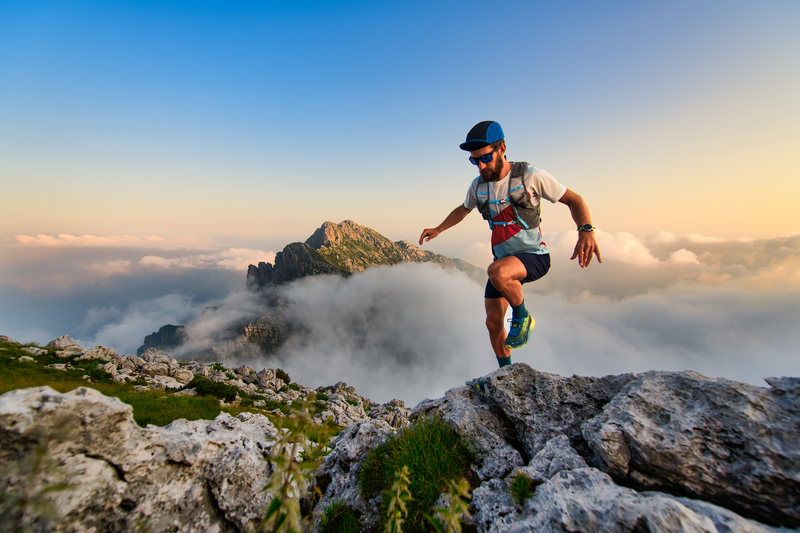
94% of researchers rate our articles as excellent or good
Learn more about the work of our research integrity team to safeguard the quality of each article we publish.
Find out more
MINI REVIEW article
Front. Neuroinform. , 25 March 2024
Volume 18 - 2024 | https://doi.org/10.3389/fninf.2024.1382372
This article is part of the Research Topic Analysis of the Nonlinear Dynamics of Brain Function from Time Series Measurements View all 4 articles
Traumatic Brain Injury (TBI) is a prevalent disorder mostly characterized by persistent impairments in cognitive function that poses a substantial burden on caregivers and the healthcare system worldwide. Crucially, severity classification is primarily based on clinical evaluations, which are non-specific and poorly predictive of long-term disability. In this Mini Review, we first provide a description of our model-free and model-based approaches within the turbulent dynamics framework as well as our vision on how they can potentially contribute to provide new neuroimaging biomarkers for TBI. In addition, we report the main findings of our recent study examining longitudinal changes in moderate-severe TBI (msTBI) patients during a one year spontaneous recovery by applying the turbulent dynamics framework (model-free approach) and the Hopf whole-brain computational model (model-based approach) combined with in silico perturbations. Given the neuroinflammatory response and heightened risk for neurodegeneration after TBI, we also offer future directions to explore the association with genomic information. Moreover, we discuss how whole-brain computational modeling may advance our understanding of the impact of structural disconnection on whole-brain dynamics after msTBI in light of our recent findings. Lastly, we suggest future avenues whereby whole-brain computational modeling may assist the identification of optimal brain targets for deep brain stimulation to promote TBI recovery.
As such, the physical phenomenon of turbulence in fluids has been an object of intense study in the scientific community for more than four centuries, starting from the insightful observations and meticulous drawings by Leonardo Da Vinci (1507) (Deco et al., 2021a) to the mathematical equations developed by Navier (1823) and eventually refined by Stokes (1843) in order to describe the turbulent regime at the microscopic level. However, as already noticed by Da Vinci, the important properties of turbulence are also to be found at the macroscopic level. One of these properties implies the effective and fast transfer of energy across fluids that Andrey Kolmogorov described in his pioneering phenomenological theory of turbulence (Kolmogorov, 1941a,b). In this ground-breaking work, Kolmogorov introduced the concept of structure functions, which allowed him to quantify the energy cascades that balance kinetics and viscous dissipation based on the correlations between two spatial points in a fluid and, as a result, demonstrate a power scaling law. Importantly, these power laws provide a mathematical foundation for Richardson's earlier concept of cascaded eddies (Richardson, 1922), i.e., a cascade of kinetic energy that is transferred from larger to smaller scales without loss in the so-called inertial subrange. At a more abstract level, effective energy transfer can be considered equivalent to efficient information processing, which makes the study of turbulence in the human brain particularly appealing as it requires the rapid integration of information across spatially distant regions.
To study turbulence in a non-hydrodynamic context such as the human brain, one needs to find an appropriate mathematical formalism. In this regard, Yoshiki Kuramoto's theory of coupled oscillators in the 1980s was crucial (Kuramoto, 1984). In fact, using coupled nonlinear oscillators, Kuramoto was able to describe turbulence in many different physical systems. Specifically, in the coupled oscillator framework, the Kuramoto local order parameter represents a spatial average of the complex phase factor of the local oscillators weighted by the coupling. Amplitude turbulence is then defined as the standard deviation of the modulus of the Kuramoto local order parameter and can be applied to the empirical data of any physical system, including the human brain. Interestingly, brain activity can be computationally modeled using Stuart-Landau coupled oscillators with a high level of accuracy, therefore providing some degree of convergence with turbulence as originated by Kuramoto's coupled oscillators. Taken together, this motivated the investigation of turbulence in the human brain by combining Kolmogorov's structure functions with Kuramoto's local order parameter as well as building a Hopf whole-brain model with Stuart-Landau oscillators to understand the causal mechanisms given rise to a turbulent regime.
