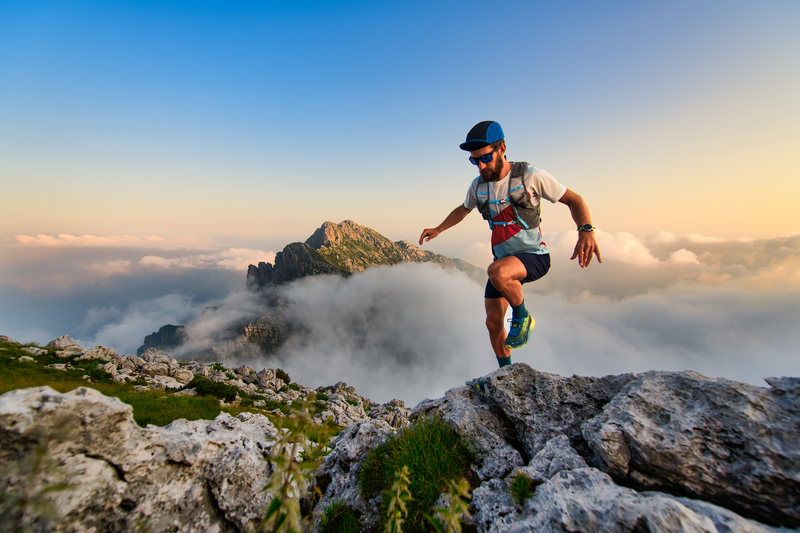
94% of researchers rate our articles as excellent or good
Learn more about the work of our research integrity team to safeguard the quality of each article we publish.
Find out more
ORIGINAL RESEARCH article
Front. Neuroinform. , 03 October 2022
Volume 16 - 2022 | https://doi.org/10.3389/fninf.2022.882126
Most stroke repetitive transcranial magnetic stimulation (rTMS) studies have used hand motor hotspots as rTMS stimulation targets; in addition, recent studies demonstrated that functional magnetic resonance imaging (fMRI) task activation could be used to determine suitable targets due to its ability to reveal individualized precise and stronger functional connectivity with motor-related brain regions. However, rTMS is unlikely to elicit motor evoked potentials in the affected hemisphere, nor would activity be detected when stroke patients with severe hemiplegia perform an fMRI motor task using the affected limbs. The current study proposed that the peak voxel in the resting-state fMRI (RS-fMRI) motor network determined by independent component analysis (ICA) could be a potential stimulation target. Twenty-one healthy young subjects underwent RS-fMRI at three visits (V1 and V2 on a GE MR750 scanner and V3 on a Siemens Prisma) under eyes-open (EO) and eyes-closed (EC) conditions. Single-subject ICA with different total number of components (20, 30, and 40) were evaluated, and then the locations of peak voxels on the left and right sides of the sensorimotor network (SMN) were identified. While most ICA RS-fMRI studies have been carried out on the group level, that is, Group-ICA, the current study performed individual ICA because only the individual analysis could guide the individual target of rTMS. The intra- (test-retest) and inter-scanner reliabilities of the peak location were calculated. The use of 40 components resulted in the highest test-retest reliability of the peak location in both the left and right SMN compared with that determined when 20 and 30 components were used for both EC and EO conditions. ICA with 40 components might be another way to define a potential target in the SMN for poststroke rTMS treatment.
Repetitive transcranial magnetic stimulation (rTMS), as a non-invasive neuromodulation technique, has been widely used to promote functional recovery in stroke patients (Barros Galvao et al., 2014; Aşkin et al., 2017; Dionisio et al., 2018; Bonin Pinto et al., 2019). Most stroke rTMS studies have used hand motor hotspots as stimulation targets (Sasaki et al., 2014; Diekhoff-Krebs et al., 2017; Long et al., 2018). Recent studies have demonstrated that functional magnetic resonance imaging (fMRI) task activation could also be considered a target due to its individualized precise and stronger functional connectivity (FC) with motor-related brain regions (Hulst et al., 2017). However, in stroke patients with severe hemiplegia, rTMS is unlikely to elicit motor evoked potentials in the affected hemisphere, nor is activity expected to be detected when these individuals perform an fMRI motor task using the affected limbs (Sasaki et al., 2014; Diekhoff-Krebs et al., 2017; Long et al., 2018).
Resting-state fMRI (RS-fMRI) FC is an approach to assessing the relationships among brain regions. This connectivity represents the temporal synchronization of spontaneous brain activity among distributed brain regions associated with particular functions (Fox et al., 2005; Cole et al., 2010). Over recent years, the combination of high spatial resolution functional magnetic resonance imaging (fMRI) and navigation systems allows researchers to locate the stimulation target with millimeter accuracy. Considering the variability of the individual brain, a precisely localized stimulation target is the key issue to improve the efficacy of stimulation treatment. A study defined the peak voxel of RS-fMRI seed-based FC in the superficial brain area as an rTMS target and then successfully altered FC while improving participants' memory performance (Wang et al., 2014b). Another recent study found that seed-based FC strength could predict local activity changes in the deep brain region (Feng et al., 2021). Stroke RS-fMRI studies have used seed-based FC analysis to investigate the abnormal FC of patients with damaged motor function (Park et al., 2011; Zheng et al., 2016). However, how an effective stimulation target for rTMS treatment should be defined remains unknown.
