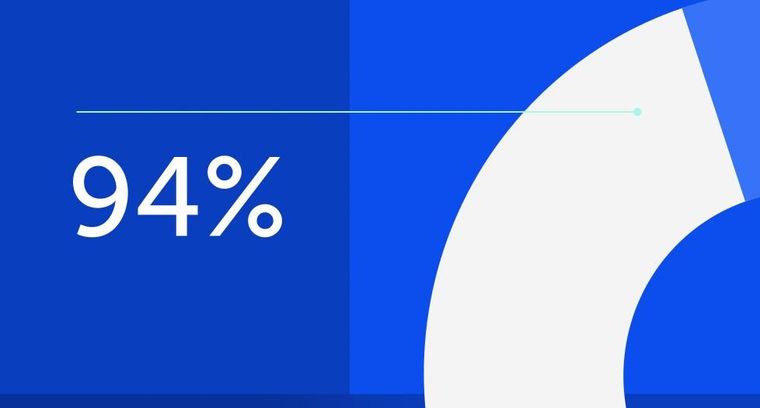
94% of researchers rate our articles as excellent or good
Learn more about the work of our research integrity team to safeguard the quality of each article we publish.
Find out more
ORIGINAL RESEARCH article
Front. Neuroinform., 08 September 2022
Volume 16 - 2022 | https://doi.org/10.3389/fninf.2022.962197
This article is part of the Research TopicMulti-Modality Imaging and Multi-Omics Approach to Pediatric Neurogenetic DisordersView all 5 articles
Rett syndrome (RTT) is a neurodevelopmental disorder caused by loss-of-function variants in the MECP2 gene, currently with no cure. Neuroimaging is an important tool for obtaining non-invasive structural and functional information about the in vivo brain. Multiple approaches to magnetic resonance imaging (MRI) scans have been utilized effectively in RTT patients to understand the possible pathological basis. This study combined developmental evaluations with clinical severity, T1-weighted imaging, and diffusion tensor imaging, aiming to explore the structural alterations in cohorts of young girls with RTT, idiopathic autism spectrum disorder (ASD), or typical development. Voxel-based morphometry (VBM) was used to determine the voxel-wised volumetric characteristics of gray matter, while tract-based spatial statistics (SPSS) was used to obtain voxel-wised properties of white matter. Finally, a correlation analysis between the brain structural alterations and the clinical evaluations was performed. In the RTT group, VBM revealed decreased gray matter volume in the insula, frontal cortex, calcarine, and limbic/paralimbic regions; TBSS demonstrated decreased fractional anisotropy (FA) and increased mean diffusivity (MD) mainly in the corpus callosum and other projection and association fibers such as superior longitudinal fasciculus and corona radiata. The social impairment quotient and clinical severity were associated with these morphometric alterations. This monogenic study with an early stage of RTT may provide some valuable guidance for understanding the disease pathogenesis. At the same time, the pediatric-adjusted analytic pipelines for VBM and TBSS were introduced for significant improvement over classical approaches for MRI scans in children.
Rett syndrome (RTT; OMIM #312750) is a neurodevelopmental disorder predominantly affecting females that is caused by loss-of-function variants in the X-linked gene encoding methyl-CpG-binding protein 2 (MECP2; Amir et al., 1999; Ip et al., 2018). RTT is characterized by relatively normal development during the first 6–18 months of life followed by stagnation regression of speech and social skills, cognitive disabilities, gait dysfunctions, and stereotypic hand movements (Neul et al., 2010; Katz et al., 2016). These behavioral impairments have been associated with global atrophy of the brain and, at the cellular level, the reduced cell body of the neuron as well as the decreased dendritic length (Reiss et al., 1993; Armstrong et al., 1995; Bauman et al., 1995; Subramaniam et al., 1997).
As a non-invasive tool, magnetic resonance imaging (MRI) has been applied to patients with RTT for localizing and quantifying anatomical differences in the past decade (Casanova et al., 1991; Reiss et al., 1993; Subramaniam et al., 1997). Some recent morphometric and volumetric studies with relatively novel methods applying voxel-based or surface-based measurements have identified selective gray matter reductions in specific areas (e.g., frontal and temporal lobes, caudate nucleus, thalamus, and cerebellum) as well as decreased FA values in the main fibers comprising the limbic system (e.g., corpus callosum, internal capsule, and frontal white matter; Carter et al., 2008; Mahmood et al., 2010; Shiohama et al., 2019). However, these previous studies used low-resolution imaging, subjects from a wide age range, or a very small sample size.
Using modern neuroimaging techniques, voxel-based morphometry (VBM) and tract-based spatial statistics (TBSS) make it possible to perform automated and unbiased whole brain analysis to determine the voxel-wised alterations of neural structures (Gunbey et al., 2017; Papma et al., 2017). It is worth mentioning that VBM was based on scans from high-resolution T1-weighted sequences and TBSS could derive data from DTI sequences even with fewer directions. Both of these two sequences are broadly available in routine clinical protocols. Unfortunately, the tissue probabilistic maps or brain templates embedded in the classical procedures of VBM and TBSS analyses were generated from scans of adults' brains. Thus, pediatric population-specific pipelines are highly recommended to solve the methodological limitations of traditional VBM and TBSS procedures when applied to datasets from children.
