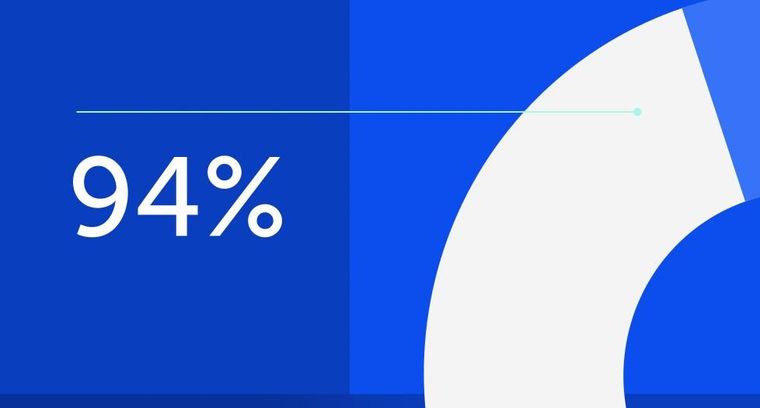
94% of researchers rate our articles as excellent or good
Learn more about the work of our research integrity team to safeguard the quality of each article we publish.
Find out more
ORIGINAL RESEARCH article
Front. Neuroinform., 03 May 2023
Volume 17 - 2023 | https://doi.org/10.3389/fninf.2023.1154916
Background: Several observational studies have explored the relationships between multiple sclerosis (MS) and breast cancer; however, whether an association exists remains unknown.
Methods: We conducted a meta-analysis of observational studies and Mendelian randomization (MR) based on genetic variants to identify the relationship between MS and breast cancer. The observational studies were searched from PubMed, Embase, Web of Science, and Scopus to assess the relationship between MS and breast cancer from inception to 07 Nov 2022. Moreover, we explored the association between genetically pre-disposed MS and breast cancer risk based on an MR study. The summary analysis for MS from two separate databases [International Multiple Sclerosis Genetics Consortium (IMSGC), FinnGen] and the summary analysis for breast cancer from Breast Cancer Association Consortium.
Results: Fifteen cohort studies involving 173,565 female MS patients were included in this meta-analysis. The correlation between MS and breast cancer was not statistically significant [relative ratio (RR) = 1.08, 95% confidence interval (CI) = 0.99–1.17]. In the MR analysis, we did not observe causal associations of genetically determined MS with breast cancer and its subtypes from both the IMSGC and FinnGen datasets.
Conclusion: The meta-analysis of observational and MR based on genetic variants does not support the correlation between MS and breast cancer.
Multiple sclerosis (MS) is a chronic autoimmune demyelinating disease (Anderson et al., 2021), and several experimental studies have found an imbalance between the inflammatory and regulatory T-cell balance in MS patients (Stephens et al., 2009; Quinn and Axtell, 2018). Because the immune system plays an initial role in MS and cancer, it is reasonable to suspect that patients with MS may have an increased incidence of cancer.
Breast cancer is the most frequent malignant tumor worldwide and is a serious threat to women’s lives and health (Sung et al., 2021). If we can identify high-risk factors for breast cancer and enhance screening, we can detect breast cancer early and thus improve the survival rate. Many researchers have explored the relationship between MS and breast cancer. Groome et al. (2022) reported a study that compared the incidence of breast cancer and colorectal cancer in MS patients through a population-based study, and Groome et al. (2022) concluded that MS patients are easily detected to have colorectal cancer but not breast cancer. Bosco-Lévy et al. (2022) found that the risk of breast cancer was higher among MS patients than among population controls (HR = 1.12, 95% CI = 1.03−1.23). Those consistencies may be caused by different study methodologies, designs, or unadjusted confounding factors (Bosco-Lévy et al., 2022). Hence, we performed a Mendelian randomization (MR) study, a new etiological investigation method, combined with previous observational studies to explore the epidemiological relationship between MS and breast cancer.
MR can identify the causal effect between risk factors and health outcomes using genetic variants as instrumental variables (Bowden and Holmes, 2019). MR can provide robust results between exposures and outcomes because mitosis allows for the random allocation of gametes to offspring, which allows for results that are not confounded by potential confounding factors and allows for a better search for the cause of the disease compared to traditional observational studies (Richmond and Davey Smith, 2022). Our study aimed to explore whether MS is a risk or protective factor for breast cancer by performing an observational meta-analysis and MR study.
The overall design of this study is shown in Figure 1. We searched four online databases, PubMed, Embase, Web of Science, and Scopus, for articles published in English up to 23 September 2022. The search keywords were (“multiple sclerosis”) and (“breast neoplasms” or “breast cancer” or “breast tumor”).
