- 1Department of Radiology, Affiliated Hospital of Nanjing University of Chinese Medicine, Nanjing, China
- 2College of Intelligence and Computing, Tianjin Key Laboratory of Cognitive Computing and Application, Tianjin University, Tianjin, China
- 3National Laboratory of Pattern Recognition, Institute of Automation, Chinese Academy of Sciences, Beijing, China
- 4Brainnetome Center, Institute of Automation, Chinese Academy of Sciences, Beijing, China
- 5Department of Magnetoencephalography, Nanjing Brain Hospital, Affiliated to Nanjing Medical University, Nanjing, China
Temporal lobe epilepsy (TLE) is a chronic neurological disorder that is divided into two subtypes, complex partial seizures (CPS) and simple partial seizures (SPS), based on clinical phenotypes. Revealing differences among the functional networks of different types of TLE can lead to a better understanding of the symbology of epilepsy. Whereas Although most studies had focused on differences between epileptic patients and healthy controls, the neural mechanisms behind the differences in clinical representations of CPS and SPS were unclear. In the context of the era of precision, medicine makes precise classification of CPS and SPS, which is crucial. To address the above issues, we aimed to investigate the functional network differences between CPS and SPS by constructing support vector machine (SVM) models. They mainly include magnetoencephalography (MEG) data acquisition and processing, construction of functional connectivity matrix of the brain network, and the use of SVM to identify differences in the resting state functional connectivity (RSFC). The obtained results showed that classification was effective and accuracy could be up to 82.69% (training) and 81.37% (test). The differences in functional connectivity between CPS and SPS were smaller in temporal and insula. The differences between the two groups were concentrated in the parietal, occipital, frontal, and limbic systems. Loss of consciousness and behavioral disturbances in patients with CPS might be caused by abnormal functional connectivity in extratemporal regions produced by post-epileptic discharges. This study not only contributed to the understanding of the cognitive-behavioral comorbidity of epilepsy but also improved the accuracy of epilepsy classification.
Introduction
According to the World Health Organization, about 50 million people worldwide suffered from epilepsy (Fiest et al., 2017). In recent years, epilepsy has been proposed to be a disorder of the brain network caused by hypersynchrony of neuronal activity (Zhang et al., 2011; Richardson, 2012). Epilepsy could be divided into focal, generalized onset, and unknown onset based on clinical manifestations and electroencephalogram (EEG) in line with the new International League Against Epilepsy ILAE criteria in 2017 (Scheffer et al., 2017). Depending on whether awareness was impaired, focal seizures were classified as aware or impaired awareness seizures, which are also known as “simple partial seizure (SPS)” and “complex partial seizure (CPS),” respectively (Falco-Walter et al., 2018).
Temporal lobe epilepsy (TLE), a common neurological disorder originates in the temporal lobe (de Lanerolle et al., 1989). Seizures types in TLE primarily incorporated SPS, which can cause focal motor or selective emotional or visual changes with relatively preserved consciousness, and CPS, which has more sophisticated clinical manifestations, including epigastric paresthesia, cognitive impairment, paresthesia, and automatisms (Proposal for Revised Clinical and Electroencephalographic Classification of Epileptic Proposal for Revised Clinical Electroencephalographic Classification of Epileptic Seizures., 1981; Depaulis et al., 1997). The EEG is mostly normal in SPS, and abnormal EEG changes were transient and were generally restricted peaks or paroxysmal activity in the temporal lobe regions (Inoue et al., 2000; Janszky et al., 2004).
During the past few decades, increasing evidence have linked epileptic cognitive impairment and loss of consciousness to diffuse brain network changes (Blume, 2002; Blumenfeld et al., 2009; Xu et al., 2009; Englot et al., 2010; González et al., 2019; Hermann et al., 2021). The duration of CPS invariably exceeded 30 s, and the discharge position was deeper. Meanwhile, CPS tended to spread to the brainstem or contralateral hemisphere, resulting in extensive neurological alterations (Stayman and Abou-Khalil, 2011; Hauf et al., 2013). “Network inhibition hypothesis” was a new theory of CPS proposed by Blumenfeld (Blumenfeld et al., 2004, 2009; Guye et al., 2006), was According to the theory, focal discharges in the temporal lobe interfered with brainstem–diencephalon arousal system, and then inhibited ascending reticular activation system, which indirectly brought forth impaired cortical function and loss of consciousness (Steriade, 1970; Motelow et al., 2015). Pathophysiological studies had shown a significant increase in slow-wave activity in the frontoparietal neocortex and an enhanced rate of diffusion of fast EEG activity from the medial temporal lobe to the contralateral side during CPS compared to SPS (Englot et al., 2017). In animal studies, it was also found that blood oxygenation level-dependent in the hippocampus of TLE increased, while it decreased in the cortex and thalamus (Motelow et al., 2015). This suggested that cortical and subcortical structures are involved in regulating consciousness. Studying alterations in resting-state brain functional connectivity could be conducive to explore the mechanisms underlying cognitive dysfunction in individuals with epilepsy. Memory deficiencies in individuals with CPS were associated with compensatory increases in the hippocampus. There was also evidence that executive dysfunction was relevant to a reduced resting-state functional connectivity in the frontoparietal lobe (Park et al., 2017; Ives-Deliperi and Butler, 2021; Li et al., 2022). Language dysfunction was associated with reduced functional connectivity in the frontotemporal lobe language network. EEG researches have revealed that even if the bilateral pikes in patients with SPS and CPS originated in disparate brain regions, the discharges spread to the same area, the temporal lobe base (Sirven et al., 1996). This indicated that SPS and CPS may have shared network nodes in the temporal lobe. However, the mechanisms by which CPS brain network alterations are associated with impaired consciousness and behavioral abnormalities have not been systematically studied.
Unlike other diseases, the first symptom defined the epilepsy type, consciousness turned into a “watershed,” differentiated SPS from CPS. Nevertheless, a flat dichotomy may result in neglecting the other clinical symptoms (Muayqil et al., 2018). Currently, there is a lack of direct studies on brain network alters among SPS and CPS individuals. Therefore, our study hypothesized that aberrant differences in functional connectivity of CPS and SPS were vital nodes involved in the regulation of brain network consciousness and behaviors. Furthermore, seizures were primarily self-reported by the patient, and even with EEG testing, the underreporting rate was still as high as 50% (Glauser et al., 2010; Elger and Hoppe, 2018; Verdru and Van Paesschen, 2020). Especially, when epilepsy originates in deep or non-dominant regions, the initial weak signal may not be obtained on scalp EEG (Benbadis et al., 2020). Compared to EEG, the sensitivity and specificity of magnetoencephalography (MEG) for localization of minute EEG activity were higher than that of EEG (van Mierlo et al., 2014). In contrast to MRI, even though functional brain networks were widely used in MRI (Salma et al., 2019; McKavanagh et al., 2021), the time span for information processing was only milliseconds to seconds when the brain was in a resting state. Therefore, the selection of MEG with higher localization accuracy for the analysis of functional connectivity differences was more promising for detecting subtle changes in the brain (Kakisaka et al., 2013; Nissen et al., 2018; van Klink et al., 2019).
Prior to this study, we preprocessed MEG data from patients with SPS and CPS and normal controls, and performed a source level analysis. The results of the coherence analysis showed that the functional connectivity of patients with both SPS and CPS was lower than that of normal individuals in the whole brain, while there was no significant difference between the two groups. Therefore, to address the problems of low efficiency and easy misdiagnosis in manual identification of EEG signals, we chose machine learning to further differentiate the differences between patients with SPS and CPS (Fallahi et al., 2021). At present, machine learning has shown sound application prospects for various neurological diseases incorporating epilepsy (Craley et al., 2020; Gleichgerrcht et al., 2020; Pavel et al., 2020). (Li et al., 2018; Bharath et al., 2019). In a previous study, SVM was able to distinguish temporal lobe epilepsy from benign epilepsy in healthy controls or central temporal spikes (Jin and Chung, 2017; Sriraam and Raghu, 2017; Yang et al., 2020). In addition, the use of SVM could also distinguish between resected and unresected regions based on preoperative interictal MEG data in epileptic patients. Therefore, the present study intends to use SVM combined with two sample selection approaches to explore the differences in functional connectivity between the two groups at rest.