In this study, we employed VBM and TBSS to obtain the voxel-wised volumetric characteristics of gray matter as well as voxel-wised properties of white matter in cohorts of young girls with RTT, idiopathic autism spectrum disorder (ASD), and typical development. In this study, we primarily aimed to investigate the morphometric abnormalities and the correlations with some clinical features in young children with RTT and secondarily tried to suggest the pediatric-adjusted analytic pipelines for VBM and TBSS.
A total of 28 girls diagnosed with Rett syndrome based on the up-to-date diagnostic criteria (Neul et al., 2010) and confirmed with MECP2 variants were consecutively recruited between February 2017 and November 2021 from the Division of Child Health Care, Children's Hospital of Fudan University. Following quality control, the imaging data of three RTT girls were excluded. Age-matched 30 typically developing (TD) girls were recruited as TD controls, and three of them were excluded after an image quality check. They had an MRI scan because they had their first episode of febrile convulsion, paroxysmal dizziness, or headache, but otherwise with normal development. Age-matched 32 ASD girls without known genetic etiology were recruited as idiopathic ASD controls, and four of them were excluded after an image quality check. ASD was diagnosed based on the Diagnostic and Statistical Manual of Mental Disorders, 5th Revision (DSM-5; Battle, 2013) and the Autism Diagnostic Observation Schedule-The Second Edition (ADOS-2; Gotham et al., 2007). Children with cerebral palsy, or other neurologic or degenerative diseases, or with recognizable lesions or abnormalities on scans, were excluded. The Griffiths Development Scales-Chinese (GDS-C), which consists of five developmental domains including gross motor, fine motor, social, language, and performance, was conducted to assess developmental and cognitive levels (Luiz et al., 2004; Tso et al., 2018). The Rett Syndrome Severity Scale (RSSS; Hoffbuhr et al., 2001; Kaufmann et al., 2012), which consists of seven domains including seizures, respiratory abnormalities, scoliosis, walking ability, hand use, speech, and sleep, was applied to assess the clinical severity of RTT.
This study was approved by the Ethics Committee of the Children's Hospital of Fudan University, and all of the procedures were in accordance with the Declaration of Helsinki.
All MRI images were scanned on a GE 3.0 Tesla Discovery MR750 system (GE Medical Systems, Milwaukee, WI) with an eight-channel head coil at the Radiology Department after parental consent. Approximately 1 h before the MRI scan, children were routinely sedated in the “Sedation Center” under the supervision of an anesthesiologist or licensed pediatrician. Chloral hydrate was given at a dose of 50 mg/kg orally, and vital signs were monitored during the scan. The high-resolution T1-weighted BRAVO (BRA in Volume imaging) sequence was performed, and the spin-echo, echo planar sequence was used to obtain the diffusion tensor imaging (DTI). T1-weighted sequence parameters: TR (repetition time) = 8.2 ms; TE (echo time) = 3.2 ms; voxel size = 1 × 1 × 1 mm3; matrix = 256 × 256; flip angle = 12°; gap = 0; FOV = 256 mm. DTI sequence parameters: 15 directions; b = 1,000 s/mm2; TR = 4,600 ms; TE = 87.4 ms; voxel size = 2*2*4 mm3; matrix = 128 × 128; flip angel = 90°; gap = 0; FOV = 240 mm; 15 b-factors 0 and 1,000 s/mm2.
Each scan was visually inspected and images containing obvious artifacts or with a low image quality rating (IQR; <0.8) were excluded. All of the original DICOM imaging files were first converted to NifTi format using the dcm2niigui toolbox within the MRIcron package (www.mricro.com/mricron).
VBM analyses were conducted using the CAT12 toolbox (http://dbm.neuro.uni-jena.de/cat/; Gaser and Dahnke, 2016) implemented in the Statistical Parametric Mapping software package (SPM12; http://www.fil.ion.ucl.ac.uk/spm/software/spm12/; Ashburner and Friston, 2005) on the platform of Matlab (version R2019b, Mathworks Inc., USA). Population-specific tissue probability maps were created using the Template-O-Matic toolbox (Wilke et al., 2008). A fast diffeomorphic registration algorithm (Diffeomorphic Anatomical Registration using Exponentiated Lie algebra, DARTEL) was then applied to these tissue probability maps to produce the templates for subsequent normalization and segmentation. Gray matter segmented images, which aligned to the population-specific template, were then submitted to the Check Sample Homogeneity through the embedded function in CAT-12. Then, the segmented gray matter images were smoothed with a 6 mm full-width at half maximum (FWHM) Gaussian kernel. At the end of this preprocessing, the modulated, normalized, and smoothed gray matter imaging data were obtained for further statistical analyses (Figure 1 for pipeline demonstration).
Figure 1. Pediatric-adjusted pipelines for VBM and TBSS. VBM, voxel-based morphometry; TBSS, tract-based spatial statistics; GM, gray matter; DTI, diffusion tensor imaging; DWI, diffusion-weighted image; FA, fractional anisotropy; MD, mean diffusivity; GLM, general linear model; TFCE, threshold-free cluster enhancement.
