- Department of Comparative Psychology, Institute of Experimental Psychology, Heinrich-Heine University Düsseldorf, Düsseldorf, Germany
Despite the rich tradition in psychology and biology, animals as research subjects have never gained a similar acceptance in microeconomics research. With this article, we counter this trend of negligence and try to convey the message that animal models are an indispensible complement to the literature on human economic decision making. This perspective review departs from a description of the similarities in economic and evolutionary theories of human and animal decision making, with particular emphasis on the optimality aspect that both classes of theories have in common. In a second part, we outline that actual, empirically observed decisions often do not conform to the normative ideals of economic and ecological models, and that many of the behavioral violations found in humans can also be found in animals. In a third part, we make a case that the sense or nonsense of the behavioral violations of optimality principles in humans can best be understood from an evolutionary perspective, thus requiring animal research. Finally, we conclude with a critical discussion of the parallels and inherent differences in human and animal research.
“The difference in mind between man and the higher animals, great as it is, certainly is one of degree and not of kind.”
The Descent of Man (Charles Darwin, 1871)
Similarities between Normative Theories of Decision Making in Economics and Biology
Why study animal decision making?
Do animals make economic decisions? Even though the answer is trivial for students of the cognitive sciences, surprise and skepticism is a common response, not only among lay people, when we answer this question with “yes.” Animals don’t think, so how can they make economic decisions, and why would it be interesting to study them? In very general terms, economics is a discipline that aims to predict how human individual decisions affect the supply and demand of (usually limited) resources. So, what are the reasons for studying animal behavior when one is ultimately interested in theories of human economic decision making?
Of course, monkeys and other animals are widely used in neuroeconomics and economically flavored neuroscience research, often for methodological reasons (see for instance Floresco et al., 1997, 2008; Shizgal, 1997; Leon and Shadlen, 1998; Platt and Glimcher, 1999; Cardinal et al., 2001; Yanagihara et al., 2001; Izawa et al., 2003, 2005; Phillips et al., 2003; Barraclough et al., 2004; Dorris and Glimcher, 2004; Sugrue et al., 2004; Kalenscher et al., 2005, 2006a; McCoy and Platt, 2005; Tobler et al., 2005; Padoa-Schioppa and Assad, 2006; Roesch et al., 2006; van den Bos et al., 2006a, b; Yang and Shadlen, 2007; Kalenscher and Pennartz, 2008; Kim et al., 2008; Gan et al., 2009; Louie and Glimcher, 2010). However, as we will argue in this article, technical reasons are not the only grounds for studying animal choice behavior. In order to fully comprehend the origins of human choice behavior, we should investigate the evolutionary roots of our decision making processes by looking at choice mechanisms and their neural substrates in animals. We maintain that, even though there might exist a many-to-one mapping of neural implementations to choice processes, careful comparisons across species can complement human microeconomics research by supplying possible answers to the question why we make decisions as we do.
Assumptions in Biological and Economic Accounts of Choice Behavior
Animal decision making has traditionally been studied assuming that animals optimize their energy intake and reproductive opportunities under evolutionary pressure, and have adapted their choice behavior accordingly. Hence, many ecological theories of decision making have in common with economic models that they focus on optimality of choice behavior, which gives both schools of thinking a normative flavor. Moreover, both schools share crucial concepts, such as equilibrium states in social exchange (Nash, 1950) or evolutionary strategies (Dawkins, 2006). In that normative tradition, until recently, economic theories departed from the assumption that decision makers have (i) stable preferences over time and context, (ii) are motivated by their material self-interest (iii) are rational (in the sense that they make internally and intertemporally consistent decisions that are in accordance with their own stable preferences), and (iv) that decision makers’ choices are made with respect to final states and not with respect to changes of states (in the sense that decisions should not be made with respect to gains or losses, but with respect to the final monetary levels1; Friedman and Savage, 1948, 1952; Varian, 2006). Despite crucial differences, there are remarkable similarities in the assumptions and implications made in economic theories and ecological models of animal foraging in the biological literature (cf. Caraco, 1983; Stephens and Krebs, 1986; Kacelnik, 2006) and, to a somewhat lesser extent, in reinforcement learning models in psychology (Thorndike, 1911; Herrnstein, 1961, 1970; Navarick and Fantino, 1974). We like to illustrate the similarities between the disciplines with two examples: decision making under risk and over time.
Decision Making Under Risk: Expected Utility Theory and Risk Sensitivity Theory
One of the dominant theories in economics for decision making under risk, expected utility theory (EU), formally prescribes choice behavior of decision makers that are assumed to behave as if they maximized expected utility. Expected utility is the sum of the probability-weighted utilities of all possible final states of an option, i.e., after the options’ prospects are integrated with the current asset level (von Neumann and Morgenstern, 1944). Assuming concave utility functions (Bernoulli, 1954), EU predicts risk aversion, as illustrated in Figure 1.
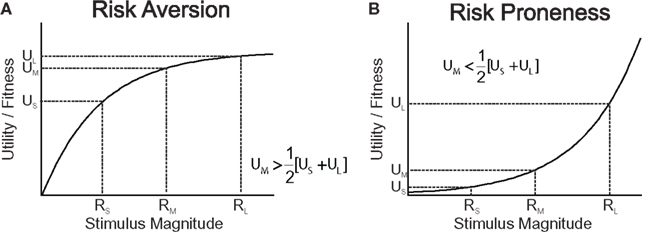
Figure 1. Utility functions can explain risk attitude according to expected utility theory and risk sensitivity theory. (A) Utility (for humans) or Darwinian fitness (for animals) as a function of the magnitude of a commodity. The utility/fitness curve is concave and is a decelerating function of the current level of stimulus magnitude (wealth/amount) because the marginal utility/fitness increment decreases with increasing level of stimulus magnitude. A concave utility/fitness function predicts risk aversion when choosing between a medium-sized, certain reward (RM) and a risky option offering large or small rewards (RS and RL) with equal probabilities. (B) A convex function predicts risk proneness.
In biology, classic optimal foraging theory assumes that evolution has favored foraging strategies that maximize the rate of energy intake as a proxy of Darwinian fitness (Charnov, 1976; Cowie, 1977). Since a given food source is progressively depleted with the time spent foraging, the marginal energy gain obtained from a given source is decreasing as a function of foraging time. Hence, the rate of gain, and thus ultimately Darwinian fitness associated with a food source is monotonically rising, but, due to its decreasing marginal gain, decelerating over time spent foraging. However, this alone is not yet sufficient to draw a parallel between optimal foraging and EU. In fact, classic optimal foraging theory posited that animals behaved as if they considered only average outcomes in the environment, and hence ignored outcome variability and risk. Consequently, inconsistent with the empirical reality (Kacelnik and Bateson, 1996; Kacelnik, 1997; Bateson and Kacelnik, 1998), classic optimal foraging models predicted risk-neutral attitudes. Risk-sensitive foraging theory has extended the classic framework of non-linear relationships between Darwinian fitness and energy gain to time- and outcome-variable, risky environments (Stephens, 1981, 2008; Stephens and Krebs, 1986; Kacelnik and Bateson, 1996). According to risk-sensitive foraging theory, if an animal chooses between two food patches offering equal average gains, but differing in outcome variance (risk), then a concave, monotonically increasing and decelerating function linking Darwinian fitness to energy gain would predict risk aversion (Figure 1A). Note that accounts referring to skewed memory representations of the risky outcomes can equally well account for risk aversion, but to a lesser extent for risk seeking (Reboreda and Kacelnik, 1991; Bateson and Kacelnik, 1996; Kacelnik and Bateson, 1997).
Decision Making Over Time: Discounted Utility Theory, Rate Maximization, and the Matching Law
Most of our decisions do not yield immediate outcomes, but outcomes that can only be realized at some point in the future. Literally all human and non-human animals tested devalue (discount) future relative to immediate outcomes (Samuelson, 1937; Knapp et al., 1959; McDiarmid and Rilling, 1965; Chung and Herrnstein, 1967; Rachlin and Green, 1972; Ainslie, 1974, 1975; Benzion et al., 1989; Green et al., 1994; Kalenscher et al., 2005, 2006a, b; Kalenscher and Pennartz, 2008). In economics, the dominant framework for decision making over time is discounted utility theory (DU; Samuelson, 1937; Koopmans, 1960; Lancaster, 1963; Fishburn and Rubinstein, 1982; Prelec and Loewenstein, 1991; Frederick et al., 2002; Kalenscher and Pennartz, 2008). In brief, DU posits that a decision maker behaves as if she maximized discounted utility, with discounted utility being the sum of the discount-factor-weighted utilities of all possible final states. Classically, DU assumed an exponentially decreasing discount function with a constant discount rate (Figure 2A; Samuelson, 1937). Constant discounting has important implications for rationality and time-consistency of preference. According to DU, it is not irrational or non-optimal per se to prefer small, short-term over large, long-term rewards, even if the preference for immediacy results in an overall reduced net gain over time. However, DU requires consistency over time. That is, if an individual prefers a small, short-term reward over a large, long-term reward, and both rewards are shifted in time by an identical time interval, then the preference for the small, short-term reward should be preserved because both rewards should be discounted by the same rate.