Studies that met the following criteria were included in our meta-analysis: (i) cohort studies of patients with MS and (ii) all published articles were in English. (iii) evaluated the association or risk between MS and breast cancer. (iv) sample size not less than 300. (v) Studies provided odds ratios (ORs), relative risks (RRs), hazard ratios (HRs), or standardized incidence ratios (SIRs) with their 95% confidence intervals (CIs) of breast cancer among MS patients. The exclusion criteria were as follows: (i) systematic review, case report, letter, meeting or meta-analysis; and (ii) insufficient data to obtain effect sizes.
Two researchers (Zhang and Fang) extracted the data independently. When disagreements arose, they were resolved through discussion. We extracted the following information: the name of the first author, country, year of publication, sample size, study period (including follow-up), and effect size (OR, RR, HR or SIR) with 95% CI. Two researchers (Fang and Zhang) evaluated the quality of the included studies using the nine-item Newcastle−Ottawa Quality scale. We have set a minimum follow-up period of 5 years, and no points will be awarded for less than 5 years or for not reporting the follow-up period. Concerning completeness of the follow-up, we set 5% as a cutoff level of loss during the follow-up. Those with a quality score of no more than 5 will be excluded.
We extracted the effect size, if available, or calculated it from available data, including OR, RR, HR, or SIR. SIRs are calculated as the ratio of the number of observed cases to the number of expected cases in the exposed population. The 95% CI for log(SIR) was constructed via the term + 1.96/[square root (O)], where O was the observed case (Alder et al., 2006). Since the absolute risk of breast cancer is low, the four types of measures are expected to have similar estimates of RR. Consequently, our final summary results are presented in the form of RR (Larsson et al., 2007). The same statistical methods have been applied in other meta-analysis articles (Siristatidis et al., 2013).
We used STATA version 14.0 (Stata Corporation, College Station, TX, USA) software for data analysis and visualization. Statistical significance is expressed as a pooled P, and a P < 0.05 was considered statistically significant. The Cochran Q-test and I2 statistics were used to measure heterogeneity; P < 0.1 and I2 value > 50% represented substantial heterogeneity (Higgins et al., 2003). The random model was used if strong heterogeneity existed, and subgroup analysis was conducted to detect potential heterogeneity. If there is no heterogeneity, the fixed-effects model will be used (DerSimonian and Laird, 2015). Sensitivity analysis was used to check data stability, and Egger’s test was used to detect publication bias.
We explored the relationship between MS and breast cancer using a two-sample MR study, which applied genetic predictors of exposure to outcome (Emdin et al., 2017). Our MR study had to follow the following three assumptions: (1) There is a strong association between the instrument variants (IVs) and the risk factor. (2) There are no associations between the IVs and any confounders. (3) IVs should not influence outcomes by confounders or other ways (Davey Smith and Hemani, 2014).
Genome-wide association studies (GWAS) of MS were derived from the International Multiple Sclerosis Genetics Consortium (IMSGC) and FinnGen datasets. In brief, the IMSGC analyzed genetic data from 15 GWASs, including 47,429 MS cases and 68,374 controls, all of whom were of European ancestry (International Multiple Sclerosis Genetics Consortium, 2019). The summary data of IMSGC were adjusted for confounding factors, age, sex, immunomodulatory drugs, batch effects, and the first 10 principal components (International Multiple Sclerosis Genetics Consortium, 2019). The diagnosis of MS was defined by the International Classification of Diseases (ICD) from the Finnish R5 release dataset, and there were 1,048 cases and 217,141 controls of Finnish ancestry. Sex, age, and 10 principal components were logistic regression covariates, and the data can also be found in MRCIEU datasets (finn-b-G6_MS). The independent single nucleotide polymorphisms (SNPs) at p < 5 × 10–8 were selected as IVs (linkage disequilibrium: R2 < 0.01; 5,000). We assessed the correlation of each IV with risk factors using the F statistic, with F < 10 representing a weak instrumental variable and exclusion (Pierce et al., 2011).
The summary GWAS of breast cancer was derived from the Breast Cancer Association Consortium (BCAC) with 122,977 cases (ER + breast cancer, 69,501; ER- breast cancer, 21,468) and 105,974 controls of European ancestry (Michailidou et al., 2017). Two genotyping arrays were used for genotyping: iCOGS arrays in 40,178 breast cancer cases and 35,314 controls1 and OncoArray in 68,242 cases and 52,367 controls2. OncoArray and iCOGS were adjusted for country and study, respectively.
Ethical approval and consent for the summary statistics were obtained from the original publication.
The Wald ratio was used to estimate the effect of exposure on the outcome for each SNP. All effects of SNPs were meta-analyzed using the inverse-variance weighted (IVW) method. The IVW method was used as the main analysis to assess the association between MS and breast cancer in our MR study, and both fixed-effect and random-effect models were performed. Moreover, MR−Egger regression, weighted median, weighted mode, simple mode, and robust adjusted profile score (MR-RAPS) were applied to detect the robustness of our results (Bowden et al., 2017).