DTI data were analyzed using the FSL software (Functional MRI of the Brain Software Library, FMRIB; Jenkinson et al., 2012). Then, the preprocessing was conducted with corrections for eddy current-induced artifacts and head motions, removing non-brain tissues, and estimating the diffusion tensor using the FDT toolbox embedded in FSL. The maps for fractional anisotropy (FA) and mean diffusivity (MD) were then calculated. Then, TBSS was applied to assess group-level differences in the DTI parameters (FA and MD; Smith et al., 2007). To adjust for pediatric imaging, a study-specific image registration procedure was applied. Then each subject's FA image was non-linearly aligned with every other's images. The FA image with the smallest average warp score obtained from registration with every other's images was identified as the “best representative” template as a target for non-linear registration. This “best representative” target image was then affine-aligned into the Montreal Neurological Institute's (MNI) 152 standard space. Every FA map was transformed non-linearly to the target image with the affine transform from that target to the MNI152 space. Then, the mean FA images and mean FA skeletons were computed as the input for TBSS. Statistical analyses were conducted based on projecting all subject's FA and MD values, respectively, onto the mean FA skeleton and submission of the 4D-projected FA and MD data (Figure 1 for pipeline demonstration).
The statistical package SPSS 20.0 was applied for the demographic and clinical data analyses. The statistical significance level was set at p < 0.05. Total intracranial volume (TIV) and age were included as nuisance covariates.
For VBM, a general linear model (GLM, specified second level) was applied on the voxel-wised level. For TBSS, non-parametric permutation tests were applied for group comparisons of both FA and MD values. The statistical maps were processed at the cluster level with threshold-free cluster enhancement (TFCE). Then, an analysis of covariance (ANCOVA) was conducted among the three groups, and significant clusters were further picked up as masks for post-hoc tests. Post-hoc t-tests were then used to compare each two groups (RTT vs. ASD; RTT vs. TD; ASD vs. TD). The significant results of ANCOVA were included as an explicit mask for the post-hoc between-group tests. Family-wise error (FWE) corrected at p < 0.05 was set as a significant level with a minimum cluster size of 100 voxels.
To further investigate the correlations between clinical features and morphometric abnormalities, we first defined regions of interest (ROIs) with significant VBM and TBSS results between the RTT group and ASD controls. Then, the specific gray matter volumes of each subject were extracted for the above gray matter ROIs using ImCalc toolbox embedded SPM12 and “fslmaths” tools from FSL. FA and MD values of each subject were extracted based on the TBSS ROIs separately applying “cluster” and “fslmaths” tools embedded in FSL. Linear regression analyses were then performed for multiple corrections with a statistically significant level at p < 0.05.
All the children from three groups are young girls with no significant differences in age (for RTT group, mean ± SD: 3.5 ± 1.25 years; for ASD group, mean ± SD: 3.6 ± 1.45 years; and for TD group, mean ± SD: 3.8 ± 1.22 years) between groups (all p-values > 0.05). Compared to the ASD group, girls with RTT exhibited significantly worse performance in all of the developmental domains of the Griffith Development Scales (all 5 domains: p < 0.0001). The RSSS clinical severity score was 5.76 ± 1.17 (mean ± SD) in the RTT group. No group differences were found in all of the imaging quality control parameters, including the six absolute head motion parameters and IQRs (for RTT group, mean ± SD: 0.886 ± 0.03; for ASD group, mean ± SD: 0.884± 0.02; and for TD group, mean ± SD: 0.883 ± 0.02; p > 0.05). Detailed demographic information and clinical characteristics are presented in Table 1.
At the global level, morphometry analysis of the whole brain showed that the RTT group exhibited a significant reduction in TIV, GMV, and WMV compared to ASD and TD controls (Table 1 and Figure 2).
Figure 2. Global-leveled ANCOVA analysis showed a significant difference in gray and white matter parameters among the three groups. RTT group exhibited significantly decreased GMV, WMV, FA, and increased MD values compared to ASD and TD controls. GMV, gray matter volume; WMV, white matter volume; FA, fractional anisotropy; MD, mean diffusivity; NS, not significant.
VBM analysis and group comparisons were conducted between RTT group (n = 25), ASD controls (n = 28), and TD controls (n = 25). Through the voxel-wise ANCOVA analysis of brain volume, we found significant differences among the three groups with 8 clusters mainly in the regions of the insula, superior frontal cortex, middle frontal cortex, inferior frontal cortex, orbitofrontal cortex, calcarine, precuneus, rectus, cuneus, putamen, and hippocampus (p < 0.05, FWE corrected).
In the post-hoc analysis, the RTT group showed significantly decreased gray matter volume compared to TD controls in four clusters (Table 2). The number of voxels in the 4 clusters was 621, 16,836, 3,818, and 1,057, respectively. The peak MNI regions were left hippocampus with coordinate (−17, −10.5, −22.5), left middle frontal cortex (−33, 49.5, −12), left calcarine (3, −99, 9), and right precuneus (−1.5, −81, 37.5), respectively. The key regions involved were the bilateral insula, calcarine, cuneus, lingual, rectus, hippocampus, and putamen, as well as the left superior frontal cortex, orbitofrontal cortex, and inferior frontal cortex (p < 0.05, FWE corrected; Figure 3). The comparison between RTT and ASD controls also showed significantly decreased gray matter volumes in 4 clusters (Table 2). The number of voxels in the four clusters was 543, 15,031, 2,483, and 1,697, respectively. The peak MNI regions were left para-hippocampus with coordinate (−27, −15, −27), left middle frontal cortex (−33, 49.5, −12), right lingual (12, −82.5, −7.5), and right precuneus (−10.5, −63, 27), respectively. The key regions involved were similar to the RTT-TD comparison, including the bilateral insula, calcarine, cuneus, lingual, rectus, orbitofrontal cortex, hippocampus, and putamen, as well as the left superior frontal cortex (p < 0.05, FWE corrected; Figure 3). However, no significant difference was found between the ASD and TD group comparisons.
