- 1 School of Medicine, University of Western Sydney, Penrith, NSW, Australia
- 2 Neuroscience Research Australia, Sydney, NSW, Australia
Postganglionic sympathetic axons in awake healthy human subjects, regardless of their identity as muscle vasoconstrictor, cutaneous vasoconstrictor, or sudomotor neurons, discharge with a low firing probability (∼30%), generate low firing rates (∼0.5 Hz) and typically fire only once per cardiac interval. The purpose of the present study was to use modeling of spike trains in an attempt to define the number of preganglionic neurons that drive an individual postganglionic neuron. Artificial spike trains were generated in 1–3 preganglionic neurons converging onto a single postganglionic neuron. Each preganglionic input fired with a mean interval distribution of either 1000, 1500, 2000, 2500, or 3000 ms and the SD varied between 0.5×, 1.0×, and 2.0× the mean interval; the discharge frequency of each preganglionic neuron exhibited positive skewness and kurtosis. Of the 45 patterns examined, the mean discharge properties of the postganglionic neuron could only be explained by it being driven by, on average, two preganglionic neurons firing with a mean interspike interval of 2500 ms and SD of 5000 ms. The mean firing rate resulting from this pattern was 0.22 Hz, comparable to that of spontaneously active muscle vasoconstrictor neurons in healthy subjects (0.40 Hz). Likewise, the distribution of the number of spikes per cardiac interval was similar between the modeled and actual data: 0 spikes (69.5 vs 66.6%), 1 spike (25.6 vs 21.2%), 2 spikes (4.3 vs 6.4%), 3 spikes (0.5 vs 1.7%), and 4 spikes (0.1 vs 0.7%). Although some features of the firing patterns could be explained by the postganglionic neuron being driven by a single preganglionic neuron, none of the emulated firing patterns generated by the firing of three preganglionic neurons matched the discharge of the real neurons. These modeling data indicate that, on average, human postganglionic sympathetic neurons are driven by two preganglionic inputs.
Introduction
Microelectrode recordings from peripheral nerves of awake human subjects have revealed common features in their discharge patterns of individual postganglionic sympathetic axons, including a probabilistic firing pattern, a tendency to fire only one spike per cardiac interval, an exponential distribution of instantaneous frequencies, and low mean firing rate (∼0.5 Hz). These features are shared across the unit types that have so far been studied: muscle vasoconstrictor (Macefield et al., 1994; Macefield and Wallin, 1999a), cutaneous vasoconstrictor (Macefield and Wallin, 1999b), and sudomotor (Macefield and Wallin, 1996) neurons in healthy subjects (for review see Macefield et al., 2002). Moreover, although the firing probability and mean firing rates of muscle vasoconstrictor neurons have been shown to be elevated in various pathophysiological states, including congestive heart failure (Macefield et al., 1999; Elam and Macefield, 2001), obstructive sleep apnea (Elam et al., 2002), chronic obstructive pulmonary disease (Ashley et al., 2010), essential hypertension (Schlaich et al., 2004), panic disorder (Lambert et al., 2006), and obesity (Lambert et al., 2007), individual neurons still tend to fire only once per cardiac interval.
Why is this so? We know that postganglionic neurons can fire multiple times in a sympathetic burst, though usually no more than four spikes per cardiac interval, and have observed that there is a shift toward fewer solitary spikes and more multiple firing in obstructive sleep apnea (Elam et al., 2002), chronic obstructive pulmonary disease (Ashley et al., 2010), panic disorder (Lambert et al., 2006), and hyperhidrosis (Macefield et al., 2008), but the biggest shift was observed during the ectopic beats associated with heart failure (Elam and Macefield, 2001). We have argued previously, based on a comparison of the firing properties of postganglionic sympathetic neurons and alpha motoneurons, that the duration of a sympathetic burst would limit the number of times a neuron can fire (Macefield and Elam, 2004), but we cannot escape the fact that the firing pattern is determined by the level of synaptic drive a given postganglionic neuron receives.
