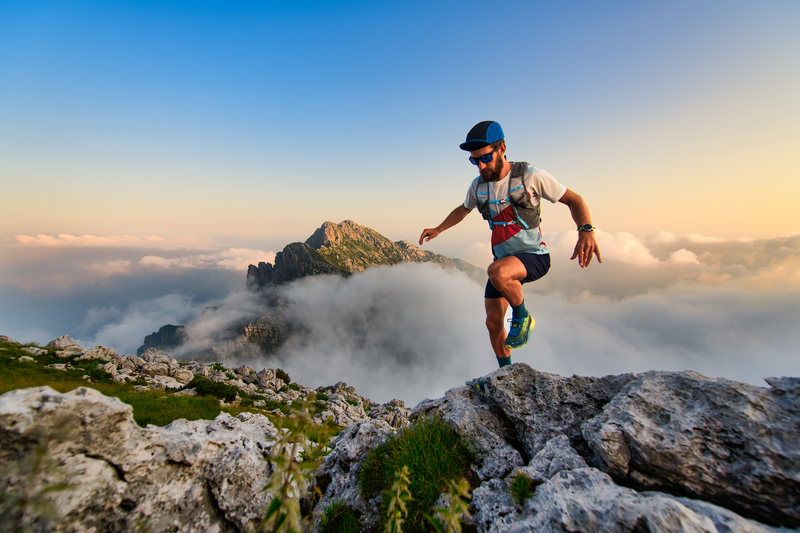
94% of researchers rate our articles as excellent or good
Learn more about the work of our research integrity team to safeguard the quality of each article we publish.
Find out more
PERSPECTIVE article
Front. Neurosci. , 02 June 2010
Sec. Neuroprosthetics
Volume 4 - 2010 | https://doi.org/10.3389/fnins.2010.00021
This article is part of the Research Topic Neuroprosthetic devices View all 5 articles
A multi-electrode array (MEA) was implanted in the dorsolateral funiculus of the cervical spinal cord to record descending information during behavior in freely moving rats. Neural signals were characterized in terms of frequency and information content. Frequency analysis revealed components both at the range of local field potentials and multi-unit activity. Coherence between channels decreased steadily with inter-contact distance and frequency suggesting greater spatial selectivity for multi-unit activity compared to local field potentials. Principal component analysis (PCA) extracted multiple channels of neural activity with patterns that correlated to the behavior, indicating multiple dimensionality of the signals. Two different behaviors involving the forelimbs, face cleaning and food reaching, generated neural signals through distinctly different combination of neural channels, which suggested that these two behaviors could readily be differentiated from recordings. This preliminary data demonstrated that descending spinal cord signals recorded with MEAs can be used to extract multiple channels of command control information and potentially be utilized as a means of communication in high level spinal cord injury subjects.
Spinal cord injury (SCI) at the cervical level results in quadriplegia with little or no function remaining in the upper limbs. Voluntary command signal generation can greatly improve such an individual’s life by utilizing those signals for the control of a neuroprosthetic device. Spinal cord lateral descending pathways above the point of injury can be a potential source for these command signals due to their involvement in producing skilled forelimb movements (Brown, 1971 , 1974 ; Armand, 1982 ; Houk et al., 1988 ; Ten Donkelaar, 1988 ; Canedo, 1997 ; Gibson et al., 1998 ; Whishaw et al., 1998 ; Iwaniuk and Whishaw, 2000 ; Van Kan and McCurdy, 2001 , 2002 ; Hermer-Vazquez et al., 2007 ). It has been shown that the cervical rubrospinal tract (RST) present in the dorsolateral column contains information required specifically for controlling forelimb movements in rats and other species (Gibson et al., 1985a ,b ; Daniel et al., 1987 ; Robinson et al., 1987 ; Houk, 1991 ; Jarratt and Hyland, 1999 ; Jiang et al., 2002 ; Craven, 2008 ). In this project, we are recording motor signals from the RST, located in the dorsolateral column, at the cervical C5/C6 level of the rat spinal cord to test if these signals can be used as a means of generating volitional command signals (Prasad and Sahin, 2006a ,b , 2009 ).