Materials and methods
Patient population
This study was part of a research program on neuroimaging in epilepsy. It consecutively recruited 40 patients with TLE between 2015 and 2020. Inclusion criteria were as follows: 1. in line with the International League Against Epilepsy (ILAE, 1989) diagnostic criteria for epilepsy; 2. partial epilepsy diagnosed by V-EEG observation and medical history; 3. the clinical and EEG characteristics were onefold SPS or CPS; 4. individuals had no visible lesions in structural MRI images.; 5. able to cooperate with the inspection and the head movement during MEG examination was no more than 5 mm. Exclusion criteria: 1. combined with a history of generalized seizure ; 2. suffered from other types of paroxysmal illness (e.g., mental illness, severe systemic illness, etc.); 3. implants that may seriously interfere with MEG and MRI data collection (such as dentures, cochlear implants, pacemakers, etc.); 4. unable to cooperate with MEG and MRI examination. Of the 40 patients we recruited, All of them underwent MEG and MRI scans at Nanjing Brain Hospital affiliated with Nanjing Medical University. All individuals have read and signed voluntary and written informed consent for the study prior to enrollment, according to the standards set by the ethical committee of Nanjing Brain Hospital of Nanjing Medical University, which approved this study.
MEG resting state acquisition
All studies were performed in a magnetically shielded room by using our 275-channel whole-head biomagnetometer (VSM MedTech, Coquitlam, BC, Canada). The full head sensor of the 275 super-conducting quantum interference device (SQUID) was used to measure the brain magnetic field in the direction perpendicular to the scalp. Three electrically active coils were placed as fiducial markers at the nasion and 1 cm anterior to the left and right tragus to measure the position of each person's head relative to the MEG sensor.
All individuals did not have seizures at least 16 h before the examination. If the frequency of seizures was less than once a week, individuals were required to stop anti-epileptic drugs for 2 days and overnight sleep deprivation (increased seizure activity). MEG recording is performed at a sample rate of 1,200 Hz for twenty 120-s recordings with the patient in the state of rest and their eyes closed to detect interictal MEG sharp waves and spikes, as well as bursts of rhythmic activity.
Anatomical MRI
MRI data were collected using a US GE Signa NV/i 1.5 T super-conducting magnetic resonance apparatus, and the head of the subject was fixed with a sponge pad. The routine anatomical MRI data were acquired to detect structural details. T1-weighted image scans were obtained, with following parameters: TR/TE = 1,750 ms/24 ms, FA = 90°, matrix = 256 × 256, FOV = 24 × 24 mm2, slice thickness = 6 mm, slice gap = 2 mm, and acquired slices = 16. Coronal T2-FLAIR-weighted image scans were also obtained, with following parameters: TR/TE = 8,400 ms/135 ms, FA = 150°, matrix = 256 × 256, FOV = 24 × 24 mm2, slice thickness = 16 mm, slice gap = 2 mm and acquired slices = 16.
Data preparation
All MEG recordings were reviewed by two experienced epileptologists at Nanjing Medical University, and the peaks of all epileptic spikes and inter epileptic discharge were marked manually based on the MEG recordings.
Artifact rejection and subtraction of inter-spikes relative-stable activity
All analyses were done with custom-written MATLAB (Mathworks, Natick, MA) scripts and FieldTrip (http://www.ru.nl/fcdonders/fieldtrip/). Firstly, all spikes and abnormal discharge before and after the 10 s time period were excluded. Then we short-cut the remaining segments with a non-overlapped length of 10 s and further extracted the sub-segments which are free from jump-like artifacts and muscular artifacts. To ensure the same length of the remaining sub-segments, we further removed the ones with a length <4 s and short-cut the others with a length of 4 s. The number of segments per subject varied from 33 to 169 at last. The total number of segments for all the individuals with CPS was 1,306, and the total number of segments for all the individuals with SPS was 1,526.
Filtering and removing artifact
The remaining signals were low-pass-filtered at 70 Hz and high-pass-filtered at 1 Hz with notch-filtered around 50 Hz (the vertical refresh rate of the LCD projector). Furthermore, to increase the speed of the following data analysis, the data were down-sampled to 100 Hz. In addition, we removed blink artifacts and ECG noise from data by using Independent Component Analysis (ICA).
Source reconstruction
We performed source reconstruction after preprocessing using a partial cannonical correlation/coherence (PCC) (Mukuta and Harada, 2014). Specifically, first, the subject-specific T1-weighted MR images were re-sliced and segmented to obtain brain/skull boundary by using FieldTrip. Then, we generated the individual cortical meshes with > 1,00,000 vertices per hemisphere by using the Freesurfer package (version 5.3.0) (surfer.nmr.mgh.harvard.edu), and we downsampled them to 8,196 nodes in all by using MNE Suite (martinos.org/mne/stable/index.html). Next, the downsampled cortical sheet was coregistered to the sensor-based coordinate system with FieldTrip. Finally, the volume conduction for the forward model was computed by using FieldTrip's “single shell” method, and the source reconstruction was computed between 1 and 40 Hz with a partial canonical correlation/coherence (PCC) method.
Functional connectivity analysis
For each segment time course, we calculated functional connectivity (FC) by the imaginary part of the coherency index between the time courses at every two sheets after source reconstruction. To reduce the dimensionality of the FC matrix, we applied a parcellation scheme according to the Destrieux Atlas, which consists of 74 parcels in the whole cortical surface (Destrieux et al., 2010). Therefore, each FC matrix was merged to a size of 74-by-74.
Classification of FC matrix between CPS and SPS
In the next step, we tried to find the functional connectivity pairs that were different between CPS and SPS groups by using a nonlinear classification method with feature extraction and leave-one-out loop. The features which contributed well and were stable for separating the two patient groups were considered to indicate the corresponding cortical pairs having obvious distinct functional connectivity between them. The operational flowchart of the proposed framework is shown in Figure 1.
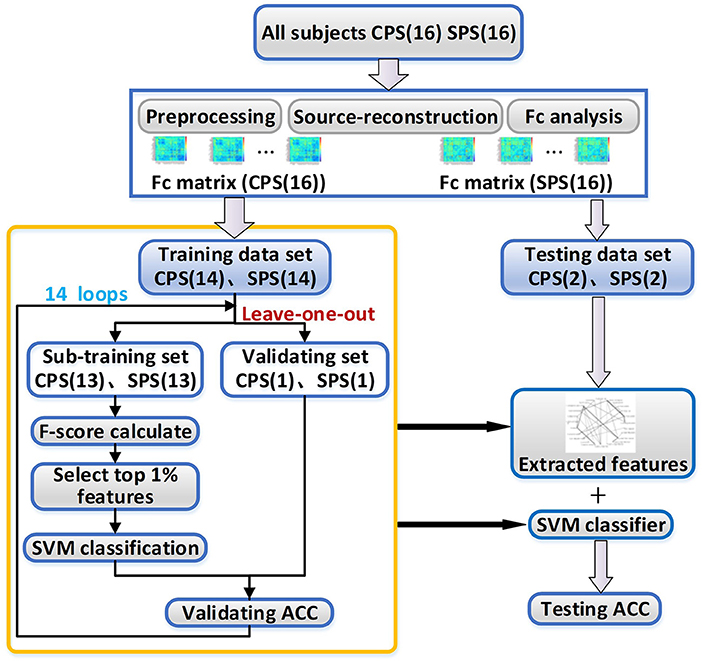
Figure 1. The working flowchart of the proposed framework. CPS, complex partial seizures; SPS, simple partial seizures; SVM, support vector machine; ACC, the accuracy rate.
Specifically, as shown in Table 1, we divided all the subjects into 16 subsets, and each subset had one patient with CPS and one with SPS. For each subset, the number of CPS FC matrices was basically equal to the number of SPS FC matrices. We randomly selected two subsets as the testing set, and the others were considered as a training set for classification.