McLachlan et al. (1997) have shown that approximately one-third of postganglionic neurons in the superior cervical ganglion of the anesthetized rat receive convergent inputs from two or more preganglionic neurons with strong synapses, the majority receiving only one strong input. Similar results have been shown for lumbar postganglionic neurons (Bratton et al., 2010). Given the probabilistic nature in which human postganglionic neurons fire, and that most can intermittently generate spike doublets or triplets with very high instantaneous frequencies (>100 Hz), we have argued that this reflects the near-coincident firing of at least two preganglionic neurons possessing strong synaptic coupling with an individual postganglionic neuron (Macefield et al., 2002). The purpose of the present study was to test the hypothesis that human postganglionic neurons are indeed driven by, on average, the inputs from two preganglionic neurons. To this end model spike trains in one to three preganglionic neurons converging onto a single model postganglionic neuron were combined in order to generate a pattern that matched the actual firing patterns of postganglionic sympathetic neurons.
Methods
Artificial spike trains were generated using the Spike Generator feature in LabChart 7 software (ADInstruments, Sydney, Australia). For each series three “preganglionic neurons” were set up to fire spontaneously with a mean interval distribution of either 1000, 1500, 2000, 2500, or 3000 ms and the data recorded on computer. The distribution was randomly generated and for each set the SD was varied between 0.5×, 1.0×, and 2.0× the mean interval. The discharge frequency of each preganglionic neuron exhibited positive skewness and kurtosis. For each stimulation pattern, i.e., a given mean interval and SD, the “postganglionic neuron” was driven by one, two, or three preganglionic neurons. Accordingly, the total number of firing patterns examined was 45. The amplitude of each preganglionic neuron was set at 1 V; for illustration purposes, background Gaussian noise (0.1 V) was added to the resultant postganglionic spike train to emulate the appearance of a real recording. In order to discriminate each unit using the Spike Histogram module in LabChart 7, the spike duration of unit 1 was set at 0.5 ms, unit 2 at 1 ms, and unit 3 at 1.5 ms. Accordingly, the firing of the “postganglionic neuron” could be configured to represent the spike train input from one, two, or three preganglionic neurons on the basis of spike duration. Spikes trains were recorded for 1020–1085 s. Spikes were sampled over 1 s every second – this represents the average cardiac interval of a subject with a mean heart rate of 60 beats/min. Comparison data were provided by combining data from our previously published unitary recordings from healthy subjects with low and high levels of muscle sympathetic nerve activity (Macefield et al., 1994; Macefield and Wallin, 1999a). The mean heart rate of the subjects from whom these combined data were obtained was 58 beats/min, similar to that of the artificially generated data. Numerical analysis was performed using Prism 5 (GraphPad Software Inc., USA). Mean firing rate was computed from the inverse of the mean interval, in order to match the method used in the analysis of unitary recordings from actual sympathetic neurons (Macefield et al., 1994).
Results
Using a variety of model spike train patterns, attempts were made to model the discharge of individual muscle vasoconstrictor neurons by combining the stochastic firing patterns of one to three input neurons (preganglionic neurons) converging onto a single output neuron (postganglionic neuron). The lower trace in Figure 1A shows a representative recording of an artificially generated spike train in a “postganglionic neuron,” together with the spike trains from two of the three “preganglionic neurons,” units 1 and 2, that converged onto this model output neuron. Note the variation in spike amplitude of the modeled postganglionic neuron, due to the underlying noise on which each standard spike is riding. Although the overall discharge frequency was low, it can be seen that intermittent high-frequency discharges can be observed: these are generated by the chance occurrence of spikes from the two preganglionic neurons converging onto the postganglionic neuron. Figure 1B shows the firing pattern when all three input neurons were driving the output neuron. Increasing the number of active preganglionic neurons increased the overall firing probability (and mean firing rate) of the postganglionic neuron, the incidence of intermittent high instantaneous frequencies also increasing.