The discovery that human brain activity is supported by turbulent dynamics was indeed recently made by using a high-quality large-scale resting-state dataset of 1,003 Human Connectome Project's participants (Deco and Kringelbach, 2020). In that study, we found that the whole-brain dynamics operates in a turbulent regime that follows a consistent power law for functional brain correlations in a broad spatial range similar to that shown by Kolmogorov in fluid dynamics and thus indicative of a cascade of information processing. More recently, using the same dataset, these findings have been extended by incorporating higher-order structure functions showing that out-of-equilibrium turbulent dynamics are based on the deviations from scale invariance within the phenomenological Kolmogorov's theory (Perl et al., 2023).
An important additional consideration in the turbulent dynamics framework concerns the concept of the brain vortex space. In fluid dynamics, the vortices are essentially capturing the rotational kinetic energy. In contrast, the brain vortex space refers to the local level of synchronization at a given spatial scale across spacetime thus capturing the level of rotationality. Based on this central concept, we can define three additional measures to study different aspects of information propagation, namely information transfer, information cascade and information cascade flow. The information transfer indicates how the information travels across space at a specific spatial scale. The other two metrics are interrelated. The information cascade flow measures how the information travels from a given spatial scale to a lower scale in consecutive time steps. The information cascade is the average of the information propagation across spatial scales. It then follows that, by calculating these additional measures, we can provide a richer description of turbulent dynamics and information processing. Since this initial implementation, the turbulent dynamics framework (Figure 1A) has also helped to discriminate between different brain states in the healthy population and altered states of consciousness (De Filippi et al., 2021; Cruzat et al., 2022; Escrichs et al., 2022), suggesting that is a valid, sensitive and reliable measure. Noteworthily, this framework has also been adapted to direct measures of fast neural dynamics such as resting-state MEG (Deco et al., 2023).
Figure 1. Whole-brain turbulent dynamics pipeline and its application to a longitudinal dataset of Traumatic Brain Injury (TBI) patients. (A) The implementation of the whole-brain turbulent dynamics framework requires resting-state functional MRI (rs-fMRI), typically using echo-planar images sampling the BOLD time course in each voxel in the brain. This is then combined with a parcellation scheme to recreate the regional time courses for each of the regions in the parcellation, in this case, the fine- grained Schaefer parcellation with 1,000 nodes (Schaefer et al., 2018). This allows for the extraction of regional time courses that are used to compute the analytic signal. The Kuramoto local order parameter can define the turbulent signatures of brain activity at different spatial scales in the vortex space. Four turbulent measures based on the Kuramoto local order parameter are calculated as potential neuroimaging biomarkers: amplitude turbulence, information cascade flow, information cascade and information transfer. (B) Left subpanel: Amplitude turbulence differs between TBI patients and healthy controls (HCs) at long distances in the brain over time, peaking at 6 months post-injury. Middle subpanel: Render brains representing the absolute difference of the node-level metastability between HCs and TBI patients at 6 months post-injury for scale λ= 0.03. Right subpanel: stage-specific resting-state networks “fingerprint” using radar plots for the number of nodes on the top 15% quantile of the absolute difference between HCs and TBI patients at 6-months post-injury comparison for λ= 0.03. Most of the nodes were ascribed to the SM, DAT, and CNT networks. TR: repetition time; LIM, limbic; CNT, control; DMN, default mode; DAT, dorsal-attention; VAT, ventral attention; VIS, visual; SM, somatosensory. Figure adapted with permission from Martínez-Molina et al. (2023).
The Hopf whole-brain modeling approach also allows to calculate other potential neuroimaging biomarkers based on the brain's reactivity to external in silico perturbations. Specifically, one can compute two additional measures: (i) susceptibility, the sensitivity of the whole-brain model to the processing of external stimulations, and (b) information capability, the standard deviation of the difference between the perturbed and unperturbed mean of the modulus of the Kuramoto local order parameter. In the context of turbulence, Deco and Kringelbach (2020) demonstrated that the optimal working point of the Hopf whole-brain model, i.e., showing the best fit to the empirical data, corresponded to a region of maximally developed amplitude turbulence. Remarkably, this was also the point where the information capability reached its maximum, further supporting the notion that amplitude turbulence is key for information processing. It is also important to note that, by incorporating the exponential distance rule (Ercsey-Ravasz et al., 2013) of anatomical connections as a cost-of-wiring principle, the Hopf whole-brain model showed an economy of anatomy that was able to reproduce turbulence. More recent refinements of this model have proved the benefits of adding the rare long-range connections found in the mammalian brain, which improved information processing (Deco et al., 2021b).