As a data-driven method, independent component analysis (ICA) decomposes whole-brain fMRI data into a given number of spatially independent components (McKeown et al., 1998; Pamilo et al., 2012). ICA has been applied in many studies, resulting in the elucidation of a host of brains intrinsic connectivity networks, such as the default mode network, visual network, sensorimotor network (SMN), language network, and auditory network (Stevens et al., 2009; Allen et al., 2011; Shirer et al., 2012; Iraji et al., 2019). RS-fMRI studies using ICA found a decreased SMN in stroke patients (Zhang et al., 2017; Zhao et al., 2018b). One of the hypotheses of the outcome of TMS in stroke treatment is to modulate sensorimotor network functions (Grefkes and Fink, 2012). Therefore, we proposed that the location of the peak voxel might be a potential stimulation target for rTMS treatment for patients with poststroke hemiplegia.
If the peak voxel is indeed a suitable stimulation target, then the reliability of the location of the peak voxel in the SMN is critical. One study reported that rTMS induced an increase in motor-cortical excitability in only 52% of healthy subjects, while the other half responded with a decrease in excitability (Hamada et al., 2013). In addition to stimulation parameters, the bias of the target may be one of the reasons for the unclear effect of rTMS. Quite a few studies have reported moderate to high test-retest reliability of ICA metrics, including well-reproducible spatial patterns (Franco et al., 2009; Meindl et al., 2010) and voxel-wise intraclass correlation (ICC) (Zuo et al., 2010). However, the reliability of the location of the peak voxel in the ICA network has not been reported. Considering the potential importance of individualized precise rTMS treatment, the reliability of peak location of single-subject ICA-derived SMN must first be assessed.
In addition, it should be noted that several factors may affect the reliability of ICC approaches. First, there is little consensus on how many components to extract for single-subject ICA. Second, RS-fMRI is unconstrained by the task and it is affected by the status of the subjects. For example, Yan and colleagues found that the FC was significantly different among the EC and EO conditions in SMN (Yan et al., 2009). Finally, the inter-scanner differences could introduce significant variability in the RS-fMRI signal (Zhao et al., 2018a). The current study investigated both intra- (test-retest) and inter-scanner reliability of the SMN peak voxel location under EO and EC conditions to evaluate whether the peak location of SMN could be a potential target for rTMS treatment.
The RS-fMRI data in the current study were acquired in previous test-retest reliability studies (Yuan et al., 2018; Zhao et al., 2018a). The current study focused on the test-retest reliability of the peak location of ICA-driven SMN, and the content of the current study had no relation to their studies.
A flowchart of the current study is shown in Figure 1. Twenty-one healthy participants (21.8 ± 1.8 years old, 11 females) with no history of neurological or psychiatric disorders were recruited. All subjects underwent RS-fMRI scans three times; the first two were performed during the first two visits (V1 and V2, approximately 2 weeks apart) with one GE 3T scanner (MR750, GE Medical Systems, Milwaukee, WI) and the last scan was performed during the third visit (V3, approximately 8 months after V2) with a Siemens 3T scanner (Prisma, Siemens Healthineers Erlangen, Germany). For scans on both GE and Siemens scanners, a gradient-echo echo-planar imaging (EPI) pulse sequence was used for blood oxygen level-dependent (BOLD) images with the following parameters: repetition time (TR) = 2,000 ms; echo time (TE) = 30 ms; flip angle (FA) = 90°; 43 slices with interleaved acquisition; matrix = 64 × 64; field of view (FOV) = 220 mm; and acquisition voxel size = 3.44 × 3.44 × 3.20 mm. For each visit, all the participants underwent two 8-min RS-fMRI sessions (i.e., EC and EO), during which they were asked to relax and either keep their eyes open naturally (without fixation) or keep their eyes closed, not to think of anything in particular, and not to fall asleep. To minimize head movement, straps and foam pads were used to fix the participants' heads comfortably during scanning.
Analysis of RS-fMRI data was performed by using DPABI_5.1 (C. G. Yan et al., 2016). Data preprocessing included (1) removing the first 10 volumes; (2) slice timing correction; (3) head motion correction; (4) spatial normalization to standard Montreal Neurological Institute (MNI) space by using an EPI template; and (5) spatial smoothing with a Gaussian kernel of 6-mm full width at half-maxima (FWHM).
Single-subject level spatial ICA was used to separate the BOLD signal into spatially independent components using the Group ICA of fMRI Toolbox (GIFT) (http://icatb.sourceforge.net/) (Calhoun et al., 2001). The RS-fMRI studies of ICA-derived SMN usually used 20 to 40 components in recent years (Smith et al., 2009; Zuo et al., 2010; Besseling et al., 2013; Shumskaya et al., 2017; Ye et al., 2017; Thomas et al., 2019; Caspers et al., 2021; Legget et al., 2021). The current study, therefore, performed single-subject ICA with three different numbers of total components (i.e., 20, 30, and 40). Independent components were estimated by the Infomax algorithm, which is based on the principle of maximum information transfer (Bell and Sejnowski, 1995). Component stability was determined by running the ICASSO toolbox, which was used to perform 20 iterations of the ICA with randomly initialized decomposition matrices and the same convergence threshold (Himberg and Hyvarinen, 2003; Himberg et al., 2004). Then, the components of a single subject were obtained and the spatial maps were then converted into z-scores (threshold = 1).