Table 2. Decreased gray matter volumes in comparisons between RTT group and controls for VBM analysis.
Figure 3. VBM and TBSS findings in young girls with RTT compared to ASD and TD controls. These figures demonstrated the ANCOVA and post-hoc statistical results of VBM analysis (left) and TBSS analysis (right) among three groups. For VBM results, the RTT group showed significantly decreased gray matter volumes (red-yellow) compared with controls (p < 0.05, FWE corrected). For TBSS results, TDD group showed significantly decreased FA values (red) and increased MD values (blue) compared with controls (p < 0.05, FWE corrected). TBSS results are overlaid on the mean FA skeleton (green).
At the global level, the RTT group showed significantly decreased FA and increased MD in comparison with ASD and TD controls (Table 1 and Figure 2).
TBSS analyses were conducted between RTT group (n = 22), ASD controls (n = 23), and TD controls (n = 23). The whole brain TBSS revealed global reductions of FA in ANCOVA. In the post-hoc analysis, the RTT group showed extensively decreased FA compared to TD controls mainly in the corpus callosum, superior longitudinal fasciculus, anterior corona radiate, and some bilateralized tracts (p = 0.002, voxel size > 100; Table 3 and Figure 3). The comparison between RTT and ASD controls also showed significantly decreased FA mainly in the similar regions of tracts as in the RTT-TD comparison. For MD, the RTT group showed increased MD values compared to TD controls mainly in the body and genu of the corpus callosum (p = 0.046, voxel size > 100). When compared with ASD controls, the RTT group showed increased MD values mainly in the body, genu, and splenium of the corpus callosum, cingulum, anterior, and posterior corona radiata (Table 3 and Figure 3). No significant difference was found between the ASD and TD group comparisons with either an FA or MD value.
Table 3. Decreased FA and increased MD values in comparisons between the RTT group and controls for TBSS analysis.
In the RTT group, correlation results showed that the developmental quotient of the GDS-C social domain was related to the gray matter volumes of the left hippocampus, calcarine, rectus, and Rolandic operculum, as well as the right precuneus and rectus after the multiple corrections (Table 4). The developmental quotient of the GDS-C motor domain was related to the general FA value of TBSS. Moreover, the RSSS clinical severity score was correlated with the gray matter volumes of right putamen and the general FA value of TBSS after the multiple corrections (Table 4). However, no significant correlations were found in the ASD group.
Table 4. Correlations between clinical data and altered gray and white matter parameters in the RTT group.
Our study demonstrated both gray and white matter morphometric features in the young RTT girls compared with age-paired ASD and TD controls. Pediatric-friendly pipelines were applied by generating study-specific tissue probability maps and templates during the preprocessing procedure. VBM analyses revealed decreased gray matter volumes mainly in the insula, frontal cortex, calcarine, and limbic/paralimbic regions. TBSS demonstrated decreased FA and increased MD mainly in the corpus callosum and other projection and association fibers such as superior longitudinal fasciculus and corona radiata. Furthermore, RTT social impairment and clinical severity were associated with these morphometric alterations. This study provides a unique opportunity to understand the underlying neuropathological mechanisms of RTT.
Since the first described as “progressive syndrome of autism” in 1983, RTT has been considered as one of the most severe subtypes of ASD for decades (Hagberg et al., 1983; Neul, 2012). However, it has been separated in the updated DSM-5 (Diagnostic and Statistical Manual of Mental Disorders, 5th edition) as an independent neurologic disorder (Battle, 2013). Clinicians and specialists claimed that there were essential differences between RTT and ASD such as sex preference, eye contact, and issues with movements (Neul, 2012). However, most of the evidence was based on behaviors. Interestingly, this study exhibited a significant difference between RTT and both ASD and TD groups, but no significant difference between ASD and TD groups in both gray and white matter comparisons. These results may suggest different neuropathological mechanisms underlying RTT and ASD, thus providing more robust evidence to support the classification of RTT as an independent neurologic disorder from ASD.
Unlike idiopathic ASD, which has not been identified with a specific gene or set of genes, RTT is more of a monogenic disorder caused by MECP2 gene variants (Neul et al., 2008, 2010). At the cellular level, the MECP2 gene has been tested to express widely throughout the mature neurons of the brain and plays a crucial role in synaptic plasticity, neuronal development, and differentiation (Chahrour et al., 2008; Lyst and Bird, 2015; Kong et al., 2022). Autopsy studies in patients with RTT revealed a reduction in brain weight and an overall decreased brain size (Armstrong et al., 1995; Bauman et al., 1995; Armstrong, 2005). Early MRI studies confirmed the findings from the autopsy that RTT patients had global brain atrophy (Reiss et al., 1993; Subramaniam et al., 1997). More recent MRI studies using voxel-based methods have provided evidence of selective alterations in related brain regions such as cerebellum, occipital cortex, and anterior frontal lobe for the gray matter, as well as the corpus callosum and fibers in the frontal area for the white matter (Carter et al., 2008; Mahmood et al., 2010; Shiohama et al., 2019). MRI studies on Mecp2-null(KO) mice showed overlapping brain areas with decreased volume, supporting the hypothesis of regional brain reduction in RTT (Saywell et al., 2006; Akaba et al., 2022). In accordance with the previous studies, our results also exhibited more regional reductions in gray matter volume and more regional alterations in white matter parameters in girls with RTT.
