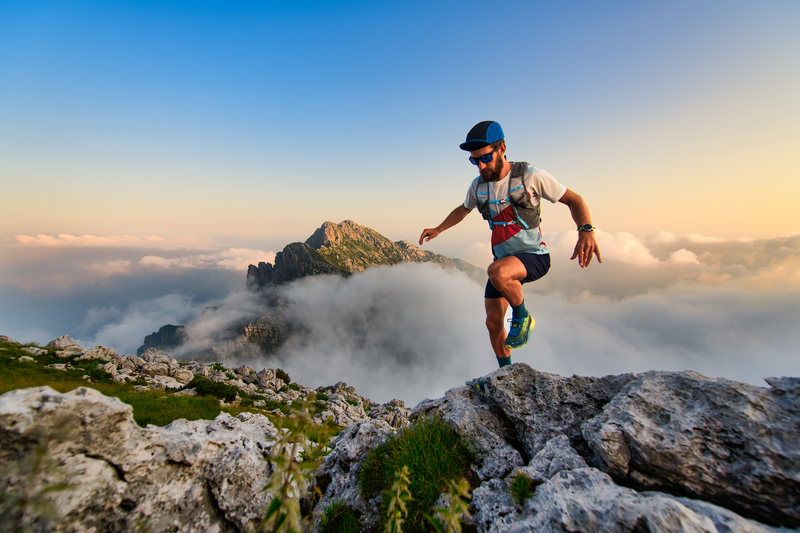
94% of researchers rate our articles as excellent or good
Learn more about the work of our research integrity team to safeguard the quality of each article we publish.
Find out more
ORIGINAL RESEARCH article
Front. Neuroinform. , 23 June 2022
Volume 16 - 2022 | https://doi.org/10.3389/fninf.2022.859309
This article is part of the Research Topic Mapping Brain Multiscale Dynamics in Health and Disease: Complex Brain Functions Need Scalable Advanced Large-scale Techniques, Modeling, and Theories View all 7 articles
Anxiety is a future-oriented unpleasant and negative mental state induced by distant and potential threats. It could be subdivided into momentary state anxiety and stable trait anxiety, which play a complex and combined role in our mental and physical health. However, no studies have systematically investigated whether these two different dimensions of anxiety share a common or distinct topological mechanism of human brain network. In this study, we used macroscale human brain morphological similarity network and functional connectivity network as well as their spatial and temporal variations to explore the topological properties of state and trait anxiety. Our results showed that state and trait anxiety were both negatively correlated with the coefficient of variation of nodal efficiency in the left frontal eyes field of volume network; state and trait anxiety were both positively correlated with the median and mode of pagerank centrality distribution in the right insula for both static and dynamic functional networks. In summary, our study confirmed that state and trait anxiety shared common human brain network topological mechanisms in the insula and the frontal eyes field, which were involved in preliminary cognitive processing stage of anxiety. Our study also demonstrated that the common brain network topological mechanisms had high spatiotemporal robustness and would enhance our understanding of human brain temporal and spatial organization.
Anxiety is a future-oriented emotional state activated by potential and distant threats (Eysenck et al., 2007; Calhoon and Tye, 2015). It is unpleasant, negative, out of proportion to the threat and can be characterized by aversive and unpleasant avoidance behaviors (Spielberger et al., 1983; Grachev and Apkarian, 2000; Eysenck et al., 2007; Modi et al., 2015). Anxiety is different from fear concerning its association with the anticipation of uncertain threats and can be more easily triggered by distant and unpredictable threats (Geng et al., 2018). Spielberger (1966) suggested that anxiety could be conceptualized into multiple dimensions by distinguishing trait anxiety from state anxiety. Specifically, trait anxiety, as a personality dimension, can be defined as an individual's predisposition to worry about future threating events (Spielberger et al., 1983), and individuals with high trait anxiety have weakened image processing on the conscious level, stronger induced sensitivity, and a tendency to overprocess relationships (Yin et al., 2016). While state anxiety reflects a temporary, transient, and subjective emotion characterized by physiological arousal and consciously perceived feelings of depression, tension, and apprehension (Spielberger et al., 1983; Endler and Kocovski, 2001). There were also some studies considering state anxiety as the state associated with the feeling of anxiety and considering trait anxiety as the frequency of anxiousness (Takagi et al., 2018). State anxiety and trait anxiety were correlated, but independent behavioral measurements that had different influences on attentional and cognitive control processes (Bishop et al., 2007; Crocker et al., 2012; Hur et al., 2015), and distinguishment between them was important for evaluating and monitoring individuals' levels of anxiety as well as developing effective means to prevent and treat anxiety-related disorders (Forrest et al., 2021). Although state and trait anxiety have independent behavioral definitions, it remained unclear whether they shared common or distinct brain network topological mechanisms.
In the neuroscience field, a large number of studies have investigated the neural underpinnings of state and trait anxiety, either common or distinct, using different experimental approaches and techniques. While most studies concluded that they had distinct neuroanatomical and functional substrates (Satpute et al., 2012; Tian et al., 2016; Saviola et al., 2020). In more detail, at the structural level, trait anxiety was associated with gray matter volume in the frontal cortex (Hu and Dolcos, 2017) and the occipital gyrus (Yin et al., 2016), as well as altered cortical thickness in the temporal cortex, the cingulate cortex, and the orbitofrontal cortex (Potvin et al., 2015); at the functional level, trait anxiety was related to regional homogeneity (ReHo) or amplitude of low-frequency fluctuations (ALFF) in the prefrontal cortex (PFC), cingulate cortex (Tian et al., 2016), orbitofrontal cortex (Xue et al., 2018), thalamus, and cerebellum (Yin et al., 2016), as well as functional connectivity (FC) in brain networks, including default mode network (DMN) (Modi et al., 2015; Saviola et al., 2020), ventral attention network (He et al., 2016), and temporo-parietal-frontal network (Modi et al., 2015; He et al., 2016). As for state anxiety, neuroimaging studies concerning structural gray matter volume or thickness are lacking (Saviola et al., 2020) and functional substrates in state anxiety mainly involved limbic regions such as the insula (Tian et al., 2016) and hippocampus (Satpute et al., 2012) in different anxiety provocation tasks. However, there were also several studies showing that there was an interaction and association between state and trait anxiety (Mathews, 1990; Williams et al., 1996). From a systematical perspective, Takagi et al. (2018) found a common brain network among state and trait anxiety in a unified analytical framework. Meanwhile, different experimental approaches revealed that state and trait anxiety were correlated with the same brain regions such as the PFC (Mataix-Cols et al., 2003; Tian et al., 2016), occipital gyrus (Yin et al., 2016; Li et al., 2020), as well as parietal-frontal network (Modi et al., 2015; He et al., 2016; Li et al., 2020). In summary, although previous studies demonstrated that state and trait anxiety shared common or distinct neural substrates, few studies provided a convincing conclusion from multiple spatial and temporal scales, as well as in both brain structure and brain function (Saviola et al., 2020).
Complex network analysis could be used to explore human brain network topological properties and enhance our comprehension on human brain network architecture and their associations with behaviors (Bullmore and Sporns, 2009; Sporns, 2011). Brain functional network construction was mainly based on FC (temporal correlation of brain activity) between brain regions (nodes) (Bullmore and Sporns, 2009) using electrophysiological or functional imaging techniques. Previous studies mostly calculated static FC that utilized all the time points (Salvador et al., 2005; He et al., 2016; Saviola et al., 2020). As temporal variability in fMRI signals has already been detected across a typical course of a single scan (Chang and Glover, 2010; Kang et al., 2011; Hutchison et al., 2013a), BOLD signal correlations would definitely show dynamic changes over time scales of seconds to minutes (Chang and Glover, 2010). Also, this dynamical nature of FC reflected a basic property of complex systems such as human brain (Liu and Duyn, 2013; Liao et al., 2015). Therefore, in this study, we would combine static and dynamic FC to construct brain functional network. In contrast, existing methods to construct brain structural network are mainly white matter tractography (Li et al., 2009; Koenis et al., 2018) and structural covariance network (Mechelli et al., 2005; Alexander-Bloch et al., 2013). As stated in our previous study (Li et al., 2021), the white matter tractography could not reliably quantify long-range structural connectivity (Jeurissen et al., 2019) and was largely affected by head motion and might involve a large number of false-positive connections (Thomas et al., 2014; Maier-Hein et al., 2017); the structural covariance network from a large number of participants only yielded one single correlation matrix but did not reveal individual differences (Alexander-Bloch et al., 2013; Li et al., 2021). Therefore, in this study we still used macroscale morphology network that was based on distributions of cortical surface characteristics (cortical volume, thickness, and surface area) from resting-state fMRI (Li et al., 2021) in order to explore the neural substrates of state and trait anxiety. Corresponding to the temporal variations of human brain functional network, we changed bin number of frequency distributions of cortical surface characteristics (area, thickness, and volume) to explore the spatial variations of brain structural network. We expected that topological properties of brain structural and functional networks, as well as their spatial and temporal variations, would efficiently distinguish state anxiety from trait anxiety.