To improve the generalization ability of the classification model as possible as we can, we adopted a leave-one-out method for the training processing in this work. For each loop, we selected 13 subsets from the training set as sub-training set and left one subset as validating set to select the best hyper-parameters for the classification model. For the sub-training set, we selected the FC features, which have stable and great contribution for separating the CPS and SPS, as the methods introduced in “Feature extraction” section. Then, we trained SVM classifier as introduction in “Support vector machine classifier”. And then, we repeated this process 14 times for all possible selection options.
After leave-one-out training, we counted the overlap rate of all appeared features among 14 times leave-one-out training loop and extracted the features with the highest (≥12/14) overlap rate as a reliable feature assemblage. Finally, we extracted the corresponding feature values in the testing dataset as the input for the classifier and performed the classification as we trained. The test accuracy rate here was calculated. The method for feature extraction and classification were introduced below in detail.
Feature extraction
To reduce the duplicate information in the FC matrix which was symmetrical, we extracted its lower triangular part and its main diagonal as the vector. Each value in the vector is a feature for classification, indicating an FC strength between a pair of cortical areas. The total number of features contained in each vector is C2,775. It is important to select the most essential features using feature selection before entering the classifier (Guyon and Elissee, 2003) when the number of features is much more than the number of subjects used in classification.
To more effectively enhance the efficiency of computation and avoid the high-dimensional and small-sample-size problem for classification, we further measured the classification capability of each feature by the F-score method (Chen et al., 2006) and removed the features which were irrelevant or has low correlation to the classification (Guyon and Elissee, 2003). F-score is a simple and generally quite an effective technique that can measure the ability of a feature to discriminate between two classes of samples. Given a set of training samples xk, k = 1, ⋯, m, then the F-score of the ith feature is defined as follows:
where is the average of the ith feature in the whole data set, whereas , are the average of the ith feature in CPS, and SPS data sets, respectively; n+ and n− represent the number of samples in CPS and SPS data sets, respectively; and and indicate the ith feature of the kth sample in the two groups' data sets, respectively. F-score is positive, and the larger the F-score of a feature is the stronger its ability to distinguish two categories of samples.
As a pretest, we calculated the F-score of 2,775 features on the whole training set first and arranged them in descending order. As shown in Figure 2, it can be found that the F-score curve of 2,775 features declines rapidly and there are a large number of feature F-scores close to zero, suggesting only a very small number of features have a good contribution to classification while the others are redundant. Therefore, in the following classification processing, we extracted the features with the top 1% F–scores from the training subset as the input for classification.
Support vector machine classifier
The SVM is one type of binary supervised learning classifier that has been widely used in recent years (Ines et al., 2013) with a high ability for generalization (Vapnik, 2000). Rajpoot et al. (2015) used AP clustering combined with SVM to study functional connectivity changes in individuals with epilepsy and normal subjects. Its basic model is a linear classifier. For linearly separable data, SVM generates a separating hyperplane which separates the data with the largest margin. For linearly inseparable data, SVM can efficiently perform a non-linear one using kernel function Φ(x) (2), mapping their inputs into high-dimensional feature spaces.
By choosing a suitable Φ(x), the SVM constructs an optimal separating hyperplane in higher-dimensional feature space to solve the linear-inseparable problem (Cantor-Rivera et al., 2015). The kernel function mentioned above may be any of the symmetric functions that satisfies the Mercel conditions (Dhanalakshmi et al., 2009). We selected the Radial Basis Function (RBF) in this work since it was the most frequently used kernel function, and there were two parameters C and γ, which must be preset before training the SVM classifier.
In the experiment, we implemented the scikit-learn, a machine learning tool package in python, to train the SVM classifier with parameter C of 1.2 and parameter γ of 5, and each sample was converted to scikit-learn input data format in this work.
Results
Five patients had obvious head movement during scanning, defined as more than a 2 mm translation. Two patients refused to participate in further research and asked to withdraw. This meant that 32 patients met the stringent inclusion criteria and were finally included in the study. Among them, 16 patients (five male, 29.1 ± 8.63 years) were suffering from CPS and 16 patients (11 male, 23.5 ± 5.66 years) from SPS. The detailed demographic and electroclinical data are summarized in Table 2.
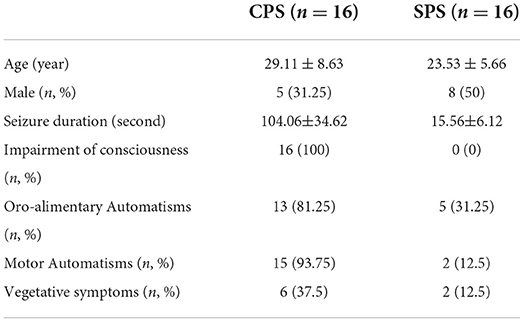
Table 2. Ictal semiology in the three groups of patients,the number of patients in each group having the concerning symptoms.
We calculated the classification accuracies from each validating subset in the leave-one-out loop to evaluate the effectiveness of our feature extraction method and SVM classifier. The results were summarized in Table 3. For all the 14 times of training, the validation accuracies varied from 72.39 to 88.73%, of which the mean is 79.87%.
After leave-one-out training, we got 28 FC features whose overlap rate was ≥12/14 among 14 times training. Figure 3 and Table 4 demonstrated the nodes and edges involved in these 28 FC features. According to the mean F-score value of each feature among 14 times training, we marked the FCs with great mean F-scores (higher than 75% of the highest mean F-score value among all the features) as a red line and colored the others in blue. They were mainly located within and between the parietal, the occipital, the frontal lobe, and the limbic system. For the temporal lobe and the insula, there was less different functional connectivity between SPS and CPS individuals. Specifically, as shown in Figure 3 and Table 4, the FCs with great mean F-scores were located between the middle frontal gyrus (G_front_middle), and the angular gyrus (G_pariet_inf-Angular), and Sulcus intermedius primus (of Jensen) (S_interm_prim-Jensen); between the Cuneus (G_cuneus) and the parieto-occipital sulcus (S_parieto_occipital), and the intraparietal sulcus (S_intrapariet_and_P_trans); and between the intraparietal sulcus (S_intrapariet_and_P_trans) and the posterior-ventral part of the cingulate gyrus (vPCC, G_cingul-Post-ventral); and between the posterior-ventral part of the cingulate gyrus (vPCC, G_cingul-Post-ventral) and the middle-posterior part of the cingulate gyrus and sulcus (pMCC, G_and_S_cingul-Mid-Post).
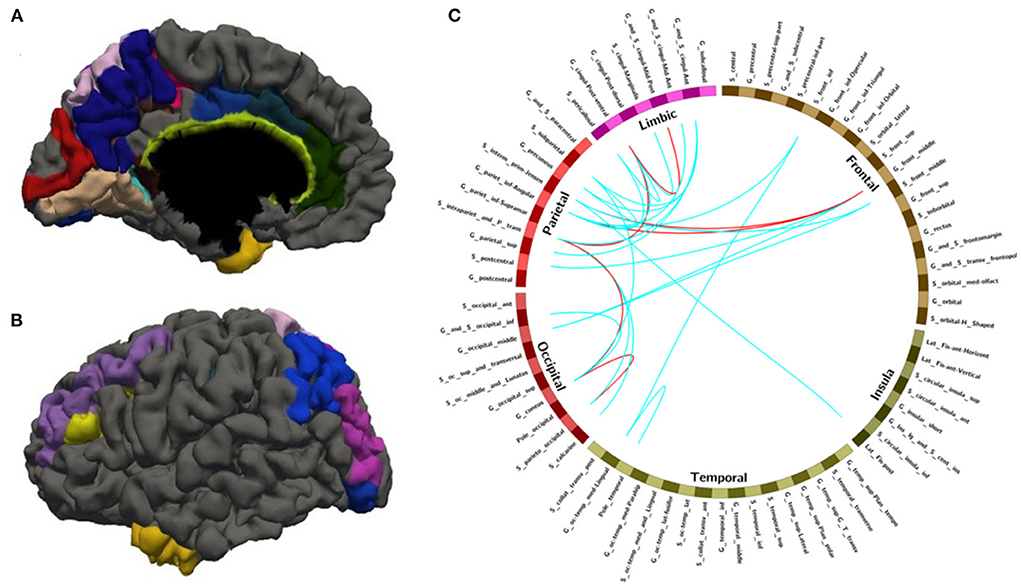
Figure 3. The nodes and edges involved in the FC features with high overlap rate (12/14) among training. (A,B) Regions involved in 28 FC features with overlapping ratios ≥12/14 across 14 training sessions. (C) Edges involved in 28 FC features with overlap ratio ≥12/14 in 14 training sessions.