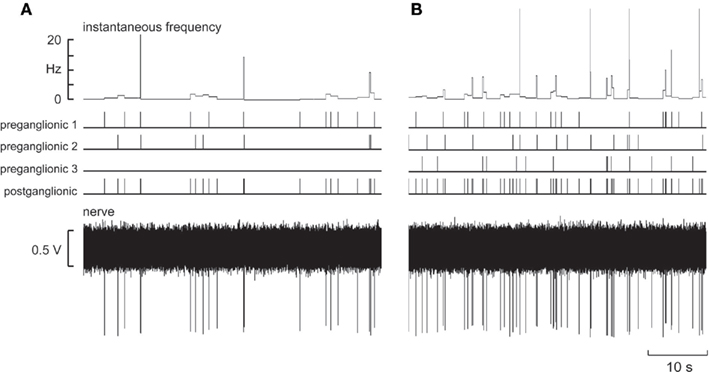
Figure 1. Model spike trains from two (A) or three (B) “preganglionic neurons” that converged onto a model “postganglionic neuron.” The lower trace shows the emulated nerve recording. The mean interspike interval was 2500 ± 5000 ms (SD).
Figure 2 shows the distribution of spikes per “cardiac interval” (1 s), calculated when one, two, or three model preganglionic neurons – discharging with a mean interspike interval of 1000 ms and a SD of 500 ms – were driving a single model postganglionic neuron. Figure 2F shows the distribution of real spikes recorded from 33 muscle vasoconstrictor neurons in healthy subjects. It is readily apparent that none of the patterns illustrated in Figures 2A–E matched the firing pattern of the real neurons: when a single preganglionic neuron was active (Figures 2A–C) the overall firing probability was too high (i.e., there were fewer cardiac intervals in which the neuron was silent) and when two or more preganglionic neurons were active the incidence of multiple firing was too high. Moreover, the mean firing rates were higher than those recorded from the real neurons. The same was true when the SD was 1× and 2× the mean interspike interval.
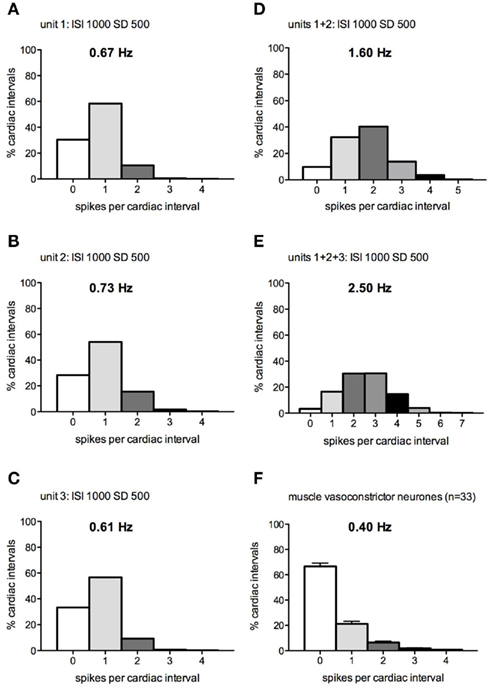
Figure 2. (A–E) Spike interval distributions for a model postganglionic neuron generating 0–4 spikes per “cardiac interval” (1 s), while being driven by 1–3 model preganglionic neurons discharging at a mean interspike interval of 1000 ± 500 ms. (F) Spike distribution of real muscle vasoconstrictor neurons (data pooled from Macefield et al., 1994; Macefield and Wallin, 1999a).
At the other extreme, Figures 3A–E shows the spike distributions calculated when one, two, or three preganglionic neurons – each firing with a mean interspike interval of 2500 ms and SD of 5000 ms – were driving the postganglionic neuron on which they converged. For the majority of intervals, the postganglionic neuron was silent: 83% of intervals when only one input neuron was active, 70% when two were active, and 57% when three input neurons were active. As the number of silent cardiac intervals decreased with an increase in the number of active preganglionic neurons, so the number of spikes generated within each cardiac interval increased. It is apparent that the distribution of the number of spikes per cardiac interval was similar between the modeled and actual data shown in Figure 3F: 0 spikes (69.5 vs 66.6%), 1 spike (25.6 vs 21.2%), 2 spikes (4.3 vs 6.4%), 3 spikes (0.5 vs 1.7%), and 4 spikes (0.1 vs 0.7%). The mean discharge interval from this pattern was 2314 ± 1453 (SD) s, corresponding to a mean firing rate of 0.22 Hz, comparable to (but somewhat lower than) that of the population of spontaneously active muscle vasoconstrictor neurons recorded in healthy subjects (0.40 Hz).
