- 1 Team PhyPA, Berlin Institute of Technology, Berlin, Germany
- 2 Swartz Center for Computational Neuroscience, Institute for Neural Computation, University of California San Diego, La Jolla, CA, USA
- 3 Brain Products, Gilching, Germany
- 4 Human Media Interaction, University of Twente, Enschede, Netherlands
Although it ranks among the oldest tools in neuroscientific research, electroencephalography (EEG) still forms the method of choice in a wide variety of clinical and research applications. In the context of brain–computer interfacing (BCI), EEG recently has become a tool to enhance human–machine interaction. EEG could be employed in a wider range of environments, especially for the use of BCI systems in a clinical context or at the homes of patients. However, the application of EEG in these contexts is impeded by the cumbersome preparation of the electrodes with conductive gel that is necessary to lower the impedance between electrodes and scalp. Dry electrodes could provide a solution to this barrier and allow for EEG applications outside the laboratory. In addition, dry electrodes may reduce the time needed for neurological exams in clinical practice. This study evaluates a prototype of a three-channel dry electrode EEG system, comparing it to state-of-the-art conventional EEG electrodes. Two experimental paradigms were used: first, event-related potentials (ERP) were investigated with a variant of the oddball paradigm. Second, features of the frequency domain were compared by a paradigm inducing occipital alpha. Furthermore, both paradigms were used to evaluate BCI classification accuracies of both EEG systems. Amplitude and temporal structure of ERPs as well as features in the frequency domain did not differ significantly between the EEG systems. BCI classification accuracies were equally high in both systems when the frequency domain was considered. With respect to the oddball classification accuracy, there were slight differences between the wet and dry electrode systems. We conclude that the tested dry electrodes were capable to detect EEG signals with good quality and that these signals can be used for research or BCI applications. Easy to handle electrodes may help to foster the use of EEG among a wider range of potential users.
Introduction
To address the issues that arise with our aging society and its related health problems it is likely that we will need technologies to aid persons in their daily work and life (assistive technology, human–computer interaction), to help persons stay physically and mentally fit as long as possible (primary prevention) and have early detection of diseases or disorders. Chatterjee and Price (2009) argue that these technologies will need to be persuasive in order for users to adhere to their use. They predict that more user-aware, ambient-aware, and context-aware smart technologies can be expected that will increase persuasion. One of the traditional tools to assess user states and users’ intentions is the use of electroencephalography (EEG) as one of the oldest imaging techniques used in clinical diagnosis and cognitive neuroscience. First described in the 1920s (Berger, 1929), a large share of neurophysiogical studies still use EEG as method of choice, because recording is non-invasive and has a high temporal resolution superior to other non-invasive methods. With the emergence of the new research field of brain–computer interfaces (BCIs), the EEG was applied as an input channel to control assistive technology for persons with physical disabilities rather than as a research tool (Wolpaw et al., 2002; Dornhege et al., 2007). Recently, features of the EEG are used as input to model user’s states to adapt human–machine interaction (HMI, Krepki et al., 2007; Cutrell and Tan, 2008; Müller et al., 2008; Zander et al., 2008, 2010; Williamson et al., 2009; Blankertz et al., 2010a; Zander and Kothe, 2011).
Several studies have shown the feasibility of BCIs as alternatives to augmented communication in severely paralyzed and even locked-in patients (Birbaumer et al., 1999; Vaughan et al., 2006; Hoffmann et al., 2008; Nijboer et al., 2008). However, these promising results remain in the scientific drawers of academics and do not reach the market. One reason for this is the unpractical usage of BCIs at home. A major concern is the long preparation time of conventional EEG systems and the use of electrode gel necessary for lowering the impedance between the electrodes and the participants’ scalp. We will refer to these electrodes as “wet” electrodes. Depending on the number of channels used, the preparation time to apply wet electrodes can vary between 20 and 60 or even more minutes. Moreover, participants can suffer from skin irritation due to long-term usage. Furthermore, when BCIs are tested with or developed for persons with severe motor paralysis, EEG is often measured while the person is sitting in a wheelchair with the head leaning against the head support. In some cases the person is even lying in bed resting the head on a pillow. Even though these patients may opt to accept the long preparation time of “wet” electrodes in return for reliable communication, they may not have the ability to wash their hair independently to remove electrode gel after BCI use. Caregivers and/or family members can wash the hair, but since this procedure can take up to an hour in a locked-in patient, current BCIs are not usable (Broermann and Nijboer, 2010). In addition, more recent BCI research aims at integrating BCI applications into human–machine systems (HMS) for healthy people (Müller et al., 2008; Zander et al., 2010, 2011). Especially in this context, EEG systems need to become easier to use with a reduced configuration phase. The application of dry electrodes could lower preparation time to only a few minutes, since the use of electrode gel is omitted. This would be the first step toward a wearable electrode system. These systems would also be beneficial for other aspects of EEG research, as described in the following section.
Casson et al. (2010) stated that the development of wearable electrodes would lead to new approaches to application of EEG in clinical practice. They suggest that wearable electrodes may be very important in long-term inpatient monitoring for example to diagnose epilepsy or sleep disorders. The authors held a survey among 21 neurologists. Eighteen neurologists agreed that there is a clinical need for wearable electrodes and 16 would consider it a major improvement in their EEG practice if wearable EEG devices were available. They believe that wearable EEG would allow a more natural sleep in sleep studies. Moreover, 10 neurologists could imagine using EEG for non-medical applications such as control of computer games or receiving feedback based upon a person’s current awareness level.
To the authors’ knowledge, there are three prototypes of such dry electrode systems: first of all, Popescu et al. (2007) designed an array of specially coated contacts comprising six electrode channels. The system was evaluated in an online BCI experiment where participants had to accomplish a motor imagination task to control a computer cursor. Performance of the dry system was compared to a traditional wet one with 64 electrodes, resulting in a degradation of the bit rate by 30%. Oehler et al. (2008) developed a capacitive EEG system with 28 channels. In a BCI experiment using steady state visually evoked potentials (SSVEPs), they compared their electrode system to a conventional one. Transferring the same information took three times longer with the capacitive system. A third system using multi-walled carbon nanotube arrays was introduced in 2007 by Ruffini et al. (2007). The system was evaluated comparing it to a wet system in a standard EEG paradigm, however, only in a few trials with one human participant. Therefore, general conclusions about the system’s performance could not be made so far. Consequently, none of these three dry electrode systems can ensure an EEG measurement that is comparable to standard EEG measurements, which is a necessity for an application of dry electrode systems in a wider context.