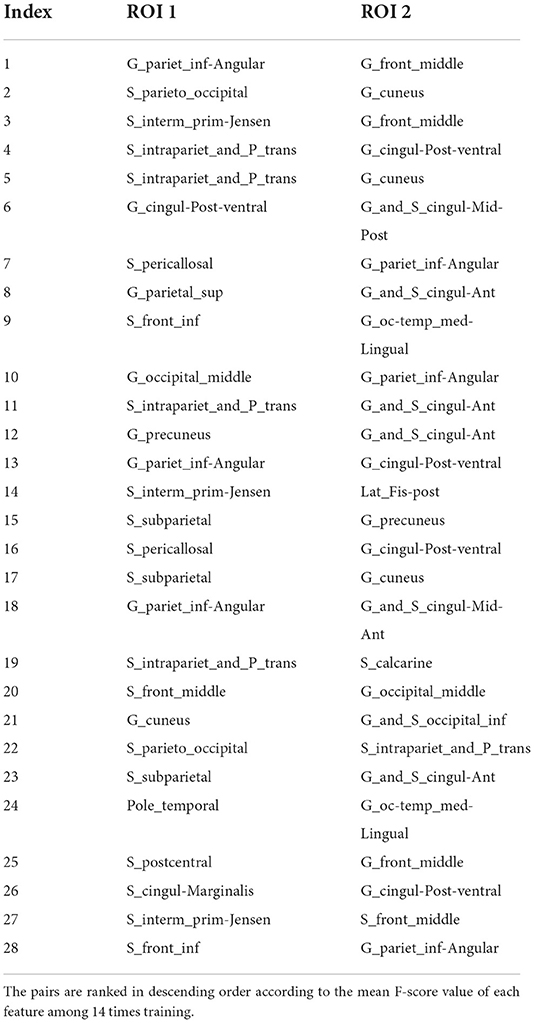
Table 4. The nodes and edges involved in the FC features with high overlap rate (12/14) among training.
According to these 28 FC features, we extracted the corresponding values in the testing dataset which contained 117 FC matrices for individuals with CPS and 114 FC matrices for individuals with SPS (as shown in the “Testing set” in Table 1). The test accuracy rate was 78.52%.
To further verify the stability of the results described above, we also adopted another criterion for sample selection in the training set. First, we mixed all the samples (FC matrices from all the time segments and all the subjects in the training set). For each time of the leave-one-out training procession, we randomly selected 90% of all the samples as a subset for training and the rest of 10% for validation. By calculating the F-score of each feature in the training subset, we took the features with top 1% F-score values as the input for the following SVM classifier. We also repeated this process 14 times. The validation accuracies in each leave-one-out loop are shown in Table 5, which varied from 78.55 to 87.21%, of which the mean is 82.69%. The final test accuracy rate reached upto 81.37%.
With random sample selection in leave-one-out training loop, we got 20 FC features with an overlap rate ≥12/14. Figure 4 and Table 6 demonstrated their nodes and edges. They were mainly located within and between the parietal, the occipital, and the frontal lobe, and the limbic system, similar to the results in Figure 3. For the temporal lobe and the insula, there was less different functional connectivity between SPS and CPS individuals, either. In Figure 4, we marked the FCs with great mean F-scores (higher than 75% of the highest mean F-score value among all the features) as a red line, and colored the others in blue. Specifically, the FCs with great mean F-scores were located between the intraparietal sulcus (S_intrapariet_and_P_trans) and the posterior-ventral part of the cingulate gyrus (vPCC, G_cingul-Post-ventral) and the cuneus (G_cuneus), between the angular gyrus (G_pariet_inf-Angular) and the middle frontal gyrus (G_front_middle) and pericallosal sulcus (S_pericallosal). Comparing the results obtained with the two different ways of sample selection, we can find that there are many coincident ROIs and edges, as shown in Figure 5 and Table 6 (marked with *).
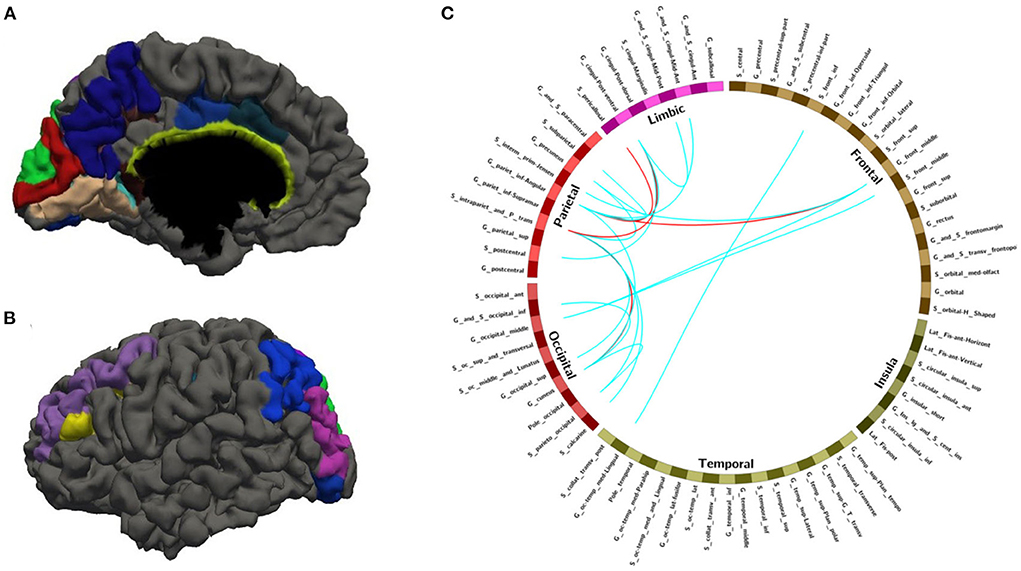
Figure 4. The nodes and edges involved in the FC features with a high overlap rate (12/14) among training with random sample selection. (A,B) Regions involved in 28 FC features with overlapping ratios ≥12/14 across 14 training sessions. (C) Edges involved in 28 FC features with overlap ratio ≥12/14 in 14 training sessions.
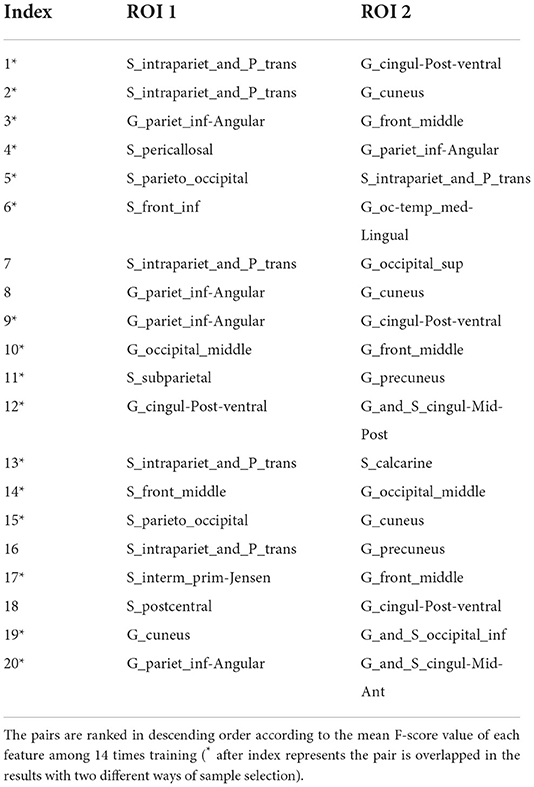
Table 6. The nodes and edges involved in the FC features with high overlap rate (12/14) among training with random sample selection.