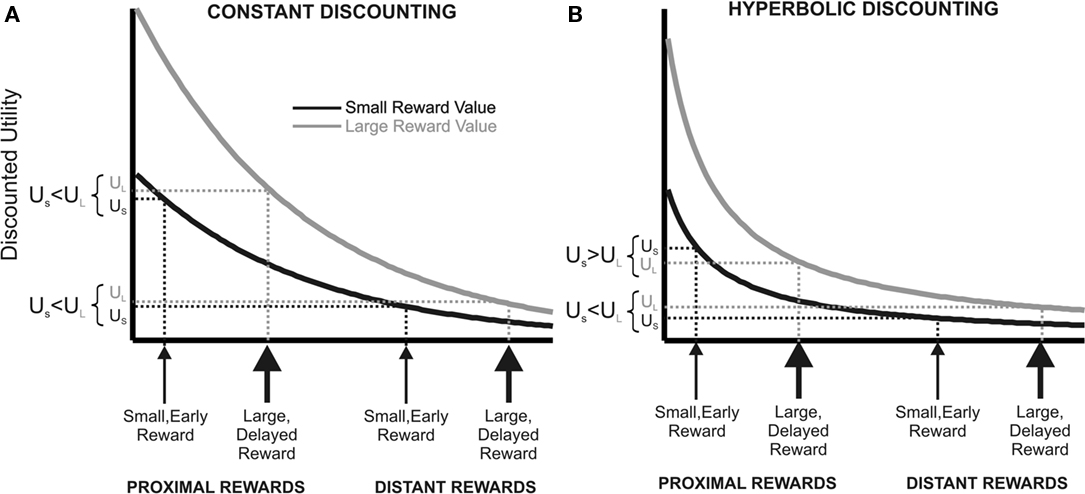
Figure 2. Constant vs. hyperbolic discounting of future events. The figure describes a choice between a small, short-term outcome or a large, long-term outcome (proximal), and another situation in which both outcomes are deferred into the future by the same time interval (distant). (A) Constant (here: exponential) utility function of a large, delayed (gray line) and small, short-term commodity (black line). With exponential discounting, preference stationarity holds when the rewards are deferred by the same time interval into the future. (B) People seem to place a premium on short-term availability of rewards, deflecting the discount into an upward direction for temporally close rewards. The resulting hyperbolic discount function can explain preference reversals over time. Due to the steeper utility decay for short delays, the utility of the small, short-term commodity is higher than the large, delayed reward for temporally proximal outcomes, but the utility order reverses when both outcomes are deferred into the future.
Animals also make decisions over time: during foraging, several time delays affect the rate of energy gain, for instance travel time between food patches, handling time of an item of prey, time between unsuccessful and successful foraging attempts etc. Behavioral ecologists assumed that animals maximizing Darwinian fitness should use foraging strategies that maximize, in the long run, the net energy gain per time unit, i.e., the ratio of food intake to the time needed to obtain or consume the food (Stephens and Krebs, 1986). For example, in a choice between large, delayed and small, short-term rewards, rate maximization predicts that animals prefer large rewards when the ratio of reward amount per time unit is higher for the large than for the small reward.
The reinforcement learning literature in psychology made predictions regarding animal and human decision making over time that are comparable to the predictions of rate maximization in biology. Thorndike’s (1911) law of effect, stating that “responses that produce a satisfying effect in a particular situation become more likely to occur again in that situation” implies that greater reinforcement (more frequent, bigger, or more preferred rewards) results in greater response rates (more frequent behaviors that produce the reward). This has led to formulation of the matching law (Herrnstein, 1961, 1970), according to which the relative selection-rate of one out of several choice options matches the relative rate of reinforcement offered by that option. Hence, the shorter the time intervals between rewards, the higher the reward rate, the more often the option offering these rewards should be chosen. In other words, as predicted by optimal foraging models, animals obeying the matching law would maximize energy gain rate in choice situations where the different choice alternatives yield different streams of reward rates (Kalenscher et al., 2003).
Similarities and Differences between Economic, Biological, and Psychological Models of Choice Behavior
The previous paragraph shows that, even though rate maximization is a prescriptive, normatively flavored theory and the matching law is a descriptive, empirically derived model, both approaches make very similar predictions. However, the similarities between rate maximization and DU are less apparent, and their correspondence is also less evident than for EU and risk sensitivity theory in decision making under risk. In fact, the differences between the two approaches seem more obvious at first glance than their similarities. For instance, DU and optimal foraging theory differ in that DU makes no prediction about the optimality of preferring small, short-term or large, long-term rewards, whereas optimal foraging theory predicts that animals should maximize long-term reward rate. On the other hand, whereas DU prescribes time-consistent preferences when adding a constant time interval to all rewards optimal foraging may predict preference reversals2. Nevertheless, despite these differences, both theories have remarkable similarities, too. Both approaches are normative and prescribe rather than describe optimal behavior. They both assume maximization of a currency (utility in DU and energy rate in optimal foraging theory). Also, they have in common that they condemn a disproportionally strong, impulsive preference for immediacy as non-optimal if it leads to discontinuous preferences over time (see Frederick et al., 2002; Kalenscher and Pennartz, 2008; Kalenscher and Tobler, 2008 for review, but see Stephens and Anderson, 2001; Stephens et al., 2004). Hence, despite the disparity of the two approaches, both frameworks would agree on the classification of a large range of different strategies as rational or as anomalous.
In summary, despite considerable differences, the normative literature in economics and ecology on choice behavior of human and non-human animals, and some of the descriptive literature in psychology, makes remarkably similar assertions and predictions about decision making under risk and over time. Presumably the most notable difference between choice theories in economics, ecology, and psychology is the focus (or lack of it) on cognitive processes. Whereas theories in economics often explicitly refrain from making any statements about the choice-underlying cognitive processes, and emphasize their pure focus on outcome instead (in many, if not most economic models, a decision maker behaves as if she maximized EU; (Samuelson, 1938; Gul and Pesendorfer, 2005), theories in cognitive psychology do precisely the opposite, i.e., put the spotlight on process, but not outcome. Note, though, that the sub-discipline in psychology that has the strongest tradition in using animal subjects, behaviorism, shares with economics the strict rejection of investigating mental process. Behaviorists are interested in describing stimulus–response or response–outcome relationships, and explicitly refuse to make any statements about the cognitive “black box” that links stimulus with outcome. Biological theories are less strict in their distinction between process and outcome, and, whereas some ecological theories have a more exclusive emphasis on one or the other, other theories combine process and outcome (e.g., Reboreda and Kacelnik, 1991; Bateson and Kacelnik, 1996; see Kacelnik, 2006 for review).
The Reality of Decision Making: Humans and Animals Show Very Similar Violations of Economic Theory
The previous paragraphs suggested that the difference in human and animal economic behavior is much smaller than one may think at first glance. This notion is corroborated by several lines of research that empirically test predictions of economic theory using non-human animals, including monkeys, rats, and pigeons (see e.g., Kagel et al., 1975, 1981, 1995; MacDonald et al., 1991; Santos and Keith Chen, 2009). These studies show that rats, for instance, comply surprisingly well to the predictions of demand theory, price theory, labor supply, decision making under risk and intertemporal choice. Yet, despite the accuracy of economic theory to account for much of human and animal behavior, we know from personal experience and countless publications in the literature on decision making that humans often fail to meet the strict assumptions made in classic economic models. Interestingly, many, if not most of the violations of the predictions of economic theory that can be observed in humans are also found in animals. The next paragraph gives a brief and selective overview of some of these violations.