Several analyses were performed to check heterogeneity and pleiotropy. Cochrane’s Q-value was used to assess heterogeneity. The MR−Egger method was based on the Instrument Strength Independent of Direct Effect (InSIDE) assumption, and the value of the intercept term is far from zero, indicating horizontal pleiotropy (Bowden et al., 2015). The weighted median is more accurate when more than half of the IVs are valid (e.g., due to pleiotropy) (Bowden et al., 2016). MR pleiotropy residual sum and outlier (MR-PRESSO) was used to detect any outlier SNPs and potential horizontal pleiotropy (Verbanck et al., 2018).
All statistical analyses were performed using R software (version 4.1.1) and STATA 12.0. A P-value of <0.05 was considered statistically significant. The “TwoSampleMR,” “mr.raps,” and “MRPRESSO” packages were applied in our MR study. F analysis was used to detect weak instrument variants (F < 10). Was used R2 to estimate the ability of instruments variants present the exposure.
The literature search with PubMed, Embase, Web of Science, and Scopus yielded 2,876 studies. After removal of duplicates, 853 studies remained, and 62 full-text articles were reviewed after screening. Finally, 15 cohort studies were enrolled in our study, which included 173,565 female MS patients (Midgard et al., 1996; Sumelahti et al., 2004; Achiron et al., 2005; Nielsen et al., 2006; Bahmanyar et al., 2009; Hemminki et al., 2012; Kingwell et al., 2012; Sun et al., 2014; Hajiebrahimi et al., 2016; Etemadifar et al., 2017; Nørgaard et al., 2019; Grytten et al., 2020; Johnson et al., 2021; Marrie et al., 2021; Bosco-Lévy et al., 2022; Mariottini et al., 2022). The quality scores of all studies were no more than 6. The flow diagram for the included and excluded studies is shown in Figure 1, and the reasons for exclusion are listed accordingly. The characteristics of all included studies are shown in Table 1.
The forest plot shows the results of this meta-analysis (Figure 2), and the results indicated that there was no relationship between MS and breast cancer [relative ratio (RR) = 1.08, 95% confidence interval (CI): 0.99–1.17]. A random-effects model was performed because of significant heterogeneity among those studies (I2 = 58.2%, p = 0.002). Several subgroup analyses were conducted to detect the potential sources of significant heterogeneity.
Subgroup analysis concerning ancestries did not find an association between MS and breast cancer in Asia or Europe (Asia: RR = 1.52, 95% CI: 0.95–2.44; Europe: RR = 1.05, 95% CI = 0.98–1.13) (Figure 3A). The subgroup analysis based on the number of MS females revealed no relationship between MS and breast cancer (<3,000: RR = 1.28, 95% CI: 0.90–1.82; 3,000–7,000: RR = 1.11, 95% CI = 0.92–1.33; >7,000: RR = 1.03, 95% CI = 0.96–1.33) (Figure 3B). Subgroup analysis did not detect the source of heterogeneity.
Figure 3. (A) Association of MS and breast cancer in subgroups stratified by ancestries and (B) number of MS females.
The results did not show any evidence of publication bias (Begg’s Test 0.553; Egger’s Test = 0.297) (Supplementary Figures 1, 2). Leave-one-out sensitivity analysis indicated that the results were robust (Supplementary Figure 3).
The detailed SNP information is shown in Supplementary Table 1. The F analysis for all SNPs was more than 10, which indicated that no weak instrument variants were detected. The R2 values were 27.3, 27.4, and 26.7% for overall breast cancer, ER+ breast cancer and ER− breast cancer, respectively. There was no evidence that MS is related to overall breast cancer (IVW-fixed OR: 1.001, 95% CI: 0.990–1.024; IVW-random OR: 1.001, 95% CI: 0.991–1.023), ER+ breast cancer (IVW-fixed OR: 1.008, 95% CI: 0.990–1.028; IVW-random OR: 1.008, 95% CI: 0.989–1.029) and ER− breast cancer (IVW-fixed OR: 0.995, 95% CI: 0.968–1.023; IVW-random OR: 0.995, 95% CI: 0.975–1.016) based on the discovery set from the IMSGC dataset, with similar results estimated in other sensitivity analyses (Table 2). MR−Egger and Cochrane’s Q-value results indicated that there was no pleiotropy in this part, and the results are also shown in Table 3. No outliers were detected by performing the MR-PRESSO test for all the estimates.