SMN components were identified by using Dice coefficient quantification with an SMN mask and careful visual inspection, as described in previous studies (Akin et al., 2017; Venkataraman et al., 2021). The SMN mask includes the bilateral pre/postcentral gyri (Pre/PostCG) and supplementary motor area (SMA), which was constructed using the Harvard-Oxford probabilistic atlas from the fMRIB Software Library (FSL) (Smith et al., 2004; Makris et al., 2006). The SMN mask was transferred to the MNI space as same as fMRI data. A previous study reported that the SMN could be represented as two separate components (i.e., left and right) when evaluated by ICA (Abou-Elseoud et al., 2010). Thus, the components with the largest and second-largest Dice coefficients with the SMN mask were selected and further confirmed by visual inspection.
The coordinates of the peak voxel within both the left and right SMN were obtained. The intra-scanner (i.e., V1 vs. V2) and inter-scanner (i.e., V1 vs. V3 and V2 vs. V3) reliability of the left and right SMN peak locations under both EC and EO conditions were estimated using ICCs according to the following equation (Shrout and Fleiss, 1979):
where Msb represents the between-subject mean square of coordinate, MSw represents the within-subject mean square of coordinate, and K is the number of sessions.
The ICCs of the X, Y, and Z axes were calculated separately, and the averaged ICCs of the three axes were obtained. Although ICCs theory is never negative, ICCs estimates can take negative values when MSb is smaller than MSw. In the current study, negative values were calculated as 0 with an understanding that the true ICCs were very low in these conditions as the previous study mentioned (Ma and MacDonald, 2021).
We performed single-subject ICA 378 times: 21 (subjects) × 2 (EO and EC) × 3 (V1, V2, V3) × 3 (20, 30, and 40 total number of components). For the 378 iterations, most of the outcomes indicated the bilateral SMN (Figures 2, 3), while 23 times yielded the left and right SMN separately (The dice coefficients of the two components were close, and the spatial components of motor areas were symmetrical) (Figure 2). For the left or right component, we used the left or right side of the SMN mask to obtain the peak location.
Figure 2. The representative SMN with the total number of components = 40. (A) The bilateral SMN of a representative subject with the total number of components = 40. (B,C) The separate left and right SMN of another subject with the total number of components = 40. The arrows indicate the peaks. Of the 378 iterations, most indicated bilateral SMN, while 23 iterations yielded the left and right SMN separately.
Figure 3. The representative SMN of 21 subjects (visit 1, total number of 40 components, EO condition).
Overall, for both EO and EC conditions, the peak location based on 40 components had the highest intra-scanner (test-retest) reliability (averaged ICC = 0.72~0.8), and was similar for EO and EC conditions (Table 1, Figure 4). The peak locations based on 20 and 30 components had low to moderate intra-scanner reliability (averaged ICC = 0.03~0.73). The intra-scanner reliability was higher than the inter-scanner reliability for the locations determined based on all the component totals under both EO and EC conditions.
Table 1. The intra- and inter-scanner reliability of peak voxels within the left and right SMN of EO/EC conditions.
Figure 4. Intra- and inter-scanner test-retest reliability of the peak location of the SMN. Overall, for both EO and EC conditions, the peak location based on a total of 40 components had the highest intra-scanner (test-retest) reliability (averaged ICC = 0.72~0.8), being similar for EO and EC conditions. The 20 and 30 components had low to moderate intra-scanner reliability (averaged ICC = 0.03~0.73). The inter-scanner reliability of the total number of components was low. ICC, intraclass correlation; EO, eyes open; EC, eyes closed; V, visit.
We also calculated the Euclidean distance of the peak location between V1 and V2 (i.e., intra-scanner distance). Tables 2–4 showed the Euclidean distance of each subject based on 20, 30, and 40 components, the peak voxels with lower ICC (20 and 30 number of components) showed very large Euclidean distance (18.85−47 mm and 21.63–33.5 mm), while the peak voxels with highest ICC (40 number of components) showed smaller Euclidean distance (18.80−21.45 mm). It should be noted that only 41% of peaks had an intra-scanner distance of 0–10 mm. This means that although the ICC was higher, the peak distance between the two visits was large.
Table 2. The Euclidean distance (mm) between the peak locations of V1 and V2 within the left/right SMN of EO/EC conditions (20 components).
Table 3. The Euclidean distance (mm) between the peak locations of V1 and V2 within the left/right SMN of EO/EC conditions (30 components).
Table 4. The Euclidean distance between the peak locations of V1 and V2 within the left/right SMN of EO/EC conditions (40 components).
To the best of our knowledge, the current RS-fMRI study was the first to evaluate the test-retest reliability of the peak voxel location using ICA; these outcomes have potential clinical significance for precise targeting of rTMS. We found that the use of 40 total components had the highest intra-scanner (test-retest) reliability for the peak voxel location of the SMN compared to that obtained when using 20 and 30 components.