In this study, spinal cord recordings were separated into lower and higher frequency components. Local field potentials (LFPs) result from simultaneously occurring activities of a large number of cellular components and they usually occupy the lower end of the frequency spectrum (Mitzdorf, 1985 ; Sanes and Donoghue, 1993 ; Heldman et al., 2006 ; Kreiman et al., 2006 ; Rasch et al., 2008 ). The higher frequency components are estimated to be the superposition of multiple action potentials generated by the axonal multi-unit activity around the microelectrode tip. The multi-unit activities and the LFPs were recorded during skilled forelimb movements and analyzed to characterize their information content as well as their capacity to differentiate between different forelimb movements.
All procedures were approved by the Institution of Animal Care and Use Committee, Rutgers University, NJ, USA. Four adult Long Evans male rats (300–350 g) were used in this study. The rats were trained for food reaching-and-grasping task through an aperture for an hour each day for 2 weeks prior to the electrode implant surgery. The animals were kept on a feed restriction diet for up to 2 weeks and their weight was maintained at approximately 85% of their initial weight thereafter. The rats were considered fully trained when they were able to grab food pellets approximately 90% of the times.
For surgery, the animals were anesthetized with sodium pentobarbital (30 mg/kg, IP) and deep anesthesia was maintained by administering additional doses of 0.2 mg/kg (IP) as needed. Dexamethasone (2 mg/kg, IM) was administered at the beginning of the surgical procedure to prevent edema in the central nervous system. Bupivacaine (0.2 ml, SC) was injected at the incision site for local anesthesia. Dorsal laminectomy was performed at the cervical C5/C6 levels to expose the spinal cord. The dura was punctured using a 27-gauge needle and then cut using microscissors. A 15-channel microelectrode array (5 × 3 matrix placed longitudinally on the spinal cord, Blackrock Microsystems, Inc, UT, USA) of dimensions 2 × 1.2 mm was implanted free-handed into the right dorsolateral funiculus through the opening in the vertebra using the dorsal entry zone as a marker to position the electrode. Figure 1 shows the implant location of the MEA in the RST at the C5 level. The electrode shanks were 1-mm long with an inter-electrode separation of 400 μm. Each shank was 80-μm thick at the base and tapered to a fine tip that had a platinum coating. A thin layer of cyanoacrylate tissue adhesive was applied around the edges of the electrode to attach it to the pia on the spinal cord. Muscles were sutured in layers using size 5.0 absorbable sutures. The wire interconnects were not sutured to the muscles in order not to create points of high stress along the wires. Six holes were drilled into the skull using a fine drill bit and metal screws were fixed to the skull. The headstage microconnector (Omnetics Connector, MN, USA) was then fixed to the skull and the metal screws using dental acrylic. Buprenorphine (0.05 mg/kg, SC) was administered twice daily for 3-days post-operatively as an analgesic. A 32-channel, 100-gain headstage amplifier (Triangle Biosystems, Inc) was used to interface the electrode array with the data acquisition card (National Instruments PCI 6259) and the computer.
Figure 1. Implant location of the micro electrode array shown on the rubrospinal tract (RST) at the C5 level of the rat spinal cord.
Recording sessions began about a week after the animals completely recovered from surgery. Each animal was recorded from 2–3 times a week for a period of 3–5 months and each recording session usually consisted of 80–90 trials. We have not been able to record for longer periods owing to connector failure, wire breakage, and electrode shanks showing high impedance. Five seconds of neural signals at a sampling rate of 30 kHz and video images at 60 fps were acquired in each trial as the rat performed the reaching or face cleaning behavior. Reaching behavior in rats took approximately 300–400 ms. A regular reaching trial was started by running the acquisition program first and then dropping the food pellet in the tray. Five second long recording segments were made so as not to lose any part of the behavior.
Data analysis was performed using MATLAB. A large reference electrode placed on the spinal cord would possibly record from the sensory pathways near the surface (spinocerebellar) and contaminate all the recording channels. Therefore, one of the electrodes (15th shank) from the array was chosen as a reference and the neural signals were recorded in a single-ended configuration with respect to that electrode. Signals from each contact were subtracted from its neighboring contact along the longitudinal direction to obtain differential recordings. Differential pairs were chosen in the longitudinal direction since the descending fibers were assumed to run along the spinal cord. Thus, seven channels of neural signals were constructed on the computer from 15 electrodes of the array. Welch’s averaged modified periodogram method for spectral estimation was used to calculate the power spectral density for the raw signals.
The coherence spectrum was computed between the single-ended recordings using Matlab’s mscohere function with a window length of 34.13 ms (1024samples) and 50% overlap. The average of the coherence spectra was taken from multiple recording sessions and then from all animals to quantify the average coherence.