Ten SNPs were selected as instrument variants (Supplementary Table 2). The F ranged from 29.6 to 259.2. In addition, MS was not a risk or protective factor for overall breast cancer (IVW-fixed OR: 1.000, 95% CI: 0.990–1.010; IVW-random OR: 1.000, 95% CI: 0.987–1.013), ER+ breast cancer (IVW-fixed OR: 1.006, 95% CI: 0.994–1.019; IVW-random OR: 1.006, 95% CI: 0.990–1.023) or ER- breast cancer (IVW-fixed OR: 0.987, 95% CI: 0.968–1.006; IVW-random OR: 0.987, 95% CI: 0.971–1.004) based on the validation set from FinnGen, with similar results assessed in other sensitivity analyses (Table 2). No heterogeneity or pleiotropy results were estimated in this MR study, and the results are also shown in Table 3. No outliers were detected by performing the MR-PRESSO test for all the estimates.
The etiology of MS is unclear, but it is currently thought to be an immune-mediated demyelinating disease involving the central nervous system (Mariottini et al., 2022; Stampanoni Bassi et al., 2022). MS and breast cancer share some common features; most notably, the incidence of both diseases is much higher in women than in men (O’Malley et al., 2015). Numerous studies have explored the relationship between them, but whether MS affects the incidence of breast cancer remains controversial (Hajiebrahimi et al., 2016; Zecca et al., 2021; Groome et al., 2022). We tried to find the association between MS and breast cancer risk through meta-analysis, and finally, we included fifteen cohort studies. However, these studies were significantly different in terms of ancestry, treatment, and other factors. To avoid these confounding factors, we used Mendelian randomization as a supplement. Ultimately, Mendelian instrumental variables were obtained in 47,429 MS patients and validated in another population including 1,048 MS patients.
Fifteen cohort studies from thirteen countries were included in our meta-analysis, and five of them reported a significant relationship between MS and breast cancer risk. Our meta-analysis found that there was no relationship between MS and breast cancer risk (meta-RR = 1.08; 95% CI = 0.99–1.17; P = 0.073). Consistently, a meta-analysis of the relationship between MS and breast cancer conducted by Catalá-López and Tobías (2010), including only five cohort studies (meta-SIR = 1.02; 95% CI = 0.75–1.40). Our updated meta-analysis included 10 additional studies and therefore had increased statistical power. Moreover, Lopez’s meta-analysis study exhibited strong heterogeneity (I2 = 75.3%), and a moderate degree of heterogeneity was found in our study (I2 = 58.2%). The meta-regression did not find the source of heterogeneity. We attempted multiple subgroup analyses but also failed to find a source of heterogeneity, suggesting that the results might be affected by other potential confounders, such as environmental exposure and treatment.
To overcome the inherent limitations of traditional observational articles, such as difficult-to-detect confounders, reverse causation, and various biases (Davey Smith and Hemani, 2014; Burgess et al., 2017), we conducted a two-sample MR method by genetic variants to further investigate the association between MS and the risk of breast cancer. MR analysis is a new epidemiological method that uses genetic variation as a tool to explore the relationship between risk factors and outcomes (Davies et al., 2018). Genetic variation as an instrument variation must meet three basic conditions: (1) Genetic variations are closely associated with exposure. (2) Genetic variations are not associated with confounding factors. (3) Genetic variations do not directly influence outcomes (Davey Smith and Hemani, 2014). The first hypothesis is easier to prove, an F statistic greater than 10 proves that there is no instrumental variable, and all SNPs in our study have an F statistic greater than 10. The proof of the second and third hypotheses is to examine horizontal pleiotropy, which is the focus and difficulty of MR research (Hemani et al., 2018). Several different sensitivity analyses were used to detect and correct for any potential pleiotropic effects on outcomes in our study. The P-value of the intercept of MR−Egger proves that there is no horizontal pleiotropy, and all sensitivity analysis and IVW method results demonstrated that MS was not associated with breast cancer risk. The same conclusion was obtained in replication practice from FinnGen consortia. The lack of detected heterogeneity suggests that the results of our MR study are relatively stable.
A hypothesis to explain the risk of breast cancer identified that chronic inflammation caused by MS may result in weakened activation of the immune system or immune protection against cancer becoming protumorigenic (Hofer et al., 2010). However, some people hold a different view: MS breaks myelin by upregulating immune system activity, which may have a protective effect against cancer. Immune-inflammation-associated helper T1 cells produce large amounts of antitumor factors that help prevent cancer proliferation (Fletcher et al., 2010). Therefore, speculation that it affects breast cancer risk by affecting the immune system remains controversial. A genotyping study found that mutations in the BRCA1 gene are very close to the MS gene, which may be why there are more cases of breast cancer in MS patients than in non-MS cohorts (Holzmann et al., 2013). Of course, this study was a single-family study, so the results may not be very significant. Another problem with this acceptance is that mutations in the BRCA1 gene account for only a small fraction of the causes of breast cancer (Paul and Paul, 2014). Furthermore, drug treatment, not the disease itself, may be the real cause of cancer (Bahmanyar et al., 2009; Dolladille et al., 2021; Bosco-Lévy et al., 2022). The development of disease-modifying therapies (MDTs) has revolutionized the treatment of MS (Florou et al., 2020). However, the modulation of innate immune mechanisms and suppression of the immune system induced by the use of DMT contribute to an increased risk of malignancies, such as breast cancer, lymphoma, and melanoma, in patients treated with these drugs for long periods of time (Conzett et al., 2011; Ragonese et al., 2017; Melamed and Lee, 2019). In addition, the protective role of CD20 B cells and cytotoxic T cells plays an important role in preventing the development of malignancies, and anti-CD20 therapy for MS has been found to play an important role in the development of cancer (Kelsey et al., 2021). A study found that cancer rates are three times higher among MS patients who receive immunotherapy than among those who never receive immunotherapy. This study found a particular trend toward breast cancer and cancer in the digestive tract, urinary tract, and skin (Lebrun et al., 2008). However, the 15 studies we included did not indicate whether patients with MS received the MDT or other types of treatment, which prevented us from performing subgroup analyses by treatment modality, which is a limitation of our study.