Here, we will now introduce a dry electrode system developed by Brain Products (Gilching, Germany). This system contains three electrodes that can be placed over the occipital lobe at the electrode positions PO7, Oz, and PO8 of the standard 10–20-system. There is no need for conductive gel; hence preparation time is reduced to roughly 5 min and it is not required to wash the hair after use. The system does not pose any health risk for the user.
The aim of the current study is to evaluate the signal quality of the dry electrode system for EEG analysis and for BCI applications. The performance of the dry electrodes was compared to a conventional wet electrode cap (actiCap, Brain Products) containing three electrodes at comparable positions, ensuring simultaneous signal acquisition of both systems. Two experiments were conducted employing neuroscientific standard paradigms, eliciting event-related potentials (ERP) and spontaneous EEG. A variant of the oddball paradigm was used to investigate ERP features (Holroyd, 2004). P300 ERPs have already been successfully used in BCI applications, e.g., the P300-speller (Farwell and Donchin, 1988; Sellers et al., 2003; Piccione et al., 2006; Sellers and Donchin, 2006; Hoffmann et al., 2008; Nijboer et al., 2008). Spontaneous EEG features were investigated by an experimental task inducing concentration (cognitive engagement) and relaxation in alternation, thus causing different levels of band power in the α-band (7–13 Hz) over occipital electrode sites (e.g., Birbaumer and Schmidt, 2005). Like ERPs, these variations of frequency power can be used in BCI applications, e.g., in the context of gaming (Nijholt et al., 2008). The averaged signals of both experiments were analyzed with standard EEG analysis. In addition, they were analyzed and classified offline on single-trial basis to evaluate their possible applicability in the field of BCI.
Dry Electrode System
The dry electrode system used in this study is essentially a modified version of the Brain Products actiCAP system with electrodes adapted in such a way as to establish a direct contact with the skin without relying on conductive gel. For this, electrodes are manufactured as a comb-like structure with a diameter of 10 mm featuring 12 small pins of 4 mm length and 2 mm diameter. For the frontal electrodes applied to the forehead, cup electrodes of 10 mm diameter are used (see Figure 1).
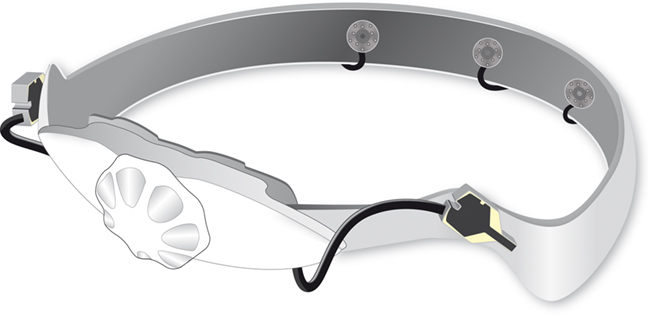
Figure 1. Sketch of the prototype dry electrode cap. The three comb-like electrodes are placed inside in the back of the cap. Ground and reference are placed laterally in the front. Moreover, there is a wheel to tighten the cap to the participants’ head.
For signal processing both digital and analog methods are employed. Digital signal processing is responsible for impedance matching and LED control, while signal transmission from the recording site to the amplifier is realized by analog methods. An optional noise suppressor is available (however, this option was not used in this study). The two frontal electrodes of the system are used as reference and ground. Signal electrodes can be variably placed on the adjustable headband. When attaching the headband, reference and ground electrodes exert a slight pressure on the forehead thus forming contact with the skin. For the signal electrodes, a conductive connection to the scalp is realized via the electrode pins that penetrates through the participants’ hair.
The current electrode locations are restricted to parietal and occipital areas. These locations are not typical in many BCI paradigms that rely on data from areas over the motor cortex or frontal midline. But, if the signals recorded at parietal areas are comparable with data from wet electrodes, it indicates that this will also be possible at other areas of the cortex. The follow up version of the prototype tested here, which is currently under development at Brain Products will also be applicable to other standard positions of the international 10/20-system, including C3, C4, Pz, Fz, and Cz electrode positions, widely used in neuroscientific and BCI research.
Experiment 1 – Oddball
Methods
Participants
Twelve students of the Berlin Institute of Technology (aged 20–28 years, seven female) took part in the experiment. All of them were neurologically healthy and reported normal or corrected-to-normal vision. They were paid 10 Euros and gave written consent to participate.
Procedure
Participants were seated comfortably in front of a monitor, wearing the two different electrode caps simultaneously. The task was explained to participants by written instructions on the screen. A simplified version of the oddball paradigm was used including four blocks, each of 2-min duration. In each block the stimulus had a different starting position. After each block, participants were instructed to name the number of deviants that occurred.
Paradigm
The experimental task was similar to classical oddball paradigms, presenting rare stimuli (deviants) in a sequence of frequent stimuli (standards; Schall, 2001). Oddball paradigms are widely used in neuroscientific research to investigate an ERP referred to as the N200 P300 complex (Polich and Kok, 1995; Linden, 2005). An EEG pattern reflecting quite similar brain mechanisms (detecting rare targets among frequent stimuli) is utilized for a certain class of BCI paradigms, as the P300 speller (Farwell and Donchin, 1988).
At the beginning of a trial a circle divided by lines into 30° angles appeared on the screen (similar to the marks on a clock, see Figure 2). The standard stimulus was a bar appearing at one of four possible starting positions (3, 6, 9, or 12 o’clock) rotating 90° (standard condition). This frequent stimulus was interrupted by a rare rotation (deviant condition), performing a snap movement. The bar rotated in a 60° angle, followed by a snap movement back to the 45° position after 100 ms. Odd rotations occurred with a probability of p = 0.1. Rotation of the bar was instantaneous; the inter-stimulus interval (ISI) between two starting figures was 1000 ms. Hence, this paradigm resulted in a 2 (tasks) × 2 (electrode system) × 3 (channels) factorial design.
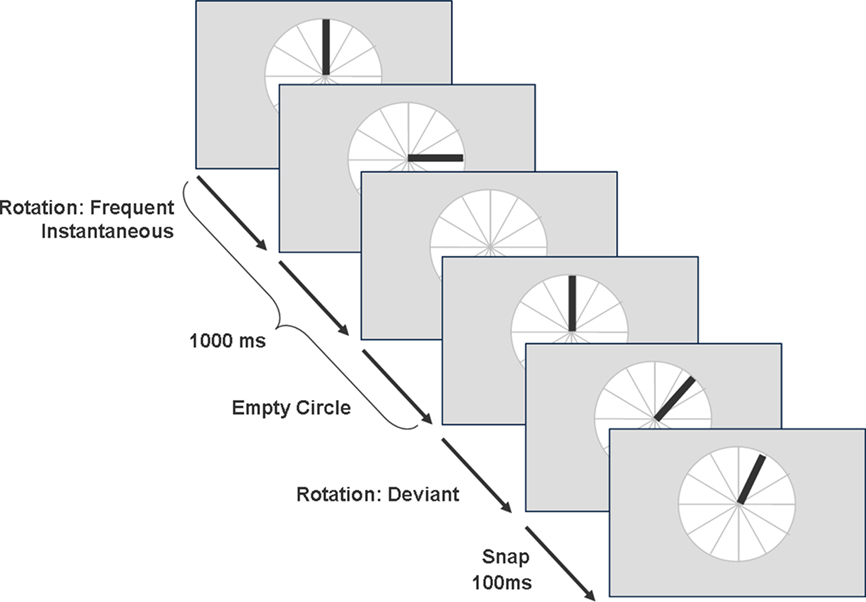
Figure 2. Example trials for the experimental task of the Oddball paradigm showing the standard and deviant condition.