In contradiction to the common assumption of unconditional self-interest (discussed, for instance, in Fehr and Fischbacher, 2002), most human individuals do care about the well-being of others, either in a prosocial way by, e.g., giving to charity or accepting costs to improve the well-being of others, or in a counter-utilitaristic way, e.g., in parochial situations where the well-being of others is reduced at a cost and without direct benefit to the actor (Fehr and Schmidt, 1999; Fehr and Fischbacher, 2002, 2003; Camerer, 2003; Baron, in press). Moreover, humans are able to cooperate with a partner even if defection would satisfy their material self-interest better in the short run (Trivers, 1971; Rilling et al., 2002). In addition to strategic considerations, such as reputation building, genuine social motives like inequity aversion and envy presumably play a role during social behavior (Fehr and Schmidt, 1999). Despite strong controversy (see, for instance, Henrich, 2004; Wynne, 2004a, b), animal behavior seems to some extent be guided by social motives, too. For instance, capuchin monkeys appear to be inequity averse (Brosnan and De Waal, 2003), and capuchin monkeys (de Waal et al., 2008), tamarins (Hauser et al., 2003), chimpanzees (de Waal and Suchak, 2010; Melis et al., 2011), rats (Rutte and Taborsky, 2007, 2008), fish (Raihani et al., 2010), and various other animals, including insects (Axelrod and Hamilton, 1981) show behavior resembling direct or generalized reciprocity. Not only great apes and monkeys (de Waal and Suchak, 2010), but also blue jays (Stephens et al., 2002), rats (Viana et al., 2010), fish (Raihani et al., 2010), and many social insects, like bees and ants (Kolmer and Heinze, 2000; Ratnieks and Helantera, 2009; Rueppell et al., 2010), cooperate with conspecifics in social situations; social insects even accept high costs, such as sacrificing their own life, if this benefits the society (Ratnieks and Helantera, 2009; Rueppell et al., 2010).
Economic models make inadequate assumptions and predictions in non-social contexts, too. For instance, the notions of internal consistency and stable preferences are often violated: human subjects frequently show intransitive preferences, preferring choice alternative A over B and B over C, but not A over C (Tversky, 1969; Kalenscher and Pennartz, 2010; Kalenscher et al., 2010), or make context-dependent choices, preferring A over B, but reversing this preference when an inferior option C is added to the pool of choice alternatives (also known as a violation of the independence axiom; Tversky and Simonson, 1993). Intransitive and context-dependent choices can occur when individuals choose between alternatives that vary along several dimensions, e.g., gain magnitude and probability. People seem to fail to treat each multidimensional option as an integrated whole, but appear to compare each attribute separately and then consider the difference between attributes instead of the difference between utilities attributes3 (Tversky, 1969; Roelofsma and Read, 2000; Brandstätter et al., 2006; Kalenscher and Pennartz, 2010; Kalenscher et al., 2010). Many animals reveal inconsistent preferences, too, even when internal homeostatic drives, such as hunger or thirst, are controlled for: honeybees, pigeons, and gray jays show intransitive preferences (Navarick and Fantino, 1972, 1975; Shafir, 1994; Waite, 2001), and hummingbirds show violations of the independence axiom and make context-dependent decisions (Bateson et al., 2003).
Choices are particularly prone to inconsistency when making decisions over time. It has been repeatedly shown that preferences reverse when the delays to both rewards are advanced or deferred in time, and basically a discontinuity of preference can often be observed when immediate outcomes become available4 (time-inconsistent preferences; Thaler and Shefrin, 1981; Benzion et al., 1989; Ainslie and Haslam, 1992; Green et al., 1994; Kirby and Herrnstein, 1995; Frederick et al., 2002; Kalenscher and Pennartz, 2008; Kalenscher and Tobler, 2008). Such non-stationarity of intertemporal preferences suggests that people add extra value to short-term availability of rewards (illustrated in Figure 2B). The disproportionally high value placed on short-term rewards is believed to be responsible for the fact that most humans find it very difficult to act in accordance with their long-term interest (Haynes, 2009). Examples include breaking diets, living unhealthy lifestyles, financial illiteracy, insufficient retirement provisions, substance abuse and even mundane issues like postponing dentist appointments. The inadequacy of DU to account for the reality of intertemporal choice behavior is even more apparent when dealing with delayed losses or aversive events. DU did not make a particular distinction between the treatment of losses and gains: the aversiveness of losses should be discounted as much as the attractiveness of gains when the outcome of a decision is more and more delayed. However, it has been shown that the attractiveness of gains is reduced faster than the aversiveness of losses (Thaler and Shefrin, 1981), implying a different discount rate for gains than for losses. An even greater challenge for DU is the observation that many human subjects prefer to expedite a loss instead of delaying it. If losses loom less when they are temporally remote, as predicted by DU, subjects should be ready to defer losses into the future. However, many subjects actually prefer to incur a loss or an aversive event immediately rather than delay it (Loewenstein, 1987; Benzion et al., 1989). Much like humans, a whole range of different animals, including monkeys, pigeons, rats, mice, and even insects show non-stationary, time-inconsistent intertemporal choices in tasks involving front-end delay (i.e., adding a common time interval to both options), and place extra value on instant outcomes (Rachlin and Green, 1972; Ainslie, 1974, 1975; Green et al., 1981; Bateson and Kacelnik, 1996; Kacelnik, 1997; Isles et al., 2003; Kalenscher et al., 2005, 2006a; Kalenscher and Pennartz, 2008; Kim et al., 2008; Louie and Glimcher, 2010). When choosing between timed aversive events, rats, much like humans, sometimes accelerate, rather than defer electric shocks (Knapp et al., 1959).
Some violations of economic theory are less intuitive than time-inconsistent preferences. Even though according to EU or other classic economic models, risk attitudes should be consistent when making decisions under risk, individuals prefer certain options when gambling for gains, but risky options when gambling to avoid losses (Kahneman and Tversky, 1979). This reversal of risk attitude has been termed the reflection effect because the reflection of the prospects around 0 (changing the sign from gains to losses) reverses the preference order. Reflection effects suggest that, apparently, choices are not made with respect to final states, but with respect to changes in final states, i.e., with respect to gains and losses. Risk attitude and the reflection effect are not only functions of objective gains and losses, but the way a problem is (verbally) presented affects the way it is cognitively treated (Kahneman and Tversky, 1979, 1984). For example, the choice of words can influence whether one and the same prospect is perceived as a choice between gains, or as a choice between losses. Such framing of a decision problem has an effect on the subjects’ risk attitude and determines whether she is risk-averse (for gains) or risk-prone (for losses). Moreover, humans show a strong overweighting of rare events, and a literal discontinuity in preference when certain outcomes become available (certainty effect; Kahneman and Tversky, 1979). Rats and various birds also show reflection effects and reversals of risk attitudes (Kacelnik and Bateson, 1996; Bateson and Kacelnik, 1998), and rats (MacDonald et al., 1991) and honeybees (Shafir et al., 2008) show certainty effects. Moreover, starlings show framing effects when making decisions under risk: they reverse their risk preference depending on whether the relative reward levels in a risky condition are higher or lower than the reward level in a standard “frame” (Marsh and Kacelnik, 2002). Another violation of economic theory includes the sunk-cost fallacy – the continued investment of money, time, effort, or resources into an unsuccessful project over a long period of time although the project clearly yields no results, and an investment into an alternative activity would promise better outcomes (Kogut, 1990; Arkes and Ayton, 1999). Pigeons commit the sunk-cost fallacy, too: their persistence to respond on a pecking key in an expected ratio schedule in which a probabilistic reward is delivered if a fixed ratio performance (a fixed number of responses) with variable response frequency requirements is met, is strongly influenced by their previous effort investment on that key (Navarro and Fantino, 2005). Finally, the endowment effect refers to the observation that goods that are in possession of a subject seem to be valued higher by the subject than goods that can be purchased (Grether and Plott, 1979). Endowment effects can be found in chimpanzees, too (Brosnan et al., 2007).
Note that the existence of these violations does not falsify EU or other economic or biological theories; it merely implies that economic theory does not apply to people (or animals) who do not meet the rationality assumptions posited by economic theory (Samuelson, 1938). However, it is of crucial interest for economists and biologists alike to understand why animals and humans so frequently and systematically violate economic or ecological theory. Only animal research offers the opportunity to use invasive tools, and thus manipulate cognitive mechanisms and their neural substrates to study the effect on economic behavior. Importantly, therefore, investigation of animal choices and their neural substrates in carefully translated experimental paradigms could yield data on the neural implementation of decision parameters that would otherwise be hard to obtain. These data should ultimately be used to update existing models of human brain function in economic decisions.
How Can Animal Models Inform Economic Research?