Several advantages of our study exist. First, our meta-analysis included fifteen cohort studies with a large study population (173,565 female patients with MS), providing more reliable results than previous meta-analyses (13,419 female patients with MS) (Catalá-López and Tobías, 2010). Second, this is the first MR study to estimate the causal relationship between MS and breast cancer. Our study design strictly followed the three assumptions of MR (VanderWeele et al., 2014). Third, we used two completely different cohorts of MS in our MR study, and the primary results and sensitivity analysis showed a robust conclusion, all of which suggest that the outcome was stable.
In conclusion, the results of this large MR study and meta-analysis do not support an association of MS with breast cancer risk, and our conclusion may provide insights for future studies.
The original contributions presented in this study are included in the article/Supplementary material, further inquiries can be directed to the corresponding author.
TF and ZZ designed this study and performed the data analyses. TF conducted the analyses and drafted the manuscript. LZ directed the analytical strategy and supervised the study from conception to completion. TF, HZ, and WW revised the manuscript draft. All authors contributed to the interpretation of data and critically revised the manuscript.
We gratefully thank American Journal Experts (www.aje.com) for English language editing.
The authors declare that the research was conducted in the absence of any commercial or financial relationships that could be construed as a potential conflict of interest.
All claims expressed in this article are solely those of the authors and do not necessarily represent those of their affiliated organizations, or those of the publisher, the editors and the reviewers. Any product that may be evaluated in this article, or claim that may be made by its manufacturer, is not guaranteed or endorsed by the publisher.
The Supplementary Material for this article can be found online at: https://www.frontiersin.org/articles/10.3389/fninf.2023.1154916/full#supplementary-material
Supplementary Figure 1 | Funnel plot of Begg’s test for publication bias.
Supplementary Figure 2 | Funnel plot of Egger’s test for publication bias.
Supplementary Figure 3 | Sensitivity analysis of the leave-one-out test for the meta-analysis.
Achiron, A., Barak, Y., Gail, M., Mandel, M., Pee, D., Ayyagari, R., et al. (2005). Cancer incidence in multiple sclerosis and effects of immunomodulatory treatments. Breast Cancer Res. Treat. 89, 265–270. doi: 10.1007/s10549-004-2229-4
Alder, N., Fenty, J., Warren, F., Sutton, A., Rushton, L., Jones, D., et al. (2006). Meta-analysis of mortality and cancer incidence among workers in the synthetic rubber-producing industry. Am. J. Epidemiol. 164, 405–420. doi: 10.1093/aje/kwj252
Anderson, A., Krysko, K., Rutatangwa, A., Krishnakumar, T., Chen, C., Rowles, W., et al. (2021). Clinical and radiologic disease activity in pregnancy and postpartum in MS. Neurol. Neuroimmunol. Neuroinflamm. 8:e959. doi: 10.1212/NXI.0000000000000959
Bahmanyar, S., Montgomery, S., Hillert, J., Ekbom, A., and Olsson, T. (2009). Cancer risk among patients with multiple sclerosis and their parents. Neurology 72, 1170–1177. doi: 10.1212/01.wnl.0000345366.10455.62
Bosco-Lévy, P., Foch, C., Grelaud, A., Sabidó, M., Lacueille, C., Jové, J., et al. (2022). Incidence and risk of cancer among multiple sclerosis patients: A matched population-based cohort study. Eur. J. Neurol. 29, 1091–1099. doi: 10.1111/ene.15226
Bowden, J., and Holmes, M. (2019). Meta-analysis and Mendelian randomization: A review. Res. Synth. Methods 10, 486–496. doi: 10.1002/jrsm.1346
Bowden, J., Davey Smith, G., and Burgess, S. (2015). Mendelian randomization with invalid instruments: Effect estimation and bias detection through Egger regression. Int. J. Epidemiol. 44, 512–525. doi: 10.1093/ije/dyv080
Bowden, J., Del Greco, M., Minelli, C., Davey Smith, G., Sheehan, N., and Thompson, J. (2017). A framework for the investigation of pleiotropy in two-sample summary data Mendelian randomization. Stat. Med. 36, 1783–1802. doi: 10.1002/sim.7221
Bowden, J., Del Greco, M., Minelli, C., Davey Smith, G., Sheehan, N., and Thompson, J. (2016). Assessing the suitability of summary data for two-sample Mendelian randomization analyses using MR−Egger regression: The role of the I2 statistic. Int. J. Epidemiol. 45, 1961–1974. doi: 10.1093/ije/dyw220
Burgess, S., Small, D., and Thompson, S. G. (2017). A review of instrumental variable estimators for Mendelian randomization. Stat. Methods Med. Res. 26, 2333–2355. doi: 10.1177/0962280215597579
Catalá-López, F., and Tobías, A. (2010). [Incidence of breast cancer in women with multiple sclerosis: Systematic review and meta-analysis of observational cohort studies]. Rev. Neurol. 51, 513–519.