Independent component analysis (ICA), which solves the blind source separation problem (Haykin, 2000 ) in signal theory, was used to extract the source signals from the spinal cord recordings. Principal component analysis (PCA) was employed as a preprocessing step before applying ICA (Brunner et al., 2009 ). PCA was applied on seven differential channels and the principal components (PCs) were ordered in a sequence of decreasing eigen values. Four PCs which contributed approximately 90% of the variance in the original signal were entered into the FastICA algorithm (Hyvarinen and Oja, 2000 ) to extract the independent sources. The contributions of each recording channel to the independent components (ICs) were determined by correlation measure. Each of the ICs was correlated, for the duration of the behavior, to each of the seven differentially obtained channels of neural signals. The recording channel was considered to be contributing to the IC if the correlation was greater than 0.7.
Power spectral density plot of a typical recording is shown in Figure 2 . The frequency range below 200 Hz, which is generally classified as LFPs, demonstrates significant power. The power density decreased steadily by frequency up to 5 kHz. The power level in the multi-unit activity range (>1 kHz) remained higher than those in a resting animal. The time-frequency spectrogram plotted in Figure 3 showed a clear shift in frequency when the animal was performing the reaching task (indicated by arrow). After the animal retrieved the food, the components at high frequencies decreased but they are still observable while the forelimb was used for holding the food pellet.
Figure 2. Power spectral density (PSD) estimate of a typical neural channel. Red trace shows the PSD when the animal was resting and the blue trace shows the PSD when the animal was reaching for food.
Figure 3. Spectrogram estimated using Welch technique while the animal was performing the behavioral task. The arrow shows the duration of the behavior.
Figure 4 shows four channels of neural signals from a trial when the rat was performing the reaching task. The neural signals generally had peak-to-peak amplitudes of about 100–200 μV. The arrow in the Figure indicates the time of occurrence for the behavior as determined from the video recordings.
Figure 4. Four selected channels of neural signals, filtered between 300–5000 Hz show the axonal population activity during the reaching behavior. The arrow marks the time of occurrence for the reach-to-grasp behavior.
Figure 5 shows the first four principal components (PCs) calculated from an episode of neural signals, four channels of which were plotted in Figure 4 . The principal components are displayed in the order of significance that they make a contribution to the neural channels. PC1 was the best correlated component to the behavior onset in this trial and others. Three or four channels accounted for 90% of the variance in the signals on average. Figure 6 shows the rectified-averaged version of the signals in Figure 5 . It can be observed from both figures that each channel records a unique pattern of activity during the behavior with some similarity. This suggests that electrode contacts record different neural components related to the behavior that is encoded by spatially distinct multi-unit of axons.
Figure 5. First four principal components (PCs) of the population activity shown in Figure 4 . The remaining three components, did not exhibit activity coincident with the behavior.
Figure 6. Rectified-averaged version of the PCs shown in Figure 5 . Neural components were observed during behavior in all channels. Although they are similar, each component has a distinct appearance.
The average coherence between single-ended recordings of 14 electrodes of the 5 × 3 array (15th electrode is used as a reference) across multiple trials from an animal were plotted in Figure 7 . Coherence was calculated for electrode separations of 400, 800, 1200, and 1600 μm. The coherence value decreased by distance for all frequencies below 2 kHz approximately, which contains both multi-unit activity as well as the LFPs. Overall, the coherence value also decreased by frequency for all distances considered except the last, the 1600 μm. The difference between the coherence vs. frequency plots for various distances is primarily due to the neural components superimposed on the baseline noise. Thus, it can be inferred from the coherence plots that frequency band for the multiunit activity is extending up to 3.5 kHz, similar to what was predicted by power spectra in Figure 2 .
Figure 7. Average coherence for all possible contact combinations in the electrode array with specific electrode separations of 400 through 1600 μm. Only longitudinal arrangements are considered. Coherence values are averaged across 20 trials from an animal. The average coherence decreases by distance and by frequency in general.
Coherence values at various frequencies were extracted from Figure 7 and plotted again in Figure 8 to demonstrate the rate of decline by distance for the multi-unit activity, which would typically peak around 1 kHz, and for LFP that is usually considered below 200 Hz. The coherence monotonously decreases with increasing electrode separation at all frequencies. The low value of coherence for 400 μm at f > 1kHz indicates that the channel crosstalk is not very strong in the multi-unit activity range even for the shortest contact separation. That is, the overlap between the axonal populations that the electrode contacts record from is not extensive.