To further compare with the previous voxel-based MRI studies in RTT patients, some gray matter volume decreased in regions overlapping with our findings, such as the frontal cortex and occipital cortex. However, the top volume reduced regions in our results also involved bilateral insula, which has not been reported in previous voxel-based studies in RTT patients. The possible reason could be that the ages of our participants are much younger (mean age of 3.5 ± 1.25 years); however, the previous voxel-based studies are either with older participants (mean age of 8.6–8.9 years; Carter et al., 2008) or with large life span (4.4–17.6 years; Shiohama et al., 2019). RTT patients usually progress through 4 clinical stages: Stage I is the early onset stage, with an onset age of 6–18 months; Stage II is the rapid destructive stage, with an onset age of 1–4 years; Stage III is the pseudo-stationary stage, with an onset age of 4–8 years; and Stage IV is the late deterioration stage, usually after 8 years (Hagberg and Witt-Engerstrom, 1986; Neul et al., 2010). The participants in previous voxel-based studies were mostly at Stages III–IV. It is worthy to mention that gross motor dysfunction, gait apraxia, stabilization issue, and truncal ataxia were usually represented by Stage III (Hagberg and Witt-Engerstrom, 1986; Neul et al., 2010). Thus, it is understandable why the previous voxel-based studies reported the cerebellum as the main affected region, which plays an important role in motor control. However, the subjects in this study were mostly in Stage II, and the results showed significant correlations between the brain morphometric features and Griffith social domain instead of the gross motor or fine motor domain. Furthermore, one study with younger RTT girls (mean age of 6 ± 2 years) applying magnetic resonance spectroscopy revealed lower NAA concentration in the insular cortex (Horska et al., 2000), which is consistent with this study. The insula is anatomically folded deep within the lateral sulcus receiving projection fibers from the thalamus, amygdala, frontal, and occipital cortex (Uddin et al., 2017; Rachidi et al., 2021). As one of the least understood brain regions, recent evidence suggests that the insula may play a role in certain higher-level functions such as socio-emotional, cognitive, and sensorimotor integration (Uddin et al., 2017). The consistency of our results between studies with younger RTT girls and the inconsistency between studies with older subjects may suggest underlying mechanisms for early-stage RTT pathogenesis. Unfortunately, similar to most patient studies, the majority of the Mecp2 animal models are with adult mice, and the early stages of development have not been well-characterized. Thus, future longitudinal studies in both human and animal models covering early age stages may allow for a more in-depth understanding of disease pathogenesis and progression.
Evidence suggested that the FA and MD values were positively related to the maturation of myelin and axon (Pecheva et al., 2018). Previous diffusion-weighted MRI studies reported reduced FA in tracts such as the corpus callosum, cingulum, and external capsule (Mahmood et al., 2010; Oishi et al., 2013). However, these studies used ROI-orientated fiber tracking instead of whole brain evaluation. In the current study, we applied TBSS, which is a fully automated approach in a voxel-wise manner for whole-brain analysis beyond a priori–defined tracts (Papma et al., 2017). Tracts with altered FA/MD values in this study were not only consistent with previously reported fibers such as corpus callosum, cingulum, and external capsule but also included other projection and association fibers, which indicated more comprehensive white matter alterations. Our current findings with comprehensive white matter alterations might provide in vivo evidence for the findings from studies on cellular levels, which propose that the MECP2 gene is expressed in nearly all the neurons and glia in the brain (Chahrour and Zoghbi, 2007; Qiu, 2018).
As a non-invasive imaging tool to obtain both structural and functional information about the in vivo brain, MRI has been widely introduced to identify specific pathological changes in the brain for neurodevelopmental disorders (Eliez and Reiss, 2000). Processing pediatric neuroimaging data is always a great challenge due to pervasive morphological changes during brain development. Considering the myelination maturation phase and brain tissue imaging contrast for MRI scans from children older than 2 years, we recommend processing study- or child-specific tissue probability maps or templates as an alternate to the standard adult templates which are embedded in the classical pipelines. To create the study-specific template for TBSS registration, we suggest simply changing the command line option to identify the “most representative” one as the target image instead of the adult FMRIB58_FA template image (https://fsl.fmrib.ox.ac.uk/fsl/fslwiki/TBSS). To create the study- or child-specific tissue probability maps for VBM preprocessing, we suggest the Template-O-Matic toolbox, which uses a large, healthy, reference database (n = 404) of children to generate matched templates for each subject based on age and gender (Wilke et al., 2008). Besides, this toolbox is quite user-friendly since the algorithm can be simply implemented in the form of the SPM toolbox. This procedure yields high-quality tissue maps to better match the individual input sample, which is a significant improvement over classical approaches, especially regarding spatial normalization and tissue segmentation processes (Wilke et al., 2008; Kozlowska et al., 2017).