Network topology means the full connection details of a network, that is, human brain connectome (Bullmore and Sporns, 2009; Rubinov and Sporns, 2010). Centrality and efficiency are two commonly used topological measures in human brain network studies. Centrality assesses the importance of a brain region (node) in facilitating functional integration and interaction across the entire network architecture (Rubinov and Sporns, 2010; Zuo et al., 2012). As for efficiency, Latora and Marchiori (2001) demonstrated that network efficiency could be used to measure the information flow within human brain network: global efficiency corresponds to long-distance interactions and reflects information integration over the whole network; nodal efficiency reflects information transfer ability of the parcel (node); whereas local efficiency reflects specialization of a single brain region (node) within the network (Latora and Marchiori, 2001; Rubinov and Sporns, 2010; Bullmore and Sporns, 2012). Global efficiency, nodal efficiency, as well as betweenness centrality, eigenvector centrality, and pagerank centrality characterized functional integration across the network architecture, while local efficiency and degree centrality focus on the information flow and transfer in local brain regions and functional segregation (Rubinov and Sporns, 2010). These topological properties have been used to successfully detect the neural basis of various behaviors and diseases (Zuo et al., 2012; Guo et al., 2016; Weiler et al., 2018; Li et al., 2021), did state and trait anxiety share a common or distinct brain network topological mechanism in both functional integration and segregation perspectives?
To determine whether state anxiety and trait anxiety shared a common or distinct topological mechanism of human brain structural and functional networks, we recruited 67 healthy participants who finished structural and resting-state fMRI scanning, followed by the assessments of state and trait anxiety. We respectively, used morphological similarity and FC method to construct human brain structural and functional networks and aimed to elucidate the topological mechanisms of state and trait anxiety from both the single-network perspective and their spatial and temporal variations perspective (Allen et al., 2014). Our study confirmed that state and trait anxiety shared common human brain network topological mechanisms in the insula and the frontal eyes field, which were involved in preliminary cognitive processing stage of anxiety. Our study also demonstrated that the common brain network topological mechanisms had high spatiotemporal robustness and would enhance our understanding of human brain temporal and spatial organization.
Participants were recruited from local community or universities by advertisements, and the initial sample included 67 datasets (32 males and 35 females; mean age = 32.79 ± 13.11; ranged from 18.59 to 64.30). All the participants underwent a detailed mental health interview by two trained psychologists using the Mini-International Neuro-Psychiatric Interview. People with a history of major neuropsychiatric illness, head injury, and alcohol and drug abuse were excluded. They were also assessed with Wechsler Adult Intelligence Scale-4th Edition (in Chinese, WAIS-IV), Schutte Self-Report Emotional Intelligence scale in Chinese Version (SSEIS), State-Trait Anxiety Inventory, Mental Health Continuum-Short Form, Emotion Regulation Questionnaire, Chinese Perceived Stress Scale, Achievement Motivation Scale, and Self-Control Scale. The institutional review board of Institute of Psychology Chinese Academy of Sciences approved this study, and written informed consent was obtained from individual participant prior to data acquisition.
The State-Trait Anxiety Inventory (STAI) (Spielberger et al., 1983) was applied to measure participants' state and trait anxiety. The scale has a total of 40 descriptive questions related to anxiety. Items 1–20 are the State Anxiety Scale (S-Al), 10 of which describe negative emotions and 10 of which describe positive emotions, which are used to assess the feelings of people at a specific and particular moment. Items 21–40 are the Trait Anxiety Inventory (T-AI), 11 of which describe negative emotions and 9 of which describe positive emotions, which are used to assess people's habitual anxiety experience and capture the dimensions of personality linked to anxiety. Each item uses a 4-point scoring method. The higher scores indicate the higher degree of anxiety. Previous studies have verified that the Chinese version of the STAI had acceptable construct validity that supported Spielberger's conception of the multidimensional nature of the S-AI and T-AI scales (Li and Lopez, 2004; Wei et al., 2015) and could be used to measure state and trait anxiety of Chinese participants. Cronbach's α coefficient for internal consistency in our sample was acceptable (state anxiety α = 0.757, trait anxiety α = 0.717).
MRI images were collected on the 3.0 T GE scanner Discovery MR750 at the Institute of Psychology Chinese Academy of Sciences. All the participants completed a T1-weighted structural MRI scan (eyes closed) with a ABI1_t1iso_fspgr sequence (TR = 6.652 ms; TE = 2.928 ms; FA = 12°; matrix = 256 × 256; slice thickness = 1 mm) and an 8-min resting-state fMRI scan (eyes open with a fixation cross) using a gradient echo EPI sequence ABI1_bold_bw_rest [TR = 2,000 ms; TE = 30 ms; FA = 90°; number of slices = 33 (interleaved); slice thickness = 3.5 mm; gap = 0.7 mm; and matrix = 64 × 64].
MRI images were preprocessed using the Connectome Computation System (http://github.com/zuoxinian/CCS) developed by our laboratory (Xu et al., 2015), which integrated several neuroimaging-related software including AFNI (Cox, 2012), FSL (Jenkinson et al., 2012), and FreeSurfer (Fischl, 2012), as well as in-house MATLAB scripts. The full pipeline of preprocessing included structural image preprocessing, functional image preprocessing, as well as quality control (Zuo et al., 2013; Xu et al., 2015). The structural preprocessing included (1) intensity inhomogeneity correction; (2) brain extraction; (3) tissue segmentation; (4) white and pial surface generation; and (5) deformation estimation between the resulting spherical mesh and a common spherical coordinate system. The functional preprocessing was the same as our previous publications (Jiang et al., 2015; Zhang et al., 2017) and included (1) excluding the first five volumes from each scan; (2) removing and interpolating of temporal spikes; (3) slice timing correction and motion correction; (4) extracting functional brain; (5) normalizing 4D global mean of image intensity; and (6) co-registration between functional and anatomical images by employing a boundary-based registration (BBR) algorithm.
Following the preprocessed individual MRI images, the CCS also provided a quality control procedure (QCP) for both functional and structural images. The QCP includes the following steps (Zuo et al., 2013): (1) brain extraction or skull stripping; (2) brain tissue segmentation; (3) pial and white surface reconstruction; (4) BBR-based functional image registration; and (5) head motion during resting-state MRI. The pipeline also computed the mean frame-wise displacement (meanFD) (Power et al., 2012) and the minimal cost of the BBR co-registration (mcBBR) for the subsequent statistical tests as covariates. All participants with bad brain extraction, bad tissue segmentation, and bad surface construction will be excluded from the subsequent analysis. One participant did not complete MRI scanning, and one participant did not pass the mental health interview. Five participants were excluded because their mcBBR was >0.65. Therefore, we had 60 participants for final group analysis. The detailed participant information and intervariable correlations are shown in Table 1.
Here, we used a macroscale brain network parcellation developed by Yeo et al. (2011) as nodes to construct human brain structural and functional network, which subdivided the entire cortical surface into 51 spatially connected parcels based on resting-state FC. We excluded the parcels whose vertex number was <50, and finally 30 parcels were reserved for final group analysis, which expanded across all the Yeo-7 networks, including visual network, somatomotor network, dorsal attention network, ventral attention network, limbic network, frontoparietal (control) network, and DMN (Table 2).
We have proposed a new method to construct structural network, including cortical volume, surface area, and cortical thickness, in our previous study (Li et al., 2021), which assessed the distribution similarity of each morphological measurement for each pair of parcels (Figure 1A), consider the cortical volume as an example). First, for each pair of parcels, we uniformly divided their volumes into 30 bins. Second, we computed the vertex frequency for each bin of the parcels, so that we got the frequency distribution histogram for each parcel. Third, the Pearson correlation coefficient of the frequency distribution histograms was calculated to estimate the volume distribution similarity, and we got a 30 → 30 morphological correlation matrix for each participant. Considering the effects of bin number on network architecture, we traversed the bin number from 24 to 36 shifting with a step size of 2 bins to get 7 structural networks to assess the spatial variations of human brain structural network.