Each of the four experimental blocks consisted of 100 trials, 90 trials of standard stimuli and 10 trials of deviant stimuli respectively, summing up to 360 trials for the standard condition and 40 deviant trials for the deviant condition over the whole experimental session.
EEG recording
Electroencephalography was recorded with two different electrode types:
On the one hand, an actiCap (Brain Products) with three standard active electrodes at occipital positions (PO7, Oz, PO8), an active ground and reference electrode at frontal positions (Fp1, Fp2) was used. On the other hand, we used the dry electrode system described above (equipped with three dry active electrodes at the same occipital positions, plus a dry active ground and reference electrode at the same frontal positions). Electrodes were placed at positions PO7, PO8, and Oz, according to the standard 10–20 system. Since both electrode caps were used simultaneously for EEG recording, one cap was placed at standard positions and the other cap approximately 1.5 cm above these positions. Upper and lower positions of the electrode sets were counterbalanced across participants. EEG was recorded at a sample rate of 500 Hz. Impedances of all electrodes were kept below 20 kΩ which is a standard calibration according to the specifications of the actiCap. The standard actiCap electrodes were connected to a 32-channel-amplifier BrainAmp DC by Brain Products. For the dry electrodes the 8-channel-amplifier V-Amp (Brain Products) was used. Both amplifiers share the same level of input noise (≤1 μVpp), hence, using two different amplifiers should not have an impact on the results of this study.
Pre-processing
To obtain ERP measures EEG was visually inspected for artifacts. EEG data containing muscle artifacts or eye blinks were excluded from further analysis. Artifact removal was performed on wet electrode data and then transferred automatically to the dry electrode data. We decided for this conservative approach to retain a standardized methodology for ERP analyses and to ensure validity of a positive assessment of dry electrodes. With defining artifact periods from the baseline of the wet electrode data, artifacts additionally generated by the usage of the dry electrode technology will be reflected in our results. A band pass filter of 0.1–15 Hz was applied. Epochs of 1000 ms length, stimulus-locked to standard stimulus rotation or deviant (odd) stimulus rotation, were extracted. A 200-ms pre-stimulus interval was used for baseline correction.
ERP measures
An ERP analysis was conducted on the data of the oddball paradigm in order to compare standard ERP measures as peak amplitude and peak latency between dry and wet electrode data. Grand average ERPs and difference curves (deviant-minus-standard) were calculated for dry and wet electrode EEG data, to obtain time windows for oddball related potentials.
According to the grand average ERPs (see Figure 3), the time window for the negativity was defined as 250–400 ms after stimulus onset; for the positivity a time window of 450–600 ms was chosen. Difference curves were calculated and plotted for each participant individually. ERP peak amplitude and latency of the difference curves were determined for each participant by choosing the minimum amplitude value for the negativity in the expected time window (250–400 ms) and the maximum amplitude value for the expected time window of the positivity (450–600 ms). Difference peak latencies were calculated for each participant, subtracting mean peak latency of the negativity by the mean peak latency of the positivity. The open source toolbox EEGLAB (Delorme and Makeig, 2004) and MATLAB (The Mathworks, Natick, USA) were utilized for pre-processing, ERP analysis, and visualization of ERP data.
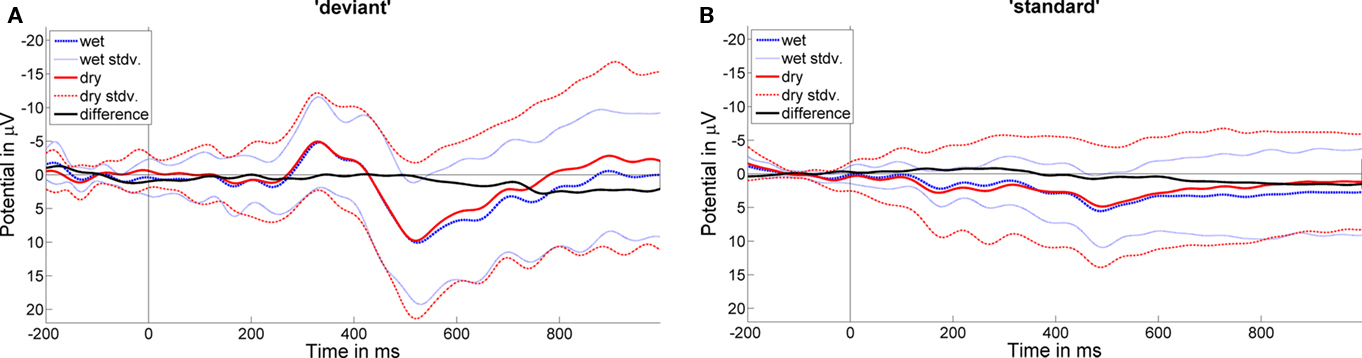
Figure 3. Grand average ERPs (12 subjects) for deviant (A) and standard (B) trials are shown for channel PO8. ERPs for dry (red) and wet (blue) electrode data are plotted together with a difference curve (black) for different data (dry-minus-wet). Dotted lines show the SDs for the grand average ERPs.
BCI analysis
The BCI single-trial analysis was conducted by calculating the offline classification error using a 10 × 5-fold cross-validation (Bishop, 2006; Blankertz et al., 2007; Zander et al., 2011) to estimate BCI classification accuracy. This procedure implies a randomized 10-fold cross-validation, which is repeated five times and the average of these results is taken as an estimation of classification accuracy. This results in less random fluctuation of the classification accuracy estimation. EEG data were downsampled to 100 Hz, epochs were generated from 0 to 800 ms relative to stimulus onset and a 0.1–15 Hz FFT band pass filter was applied. For classification a modified version of the pattern matching method (Blankertz et al., 2002, 2010b; Zander et al., 2011) was utilized. Trials were partitioned into sixtime windows of 50 ms length according to the shape of the ERP. Calculating the mean of each time window and single trial for the three channels resulted in an 18-dimensional feature space (3 channels × 6 time windows). As a classifier, regularized linear discriminant analysis (rLDA) was used. BCI analysis was conducted with the PhyPA BCI toolbox (Zander et al., 2008, 2011).