Animal Behavior Offers Insight into the Evolutionary Roots of Decision Making
Of course, one of the foremost reasons to use animals in decision research is methodological, as briefly touched upon in the introduction. Neuroeconomics aims to reveal the neural processes underlying economic decision making. The majority of the most accurate and promising technologies used in neuroscience research cannot be used on humans for ethical and practical reasons, including basic manipulations such as lesion studies, electrophysiology, microdialysis, psychopharmacology and others. As trivial as it is to point out, animal models are therefore an indispensible and crucial tool in neuroeconomic research. However, we hope to have convinced the reader by now that methodological reasons are not the only grounds to use animal subjects for studying economic decision making.
In the previous chapters, we have emphasized the parallels in the theoretical models on human and animal decision making and pointed up the correspondence in actual economic behavior. The similarities in compliance with and violations of the predictions of economic theory suggest that some rudiments of human decision patterns can also be found in non-human animals. This implies that human and animal decision making may share evolutionary roots: presumably, the way we make decisions today is the result of natural selection, so that the choice mechanisms found in modern humans probably once (and maybe still today) equipped decision makers in the best possible way to adapt to and deal with the intricacies of the environments in which they evolved. This offers unique insights into the sense or nonsense of violating optimality principles, such as variable and time-inconsistent preferences.
Several authors argue that, although the decision rules used by modern humans provided the highest possible fitness increases to animals in the environments in which they evolved, they may actually be maladaptive today and fail to perform well in the intricate and flexible environmental structure of modern societies (Kahneman and Tversky, 1996). Take the example of time-inconsistent intertemporal choices: Stephens (2002, 2008) maintains that the short-sighted, over-impulsive decision mechanisms observed in animals when making intertemporal decisions are actually well adapted to meet the challenges an animal is facing in a real environment. An animal’s real environment does, for example, have a sequential foreground/background structure. Sequential means that decisions are usually not between binary options (choose either A or B, which is usually the case in laboratory experiments), but between a sequence of options, such as the decision whether to stay in a currently exploited, depleting food patch or leave to another food patch (which involves traveling time/effort and the risk of not finding an equivalent one), or whether to attack a prey item or continue searching for better prey. Foreground/background means that an animal usually follows a default strategy (background: searching for food) that needs to be put on hold for the time being if a potential food item is encountered (foreground). Several authors (Stephens and Anderson, 2001; Stephens, 2002, 2008) have shown that animals making non-optimal decisions in artificial laboratory situations actually choose optimally in economically equivalent, but sequential foreground/background choice situations that meet the criteria of higher ecological validity. Hence, the very same choice rules that perform well in these “natural” choice scenarios, produce deviations from normative models, such as impulsive, delay-oversensitive preferences in artificial laboratory settings. If the choice mechanisms humans employ to make intertemporal decisions share common evolutionary roots with non-human animals, then natural selection has favored decision rules that are optimally adapted to the sequential foreground/background environment of foraging animals, but the same decision rules may fail to perform well in our “modern” binary-choice environments where over-impulsiveness is a vice, not a virtue.
In contrast to the view that violations of normative ideals are maladaptive, others (Gigerenzer and Goldstein, 1996; Goldstein and Gigerenzer, 2002; Hutchinson and Gigerenzer, 2005; Brandstätter et al., 2006) maintain that human decision rules are as adaptive now as they were when they evolved because the benefits of these rules, such as computational speed and accuracy, outweighed the drawbacks, such as the occasional non-optimal choice, back then as much as now. According to this view, comparative research yields optimality criteria that were previously not recognized or considered in traditional economic models (Hutchinson and Gigerenzer, 2005). Comparative research may thus contribute to modifying and amending existing normative theories to improve their descriptive validity and explanatory power. For example, a decision maker who maximized expected utility for decision making under risk should behave as if she integrated each outcome’s probability with the utility of the outcome, and compared these integrated utilities across outcomes. Such a computation involves several mental transformations: translation of objective reward magnitude into utility, multiplication with an accurate mental representation of probability, repeating this for every option, and comparing these integrated expected utilities across options. Several authors argued that the direct comparison of the attributes, i.e., comparing probabilities and reward magnitudes separately, provides in most cases a much more accurate, fast, precise, easy, and less error-prone estimation of the best option than the “economically sound” way, albeit at the cost of making inconsistent choices in special circumstances (Russo and Dosher, 1983). It has been argued that such decision rules evolved because they maximized Darwinian fitness in animals (Houston, 1997) and prevail because they continue to produce near-optimal decision outcomes in humans, too (Russo and Dosher, 1983; Brandstätter et al., 2006).
However, identifying the evolutionary basis of choice behavior is not the only reason to conduct animal research on economic decision making. The analysis of animal behavior is a convenient, economical, and sound way to test competing economic decision models in optimally controlled experimental environments. The cultural, cognitive and motivational confounds and the experimental design issues related to differences in belief- and value-systems that are very difficult to overcome in human research are not an issue in animal studies. Moreover, animal models allow for experimental manipulations, including real appetitive and aversive consequences, that are for practical and ethical reasons not implementable in human research. As pointed out by Kagel et al. (1995), animals are a mean to probe the elementary principles of microeconomic theory: if these basic principles fail to account for the behavior of simple organisms, such as rats or pigeons, in simple choice situations, such as Skinner box experiments, how can they be trusted in much more complex situations involving much more complex organisms, such as our worldwide economic systems with human actors? Or, in other words, “[…] a theory that works well across species has a greater likelihood of being valid than one that works will with only one, or a limited set of, species.” (Kagel et al., 1995, p. 4).
Economic research on animals is often criticized on the grounds that animals are considered irrational and instinct-driven. Hence, how could economic theory that relies on the assumption of rational decision makers be applied to animal behavior? In addition to the problematic usage of the word “instinct,” we hope to have conveyed the message that the differences in animal and human behavior and cognition may be a matter of degree, and not of kind, as testified by the remarkable conformity of animal decision making with the principles of microeconomic theory (Kagel et al., 1995), and also with the parallels between human and animal behavior in violating them.
In sum, we argue that neither pure theoretical reasoning nor exclusive experimentation with human subjects will be sufficient to obtain a comprehensive picture of human decision making, including where humans conform to the principles of rational choice models and where and why humans violate them. We maintain that, in order to understand not only how we make decisions, but also to investigate why our decisions are what they are, it is imperative to know the reserve constraints, evolutionary pressures, and adaptive benefits that molded the choice behavior in the first place, aiming to add construct validity to the models. We conclude that the prime way to obtain access to the evolutionary pressures shaping our decision mechanisms and to identify common denominators in choice behavior is to study animal behavior and its neural mechanisms.
Caution is Required When Drawing Parallels between Human and Animal Behavior
In the previous paragraphs, we have emphasized the parallels in the theoretical models on human and animal decision making and pointed up the similarities in economic behavior. Admittedly, we conveniently skipped the discussion of the differences in theories and behavior. However, if we want to use animal models to explain human behavior, it is imperative to know not only the similarities, but also the differences across species. Moreover, it is dangerous to draw cross-species parallels in behavior and mechanism too quickly since many findings may have face validity only. That is, because a problem can be solved by a plethora of different mechanisms, it is quite possible that human and animal economic choice behavior, and many of the violations of rational choice observed across species, appear similar and are therefore given identical names, while the underlying cognitive and neural processes may be fundamentally different. Even though this is less of a predicament for traditional economics given that economics is conventionally only interested in prescribing choice–outcome relations, not in identifying the choice-underyling mental and neural processes, this is particularly problematic for disciplines interested in those very processes, including cognitive psychology, comparative biology, and neuroscience. Therefore, much of comparative research aims to identify which mental processes are comparable across species, and which ones are not (Rosati et al., 2007).