In this Mini Review, we first describe the findings from our recent study that point to potential neuroimaging biomarkers for TBI using a model-free and model-based approach within the turbulent dynamics framework. Next, we discuss how the model-based approach combined with a simulated attack in that study helped us to understand the effect of structural disconnection. Finally, we propose that deep brain stimulation treatments for TBI could benefit from presurgical assessment of clinical outcomes using whole-brain computational modeling.
Traumatic Brain Injury (TBI) poses a global burden on death and disability only second to cancer according to a recent estimate (National Academies of Sciences, Engineering, and Medicine, 2022), thus representing a significant public health concern. More specifically, recent reports provide an estimate of 50–60 million people with TBI per year worldwide (Feigin et al., 2013). TBI can result from diverse causes including falls, sports injuries, vehicle collisions, intimate partner violence, and military incidents affecting different age groups, from babies to the elderly (National Academies of Sciences, Engineering, and Medicine, 2022). Patients with TBI show a wide spectrum of symptoms, ranging from physical, behavioral and emotional to cognitive (Hoofien et al., 2001; Dikmen et al., 2009; Cantor et al., 2013; Wilde et al., 2022). This wide variation in the clinical manifestations of TBI is likely due to the complexity of the brain's organization, as well as to the patterns and extent of damage caused by external forces leading to TBI. In addition to focal brain damage, rapid acceleration and deceleration forces at the time of brain injury damage the axonal membrane resulting in the so-called diffuse axonal injury (DAI) (Martínez-Molina et al., 2022) that is thought to underlie alterations in brain network connectivity (Sharp et al., 2014). Moreover, DAI and neuroinflammation after TBI might influence the development of neurodegenerative disorders such as Alzheimer's disease (AD), which is a frequent late complication in these patients (Sharp et al., 2014). Given that impairment in executive functioning is one of the most common symptons after TBI, it is conceivable that DAI affects the long-range connections that shape the brain's information transmission capabilities across time and space (Deco et al., 2021b) and contribute to sustain critical dynamics in the presence of the slow information transfer between neurons (Deco et al., 2023).
Historically, patients with TBI have been classified into mild, moderate, and severe diagnostic categories based exclusively on clinical features such as the level of consciousness as evaluated with the Glasgow Coma Scale (GCS) (Teasdale and Jennett, 1974) or duration of post-traumatic amnesia (PTA) (Marshman et al., 2013). However, both the GCS score and the duration of PTA have been shown to be poorly reflective of pathophysiological mechanisms (King et al., 1997; Zuercher et al., 2009). This makes it clear that patients' stratification should be informed by objective measures of pathophysiology such as neuroimaging, neuroelectrophysiological and fluid biomarkers (Orešič et al., 2016; Majdan et al., 2017; Thelin et al., 2019). The discovery of new biomarkers could also help to better monitor the longitudinal progression of TBI and identify patients at high risk for developing neurodegenerative disease secondary to TBI. Although blood biomarkers are accessible cost-effective promising tools for TBI diagnosis and prognosis in primary care settings, they do not allow to assess the brain's abnormality patterns associated with TBI. A multi-modal approach that integrates neuroimaging biomarkers can circumvent this limitation and contribute to provide a more comprehensive understanding of the disease and its progression. Such an approach including biomarker panels combined with machine learning algorithms may help to identify specific injury profiles (Wilde et al., 2022). In this direction, some studies have started to explore the discriminatory power of a combination of fluid biomarkers in patients with suspected mild TBI with and without neuroimaging findings (Gill et al., 2018; Edwards et al., 2020).
Resting-state fMRI (rs-fMRI) data are increasingly being used in the development of new neuroimaging biomarkers for neurological and neuropsychiatric populations (Deco and Kringelbach, 2014) as these sequences have better signal-to-noise ratio compared to task-based studies, can be acquired in patients who may not be able to perform tasks and can be automatically preprocessed with currently available software tools (Whitfield-Gabrieli and Nieto-Castanon, 2012), which can facilitate their translation from research into clinical practice. According to the Food and Drug Administration (FDA), a biomarker can be defined as “a characteristic that is measured as an indicator of normal biological processes, pathogenic processes, or responses to an exposure or intervention, including therapeutic interventions” with the ideal requirements of a biomarker including being sensitive, specific, reproducible and operational among others (Wilde et al., 2022). In this regard, the turbulent dynamics framework holds great promise to provide new neuroimaging biomarkers as: (i) it can be easily computed from rs-fMRI data, (ii) captures global functional brain damage due to structural disconnection at multiple spatial scales, and (iii) reflects regional abnormalities when calculated at node-level providing specific “fingerprints” potentially useful for distinguishing between different subgroups of patients. This potential is very much in line with the possibility to combine the turbulent framework with the biotype approach, the latter being a data-driven strategy to identify clusters of patients (Brucar et al., 2023). In the field of computational neuropsychiatry, this approach has been used to cluster patients with major depressive disorder based on rs-fMRI data (Drysdale et al., 2017). Interestingly, the authors found different biotypes associated with specific clinical symptoms that showed a differential response to treatment with repetitive transcranial magnetic stimulation (rTMS), suggesting the predictive value of the biotype-based classification.