Figure 8. Coherence at different frequencies as a function of electrode separation extracted from Figure 7 . Average coherence steadily decreases by distance for the range (400–1600 μm) and frequencies considered.
The mean percent contributions of each differential neural channel to the independent sources (computed from the same neural signals) are plotted in Figure 9 across multiple trials of face cleaning and food reaching behaviors. The bar plots show the electrode contributions (i.e. number of hits) in mean percentages after being normalized by the total number of trials for each behavior. Four recording sessions (200 reaching trials and 70 cleaning trials) were used. The distribution of activity across the neural channels seems to be very different for each behavior. Neural channels 4 and 5 alone may be sufficient to differentiate between the two behavior types since they rarely occur together.
Figure 9. Relative contributions of neural channels to the independent components during two different behaviors. reaching for food and face cleaning. See text for the analysis details. The distribution of percentages across the neural channels presents distinctly different patterns for each behavior.
Brain-computer interfaces (BCI) based on implantable multi-electrode recordings were first investigated in rodents (O’Keefe and Dostrovsky, 1971 ; Nicolelis et al., 1993 , 1995 , 1998 ; Wilson and McNaughton, 1993 ; Chapin et al., 1999 ) and later in non-human primates (Baker et al., 1999 ; Decharms et al., 1999 ; Wessberg et al., 2000 ; Serruya et al., 2002 ; Taylor et al., 2002 ; Carmena et al., 2003 ; Nicolelis et al., 2003 ; Hatsopoulos et al., 2004 ; Musallam et al., 2004 ; Lebedev et al., 2005 ; Santhanam et al., 2006 ; Jackson and Fetz, 2007 ). Recent studies have shown monkeys with implanted electrodes to be able to feed themselves by controlling a robotic arm and gripper (Velliste et al., 2008 ). Recently, these multi-electrodes were tested in tetraplegic human trials, where recorded signals controlled a computer cursor (Hochberg et al., 2006 ; Truccolo et al., 2008 ). However, the technology has not yet been transferred to clinics owing to several issues (see Nicolelis and Lebedev, 2009 for discussion). A spinal cord computer interface based on signals recorded from the descending dorsolateral pathways presented in this study provides an alternative approach to BCI.
The corticospinal tract (CST) contained in the dorsolateral funiculus is the primary descending motor pathway and the rubrospinal tract (RST, site for electrode implantation in this study) is vestigial in humans. The dorsolateral funiculus in rats consists mainly of the RST fibers (Raineteau et al., 2002 ), which is involved in producing skilled movements (Gibson et al., 1985a ,b ; Daniel et al., 1987 ; Robinson et al., 1987 ; Houk, 1991 ; Whishaw et al., 1998 ; Jarratt and Hyland, 1999 ; Jiang et al., 2002 ; Craven, 2008 ). The same implant site in humans would record signals from the CST only, which is the primary motor tract in humans. The rat serves as an excellent animal model to study the descending signals controlling the forelimbs because of several similarities in forelimb movements and their velocity profiles compared to humans (Whishaw et al., 1992 ; Sacrey et al., 2009 ) and because of the distinct anatomical locations of the CST and RST (Raineteau et al., 2002 ). Therefore, these motor tracts can be studied individually in rats to ascertain their role in producing voluntary forelimb movements.
Kuchler et. al. (2002) showed that the motor neuron pools for the control of the forelimbs reside in the C4-T1 spinal segments in the rat. They also reported that bouton-like endings of the RST axons and axon collaterals could be observed at all levels of the spinal cord segments they examined (C5-T1) in rats, terminating mostly in laminae V, VI, and dorsal VII. In this study, C5–C6 segments were chosen for recordings of the RST activity. Electrodes implanted at a higher level, such as C2–C3, could have compromised the diaphragm function.
The spinal cord recordings from the rubrospinal tract showed activity patterns that were strongly correlated with the timing of the behavior involving the forelimbs in all behavioral trials. This suggests that neural signals controlling the forelimb movements can be recorded from the descending tracts of the cervical spinal cord. Signal amplitudes are well above the noise level reaching 100 μV and higher, although individual action potentials are not discernible because of the large number of axons in the vicinity of electrode tips. Frequency plots indicated the presence of strong LFPs at the lower end of the spectrum as well as multi-unit activity.