Statistical analysis
Differences of negative and positive peak amplitudes between dry and wet electrode data were tested for significance by conducting a 2 (electrode type) × 3 (channels) repeated-measures ANOVA, with two within-subject factors. An ANOVA was calculated for each dependent measure: the peak amplitude of the negativity and positivity of the according difference curve. Greenhouse–Geisser corrected degrees of freedom and significant values were used when the assumption of sphericity was not met. Since the goal was to fulfill the null hypothesis, i.e., to show that there are no differences in ERP measures between dry and wet EEG data, the alpha significance level was set to p > 0.1 to control for the beta error (Bortz, 2005). Correlations between dry and wet difference latencies were obtained by calculating bivariate correlations (Pearson correlation), which were tested for significance by a one sample t-test against zero. The probability of getting a correlation as large as the resulting one by chance, if true correlation was zero, was evaluated against a significance level of 0.05. Classification accuracies were tested for significant differences between wet and dry electrode data by using a paired sample t-test.
Results
ERP measures
Figure 2 shows grand average ERPs for wet and dry electrode data for standard and deviant trials at electrode PO8. The respective difference curves are plotted to show differences in ERP shape for the two electrode systems. The morphology of the dry vs. wet mean ERPs is almost identical except for some differences in SD revealing a higher SD for the dry electrode data in the standard condition.
For the peak amplitude measure, analysis of variances revealed that neither the main factor of electrode type [dry vs. wet electrode system; negativity: F(11) = 0.2, p > 0.1, positivity: F(11) = 0.15, p > 0.1], nor the factor channel [PO7, Oz, PO8; negativity: F(11) = 1.54, p > 0.1, positivity: F(11) = 1.87, p > 0.1] had significant effects on the peak amplitude. Accordingly, there were no significant differences between wet and dry electrode data or channels. Table 1 shows mean values and SD for the respective peak amplitude values and difference peak latencies.
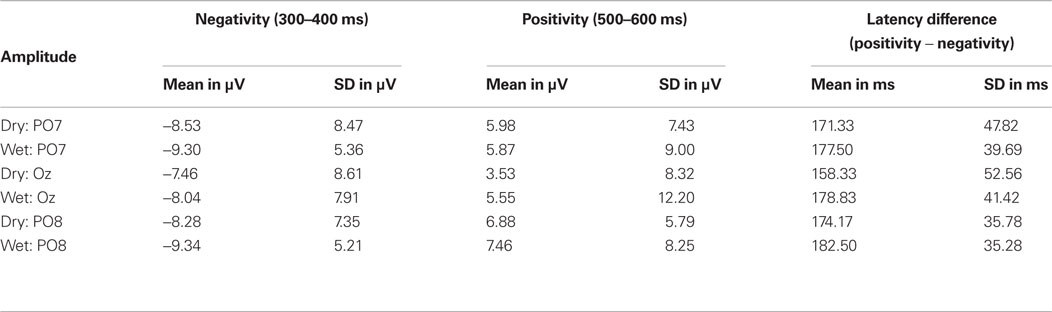
Table 1. Event-related potential results. Mean values and SD for peak amplitudes and latency differences for the negativity and positivity.
The mean difference peak latency (positive minus negative peak latency) was 171.33 ms for dry vs. 177.50 ms for wet electrode data at channel PO7, 158.33 ms (dry) vs. 178.83 ms (wet) at channel Oz and 174.17 ms (dry) vs. 182.50 ms (wet) at channel PO8. Bivariate correlations for difference peak latencies between dry and wet electrode data were high and significant for all electrodes (PO7: r = 0.64, p < 0.05; Oz: r = 0.65, p < 0.05; PO8: r = 0.65, p < 0.05).
BCI analysis
Classification results are shown in Table 2, mean classification accuracy was 77.8% for the wet and 72.1% for the dry electrode data. Classification results for all participants are shown in Table 2. Differences in classification accuracy between wet and dry electrode data [t(11) = −2.64, p < 0.05] were significant. The accuracy difference was approximately 6%.
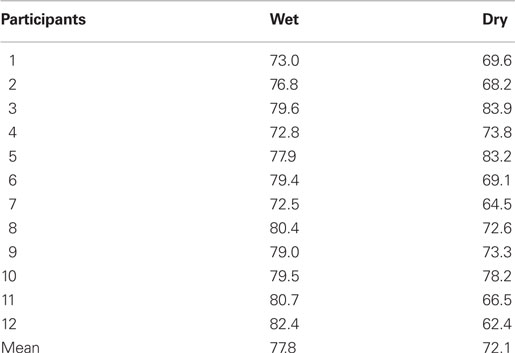
Table 2. Results of BCI analysis. Shown is the offline classification accuracy, calculated by cross-validation for each participant for dry and wet electrode data.
Discussion
The grand average ERP morphology for dry and wet electrode data is almost identical except for a higher SD for dry electrode data in the standard condition. This reveals that the signal-to-noise ratio for the dry electrode EEG data is not as good as for the wet electrode EEG data, still showing only slight differences in the grand average. Statistical analysis of standard ERP measures revealed no significant differences between wet and dry electrode EEG data with respect to peak amplitudes. In addition, mean difference peak latencies (positivity minus negativity) showed a high significant correlation between wet and dry electrode data across subjects. This indicates that there is no time lag induced by the dry electrode system. It can be assumed that the dry electrodes are suitable for standard ERP analysis, with respect to ERP measures investigated here.
Statistical analysis of the results of the BCI classification accuracy revealed significant differences between dry and wet electrode data. Regarding BCI single-trial analysis, the results of the dry electrode data were deteriorated by approximately 6% classification accuracy with a mean of 72.1% (dry) compared to 77.8% (wet) classification accuracy. The used paradigm was a rotating stimulus inducing an oddball ERP with a delayed timing and different morphology compared to standard oddball paradigms. Nonetheless, all measures, standard ERP peak amplitude and latency as well as BCI classification accuracy revealed that both EEG systems lead to comparable analysis results in the ERP domain.
Experiment 2 – Alpha Paradigm
Methods
Participants
Like in Experiment 1, 12 students (some of them had already participated in the first experiment) of the Berlin Institute of Technology (age: 20–28 years, eight female) took part in the experiment. All of them were neurologically healthy and reported normal or corrected-to-normal vision. They were paid 10 Euros and gave written consent to participate.