There were some limitations to be considered. First, the current MRI scans were mainly structural, so future studies of functional connectivity may provide more in-depth evidence for our conclusions. Second, this study is cross-sectional. However, RTT is a postnatal neurodevelopmental disorder with symptoms getting worse with age. Thus, future longitudinal studies in both human and animal models may allow a better understanding of disease pathogenesis and progression.
This study applied VBM for voxel-wised volumetric characteristics of gray matter and TBSS for white matter analysis in young girls with RTT using pediatric-adjusted analytic pipelines. Decreased gray matter in the insula, frontal cortex, and limbic/paralimbic regions as well as altered FA and MD values in the corpus callosum and other projection and association fibers provided some new guidance to further understand the underlying mechanisms in RTT.
The original contributions presented in the study are included in the article/supplementary material, further inquiries can be directed to the corresponding author/s.
The studies involving human participants were reviewed and approved by the Ethics Committee of Children's Hospital of Fudan University. Written informed consent to participate in this study was provided by the participants' legal guardian/next of kin.
DL and LM analyzed and interpreted data and wrote and reviewed the manuscript. QX designed the research, analyzed and interpreted the data, and reviewed the manuscript. XX interpreted the data and reviewed the manuscript. ZQ interpreted the data and reviewed the manuscript. HL and CH collected the clinical data. BZ and KZ prepared the data and reviewed the manuscript. All authors contributed to the article and approved the submitted version.
This study was supported in part by the National Natural Science Foundation of China (NSFC, No. 81701129, 82171540) and the Key Subject Construction Project of Shanghai Municipal Health Commission (No. shslczdzk02903).
The authors declare that the study was conducted in the absence of any commercial or financial relationships that could be construed as a potential conflict of interest.
The reviewer YY declared a shared parent affiliation with the authors to the handling editor at the time of review.
All claims expressed in this article are solely those of the authors and do not necessarily represent those of their affiliated organizations, or those of the publisher, the editors and the reviewers. Any product that may be evaluated in this article, or claim that may be made by its manufacturer, is not guaranteed or endorsed by the publisher.
Akaba, Y., Shiohama, T., Komaki, Y., Seki, F., Ortug, A., Sawada, D., et al. (2022). Comprehensive volumetric analysis of Mecp2-null mouse model for rett syndrome by T2-weighted 3D magnetic resonance imaging. Front. Neurosci. 16:885335. doi: 10.3389/fnins.2022.885335
Amir, R. E., Van den Veyver, I. B., Wan, M., Tran, C. Q., Francke, U., and Zoghbi, H. Y. (1999). Rett syndrome is caused by mutations in X-linked MECP2, encoding methyl-CpG-binding protein 2. Nat. Genet. 23, 185–188. doi: 10.1038/13810
Armstrong, D., Dunn, J. K., Antalffy, B., and Trivedi, R. (1995). Selective dendritic alterations in the cortex of Rett syndrome. J. Neuropathol. Exp. Neurol. 54, 195–201. doi: 10.1097/00005072-199503000-00006
Armstrong, D. D. (2005). Neuropathology of Rett syndrome. J. Child Neurol. 20, 747–753. doi: 10.1177/08830738050200082401
Ashburner, J., and Friston, K. J. (2005). Unified segmentation. Neuroimage 26, 839–851. doi: 10.1016/j.neuroimage.2005.02.018
Battle, D. E. (2013). Diagnostic and statistical manual of mental disorders (DSM). Codas 25, 191–192. doi: 10.1590/s2317-17822013000200017
Bauman, M. L., Kemper, T. L., and Arin, D. M. (1995). Microscopic observations of the brain in Rett syndrome. Neuropediatrics 26, 105–108. doi: 10.1055/s-2007-979737
Carter, J. C., Lanham, D. C., Pham, D., Bibat, G., Naidu, S., and Kaufmann, W. E. (2008). Selective cerebral volume reduction in Rett syndrome: a multiple-approach MR imaging study. Am. J. Neuroradiol. 29, 436–441. doi: 10.3174/ajnr.A0857
Casanova, M. F., Naidu, S., Goldberg, T. E., Moser, H. W., Khoromi, S., Kumar, A., et al. (1991). Quantitative magnetic resonance imaging in Rett syndrome. J. Neuropsychiatry Clin. Neurosci. 3, 66–72. doi: 10.1176/jnp.3.1.66
Chahrour, M., Jung, S. Y., Shaw, C., Zhou, X., Wong, S. T., Qin, J., et al. (2008). MeCP2, a key contributor to neurological disease, activates and represses transcription. Science 320, 1224–1229. doi: 10.1126/science.1153252