Figure 1. The workflow for the construction and thresholding of morphological (structural) and functional network. (A) For each pair of parcels such as the left SomMot and the left DorsAttn_Post of right hemisphere, we uniformly divided their volumes into 30 bins (5.4–61.2). Each bin will have the width of (61.2–5.4)/30 = 1.68, and the frequency distribution histograms are presented in the upper. By computing the Pearson's correlation of two sets of frequencies, we got the similarity of these two parcels. Then, we changed the bin number from 24 to 36 with a step of 2 bins to get 7 structural networks. (B) For each pair of parcels such as the left SomMot and the left DorsAttn_Post of right hemisphere, we used the sliding window method and segmented the total 235 time points of each parcel into windows of 50 TRs (100 s) shifting with a step size of 1 TR to obtain 186 functional networks. And then we showed the thresholding schemes of the network: we first got absolute value of each connection in the network and then applied the data-driven thresholding scheme based on orthogonal minimal spanning trees (OMST) to get the strongest and the most important connections. Finally, we calculated the topological measures based on the connection networks to explore the correlations between anxiety and human brain topological properties.
For each parcel of cortical surface, we calculated an average time sequence (235 time points), and then we computed the Pearson correlation coefficient of time series on each pair of parcels to get a 30 → 30 correlation matrix for each participant. The sliding window approach (Allen et al., 2014) was then used to calculate the dynamic FC between each pair of parcels to assess the temporal variations of human brain functional network (see Figure 1B). A total of 50 TRs were selected as the window length, on the one hand, which covered the low-frequency band of interest (0.01–0.1 Hz) with an adequate number of time points (at least one period) (Liao et al., 2015), on the other hand, which optimized the balance between the specificity (long enough to detect convincing dynamic fluctuations) and sensitivity (short enough to permit real dynamic variations) of dynamic functional connections (He et al., 2018; Li et al., 2018; Guo et al., 2020). Finally, the total 235 time points of each parcel were segmented into windows of 50 TRs (100 s) shifting with a step size of 1 TR, and we obtained 186 functional networks. We also have tried other window lengths (60 and 70 TRs), and our conclusions were not changed.
After constructing brain structural and functional networks, we used orthogonal minimal spanning trees [OMST, (Dimitriadis et al., 2017)], which was a threshold-free method to extract the strongest and the most important connections of a network, to get an undirected weighted graph. Then, we computed topological properties of human brain network, network efficiency and centrality, and the whole pipeline of data analysis is shown in Figure 1.
Network topology means the full details of network connections, and here we mainly considered two types of topological measurements, namely, network efficiency and centrality. In more detail, we applied graph theory (Achard et al., 2006) to compute network efficiency, including global efficiency (Eglob), nodal efficiency (Enodal), and local efficiency (Elocal), as well as network centrality, including degree centrality (DC), betweenness centrality (BC), eigenvector centrality (EC), and pagerank centrality (PC), and the specific calculation tools included the Brain Connectivity Toolbox (http://www.brain-connectivity-toolbox.net) (Rubinov and Sporns 2010) and the Connectome Computation System (http://github.com/zuoxinian/CCS) scripts (Xu et al., 2015).
Global efficiency for a network G is defined as follows:
where N is the number of nodes (brain regions) and Lijis the shortest path length between node i and node j in network G (Latora and Marchiori, 2003). Global efficiency refers to the overall information transfer efficiency between any two nodes (brain regions) in the whole brain, which is a long-distance information transmission in the network and reflects a global measure of the information transmission efficiency of the whole network (Latora and Marchiori, 2003).
Nodal efficiency of node i is defined as follows:
where N and Lij are the same as that in Equation (1). The nodal efficiency represents the importance of the node for information transfer in the network, and the global efficiency is the average of the node efficiency of all nodes (brain regions) in the whole brain.
Local efficiency of node i is defined as follows:
where Gi is a subgraph and composed of the nodes that connect to node i (not including node i) directly and interconnected edges. The average of the reciprocals of the shortest paths between any two nodes within the subgraph is the local efficiency. Local efficiency indicates the information transfer ability in the given subgraph, and more densely clustered connections between topological neighbors indicated higher local efficiency (Latora and Marchiori, 2003; Bullmore and Sporns, 2012).
Degree centrality of node i is defined as follows:
where aij is the connection status between i and j: aij = 1 when i and j are connected and aij= 0 when i and j are not connected. DC is the number of links connected to a node and represents the most local and directly quantifiable centrality measure, and higher DC of the node indicates more important role in the network (Rubinov and Sporns, 2010; Zuo et al., 2012).
Betweenness centrality of node i is defined as follows:
where Lhj is the number of shortest paths between node h and node j, and Lhj(i) is the number of shortest paths between h and j that pass through node i. BC represents the fraction of all shortest paths in the network that pass through a given node. Important and bridging nodes that connect disparate parts of the network exhibited a high BC (Freeman, 1979; Rubinov and Sporns, 2010).
Eigenvector centrality of node i is defined as follows:
where μj(i) is the ith component of the jth eigenvector of the adjacency matrix aij, and λ1 is the corresponding jth eigenvalue. N is the number of nodes, and aij is the association matrix. Nodes have high EC if they connect to other nodes that have high EC, which means higher EC scores indicate a more central and important role of the node in the network (Bonacich, 1972; van Duinkerken et al., 2017).
Pagerank centrality of node i is defined as follows:
The pagerank measure was introduced originally by Google to rank web pages. In the graph theory, Pagerank represents the “importance” of nodes assuming that the importance of a node is the expected sum of the importance of all connected nodes and the direction of edges (Gleich, 2015; Henni et al., 2018). The Google pagerank centrality algorithm is a variant of EC, which introduces a small probability (1–d = 0.15, d is damping factor) of random damping to handle walking traps on a graph (Boldi et al., 2009).
Besides the above topological properties themselves, we also calculated the coefficient of variation (CV) of the topological properties to explore the temporal and spatial variations underlying state and trait anxiety. Meanwhile, we divided the value of topological measures in 186 functional brain networks into frequency distribution histograms whose bin number was 20 and calculated medians, modes, and half width at half maxima (FWHMs) of the histograms, as well as medians, modes, and FWHMs of the histogram-fitted curves.
To investigate topological mechanisms of human brain structural and functional networks underlying state and trait anxiety, we used general linear model to calculate the partial correlations between topological properties (E, efficiency; C, centrality; CV, coefficient of variation) and anxiety. In more detail, for structural network, we considered age, gender, education, intracranial volume (ICV), and total volume for volume network (total area for area network or mean thickness for thickness network) as covariates. The detailed statistical model is shown in the following equation:
For functional network, we considered age, gender, education, mcBBR, and meanFD as covariates. The detailed statistical model is shown in the following equation:
False discovery rate (FDR, q < 0.05) correction for 30 parcels was used to control type 1 error over multiple tests, and all the statistical analyses were performed using MATLAB scripts in this study.
Table 1 presents detailed information about state-anxiety and trait-anxiety for the entire group of participants, including their average and standard deviation. There was no significant correlation between anxiety and demographic variables such as age and education. However, state and trait anxiety were significantly correlated, which demonstrated that the two dimensions of anxiety might share some common components.
We used morphological similarity network (bin number = 30) of cortical volume, surface area, cortical thickness, and FC network (time point = 235) to explore whether state anxiety and trait anxiety had common or distinct topological mechanisms. For functional network, we found state anxiety (r = 0.5509, corrected p = 0.0004) and trait anxiety (r = 0.4505, corrected p = 0.0168) were both significantly positively correlated with pagerank centrality in the RH_SalVentAttn_FrOper (Figures 2A,B), which mapped to the opercular part of the inferior frontal gyrus, and the inferior and superior of the insula (Destrieux et al., 2010).
Figure 2. Brain regions with significant correlations between topological properties of human brain network and anxiety. Pagerank centrality in the RH_SalVentAttn_FrOper (the opercular part of the inferior frontal gyrus, the inferior and superior insula of right hemisphere) of human brain functional networks was not only positively correlated with state anxiety [(A) r = 0.5509, corrected p = 0.0004], but also was positively correlated with trait anxiety [(B) r = 0.4505, corrected p = 0.0168].
Using different spatial scales during the construction of human brain morphological similarity network, we got spatial variations of human brain structural network. Using sliding-window method, we got temporal variations of human brain functional network. The detailed topological properties for different spatial scales and different time slots are illustrated in Supplementary Figure 1.