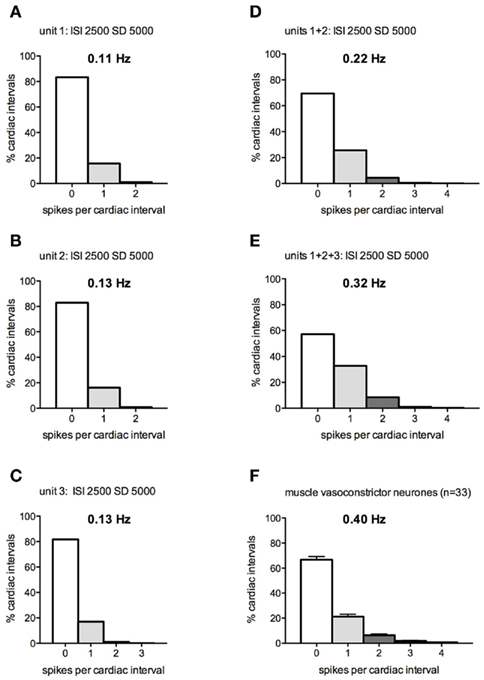
Figure 3. (A–E) Spike interval distributions for a postganglionic neuron generating 0–4 spikes per “cardiac interval” (1 s), while being driven by 1–3 model preganglionic neurons discharging at a mean interspike interval of 2500 ± 5000 ms. (F) Spike distribution of real muscle vasoconstrictor neurons (data pooled from Macefield et al., 1994; Macefield and Wallin, 1999a).
Table 1 shows the spike distributions for the model postganglionic neurons driven by one, two, or three model preganglionic neurons, each firing with a mean interspike interval of 1000, 1500, 2000, or 2500 ms and SDs of 0.5×, 1.0×, or 2.0× that of the mean interval. With the exception of some that were greater than 1 Hz, the mean firing rates of the model postganglionic neurons were all within the physiological range recorded from real muscle vasoconstrictor neurons. And while some of the patterning could be partially explained by a postganglionic neuron being driven by one preganglionic neuron exclusively (see asterisks in Table 1), there were no firing distributions generated by the summed inputs of all three preganglionic neurons that matched those of the real muscle vasoconstrictor neurons. Indeed, of the 45 patterns examined, the mean discharge properties of the model postganglionic neuron could best be explained by it being driven by – on average – two preganglionic neurons firing with a mean interspike interval of 2500 ms and SD of 5000 ms (Figure 3). Table 1 also shows that for all patterns the incidence of short interspike intervals was very low; intervals ≤20 ms typically occurred <2% of the time.