Procedure
Participants performed four blocks of the alpha paradigm described below, with a duration of 5 min each, including short breaks between blocks.
Paradigm
The experimental paradigm included two conditions (“relaxed” vs. “engaged”). In the “relaxed” condition participants were instructed to relax and close their eyes for 6 s, indicated by the phrase “Please relax now.” appearing on the screen (see Figure 4). The starting point for this period was cued by a high tone, the end by a lower tone. The task of the “engaged” condition was to find a word by concatenating letters appearing shortly at random locations on screen, indicated by “Do you recognize the word?”. These letters were successively shown on a dynamic background with different gray-scaled shades in a 6-s period. Shades were changing shape and color which made letter detection difficult and strenuous. In addition, letters had to be memorized until a word was recognized putting load on working memory during this condition in contrast to the “relaxed” condition. In the end of each trial, participants were instructed to name the recognized word. In sum, participants performed 52 trials of the “relaxed” condition and 52 trials of the “engaged” condition randomly distributed across the four experimental blocks. This paradigm reflects a common strategy used in psychophysiological experiments and induces an event-related desynchronization (ERD) of the alpha rhythm recorded at parietal areas. As ERD is a common feature used in BCI paradigms (Pfurtscheller et al., 1997), the results from this paradigm are a good indicator whether the tested electrodes will be applicable in frequency based BCI applications.
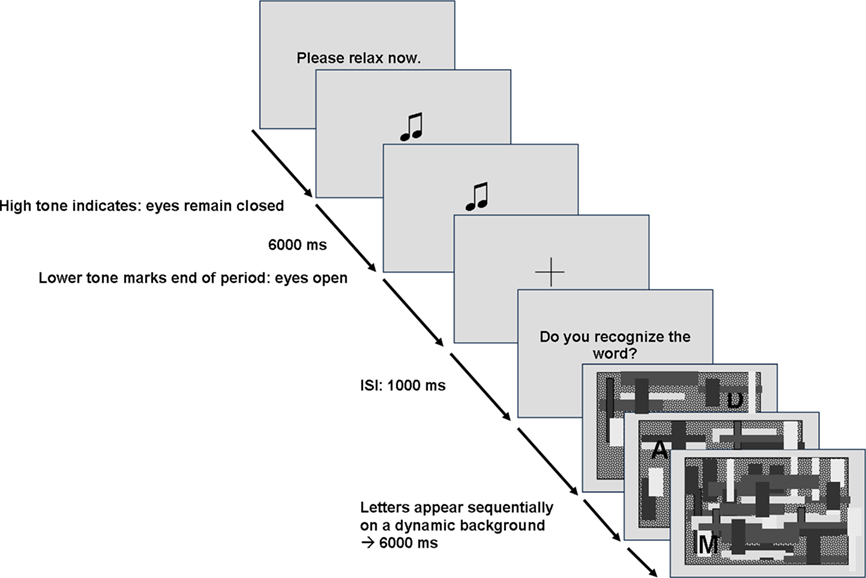
Figure 4. Example trials for the experimental task of the paradigm to induce alpha activity. In the “engaged” condition, participants had to recognize a word in a sequence of letters presented on noisy background. In the “relaxed” condition, participants had to close their eyes and relax indicated by a high tone.
EEG recording
Electroencephalography recording was conducted in the same way as in Experiment 1.
Pre-processing
Artifact removal was identical to Experiment 1. Epochs extracted lasted 6 s from beginning of the task to the end of this period.
EEG frequency analysis
In order to determine whether the frequency characteristics of the dry electrode data are comparable to the wet electrode data, three different measures were used. First power spectral densities of dry and wet electrode data were compared. For this, spectral densities in the range of 0.1–40 Hz were computed using the EEGLAB function “spectopo.” This was done separately for each electrode and experimental condition (“relaxed,” “engaged”), both for the spectral densities of individual subjects as well as for the densities averaged across all participants (grand average).
Second, the mean band power in the alpha band (7–13 Hz), which was expected to be pronounced in the “relaxed” condition, was investigated more closely. Mean band power for each single trial of the “relaxed” and “engaged” condition was determined for each channel and participant. The resulting single-trial band power values were normalized for each condition and participant by the band power mean of the corresponding data set. Normalized single-trial band power values were averaged across trials and channels for each condition. Mean difference values between conditions were calculated (“relaxed” minus “engaged”) resulting in one normalized mean difference band power value per participant.
As a third dependent measure, the time course of the band power in the alpha band range was examined. For each trial, a sliding window of 100 data points length was shifted over the signal with a window overlap of 99 data points for two successive shifts. The signal in each window was transformed by multiplying it with a Gaussian window of the same length to avoid leakage effects. Then, the normalized band power of the transformed signal was calculated. The resulting band power time courses were averaged across trials, yielding 12 vectors (3 channels × 2 conditions × 2 electrode sets) for each participant containing the time course information of the band power in the alpha band.
BCI analysis
To assess the potential of the dry electrodes for BCI applications, classification accuracies between dry and wet electrode data were compared. For this, logarithmic band power values (Solis-Escalante et al., 2010; Zander et al., 2011) were used for feature extraction. Based on these features, classification was performed using a rLDA. Classification accuracies were estimated using 10 × 10-fold cross-validation (Bishop, 2006; Blankertz et al., 2007; Zander et al., 2011).
Statistical Analysis
For power spectral densities in the range of 0.1–40 Hz, bivariate correlations (Pearson Correlation) between dry and wet electrode data were calculated for each participant and electrode as well as for the grand average mean. For these individual and grand average correlations, one sample t-tests against zero were performed for each electrode, again at a significance level of p < 0.05.
Mean difference band power values were tested for significant differences across participants between wet and dry electrode data using paired sample t-tests. The alpha significance level was again set to p > 0.1 to control for the beta error. For band power time courses in the alpha band, bivariate correlations between wet and dry electrode data were calculated for each condition, channel, and participant. Classification accuracies of dry and wet electrode data were compared with a paired sample t-test. The significance level was raised again to p = 0.1 to control for the beta error.
Results
Frequency spectra
The mean of individual correlations (subjects × channels) between dry and wet electrode power spectral densities was r = 0.97 (SD = 0.03) with all individual correlation values above r > 0.86. For the grand average power spectral densities, the respective correlation coefficients were above r > 0.98 (mean: 0.99, SD: 0.01) for all three channels. All correlations reached a significant value of p < 0.05. Figure 5 shows two examples for grand average power spectral density plots for both conditions.