For human brain structural network at different spatial scales, we found that the CV of degree centrality was negatively correlated with trait anxiety in the LH_Default_PFC (corresponding to PFC) (r = −0.4163, corrected p = 0.0471), and CV of nodal efficiency was significantly negatively correlated with both state anxiety (r = −0.4175, corrected p = 0.0455) and trait anxiety (r = −0.4186, corrected p = 0.0441) in the LH_DorsAttn_FEF (corresponding to frontal eyes field) of volume network (Figure 3). There were no significant results in other morphological networks.
Figure 3. The partial correlations between the spatial variations of human brain structural networks and anxiety: CV of degree centrality in the LH_Default_PFC (the PFC of left hemisphere) of volume network with trait anxiety [(A) r = −0.4163, corrected p = 0.0471]; CV of nodal efficiency in the LH_DorsAttn_FEF (the frontal eyes field of left hemisphere) of volume network with trait anxiety [(B) r = −0.4186, corrected p = 0.0441]; and CV of nodal efficiency in the LH_DorsAttn_FEF of volume network with state anxiety [(C) r = −0.4175, corrected p = 0.0455].
Using dynamic functional networks acquired from sliding window, we found both state and trait anxiety were positively correlated with median of pagerank centrality (state anxiety: r = 0.5121, corrected p = 0.0019; trait anxiety: r = 0.4674, corrected p = 0.0096) and median of degree centrality (state anxiety: r = 0.447, corrected p = 0.0187; trait anxiety: r = 0.4406, corrected p = 0.0228) in the RH_SalVentAttn_FrOper (Figure 4). We also found both state and trait anxiety were positively correlated with median of betweenness centrality (state anxiety: r = 0.3991, corrected p = 0.0381; trait anxiety: r = 0.4322, corrected p = 0.0295) and mode of degree centrality (state anxiety: r = 0.4289, corrected p = 0.0326; trait anxiety: r = 0.4308, corrected p = 0.0308) in the RH_SalVentAttn_FrOper (Supplementary Figure 3); trait anxiety was positively associated with median (r = 0.3991, corrected p = 0.0381) and mode (r = 0.4265, corrected p = 0.0325) of pagerank centrality in the LH_SalVentAttn_FrOper, median (r = 0.4104, corrected p = 0.0381) and FWHM (r = 0.4242, corrected p = 0.0360) of betweenness centrality in the LH_SalVentAttn_FrOper, and mode (r = 0.4909, corrected p = 0.0325) of pagerank centrality in the RH_SalVentAttn_FrOper (Supplementary Figure 2). Besides the above parcel-wise network, we also examined vertex-wise functional network and got similar results as shown in Supplementary Table 1. We also tried group comparisons between high-anxiety and low-anxiety groups, and found that both state and trait anxiety were associated with global efficiency of area similarity network (Supplementary Tables 2–8).
Figure 4. The partial correlations between the temporal variations of topological measures of human brain functional networks and anxiety: median of pagerank centrality in the RH_SalVentAttn_FrOper (the opercular part of the inferior frontal gyrus, the inferior and superior insula of right hemisphere) with state anxiety [(A) r = 0.5121, corrected p = 0.0019]; median of degree centrality in the RH_SalVentAttn_FrOper with state anxiety [(B) r = 0.447, corrected p = 0.0187]; median of pagerank centrality in the RH_SalVentAttn_FrOper with trait anxiety [(C) r = 0.4674, corrected p = 0.0096]; and median of degree centrality in the RH_SalVentAttn_FrOper with trait anxiety [(D) r = 0.4406, corrected p = 0.0228].
To examine whether state and trait anxiety share common or distinct neural substrates, we conducted systematical studies on the associations of anxiety with topological properties of human brain network, including morphological similarity network and its spatial variations, as well as FC network and its temporal variations, from both parcel-wise and vertex-wise perspectives. Our results showed that (1) in the static functional network, state and trait anxiety were both positively correlated with pagerank centrality in the right insula; (2) in the dynamic functional network, state and trait anxiety were both positively correlated with median and mode of pagerank and degree centrality in the right insula; and (3) state and trait anxiety were both negatively correlated with CV of nodal efficiency in the left frontal eyes field of volume network. In summary, our results demonstrated that there might be a common neural and topological mechanism between state and trait anxiety in different network conditions.
At the human brain functional level, we found that pagerank centrality of the anterior insula, which mostly occupied the right frontal operculum (RH_SalVentAttn_FrOper), was significantly positively correlated with state and trait anxiety in both static and dynamic functional networks. This was in line with previous studies indicating the activations of the insula in healthy participants' anxiety provocation tasks (Tian et al., 2016; Geng et al., 2018; Dammann et al., 2020). The insula has been proved to play an important role in subjective emotional processing and internal body awareness (Critchley et al., 2004; Craig, 2009; Singer et al., 2009; Tranel et al., 2009; Ernst et al., 2013), anticipation of future uncertain threat (Geng et al., 2018) and unpleasant negative stimuli (Herwig et al., 2007; Simmons et al., 2011; Lutz et al., 2013), risk and uncertainty in decision-making (Grinband et al., 2006; Clark et al., 2008), as well as visual and auditory awareness (Bushara et al., 2001; Kondo and Kashino, 2007). The positive correlations between topological properties of the insula and anxiety indicated that healthy participants with higher state and trait anxiety might be prone to pay more attention and awareness to internal body feelings and anticipate the risky and uncertain future events or threats due to the activations of the insula. Meanwhile, the role of the insula was also reflected in anxiety disorders, including social anxiety disorder (Duval et al., 2018; Atmaca et al., 2021) and generalized anxiety disorder (Shah et al., 2009; Cui et al., 2020), where they found more interoceptive body awareness, deficits in attentional control and emotion modulation, as well as hyperactive to general emotional images in patients compared to healthy controls. All these studies together demonstrated the connections between the insula and anxiety.
While at the human brain structural level, we found that spatial variations (CV) of nodal efficiency in the left frontal eyes field (FEF) was negatively correlated with state and trait anxiety, which reflected that decreased information transfer ability and efficiency of the FEF contributed to higher state and trait anxiety. The FEF, located in the superior precentral sulcus of the superior frontal sulcus corresponding to Brodmann's area 6 (Vernet et al., 2014), was not only involved in preparing and triggering various eye movements (Nyffeler et al., 2004; Nagel et al., 2008; Yang and Kapoula, 2011; Jaun-Frutiger et al., 2013), but also important in cognitive processes, including attentional orienting (Muggleton et al., 2003; Grosbras et al., 2005), visual awareness (Smith et al., 2005; Quentin et al., 2013), and perceptual modulation (Thompson et al., 1996). The negative correlations indicated participants with high state and trait anxiety had weakened ability and efficiency of attention control as well as perceptual modulation, and might show a tendency to increase more attention to unpleasant threats and stimuli, which was consistent with the attention control theory (Eysenck et al., 2007).
In this study, we conducted systematical studies to confirm that state and trait anxiety shared common brain network topological mechanisms. We found topological properties of the insula and the FEF were both correlated with state and trait anxiety, respectively, at human brain functional and structural level, which suggested that healthy participants who tended to feel anxious in daily life (trait anxiety) might share the same brain network topological patterns during anxious events and threats evocation (state anxiety). Previous studies have demonstrated that there was an interaction and association between state anxiety and trait anxiety (Mathews, 1990; Williams et al., 1996). More importantly, Takagi et al. (2018) have directly demonstrated that trait and state anxiety shared the same brain network and had a substantial biological interrelationship in a unified analytical framework. They hypothesized that participants with high trait anxiety would become increasingly anxious when facing unanticipated anxiety-related events and further enhance their levels of state anxiety. In contrast, participants with low trait anxiety would show a resistant and defensive response to the threats to reduce their levels of state anxiety. In more detail, the insula and the FEF were both involved in preliminary cognitive processing stage awareness (whether external or internal; visual or auditory), which indicated that state and trait anxiety shared the same brain topology mechanisms in alarming and sensing anxiety-related signals. Meanwhile, we also found that spatial variations (CV) of degree centrality in the PFC were only negatively correlated with trait anxiety but not state anxiety. The PFC generally acts as a role in emotion regulation and generation (Dixon et al., 2017), as well as integrates internal and external stimuli, to influence behavioral reactions (Bickart et al., 2012; Lindquist et al., 2012). We inferred that state and trait anxiety might use different human brain network topological mechanisms in the subsequent processes of coping with anxiety-related threats or events.