Moreover, as the previous paragraphs illustrated, caution is even more warranted when drawing parallels between human and animal economic behavior since the experimental designs used may bias results and their interpretation. For obvious reasons, the procedures and designs used to elicit the behaviors in humans and animals described in the preceding paragraphs have several fundamental differences. The most evident difference lies in the incentivization used to motivate human and animal participants: whereas humans are usually paid contingent on their choices or are instructed to imagine virtual payoffs, animals receive food or liquid rewards. Food and money are essentially dissimilar commodities: whereas food is a primary reward (eliciting a strong direct hedonic response), money is a powerful secondary reinforcer (money itself does not produce a hedonic response, only its association with primary reinforcers makes it a reward). This calls into question in how far the results of comparative intertemporal choice experiments are commensurable. Moreover, during intertemporal choice studies, the delays associated with money in human studies are usually in the range of months or years, whereas the delays associated with food rewards in animal studies are usually in the range of seconds. Even though one may dismiss comparative human and animal research as non-commensurable in principle based on these grounds, recent attempts in the intertemporal choice literature to match the experimental procedures used in humans to the ones common in animal research suggest the opposite. The incentives used in these studies involved primary rewards, such as liquids (McClure et al., 2007) and pictures of the opposite sex (Hayden et al., 2007) and involved much shorter delays. The results show that human participants exhibited the same behavioral patterns found with secondary reinforcers, such as money. Moreover, the neural networks involved in making intertemporal decisions for primary rewards, with delays in the seconds to minutes range, were remarkably similar to the networks involved in financial intertemporal decisions with delays in the range of days to months (McClure et al., 2004, 2007). The procedure-independence of behavior and neural mechanism suggests that the agreement of intertemporal choice behavior in animals and humans cannot easily be dismissed based on procedural grounds alone. In partial support of this, in an experimental design that was manufactured to allow best-possible comparison between species, Rosati et al. (2007) showed that humans were generally only as delay-tolerant, and often even slightly less patient than chimpanzees and bonobos when waiting for future primary rewards. Because of the parallel in delay tolerance between humans and apes, and since the degree of patience exhibited by the apes exceeded the level predicted by common short-term maximization models, the authors conclude that the capacity to endure long delays for food evolved before the human lineage split, suggesting that apes and humans share common intertemporal choice mechanisms, at least for primary rewards. However, because humans were substantially more patient to wait for monetary rewards, they also suggested that the human ability to delay gratification for secondary reinforcers is unprecedented in the animal kingdom, raising doubts about whether the mental processes for primary and secondary rewards are identical. Nevertheless, given the indications discussed above, the overall evidence implies that the procedural differences in studies using primary and secondary reinforcers, and the mental processes involved, may be less significant than feared.
Also in the domain of decision making under risk, there are elementary differences in the typical procedures used in animal and human research. In humans, subjects are usually instructed about the probabilities of a gain or a loss, often in one-shot scenarios (only one question asked, no repetitions). By contrast, because animals cannot be verbally instructed, reward probabilities are usually operationalized as reward frequencies in multi-trial settings, so that a reward with a probability of 0.8 implies that, on average, 8 out of 10 trials are rewarded. It is likely that extracting reward probabilities from reward frequencies involves fundamentally different cognitive and neural systems than when being instructed about these attributes in one-shot sessions. Moreover, several authors pointed out that tasks involving probabilistic rewards may not be interpreted by the animal subject as a decision under risk, but as an intertemporal choice (Rachlin et al., 1986; Hayden and Platt, 2007; Kalenscher, 2007). According to this idea, it is possible that reward frequencies are construed as delays so that, for instance, a reward with a probability of 0.5 is perceived as a reward with a delay of, on average, two trials duration. However, here again, several lines of evidence suggest that procedural differences are not necessarily a blackout argument to dismiss any belief in a similarity between human and animal decision making under risk. For instance, some behavioral patterns in human behavior that are found when probabilities are instructed can also be found when probabilities have to be extracted from reward frequencies. For example, in a study touched upon above, Fantino and Navarro found that humans and pigeons were equally likely to commit the sunk-cost fallacy in a task in which the (human and avian) participants had to decide whether to persist to respond in an expected ratio schedule, or abort a trial and start a new one (Navarro and Fantino, 2005). By contrast, others argue that probabilities extracted from experience induce different behavioral biases than instructed probabilities (Hertwig et al., 2004). For instance, the typical overweighting of rare events when probabilities are instructed (Kahneman and Tversky, 1979) contrasts with a characteristic underweighting of low likelihoods when probabilities are extracted from experience (Hertwig et al., 2004). However, comparative research with bees and humans suggest that this divergence of evidence between descriptive and experience-based likelihood extraction can be attributed to perceptual noise when extracting information from experience, and the original overweighting, in both bees and humans, can be reinstated when perceptual noise is reduced (Shafir et al., 2008). This would suggest, again, that procedural differences are less significant than feared. However, another study found that preference reversals (here: the tendency to place higher value on a high risk, high magnitude gamble, but to prefer a low risk, low magnitude gamble) are reduced when human subjects extract probabilities from experience, and not from description (Chu and Chu, 1990).
In conclusion, it is still under debate whether animal models for decision under risk are suitable approximations of human decision making, given the controversy whether the mental processes involved when extracting probability information from experience or instruction are identical. Nevertheless, at least some evidence suggests that animals and humans behave similarly when procedural differences are controlled for, implying, but not proving, that the underlying choice mechanisms may be similar, too. We want to stress that this uncertainty is by no means reducing the necessity of animal research. On the contrary, because parallels in brain functioning cannot always straightforwardly be drawn between humans and animals at large, but also between different species of animals and their diverging brain connectivity involved in choice behavior, exactly those differences between species can be highly informative and can shed light on the function of the underlying systems. For example, the conclusion that the cognitive and neural mechanisms underlying intertemporal choice are identical across species is not always straightforwardly supported by the empirical reality: because activity in human ventral striatum is often positively correlated with impulsiveness, present-bias, or with choices of the most temporally proximal option, as revealed by functional magnetic resonance imaging (Hariri et al., 2006; Glimcher et al., 2007; Kable and Glimcher, 2007), one would expect that less ventral striatum activity would predict more delay tolerance. However, the opposite has been found in animal research: rats with lesioned ventral striatum are more, not less impulsive, compared to control rats (Cardinal et al., 2001), suggesting that the integrity of ventral striatum is necessary for maintaining self-control (in the sense of delay-tolerance). Even though it is impossible to entirely rule out that the ventral striatum plays a functionally different role in rats and humans during intertemporal choice, this explanation is unlikely because the ventral striatum is phylogenetically identical and homologous across species (Reiner et al., 1984; Pennartz et al., 1994; Durstewitz et al., 1999; Mezey and Csillag, 2002; Izawa et al., 2003). It is more likely that the ventral striatum plays a role in optimizing decisions over time, according to which too much or too little ventral striatal activity results in suboptimal, impulsive decisions. This idea is supported by a wealth of findings in the psychopharmacology literature suggesting that both amplifying and antagonizing the dopaminergic input into the ventral striatum increases impulsiveness in rats and humans (Evenden and Ryan, 1996; Cardinal et al., 2000; Cardinal, 2006; Boettiger et al., 2007; Buckholtz et al., 2010; Pine et al., 2010). We conclude from this example that comparative research provides better answers to the question of the role of ventral striatum in intertemporal choice than research with either species alone. Hence, what is needed is a comprehensive comparative approach, preferably across humans and multiple species of non-human animals, in which lab studies on animal and human economic decision making are additionally complemented by field studies to probe the theories in real-world environments.
Conclusion
In this perspective review, we have argued that, even though the contexts wherein economic decisions made by humans on the one hand and animals on the other can be vastly different, economic theory can be remarkably successfully applied to human and animal behavior alike. We regard the critical examination of economic theory the prime objective of performing experiments in decision making and we maintain that in this light it is imperative to include animal models in the arsenal at our disposal. In the worst case, results obtained in animals will corroborate those obtained from humans, strengthening the existing theory. Preferably, though, comparative research will uncover inconsistencies in choice behavior between humans and animals that allow for an improved, more comprehensive description of choice behavior and possibly force us to re-think the basis of economic theory in the light of the evolutionary roots of choice.
A potential problem using this approach remains that results obtained with human and animal choice paradigms could have only face validity in reproducing each others’ findings, and could diverge in the underlying cognitive processes subserving economic decision making. Rather than viewing this as a discredit to comparative research, we see underconstrainment of the cognitive processes governing choice behavior as an invitation to bridge the fields of biology, psychology and economics in further, careful probing of the neural basis of economics decision making across species.
Conflict of Interest Statement
The authors declare that the research was conducted in the absence of any commercial or financial relationships that could be construed as a potential conflict of interest.
Footnotes
- ^For example, a decision maker should be indifferent between these two cases: (a) an individual has $0 and wins $100 (final state $100), or, (b) an individual is first endowed with $200 but then loses $100 of this endowment (final state $100).
- ^Assume an animal chooses between (a) two food items delivered after 2 s (rate: one item per second) and, (b), four food items delivered after 8 s (rate: 1/2 items per second; hence a > b). If both rewards were delayed by 10 s, the energy rate for option (a′) would change to 0.17 items per second and for option (b′) to 0.22 items per second. Optimal foraging theory predicts preference for a over b, but b′ over a′; DU prescribes consistent preference because of constant discounting, i.e., a over b and a′ over b′. Note that the rate maximization model can be modified to match DU, for instance by assuming a non-linear value function linking Darwinian fitness to energy gain.