Dimensionality of the signals was investigated through PCA, which indicated that there are at least half as many principal components as the number of electrodes that account for most of the variation in the signals. Each one of these components is anticipated to encode different aspects (e.g. control of different muscle groups) of the behavior that are needed in various phases of the behavior.
Spatial selectivity of the electrode was investigated via calculation of coherence as a function of contact separation. Selectivity varied both as a function of distance and frequency. Signals toward the lower end of the frequency spectrum (1–100 Hz) showed less selectivity (higher coherence) compared to signals at higher frequencies. This agrees with the reports that the spatial spread of LFPs is larger than the multi-spike activity (Henze et al., 2000 ). The coherence plot also suggested that the average coherence at all frequencies was greater for shorter inter-contact differences than larger. However, the coherence values were still small for the shortest distance considered (400 μm). Therefore, it may be concluded that even the electrodes at the shortest distance are recording from axonal population that are not overlapping extensively. LFPs, on the other hand, have higher coherence as they represent the summation of field potentials from a large population of cells that are not very near the electrode tips. Such a spatially distributed, distant field is recorded by all electrodes as a common voltage that increases the correlation between all channels. Since the extracellular potentials around the cell bodies are larger than that of the axons and the LFP signals tend to originate from large and far neural activity, we speculate that the LFPs recorded by these microelectrodes must be due to the activity of the neuronal bodies in the gray matter. The coherence (crosstalk) could be reduced between the neural channels by taking the difference of single-ended recordings with respect to a reference electrode. This method effectively removed any common-mode signal recorded by neighboring electrodes. The largest coherence between any contact pair was reduced below 0.08 by converting the single-ended signals to differential pairs.
Principal component analysis was successful in isolating the behavior into a few components, performed either on the single-ended or differential signals. PC1 usually accounted for approximately 70–80% of the variance in the neural signals recorded in single-ended configuration. In this case, PC1 might be accounting for common-mode signals which result from distant sources away from the microelectrode tip. However, the variance accounted by PC1 after obtaining the differential signals reduced to approximately 50% and 3–4 PCs were generally required to account for 90% of variance in the data. PCA was able to extract multiple uncorrelated components from the differential neural signals. These multiple components can be used as individual channels of control in a spinal cord computer interface.
ICA may be a better method for signal separation as it takes higher order statistics into account whereas PCA is based on the second order covariance matrix (Haykin, 2000 ; Makeig et al., 2004 ). The neural signals recorded from the spinal cord descending tracts can be visualized as being generated from a number of sources distributed near the tips of the microelectrode array (Tie and Sahin, 2005 ). Each source represents a group of fibers carrying a unique component of the control signals in this paradigm. Independent component analysis (ICA) method was used to separate these signal sources in this study. The result of this analysis suggested that the two behaviors can be differentiated based on the location of the independent sources. As a control, the frequency components remained near baseline when the animal was quiet and resting compared to the spectrum reaching up to 3.5 kHz when the animal was performing either of the two tasks. Two different behaviors were compared in order to observe the change in the signal contribution pattern to each electrode (Figure 9 ). Reaching and face cleaning both involve flexion and extension of the forelimbs, however they are very distinct in terms of the complexity of the movement. Reaching is a more complex behavior and can be considered as a skilled task compared to face cleaning.
The authors declare that the research was conducted in the absence of any commercial or financial relationships that could be construed as a potential conflict of interest.
This study was supported by a NIH grant R21 NIH/NICDS HD056963-01 A2 and a graduate fellowship to Abhishek Prasad from New Jersey Commission on Spinal Cord Research. The authors would also like to thank Jonathan Groth and Nicholas Sachs, PhD for their help during experiments.
Armand, J. (1982). The origin, course and terminations of corticospinal fibers in various mammals. Prog. Brain Res. 57, 329–360.
Baker, S. N., Philbin, N., Spinks, R., Pinches, E. M., Wolpert, D. M., MacManus, D. G., Pauluis, Q., and Lemon, R. N. (1999). Multiple single unit recording in the cortex of monkeys using independently moveable microelectrodes. J. Neurosci. Methods 94, 5–17.
Brown, L. T. Jr (1971). Projections and termination of the corticospinal tract in rodents. Exp. Brain Res. 13, 432–450.