To the best of our knowledge, we are the first to not only explore the topological properties underlying state and trait anxiety using MRI technique but also verify that the common topological properties of human brain structural and functional networks underlying state and trait anxiety had temporospatial robustness. Human brain is one of the most complicated systems in the world, and it must coordinate complicated information at different spatial and temporal scales. Based on temporal correlations of BOLD time series, functional network could be used to detect human brain functional organization at different temporal scales (Friston, 2011; Allen et al., 2014; Calhoun Vince et al., 2014). Traditional FC method employed correlation coefficient of the time series of the entire scan across brain regions without capturing the temporal variations, while dynamic FC could quantify temporal alterations in FC metrics across multiple temporal scales (Hutchison et al., 2013b). Dynamic FC was proved to be a sensitive and specific marker of mental illness (Calhoun Vince et al., 2014; Yao et al., 2017). In more detail, previous studies have applied dynamic FC to various diseases such as generalized anxiety disorder (Yao et al., 2017; Chen et al., 2020), schizophrenia (Damaraju et al., 2014; Du et al., 2017), and bipolar disorder (Rashid et al., 2016; Nguyen et al., 2017). All these validated that dynamic FC was useful and efficient in characterizing human brain functional organization at different temporal scales. Corresponding to functional network, we also changed bin number (spatial scales to measure morphological distribution) and gained different morphological similarity resolutions to investigate spatial variations of human brain structural network underlying state and trait anxiety. Previous studies have demonstrated that morphological similarity might be the most accurate and robust method to reflect information transfer between brain regions (Seidlitz et al., 2018; Li et al., 2021), and different spatial resolutions of measuring morphological similarity could detect spatial robustness of human brain structural network underlying state and trait anxiety. This was the first study to examine spatial robustness of human brain networks and characterized the dependence of topological mechanisms of anxiety on spatial resolution. The above temporal and spatial robustness studies would enhance our understanding of temporal and spatial organization of human brain networks. In summary, our study systematically examined the topological mechanisms of state and trait anxiety from both structural and functional networks perspectives, as well as from their spatial and temporal variations perspectives. Our results indicated that state and trait anxiety shared common brain network topology mechanisms, and the common topological mechanisms exhibited high temporospatial robustness.
In conclusion, we explored topological properties underlying state and trait anxiety based on human brain structural and functional network, as well as their spatial and temporal variations. We found state and trait anxiety were both positively correlated with pagerank centrality in the right insula, median and mode of pagerank, and degree centrality in the right insula and negatively correlated with CV of nodal efficiency in the left frontal eyes field of volume network. Our results demonstrated that state and trait anxiety shared common brain network topological mechanisms with high temporospatial robustness and would enhance our understanding of spatial and temporal organization of human brain.
Several limitations should be taken into consideration for this study. First, our sample size was small but across a large age span, and we need to increase the number of participants in future studies. Second, our study only analyzed human brain information of cerebral cortex but did not involve subcortical data. Anxiety as a negative emotional state, subcortical information such as amygdala, hippocampus, and caudate should be considered in future explorations. Finally, we only conducted inter-regional correlations and intra-regional vertex-wise analysis, and whole-brain vertex-wise analysis required immense memory storage or efficient computational capacity.
The raw data supporting the conclusions of this article will be made available upon request to the corresponding author.
The studies involving human participants were reviewed and approved by the Institutional Review Board of Institute of Psychology Chinese Academy of Sciences. The patients/participants provided their written informed consent to participate in this study.
LJ: conceptualization and methodology. YL and LJ: formal analysis, investigation, and writing and editing. All authors contributed to the article and approved the submitted version.
This study was supported by the National Natural Science Foundation of China (11674388).
The authors declare that the research was conducted in the absence of any commercial or financial relationships that could be construed as a potential conflict of interest.
All claims expressed in this article are solely those of the authors and do not necessarily represent those of their affiliated organizations, or those of the publisher, the editors and the reviewers. Any product that may be evaluated in this article, or claim that may be made by its manufacturer, is not guaranteed or endorsed by the publisher.
The Supplementary Material for this article can be found online at: https://www.frontiersin.org/articles/10.3389/fninf.2022.859309/full#supplementary-material
Achard, S., Salvador, R., Whitcher, B., Suckling, J., and Bullmore, E. (2006). A resilient, low-frequency, small-world human brain functional network with highly connected association cortical hubs. J. Neurosci. 26, 63–72. doi: 10.1523/JNEUROSCI.3874-05.2006
Alexander-Bloch, A. F., Vértes, P. E., Stidd, R., Lalonde, F., Clasen, L., Rapoport, J., et al. (2013). The anatomical distance of functional connections predicts brain network topology in health and schizophrenia. Cereb. Cortex 23, 127–138. doi: 10.1093/cercor/bhr388
Allen, E. A., Damaraju, E., Plis, S. M., Erhardt, E. B., Eichele, T., and Calhoun, V. D. (2014). Tracking whole-brain connectivity dynamics in the resting state. Cereb. Cortex 24, 663–676. doi: 10.1093/cercor/bhs352
Atmaca, M., Koc, M., Mermi, O., Korkmaz, S., Aslan, S., and Yildirim, H. (2021). Insula volumes are altered in patients with social anxiety disorder. Behav. Brain Res. 400, 113012–113012. doi: 10.1016/j.bbr.2020.113012
Bickart, K. C., Hollenbeck, M. C., Barrett, L. F., and Dickerson, B. C. (2012). Intrinsic amygdala-cortical functional connectivity predicts social network size in humans. J. Neurosci. 32, 14729–14741. doi: 10.1523/JNEUROSCI.1599-12.2012
Bishop, S. J., Jenkins, R., and Lawrence, A. D. (2007). Neural processing of fearful faces: effects of anxiety are gated by perceptual capacity limitations. Cereb. Cortex 17, 1595–1603. doi: 10.1093/cercor/bhl070
Boldi, P., Santini, M., and Vigna, S. (2009). PageRank: functional dependencies. ACM Trans. Inform. Syst. 27, 1–23. doi: 10.1145/1629096.1629097
Bonacich, P. (1972). Factoring and weighting approaches to status scores and clique identification. J. Math. Sociol. 2, 113–120. doi: 10.1080/0022250X.1972.9989806
Bullmore, E., and Sporns, O. (2009). Complex brain networks: graph theoretical analysis of structural and functional systems. Nat. Rev. Neurosci. 10, 186–198. doi: 10.1038/nrn2575
Bullmore, E., and Sporns, O. (2012). The economy of brain network organization. Nat. Rev. Neurosci. 13, 336–349. doi: 10.1038/nrn3214
Bushara, K. O., Grafman, J., and Hallett, M. (2001). Neural correlates of auditory-visual stimulus onset asynchrony detection. J. Neurosci. 21, 300–304. doi: 10.1523/JNEUROSCI.21-01-00300.2001
Calhoon, G. G., and Tye, K. M. (2015). Resolving the neural circuits of anxiety. Nat. Neurosci. 18, 1394–1404. doi: 10.1038/nn.4101
Calhoun Vince, D., Miller, R., Pearlson, G., and Adal,i, T. (2014). The chronnectome: time-varying connectivity networks as the next frontier in fMRI data discovery. Neuron 84, 262–274. doi: 10.1016/j.neuron.2014.10.015
Chang, C., and Glover, G. H. (2010). Time-frequency dynamics of resting-state brain connectivity measured with fMRI. Neuroimage 50, 81–98. doi: 10.1016/j.neuroimage.2009.12.011