- ^For example, assume a decision maker chooses between three gambles: (A) win $40 with probability p = 0.4, (B) win $45 with p = 0.35, and (C) win $50 with p = 0.3. This individual may consider the difference in probability between gambles A and B (Δp) = 0.05) too small to care about, and consequently chooses the gamble with the higher gain magnitude [Δ(gain) = $5], hence A > B. The same logic applies to choices between B and C, hence B > C. However, the difference in probability between A and C [(Δp) = 0.1] may exceed a cognitive “threshold,” and, because of the participant’s risk aversion, she may choose the safer gamble with the higher probability, hence C > A.
- ^For example, when given the choice between receiving $10 today, or $20 in a year, many individuals prefer $10 today. If both options were shifted into the future by, say, 50 years (now the choice is between $10 in 50 years and $20 in 51 years), most individuals would prefer the more delayed $20.
References
Ainslie, G. (1975). Specious reward: a behavioral theory of impulsiveness and impulse control. Psychol. Bull. 82, 463–496.
Ainslie, G., and Haslam, N. (1992). “Self-Control,” in Choice over Time, eds G. Loewenstein and J. Elster (New York: Russell Sage Foundation), 177–209.
Arkes, H. R., and Ayton, P. (1999). The sunk cost and concorde effects: are humans less rational than lower animals? Psychol. Bull. 125, 591–600.
Baron, J. (in press). “Parochialism as a result of cognitive biases,” in Understanding Social Action, Promoting Human Rights, eds R. Goodman, D. Jinks, and A. K. Woods (New York: Oxford University Press).
Barraclough, D. J., Conroy, M. L., and Lee, D. (2004). Prefrontal cortex and decision making in a mixed-strategy game. Nat. Neurosci. 7, 404–410.
Bateson, M., Healy, S. D., and Hurly, T. A. (2003). Context-dependent foraging decisions in rufous hummingbirds. Proc. Biol. Sci. 270, 1271–1276.
Bateson, M., and Kacelnik, A. (1996). Preferences for fixed and variable food sources: variability in amount and delay. J. Exp. Anal. Behav. 63, 313–329.
Bateson, M., and Kacelnik, A. (1998). “Risk-sensitive foraging: decision-making in variable environments,” in Cognitive Ecology, ed. R. Dukas (Chicago, IL: University of Chicago Press), 297–341.
Benzion, U., Rapoport, A., and Yagil, J. (1989). Discount rates inferred from decisions: an experimental study. Manage. Sci. 35, 270–284.
Bernoulli, D. (1954). Exposition of a new theory on the measurement of risk. Econometrica 22, 23–36.
Boettiger, C. A., Mitchell, J. M., Tavares, V. C., Robertson, M., Joslyn, G., D’Esposito, M., and Fields, H. L. (2007). Immediate reward bias in humans: fronto-parietal networks and a role for the catechol-O-methyltransferase 158(Val/Val) genotype. J. Neurosci. 27, 14383–14391.
Brandstätter, E., Gigerenzer, G., and Hertwig, R. (2006). The priority heuristic: making choices without trade-offs. Psychol. Rev. 113, 409–432.
Brosnan, S. F., Jones, O. D., Lambeth, S. P., Mareno, M. C., Richardson, A. S., and Schapiro, S. J. (2007). Endowment effects in chimpanzees. Curr. Biol. 17, 1704–1707.
Buckholtz, J. W., Treadway, M. T., Cowan, R. L., Woodward, N. D., Li, R., Ansari, M. S., Baldwin, R. M., Schwartzman, A. N., Shelby, E. S., Smith, C. E., Kessler, R. M., and Zald, D. H. (2010). Dopaminergic network differences in human impulsivity. Science 329, 532.
Camerer, C. F. (2003). Behavioral Game Theory: Experiments in Strategic Interaction, 1st Edn. Princeton, NJ: Princeton University Press.
Caraco, T. (1983). White-crowned sparrows (Zonotrichia leucophrys): foraging preferences in a risky environment. Behav. Ecol. Sociobiol. (Print) 12, 63–69.
Cardinal, R. N. (2006). Neural systems implicated in delayed and probabilistic reinforcement. Neural Netw. 19, 1277–1301.
Cardinal, R. N., Pennicott, D. R., Sugathapala, C. L., Robbins, T. W., and Everitt, B. J. (2001). Impulsive choice induced in rats by lesions of the nucleus accumbens core. Science 292, 2499–2501.
Cardinal, R. N., Robbins, T. W., and Everitt, B. J. (2000). The effects of d-amphetamine, chlordiazepoxide, alpha-flupenthixol and behavioural manipulations on choice of signalled and unsignalled delayed reinforcement in rats. Psychopharmacology (Berl.) 152, 362–375.
Charnov, E. L. (1976). Optimal foraging: the marginal value theorem. Theor. Popul. Biol. 9, 129–136.
Chu, Y. P., and Chu, R. L. (1990). The subsidence of preference reversals in simplified and marketlike experimental settings: a note. Am. Econ. Rev. 80, 902–911.
Chung, S. H., and Herrnstein, R. J. (1967). Choice and delay of reinforcement. J. Exp. Anal. Behav. 10, 67–74.
de Waal, F. B., Leimgruber, K., and Greenberg, A. R. (2008). Giving is self-rewarding for monkeys. Proc. Natl. Acad. Sci. U.S.A. 105, 13685–13689.
de Waal, F. B., and Suchak, M. (2010). Prosocial primates: selfish and unselfish motivations. Philos. Trans. R. Soc. Lond. B Biol. Sci. 365, 2711–2722.
Dorris, M. C., and Glimcher, P. W. (2004). Activity in posterior parietal cortex is correlated with the relative subjective desirability of action. Neuron 44, 365–378.
Durstewitz, D., Kröner, S., and Güntürkün, O. (1999). The dopaminergic innervation of the avian telencephalon. Prog. Neurobiol. 59, 161–195.
Evenden, J. L., and Ryan, C. N. (1996). The pharmacology of impulsive behaviour in rats: the effects of drugs on response choice with varying delays of reinforcement. Psychopharmacology (Berl.) 128, 161–170.
Fehr, E., and Fischbacher, U. (2002). Why social preferences matter – the impact of non-selfish motives on competition, cooperation and incentives. Econ. J. 112, C1–C33.
Fehr, E., and Schmidt, K. M. (1999). A theory of fairness, competition and cooperation. Q. J. Econ. 114, 817–868.
Floresco, S. B., Seamans, J. K., and Phillips, A. G. (1997). Selective roles for hippocampal, prefrontal cortical, and ventral striatal circuits in radial-arm maze tasks with or without a delay. J. Neurosci. 17, 1880–1890.
Floresco, S. B., St Onge, J. R., Ghods-Sharifi, S., and Winstanley, C. A. (2008). Cortico-limbic-striatal circuits subserving different forms of cost-benefit decision making. Cogn. Affect. Behav. Neurosci. 8, 375–389.
Frederick, S., Loewenstein, G., and O’Donoghue, T. (2002). Time discounting and time preference: a critical review. J. Econ. Lit. 40, 351–401.
Friedman, M., and Savage, L. J. (1948). The utility analysis of choices involving risk. J. Polit. Econ. 56, 279–304.
Friedman, M., and Savage, L. J. (1952). The expected-utility hypothesis and the measurability of utility. J. Polit. Econ. 60, 463–474.
Gan, J. O., Walton, M. E., and Phillips, P. E. (2009). Dissociable cost and benefit encoding of future rewards by mesolimbic dopamine. Nat. Neurosci. 13, 25–27.
Gigerenzer, G., and Goldstein, D. G. (1996). Reasoning the fast and frugal way: models of bounded rationality. Psychol. Rev. 103, 650–669.
Glimcher, P. W., Kable, J., and Louie, K. (2007). Neuroeconomic studies of impulsivity: now or just as soon as possible? Am. Econ. Rev. 97, 142–147.
Goldstein, D. G., and Gigerenzer, G. (2002). Models of ecological rationality: the recognition heuristic. Psychol. Rev. 109, 75–90.
Green, L., Fisher, E.B., Perlow, S., and Sherman, L. (1981). Preference reversal and self-control: choice as a function of reward amount and delay. Behav. Anal. Lett. 1, 43–51.
Green, L., Fristoe, N., and Myerson, J. (1994). Temporal discounting and preference reversals in choice between delayed outcomes. Psychon. Bull. Rev. 1, 383–389.
Grether, D. M., and Plott, C. R. (1979). Economic theory of choice and the preference reversal phenomenon. Am. Econ. Rev. 69, 623–638.
Hariri, A. R., Brown, S. M., Williamson, D. E., Flory, J. D., de Wit, H., and Manuck, S. B. (2006). Preference for immediate over delayed rewards is associated with magnitude of ventral striatal activity. J. Neurosci. 26, 13213–13217.
