. Neurosci. 28, 12176–12182. doi: 10.1523/JNEUROSCI.3479-08.2008
Knickmeyer, R. C., Styner, M., Short, S. J., Lubach, G. R., Kang, C., Hamer, R., et al. (2010). Maturational trajectories of cortical brain development through the pubertal transition: unique species and sex differences in the monkey revealed through structural magnetic resonance imaging. Cereb. Cortex 20, 1053–1063. doi: 10.1093/cercor/bhp166
Kulkarni, J., Gurvich, C., Gilbert, H., Mehmedbegovic, F., Mu, L., Marston, N., et al. (2008). Hormone modulation: a novel therapeutic approach for women with severe mental illness. Aust. N. Z. J. Psychiatry 42, 83–88. doi: 10.1080/00048670701732715
Lacreuse, A., and Herndon, J. G. (2009). “Non-human primate models of cognitive aging,” in Animal Models of Human Cognitive Aging, eds J. L. Bizon and A. Woods (New York, NY: Humana Press). 29–59.
Lebel, C., Walker, L., Leemans, A., Phillips, L., and Beaulieu, C. (2008). Microstructural maturation of the human brain from childhood to adulthood. Neuroimage 40, 1044–1055. doi: 10.1016/j.neuroimage.2007.12.053
Lebherz, C., Maguire, A. M., Auricchio, A., Tang, W., Aleman, T. S., Wei, Z., et al. (2005). Non-human primate models for diabetic ocular neovascularization using AAV2-mediated overexpression of vascular endothelial growth factor. Diabetes 54, 1141–1149. doi: 10.2337/diabetes.54.4.1141
Lenroot, R. K., and Giedd, J. N. (2006). Brain development in children and adolescents: insights from anatomical magnetic resonance imaging. Neurosci. Biobehav. Rev. 30, 718–29. doi: 10.1016/j.neubiorev.2006.06.001
Lenroot, R. K., Gogtay, N., Greenstein, D. K., Wells, E. M., Wallace, G. L., Clasen, L. S., et al. (2007). Sexual dimorphism of brain developmental trajectories during childhood and adolescence. Neuroimage 36, 1065–1073. doi: 10.1016/j.neuroimage.2007.03.053
Liu, Z., Wang, Y., Gerig, G., Gouttard, S., Tao, R., Fletcher, T., et al. (2010). Quality control of diffusion weighted images. Proc. SPIE Int. Soc. Opt. Eng. 7628:76280J1-9. doi: 10.1117/12.844748
Lubach, G. R., and Coe, C. L. (2006). Preconception maternal iron status is a risk factor for iron deficiency in infant rhesus monkeys (Macaca mulatta). J. Nutr. 136, 2345–2349.
Machado, C. J., and Bachevalier, J. (2003). Non-human primate models of childhood psychopathology: the promise and the limitations. J. Child Psychol. Psychiatry 44, 64–87. doi: 10.1111/1469-7610.00103
Makris, N., Papadimitriou, G. M., van der Kouwe, A., Kennedy, D. N., Hodge, S. M., Dale, A. M., et al. (2007). Frontal connections and cognitive changes in normal aging rhesus monkeys: A DTI study. Neurobiol. Aging. 28, 1556–1567. doi: 10.1016/j.neurobiolaging.2006.07.005
Moffitt, T. E. (1990). Juvenile delinquency and attention deficit disorder: boys' developmental trajectories from age 3 to age 15. Child Dev. 61, 893–910. doi: 10.2307/1130972
Moffitt, T. E., and Caspi, A. (2001). Childhood predictors differentiate life-course persistent and adolescence-limited antisocial pathways among males and females. Dev. Psychopathol. 13, 355–375. doi: 10.1017/S0954579401002097
Nopoulos, P., Flaum, M., and Andreasen, N. C. (1997). Sex differences in brain morphology in schizophrenia. Am. J. Psychiatry 154, 1648–1654. doi: 10.1176/ajp.154.12.1648
Oguz, I., Farzinfar, M., Matsui, J., Budin, F., Liu, Z., Gerig, G., et al. (2014). DTIPrep: quality control of diffusion-weighted images. Front. Neuroinform. 8:4. doi: 10.3389/fninf.2014.00004
Payne, C., Machado, C. J., Bliwise, N. G., and Bachevalier, J. (2010). Maturation of the hippocampal formation and amygdala in Macaca mulatta: a volumetric magnetic resonance imaging study. Hippocampus 20, 922–935. doi: 10.1002/hipo.20688
Pfefferbaum, A., Mathalon, D. H., Sullivan, E. V., Rawles, J. M., Zipursky, R. B., and Lim, K. O. (1994). A quantitative magnetic resonance imaging study of changes in brain morphology from infancy to late adulthood. Arch. Neurol. 51, 874–887. doi: 10.1001/archneur.1994.00540210046012
Price, K. C., and Coe, C. L. (2000). Maternal constraint on fetal growth patterns in the rhesus monkey (Macaca mulatta): the intergenerational link between mothers and daughters. Hum. Reprod. 15, 452–457. doi: 10.1093/humrep/15.2.452
Rakic, P., Bourgeois, J. P., Eckenhoff, M. F., Zecevic, N., and Goldman-Rakic, P. S. (1986). Concurrent overproduction of synapses in diverse regions of the primate cerebral cortex. Science 232, 232–235. doi: 10.1126/science.3952506
Rakic, P., and Goldman-Rakic, P. S. (1982). The development and modifiability of the cerebral cortex. Overview. Neurosci. Res. Program Bull. 20, 433–438.