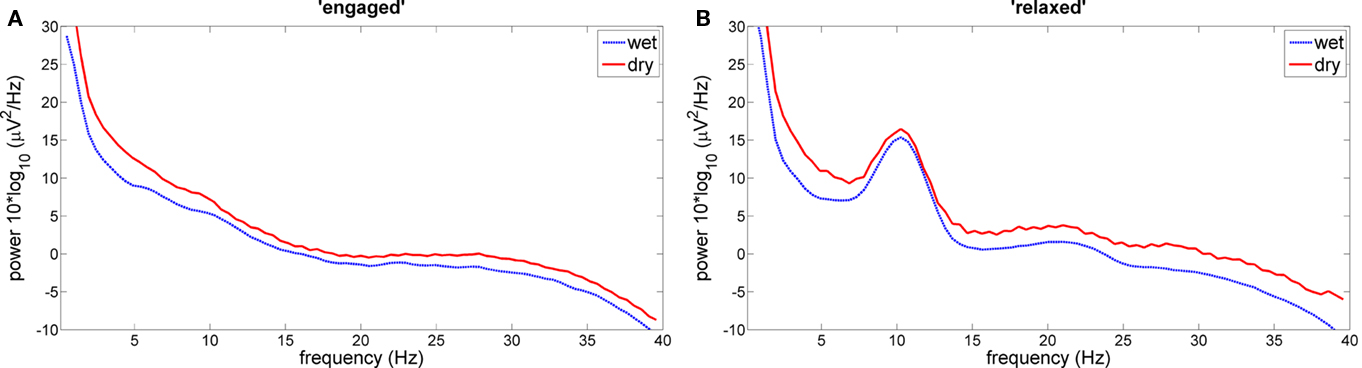
Figure 5. Grand average power spectral densities at PO7. Data are averaged across subjects during relaxation (A) and during mental engagement (B).
The grand average band power difference between conditions “relaxed” and “engaged” was bprel-eng = 2.68 (SD = 1.20) for dry electrode data and bprel-eng = 2.54 (SD: 0.82) for wet electrode data. The t-test for individual mean bp differences between conditions yielded no significant differences between wet and dry electrode data [t(11) = 0.34, p > 0.1].
For alpha band power time courses, mean correlation across participants and channels for the relaxed condition was r = 0.74 (PO7: r = 0.72, Oz: r = 0.73, PO8: r = 0.76). These correlations were significant for all participants (all p values < 0.05). For the “engaged” condition the mean band power correlation was r = 0.63 (PO7: r = 0.63, Oz: r = 0.66, PO8: r = 0.61). For two subjects, the correlation was not significant at PO7 and for one of these not significant at Oz. For all other participants and channels, correlations were significant. Figure 6 shows the time course of the alpha band power of a participant with high correlations between the two EEG systems (participant 8).
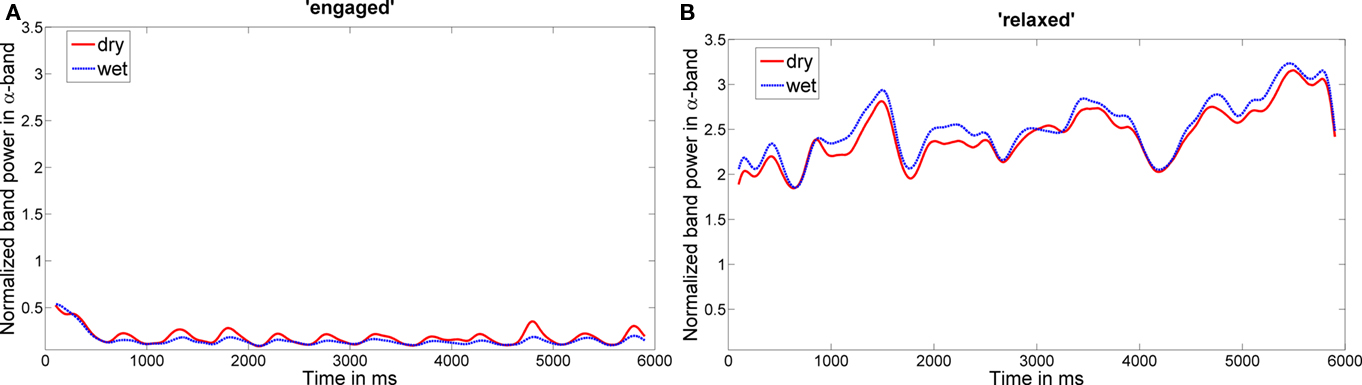
Figure 6. Time course of alpha band power. Examples (participant 8) for the time course of the alpha band power for the experimental conditions “engaged” (A) and “relaxed” (B).
BCI analysis
Table 3 lists the classification accuracies of individual participants for the different electrode sets. Mean classification accuracy for dry electrode data was 90.7 and 94.0% for wet electrode data. Comparing classification accuracies between wet and dry electrode condition no significant differences were found between the two electrode systems [t(11) = 0.67, p > 0.1].
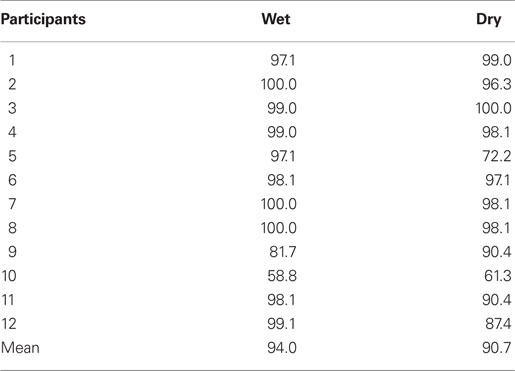
Table 3. Brain–computer interface results of the alpha paradigm. Classification results for all participants.
Discussion
The consistently high correlations between the frequency spectra of the dry and wet electrode data suggest that both electrode sets are equally capable of measuring spontaneous EEG. This is also supported by the band power analysis of the alpha band. Band power differences between “relaxed” and “engaged” condition do not differ significantly from each other. With respect to the time course of the alpha band power, it was evident that there were significant correlations across all participants for the “relaxed” condition and predominantly significant correlations in the “engaged” condition. One possible explanation for the sporadic not significant correlations in the “engaged” condition, respecting two participants, could be the fact that the dry data was not preprocessed individually for artifacts. Artifact removal was performed by visual inspection on the wet electrode data. These rejection time windows were automatically transferred to the dry electrode data to make a comparison of both electrode systems possible and to rule out possible influences of manual artifact removal on the dry electrode data quality. Hence, artifacts occurring only in the dry electrode system, e.g., due to sweat or movement of individual electrodes remained in the data and thereby could have contaminated experimental trials.
Mean classification accuracies were above 90% for the data of both electrode sets and the distributions did not differ significantly from each other. However, for some participants, there were larger differences varying in both directions.