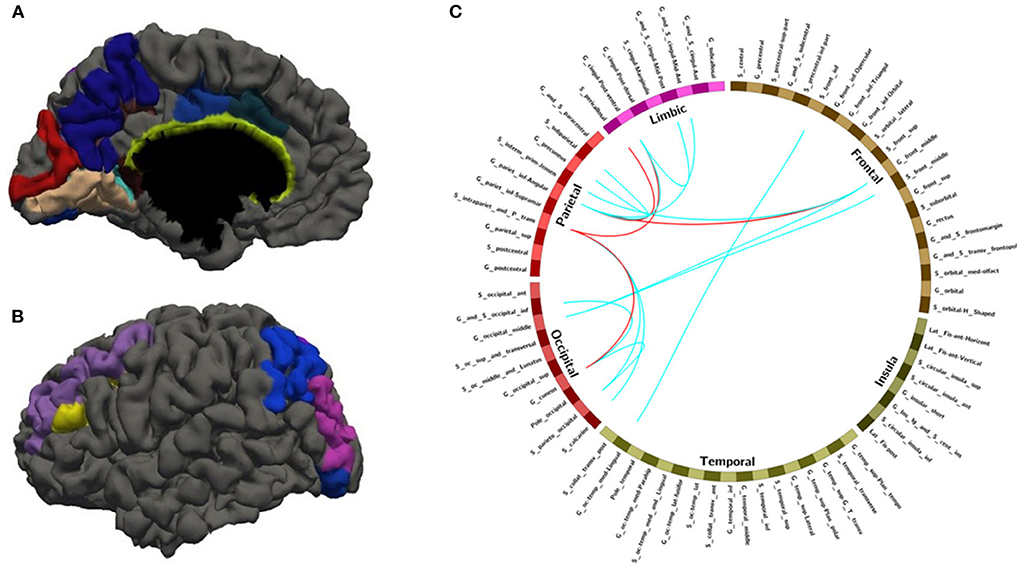
Figure 5. The overlapped ROIs and edges in both approaches (A,B) Regions involved in 28 FC features with overlapping ratios ≥12/14 across 14 training sessions. (C) Edges involved in 28 FC features with overlap ratio ≥12/14 in 14 training sessions. Red line: feature mean functional connections greater than the remaining 75% are marked with a red line. Blue line: no more than the remaining 75% of the feature mean functional connections are marked with blue lines.
Discussion
Summary
In our pre-experiment, we found a significant decrease in functional connectivity in both groups of patients compared to healthy controls, which was consistent with previous studies (Ives-Deliperi and Butler, 2021). To date, few studies have focused on the resting state of SPS and CPS, especially the analysis of differences in brain functional connectivity between the two groups. We evaluated subjects' FC for exploring network connection markers for TLE with altered consciousness status. The synchronicity of activities between network nodes was calculated at the brain network level. A higher mean F-score value indicated a stronger activity of network nodes and a greater connectivity with other nodes. The network connection differences of CPS from SPS were distinguished by SVM. In this way, dissimilar regions in the functional connectivity of global networks in SPS and CPS were determined. The following primary results were made: (1) The SVM classification model used the optimal feature set of 28 functional connections calculated from MEG data to distinguish the CPS subjects from SPS at a mean accuracy of 81.37% (sensitivity = 81.1%; specificity = 81.54%) on test data. (2) Compared with SPS, individuals with CPS revealed a hyper-connectivity in several primary regions including intraparietal sulcus, transverse parietal sulcus of brissaud, middle frontal gyrus, callosal suleus, ventral posterior cingulate gyrus, cuneus, and inferior parietal marginal angular gyrus. By comparing the differences in FC between SPS and CPS, it was possible to explore the pathological basis of consciousness impairment and cognitive abnormalities.
Relationship between CPS network connections and consciousness
There was no significant relationship between the occurrence of impaired consciousness in CPS and the functional connectivity of the epileptic region of origin (Najm, 2018). We did find that CPS and SPS functional connectivity differences were concentrated in extratemporal lobe regions. It was mainly distributed between the parietal, occipital, frontal. and limbic systems. This implied that the occurrence of CPS was not only associated with structural damages in the temporal lobe but also with abnormal brain network connectivity in extratemporal brain regions (Englot et al., 2010). Abnormal functional connectivity in these brain regions might accelerate the outward diffusion of temporal lobe discharge (Yoo et al., 2014; Sirin et al., 2020). This may lead to individuals who showed up with the loss of consciousness (Li et al., 2020).
Our observations were consistent with the “network inhibition hypothesis.” If seizures spread beyond the epileptogenic zone, consciousness may be vulnerable to impairment Bancaud et al., 1994; So, 1995; Norden and Blumenfeld, 2002; Blumenfeld et al., 2004; Englot et al., 2009, 2010. Previous studies have shown that the activation state of the frontoparietal region was associated with loss of consciousness (Untergehrer et al., 2014). CPS also had a distinct “spatial shift of slow waves”, that was, after a seizure, slow waves spread from the frontal cortex to the contralateral parietal and temporal lobes. However, this phenomenon was not found in SPS individuals (Yang et al., 2012). Similar results were seen in neuroimaging. Both SPECT and fMRI detected a reduction in subcortical cerebral blood oxygen level-dependent signals in the frontoparietal region in patients with generalized epilepsy (Gotman et al., 2005; Bai et al., 2010). Similarly, individuals with other unconscious states, such as coma, anesthesia, and brain death, showed impaired functional integration of the resembled cortex (Noirhomme et al., 2010; Crone et al., 2013; Gruenbaum, 2021). Therefore, we speculated that in TLE, the presence of abnormal functional connectivity in the frontoparietal cortex had led to impairment of consciousness. Aberrant neuronal discharge in patients with CPS activated significant network disturbances at a later stage, which in turn led to frontoparietal network abnormalities that clinically manifested as impaired consciousness.
Possible causes of automatism in patients with CPS
Increased epilepsy network coherence was a pathophysiology of epilepsy semiotics (Chauvel and McGonigal, 2014). Semiology depended on the interaction of epileptogenic focus and dissemination targets (Maillard et al., 2004). Automatism was considered to be one of the most common symptoms of CPS. About 75% of individuals with CPS might present with buccal and tongue movements, including smacking, swallowing, and spitting, called oral automatisms (OAAs) (Maldonado et al., 1988; Janati et al., 1990; Kramer et al., 1997).
Automatisms had been proposed to be associated with widespread cortical excitation. Several previous stimulation studies had shown that stimulation of frontal, insular, and temporal cortex or amygdala regions could induce automatisms (Maestro et al., 2008). The mechanism by which OAAs arose was the synchronous propagation of brain waves in the temporal–insular–parietal lobes, disturbing the cortical masticatory region. The abnormal cerebral cortex triggered the emergence of oral movements (Aupy et al., 2018). Similarly, individuals with preserved verbal responsiveness had lower frequencies of perfusion in ipsilateral parietal regions during interictal episodes of automatisms (Park et al., 2018). In our study, patients in the CPS group exhibited more complex clinical symptoms in addition to impaired consciousness compared to patients with SPS. There was a prominent difference in the functional connectivity in the CPS group. This further demonstrates that the clinical behavioral differences in patients with CPS were likely due to alterations in network connectivity between the limbic system and parietal lobes during the late stages of neuronal firing.
Limitations
The present study has several limitations. First, since the number of features was much more than the number of subjects used in classification, we used the F-score method to measure the classification ability of each input feature so as to select the most important edges for making accurate predictions (Guyon and Elissee, 2003). However, the F-score method did not take the mutual information between features into account (Chen et al., 2006), and it can only be used for two classifications. Once the number of classifications was more than two, the F-score method will fail. Second, an insufficient sample size of subjects was still a factor that cannot be ignored due to the limitation of the research. Due to the small sample size of individuals with simple clinical SPS or CPS, to strictly enroll the criteria, we only absorbed individuals whose timing of onset was short and the frequency of clinical seizure was low. Thus, there were only individuals who were up to standard with a single seizure form.