Chen, Y., Cui, Q., Xie, A., Pang, Y., Sheng, W., Tang, Q., et al. (2020). Abnormal dynamic functional connectivity density in patients with generalized anxiety disorder. J. Affect. Disord. 261, 49–57. doi: 10.1016/j.jad.2019.09.084
Clark, L., Bechara, A., Damasio, H., Aitken, M. R. F., Sahakian, B. J., and Robbins, T. W. (2008). Differential effects of insular and ventromedial prefrontal cortex lesions on risky decision-making. Brain 131, 1311–1322. doi: 10.1093/brain/awn066
Cox, R. W. (2012). AFNI: What a long strange trip it's been. NeuroImage 62, 743–747. doi: 10.1016/j.neuroimage.2011.08.056
Craig, A. D. (2009). How do you feel—now? The anterior insula and human awareness. Nat. Rev. Neurosci. 10, 59–70. doi: 10.1038/nrn2555
Critchley, H. D., Wiens, S., Rotshtein, P., Öhman, A., and Dolan, R. J. (2004). Neural systems supporting interoceptive awareness. Nat. Neurosci. 7, 189–195. doi: 10.1038/nn1176
Crocker, L. D., Heller, W., Spielberg, J. M., Warren, S. L., Bredemeier, K., Sutton, B. P., et al. (2012). Neural mechanisms of attentional control differentiate trait and state negative affect. Front. Psychol. 3, 298. doi: 10.3389/fpsyg.2012.00298
Cui, H., Zhang, B., Li, W., Li, H., Pang, J., Hu, Q., et al. (2020). Insula shows abnormal task-evoked and resting-state activity in first-episode drug-naïve generalized anxiety disorder. Depress. Anxiety 37, 632–644. doi: 10.1002/da.23009
Damaraju, E., Allen, E. A., Belger, A., Ford, J. M., McEwen, S., Mathalon, D. H., et al. (2014). Dynamic functional connectivity analysis reveals transient states of dysconnectivity in schizophrenia. NeuroImage Clin. 5, 298–308. doi: 10.1016/j.nicl.2014.07.003
Dammann, J., Klepzig, K., Schenkenberger, E., Kordass, B., and Lotze, M. (2020). Association of decrease in insula fMRI activation with changes in trait anxiety in patients with craniomandibular disorder (CMD). Behav. Brain Res. 379, 112327. doi: 10.1016/j.bbr.2019.112327
Destrieux, C., Fischl, B., Dale, A., and Halgren, E. (2010). Automatic parcellation of human cortical gyri and sulci using standard anatomical nomenclature. Neuroimage 53, 1–15. doi: 10.1016/j.neuroimage.2010.06.010
Dimitriadis, S. I., Salis, C., Tarnanas, I., and Linden, D. E. (2017). Topological filtering of dynamic functional brain networks unfolds informative chronnectomics: a novel data-driven thresholding scheme based on orthogonal minimal spanning trees (OMSTs). Front. Neuroinform. 11, 28. doi: 10.3389/fninf.2017.00028
Dixon, M. L., Thiruchselvam, R., Todd, R., and Christoff, K. (2017). Emotion and the prefrontal cortex: an integrative review. Psychol. Bull. 143, 1033–1081. doi: 10.1037/bul0000096
Du, Y., Pearlson, G. D., Lin, D., Sui, J., Chen, J., Salman, M., et al. (2017). Identifying dynamic functional connectivity biomarkers using GIG-ICA: application to schizophrenia, schizoaffective disorder, and psychotic bipolar disorder. Hum. Brain Mapp. 38, 2683–2708. doi: 10.1002/hbm.23553
Duval, E. R., Joshi, S. A., Russman Block, S., Abelson, J. L., and Liberzon, I. (2018). Insula activation is modulated by attention shifting in social anxiety disorder. J. Anxiety Disord. 56, 56–62. doi: 10.1016/j.janxdis.2018.04.004
Endler, N. S., and Kocovski, N. L. (2001). State and trait anxiety revisited. J. Anxiety Disord. 15, 231–245. doi: 10.1016/S0887-6185(01)00060-3
Ernst, J., Böker, H., Hättenschwiler, J., Schüpbach, D., Northoff, G., Seifritz, E., et al. (2013). The association of interoceptive awareness and alexithymia with neurotransmitter concentrations in insula and anterior cingulate. Soc. Cogn. Affect. Neurosci. 9, 857–863. doi: 10.1093/scan/nst058
Eysenck, M. W., Derakshan, N., Santos, R., and Calvo, M. G. (2007). Anxiety and cognitive performance: attentional control theory. Emotion 7, 336–353. doi: 10.1037/1528-3542.7.2.336
Forrest, S. J., Siegert, R. J., Krägeloh, C. U., Landon, J., and Medvedev, O. N. (2021). Generalizability theory distinguishes between state and trait anxiety. Psychol. Assess. 33, 1080–1088. doi: 10.1037/pas0001060
Freeman, L. C. (1979). Centrality in social networks conceptual clarification. Soc. Netw. 1, 215–239. doi: 10.1016/0378-8733(78)90021-7
Friston, K. J. (2011). Functional and effective connectivity: a review. Brain Connect. 1, 13–36. doi: 10.1089/brain.2011.0008
Geng, H., Wang, Y., Gu, R., Luo, Y. J., Xu, P., Huang, Y., et al. (2018). Altered brain activation and connectivity during anticipation of uncertain threat in trait anxiety. Hum. Brain Mapp. 39, 3898–3914. doi: 10.1002/hbm.24219
Grachev, I. D., and Apkarian, A. V. (2000). Anxiety in healthy humans is associated with orbital frontal chemistry. Mol. Psychiatry 5, 482–488. doi: 10.1038/sj.mp.4000778
Grinband, J., Hirsch, J., and Ferrera, V. P. (2006). A neural representation of categorization uncertainty in the human brain. Neuron 49, 757–763. doi: 10.1016/j.neuron.2006.01.032
Grosbras, M.-H., Laird, A. R., and Paus, T. (2005). Cortical regions involved in eye movements, shifts of attention, and gaze perception. Hum. Brain Mapp. 25, 140–154. doi: 10.1002/hbm.20145
Guo, X., Duan, X., Chen, H., He, C., Xiao, J., Han, S., et al. (2020). Altered inter- and intrahemispheric functional connectivity dynamics in autistic children. Hum. Brain Mapp. 41, 419–428. doi: 10.1002/hbm.24812
Guo, Z., Liu, X., Hou, H., Wei, F., Liu, J., and Chen, X. (2016). Abnormal degree centrality in Alzheimer's disease patients with depression: a resting-state functional magnetic resonance imaging study. Exp. Gerontol. 79, 61–66. doi: 10.1016/j.exger.2016.03.017
He, C., Chen, Y., Jian, T., Chen, H., Guo, X., Wang, J., et al. (2018). Dynamic functional connectivity analysis reveals decreased variability of the default-mode network in developing autistic brain. Autism Res. 11, 1479–1493. doi: 10.1002/aur.2020
He, Y., Xu, T., Zhang, W., and Zuo, X. N. (2016). Lifespan anxiety is reflected in human amygdala cortical connectivity. Hum. Brain Mapp. 37, 1178–1193. doi: 10.1002/hbm.23094
Henni, K., Mezghani, N., and Gouin-Vallerand, C. (2018). Unsupervised graph-based feature selection via subspace and pagerank centrality. Expert Syst. Appl. 114, 46–53. doi: 10.1016/j.eswa.2018.07.029
Herwig, U., Abler, B., Walter, H., and Erk, S. (2007). Expecting unpleasant stimuli – an fMRI study. Psychiatry Res. Neuroimaging 154, 1–12. doi: 10.1016/j.pscychresns.2006.02.007
Hu, Y., and Dolcos, S. (2017). Trait anxiety mediates the link between inferior frontal cortex volume and negative affective bias in healthy adults. Soc. Cogn. Affect. Neurosci. 12, 775–782. doi: 10.1093/scan/nsx008
Hur, J., Miller, G. A., McDavitt, J. R. B., Spielberg, J. M., Crocker, L. D., Infantolino, Z. P., et al. (2015). Interactive effects of trait and state affect on top-down control of attention. Soc. Cogn. Affect. Neurosci. 10, 1128–1136. doi: 10.1093/scan/nsu163
Hutchison, R. M., Womelsdorf, T., Allen, E. A., Bandettini, P. A., Calhoun, V. D., Corbetta, M., et al. (2013a). Dynamic functional connectivity: promise, issues, and interpretations. Neuroimage 80, 360–378. doi: 10.1016/j.neuroimage.2013.05.079
Hutchison, R. M., Womelsdorf, T., Gati, J. S., Everling, S., and Menon, R. S. (2013b). Resting-state networks show dynamic functional connectivity in awake humans and anesthetized macaques. Hum. Brain Mapp. 34, 2154–2177. doi: 10.1002/hbm.22058
Jaun-Frutiger, K., Cazzoli, D., Müri, R. M., Bassetti, C. L., and Nyffeler, T. (2013). The frontal eye field is involved in visual vector inversion in humans—a theta burst stimulation study. PLoS ONE 8, e83297. doi: 10.1371/journal.pone.0083297
Jenkinson, M., Beckmann, C. F., Behrens, T. E. J., Woolrich, M. W., and Smith, S. M. (2012). FSL. NeuroImage 62, 782–790. doi: 10.1016/j.neuroimage.2011.09.015
Jeurissen, B., Descoteaux, M., Mori, S., and Leemans, A. (2019). Diffusion MRI fiber tractography of the brain. NMR Biomed. 32, e3785. doi: 10.1002/nbm.3785
Jiang, L., Xu, Y., Zhu, X. T., Yang, Z., Li, H. J., and Zuo, X. N. (2015). Local-to-remote cortical connectivity in early- and adulthood-onset schizophrenia. Transl. Psychiatry 5, e566. doi: 10.1038/tp.2015.59
Kang, J., Wang, L., Yan, C., Wang, J., Liang, X., and He, Y. (2011). Characterizing dynamic functional connectivity in the resting brain using variable parameter regression and Kalman filtering approaches. NeuroImage 56, 1222–1234. doi: 10.1016/j.neuroimage.2011.03.033