General Discussion
In this paper we aimed to investigate the data quality of dry electrodes for recording EEG patterns which are commonly used in EEG analyses and which are similar to those patterns used in common BCI paradigms. In contrast to other studies on dry electrode systems, which focus on BCIs transfer rates (Popescu et al., 2007; Oehler et al., 2008), we report here results on common EEG analyzes and the abstract measure of classification accuracy. To summarize, we found that the presented system indeed is capable of recording reliable EEG signals. The recorded signals show only slight differences to the data recorded with standard wet EEG electrodes.
In two experiments considering ERPs as well as spontaneous EEG, we were able to show that the Brain Products dry electrode set is capable of recording EEG signals for psychophysiological research and signals used in BCIs. Compared to a set of standard wet electrodes consisting of the same number of channels, there was almost no difference in standard ERP and frequency measures. ERP peak latency measures revealed that there is no time lag induced by the dry electrode system. In addition, there was no significant performance degradation for the BCI classification with respect to the frequency domain. The slightly degraded classification results of the oddball paradigm for the dry electrode set remain to be investigated. It might be possible that the paradigm is not suitable for single-trial analysis at occipital sites. Moreover, it could be due to the small number of trials. The chosen approach of conservative artifact removal only based on time periods taken from the baseline data of wet electrodes ensures the validity of our results.
In sum, the dry electrode system investigated here showed in most cases no performance degradation in EEG and BCI measures. But still, as we were only able to record data from parietal areas due to the setup of the used prototype of the headset, we could only investigate patterns similar to those commonly used in BCI applications. Hence, the presented results are only the first step toward the usage of dry electrodes for BCI. Next steps should be the development of systems with more electrodes which possibly could be placed at any site of the international 10/20-system. With that, these new electrodes could open new opportunities to apply BCI in the context of HMI, e.g., as gadget in the gaming context, thus moving it out of the clinical context. Moreover, patients with severe motor impairment could benefit from this new technology because handling of the dry electrode system overcomes many of the restrictions of conventional EEG systems. Dry electrodes may also reduce the time needed for a neurological exam, thereby reducing costs for the health care system.
Conclusion and Outlook
This study presented a prototype of a dry electrode cap which shows that the investigated electrodes provide a high potential to be applicable in psychophysiological and BCI research. This system represents a crucial step to move BCIs from laboratories to people’s homes. The next step would be using these electrodes in conventional BCI applications – including online feedback. Also interesting would be a detailed comparison of differences between the developments of artifacts in dry and wet electrodes. Here, a shift to a new baseline consisting of the intersection of artifact periods from both data streams would be useful. Therefore, the mainly mechanical problem of placing dry electrodes on the top of the head still needs to be solved. A newer version of this prototype, which currently is under development, will provide electrodes over motor cortex and frontal midline areas – to enable, for example, control by motor imagery. Final goal could be a high-density electrode array which would be capable of rendering a much broader range of EEG studies and BCI applications. We will continue on tracking efforts in this direction and hopefully will be able to test diverse prototypes in our laboratory in the near future.
Conflict of Interest Statement
The authors declare that the research was conducted in the absence of any commercial or financial relationships that could be construed as a potential conflict of interest.
Acknowledgments
The authors gratefully acknowledge the support by the company Brain Products with providing us the first prototype of the actiCap Dry System. Also, we thank Mandy Dotzauer for advice on earlier versions of the manuscript.
References
Berger, H. (1929). über das Elektroenkephalogramm des Menschen. Eur. Arch. Psychiatry Clin. Neurosci. 87, 527–570.
Birbaumer, N., Ghanayim, N., Hinterberger, T., Iversen, I., Kotchoubey, B., Kübler, A., Perelmouter, J., Taub, E., and Flor, H. (1999). A spelling device for the paralysed. Nature 398, 297–298.
Blankertz, B., Dornhege, G., Krauledat, M., Müller, K.-R., and Curio, G. (2007). The non-invasive Berlin brain-computer interface: fast acquisition of effective performance in untrained subjects. Neuroimage 37, 539–550.
Blankertz, B., Schäfer, C., Dornhege, G., and Curio, G. (2002). Single trial detection of EEG error potentials: a tool for increasing BCI transmission rates. Artif. Neural Netw. ICANN 2002, 1137–1143.
Blankertz, B., Tangermann, M., Vidaurre, C., Fazli, S., Sannelli, C., Haufe, S., Maeder, C., Ramsey, L., Curio, G., and Müller, K.-R. (2010a). Non-medical uses of BCI technology. Front. Neuropro. 4:198. doi: 10.3389/fnins.2010.00198
Blankertz, B., Lemm, S., Treder, M., Haufe, S., and Müller, K.-R. (2010b). Single-trial analysis and classification of ERP components – a tutorial. Neuroimage. 56, 814–825.
Broermann, U., and Nijboer, F. (2010). “Brain–computer interfaces for communication and control in locked-in patients,” in Brain–Computer Interfaces, eds B. Graimann, G. Pfurtscheller, and B. Z. Allison (Berlin: Springer), 185–201.
Casson, A. J., Yates, D. C., Smith, S. J. M., Duncan, J. S., and Rodriguez-Villegas, E. (2010). Wearable electroencephalography – what is it, why is it needed and what does it entail? IEEE Eng. Med. Biol. 44–56.
Chatterjee, S., and Price, A. (2009). Healthy living with persuasive technologies: framework, issues, and challenges. J. Am. Med. Inform. Assoc. 16, 171–178. doi: 10.1197/jamia.M2859
Cutrell, E., and Tan, D. (2008). “BCI for Passive Input in HCI,” in Proceedings of ACM CHI 2008 (New York: ACM Press).
Delorme, A., and Makeig, S. (2004). EEGLAB: an open source toolbox for analysis of single trial EEG dynamics including independent component analysis. J. Neurosci. Methods 134, 9–21.
Dornhege, G., Millan, J., Hinterberger, T., McFarland, D., and Müller, K.-R. (eds). (2007). Toward Brain Computer Interfacing. Cambridge, MA: MIT Press.
Farwell, L. A., and Donchin, E. (1988). Talking off the top of your head: toward a mental prosthesis utilizing event-related brain potentials. Electroencephalogr. Clin. Neurophysiol. 70, 510–523.
Hoffmann, U., Vesin, J. M., Ebrahimi, T., and Diserens, K. (2008). An efficient P300-based brain-computer interface for disabled subjects. J. Neurosci. Methods 167, 115–125.
Holroyd, C. B. (2004). “A note on the oddball N200 and the feedback ERN,” in Errors, Conflicts, and The Brain. Current Opinions on Performance Monitoring, eds M. Ullsperger and M. Falkenstein (Leipzig: Max-Planck Institute for Human Cognitive and Brain Sciences), 211–218.