Hauser, M. D., Chen, M. K., Chen, F., and Chuang, E. (2003). Give unto others: genetically unrelated cotton-top tamarin monkeys preferentially give food to those who altruistically give food back. Proc. Biol. Sci. 270, 2363–2370.
Hayden, B. Y., Parikh, P. C., Deaner, R. O., and Platt, M. L. (2007). Economic principles motivating social attention in humans. Proc. Biol. Sci. 274, 1751–1756.
Hayden, B. Y., and Platt, M. L. (2007). Temporal discounting predicts risk sensitivity in rhesus macaques. Curr. Biol. 17, 49–53.
Haynes, L. (2009). Delaying Gratification. London: Parliamentary Office of Science and Technology of the United Kingdom, 1–4.
Herrnstein, R. J. (1961). Relative and absolute strength of response as a function of frequency of reinforcement. J. Exp. Anal. Behav. 4, 267–272.
Hertwig, R., Barron, G., Weber, E. U., and Erev, I. (2004). Decisions from experience and the effect of rare events in risky choice. Psychol. Sci. 15, 534–539.
Houston, A. I. (1997). Natural selection and context-dependent values. Proc. R. Soc. Lond. B Biol. Sci. 264, 1539–1541.
Hutchinson, J. M., and Gigerenzer, G. (2005). Simple heuristics and rules of thumb: where psychologists and behavioural biologists might meet. Behav. Processes 69, 97–124.
Isles, A.R., Humby, T., and Wilkinson, L.S. (2003). Measuring impulsivity in mice using a novel operant delayed reinforcement task: effects of behavioural manipulations and d-amphetamine. Psychopharmacol. (Berl). 170, 376–382.
Izawa, E., Aoki, N., and Matsushima, T. (2005). Neural correlates of the proximity and quantity of anticipated food rewards in the ventral striatum of domestic chicks. Eur. J. Neurosci. 22, 1502–1512.
Izawa, E., Zachar, G., Yanagihara, S., and Matsushima, T. (2003). Localized lesion of caudal part of lobus parolfactorius caused impulsive choice in the domestic chick: evolutionarily conserved function of ventral striatum. J. Neurosci. 23, 1894–1902.
Kable, J. W., and Glimcher, P. W. (2007). The neural correlates of subjective value during intertemporal choice. Nat. Neurosci. 10, 1625–1633.
Kacelnik, A. (1997). Normative and descriptive models of decision making: time discounting and risk sensitivity. Ciba Found. Symp. 208, 51–67; discussion 67–70.
Kacelnik, A. (2006). “Meanings of rationality,” in Rational Animals? eds M. Nudds and S. Hurley (Oxford: Oxford University Press), 87–106.
Kacelnik, A., and Bateson, M. (1996). Risky theories – the effects of variance on foraging decisions. Am. Zool. 36, 402–434.
Kacelnik, A., and Bateson, M. (1997). Risk-sensitivity: crossroads for theories of decision-making. Trends Cogn. Sci. (Regul. Ed.) 1, 304–309.
Kagel, J. H., Battalio, R. C., and Green, L. (1995). Economic Choice Theory – An Experimental Analysis of Animal Behavior, 1st Edn. Cambridge: Cambridge University Press.
Kagel, J. H., Battalio, R. C., Rachlin, H., Basmann, R. L., Green, L., and Klemm, W. R. (1975). Experimental studies of consumer demand behavior using laboratory animals. Econ. Inq. 13, 22–38.
Kagel, J. H., Battalio, R. C., Rachlin, H., and Green, L. (1981). Demand curves for animal consumers. Q. J. Econ. 96, 1–15.
Kahneman, D., and Tversky, A. (1979). Prospect theory: an analysis of decision under risk. Econometrica 47, 263–291.
Kahneman, D., and Tversky, A. (1996). On the reality of cognitive illusions. Psychol. Rev. 103, 583–591.
Kalenscher, T., Diekamp, B., and Güntürkün, O. (2003). Neural architecture of choice behaviour in a concurrent interval schedule. Eur. J. Neurosci. 18, 2627–2637.
Kalenscher, T., Ohmann, T., and Gunturkun, O. (2006a). The neuroscience of impulsive and self-controlled decisions. Int. J. Psychophysiol. 62, 203–211.
Kalenscher, T., Ohmann, T., Windmann, S., Freund, N., and Gunturkun, O. (2006b). Single forebrain neurons represent interval timing and reward amount during response scheduling. Eur. J. Neurosci. 24, 2923–2931.
Kalenscher, T., and Pennartz, C. (2010). “Do intransitive choices reflect genuinely context-dependent preferences,” in Attention and Performance XIII: Decision Making, eds M. R. Delgado, E. Phelps, and T. Robbins (Vermont: Oxford University Press), 101–124.
Kalenscher, T., and Pennartz, C. M. (2008). Is a bird in the hand worth two in the future? The neuroeconomics of intertemporal decision-making. Prog. Neurobiol. 84, 284–315.
Kalenscher, T., and Tobler, P. N. (2008). “Comparing risky and inter-temporal decisions; views from psychology, ecology and microeconomics,” in Psychology of Decision Making in Economics, Business and Finance, ed. K. P. Hoffmann (New York: Nova Science Publishers), 111–135.
Kalenscher, T., Tobler, P. N., Huijbers, W., Daselaar, S. M., and Pennartz, C. M. (2010). Neural signatures of intransitive preferences. Front. Hum. Neurosci. 4:49. doi: 10.3389/fnhum.2010.00049
Kalenscher, T., Windmann, S., Diekamp, B., Rose, J., Güntürkün, O., and Colombo, M. (2005). Single units in the pigeon brain integrate reward amount and time-to-reward in an impulsive choice task. Curr. Biol. 15, 594–602.
Kim, S., Hwang, J., and Lee, D. (2008). Prefrontal coding of temporally discounted values during intertemporal choice. Neuron 59, 161–172.
Kirby, K. N., and Herrnstein, R. J. (1995). Preference reversals due to myopic discounting of delayed reward. Psychol. Sci. 6, 83–89.
Knapp, R. K., Kause, R. H., and Perkins, C. C. (1959). Immediate vs. delayed shock in t-maze performance. J. Exp. Psychol. 58, 357–362.
Kolmer, K., and Heinze, J. (2000). Rank orders and division of labour among unrelated cofounding ant queens. Proc. Biol. Sci. 267, 1729–1734.
Leon, M. I., and Shadlen, M. N. (1998). Exploring the neurophysiology of decisions. Neuron 21, 669–672.
Loewenstein, G. (1987). Anticipation and the valuation of delayed consumption. Econ. J. 97, 666–684.
Louie, K., and Glimcher, P. W. (2010). Separating value from choice: delay discounting activity in the lateral intraparietal area. J. Neurosci. 30, 5498–5507.
MacDonald, D. H., Kagel, J. H., and Battalio, R. C. (1991). Animals’ choices over uncertain outcomes, further experimental results. Econ. J. 101, 1065–1084.
Marsh, B., and Kacelnik, A. (2002). Framing effects and risky decisions in starlings. Proc. Natl. Acad. Sci. U.S.A. 99, 3352–3355.
McClure, S. M., Ericson, K. M., Laibson, D. I., Loewenstein, G., and Cohen, J. D. (2007). Time discounting for primary rewards. J. Neurosci. 27, 5796–5804.
McClure, S. M., Laibson, D. I., Loewenstein, G., and Cohen, J. D. (2004). Separate neural systems value immediate and delayed monetary rewards. Science 306, 503–507.
McCoy, A. N., and Platt, M. L. (2005). Risk-sensitive neurons in macaque posterior cingulate cortex. Nat. Neurosci. 8, 1220–1227.
McDiarmid, C. G., and Rilling, M. E. (1965). Reinforcement delay and reinforcement rate as determinants of schedule preference. Psychon. Sci. 2, 195–196.
Melis, A. P., Warneken, F., Jensen, K., Schneider, A. C., Call, J., and Tomasello, M. (2011). Chimpanzees help conspecifics obtain food and non-food items. Proc. Biol. Sci. 278, 1405–1413.
Mezey, S., and Csillag, A. (2002). Selective striatal connections of midbrain dopaminergic nuclei in the chick (Gallus domesticus). Cell Tissue Res. 308, 35–46.
Navarick, D. J., and Fantino, E. (1972). Transitivity as a property of choice. J. Exp. Anal. Behav. 18, 389–401.
Navarick, D. J., and Fantino, E. (1974). Stochastic transitivity and unidimensional behavior theories. Psychol. Rev. 81, 426–441.