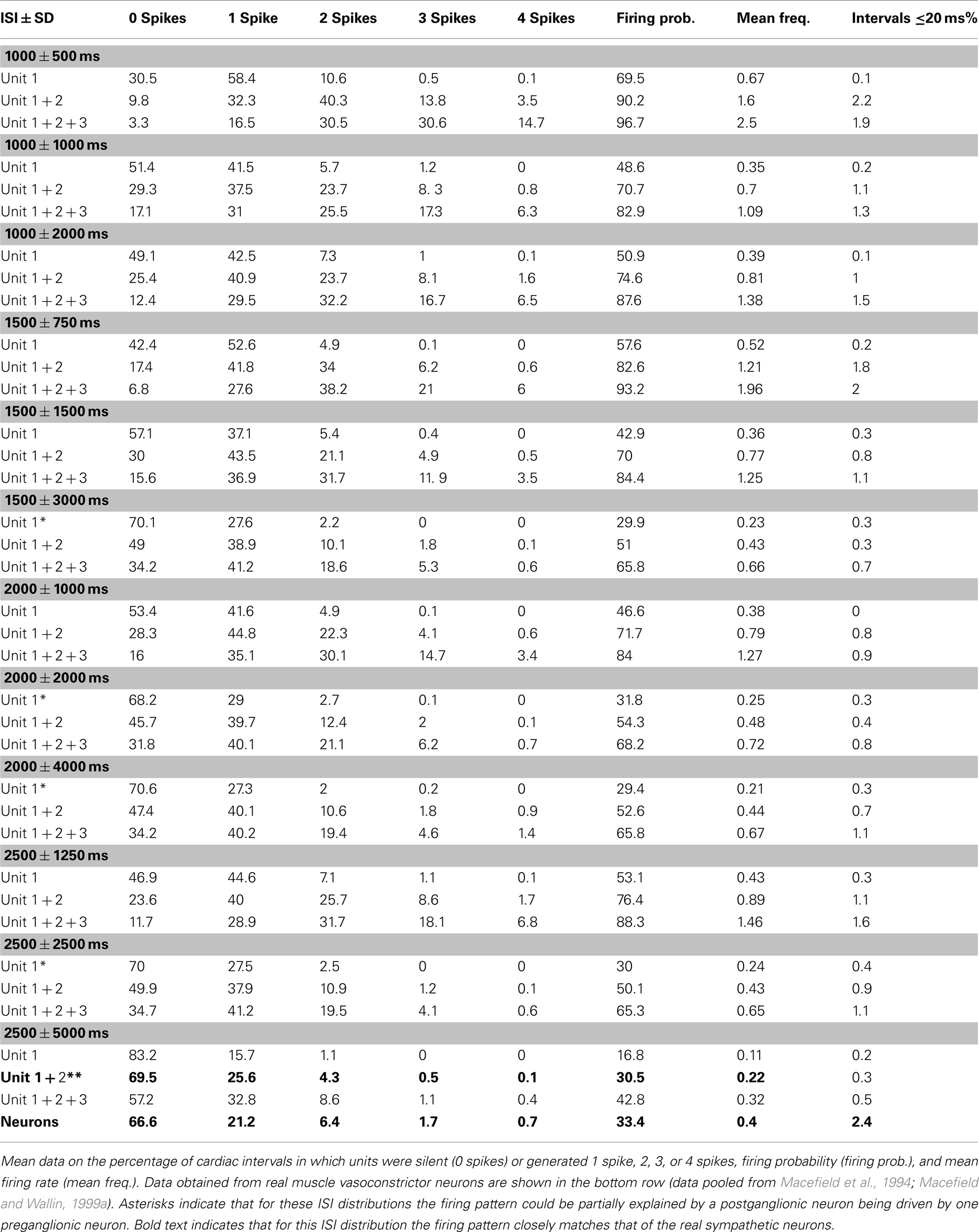
Table 1. Firing patterns of model postganglionic neurons driven by 1, 2, or 3 model preganglionic neurons with differing interspike interval (ISI) distributions.
Discussion
This study has shown that the characteristic firing pattern of postganglionic muscle vasoconstrictor neurons recorded from awake healthy human subjects can be emulated by a limited set of patterns of firing in the preganglionic neurons. Obviously, it is not possible to directly record from preganglionic neurons in awake human subjects, which would require inserting a microelectrode into the intermediolateral column of the spinal cord or the white rami that connect the cord to the sympathetic chain. Accordingly, this modeling study was an attempt to increase our understanding of how the sympathetic nervous system grades its output, given that the discharge properties of postganglionic neurons are remarkably consistent in healthy subjects (Macefield et al., 2002). A similar modeling approach had been used by McLachlan et al. (1998) to compare the firing of simulated postganglionic neurons and real postganglionic neurons recorded from the superior cervical ganglion in the rat.