In addition to improved diagnosis, the turbulent dynamics framework could also provide prognostic biomarkers to characterize the longitudinal recovery trajectory after TBI. In our recent study (Martínez-Molina et al., 2023), we investigated the potential of the turbulent dynamics framework and whole-brain modeling to provide us with neuroimaging biomarkers that uncover TBI progression during one year of spontaneous recovery using a publicly available rs-fMRI dataset (Roy et al., 2017) with moderate-severe patients at the chronic stage. In our study (Figure 1B), we provided evidence of significantly reduced global amplitude turbulence in TBI patients at long distances in the brain, which, as mentioned above, suggests disruptions in the long-range connections that enhance information processing across time and space (Deco et al., 2021b). Node-level turbulence revealed specific resting-state networks “fingerprints” showing the difference between TBI patients and healthy controls (HCs), which could help to assess the neurobiological effectiveness of targeted treatments. Furthermore, we explored the behavioral relevance of these findings by analyzing the correlation between turbulent brain dynamics and a neuropsychological battery to evaluate executive function focusing on attention and working memory. Our results extended previous findings on the association between metastability and cognitive performance (Hellyer et al., 2015) by showing that, at baseline, working memory scores in TBI patients correlated with information cascade flow and amplitude turbulence in the default mode network at long distances. The results from the model-based approach showed a decrease in the global coupling strength in all time points when fitting the model of TBI patients (Martínez-Molina et al., 2023). TBI patients were also characterized by a U-shaped reduction in the susceptibility and information capability during the 1-year recovery trajectory.
This promising preliminary evidence could be further refined by examining the relationship between turbulent brain dynamics and genomic information, which would shed light on the neurobiological basis associated with disruptions in the turbulent regime with a particular focus on neuroinflammation. Indeed, the neuroinflammatory response to injury triggered by microglia activation after TBI can persist for months to years (Ramlackhansingh et al., 2011; Shitaka et al., 2011; Johnson et al., 2013). This persistent neuroinflammation might induce the propagation of abnormal proteins, and could be a causal factor in the subsequent neurodegeneration and further cognitive decline often seen after TBI (Gentleman, 2013). Recent studies have started to incorporate the gene expression profiles obtained from blood samples in Alzheimer's disease (AD) patients and investigated their relationship with neuroimaging biomarkers (Zhao et al., 2023). A gene-enrichment analysis revealed that the genes associated with a significant neuroimaging biomarker for AD were involved in immunity-related processes. Given the abovementioned neuroinflammatory response and risk for neurodegeration after TBI, future gene-enrichment analysis could help to ascertain whether genes associated with neuroinflammation and neurodegeneration might play a role in the underlying neurobiology captured by neuroimaging biomarkers obtained using turbulent dynamics and whole-brain modeling perturbation protocols.
By simulating normal spontaneous brain function, whole-brain computational modeling can provide a unique tool for understanding and predicting the impact of structural connectivity damage on brain dynamics and, more specifically, the effect of anatomical location and extent of the lesion (Alstott et al., 2009). Such an endeavor is particularly relevant to understand the functional consequences of lesions in stroke (Idesis et al., 2023) and TBI patients (Martínez-Molina et al., 2023), often presenting a heterogeneous pattern of lesions. Using the abovementioned longitudinal rs-fMRI dataset, we applied a simulated attack approach (Medaglia et al., 2022) to explore the influence of focal lesions on the brain's responsiveness to in silico perturbations of the whole-brain model (Martínez-Molina et al., 2023). In brief, the overlap between the patient's specific lesion mask and the parcellation scheme was calculated and used to create a group lesion mask to which we applied two structural disconnection methods: one weighted and one binary, the latter being more aggressive with a full deletion of the lesioned node's connectivity. Both approaches of structural disconnection applied to TBI patients led to decreased reactivity to external perturbations. Of note, the lowest values were found for the most aggressive binary approach. This indicates that, at the group level, the effects of lesions on the brain's reactivity are more prominent when there is a great degree of lesion overlap in the patients under study.
Although at present there is no gold standard treatment to promote the recovery of TBI-related cognitive impairments, deep brain stimulation (DBS) within the central lateral (CL) nucleus of the thalamus and the associated medial dorsal tegmental tract has been recently shown to improve executive function in six moderate-severe TBI patients at the chronic stage (Schiff et al., 2023). The CL thalamic neurons project to frontal and striatal regions and might contribute to reverse the cognitive deficits associated with disrupted frontostriatal connectivity after TBI (De Simoni et al., 2018). Despite the beneficial effects of the DBS therapy, there was considerable interindividual variability in the level of efficacy. In this regard, whole-brain computational modeling could help to predict the clinical outcomes of DBS presurgically in order to inform the decision to undergo such an invasive procedure. On the other hand, the selection of the stimulation target was based primarily on their previous work with a single patient in the minimally conscious state (Schiff et al., 2007) and non-human primates studies (Baker et al., 2016; Janson et al., 2021). While this is a scientifically valid approach, whole-brain computational modeling combined with individual structural connectivity could enable the exploration of the optimal brain targets for an individual patient. Although with less spatial resolution than implantable DBS, transcranial temporal interference stimulation (tTIS) (Grossman et al., 2017; Violante et al., 2023) stands as a non-invasive alternative that could also be used to electrically estimulate the striatum (Wessel et al., 2023) and might improve executive dysfunction in TBI patients with altered caudate connectivity (De Simoni et al., 2018). Regardless of the neurostimulation technique used, much more future research is needed before the translation of whole-brain modeling to the clinical setting for optimal brain targeting. In this sense, we envisage three main avenues for future work: (i) improve patient-specific whole-brain computational models to mitigate the impact of overfitting and measurement noise, (ii) study and predict the DBS- or tTIS-induced structural and functional changes as in van Hartevelt et al. (2014), and (iii) evaluate how well the whole-brain model is able to replicate the changes induced by DBS or tTIS after in silico stimulation of the same brain targets.