Reiss, A. L., Abrams, M. T., Singer, H. S., Ross, J. L., and Denckla, M. B. (1996). Brain development, gender and IQ in children. A volumetric imaging study. Brain 119, 1763–1774. doi: 10.1093/brain/119.5.1763
Schaefer, G. B., Thompson, J. N. Jr., Bodensteiner, J. B., Hamza, M., Tucker, R. R., Marks, W., et al. (1990). Quantitative morphometric analysis of brain growth using magnetic resonance imaging. J. Child Neurol. 5, 127–130. doi: 10.1177/088307389000500211
Scott, J. A., Grayson, D., Fletcher, E., Lee, A., Bauman, M. D., Schumann, C. M., et al. (2016). Longitudinal analysis of the developing rhesus monkey brain using magnetic resonance imaging: birth to adulthood. Brain Struct. Funct. 21, 2847–2871. doi: 10.1007/s00429-015-1076-x
Segerstrom, S. C., Lubach, G. R., and Coe, C. L. (2006). Identifying immune traits and biobehavioral correlates,:generalizability and reliability of immune responses in rhesus macaques. Brain Behav. Immun. 20, 349–358. doi: 10.1016/j.bbi.2005.09.007
Sowell, E. R., Thompson, P. M., Leonard, C. M., Welcome, S. E., Kan, E., and Toga, A. W. (2004). Longitudinal mapping of cortical thickness and brain growth in normal children. J. Neurosci. 24, 8223–8231. doi: 10.1523/JNEUROSCI.1798-04.2004
Stepanyants, A., Hof, P. R., and Chklovskii, D. B. (2002). Geometry and structural plasticity of synaptic connectivity. Neuron 34, 275–288. doi: 10.1016/S0896-6273(02)00652-9
Styner, M., Knickmeyer, R., Coe, C., Short, S. J., and Gilmore, J. (2008). “Automatic regional analysis of DTI properties in the developmental macaque brain,” in Proc. of SPIE Vol. 6914, Medical Imaging 2008: Image Processing, 69142K eds J. M. Reinhardt and J. P. W. Pluim (San Diego, CA). doi: 10.1117/12.772353
Sullivan, E. V., Sable, H. J. K., Strother, W. N., Friedman, D. P., Davenport, A., Tillman-Smith, H., et al. (2005). Neuroimaging of rodent and primate models of alcoholism: initial reports from the integrative neuroscience initiative on alcoholism. Alcohol. Clin. Exp. Res. 29, 287–294. doi: 10.1097/01.alc.0000153546.39946.ec
Szatmari, P., Offord, D. R., and Boyle, M. H. (1989). Ontario child health study: prevalence of attention deficit disorder with hyperactivity. J. Child Psychol. Psychiatry 30, 219–230. doi: 10.1111/j.1469-7610.1989.tb00236.x
Tustison, N. J., Avants, B. B., Cook, P. A., Zheng, Y., Egan, A., Yushkevich, P. A., et al. (2010). N4ITK: Improved N3 bias correction. IEEE Trans. Med. Imaging 29, 1310–1320. doi: 10.1109/TMI.2010.2046908
Van Leemput, K., Maes, F., Vandermeulen, D., and Suetens, P. (1999). Automated model-based tissue classification of MR images of the brain. IEEE Trans. Med. Imaging 18, 885–896. doi: 10.1109/42.811268
Verde, A. R., Budin, F., Berger, J.-B., Gupta, A., Farzinfar, M., Kaiser, A., et al. (2014). UNC-Utah NA-MIC framework for DTI fiber tract analysis. Front. Neuroinform. 7:51. doi: 10.3389/fninf.2013.00051
Keywords: neuroimaging, non-human primate, macaque, computational atlases, magnetic resonance imaging, diffusion tensor imaging, brain development
Citation: Young JT, Shi Y, Niethammer M, Grauer M, Coe CL, Lubach GR, Davis B, Budin F, Knickmeyer RC, Alexander AL and Styner MA (2017) The UNC-Wisconsin Rhesus Macaque Neurodevelopment Database: A Structural MRI and DTI Database of Early Postnatal Development. Front. Neurosci. 11:29. doi: 10.3389/fnins.2017.00029
Received: 22 September 2016; Accepted: 16 January 2017;
Published: 02 February 2017.
Edited by:
Maxime Descoteaux, Université de Sherbrooke, CanadaReviewed by:
Laurent Petit, French National Centre for Scientific Research (CNRS), FranceBassem Hiba, French National Centre for Scientific Research (CNRS), France
Copyright © 2017 Young, Shi, Niethammer, Grauer, Coe, Lubach, Davis, Budin, Knickmeyer, Alexander and Styner. This is an open-access article distributed under the terms of the Creative Commons Attribution License (CC BY). The use, distribution or reproduction in other forums is permitted, provided the original author(s) or licensor are credited and that the original publication in this journal is cited, in accordance with accepted academic practice. No use, distribution or reproduction is permitted which does not comply with these terms.
*Correspondence: Martin A. Styner, styner@cs.unc.edu