Koenis, M. M. G., Brouwer, R. M., Swagerman, S. C., van Soelen, I. L. C., Boomsma, D. I., and Hulshoff Pol, H. E. (2018). Association between structural brain network efficiency and intelligence increases during adolescence. Hum. Brain Mapp. 39, 822–836. doi: 10.1002/hbm.23885
Kondo, H. M., and Kashino, M. (2007). Neural mechanisms of auditory awareness underlying verbal transformations. NeuroImage 36, 123–130. doi: 10.1016/j.neuroimage.2007.02.024
Latora, V., and Marchiori, M. (2001). Efficient behavior of small-world networks. Phys. Rev. Lett. 87, 198701. doi: 10.1103/PhysRevLett.87.198701
Latora, V., and Marchiori, M. (2003). Economic small-world behavior in weighted networks. Euro. Phys. J. B 32, 249–263. doi: 10.1140/epjb/e2003-00095-5
Li, C., Qiao, K., Mu, Y., and Jiang, L. (2021). Large-Scale morphological network efficiency of human brain: cognitive intelligence and emotional intelligence. Front. Aging Neurosci. 13, 605158. doi: 10.3389/fnagi.2021.605158
Li, H. C. W., and Lopez, V. (2004). The reliability and validity of the Chinese version of the trait anxiety scale for children. Res. Nurs. Health 27, 426–434. doi: 10.1002/nur.20045
Li, K., Zhang, M., Zhang, H., Li, X., Zou, F., Wang, Y., et al. (2020). The spontaneous activity and functional network of the occipital cortex is correlated with state anxiety in healthy adults. Neurosci. Lett. 715, 134596. doi: 10.1016/j.neulet.2019.134596
Li, R., Liao, W., Yu, Y., Chen, H., Guo, X., Tang, Y. L., et al. (2018). Differential patterns of dynamic functional connectivity variability of striato-cortical circuitry in children with benign epilepsy with centrotemporal spikes. Hum. Brain Mapp. 39, 1207–1217. doi: 10.1002/hbm.23910
Li, Y., Liu, Y., Li, J., Qin, W., Li, K., Yu, C., et al. (2009). Brain anatomical network and intelligence. PLoS Comput. Biol. 5, e1000395. doi: 10.1371/journal.pcbi.1000395
Liao, X., Yuan, L., Zhao, T., Dai, Z., Shu, N., Xia, M., et al. (2015). Spontaneous functional network dynamics and associated structural substrates in the human brain. Front. Hum. Neurosci. 9, 478. doi: 10.3389/fnhum.2015.00478
Lindquist, K. A., Wager, T. D., Kober, H., Bliss-Moreau, E., and Barrett, L. F. (2012). The brain basis of emotion: a meta-analytic review. Behav. Brain Sci. 35, 121–143. doi: 10.1017/S0140525X11000446
Liu, X., and Duyn, J. H. (2013). Time-varying functional network information extracted from brief instances of spontaneous brain activity. Proc. Natl. Acad. Sci. U.S.A. 110, 4392–4397. doi: 10.1073/pnas.1216856110
Lutz, A., McFarlin, D. R., Perlman, D. M., Salomons, T. V., and Davidson, R. J. (2013). Altered anterior insula activation during anticipation and experience of painful stimuli in expert meditators. NeuroImage 64, 538–546. doi: 10.1016/j.neuroimage.2012.09.030
Maier-Hein, K. H., Neher, P. F., Houde, J.-C., Côt,é, M.-A, Garyfallidis, E., Zhong, J., et al. (2017). The challenge of mapping the human connectome based on diffusion tractography. Nat. Commun. 8, 1349. doi: 10.1038/s41467-017-01285-x
Mataix-Cols, D., Cullen, S., Lange, K., Zelaya, F., Andrew, C., Amaro, E., et al. (2003). Neural correlates of anxiety associated with obsessive-compulsive symptom dimensions in normal volunteers. Biol. Psychiatry 53, 482–493. doi: 10.1016/S0006-3223(02)01504-4
Mathews, A. (1990). Why worry? The cognitive function of anxiety. Behav Res Ther. 28, 455–468. doi: 10.1016/0005-7967(90)90132-3
Mechelli, A., Friston, K. J., Frackowiak, R. S., and Price, C. J. (2005). Structural covariance in the human cortex. J. Neurosci. 25, 8303–8310. doi: 10.1523/JNEUROSCI.0357-05.2005
Modi, S., Kumar, M., Kumar, P., and Khushu, S. (2015). Aberrant functional connectivity of resting state networks associated with trait anxiety. Psychiatry Res. 234, 25–34. doi: 10.1016/j.pscychresns.2015.07.006
Muggleton, N. G., Juan, C.-H., Cowey, A., and Walsh, V. (2003). Human frontal eye fields and visual search. J. Neurophysiol. 89, 3340–3343. doi: 10.1152/jn.01086.2002
Nagel, M., Sprenger, A., Lencer, R., Kömpf, D., Siebner, H., and Heide, W. (2008). Distributed representations of the “preparatory set” in the frontal oculomotor system: a TMS study. BMC Neurosci. 9, 89. doi: 10.1186/1471-2202-9-89
Nguyen, T. T., Kovacevic, S., Dev, S. I., Lu, K., Liu, T. T., and Eyler, L. T. (2017). Dynamic functional connectivity in bipolar disorder is associated with executive function and processing speed: a preliminary study. Neuropsychology 31, 73–83. doi: 10.1037/neu0000317
Nyffeler, T., Bucher, O., Pflugshaupt, T., Von Wartburg, R., Wurtz, P., Hess, C. W., et al. (2004). Single-pulse transcranial magnetic stimulation over the frontal eye field can facilitate and inhibit saccade triggering. Eur. J. Neurosci. 20, 2240–2244. doi: 10.1111/j.1460-9568.2004.03667.x
Potvin, O., Catheline, G., Bernard, C., Meillon, C., Bergua, V., Allard, M., et al. (2015). Gray matter characteristics associated with trait anxiety in older adults are moderated by depression. Int. Psychogeriatr. 27, 1813–1824. doi: 10.1017/S1041610215000836
Power, J. D., Barnes, K. A., Snyder, A. Z., Schlaggar, B. L., and Petersen, S. E. (2012). Spurious but systematic correlations in functional connectivity MRI networks arise from subject motion. NeuroImage 59, 2142–2154. doi: 10.1016/j.neuroimage.2011.10.018
Quentin, R., Chanes, L., Migliaccio, R., Valabrègue, R., and Valero-Cabré, A. (2013). Fronto-tectal white matter connectivity mediates facilitatory effects of non-invasive neurostimulation on visual detection. NeuroImage 82, 344–354. doi: 10.1016/j.neuroimage.2013.05.083
Rashid, B., Arbabshirani, M. R., Damaraju, E., Cetin, M. S., Miller, R., Pearlson, G. D., et al. (2016). Classification of schizophrenia and bipolar patients using static and dynamic resting-state fMRI brain connectivity. NeuroImage 134, 645–657. doi: 10.1016/j.neuroimage.2016.04.051
Rubinov, M., and Sporns, O. (2010). Complex network measures of brain connectivity: uses and interpretations. Neuroimage 52, 1059–1069. doi: 10.1016/j.neuroimage.2009.10.003
Salvador, R., Suckling, J., Coleman, M. R., Pickard, J. D., Menon, D., and Bullmore, E. (2005). Neurophysiological architecture of functional magnetic resonance images of human brain. Cereb. Cortex 15, 1332–1342. doi: 10.1093/cercor/bhi016
Satpute, A. B., Mumford, J. A., Naliboff, B. D., and Poldrack, R. A. (2012). Human anterior and posterior hippocampus respond distinctly to state and trait anxiety. Emotion 12, 58–68. doi: 10.1037/a0026517
Saviola, F., Pappaianni, E., Monti, A., Grecucci, A., Jovicich, J., and De Pisapia, N. (2020). Trait and state anxiety are mapped differently in the human brain. Sci. Rep. 10, 11112. doi: 10.1038/s41598-020-68008-z
Seidlitz, J., Vasa, F., Shinn, M., Romero-Garcia, R., Whitaker, K. J., Vertes, P. E., et al. (2018). Morphometric Similarity Networks Detect Microscale Cortical Organization and Predict Inter-Individual Cognitive Variation. Neuron 97, 231–247.e7. doi: 10.1016/j.neuron.2017.11.039
Shah, S. G., Klumpp, H. P., Angstadt, M., Nathan, P. J., and Phan, K. L. (2009). Amygdala and insula response to emotional images in patients with generalized social anxiety disorder. J. Psychiatry Neurosci. 34, 296–302
Simmons, A. N., Stein, M. B., Strigo, I. A., Arce, E., Hitchcock, C., and Paulus, M. P. (2011). Anxiety positive subjects show altered processing in the anterior insula during anticipation of negative stimuli. Hum. Brain Mapp. 32, 1836–1846. doi: 10.1002/hbm.21154
Singer, T., Critchley, H. D., and Preuschoff, K. (2009). A common role of insula in feelings, empathy and uncertainty. Trends Cogn. Sci. 13, 334–340. doi: 10.1016/j.tics.2009.05.001
Smith, D. T., Jackson, S. R., and Rorden, C. (2005). Transcranial magnetic stimulation of the left human frontal eye fields eliminates the cost of invalid endogenous cues. Neuropsychologia 43, 1288–1296. doi: 10.1016/j.neuropsychologia.2004.12.003
Spielberger, C. D. (1966). “Chapter 14—the effects of anxiety on complex learning and academic achievement,” Anxiety and Behavior. Elsevier Inc., 361–398. doi: 10.1016/B978-1-4832-3131-0.50019-6
Spielberger, C. D., Gorsuch, R. L., Lushene, R., Vagg, P. R., and Jacobs, G. A. (1983). Manual for the State-Trait Anxiety Inventory. Palo Alto, CA: Consulting Psychologists Press.