In summary, in this Mini Review, we have tried to show some progress in the discovery of neuroimaging biomarkers for TBI based on model-free and model-based approaches within the turbulent dynamics framework that have strong potential for application in future clinical practice and treatment trials. The complexity and heterogeneity of TBI calls for a combination of clinical variables and objective pathophysiological biomarkers to improve diagnosis—which to date relies primarily on non-specific clinical evaluations that poorly predict long-term disability—, prognosis and prediction of treatment efficacy. Furthermore, we have discussed how whole-brain computational models can increase our understanding of the effect of focal lesions and to identify optimal stimulation targets which, ultimately, can help to alleviate the long-term suffering associated with TBI.
NM-M: Conceptualization, Project administration, Writing—original draft. YS-P: Methodology, Writing—review & editing. AE: Methodology, Writing—review & editing. MK: Conceptualization, Methodology, Writing—review & editing. GD: Conceptualization, Methodology, Supervision, Writing—review & editing.
The author(s) declare that financial support was received for the research, authorship, and/or publication of this article. NM-M was supported by the Beatriu de Pinós programme (grant agreement no. 2019-BP-00032) from the European Union's Horizon 2020 research and innovation programme under the Marie Sklodowska-Curie agreement no. 801370. YS-P was supported by European Union's Horizon 2020 research and innovation program under the Marie Sklodowska-Curie grant 896354 and the project NEurological MEchanismS of Injury, and Sleep-like cellular dynamics (NEMESIS) (ref. 101071900) funded by the EU ERC Synergy Horizon Europe. AE was supported by the project eBRAIN-Health—Actionable Multilevel Health Data (id 101058516), funded by EU Horizon Europe, and the NODYN Project PID2022-136216NB-I00 financed by the MCIN/AEI/10.13039/501100011033/ FEDER, UE., the Ministry of Science and Innovation, the State Research Agency and the European Regional Development Fund. MK was supported by the Center for Music in the Brain, funded by the Danish National Research Foundation (DNRF117), and Centre for Eudaimonia and Human Flourishing at Linacre College funded by the Pettit and Carlsberg Foundations. GD was also supported by the project NEurological MEchanismS of Injury, and Sleep-like cellular dynamics (NEMESIS) (ref. 101071900) funded by the EU ERC Synergy Horizon Europe and the NODYN Project PID2022-136216NB-I00 financed by the MCIN/AEI/10.13039/501100011033/FEDER, UE., the Ministry of Science and Innovation, the State Research Agency and the European Regional Development Fund.
The authors declare that the research was conducted in the absence of any commercial or financial relationships that could be construed as a potential conflict of interest.
All claims expressed in this article are solely those of the authors and do not necessarily represent those of their affiliated organizations, or those of the publisher, the editors and the reviewers. Any product that may be evaluated in this article, or claim that may be made by its manufacturer, is not guaranteed or endorsed by the publisher.