Navarick, D. J., and Fantino, E. (1975). Stochastic transitivity and the unidimensional control of choice. Learn. Motiv. 6, 179–201.
Navarro, A. D., and Fantino, E. (2005). The sunk cost effect in pigeons and humans. J. Exp. Anal. Behav. 83, 1–13.
Padoa-Schioppa, C., and Assad, J. A. (2006). Neurons in the orbitofrontal cortex encode economic value. Nature 441, 223–226.
Pennartz, C. M., Groenewegen, H. J., and Lopes da Silva, F. H. (1994). The nucleus accumbens as a complex of functionally distinct neuronal ensembles: an integration of behavioural, electrophysiological and anatomical data. Prog. Neurobiol. 42, 719–761.
Phillips, P. E., Stuber, G. D., Heien, M. L., Wightman, R. M., and Carelli, R. M. (2003). Subsecond dopamine release promotes cocaine seeking. Nature 422, 614–618.
Pine, A., Shiner, T., Seymour, B., and Dolan, R. J. (2010). Dopamine, time, and impulsivity in humans. J. Neurosci. 30, 8888–8896.
Platt, M. L., and Glimcher, P. W. (1999). Neural correlates of decision variables in parietal cortex. Nature 400, 233–238.
Prelec, D., and Loewenstein, G. (1991). Decision making over time and under uncertainty: a common approach. Manage. Sci. 37, 770–786.
Rachlin, H., and Green, L. (1972). Commitment, choice and self control. J. Exp. Anal. Behav. 17, 15–22.
Rachlin, H., Logue, A. W., Gibbon, J., and Frankel, M. (1986). Cognition and behavior in studies of choice. Psychol. Rev. 93, 33–45.
Raihani, N. J., Grutter, A. S., and Bshary, R. (2010). Punishers benefit from third-party punishment in fish. Science 327, 171.
Ratnieks, F. L., and Helantera, H. (2009). The evolution of extreme altruism and inequality in insect societies. Philos. Trans. R. Soc. Lond. B Biol. Sci. 364, 3169–3179.
Reboreda, J. C., and Kacelnik, A. (1991). Risk sensitivity in starlings: variability in food amount and food delay. Behav. Ecol. 2, 301–308.
Reiner, A., Brauth, S. E., and Karten, H. J. (1984). Evolution of the amniote basal ganglia. Trends Neurosci. 7, 320–325.
Rilling, J., Gutman, D., Zeh, T., Pagnoni, G., Berns, G., and Kilts, C. (2002). A neural basis for social cooperation. Neuron 35, 395–405.
Roelofsma, P. H. M. P., and Read, D. (2000). Intransitive intertemporal choice. J. Behav. Decis. Mak. 13, 161–177.
Roesch, M. R., Taylor, A. R., and Schoenbaum, G. (2006). Encoding of time-discounted rewards in orbitofrontal cortex is independent of value representation. Neuron 51, 509–520.
Rosati, A. G., Stevens, J. R., Hare, B., and Hauser, M. D. (2007). The evolutionary origins of human patience: temporal preferences in chimpanzees, bonobos, and human adults. Curr. Biol. 17, 1663–1668.
Rueppell, O., Hayworth, M. K., and Ross, N. P. (2010). Altruistic self-removal of health-compromised honey bee workers from their hive. J. Evol. Biol. 23, 1538–1546.
Russo, J. E., and Dosher, B. A. (1983). Strategies for multiattribute binary choice. J. Exp. Psychol. Learn. Mem. Cogn. 9, 676–696.
Rutte, C., and Taborsky, M. (2007). Generalized reciprocity in rats. PLoS Biol. 5, e196. doi: 10.1371/journal.pbio.0050196
Rutte, C., and Taborsky, M. (2008). The influence of social experience on cooperative behaviour of rats (Rattus norvegicus): direct vs generalised reciprocity. Behav. Ecol. Sociobiol. (Print) 62, 499–505.
Santos, L. R., and Keith Chen, M. (2009). “The evolution of rational and irrational economic behavior: evidence and insight from a non-human primate species,” in Neuroeconomics – Decision Making and the Brain, 1st Edn, eds P. W. Glimcher, C. F. Camerer, E. Fehr, and R. A. Poldrack (London: Academic Press), 81–93.
Shafir, S. (1994). Intransitivity of preferences in honey bees: support for “comparative” evaluation of foraging options. Anim. Behav. 48, 55–67.
Shafir, S., Reich, T., Tsur, E., Erev, I., and Lotem, A. (2008). Perceptual accuracy and conflicting effects of certainty on risk-taking behaviour. Nature 453, 917–920.
Stephens, D. W. (2002). Discrimination, discounting and impulsivity: a role for an informational constraint. Philos. Trans. R. Soc. Lond. B Biol. Sci. 357, 1527–1537.
Stephens, D. W. (2008). Decision ecology: foraging and the ecology of animal decision making. Cogn. Affect. Behav. Neurosci. 8, 475–484.
Stephens, D. W., and Anderson, D. (2001). The adaptive value of preference for immediacy: when shortsighted rules have farsighted consequences. Behav. Ecol. 12, 330–339.
Stephens, D. W., Kerr, B., and Fernandez-Juricic, E. (2004). Impulsiveness without discounting: the ecological rationality hypothesis. Proc. R. Soc. Lond. B Biol. Sci. 271, 2459–2465.
Stephens, D. W., and Krebs, J. R. (1986). Foraging Theory. Monographs in Behavior and Ecology. Princeton, NJ: University Press.
Stephens, D. W., McLinn, C. M., and Stevens, J. R. (2002). Discounting and reciprocity in an iterated prisoner’s dilemma. Science 298, 2216–2218.
Sugrue, L. P., Corrado, G. S., and Newsome, W. T. (2004). Matching behavior and the representation of value in the parietal cortex. Science 304, 1782–1787.
Thaler, R. H., and Shefrin, H. M. (1981). An economic theory of self-control. J. Polit. Econ. 89, 392–406.
Tobler, P. N., Fiorillo, C. D., and Schultz, W. (2005). Adaptive coding of reward value by dopamine neurons. Science 307, 1642–1645.
van den Bos, R., Houx, B. B., and Spruijt, B. M. (2006a). The effect of reward magnitude differences on choosing disadvantageous decks in the Iowa gambling task. Biol. Psychol. 71, 155–161.
van den Bos, R., Lasthuis, W., den Heijer, E., van der Harst, J., and Spruijt, B. (2006b). Toward a rodent model of the Iowa gambling task. Behav. Res. Methods 38, 470–478.
Varian, H. R. (2006). Intermediate Microeconomics – A Modern Approach, 7th Edn. New York: W.W. Norton & Company.
Viana, D. S., Gordo, I., Sucena, E., and Moita, M. A. (2010). Cognitive and motivational requirements for the emergence of cooperation in a rat social game. PLoS ONE 5, e8483. doi:10.1371/journal.pone.0008483
von Neumann, J., and Morgenstern, O. (1944). Theory of Games and Economic Behavior. Princeton, NJ: University Press.
Waite, T. A. (2001). Intransitive preferences in hoarding gray jays (Perisoreus canadensis). Behav. Ecol. Sociobiol. (Print) 50, 116–121.
Yanagihara, S., Izawa, E., Koga, K., and Matsushima, T. (2001). Reward-related neuronal activities in basal ganglia of domestic chicks. Neuroreport 12, 1431–1435.
Keywords: neuroeconomics, decision making, animals, reward, optimal foraging, behavioral ecology, ethology, rational
Citation: Kalenscher T and van Wingerden M (2011) Why we should use animals to study economic decision making – a perspective. Front. Neurosci. 5:82. doi: 10.3389/fnins.2011.00082
Received: 13 April 2011;
Paper pending published: 02 May 2011;
Accepted: 06 June 2011;
Published online: 17 June 2011.
Edited by:
Michael Platt, Duke University, USAReviewed by:
Veit Stuphorn, Johns Hopkins University, USABenjamin Hayden, Duke University Medical Center, USA
Copyright: © 2011 Kalenscher and van Wingerden. This is an open-access article subject to a non-exclusive license between the authors and Frontiers Media SA, which permits use, distribution and reproduction in other forums, provided the original authors and source are credited and other Frontiers conditions are complied with.
*Correspondence: Tobias Kalenscher, Department of Comparative Psychology, Institute of Experimental Psychology, Heinrich-Heine University Düsseldorf, Universitätsstrasse 1, 40225 Düsseldorf, Germany. e-mail: tobias.kalenscher@gmail.com