Sporns, O. (2011). The human connectome: a complex network. Ann. N. Y. Acad. Sci. 1224, 109–125. doi: 10.1111/j.1749-6632.2010.05888.x
Takagi, Y., Sakai, Y., Abe, Y., Nishida, S., Harrison, B. J., Martinez-Zalacain, I., et al. (2018). A common brain network among state, trait, and pathological anxiety from whole-brain functional connectivity. Neuroimage 172, 506–516. doi: 10.1016/j.neuroimage.2018.01.080
Thomas, C., Ye, F. Q., Irfanoglu, M. O., Modi, P., Saleem, K. S., Leopold, D. A., et al. (2014). Anatomical accuracy of brain connections derived from diffusion MRI tractography is inherently limited. Proc. Natl. Acad. Sci. U.S.A. 111, 16574–16579. doi: 10.1073/pnas.1405672111
Thompson, K. G., Hanes, D. P., Bichot, N. P., and Schall, J. D. (1996). Perceptual and motor processing stages identified in the activity of macaque frontal eye field neurons during visual search. J. Neurophysiol. 76, 4040–4055. doi: 10.1152/jn.1996.76.6.4040
Tian, X., Wei, D., Du, X., Wang, K., Yang, J., Liu, W., et al. (2016). Assessment of trait anxiety and prediction of changes in state anxiety using functional brain imaging: a test-retest study. Neuroimage 133, 408–416. doi: 10.1016/j.neuroimage.2016.03.024
Tranel, D., Feinstein, J. S., Khalsa, S. S., and Rudrauf, D. (2009). The pathways of interoceptive awareness. Nat. Neurosci. 12, 1494–1496. doi: 10.1038/nn.2411
van Duinkerken, E., Schoonheim, M. M., Moll, A. C., Landeira-Fernandez, J, Klein, M., Diamant, M., et al. (2017). Altered eigenvector centrality is related to local resting-state network functional connectivity in patients with longstanding type 1 diabetes mellitus. Hum. Brain Mapp. 38, 3623–3636. doi: 10.1002/hbm.23617
Vernet, M., Quentin, R., Chanes, L., Mitsumasu, A., and Valero-Cabre, A. (2014). Frontal eye field, where art thou? Anatomy, function, and non-invasive manipulation of frontal regions involved in eye movements and associated cognitive operations. Front. Integr. Neurosci. 8:66. doi: 10.3389/fnint.2014.00088
Wei, D., Du, X., Li, W., Chen, Q., Li, H., Hao, X., et al. (2015). Regional gray matter volume and anxiety-related traits interact to predict somatic complaints in a non-clinical sample. Soc. Cogn. Affect. Neurosci. 10, 122–128. doi: 10.1093/scan/nsu033
Weiler, M., Casseb, R. F., de Campos, B. M., Teixeira, C. V., Carletti-Cassani, A. F., Vicentini, J. E., et al. (2018). Cognitive reserve relates to functional network efficiency in Alzheimer's disease. Front. Aging Neurosci. 10, 255. doi: 10.3389/fnagi.2018.00255
Williams, J., Mathews, A., and MacLeod, C. (1996). The emotional stroop task and psychopathology. Psychol. Bull. 120, 3–24. doi: 10.1037/0033-2909.120.1.3
Xu, T., Yang, Z., Jiang, L., Xing, X.-X., and Zuo, X.-N. (2015). A connectome computation system for discovery science of brain. Sci. Bull. 60, 86–95. doi: 10.1007/s11434-014-0698-3
Xue, S. W., Lee, T. W., and Guo, Y. H. (2018). Spontaneous activity in medial orbitofrontal cortex correlates with trait anxiety in healthy male adults. J. Zhejiang Univ. Sci. B 19, 643–653. doi: 10.1631/jzus.B1700481
Yang, Q., and Kapoula, Z. (2011). Distinct control of initiation and metrics of memory-guided saccades and vergence by the FEF: a TMS study. PLoS ONE 6, e20322. doi: 10.1371/journal.pone.0020322
Yao, Z., Liao, M., Hu, T., Zhang, Z., Zhao, Y., Zheng, F., et al. (2017). An effective method to identify adolescent generalized anxiety disorder by temporal features of dynamic functional connectivity. Front. Hum. Neurosci. 11, 492. doi: 10.3389/fnhum.2017.00492
Yeo, B. T., Krienen, F. M., Sepulcre, J., Sabuncu, M. R., Lashkari, D., Hollinshead, M., et al. (2011). The organization of the human cerebral cortex estimated by intrinsic functional connectivity. J. Neurophysiol. 106, 1125–1165. doi: 10.1152/jn.00338.2011
Yin, P., Zhang, M., Hou, X., Tan, Y., Fu, Y., and Qiu, J. (2016). The brain structure and spontaneous activity baseline of the behavioral bias in trait anxiety. Behav. Brain Res. 312, 355–361. doi: 10.1016/j.bbr.2016.06.036
Zhang, Y. W., Zhao, Z. L., Qi, Z., Hu, Y., Wang, Y. S., Sheng, C., et al. (2017). Local-to-remote cortical connectivity in amnestic mild cognitive impairment. Neurobiol. Aging 56, 138–149. doi: 10.1016/j.neurobiolaging.2017.04.016
Zuo, X. N., Ehmke, R., Mennes, M., Imperati, D., Castellanos, F. X., Sporns, O., et al. (2012). Network centrality in the human functional connectome. Cereb. Cortex 22, 1862–1875. doi: 10.1093/cercor/bhr269
Keywords: MRI, human brain, anxiety, centrality, efficiency
Citation: Li Y and Jiang L (2022) State and Trait Anxiety Share Common Network Topological Mechanisms of Human Brain. Front. Neuroinform. 16:859309. doi: 10.3389/fninf.2022.859309
Received: 21 January 2022; Accepted: 04 May 2022;
Published: 23 June 2022.
Edited by:
Dong Song, University of Southern California, United StatesReviewed by:
Yonggui Yuan, Southeast University, ChinaCopyright © 2022 Li and Jiang. This is an open-access article distributed under the terms of the Creative Commons Attribution License (CC BY). The use, distribution or reproduction in other forums is permitted, provided the original author(s) and the copyright owner(s) are credited and that the original publication in this journal is cited, in accordance with accepted academic practice. No use, distribution or reproduction is permitted which does not comply with these terms.
*Correspondence: Lili Jiang, amlhbmdsbEBwc3ljaC5hYy5jbg==
Disclaimer: All claims expressed in this article are solely those of the authors and do not necessarily represent those of their affiliated organizations, or those of the publisher, the editors and the reviewers. Any product that may be evaluated in this article or claim that may be made by its manufacturer is not guaranteed or endorsed by the publisher.
Research integrity at Frontiers
Learn more about the work of our research integrity team to safeguard the quality of each article we publish.