Chahrour, M., and Zoghbi, H. Y. (2007). The story of Rett syndrome: from clinic to neurobiology. Neuron 56, 422–437. doi: 10.1016/j.neuron.2007.10.001
Eliez, S., and Reiss, A. L. (2000). MRI neuroimaging of childhood psychiatric disorders: a selective review. J. Child Psychol. Psychiatry 41, 679–694. doi: 10.1111/1469-7610.00656
Gaser, C., and Dahnke, R. (2016). “CAT-A computational anatomy toolbox for the analysis of structural MRI data,” in 22nd Annual Meeting of the Organization For Human Brain Mapping. Available online at: https://www.biorxiv.org/content/10.1101/2022.06.11.495736v1.full.pdf
Gotham, K., Risi, S., Pickles, A., and Lord, C. (2007). The autism diagnostic observation schedule: revised algorithms for improved diagnostic validity. J. Autism Dev. Disord. 37, 613–627. doi: 10.1007/s10803-006-0280-1
Gunbey, H. P., Bilgici, M. C., Aslan, K., Has, A. C., Ogur, M. G., Alhan, A., et al. (2017). Structural brain alterations of Down's syndrome in early childhood evaluation by DTI and volumetric analyses. Eur. Radiol. 27, 3013–3021. doi: 10.1007/s00330-016-4626-6
Hagberg, B., Aicardi, J., Dias, K., and Ramos, O. (1983). A progressive syndrome of autism, dementia, ataxia, and loss of purposeful hand use in girls: Rett's syndrome: report of 35 cases. Ann. Neurol. 14, 471–479. doi: 10.1002/ana.410140412
Hagberg, B., and Witt-Engerstrom, I. (1986). Rett syndrome: a suggested staging system for describing impairment profile with increasing age towards adolescence. Am. J. Med. Genet. Suppl. 1, 47–59. doi: 10.1002/ajmg.1320250506
Hoffbuhr, K., Devaney, J. M., LaFleur, B., Sirianni, N., Scacheri, C., Giron, J., et al. (2001). MeCP2 mutations in children with and without the phenotype of Rett syndrome. Neurology 56, 1486–1495. doi: 10.1212/WNL.56.11.1486
Horska, A., Naidu, S., Herskovits, E. H., Wang, P. Y., Kaufmann, W. E., and Barker, P. B. (2000). Quantitative 1H MR spectroscopic imaging in early Rett syndrome. Neurology 54, 715–722. doi: 10.1212/WNL.54.3.715
Ip, J. P. K., Mellios, N., and Sur, M. (2018). Rett syndrome: insights into genetic, molecular and circuit mechanisms. Nat. Rev. Neurosci. 19, 368–382. doi: 10.1038/s41583-018-0006-3
Jenkinson, M., Beckmann, C. F., Behrens, T. E., Woolrich, M. W., and Smith, S. M. (2012). Fsl. Neuroimage 62, 782–790. doi: 10.1016/j.neuroimage.2011.09.015
Katz, D. M., Bird, A., Coenraads, M., Gray, S. J., Menon, D. U., Philpot, B. D., et al. (2016). Rett syndrome: crossing the threshold to clinical translation. Trends Neurosci. 39, 100–113. doi: 10.1016/j.tins.2015.12.008
Kaufmann, W. E., Tierney, E., Rohde, C. A., Suarez-Pedraza, M. C., Clarke, M. A., Salorio, C. F., et al. (2012). Social impairments in Rett syndrome: characteristics and relationship with clinical severity. J. Intellect. Disabil. Res. 56, 233–247. doi: 10.1111/j.1365-2788.2011.01404.x
Kong, Y., Li, Q. B., Yuan, Z. H., Jiang, X. F., Zhang, G. Q., Cheng, N., et al. (2022). Multimodal neuroimaging in Rett syndrome with MECP2 mutation. Front. Neurol. 13:838206. doi: 10.3389/fneur.2022.838206
Kozlowska, K., Griffiths, K. R., Foster, S. L., Linton, J., Williams, L. M., and Korgaonkar, M. S. (2017). Grey matter abnormalities in children and adolescents with functional neurological symptom disorder. Neuroimage Clin. 15, 306–314. doi: 10.1016/j.nicl.2017.04.028
Luiz, D. M., Foxcroft, C. D., and Tukulu, A. N. (2004). The denver II scales and the griffiths scales of mental development: a correlational study. J. Child Adolesc. Ment. Health 16, 77–81. doi: 10.2989/17280580409486573
Lyst, M. J., and Bird, A. (2015). Rett syndrome: a complex disorder with simple roots. Nat. Rev. Genet. 16, 261–275. doi: 10.1038/nrg3897
Mahmood, A., Bibat, G., Zhan, A. L., Izbudak, I., Farage, L., Horska, A., et al. (2010). White matter impairment in Rett syndrome: diffusion tensor imaging study with clinical correlations. Am. J. Neuroradiol. 31, 295–299. doi: 10.3174/ajnr.A1792
Neul, J. L. (2012). The relationship of Rett syndrome and MECP2 disorders to autism. Dialogues Clin. Neurosci. 14, 253–262. doi: 10.31887/DCNS.2012.14.3/jneul
Neul, J. L., Fang, P., Barrish, J., Lane, J., Caeg, E. B., Smith, E. O., et al. (2008). Specific mutations in methyl-CpG-binding protein 2 confer different severity in Rett syndrome. Neurology 70, 1313–1321. doi: 10.1212/01.wnl.0000291011.54508.aa
Neul, J. L., Kaufmann, W. E., Glaze, D. G., Christodoulou, J., Clarke, A. J., Bahi-Buisson, N., et al. (2010). Rett syndrome: revised diagnostic criteria and nomenclature. Ann. Neurol. 68, 944–950. doi: 10.1002/ana.22124