Brunner, C., Naeem, M., and Pfurtscheller, G. (2009). Dimensionality reduction and channel selection of motor imagery electroencephalographic data. Comput. Intell Neurosci. [Epub ahead of print]. doi: 10.1155/2009/537504
Canedo, A. (1997). Primary motor cortex influences on the descending and ascending systems. Prog. Neurobiol. 51, 287–335.
Carmena, J. M., Lebedev, M. A., Crist, R. E., O’Doherty, J. E., Santucci, D. M., Dimitrov, D. F., Patil, P. G., Henriquez, C. S., and Nicolelis, M. A. L. (2003). Learning to control a brain-machine interface for reaching and grasping by primates. PLoS Biol. 1, e42. doi:10.1371/journal.pbio.0000042.
Chapin, J. K., Moxon, K. A., Markowitz, R. S., and Nicolelis, M. A. L. (1999). Real-time control of a robot arm using simultaneously recorded neurons in the motor cortex. Nat. Neurosci. 2, 664–670.
Craven, J. (2008). Major ascending and descending tracts in the spinal cord. Anaesth. Intensive Care Med. 9, 1–2.
Daniel, H., Billard, J. M., Angaut, P., and Batini, C. (1987). The interposito-rubrospinal system. Anatomical tracing of a motor control pathway in the rat. Neurosci. Res. 5, 87–112.
Decharms, R. C., Blake, D. T., and Merzenich, M. M. (1999). A multielectrode implant device for the cerebral cortex. J. Neurosci. Methods 93, 27–35.
Gibson, A. R., Horn, K. M., Pong, M., and Van Kan, P. L. E. (1998). “Construction of a reach-to-grasp,” in Novartis Found. Symp. 218, 233–251.
Gibson, A. R., Houk, J. C., and Kohlerman, N. J. (1985a). Magnocellular red nucleus activity during different types of limb movement in the macaque monkey. J. Physiol. 358, 527–549.
Gibson, A. R., Houk, J. C., and Kohlerman, N. J. (1985b). Relation between red nucleus discharge and movement parameters in trained macaque monkeys. J. Physiol. 358, 551–570.
Hatsopoulos, N. G., Joshi, J., and O’Leary, J. G. (2004). Decoding continuous and discrete motor behaviors using motor and premotor cortical ensembles. J. Neurophysiol. 92, 1165–1174.
Haykin, S. (ed) (2000). Unsupervised Adaptive Filtering: Volume 1, Blind Source Separation. New York: Wiley.
Heldman, D. A., Wang, W., Chan, S. S., and Moran, D. W. (2006). Local field potential spectral tuning in motor cortex during reaching. IEEE Trans. Neural. Syst. Rehabil. Eng. 14, 180–183.
Henze, D. A., Borhegyi, Z., Csicsvari, J., Mamiya, A., Harris, K. D., and Buzsáki, G. (2000). Intracellular features predicted by extracellular recordings in the hippocampus in vivo. J. Neurophysiol. 84, 390–400.
Hermer-Vazquez, L., Hermer-Vazquez, R., and Chapin, J. K. (2007). The reach-to-grasp-food task for rats: a rare case of modularity in animal behavior? Behav. Brain Res. 177, 322–328.
Hochberg, L. R., Serruya, M. D., Friehs, G. M., Mukand, J. A., Saleh, M., Caplan, A. H., Branner, A., Chen, D., Penn, R. D., and Donoghue, J. P. (2006). Neuronal ensemble control of prosthetic devices by a human with tetraplegia. Nature 442, 164–171.
Houk, J. C., Gibson, A. R., Harvey, C. F., Kennedy, P. R., and Van Kan, P. L. E. (1988). Activity of primate magnocellular red nucleus related to hand and finger movements. Behav. Brain Res. 28, 201–206.
Hyvarinen, A., and Oja, E. (2000). Independent component analysis: algorithms and applications. Neural Netw. 13, 411–430.
Iwaniuk, A. N., and Whishaw, I. Q. (2000). On the origin of skilled forelimb movements. Trends Neurosci. 23, 372–376.
Jackson, A., and Fetz, E. E. (2007). Compact movable microwire array for long-term chronic unit recording in cerebral cortex of primates. J. Neurophysiol. 98, 3109–3118.
Jarratt, H., and Hyland, B. (1999). Neuronal activity in rat red nucleus during forelimb reach-to-grasp movements. Neuroscience 88, 629–642.