Of the 45 spike emulation patterns examined, the discharge properties of a typical postganglionic muscle vasoconstrictor neuron could best be explained by it being driven by, on average, two preganglionic neurons firing with a mean interspike interval of 2500 ms and SD of 5000 ms: firing probability and the numbers of spikes generated within a cardiac interval matched those of spontaneously active muscle vasoconstrictor neurons; mean firing rate was also in the physiological range (Macefield et al., 1994; Macefield and Wallin, 1999a). Moreover, given that the firing patterns are similar for cutaneous vasoconstrictor and sudomotor neurons in healthy subjects exhibiting cold-induced cutaneous vasoconstriction (Macefield and Wallin, 1999b) or heat-induced sweating (Macefield and Wallin, 1996), the current modeling data can also explain the discharge properties of these neurons. It should be pointed out that some postganglionic neurons comprising the population of real neurons recorded do discharge with a pattern that closely reflects the input from a single preganglionic neuron, but none discharged with a pattern that reflected the combined inputs from three preganglionic neurons. Moreover, individual neurons vary in their firing probabilities, with some having low and some high firing probabilities. Such patterns have also been observed when using wavelet decomposition techniques to extract the firing of individual spikes comprising a multiunit recording (Steinback et al., 2010; Salmanpour et al., 2011). Nevertheless, on average, one can conclude that the population behavior is of each postganglionic neuron being driven, on average, by two preganglionic neurons. This then provides us with an upper limit as to how many preganglionic neurons are responsible for the generation of action potentials in a single postganglionic neuron: two.
We know from studies in the anesthetized rat that some postganglionic neurons in the superior cervical ganglion, which supply many different tissues, are driven by two (rarely three) preganglionic neurons with “strong” synapses, but that most are driven by only one (McLachlan et al., 1997, 1998; Li and Horn, 2006; Rimmer and Horn, 2010). Moreover, close temporal coincidence of EPSPs from two preganglionic neurons with “weak” synapses can bring the postganglionic neuron to threshold (Rimmer and Horn, 2010). Like those in the superior cervical ganglion, most postganglionic neurons recorded from a lumbar sympathetic ganglion in the rat – the majority of which are vasoconstrictor in function – are driven by one “primary” preganglionic neuron, but inputs from two (or rarely three) “secondary” preganglionic neurons can occasionally contribute to the firing of a single postganglionic neuron (Bratton et al., 2010). The current modeling study suggests that the synaptic organization of postganglionic neurons in awake human subjects is similar to that seen in the anesthetized rat. However, it should be noted that the studies of ganglionic transmission in the superior cervical ganglion (McLachlan et al., 1997, 1998) were made under conditions in which the postganglionic neurons were hyperpolarized, which may have blocked the production of action potentials from “weak inputs,” whereas the studies conducted in a lumbar sympathetic ganglion (Bratton et al., 2010) were made at the resting membrane potential. Nevertheless, both sets of studies were undertaken in very reduced preparations, and the influence of anesthesia may well have affected the underlying firing properties of both the preganglionic neurons and the postganglionic neurons to which they project. Despite these differences between the rat and human studies, it would appear that the underlying ganglionic neurophysiology is remarkably similar.
If human postganglionic neurons are driven by, on average, two preganglionic neurons then this fits with our interpretation of how short “spike doublets” are generated in muscle vasoconstrictor, cutaneous vasoconstrictor, and sudomotor neurons – by the chance occurrence of temporally coincident action potentials in the preganglionic neurons that synapse onto a given postganglionic neuron (Macefield et al., 2002). Indeed, we had argued that this observation alone suggests that each postganglionic neuron is driven by two preganglionic neurons, so the current modeling study lends credence to this interpretation. Importantly, we had noted previously that “although the generation of high instantaneous frequencies requires an increase in synaptic drive to the neuron, these events were usually not associated with large bursts – rather they occurred essentially randomly” (Macefield et al., 2002).
Because our model preganglionic neurons were set up to fire stochastically, there were no constraints as to whether the discharge of two or three closely firing neurons could influence a postganglionic neuron. Physiologically, of course, the capacity of a postganglionic neuron to follow excitatory postsynaptic potentials and generate action potentials is limited by the absolute refractory period of the neuron (∼2 ms); indeed, our recordings from single postganglionic neurons occasionally revealed instantaneous frequencies of 400 Hz, approaching the theoretical limit of 500 Hz (Macefield et al., 1994, 2002; Macefield and Wallin, 1996, 1999a,b). The model neurons could generate frequencies that were artificially higher than this, but could also generate intermittent peak instantaneous frequencies that were similar to those recorded from real neurons. Nevertheless, the incidence of short interspike intervals (≤20 ms) was very low, typically < 2%.