Conzett, K., Kolm, I., Jelcic, I., Kamarachev, J., Dummer, R., Braun, R., et al. (2011). Melanoma occurring during treatment with fingolimod for multiple sclerosis: A case report. Arch. Dermatol. 147, 991–992. doi: 10.1001/archdermatol.2011.212
Davey Smith, G., and Hemani, G. (2014). Mendelian randomization: Genetic anchors for causal inference in epidemiological studies. Hum. Mol. Genet. 23, R89–R98. doi: 10.1093/hmg/ddu328
Davies, N., Holmes, M., and Davey Smith, G. (2018). Reading Mendelian randomization studies: A guide, glossary, and checklist for clinicians. BMJ 362:k601. doi: 10.1136/bmj.k601
DerSimonian, R., and Laird, N. (2015). Meta-analysis in clinical trials revisited. Contemp. Clin. Trials 45(Pt A), 139–145. doi: 10.1016/j.cct.2015.09.002
Dolladille, C., Chrétien, B., Peyro-Saint-Paul, L., Alexandre, J., Dejardin, O., Fedrizzi, S., et al. (2021). Association between disease-modifying therapies prescribed to persons with multiple sclerosis and cancer: A WHO pharmacovigilance database analysis. Neurotherapeutics 18, 1657–1664. doi: 10.1007/s13311-021-01073-y
Emdin, C., Khera, A., and Kathiresan, S. (2017). Mendelian randomization. JAMA 318, 1925–1926. doi: 10.1001/jama.2017.17219
Etemadifar, M., Jahanbani-Ardakani, H., Ghaffari, S., Fereidan-Esfahani, M., Changaei, H., Aghadoost, N., et al. (2017). Cancer risk among patients with multiple sclerosis: A cohort study in Isfahan, Iran. Caspian J. Intern. Med. 8, 172–177.
Fletcher, J., Lalor, S., Sweeney, C., Tubridy, N., and Mills, K. H. (2010). T cells in multiple sclerosis and experimental autoimmune encephalomyelitis. Clin Exp. Immunol. 162, 1–11. doi: 10.1111/j.1365-2249.2010.04143.x
Florou, D., Katsara, M., Feehan, J., Dardiotis, E., and Apostolopoulos, V. (2020). Anti-CD20 agents for multiple sclerosis: Spotlight on ocrelizumab and ofatumumab. Brain Sci. 10:758. doi: 10.3390/brainsci10100758
Groome, P., Webber, C., Maxwell, C., McClintock, C., Seitz, D., Mahar, A., et al. (2022). Multiple sclerosis and the cancer diagnosis: Diagnostic route, cancer stage, and the diagnostic interval in breast and colorectal cancer. Neurology 98, e1798–e1809. doi: 10.1212/WNL.0000000000200163
Grytten, N., Myhr, K., Celius, E., Benjaminsen, E., Kampman, M., Midgard, R., et al. (2020). Risk of cancer among multiple sclerosis patients, siblings, and population controls: A prospective cohort study. Mult. Scler. 26, 1569–1580. doi: 10.1177/1352458519877244
Hajiebrahimi, M., Montgomery, S., Burkill, S., and Bahmanyar, S. (2016). Risk of premenopausal and postmenopausal breast cancer among multiple sclerosis patients. PLoS One 11:e0165027. doi: 10.1371/journal.pone.0165027
Hemani, G., Bowden, J., and Davey Smith, G. (2018). Evaluating the potential role of pleiotropy in Mendelian randomization studies. Hum. Mol. Genet. 27, R195–R208. doi: 10.1093/hmg/ddy163
Hemminki, K., Liu, X., Ji, J., Försti, A., Sundquist, J., and Sundquist, K. (2012). Effect of autoimmune diseases on risk and survival in female cancers. Gynecol. Oncol. 127, 180–185. doi: 10.1016/j.ygyno.2012.07.100
Higgins, J., Thompson, S., Deeks, J., and Altman, D. (2003). Measuring inconsistency in meta-analyses. Bmj. 327, 557–560. doi: 10.1136/bmj.327.7414.557
Hofer, S., Linnebank, M., Weller, M., Bahmanyar, S., Montgomery, S., Hillert, J., et al. (2010). Cancer risk among patients with multiple sclerosis and their parents. Neurology 74, 614–5; authorrely 5. doi: 10.1212/WNL.0b013e3181c777b7
Holzmann, C., Bauer, I., and Meyer, P. (2013). Co-occurrence of multiple sclerosis and cancer in a BRCA1 positive family. Eur. J. Med. Genet. 56, 577–579. doi: 10.1016/j.ejmg.2013.07.006
International Multiple Sclerosis Genetics Consortium. (2019). Multiple sclerosis genomic map implicates peripheral immune cells and microglia in susceptibility. Science 365:eaav7188.