Jiang, M. C., Alheid, G. F., Nunzi, M. G., and Houk, J. C. (2002). Cerebellar input to magnocellular neurons in the red nucleus of the mouse: synaptic analysis in horizontal brain slices incorporating cerebello-rubral pathways. Neuroscience 110, 105–121.
Kreiman, G., Hung, C. P., Kraskov, A., Quiroga, R. Q., Poggio, T., and DiCarlo, J. J. (2006). Object selectivity of local field potentials and spikes in the macaque inferior temporal cortex. Neuron 49, 433–445.
Kuchler, M., Fouad, K., Weinmann, O., Schwab, M. E., and Raineteau, O. (2002). Red nucleus projections to distinct motor neuron pools in the rat spinal cord. J. Comp. Neurol. 448, 349–359.
Lebedev, M. A., Carmena, J. M., O’Doherty, J. E., Zacksenhouse, M., Henriquez, C. S., Principe, J. C., and Nicolelis, M. A. L. (2005). Cortical ensemble adaptation to represent velocity of an artificial actuator controlled by a brain-machine interface. J. Neurosci. 25, 4681–4693.
Makeig, S., Debener, S., Onton, J., and Delorme, A. (2004). Mining event-related brain dynamics. Trends Cogn. Sci. (Regul. Ed.) 8, 204–210.
Mitzdorf, U. (1985). Current source-density method and application in cat cerebral cortex: Investigation of evoked potentials and EEG phenomena. Physiol. Rev. 65, 37–100.
Musallam, S., Corneil, B. D., Greger, B., Scherberger, H., and Andersen, R. A. (2004). Cognitive control signals for neural prosthetics. Science 305, 258–262.
Nicolelis, M. A., Ghazanfar, A. A., Stambaugh, C. R., Oliveira, L. M., Laubach, M., Chapin, J. K., Nelson, R. J., and Kaas, J. H. (1998). Simultaneous encoding of tactile information by three primate cortical areas. Nat. Neurosci. 1, 621–630.
Nicolelis, M. A. L., Baccala, L. A., Lin, R. C. S., and Chapin, J. K. (1995). Sensorimotor encoding by synchronous neural ensemble activity at multiple levels of the somatosensory system. Science 268, 1353–1358.
Nicolelis, M. A. L., Dimitrov, D., Carmena, J. M., Crist, R., Lehew, G., Kralik, J. D., and Wise, S. P. (2003). Chronic, multisite, multielectrode recordings in macaque monkeys. Proc. Natl. Acad. Sci. U.S.A. 100, 11041–11046.
Nicolelis, M. A. L., and Lebedev, M. A. (2009). Principles of neural ensemble physiology underlying the operation of brain-machine interfaces. Nat. Rev. Neurosci. 10, 530–540.
Nicolelis, M. A. L., Lin, R. C. S., Woodward, D. J., and Chapin, J. K. (1993). Induction of immediate spatiotemporal changes in thalamic networks by peripheral block of ascending cutaneous information. Nature 361, 533–536.
O’Keefe, J., and Dostrovsky, J. (1971). The hippocampus as a spatial map. Preliminary evidence from unit activity in the freely-moving rat. Brain Res. 34, 171–175.
Prasad, A., and Sahin, M. (2006a). Extraction of motor activity from the cervical spinal cord of behaving rats. J. Neural Eng. 3, 287–292.
Prasad, A., and Sahin, M. (2006b). Multi-channel recordings of the motor activity from the spinal cord of behaving rats. Conf. Proc. IEEE Eng. Med. Biol. Soc. 1, 2288–2291.
Prasad, A., and Sahin, M. (2009). Spinal cord recordings in rats during skilled reaching task. Conf. Proc. IEEE Eng. Med. Biol. Soc. 1, 582–585.
Raineteau, O., Fouad, K., Bareyre, F. M., and Schwab, M. E. (2002). Reorganization of descending motor tracts in the rat spinal cord. Eur. J. Neurosci. 16, 1761–1771.
Rasch, M. J., Gretton, A., Murayama, Y., Maass, W., and Logothetis, N. K. (2008). Inferring spike trains from local field potentials. J. Neurophysiol. 99, 1461–1476.
Robinson, F. R., Houk, J. C., and Gibson, A. R. (1987). Limb specific connections of the cat magnocellular red nucleus. J. Comp. Neur. 257, 553–577.
Sacrey, L. A. R., Alaverdashvili, M., and Whishaw, I. Q. (2009). Similar hand shaping in reaching-for-food (skilled reaching) in rats and humans provides evidence of homology in release, collection, and manipulation movements. Behav. Brain Res. 204, 153–161.