Alstott, J., Breakspear, M., Hagmann, P., Cammoun, L., and Sporns, O. (2009). Modeling the impact of lesions in the human brain. PLoS Comput. Biol. 5:e1000408. doi: 10.1371/journal.pcbi.1000408
Baker, J. L., Ryou, J.-W., Wei, X. F., Butson, C. R., Schiff, N. D., and Purpura, K. P. (2016). Robust modulation of arousal regulation, performance, and frontostriatal activity through central thalamic deep brain stimulation in healthy nonhuman primates. J. Neurophysiol. 116, 2383–2404. doi: 10.1152/jn.01129.2015
Brucar, L. R., Feczko, E., Fair, D. A., and Zilverstand, A. (2023). Current approaches in computational psychiatry for the data-driven identification of brain-based subtypes. Biol. Psychiatry 93, 704–716. doi: 10.1016/j.biopsych.2022.12.020
Cantor, J. B., Gordon, W., and Gumber, S. (2013). What is post TBI fatigue? NeuroRehabilitation 32, 875–883. doi: 10.3233/NRE-130912
Cruzat, J., Perl, Y. S., Escrichs, A., Vohryzek, J., Timmermann, C., Roseman, L., et al. (2022). Effects of classic psychedelic drugs on turbulent signatures in brain dynamics. Netw. Neurosci. 6, 1104–1124. doi: 10.1162/netn_a_00250
De Filippi, E., Uribe, C., Avila-Varela, D. S., Martínez-Molina, N., Gashaj, V., Pritschet, L., et al. (2021). The menstrual cycle modulates whole-brain turbulent dynamics. Front. Neurosci. 15:753820. doi: 10.3389/fnins.2021.753820
De Simoni, S., Jenkins, P. O., Bourke, N. J., Fleminger, J. J., Hellyer, P. J., Jolly, A. E., et al. (2018). Altered caudate connectivity is associated with executive dysfunction after traumatic brain injury. Brain 141, 148–164. doi: 10.1093/brain/awx309
Deco, G., Kemp, M., and Kringelbach, M. L. (2021a). Leonardo da Vinci and the search for order in neuroscience. Curr. Biol. 31, R704–R709. doi: 10.1016/j.cub.2021.03.098
Deco, G., and Kringelbach, M. L. (2014). Great expectations: using whole-brain computational connectomics for understanding neuropsychiatric disorders. Neuron 84, 892–905. doi: 10.1016/j.neuron.2014.08.034
Deco, G., and Kringelbach, M. L. (2020). Turbulent-like dynamics in the human brain. Cell Rep. 33:108471. doi: 10.1016/j.celrep.2020.108471
Deco, G., Liebana Garcia, S., Sanz Perl, Y., Sporns, O., and Kringelbach, M. L. (2023). The effect of turbulence in brain dynamics information transfer measured with magnetoencephalography. Commun. Phys. 6, 1–8. doi: 10.1038/s42005-023-01192-2
Deco, G., Sanz Perl, Y., Vuust, P., Tagliazucchi, E., Kennedy, H., and Kringelbach, M. L. (2021b). Rare long-range cortical connections enhance human information processing. Curr. Biol. 31, 4436–4448.e5. doi: 10.1016/j.cub.2021.07.064
Dikmen, S. S., Corrigan, J. D., Levin, H. S., Machamer, J., Stiers, W., and Weisskopf, M. G. (2009). Cognitive outcome following traumatic brain injury. J. Head Trauma Rehabil. 24, 430–438. doi: 10.1097/HTR.0b013e3181c133e9
Drysdale, A. T., Grosenick, L., Downar, J., Dunlop, K., Mansouri, F., Meng, Y., et al. (2017). Resting-state connectivity biomarkers define neurophysiological subtypes of depression. Nat. Med. 23, 28–38. doi: 10.1038/nm.4246
Edwards, K. A., Pattinson, C. L., Guedes, V. A., Peyer, J., Moore, C., Davis, T., et al. (2020). Inflammatory cytokines associate with neuroimaging after acute mild traumatic brain injury. Front. Neurol. 11:348. doi: 10.3389/fneur.2020.00348
Ercsey-Ravasz, M., Markov, N. T., Lamy, C., Van Essen, D. C., Knoblauch, K., Toroczkai, Z., et al. (2013). A predictive network model of cerebral cortical connectivity based on a distance rule. Neuron 80, 184–197. doi: 10.1016/j.neuron.2013.07.036
Escrichs, A., Perl, Y. S., Uribe, C., Camara, E., Türker, B., Pyatigorskaya, N., et al. (2022). Unifying turbulent dynamics framework distinguishes different brain states. Commun. Biol. 5:638. doi: 10.1038/s42003-022-03576-6
Feigin, V. L., Theadom, A, Barker-Collo, S., Starkey, N. J., McPherson, K., and Kahan, M. Incidence of traumatic brain injury in New Zealand: a population-based study. Lancet Neurol. (2013) 12:53–64. doi: 10.1016/S1474-4422(12)70262-4.