Conclusions
In this research, we divided the individuals with TLE into two subtypes according to different clinical symptoms, SPS and CPS, and constructed an SVM classifier to classify the functional connectivity corresponding to these two types of individuals. Finally, a classification accuracy of 78.52% was achieved when we used the already existing SVM classifier on the testing set. To further verify the stability of the results above, we redesigned the data set allocation method and extracted the features, and finally obtained 81.37% classification accuracy. The final results showed that the nodes and edges involved in these features extracted by the above two methods had a high coincidence. Moreover, the two groups of individuals had significant differences in the connection between the parietal lobe and other brain regions. This finding may provide an idea for studying the pathogenesis of CPS or new thinking that can be used to research refractory epilepsy.
Data availability statement
The original contributions presented in the study are included in the article/supplementary material, further inquiries can be directed to the corresponding author.
Ethics statement
The studies involving human participants were reviewed and approved by Ethics Committee of Affiliated Hospital of Nanjing University of Traditional Chinese Medicine. The patients/participants provided their written informed consent to participate in this study.
Author contributions
TW contributed to conceptualization, methodology, software, data curation, and writing. YW contributed to conceptualization, methodology, and supervision. ZL and YL contributed to methodology and software. YZ and YW contributed to writing the manuscript. YZ and XX contributed to conceptualization and supervision. All authors have read and agreed to the published version of the manuscript.
Funding
This work was partially supported by the National Natural Science Foundation of China (Grant No. 82172022).
Conflict of interest
The authors declare that the research was conducted in the absence of any commercial or financial relationships that could be construed as a potential conflict of interest.
Publisher's note
All claims expressed in this article are solely those of the authors and do not necessarily represent those of their affiliated organizations, or those of the publisher, the editors and the reviewers. Any product that may be evaluated in this article, or claim that may be made by its manufacturer, is not guaranteed or endorsed by the publisher.
References
Aupy, J., Noviawaty, I., Krishnan, B., Suwankpakdee, P., Bulacio, J., Gonzalez-Martinez, J., et al. (2018). Insulo-opercular cortex generates oroalimentary automatisms in temporal seizures. Epilepsia. 59, 583–594. doi: 10.1111/epi.14011
Bai, X., Vestal, M., Berman, R., Negishi, M., Spann, M., Vega, C., et al. (2010). Dynamic time course of typical childhood absence seizures: EEG, behavior, and functional magnetic resonance imaging. J. Neurosci. 30, 5884–5893. doi: 10.1523/JNEUROSCI.5101-09.2010
Bancaud, J., Brunet-Bourgin, F., Chauvel, P., and Halgren, E. (1994). Anatomical origin of déjà vu and vivid 'memories' in human temporal lobe epilepsy. Brain. 117, 71–90. doi: 10.1093/brain/117.1.71
Benbadis, S. R., Beniczky, S., Bertram, E., MacIver, S., and Mosh,é, S. L. (2020). The role of EEG in patients with suspected epilepsy. Epileptic Dis. 22, 143–155. doi: 10.1684/epd.2020.1151
Bharath, R. D., Panda, R., Raj, J., Bhardwaj, S., Sinha, S., Chaitanya, G., et al. (2019). Machine learning identifies “rsfMRI epilepsy networks” in temporal lobe epilepsy. Eur. Radiol. 29, 3496–3505. doi: 10.1007/s00330-019-5997-2
Blume, W. T. (2002). Complex partial seizures. Clinical description and diagnosis. Adv. Exp. Med. Biol. 497, 9–18. doi: 10.1007/978-1-4615-1335-3_2
Blumenfeld, H., McNally, K. A., Vanderhill, S. D., Paige, A. L., Chung, R., Davis, K., et al. (2004). Positive and negative network correlations in temporal lobe epilepsy. Cerebral Cortex, 14, 892–902. doi: 10.1093/cercor/bhh048
Blumenfeld, H., Varghese, G. I., Purcaro, M. J., Motelow, J. E., Enev, M., McNally, K. A., et al. (2009). Cortical and subcortical networks in human secondarily generalized tonic-clonic seizures. Brain. 132, 999–1012. doi: 10.1093/brain/awp028
Cantor-Rivera, D., Khan, A. R., Goubran, M., Mirsattari, S. M., and Peters, T. M. (2015). Detection of temporal lobe epilepsy using support vector machines in multi-parametric quantitative MR imaging. Comput. Med. Imaging Graph. 41, 14–28. doi: 10.1016/j.compmedimag.2014.07.002
Chauvel, P., and McGonigal, A. (2014). Emergence of semiology in epileptic seizures. Epilepsy Behav. 38, 94–103. doi: 10.1016/j.yebeh.2013.12.003
Chen, H., Dai, W. -L., Yang, X. -L., Gao, R., Cao, Y., Li, H., et al. (2006). Studies on the structural change of a reaction-controlled phase-transfer π−C5H5NC16H33]3{PO4[WO3]4 catalyst during the selective oxidation of cyclopentene to glutaric acid with aqueous H2sbO2. Appl. Cataly. A Gen. 309, 62–69. doi: 10.1016/j.apcata.2006.04.037
Craley, J., Johnson, E., and Venkataraman, A. (2020). A spatio-temporal model of seizure propagation in focal epilepsy. IEEE Trans. Med. Imaging. 39, 1404–1418. doi: 10.1109/TMI.2019.2950252
Crone, J. S., Soddu, A., Höller, Y., Vanhaudenhuyse, A., Schurz, M., Bergmann, J., et al. (2013). Altered network properties of the fronto-parietal network and the thalamus in impaired consciousness. NeuroImage. Clini. 4, 240–248. doi: 10.1016/j.nicl.2013.12.005
de Lanerolle, N. C., Kim, J. H., Robbins, R. J., and Spencer, D. D. (1989). Hippocampal interneuron loss and plasticity in human temporal lobe epilepsy. Brain Res. 495, 387–395. doi: 10.1016/0006-8993(89)90234-5
Depaulis, A., Helfer, V., Deransart, C., and Marescaux, C. (1997). Anxiogenic-like consequences in animal models of complex partial seizures. Neuroscience and biobehavioral reviews, 21, 767–774. doi: 10.1016/S0149-7634(96)00060-7
Destrieux, C., Fischl, B., Dale, A., and Halgren, E. (2010). Automatic parcellation of human cortical gyri and sulci using standard anatomical nomenclature. NeuroImage. 53, 1–15. doi: 10.1016/j.neuroimage.2010.06.010
Dhanalakshmi, V., Kumar, M. A., Rekha, R. U., Kumar, C. A., and Rajendran, S. (2009). “Morphological analyzer for agglutinative languages using machine learning approaches,” in International Conference on Advances in Recent Technologies in Communication & Computing (IEEE).