The current study proposed that the location of the voxel with peak FC might be a potential stimulation target for rTMS treatment of poststroke hemiplegia. Motor dysfunction in stroke patients was viewed as a system-level disruption of brain networks (Wang et al., 2010, 2014a) without a common local lesion. Previous RS-fMRI studies have found decreased brain activity of the SMN in stroke patients by using either ICA (Zhang et al., 2017; Zhao et al., 2018b) or seed-based FC analysis (Zheng et al., 2016; Tsuchimoto et al., 2019). Another study conducted an assessment to determine the peak voxel in the ipsilesional precentral gyrus during group-level task activation that could be used as a seed region of interest (ROI) for RS-fMRI FC analysis and found decreased connectivity of the ipsilesional M1 with the contralesional SMN (Zheng et al., 2016). Seed-based FC has been successfully used to define the rTMS target for modulating brain functions in healthy subjects (Wang et al., 2014b). Although abnormal FC of the SMN was reported in the above-mentioned studies, it is certainly not easy to define a common seed ROI for FC-guided rTMS of poststroke hemiplegia due to its diverse lesion foci. Precentral activation-based FC is unable to comprehensively and objectively reflect all poststroke motor functions, especially the functions of the SMN. Moreover, there is compelling evidence that the neural correlates driving hand movements are rather dependent on the regulation of the interactions among regions of the motor network (Solodkin et al., 2004; Swinnen and Wenderoth, 2004; Wiesendanger and Serrien, 2004). Importantly, the precentral gyrus may no longer be a representation of the SMN when hemiplegia occurs after a stroke. Graph theory studies have reported that the degree and betweenness centrality of some hub nodes significantly decreased when the function of the network was affected; hence, these nodes were no longer hubs (Pandit et al., 2013; Aerts et al., 2016). Although the reliability of the SMN peak location in the current study was based on healthy subjects and not on stroke patients, the previous study had indicated that the response to plasticity-inducing intervention might depend on how the stimulation interacts with the pre-existing connectivity in the functional network (Diekhoff-Krebs et al., 2017). And for stroke patients, the peak location of the ipsilateral hemisphere SMN is still the node with the strongest functional connectivity. Thus, defining a location that represents the neural activity of the whole SMN can yet be regarded as a kind of method to identify rTMS therapeutic targets in structurally damaged brains.
As a data-driven method, ICA could provide complementary and valuable information for investigating the mechanism of impaired function of the brain network in diverse neurologic diseases. For example, the most consistent finding obtained by using seed-based FC in previous RS-fMRI studies was that patients with motor impairment after stroke show decreased connectivity of the ipsilesional precentral gyrus with the contralesional SMN (Park et al., 2011; Zheng et al., 2016), while ICA studies reported decreased connectivity in both the ipsilesional and contralesional precentral and postcentral gyrus (S1) (Zhang et al., 2017; Zhao et al., 2018b). As the core network is vulnerable to stroke lesions, ICA-derived SMN may yield results that lead to a more comprehensive understanding of network impairment in stroke patients with hemiplegia compared to the results obtained from seed-based FC. Moreover, we found that 51% of the total 740 peaks (348 for both left and right, and 16 peaks were excluded because of an unidentified SMN component) were in the precentral gyrus, 46% of the peaks were in the postcentral gyrus, and 3% of the peaks were in the SMA. Notably, studies of stroke have demonstrated that abnormal local brain activity within the postcentral gyrus was associated with somatosensory deficits (Liang et al., 2018; Jiang et al., 2019), while increased fMRI task activation was found in the precentral gyrus and appeared in the postcentral gyrus along with motor function recovery (Luft et al., 2004). In addition, the peak location of the ICA network represents the position with the strongest temporal synchronization of spontaneous brain activity with other regions within the functional network (Damoiseaux et al., 2008), and the peak location of the postcentral as the strongest functional connectivity within SMN may be the hub that modulates the network, not just the precentral gyrus. The findings mentioned earlier lead to one hypothesis regarding whether the SMN peak in the precentral or postcentral gyrus could be a potential target for rTMS to modulate SMN functions and further improve motor symptoms.
The current study proposed that the peak location in the ICA-derived SMN could be a potential stimulation target for rTMS treatment of poststroke hemiplegia. However, the peak location may vary due to the number of components, and therefore, the current study tested the intra- and inter-scanner reliability of the peak SMN location in which the total number of components was a factor. Previous studies focused on RS-fMRI ICA found that functionally connected regions would be split into separate subnetworks with a high number of components (Van de Ven et al., 2004), while the separated subnetworks would be mixed with various components, such as noise, when too few components were set (Van de Ven et al., 2004; Bartels and Zeki, 2005). To date, there is no accepted number of components for the separation of individual SMNs, and previous studies have used different numbers of components for different purposes. The current study measured the test-retest reliability of individualized SMNs with three different numbers of total components that were commonly used in previous ICA studies. Among the three different numbers of total components, we found that the use of 40 components resulted in the highest intra-scanner reliability of the SMN peak location. Given the number of components has been an uncertain question of ICA, it could be a reference to obtaining a stable location as an rTMS target. Further analysis of Euclidean distance between two visits showed that the peak voxels with lower ICC (20 and 30 number of components) shower very large Euclidean distance, while the peak voxels with highest ICC showed smaller Euclidean distance. Although several subjects showed large distance (>10 mm), the average Euclidean distance of 40 components is much smaller than 20 and 30. Future studies could focus on improving the reliability of single-subject ICA network peak locations. It should be noted that the reliability of the SMN peak location in the current study was based on healthy subjects and not on stroke patients. To verify the feasibility of the proposed method on stroke patients, future studies should measure the test-retest reliability of peak voxels on stroke patients. The high test-retest reliability is a suitable characteristic that makes this location a potential rTMS target for hemiplegia poststroke and other neuropsychiatric disorders.
The first limitation of the current study was the relatively short RS-fMRI scan (8 min). It has been reported that the reliability of FC could be greatly improved by increasing the scanning time (Mueller et al., 2015). Longer scanning times in future studies may improve the reliability of the peak location of the ICA-driven network.