Gentleman, S. M. (2013). Review: microglia in protein aggregation disorders: friend or foe? Neuropathol. Appl. Neurobiol. 39, 45–50. doi: 10.1111/nan.12017
Gill, J., Latour, L., Diaz-Arrastia, R., Motamedi, V., Turtzo, C., Shahim, P., et al. (2018). Glial fibrillary acidic protein elevations relate to neuroimaging abnormalities after mild TBI. Neurology 91, e1385–e1389. doi: 10.1212/WNL.0000000000006321
Grossman, N., Bono, D., Dedic, N., Kodandaramaiah, S. B., Rudenko, A., Suk, H.-J., et al. (2017). Noninvasive deep brain stimulation via temporally interfering electric fields. Cell 169, 1029–1041.e16. doi: 10.1016/j.cell.2017.05.024
Hellyer, P. J., Scott, G., Shanahan, M., Sharp, D. J., and Leech, R. (2015). Cognitive flexibility through metastable neural dynamics is disrupted by damage to the structural connectome. J. Neurosci. 35, 9050–9063. doi: 10.1523/JNEUROSCI.4648-14.2015
Hoofien, D., Gilboa, A., Vakil, E., and Donovick, P. J. (2001). Traumatic brain injury (TBI) 10-20 years later: a comprehensive outcome study of psychiatric symptomatology, cognitive abilities and psychosocial functioning. Brain Inj. 15, 189–209. doi: 10.1080/026990501300005659
Idesis, S., Allegra, M., Vohryzek, J., Sanz Perl, Y., Faskowitz, J., Sporns, O., et al. (2023). A low dimensional embedding of brain dynamics enhances diagnostic accuracy and behavioral prediction in stroke. Sci. Rep. 13:15698. doi: 10.1038/s41598-023-42533-z
Janson, A. P., Baker, J. L., Sani, I., Purpura, K. P., Schiff, N. D., and Butson, C. R. (2021). Selective activation of central thalamic fiber pathway facilitates behavioral performance in healthy non-human primates. Sci. Rep. 11:23054. doi: 10.1038/s41598-021-02270-7
Johnson, V. E., Stewart, J. E., Begbie, F. D., Trojanowski, J. Q., Smith, D. H., and Stewart, W. (2013). Inflammation and white matter degeneration persist for years after a single traumatic brain injury. Brain 136 (Pt 1), 28–42. doi: 10.1093/brain/aws322
King, N. S., Crawford, S., Wenden, F. J., Moss, N. E., Wade, D. T., and Caldwell, F. E. (1997). Measurement of post-traumatic amnesia: how reliable is it? J. Neurol. Neurosurg. Psychiatr. 62, 38–42. doi: 10.1136/jnnp.62.1.38
Kolmogorov, A. (1941b). The local structure of turbulence in incompressible viscous fluid for very large Reynolds numbers. Proc. USSR Acad. Sci. 30, 299–303.
Majdan, M., Brazinova, A., Rusnak, M., and Leitgeb, J. (2017). Outcome prediction after traumatic brain injury: comparison of the performance of routinely used severity scores and multivariable prognostic models. J. Neurosci. Rural Pract. 8, 20–29. doi: 10.4103/0976-3147.193543
Marshman, L. A. G., Jakabek, D., Hennessy, M., Quirk, F., and Guazzo, E. P. (2013). Post-traumatic amnesia. J. Clin. Neurosci. 20, 1475–1481. doi: 10.1016/j.jocn.2012.11.022
Martínez-Molina, N., Escrichs, A., Sanz-Perl, Y., Sihvonen, A. J., Särkämö, T., Kringelbach, M. L., et al. (2023). The evolution of whole-brain turbulent dynamics during recovery from traumatic brain injury. Netw. Neurosci. 8, 158–177. doi: 10.1162/netn_a_00346
Martínez-Molina, N., Siponkoski, S.-T., and Särkämö, T. (2022). Cognitive efficacy and neural mechanisms of music-based neurological rehabilitation for traumatic brain injury. Ann. N. Y. Acad. Sci. 1515, 20–32. doi: 10.1111/nyas.14800
Medaglia, J. D., Erickson, B. A., Pustina, D., Kelkar, A. S., DeMarco, A. T., Dickens, J. V., et al. (2022). Simulated attack reveals how lesions affect network properties in poststroke aphasia. J. Neurosci. 42, 4913–4926. doi: 10.1523/JNEUROSCI.1163-21.2022
National Academies of Sciences Engineering and Medicine (2022). Traumatic Brain Injury: A Roadmap for Accelerating Progress. Washington, DC: National Academies Press.
Orešič, M., Posti, J. P., Kamstrup-Nielsen, M. H., Takala, R. S. K., Lingsma, H. F., Mattila, I., et al. (2016). Human serum metabolites associate with severity and patient outcomes in traumatic brain injury. EBioMedicine 12, 118–126. doi: 10.1016/j.ebiom.2016.07.015
Perl, Y. S., Mininni, P., Tagliazucchi, E., Kringelbach, M. L., and Deco, G. (2023). Scaling of whole-brain dynamics reproduced by high-order moments of turbulence indicators. Phys. Rev. Res. 5:033183. doi: 10.1103/PhysRevResearch.5.033183
Ramlackhansingh, A. F., Brooks, D. J., Greenwood, R. J., Bose, S. K., Turkheimer, F. E., Kinnunen, K. M., et al. (2011). Inflammation after trauma: microglial activation and traumatic brain injury. Ann. Neurol. 70, 374–383. doi: 10.1002/ana.22455
Richardson, L. (1922). Weather Prediction by Numerical Process. Cambridge: Cambridge University Press.