Elger, C. E., and Hoppe, C. (2018). Diagnostic challenges in epilepsy: seizure under-reporting and seizure detection. Lancet. Neurol. 17, 279–288. doi: 10.1016/S1474-4422(18)30038-3
Englot, D. J., D'Haese, P. F., Konrad, P. E., Jacobs, M. L., Gore, J. C., Abou-Khalil, B. W., et al. (2017). Functional connectivity disturbances of the ascending reticular activating system in temporal lobe epilepsy. J. Neurol. 88, 925–932. doi: 10.1136/jnnp-2017-315732
Englot, D. J., Modi, B., Mishra, A. M., DeSalvo, M., Hyder, F., and Blumenfeld, H. (2009). Cortical deactivation induced by subcortical network dysfunction in limbic seizures. J. Neurosci. 29, 13006–13018. doi: 10.1523/JNEUROSCI.3846-09.2009
Englot, D. J., Yang, L., Hamid, H., Danielson, N., Bai, X., Marfeo, A., et al. (2010). Impaired consciousness in temporal lobe seizures: role of cortical slow activity. Brain. 133, 3764–3777. doi: 10.1093/brain/awq316
Falco-Walter, J. J., Scheffer, I. E., and Fisher, R. S. (2018). The new definition and classification of seizures and epilepsy. Epilepsy Res. 139, 73–79. doi: 10.1016/j.eplepsyres.2017.11.015
Fallahi, A., Pooyan, M., Lotfi, N., Baniasad, F., Tapak, L., Mohammadi-Mobarakeh, N., et al. (2021). Dynamic functional connectivity in temporal lobe epilepsy: a graph theoretical and machine learning approach. Neurol.Sci. 42, 2379–2390. doi: 10.1007/s10072-020-04759-x
Fiest, K. M., Sauro, K. M., Wiebe, S., Patten, S. B., Kwon, C. S., Dykeman, J., et al. (2017). Prevalence and incidence of epilepsy: A systematic review and meta-analysis of international studies. Neurology. 88, 296–303. doi: 10.1212/WNL.0000000000003509
Glauser, T. A., Cnaan, A., Shinnar, S., Hirtz, D. G., Dlugos, D., Masur, D., et al. (2010). Ethosuximide, valproic acid, and lamotrigine in childhood absence epilepsy. N. Engl. J. Med. 362, 790–799. doi: 10.1056/NEJMoa0902014
Gleichgerrcht, E., Keller, S. S., Drane, D. L., Munsell, B. C., Davis, K. A., Kaestner, E., et al. (2020). Temporal lobe epilepsy surgical outcomes can be inferred based on structural connectome hubs: a machine learning study. Ann. Neurol. 88, 970–983. doi: 10.1002/ana.25888
González, H., Chakravorti, S., Goodale, S. E., Gupta, K., Claassen, D. O., Dawant, B., et al. (2019). Thalamic arousal network disturbances in temporal lobe epilepsy and improvement after surgery. J. Neurol. 90, 1109–1116. doi: 10.1136/jnnp-2019-320748
Gotman, J., Grova, C., Bagshaw, A., Kobayashi, E., Aghakhani, Y., and Dubeau, F. (2005). Generalized epileptic discharges show thalamocortical activation and suspension of the default state of the brain. Proc. Natl. Acad. Sci. U.S.A. 102, 15236–15240. doi: 10.1073/pnas.0504935102
Gruenbaum, B. F. (2021). Comparison of anaesthetic- and seizure-induced states of unconsciousness: a narrative review. Br. J. Anaesth. 126, 219–229. doi: 10.1016/j.bja.2020.07.056
Guye, M., Régis, J., Tamura, M., Wendling, F., McGonigal, A., Chauvel, P., et al. (2006). The role of corticothalamic coupling in human temporal lobe epilepsy. Brain. 129, 1917–1928. doi: 10.1093/brain/awl151
Guyon, I., and Elissee, A. (2003). An introduction to variable and feature selection. J. Mach. Learn. Res. 3, 1157–1182. Available online at: https://www.jmlr.org/papers/volume3/guyon03a/guyon03a.pdf
Hauf, M., Wiest, R., Schindler, K., Jann, K., Dierks, T., Strik, W., et al. (2013). Common mechanisms of auditory hallucinations-perfusion studies in epilepsy. Psychiat. Res. 211, 268–270. doi: 10.1016/j.pscychresns.2012.06.007
Hermann, B. P., Struck, A. F., Busch, R. M., Reyes, A., Kaestner, E., and McDonald, C. R. (2021). Neurobehavioural comorbidities of epilepsy: towards a network-based precision taxonomy. Neurology. 17, 731–746. doi: 10.1038/s41582-021-00555-z
Ines, H., Slim, Y., and Noureddine, E. (2013). “EEG classification using support vector machine,” in International Multi-conference on Systems (IEEE).
Inoue, Y., Mihara, T., Matsuda, K., Tottori, T., Otsubo, T., and Yagi, K. (2000). Absence of simple partial seizure in temporal lobe epilepsy: its diagnostic and prognostic significance. Epilepsy Res. 38, 133–138. doi: 10.1016/S0920-1211(99)00081-9
Ives-Deliperi, V., and Butler, J. T. (2021). Mechanisms of cognitive impairment in temporal lobe epilepsy: A systematic review of resting-state functional connectivity studies. Epilepsy Behavio. 115, 107686. doi: 10.1016/j.yebeh.2020.107686
Janati, A., Nowack, W. J., Dorsey, S., and Chesser, M. Z. (1990). Correlative study of interictal electroencephalogram and aura in complex partial seizures. Epilepsia. 31, 41–46. doi: 10.1111/j.1528-1157.1990.tb05358.x
Janszky, J., Schulz, R., and Ebner, A. (2004). Simple partial seizures (isolated auras) in medial temporal lobe epilepsy. Seizure. 13, 247–249. doi: 10.1016/S1059-1311(03)00192-4
Jin, S. H., and Chung, C. K. (2017). Electrophysiological resting-state biomarker for diagnosing mesial temporal lobe epilepsy with hippocampal sclerosis. Epilepsy Res. 129, 138–145. doi: 10.1016/j.eplepsyres.2016.11.018
Kakisaka, Y., Gupta, A., Enatsu, R., Wang, Z. I., Alexopoulos, A. V., Mosher, J. C., et al. (2013). Magnetoencephalography reveals a unique neurophysiological profile of focal-onset epileptic spasms. Tohoku J. Exp. Med. 229, 147–151. doi: 10.1620/tjem.229.147
Kramer, U., Riviello, J. J. Jr, Carmant, L., Black, P. M., Madsen, J., and Holmes, G. L. (1997). Clinical characteristics of complex partial seizures: a temporal versus a frontal lobe onset. Seizure. 6, 57–61. doi: 10.1016/S1059-1311(97)80054-4
Li, R., Hu, C., Wang, L., Liu, D., Liu, D., Liao, W., et al. (2020). Disruption of functional connectivity among subcortical arousal system and cortical networks in temporal lobe epilepsy. Brain Imaging Behav. 14, 762–771. doi: 10.1007/s11682-018-0014-y
Li, Y., Cui, W., Luo, M., Li, K., and Wang, L. (2018). Epileptic seizure detection based on time-frequency images of EEG signals using gaussian mixture model and gray level co-occurrence matrix features. Int. J. Neural Syst. 28, 1850003. doi: 10.1142/S012906571850003X
Li, Z., Jiang, C., Xiang, W., Qi, Z., Gao, Q., Peng, K., et al. (2022). Brain structural connectivity sub typing in unilateral temporal lobe epilepsy. Brain Imaging Behav. 10.1007/s11682-022-00691-0. doi: 10.1007/s11682-022-00691-0
Maestro, I., Carreño, M., Donaire, A., Rumi,à, J., Conesa, G., Bargall,ó, N., et al. (2008). Oroalimentary automatisms induced by electrical stimulation of the fronto-opercular cortex in a patient without automotor seizures. Epilepsy Behavior. 13, 410–412. doi: 10.1016/j.yebeh.2008.03.013
Maillard, L., Vignal, J. P., Gavaret, M., Guye, M., Biraben, A., McGonigal, A., et al. (2004). Semiologic and electrophysiologic correlations in temporal lobe seizure subtypes. Epilepsia. 45, 1590–1599. doi: 10.1111/j.0013-9580.2004.09704.x
Maldonado, H. M., Delgado-Escueta, A. V., Walsh, G. O., Swartz, B. E., and Rand, R. W. (1988). Complex partial seizures of hippocampal and amygdalar origin. Epilepsia. 29, 420–433. doi: 10.1111/j.1528-1157.1988.tb03741.x
McKavanagh, A., Kreilkamp, B., Chen, Y., Denby, C., Bracewell, M., Das, K., et al. (2021). Altered structural brain networks in refractory and nonrefractory idiopathic generalized epilepsy. Brain Connect. 10.1089/brain.2021.0035. doi: 10.1089/brain.2021.0035
Motelow, J. E., Li, W., Zhan, Q., Mishra, A. M., Sachdev, R. N., Liu, G., et al. (2015). Decreased subcortical cholinergic arousal in focal seizures. Neuron. 85, 561–572. doi: 10.1016/j.neuron.2014.12.058
Muayqil, T. A., Alanazy, M. H., Almalak, H. M., Alsalman, H. K., Abdulfattah, F. W., Aldraihem, A. I., et al. (2018). Accuracy of seizure semiology obtained from first-time seizure witnesses. BMC Neurol. 18, 135. doi: 10.1186/s12883-018-1137-x
Mukuta, Y., and Harada, T. (2014). “Probabilistic partial canonical correlation analysis,” in Proceedings of the 31st International Conference on Machine Learning, Vol. 32 (PMLR), 1449–1457.