Johnson, D., Reynolds, K., Poole, B., Montierth, M., Todd, V., Barnado, A., et al. (2021). Contribution of viral infection to risk for cancer in systemic lupus erythematosus and multiple sclerosis. PLoS One 16:e0243150. doi: 10.1371/journal.pone.0243150
Kelsey, A., Casinelli, G., Tandon, M., and Sriwastava, S. (2021). Breast carcinoma after ocrelizumab therapy in multiple sclerosis patients: A case series and literature review. J. Cent. Nerv. Syst. Dis. 13:11795735211037785. doi: 10.1177/11795735211037785
Kingwell, E., Bajdik, C., Phillips, N., Zhu, F., Oger, J., Hashimoto, S., et al. (2012). Cancer risk in multiple sclerosis: Findings from British Columbia, Canada. Brain. 135(Pt 10), 2973–2979. doi: 10.1093/brain/aws148
Larsson, S., Mantzoros, C., and Wolk, A. (2007). Diabetes mellitus and risk of breast cancer: A meta-analysis. Int. J. Cancer 121, 856–862. doi: 10.1002/ijc.22717
Lebrun, C., Debouverie, M., Vermersch, P., Clavelou, P., Rumbach, L., de Seze, J., et al. (2008). Cancer risk and impact of disease-modifying treatments in patients with multiple sclerosis. Mult. Scler. 14, 399–405. doi: 10.1177/1352458507083625
Mariottini, A., Forci, B., Gualdani, E., Romoli, M., Repice, A., Barilaro, A., et al. (2022). Incidence of malignant neoplasms and mortality in people affected by multiple sclerosis in the epoch of disease-modifying treatments: A population-based study on Tuscan residents. Mult. Scler. Relat. Disord. 60:103679. doi: 10.1016/j.msard.2022.103679
Marrie, R., Maxwell, C., Mahar, A., Ekuma, O., McClintock, C., Seitz, D., et al. (2021). Cancer incidence and mortality rates in multiple sclerosis: A matched cohort study. Neurology 96, e501–e512. doi: 10.1212/WNL.0000000000011219
Melamed, E., and Lee, M. (2019). Multiple sclerosis and cancer: The ying-yang effect of disease modifying therapies. Front. Immunol. 10:2954. doi: 10.3389/fimmu.2019.02954
Michailidou, K., Lindström, S., Dennis, J., Beesley, J., Hui, S., Kar, S., et al. (2017). Association analysis identifies 65 new breast cancer risk loci. Nature 551, 92–94. doi: 10.1038/nature24284
Midgard, R., Glattre, E., Grønning, M., Riise, T., Edland, A., and Nyland, H. (1996). Multiple sclerosis and cancer in Norway. A retrospective cohort study. Acta Neurol. Scand. 93, 411–415. doi: 10.1111/j.1600-0404.1996.tb00019.x
Nielsen, N., Rostgaard, K., Rasmussen, S., Koch-Henriksen, N., Storm, H., Melbye, M., et al. (2006). Cancer risk among patients with multiple sclerosis: A population-based register study. Int. J. Cancer 118, 979–984. doi: 10.1002/ijc.21437
Nørgaard, M., Veres, K., Didden, E., Wormser, D., and Magyari, M. (2019). Multiple sclerosis and cancer incidence: A Danish nationwide cohort study. Mult. Scler. Relat. Disord. 28, 81–85. doi: 10.1016/j.msard.2018.12.014
O’Malley, P., Mulla, Z., and Nesic, O. (2015). Multiple sclerosis and breast cancer. J. Neurol. Sci. 356, 137–141. doi: 10.1016/j.jns.2015.06.033
Paul, A., and Paul, S. (2014). The breast cancer susceptibility genes (BRCA) in breast and ovarian cancers. Front. Biosci. 19:605–618. doi: 10.2741/4230
Pierce, B., Ahsan, H., and Vanderweele, T. (2011). Power and instrument strength requirements for Mendelian randomization studies using multiple genetic variants. Int. J. Epidemiol. 40, 740–752. doi: 10.1093/ije/dyq151
Quinn, J., and Axtell, R. (2018). Emerging role of follicular T Helper cells in multiple sclerosis and experimental autoimmune encephalomyelitis. Int. J. Mol. Sci. 19:3233. doi: 10.3390/ijms19103233
Ragonese, P., Aridon, P., Vazzoler, G., Mazzola, M., Lo Re, V., Lo Re, M., et al. (2017). Association between multiple sclerosis, cancer risk, and immunosuppressant treatment: A cohort study. BMC Neurol. 17:155. doi: 10.1186/s12883-017-0932-0