Oishi, K., Faria, A. V., Yoshida, S., Chang, L., and Mori, S. (2013). Quantitative evaluation of brain development using anatomical MRI and diffusion tensor imaging. Int. J. Dev. Neurosci. 31, 512–524. doi: 10.1016/j.ijdevneu.2013.06.004
Papma, J. M., Jiskoot, L. C., Panman, J. L., Dopper, E. G., den Heijer, T., Donker Kaat, L., et al. (2017). Cognition and gray and white matter characteristics of presymptomatic C9orf72 repeat expansion. Neurology 89, 1256–1264. doi: 10.1212/WNL.0000000000004393
Pecheva, D., Kelly, C., Kimpton, J., Bonthrone, A., Batalle, D., Zhang, H., et al. (2018). Recent advances in diffusion neuroimaging: applications in the developing preterm brain. F1000Res 7:F1000. doi: 10.12688/f1000research.15073.1
Qiu, Z. (2018). Deciphering MECP2-associated disorders: disrupted circuits and the hope for repair. Curr. Opin. Neurobiol. 48, 30–36. doi: 10.1016/j.conb.2017.09.004
Rachidi, I., Minotti, L., Martin, G., Hoffmann, D., Bastin, J., David, O., et al. (2021). The insula: a stimulating island of the brain. Brain Sci. 11:1533. doi: 10.3390/brainsci11111533
Reiss, A. L., Faruque, F., Naidu, S., Abrams, M., Beaty, T., Bryan, R. N., et al. (1993). Neuroanatomy of Rett syndrome: a volumetric imaging study. Ann. Neurol. 34, 227–234. doi: 10.1002/ana.410340220
Saywell, V., Viola, A., Confort-Gouny, S., Le Fur, Y., Villard, L., and Cozzone, P. J. (2006). Brain magnetic resonance study of Mecp2 deletion effects on anatomy and metabolism. Biochem. Biophys. Res. Commun. 340, 776–783. doi: 10.1016/j.bbrc.2005.12.080
Shiohama, T., Levman, J., and Takahashi, E. (2019). Surface- and voxel-based brain morphologic study in Rett and Rett-like syndrome with MECP2 mutation. Int. J. Dev. Neurosci. 73, 83–88. doi: 10.1016/j.ijdevneu.2019.01.005
Smith, S. M., Johansen-Berg, H., Jenkinson, M., Rueckert, D., Nichols, T. E., Miller, K. L., et al. (2007). Acquisition and voxelwise analysis of multi-subject diffusion data with tract-based spatial statistics. Nat. Protoc. 2, 499–503. doi: 10.1038/nprot.2007.45
Subramaniam, B., Naidu, S., and Reiss, A. L. (1997). Neuroanatomy in Rett syndrome: cerebral cortex and posterior fossa. Neurology 48, 399–407. doi: 10.1212/WNL.48.2.399
Tso, W. W. Y., Wong, V. C. N., Xia, X., Faragher, B., Li, M., Xu, X., et al. (2018). The Griffiths Development Scales-Chinese (GDS-C): a cross-cultural comparison of developmental trajectories between Chinese and British children. Child Care Health Dev. 44, 378–383. doi: 10.1111/cch.12548
Uddin, L. Q., Nomi, J. S., Hebert-Seropian, B., Ghaziri, J., and Boucher, O. (2017). Structure and function of the human insula. J. Clin. Neurophysiol. 34, 300–306. doi: 10.1097/WNP.0000000000000377
Keywords: voxel-based morphometry, tract-based spatial statistics, Rett, MECP2, children
Citation: Li D, Mei L, Li H, Hu C, Zhou B, Zhang K, Qiao Z, Xu X and Xu Q (2022) Brain structural alterations in young girls with Rett syndrome: A voxel-based morphometry and tract-based spatial statistics study. Front. Neuroinform. 16:962197. doi: 10.3389/fninf.2022.962197
Received: 06 June 2022; Accepted: 15 August 2022;
Published: 08 September 2022.
Edited by:
Jingwen Yan, Indiana University, Purdue University Indianapolis, United StatesReviewed by:
Ye Yao, Fudan University, ChinaCopyright © 2022 Li, Mei, Li, Hu, Zhou, Zhang, Qiao, Xu and Xu. This is an open-access article distributed under the terms of the Creative Commons Attribution License (CC BY). The use, distribution or reproduction in other forums is permitted, provided the original author(s) and the copyright owner(s) are credited and that the original publication in this journal is cited, in accordance with accepted academic practice. No use, distribution or reproduction is permitted which does not comply with these terms.
*Correspondence: Qiong Xu, eHVxaW9uZ0BmdWRhbi5lZHUuY24=; Xiu Xu, eHV4aXVAc2htdS5lZHUuY24=; Zhongwei Qiao, cWlhb3pod2VpQDE2My5jb20=
†These authors have contributed equally to this work
Disclaimer: All claims expressed in this article are solely those of the authors and do not necessarily represent those of their affiliated organizations, or those of the publisher, the editors and the reviewers. Any product that may be evaluated in this article or claim that may be made by its manufacturer is not guaranteed or endorsed by the publisher.
Research integrity at Frontiers
Learn more about the work of our research integrity team to safeguard the quality of each article we publish.