Najm, I. M. (2018). Mapping brain networks in patients with focal epilepsy. The Lancet. 17, 295–297. doi: 10.1016/S1474-4422(18)30090-5
Nissen, I. A., Stam, C. J., van Straaten, E., Wottschel, V., Reijneveld, J. C., Baayen, J. C., et al. (2018). Localization of the epileptogenic zone using interictal MEG and machine learning in a large cohort of drug-resistant epilepsy patients. Front. Neurol. 9, 647. doi: 10.3389/fneur.2018.00647
Noirhomme, Q., Soddu, A., Lehembre, R., Vanhaudenhuyse, A., Boveroux, P., Boly, M., et al. (2010). Brain connectivity in pathological and pharmacological coma. Front. Neurol. 4, 160. doi: 10.3389/fnsys.2010.00160
Norden, A. D., and Blumenfeld, H. (2002). The role of subcortical structures in human epilepsy. Epilepsy Behav. 3, 219–231. doi: 10.1016/S1525-5050(02)00029-X
Park, C. H., Choi, Y. S., Jung, A. R., Chung, H. K., Kim, H. J., Yoo, J. H., et al. (2017). Seizure control and memory impairment are related to disrupted brain functional integration in temporal lobe epilepsy. J. Neuropsychiatry Clin. Neurosci. 29, 343–350. doi: 10.1176/appi.neuropsych.16100216
Park, H. R., Seong, M. J., Shon, Y. M., Joo, E. Y., Seo, D. W., and Hong, S. B. (2018). SPECT perfusion changes during ictal automatisms with preserved responsiveness in patients with right temporal lobe epilepsy. Epilepsy Behav. 80, 11–14. doi: 10.1016/j.yebeh.2017.12.030
Pavel, A. M., Rennie, J. M., de Vries, L. S., Blennow, M., Foran, A., Shah, D. K., et al. (2020). A machine-learning algorithm for neonatal seizure recognition: a multicentre, randomised, controlled trial. The Lancet. 4, 740–749. doi: 10.1016/S2352-4642(20)30239-X
Proposal for Revised Clinical and Electroencephalographic Classification of Epileptic Seizures. (1981). From the commission on classification and terminology of the International League Against Epilepsy. Epilepsia. 22, 489–501. doi: 10.1111/j.1528-1157.1981.tb06159.x
Rajpoot, K., Riaz, A., Majeed, W., and Rajpoot, N. (2015). Functional connectivity alterations in epilepsy from resting-state functional MRI. PloS ONE. 10, e0134944. doi: 10.1371/journal.pone.0134944
Richardson, M. P. (2012). Large scale brain models of epilepsy: dynamics meets connectomics. J. Neurol. Neurosurg. Psychiatry. 83, 1238–1248. doi: 10.1136/jnnp-2011-301944
Salma, Z., Hanen, H. K., Salma, S., Olfa, H., Nouha, F., Mariem, D., et al. (2019). Headaches and their relationships to epileptic seizures. Epilepsy Behav. 90, 233–237. doi: 10.1016/j.yebeh.2018.11.012
Scheffer, I. E., Berkovic, S., Capovilla, G., Connolly, M. B., French, J., Guilhoto, L., et al. (2017). ILAE classification of the epilepsies: position paper of the ILAE Commission for Classification and Terminology. Epilepsia. 58, 512–521. doi: 10.1111/epi.13709
Sirin, N. G., Kurt, E., Ulasoglu-Yildiz, C., Kicik, A., Bayram, A., Karaaslan, Z., et al. (2020). Functional connectivity analysis of patients with temporal lobe epilepsy displaying different ictal propagation patterns. Epileptic Dis. 22, 623–632. doi: 10.1684/epd.2020.1210
Sirven, J. I., Sperling, M. R., French, J. A., and O'Connor, M. J. (1996). Significance of simple partial seizures in temporal lobe epilepsy. Epilepsia. 37, 450–454. doi: 10.1111/j.1528-1157.1996.tb00590.x
So, E. L. (1995). Classifications and epidemiologic considerations of epileptic seizures and epilepsy. Neuroimaging Clin. N. Am. 5, 513–526.
Sriraam, N., and Raghu, S. (2017). Classification of focal and non focal epileptic seizures using multi-features and SVM classifier. J. Medical Syst. 41, 160. doi: 10.1007/s10916-017-0800-x
Stayman, A., and Abou-Khalil, B. (2011). FDG-PET in the diagnosis of complex partial status epilepticus originating from the frontal lobe. Epilepsy Behav. 20, 721–724. doi: 10.1016/j.yebeh.2011.02.001
Steriade, M. (1970). Ascending control of thalamic and cortical responsiveness. Int. Rev. Neurobiol. 12, 87–144. doi: 10.1016/S0074-7742(08)60059-8
Untergehrer, G., Jordan, D., Kochs, E. F., Ilg, R., and Schneider, G. (2014). Fronto-parietal connectivity is a non-static phenomenon with characteristic changes during unconsciousness. PloS ONE. 9, e87498. doi: 10.1371/journal.pone.0087498
van Klink, N., Mooij, A., Huiskamp, G., Ferrier, C., Braun, K., Hillebrand, A., et al. (2019). Simultaneous MEG and EEG to detect ripples in people with focal epilepsy. Clin Neurophysiol. 130, 1175–1183. doi: 10.1016/j.clinph.2019.01.027
van Mierlo, P., Papadopoulou, M., Carrette, E., Boon, P., Vandenberghe, S., Vonck, K., et al. (2014). Functional brain connectivity from EEG in epilepsy: seizure prediction and epileptogenic focus localization. Prog. Neurobiol. 121, 19–35. doi: 10.1016/j.pneurobio.2014.06.004
Verdru, J., and Van Paesschen, W. (2020). Wearable seizure detection devices in refractory epilepsy. Acta Neurologica Belgica. 120, 1271–1281. doi: 10.1007/s13760-020-01417-z
Xu, Q., Zhou, H., Wang, Y., and Huang, J. (2009). Fuzzy support vector machine for classification of EEG signals using wavelet-based features. Med. Eng. Phys. 31, 858–865. doi: 10.1016/j.medengphy.2009.04.005
Yang, L., Worrell, G. A., Nelson, C., Brinkmann, B., and He, B. (2012). Spectral and spatial shifts of post-ictal slow waves in temporal lobe seizures. Brain. 135, 3134–3143. doi: 10.1093/brain/aws221
Yang, W., Joo, M., Kim, Y., Kim, S. H., and Chung, J. M. (2020). “Hybrid machine learning scheme for classification of bects and TLE patients using EEG brain signals,” in IEEE Access (IEEE).
Yoo, J. Y., Farooque, P., Chen, W. C., Youngblood, M. W., Zaveri, H. P., Gerrard, J. L., et al. (2014). Ictal spread of medial temporal lobe seizures with and without secondary generalization: an intracranial electroencephalography analysis. Epilepsia. 55, 289–295. doi: 10.1111/epi.12505
Keywords: temporal lobe epilepsy, resting-state functional connectivity, MEG, machine learning, classification
Citation: Wang Y, Li Z, Zhang Y, Long Y, Xie X and Wu T (2022) Classification of partial seizures based on functional connectivity: A MEG study with support vector machine. Front. Neuroinform. 16:934480. doi: 10.3389/fninf.2022.934480
Received: 02 May 2022; Accepted: 27 June 2022;
Published: 18 August 2022.
Edited by:
Ke Liu, Chongqing University of Posts and Telecommunications, ChinaReviewed by:
Jinping Xu, Shenzhen Institutes of Advanced Technology (CAS), ChinaChao Wang, Shenzhen University, China
Copyright © 2022 Wang, Li, Zhang, Long, Xie and Wu. This is an open-access article distributed under the terms of the Creative Commons Attribution License (CC BY). The use, distribution or reproduction in other forums is permitted, provided the original author(s) and the copyright owner(s) are credited and that the original publication in this journal is cited, in accordance with accepted academic practice. No use, distribution or reproduction is permitted which does not comply with these terms.
*Correspondence: Ting Wu, ZnN5eTAwNTk4QG5qdWNtLmVkdS5jbg==
†These authors have contributed equally to this work and share first authorship