Roy, A., Bernier, R. A., Wang, J., Benson, M., French, J. J., Good, D. C., et al. (2017). The evolution of cost-efficiency in neural networks during recovery from traumatic brain injury. PLoS ONE 12:e0170541. doi: 10.1371/journal.pone.0170541
Schaefer, A., Kong, R., Gordon, E. M., Laumann, T. O., Zuo, X.-N., Holmes, A. J., et al. (2018). Local-global parcellation of the human cerebral cortex from intrinsic functional connectivity MRI. Cereb. Cortex 28, 3095–3114. doi: 10.1093/cercor/bhx179
Schiff, N. D., Giacino, J. T., Butson, C. R., Choi, E. Y., Baker, J. L., O'Sullivan, K. P., et al. (2023). Thalamic deep brain stimulation in traumatic brain injury: a phase 1, randomized feasibility study. Nat. Med. 29, 3162–3174. doi: 10.1038/s41591-023-02638-4
Schiff, N. D., Giacino, J. T., Kalmar, K., Victor, J. D., Baker, K., Gerber, M., et al. (2007). Behavioural improvements with thalamic stimulation after severe traumatic brain injury. Nature 448, 600–603. doi: 10.1038/nature06041
Sharp, D. J., Scott, G., and Leech, R. (2014). Network dysfunction after traumatic brain injury. Nat. Rev. Neurol. 10, 156–166. doi: 10.1038/nrneurol.2014.15
Shitaka, Y., Tran, H. T., Bennett, R. E., Sanchez, L., Levy, M. A., Dikranian, K., et al. (2011). Repetitive closed-skull traumatic brain injury in mice causes persistent multifocal axonal injury and microglial reactivity. J. Neuropathol. Exp. Neurol. 70, 551–567. doi: 10.1097/NEN.0b013e31821f891f
Teasdale, G., and Jennett, B. (1974). Assessment of coma and impaired consciousness. A practical scale. Lancet 2, 81–84. doi: 10.1016/S0140-6736(74)91639-0
Thelin, E., Al Nimer, F., Frostell, A., Zetterberg, H., Blennow, K., Nyström, H., et al. (2019). A serum protein biomarker panel improves outcome prediction in human traumatic brain injury. J. Neurotrauma 36, 2850–2862. doi: 10.1089/neu.2019.6375
van Hartevelt, T. J., Cabral, J., Deco, G., Müller, A., Green, A. L., Aziz, T. Z., et al. (2014). Neural plasticity in human brain connectivity: the effects of long term deep brain stimulation of the subthalamic nucleus in Parkinson's disease. PLoS ONE 9:e86496. doi: 10.1371/journal.pone.0086496
Violante, I. R., Alania, K., Cassarà, A. M., Neufeld, E., Acerbo, E., Carron, R., et al. (2023). Non-invasive temporal interference electrical stimulation of the human hippocampus. Nat. Neurosci. 26, 1994–2004. doi: 10.1038/s41593-023-01456-8
Wessel, M. J., Beanato, E., Popa, T., Windel, F., Vassiliadis, P., Menoud, P., et al. (2023). Noninvasive theta-burst stimulation of the human striatum enhances striatal activity and motor skill learning. Nat. Neurosci. 26, 2005–2016. doi: 10.1038/s41593-023-01457-7
Whitfield-Gabrieli, S., and Nieto-Castanon, A. (2012). Conn: a functional connectivity toolbox for correlated and anticorrelated brain networks. Brain Connect. 2, 125–141. doi: 10.1089/brain.2012.0073
Wilde, E. A., Wanner, I.-B., Kenney, K., Gill, J., Stone, J. R., Disner, S., et al. (2022). A framework to advance biomarker development in the diagnosis, outcome prediction, and treatment of traumatic brain injury. J. Neurotrauma 39, 436–457. doi: 10.1089/neu.2021.0099
Zhao, K., Chen, P., Alexander-Bloch, A., Wei, Y., Dyrba, M., Yang, F., et al. (2023). A neuroimaging biomarker for individual brain-related abnormalities in neurodegeneration (IBRAIN): a cross-sectional study. eClinicalMedicine 65:102276. doi: 10.1016/j.eclinm.2023.102276
Keywords: traumatic brain injury, nonlinear brain dynamics, turbulence, whole-brain modeling, neuroimaging biomarkers, stratified neurology
Citation: Martínez-Molina N, Sanz-Perl Y, Escrichs A, Kringelbach ML and Deco G (2024) Turbulent dynamics and whole-brain modeling: toward new clinical applications for traumatic brain injury. Front. Neuroinform. 18:1382372. doi: 10.3389/fninf.2024.1382372
Received: 05 February 2024; Accepted: 01 March 2024;
Published: 25 March 2024.
Edited by:
Feliberto De La Cruz, University Hospital Jena, GermanyReviewed by:
Paul Rapp, Uniformed Services University of the Health Sciences, United StatesCopyright © 2024 Martínez-Molina, Sanz-Perl, Escrichs, Kringelbach and Deco. This is an open-access article distributed under the terms of the Creative Commons Attribution License (CC BY). The use, distribution or reproduction in other forums is permitted, provided the original author(s) and the copyright owner(s) are credited and that the original publication in this journal is cited, in accordance with accepted academic practice. No use, distribution or reproduction is permitted which does not comply with these terms.
*Correspondence: Noelia Martínez-Molina, bm9lbGlhLm1hcnRpbmV6QHVwZi5lZHU=
Disclaimer: All claims expressed in this article are solely those of the authors and do not necessarily represent those of their affiliated organizations, or those of the publisher, the editors and the reviewers. Any product that may be evaluated in this article or claim that may be made by its manufacturer is not guaranteed or endorsed by the publisher.
Research integrity at Frontiers
Learn more about the work of our research integrity team to safeguard the quality of each article we publish.