The second limitation was that the current results showed only 41% peaks of number of 40 components had an intra-scanner Euclidean distance of 0–10 mm. Although the ICC was higher, according to the results of Euclidean distance analysis, some subjects' peak distance between two visits was large. The specific reasons for this difference are not clear for now, and future studies could focus on improving the reliability of single-subject ICA network peak locations.
The third limitation was that the inter-scanner spans short and long term but does not balance the order of scanning machines. Future studies should balance the scanning order to explore inter-scanner reliability.
The datasets presented in this study can be found in online repositories. The names of the repository/repositories and accession number(s) can be found at: https://www.nitrc.org/.
The studies involving human participants were reviewed and approved by Ethics Committee of the Center for Cognition and Brain Disorders (CCBD) at Hangzhou Normal University (HZNU). The patients/participants provided their written informed consent to participate in this study.
Y-SH, JW, and Y-FZ analyzed the data and wrote the paper. JY, QG, and Z-JF processed the data. All authors contributed to the article and approved the submitted version.
This study was supported by NSFC (82071537, 81520108016), Key Realm R&D Program of Guangdong Province (2019B030335001), Key Medical Discipline of Hangzhou, and The Cultivation Project of the Province-Leveled Preponderant Characteristic Discipline of Hangzhou Normal University (18JYXK046, 20JYXK004).
The authors declare that the research was conducted in the absence of any commercial or financial relationships that could be construed as a potential conflict of interest.
All claims expressed in this article are solely those of the authors and do not necessarily represent those of their affiliated organizations, or those of the publisher, the editors and the reviewers. Any product that may be evaluated in this article, or claim that may be made by its manufacturer, is not guaranteed or endorsed by the publisher.
Abou-Elseoud, A., Starck, T., Remes, J., Nikkinen, J., Tervonen, O., and Kiviniemi, V. (2010). The effect of model order selection in group PICA. Hum. Brain Mapp. 31, 1207–1216. doi: 10.1002/hbm.20929
Aerts, H., Fias, W., Caeyenberghs, K., and Marinazzo, D. (2016). Brain networks under attack: robustness properties and the impact of lesions. Brain 139, 3063–3083. doi: 10.1093/brain/aww194
Akin, B., Lee, H. L., Hennig, J., and LeVan, P. (2017). Enhanced subject-specific resting-state network detection and extraction with fast fMRI. Hum. Brain Mapp. 38, 817–830. doi: 10.1002/hbm.23420
Allen, E. A., Erhardt, E. B., Damaraju, E., Gruner, W., Segall, J. M., Silva, R. F., et al. (2011). A baseline for the multivariate comparison of resting-state networks. Front. Syst. Neurosci. 5, 2. doi: 10.3389/fnsys.2011.00002
Aşkin, A., Tosun, A., and Demirdal, Ü. S. (2017). Effects of low-frequency repetitive transcranial magnetic stimulation on upper extremity motor recovery and functional outcomes in chronic stroke patients: a randomized controlled trial. Somatosens. Mot. Res. 34, 102–107. doi: 10.1080/08990220.2017.1316254
Barros Galvao, S. C., Borba Costa dos Santos, R., Borba dos Santos, P., Cabral, M. E., and Monte-Silva, K. (2014). Efficacy of coupling repetitive transcranial magnetic stimulation and physical therapy to reduce upper-limb spasticity in patients with stroke: a randomized controlled trial. Arch. Phys. Med. Rehabil. 95, 222–229. doi: 10.1016/j.apmr.2013.10.023
Bartels, A., and Zeki, S. (2005). Brain dynamics during natural viewing conditions–a new guide for mapping connectivity in vivo. Neuroimage 24, 339–349. doi: 10.1016/j.neuroimage.2004.08.044
Bell, A. J., and Sejnowski, T. J. (1995). An information-maximization approach to blind separation and blind deconvolution. Neural Comput. 7, 1129–1159. doi: 10.1162/neco.1995.7.6.1129
Besseling, R. M., Jansen, J. F., Overvliet, G. M., van der Kruijs, S. J., Vles, J. S., Ebus, S. C., et al. (2013). Reduced functional integration of the sensorimotor and language network in rolandic epilepsy. Neuroimage Clin. 2, 239–246. doi: 10.1016/j.nicl.2013.01.004
Bonin Pinto, C., Morales-Quezada, L., de Toledo Piza, P. V., Zeng, D., Saleh Vélez, F. G., Ferreira, I. S., et al. (2019). Combining fluoxetine and rtms in poststroke motor recovery: a placebo-controlled double-blind randomized phase 2 clinical trial. Neurorehabil. Neural Repair 33, 643–655. doi: 10.1177/1545968319860483
Calhoun, V. D., Adali, T., Pearlson, G. D., and Pekar, J. J. (2001). A method for making group inferences from functional MRI data using independent component analysis. Hum. Brain Mapp. 14, 140–151. doi: 10.1002/hbm.1048
Caspers, J., Rubbert, C., Eickhoff, S. B., Hoffstaedter, F., Südmeyer, M., Hartmann, C. J., et al. (2021). Within- and across-network alterations of the sensorimotor network in Parkinson's disease. Neuroradiology 63, 2073–2085. doi: 10.1007/s00234-021-02731-w