Limitations
There were several assumptions made in this study. First, the discharge of the model preganglionic neurons was composed of randomly generated interspike intervals, whereas we know that individual muscle vasoconstrictor neurons are constrained by the arterial baroreceptors to fire with a tight cardiac rhythmicity, and that even cutaneous vasoconstrictor and sudomotor neurons do exhibit some cardiac rhythmicity (Macefield and Wallin, 1996, 1999b). Nevertheless, like the real neurons, the distribution of firing rates was exponential: it was positively skewed with a positive kurtosis (Macefield et al., 2002). In many respects, the randomly occurring spikes largely mimicked that of actual postganglionic neurons, the firing of which appears to be probabilistic in nature. We know that the interspike intervals of neurons in the superior cervical ganglion of the anesthetized rat are exponentially distributed, that bursts of impulses occur through chance, and that cardiac and respiratory rhythms can entrain the discharge (McLachlan et al., 1998). Second, as noted above, there were no biophysical constraints as to the shortest interspike interval, but given that these were rare I don’t believe this has a major impact on the interpretation. Third, it is assumed that the presynaptic neurons are exclusively excitatory – dominated by “strong” or “primary” inputs in which there is faithful synaptic security between a given preganglionic neuron and the postganglionic neuron it supplies. And fourth, the mean firing rate of each of the model preganglionic neurons was equal: this is not unreasonable, but assumes that the descending excitatory drive from the medulla is distributed uniformly within the pool of preganglionic neurons in the spinal cord.
Conclusion
Not withstanding these limitations, on the basis of modeling the firing properties of preganglionic neurons and comparing these with the firing of real postganglionic neurons it is concluded that the firing properties of single muscle vasoconstrictor neurons recorded in awake human subjects appear to be an emergent property of the probabilistic discharge of – on average – two preganglionic neurons converging onto the one postganglionic neuron. None of the firing patterns generated by the convergence of three preganglionic neurons matched the discharge of the real postganglionic neurons.
Conflict of Interest Statement
The author declares that the research was conducted in the absence of any commercial or financial relationships that could be construed as a potential conflict of interest.
References
Ashley, C., Burton, D., Sverrisdottir, Y. B., Sander, M., McKenzie, D. K., and Macefield, V. G. (2010). Firing probability and mean firing rates of human muscle vasoconstrictor neurones are elevated during chronic asphyxia. J. Physiol. 588, 701–711.
Bratton, B., Davies, P., Jänig, W., and McAllen, R. (2010). Ganglionic transmission in a vasomotor pathway studied in vivo. J. Physiol. 588, 1647–1659.
Elam, M., and Macefield, V. G. (2001). Multiple firing of single muscle vasoconstrictor neurons during cardiac dysrhythmias in human heart failure. J. Appl. Physiol. 91, 717–724.
Elam, M., Macefield, V. G., and McKenzie, D. (2002). The firing pattern of single muscle vasoconstrictor neurons in awake patients with the obstructive sleep apnoea syndrome. J. Appl. Physiol. 93, 297–303.
Lambert, E., Hotchkin, E., Alvarenga, M., Pier, C., Richards, J., Barton, D., Dawood, T., Esler, M., and Lambert, G. (2006). Single-unit analysis of sympathetic nervous discharges in patients with panic disorder. J. Physiol. 570, 637–643.
Lambert, E., Straznicky, N., Schlaich, M., Esler, M., Dawood, T., Hotchkin, E., and Lambert, G. (2007). Differing pattern of sympathoexcitation in normal-weight and obesity-related hypertension. Hypertension 50, 862–868.
Li, C., and Horn, J. P. (2006). Physiological classification of sympathetic neurons in the rat superior cervical ganglion. J. Neurophysiol. 95, 187–195.
Macefield, V. G., and Elam, M. (2004). Comparison of the firing patterns of human postganglionic sympathetic neurones and spinal α motoneurones during brief bursts. Exp. Physiol. 89, 82–88.