Richmond, R., and Davey Smith, G. (2022). Mendelian randomization: Concepts and scope. Cold Spring Harb. Perspect. Med. 12:a040501. doi: 10.1101/cshperspect.a040501
Siristatidis, C., Sergentanis, T., Kanavidis, P., Trivella, M., Sotiraki, M., Mavromatis, I., et al. (2013). Controlled ovarian hyperstimulation for IVF: Impact on ovarian, endometrial and cervical cancer–a systematic review and meta-analysis. Hum. Reprod. Update 19, 105–123. doi: 10.1093/humupd/dms051
Stampanoni Bassi, M., Iezzi, E., and Centonze, D. (2022). Multiple sclerosis: Inflammation, autoimmunity and plasticity. Handb. Clin. Neurol. 184, 457–470. doi: 10.1016/B978-0-12-819410-2.00024-2
Stephens, L., Malpass, K., and Anderton, S. (2009). Curing CNS autoimmune disease with myelin-reactive Foxp3+ Treg. Eur. J. Immunol. 39, 1108–1117. doi: 10.1002/eji.200839073
Sumelahti, M., Pukkala, E., and Hakama, M. (2004). Cancer incidence in multiple sclerosis: A 35-year follow-up. Neuroepidemiology 23, 224–227. doi: 10.1159/000079947
Sun, L., Lin, C., Chung, C., Liang, J., Sung, F., and Kao, C. (2014). Increased breast cancer risk for patients with multiple sclerosis: A nationwide population-based cohort study. Eur. J. Neurol. 21, 238–244. doi: 10.1111/ene.12267
Sung, H., Ferlay, J., Siegel, R., Laversanne, M., Soerjomataram, I., Jemal, A., et al. (2021). Global cancer statistics 2020: GLOBOCAN estimates of incidence and mortality worldwide for 36 cancers in 185 countries. CA Cancer J. Clin. 71, 209–249. doi: 10.3322/caac.21660
VanderWeele, T., Tchetgen Tchetgen, E., Cornelis, M., and Kraft, P. (2014). Methodological challenges in Mendelian randomization. Epidemiology 25, 427–435. doi: 10.1097/EDE.0000000000000081
Verbanck, M., Chen, C., Neale, B., and Do, R. (2018). Detection of widespread horizontal pleiotropy in causal relationships inferred from Mendelian randomization between complex traits and diseases. Nat. Genet. 50, 693–698. doi: 10.1038/s41588-018-0099-7
Keywords: multiple sclerosis, breast cancer, Mendelian randomization, meta-analysis, genetic variants
Citation: Fang T, Zhang Z, Zhou H, Wu W and Zou L (2023) Multiple sclerosis and breast cancer risk: a meta-analysis of observational and Mendelian randomization studies. Front. Neuroinform. 17:1154916. doi: 10.3389/fninf.2023.1154916
Received: 31 January 2023; Accepted: 27 March 2023;
Published: 03 May 2023.
Edited by:
Anneke Van Der Walt, Faculty of Medicine, Nursing and Health Sciences, Monash University, AustraliaReviewed by:
Francesca Bridge, Monash University, AustraliaCopyright © 2023 Fang, Zhang, Zhou, Wu and Zou. This is an open-access article distributed under the terms of the Creative Commons Attribution License (CC BY). The use, distribution or reproduction in other forums is permitted, provided the original author(s) and the copyright owner(s) are credited and that the original publication in this journal is cited, in accordance with accepted academic practice. No use, distribution or reproduction is permitted which does not comply with these terms.
*Correspondence: Liqun Zou, em91bGlxdW4xOTcxQDE2My5jb20=
Disclaimer: All claims expressed in this article are solely those of the authors and do not necessarily represent those of their affiliated organizations, or those of the publisher, the editors and the reviewers. Any product that may be evaluated in this article or claim that may be made by its manufacturer is not guaranteed or endorsed by the publisher.
Research integrity at Frontiers
Learn more about the work of our research integrity team to safeguard the quality of each article we publish.