Sanes, J. N., and Donoghue, J. P. (1993). Oscillations in local field potentials of the primate motor cortex during voluntary movement. Proc. Natl. Acad. Sci. U.S.A. 90, 4470–4474.
Santhanam, G., Ryu, S. I., Yu, B. M., Afshar, A., and Shenoy, K. V. (2006). A high-performance brain-computer interface. Nature 442, 195–198.
Serruya, M. D., Hatsopoulos, N. G., Paninski, L., Fellows, M. R., and Donoghue, J. P. (2002). Instant neural control of a movement signal. Nature 416, 141–142.
Taylor, D. M., Tillery, S. I. H., and Schwartz, A. B. (2002). Direct cortical control of 3D neuroprosthetic devices. Science 296, 1829–1832.
Ten Donkelaar, H. J. (1988). Evolution of the red nucleus and rubrospinal tract. Behav. Brain Res. 28, 9–20.
Tie, Y., and Sahin, M. (2005). Separation of spinal cord motor signals using the FastICA method. J. Neural Eng. 2, 90–96.
Truccolo, W., Friehs, G. M., Donoghue, J. P., and Hochberg, L. R. (2008). Primary motor cortex tuning to intended movement kinematics in humans with tetraplegia. J. Neurosci. 28, 1163–1178.
Van Kan, P. L. E., and McCurdy, M. L. (2001). Role of primate magnocellular red nucleus neurons in controlling hand preshaping during reaching to grasp. J. Neurophysiol. 85, 1461–1478.
Van Kan, P. L. E., and McCurdy, M. L. (2002). Contribution of primate magnocellular red nucleus to timing of hand preshaping during reaching to grasp. J. Neurophysiol. 87, 1473–1487.
Velliste, M., Perel, S., Spalding, M. C., Whitford, A. S., and Schwartz, A. B. (2008). Cortical control of a prosthetic arm for self-feeding. Nature 453, 1098–1101.
Wessberg, J., Stambaugh, C. R., Kralik, J. D., Beck, P. D., Laubach, M., Chapin, J. K., Kim, J., Biggs, S. J., Srinivasan, M. A., and Nicolelis, M. A. L. (2000). Real-time prediction of hand trajectory by ensembles of cortical neurons in primates. Nature 408, 361–365.
Whishaw, I. Q., Gorny, B., and Sarna, J. (1998). Paw and limb use in skilled and spontaneous reaching after pyramidal tract, red nucleus and combined lesions in the rat: Behavioral and anatomical dissociations. Behav. Brain Res. 93, 167–183.
Whishaw, I. Q., Pellis, S. M., and Gorny, B. P. (1992). Skilled reaching in rats and humans: Evidence for parallel development or homology. Behav. Brain Res. 47, 59–70.
Keywords: rubrospinal tract, brain-computer, neural interface
Citation: Prasad A and Sahin M (2010) Characterization of neural activity recorded from the descending tracts of the rat spinal cord. Front. Neurosci. 4:21. doi: 10.3389/fnins.2010.00021
This article was submitted to Frontiers in Neuroprosthetics, a specialty of Frontiers in Neuroscience.
Received: 02 January 2010;
Paper pending published: 14 January 2010;
Accepted: 06 April 2010;
Published online: 02 June 2010
Edited by:
Victor Pikov, Huntington Medical Research Institutes, USAReviewed by:
Robert Gaunt, University of Alberta, CanadaCopyright: © 2010 Prasad and Sahin. This is an open-access article subject to an exclusive license agreement between the authors and the Frontiers Research Foundation, which permits unrestricted use, distribution, and reproduction in any medium, provided the original authors and source are credited.
*Correspondence: Mesut Sahin, Department of Biomedical Engineering, New Jersey Institute of Technology, Fenster Building, (Room 617), 323 Martin Luther King Jr Blvd., University Heights, Newark, NJ 07102, USA. e-mail:c2FoaW5AbmppdC5lZHU=
Disclaimer: All claims expressed in this article are solely those of the authors and do not necessarily represent those of their affiliated organizations, or those of the publisher, the editors and the reviewers. Any product that may be evaluated in this article or claim that may be made by its manufacturer is not guaranteed or endorsed by the publisher.
Research integrity at Frontiers
Learn more about the work of our research integrity team to safeguard the quality of each article we publish.