Cole, D. M., Smith, S. M., and Beckmann, C. F. (2010). Advances and pitfalls in the analysis and interpretation of resting-state FMRI data. Front. Syst. Neurosci. 4, 8. doi: 10.3389/fnsys.2010.00008
Damoiseaux, J. S., Beckmann, C. F., Arigita, E. J., Barkhof, F., Scheltens, P., Stam, C. J., et al. (2008). Reduced resting-state brain activity in the “default network” in normal aging. Cereb. Cortex 18, 1856–1864. doi: 10.1093/cercor/bhm207
Diekhoff-Krebs, S., Pool, E. M., Sarfeld, A. S., Rehme, A. K., Eickhoff, S. B., Fink, G. R., et al. (2017). Interindividual differences in motor network connectivity and behavioral response to iTBS in stroke patients. Neuroimage Clin. 15, 559–571. doi: 10.1016/j.nicl.2017.06.006
Dionisio, A., Duarte, I. C., Patricio, M., and Castelo-Branco, M. (2018). The use of repetitive transcranial magnetic stimulation for stroke rehabilitation: a systematic review. J. Stroke Cerebrovasc. Dis. 27, 1–31. doi: 10.1016/j.jstrokecerebrovasdis.2017.09.008
Feng, Z. J., Deng, X. P., Zhao, N., Jin, J., Yue, J., Hu, Y. S., et al. (2021). Resting-state fMRI functional connectivity strength predicts local activity change in the dorsal cingulate cortex: a multi-target focused rTMS study. Cereb. Cortex. 32, 2773–2784. doi: 10.1093/cercor/bhab380
Fox, M. D., Snyder, A. Z., Vincent, J. L., Corbetta, M., Van Essen, D. C., and Raichle, M. E. (2005). The human brain is intrinsically organized into dynamic, anticorrelated functional networks. Proc. Natl. Acad. Sci. USA. 102, 9673–9678. doi: 10.1073/pnas.0504136102
Franco, A. R., Pritchard, A., Calhoun, V. D., and Mayer, A. R. (2009). Interrater and intermethod reliability of default mode network selection. Hum. Brain Mapp. 30, 2293–2303. doi: 10.1002/hbm.20668
Grefkes, C., and Fink, G. R. (2012). Disruption of motor network connectivity post-stroke and its noninvasive neuromodulation. Curr. Opin. Neurol. 25, 670–675. doi: 10.1097/WCO.0b013e3283598473
Hamada, M., Murase, N., Hasan, A., Balaratnam, M., and Rothwell, J. C. (2013). The role of interneuron networks in driving human motor cortical plasticity. Cereb. Cortex 23, 1593–1605. doi: 10.1093/cercor/bhs147
Himberg, J., and Hyvarinen, A. (2003). “Icasso: software for investigating the reliability of ICA estimates by clustering and visualization,” in Processeding IEEE Workshop on Neural Networks for Signal Processing (NNSP2003) (Toulouse: IEEE Press), 259–268.
Himberg, J., Hyvärinen, A., and Esposito, F. (2004). Validating the independent components of neuroimaging time series via clustering and visualization. Neuroimage 22, 1214–1222. doi: 10.1016/j.neuroimage.2004.03.027
Hulst, H. E., Goldschmidt, T., Nitsche, M. A., de Wit, S. J., van den Heuvel, O. A., Barkhof, F., et al. (2017). rTMS affects working memory performance, brain activation and functional connectivity in patients with multiple sclerosis. J. Neurol. Neurosurg. Psychiatry 88, 386–394. doi: 10.1136/jnnp-2016-314224
Iraji, A., Deramus, T. P., Lewis, N., Yaesoubi, M., Stephen, J. M., Erhardt, E., et al. (2019). The spatial chronnectome reveals a dynamic interplay between functional segregation and integration. Hum. Brain Mapp. 40, 3058–3077. doi: 10.1002/hbm.24580
Jiang, C., Yi, L., Cai, S., and Zhang, L. (2019). Ischemic stroke in pontine and corona radiata: location specific impairment of neural network investigated with resting state fMRI. Front. Neurol. 10, 575. doi: 10.3389/fneur.2019.00575
Legget, K. T., Wylie, K. P., Cornier, M. A., Berman, B. D., and Tregellas, J. R. (2021). Altered between-network connectivity in individuals prone to obesity. Physiol. Behav. 229, 113242. doi: 10.1016/j.physbeh.2020.113242
Liang, L., Hu, R., Long, W., Feng, B., and Song, R. (2018). “Altered regional homogeneity and amplitude of low-frequency fluctuations in sub-acute ischemic stroke: a resting-state fMRI study,” in 2018 IEEE International Conference on Cyborg and Bionic Systems (CBS), 637–640. doi: 10.1109/CBS.2018.8612170
Long, H., Wang, H., Zhao, C., Duan, Q., Feng, F., Hui, N., et al. (2018). Effects of combining high- and low-frequency repetitive transcranial magnetic stimulation on upper limb hemiparesis in the early phase of stroke. Restor. Neurol. Neurosci. 36, 21–30. doi: 10.3233/RNN-170733
Luft, A. R., McCombe-Waller, S., Whitall, J., Forrester, L. W., Macko, R., Sorkin, J. D., et al. (2004). Repetitive bilateral arm training and motor cortex activation in chronic stroke: a randomized controlled trial. JAMA 292, 1853–1861. doi: 10.1001/jama.292.15.1853
Ma, Y., and MacDonald, A. W III. (2021). Impact of independent component analysis dimensionality on the test-retest reliability of resting-state functional connectivity. Brain Connect. 11, 875–886. doi: 10.1089/brain.2020.0970
Makris, N., Goldstein, J. M., Kennedy, D., Hodge, S. M., Caviness, V. S., Faraone, S. V., et al. (2006). Decreased volume of left and total anterior insular lobule in schizophrenia. Schizophr. Res. 83, 155–171. doi: 10.1016/j.schres.2005.11.020
McKeown, M. J., Makeig, S., Brown, G. G., Jung, T. P., Kindermann, S. S., Bell, A. J., et al. (1998). Analysis of fMRI data by blind separation into independent spatial components. Hum. Brain Mapp. 6, 160–188.