Krepki, R., Blankertz, B., Curio, G., and Müller, K.-R. (2007). The Berlin brain computer interface – towards a new communication channel for online control in gaming applications. J. Multimed. Tools Appl. 33, 73–90.
Linden, D. E. J. (2005). The P300: where in the brain is it produced and what does it tell us? Neuroscientist 11, 563–576.
Müller, K.-R., Tangermann, M., Dornhege, G., Krauledat, M., Curio, G., and Blankertz, B. (2008). Machine learning for real-time single-trial analysis: from brain-computer interfacing mental state monitoring. J. Neurosci. Methods 167, 82–90.
Nijboer, F., Sellers, E. W., Mellinger, J., Jordan, M. A., Matuz, T., Furdea, A., Halder, S., Mochty, U., Krusienski, D. J., Vaughan, T. M., Wolpaw, J. R., Birbaumer, N., and Kübler, A. (2008). A P300-based brain-computer interface for people with amyotrophic lateral sclerosis. Clin. Neurophysiol. 119, 1909–1916.
Nijholt, A., van Erp, J., and Heylen, D. K. J. (2008). “Brain gain: BCI for HCI and games,” in Proceedings AISB Symposium Brain Computer Interfaces and Human Computer Interaction: A Convergence of Ideas, Aberdeen, 32–35.
Oehler, M., Neumann, P., Becker, M., Curio, G., and Schilling, M. (2008). “Extraction of SSVEP signals of a capacitive EEG helmet for human machine interface,” in Proceedings of the Annual International Conference of the IEEE Engineering in Medicine and Biology Society. IEEE Engineering in Medicine and Biology Society (Vancouver: IEEE Service Center), 4495–4498.
Pfurtscheller, G., Neuper, C., Flotzinger, D., and Pregenzer, M. (1997). EEG-based discrimination between imagination of right and left hand movement. Electroencephalogr. Clin. Neurophysiol. 103, 642–651.
Piccione, F., Giorgi, F., Tonin, P., Priftis, K., Giove, S., Silvoni, S., Palmas, G., and Beverina, F. (2006). P300-based brain computer interface: reliability and performance in healthy and paralysed participants. Clin. Neurophysiol. 117, 531–537.
Polich, J., and Kok, A. (1995). Cognitive and biological determinants of P300: an integrative review. Biol. Psychol. 41, 103–146.
Popescu, F., Fazli, S., Badower, J., Blankertz, B., and Müller, K.-R. (2007). Single trial classification of motor imagination using 6 dry EEG electrodes. PloS ONE 2, e637. doi: 10.1371/journal.pone.0000637
Ruffini, G., Dunne, S., Esteve, F., Cester, I., Watts, P. C. P., Silva, S. R. P., Grau, C., Fuentemilla, L., Marco-Pallarés, J., and Vandecasteele, B. (2007). “ENOBIO dry electrophysiology electrode; first human trial plus wireless electrode system,” in Proceedings of the Annual International Conference of the IEEE Engineering in Medicine and Biology Society. IEEE Engineering in Medicine and Biology Society. Conference (Lyon: IEEE Service Center), 6690–6694.
Sellers, E., Schalk, G., and Donchin, E. (2003). The P300 as a typing tool: tests of brain computer interface with an ALS patient. Psychophysiology 40(Suppl. 1), :77.
Sellers, E. W., and Donchin, E. (2006). A P300-based brain-computer interface: initial tests by ALS patients. Clin. Neurophysiol. 117, 538–548.
Solis-Escalante, T., Müller-Putz, G., Brunner, C., Kaiser, V., and Pfurtscheller, G. (2010). Analysis of sensorimotor rhythms for the implementation of a brain switch for healthy subjects. Biomed. Signal Process. Control 5, 15–20.
Vaughan, T. M., McFarland, D. J., Schalk, G., Sarnacki, W. A., Krusienski, D. J., Sellers, E. W., and Wolpaw, J. R. (2006). The Wadsworth BCI Research and Development Program: at home with BCI. IEEE Trans. Neural Syst. Rehabil. Eng. 14, 229–233.
Williamson, J., Murray-Smith, R., Blankertz, B., Krauledat, M., and Müller, K.-R. (2009). Designing for uncertain, asymmetric control: interaction design for brain-comp uter interfaces. Int. J. Hum. Comput. Stud. 67, 827–841.
Wolpaw, J. R., Birbaumer, N., McFarland, D. J., Pfurtscheller, G., and Vaughan, T. M. (2002). Brain-computer Interfaces for communication and control. Clin. Neurophysiol. 113, 767–791.
Zander, T. O., Ihme, K., Gaertner, M., and Roetting, M. (2011). A public data hub for benchmarking common brain–computer interface algorithms. J. Neural Eng. 8, 025081.
Zander, T. O., and Kothe, C. (2011). Towards passive brain–computer interfaces: applying brain-computer interface technology to human–machine systems in general. J. Neural Eng. 8, 025005.
Zander, T. O., Kothe, C., Jatzev, S., and Gaertner, M. (2010). “Enhancing human-computer interaction with input from active and passive brain-computer interfaces,” in Brain-Computer Interfaces: Applying our Minds to Human-Computer Interaction, eds D. S. Tan and A. Nijholt (London: Springer), 181–199.
Keywords: EEG, dry electrodes, brain–computer interfaces, human–machine interaction, event-related potentials
Citation: Zander TO, Lehne M, Ihme K, Jatzev S, Correia J, Kothe C, Picht B and Nijboer F (2011) A dry EEG-system for scientific research and brain–computer interfaces. Front. Neurosci. 5:53. doi: 10.3389/fnins.2011.00053
Received: 08 October 2010;
Paper pending published: 26 November 2010;
Accepted: 30 March 2011;
Published online: 26 May 2011.
Edited by:
Niels Birbaumer, Istituto di Ricovero e Cura a Carattere Scientifi co Ospedale San Camillo, ItalyReviewed by:
Niels Birbaumer, Istituto di Ricovero e Cura a Carattere Scientifi co Ospedale San Camillo, ItalyKlaus R. Mueller, Technical University, Germany
Copyright: © 2011 Zander, Lehne, Ihme, Jatzev, Correia, Kothe, Picht and Nijboer. This is an open-access article subject to a non-exclusive license between the authors and Frontiers Media SA, which permits use, distribution and reproduction in other forums, provided the original authors and source are credited and other Frontiers conditions are complied with.
*Correspondence: Thorsten Oliver Zander, Team PhyPA, Department of Human-Machine Systems, Berlin Institute of Technology, Franklinstraße 28/29, 10587 Berlin, Germany. e-mail: tzander@gmail.com