Macefield, V. G., Elam, M., and Wallin, B. G. (2002). Firing properties of single postganglionic sympathetic neurones recorded in awake human subjects. Auton. Neurosci. 95, 146–159.
Macefield, V. G., Rundqvist, B., Sverrisdottir, Y. B., Wallin, B. G., and Elam, M. (1999). Firing properties of single muscle vasoconstrictor neurons in the sympathoexcitation associated with congestive heart failure. Circulation 100, 1708–1713.
Macefield, V. G., Sverrisdottir, Y. B., Elam, E., and Harris, J. (2008). Firing properties of sudomotor neurones in hyperhidrosis and thermal sweating. Clin. Auton. Res. 18, 325–330.
Macefield, V. G., and Wallin, B. G. (1996). Discharge behaviour of single sympathetic neurones innervating human sweat glands. J. Auton. Nerv. Syst. 61, 277–286.
Macefield, V. G., and Wallin, B. G. (1999a). Firing properties of single vasoconstrictor motoneurones in subjects with high levels of muscle sympathetic activity. J. Physiol. 516, 293–301.
Macefield, V. G., and Wallin, B. G. (1999b). Respiratory and cardiac modulation of single vasoconstrictor and sudomotor neurones to human skin. J. Physiol. 516, 303–314.
Macefield, V. G., Wallin, B. G., and Vallbo, Å. B. (1994). The discharge behaviour of single vasoconstrictor motoneurones in human muscle nerves. J. Physiol. 481, 799–809.
McLachlan, E. M., Davies, P. J., Häbler, H. J., and Jamieson, J. (1997). On-going and reflex synaptic events in rat superior cervical ganglion cells. J. Physiol. 501, 165–182.
McLachlan, E. M., Habler, H. J., Jamieson, J., and Davies, P. J. (1998). Analysis of the periodicity of synaptic events in neurones in the superior cervical ganglion of anaesthetized rats. J. Physiol. (Lond.) 511, 461–478.
Rimmer, K., and Horn, J. P. (2010). Weak and straddling secondary nicotinic synapses can drive firing in rat sympathetic neurons and thereby contribute to ganglionic amplification. Front. Neurol. 1:130. doi:10.3389/fneur.2010.00130
Salmanpour, A., Brown, L. J., Steinback, C. D., Usselman, C. W., Goswami, R., and Shoemaker, J. K. (2011). Relationship between size and latency of action potentials in human muscle sympathetic nerve activity. J. Neurophysiol. 105, 2830–2842.
Schlaich, M. P., Lambert, E., Kaye, D. M., Krozowski, Z., Campbell, D. J., Lambert, G., Hastings, J., Aggarwal, A., and Esler, M. D. (2004). Sympathetic augmentation in hypertension: role of nerve firing, norepinephrine reuptake, and angiotensin neuromodulation. Hypertension 43, 169–175.
Keywords: sympathetic nervous system, human, preganglionic neuron, postganglionic neuron, single-unit, microneurography
Citation: Macefield VG (2011) On the number of preganglionic neurons driving human postganglionic sympathetic neurons: a comparison of modeling and empirical data. Front. Neurosci. 5:132. doi: 10.3389/fnins.2011.00132
Received: 02 October 2011;
Accepted: 14 November 2011;
Published online: 06 December 2011.
Edited by:
Stuart Mazzone, University of Queensland, AustraliaReviewed by:
Elisabeth Lambert, Baker IDI Heart and Diabetes Institute, AustraliaAnthony (Tony) E. Pickering, University of Bristol, UK
J. Kevin Shoemaker, The University of Western Ontario, Canada
Copyright: © 2011 Macefield. This is an open-access article distributed under the terms of the Creative Commons Attribution Non Commercial License, which permits non-commercial use, distribution, and reproduction in other forums, provided the original authors and source are credited.
*Correspondence: Vaughan G. Macefield, School of Medicine, University of Western Sydney, Locked Bag 1797, Penrith, NSW 2751, Australia. e-mail:di5tYWNlZmllbGRAdXdzLmVkdS5hdQ==