Meindl, T., Teipel, S., Elmouden, R., Mueller, S., Koch, W., Dietrich, O., et al. (2010). Test-retest reproducibility of the default-mode network in healthy individuals. Hum. Brain Mapp. 31, 237–246. doi: 10.1002/hbm.20860
Mueller, S., Wang, D., Fox, M. D., Pan, R., Lu, J., Li, K., et al. (2015). Reliability correction for functional connectivity: theory and implementation. Hum. Brain Mapp. 36, 4664–4680. doi: 10.1002/hbm.22947
Pamilo, S., Malinen, S., Hlushchuk, Y., Seppä, M., Tikka, P., and Hari, R. (2012). Functional subdivision of group-ICA results of fMRI data collected during cinema viewing. PLoS ONE 7, e42000. doi: 10.1371/journal.pone.0042000
Pandit, A. S., Expert, P., Lambiotte, R., Bonnelle, V., Leech, R., Turkheimer, F. E., et al. (2013). Traumatic brain injury impairs small-world topology. Neurology 80, 1826–1833. doi: 10.1212/WNL.0b013e3182929f38
Park, C. H., Chang, W. H., Ohn, S. H., Kim, S. T., Bang, O. Y., Pascual-Leone, A., et al. (2011). Longitudinal changes of resting-state functional connectivity during motor recovery after stroke. Stroke 42, 1357–1362. doi: 10.1161/STROKEAHA.110.596155
Sasaki, N., Kakuda, W., and Abo, M. (2014). Bilateral high- and low-frequency rTMS in acute stroke patients with hemiparesis: a comparative study with unilateral high-frequency rTMS. Brain Inj. 28, 1682–1686. doi: 10.3109/02699052.2014.947626
Shirer, W. R., Ryali, S., Rykhlevskaia, E., Menon, V., and Greicius, M. D. (2012). Decoding subject-driven cognitive states with whole-brain connectivity patterns. Cereb. Cortex 22, 158–165. doi: 10.1093/cercor/bhr099
Shrout, P. E., and Fleiss, J. L. (1979). Intraclass correlations: uses in assessing rater reliability. Psychol. Bull. 86, 420–428. doi: 10.1037/0033-2909.86.2.420
Shumskaya, E., van Gerven, M. A., Norris, D. G., Vos, P. E., and Kessels, R. P. (2017). Abnormal connectivity in the sensorimotor network predicts attention deficits in traumatic brain injury. Exp. Brain Res. 235, 799–807. doi: 10.1007/s00221-016-4841-z
Smith, S. M., Fox, P. T., Miller, K. L., Glahn, D. C., Fox, P. M., Mackay, C. E., et al. (2009). Correspondence of the brain's functional architecture during activation and rest. Proc. Natl. Acad. Sci. USA. 106, 13040–13045. doi: 10.1073/pnas.0905267106
Smith, S. M., Jenkinson, M., Woolrich, M. W., Beckmann, C. F., Behrens, T. E., Johansen-Berg, H., et al. (2004). Advances in functional and structural MR image analysis and implementation as FSL. Neuroimage 23(Suppl. 1), S208–219. doi: 10.1016/j.neuroimage.2004.07.051
Solodkin, A., Hlustik, P., Chen, E. E., and Small, S. L. (2004). Fine modulation in network activation during motor execution and motor imagery. Cereb. Cortex 14, 1246–1255. doi: 10.1093/cercor/bhh086
Stevens, M. C., Kiehl, K. A., Pearlson, G. D., and Calhoun, V. D. (2009). Brain network dynamics during error commission. Hum. Brain Mapp. 30, 24–37. doi: 10.1002/hbm.20478
Swinnen, S. P., and Wenderoth, N. (2004). Two hands, one brain: cognitive neuroscience of bimanual skill. Trends Cogn. Sci. 8, 18–25. doi: 10.1016/j.tics.2003.10.017
Thomas, S. A., Christensen, R. E., Schettini, E., Saletin, J. M., Ruggieri, A. L., MacPherson, H. A., et al. (2019). Preliminary analysis of resting state functional connectivity in young adults with subtypes of bipolar disorder. J. Affect. Disord. 246, 716–726. doi: 10.1016/j.jad.2018.12.068
Tsuchimoto, S., Shindo, K., Hotta, F., Hanakawa, T., Liu, M., and Ushiba, J. (2019). Sensorimotor connectivity after motor exercise with neurofeedback in post-stroke patients with hemiplegia. Neuroscience 416, 109–125. doi: 10.1016/j.neuroscience.2019.07.037
Van de Ven, V. G., Formisano, E., Prvulovic, D., Roeder, C. H., and Linden, D. E. (2004). Functional connectivity as revealed by spatial independent component analysis of fMRI measurements during rest. Hum. Brain Mapp. 22, 165–178. doi: 10.1002/hbm.20022
Venkataraman, A., Zhuang, Y., Marsella, J., Tivarus, M. E., Qiu, X., Wang, L., et al. (2021). Functional MRI correlates of sleep quality in HIV. Nat. Sci. Sleep 13, 291–301. doi: 10.2147/NSS.S291544
Wang, C., Qin, W., Zhang, J., Tian, T., Li, Y., Meng, L., et al. (2014a). Altered functional organization within and between resting-state networks in chronic subcortical infarction. J. Cereb. Blood Flow Metab. 34, 597–605. doi: 10.1038/jcbfm.2013.238
Wang, J. X., Rogers, L. M., Gross, E. Z., Ryals, A. J., Dokucu, M. E., Brandstatt, K. L., et al. (2014b). Targeted enhancement of cortical-hippocampal brain networks and associative memory. Science 345, 1054–1057. doi: 10.1126/science.1252900
Wang, L., Yu, C., Chen, H., Qin, W., He, Y., Fan, F., et al. (2010). Dynamic functional reorganization of the motor execution network after stroke. Brain 133, 1224–1238. doi: 10.1093/brain/awq043
Wiesendanger, M., and Serrien, D. J. (2004). The quest to understand bimanual coordination. Prog. Brain Res. 143, 491–505. doi: 10.1016/S0079-6123(03)43046-X
Yan, C., Liu, D., He, Y., Zou, Q., Zhu, C., Zuo, X., et al. (2009). Spontaneous brain activity in the default mode network is sensitive to different resting-state conditions with limited cognitive load. PLoS ONE 4, e5743. doi: 10.1371/journal.pone.0005743
Yan, C. G., Wang, X. D., Zuo, X. N., and Zang, Y. F. (2016). DPABI: data processing and analysis for (resting-state) brain imaging. Neuroinformatics 14, 339–351. doi: 10.1007/s12021-016-9299-4
Ye, Z., Hammer, A., and Münte, T. F. (2017). Pramipexole modulates interregional connectivity within the sensorimotor network. Brain Connect. 7, 258–263. doi: 10.1089/brain.2017.0484
Yuan, L. X., Wang, J. B., Zhao, N., Li, Y. Y., Ma, Y., Liu, D. Q., et al. (2018). Intra- and Inter-scanner reliability of scaled subprofile model of principal component analysis on ALFF in resting-state fMRI under eyes open and closed conditions. Front. Neurosci. 12, 311. doi: 10.3389/fnins.2018.00311
Zhang, Y., Wang, L., Yang, J., Yan, R., Zhang, J., Sang, L., et al. (2017). Abnormal functional networks in resting-state of the sub-cortical chronic stroke patients with hemiplegia. Brain Res. 1663, 51–58. doi: 10.1016/j.brainres.2017.02.012
Zhao, N., Yuan, L. X., Jia, X. Z., Zhou, X. F., Deng, X. P., He, H. J., et al. (2018a). Intra- and inter-scanner reliability of voxel-wise whole-brain analytic metrics for resting state fMRI. Front. Neuroinform. 12, 54. doi: 10.3389/fninf.2018.00054
Zhao, Z., Wu, J., Fan, M., Yin, D., Tang, C., Gong, J., et al. (2018b). Altered intra- and inter-network functional coupling of resting-state networks associated with motor dysfunction in stroke. Hum. Brain Mapp. 39, 3388–3397. doi: 10.1002/hbm.24183
Zheng, X., Sun, L., Yin, D., Jia, J., Zhao, Z., Jiang, Y., et al. (2016). The plasticity of intrinsic functional connectivity patterns associated with rehabilitation intervention in chronic stroke patients. Neuroradiology 58, 417–427. doi: 10.1007/s00234-016-1647-4
Keywords: inter-scanner reliability, intra-scanner reliability, ICA, SMN, peak location
Citation: Hu Y-S, Yue J, Ge Q, Feng Z-J, Wang J and Zang Y-F (2022) Test-retest reliability of peak location in the sensorimotor network of resting state fMRI for potential rTMS targets. Front. Neuroinform. 16:882126. doi: 10.3389/fninf.2022.882126
Received: 23 February 2022; Accepted: 15 September 2022;
Published: 03 October 2022.
Edited by:
Ludovico Minati, Tokyo Institute of Technology, JapanReviewed by:
Lubin Wang, Beijing Institute of Basic Medical Sciences, ChinaCopyright © 2022 Hu, Yue, Ge, Feng, Wang and Zang. This is an open-access article distributed under the terms of the Creative Commons Attribution License (CC BY). The use, distribution or reproduction in other forums is permitted, provided the original author(s) and the copyright owner(s) are credited and that the original publication in this journal is cited, in accordance with accepted academic practice. No use, distribution or reproduction is permitted which does not comply with these terms.
*Correspondence: Jue Wang, anVlZmlyc3RAY2RzdS5lZHUuY24=; Yu-Feng Zang, emFuZ3lmQGh6bnUuZWR1LmNu
Disclaimer: All claims expressed in this article are solely those of the authors and do not necessarily represent those of their affiliated organizations, or those of the publisher, the editors and the reviewers. Any product that may be evaluated in this article or claim that may be made by its manufacturer is not guaranteed or endorsed by the publisher.
Research integrity at Frontiers
Learn more about the work of our research integrity team to safeguard the quality of each article we publish.