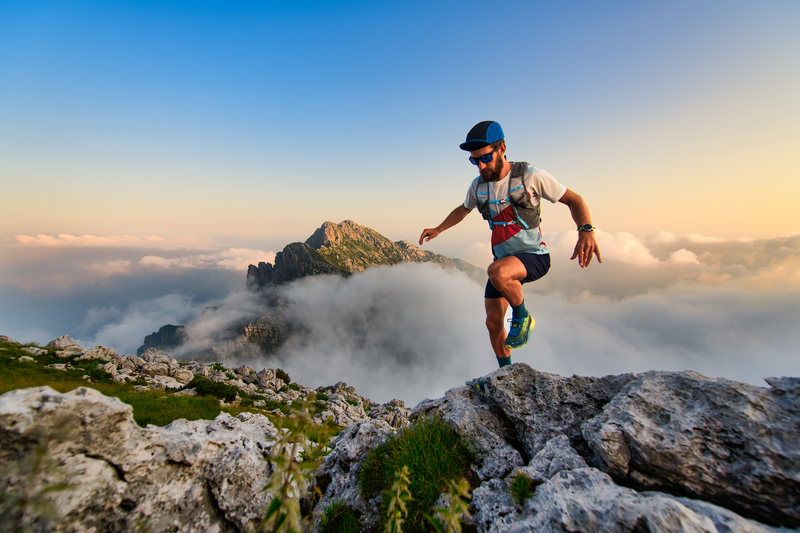
94% of researchers rate our articles as excellent or good
Learn more about the work of our research integrity team to safeguard the quality of each article we publish.
Find out more
ORIGINAL RESEARCH article
Front. Neuroinform. , 21 May 2019
Volume 13 - 2019 | https://doi.org/10.3389/fninf.2019.00036
This article is part of the Research Topic Nanoscale Neurocartography: Approaches for Mapping the Brain at the Neuron and Synapse Level View all 4 articles
The process through which neurons are labeled is a key methodological choice in measuring neuron morphology. However, little is known about how this choice may bias measurements. To quantify this bias we compare the extracted morphology of neurons collected from the same rodent species, experimental condition, gender distribution, age distribution, brain region and putative cell type, but obtained with 19 distinct staining methods. We found strong biases on measured features of morphology. These were largest in features related to the coverage of the dendritic tree (e.g., the total dendritic tree length). Understanding measurement biases is crucial for interpreting morphological data.
There are many techniques through which neuron morphologies may be imaged. These techniques can be classified based on two factors: how they target neurons for imaging, and how the axons and dendrites of the probed neurons are labeled so they are visible for imaging. Neurons may be targeted through their propensity to take up heavy metals or genetic markers, through immunohistochemistry or by direct injection (Elston et al., 1999; Jacobs et al., 2001; Travis et al., 2005; Donohue and Ascoli, 2011; Parekh and Ascoli, 2013, 2015; Carter and Shieh, 2015). Neurons may be labeled using a variety of heavy metals, fluorescent or chromogenic labels are used to allow imaging. We may expect that both targeting and labeling aspects will introduce biases upon the resulting reconstructions of morphology.
Staining with heavy metals remains one of the key imaging techniques. Golgi staining is the oldest such method. In Golgi staining, silver nitrate is introduced to fixed tissue, and the metal is taken up by a sub-population of neurons through a mechanism that remains largely uncharacterized. Neurons are stained in their entirety and then imaged with light microscopy (Koyama, 2013). The method can be subdivided into Rapid Golgi, Golgi-Kopsch, and Golgi-Cox (Koyama, 2013) and each version labels a subset of neurons. Other heavy metals such as osmium and lead can instead be used for dense labeling, which is popular for EM data (Watson, 1958; Tapia et al., 2012). Or, alternatively, lipophilic dyes such as DiOlistics can be introduced ballistically to neurons and allow for Golgi-like staining (Staffend and Meisel, 2011). Since the biological mechanism of heavy staining method is mainly unknown, the extract morphology may be subject to selection bias (Staffend and Meisel, 2011). Because it is relatively simple to perform compared to other methods, heavy metals are a popular staining method for fixed tissues.
A more recent approach is to target neurons through genetic markers. Fluorescent proteins such as green fluorescent protein (GFP), red fluorescent protein (RFP), and yellow fluorescent protein (YFP) can be introduced transgenically to be expressed in neurons, and then imaged through fluorescent microscopy to reveal morphology (Marshall et al., 1995). The use of fluorescent proteins may be limited to animals for which good genetic tools exist. Fluorescent proteins are introduced under the control of promoter regions that are active for known cell-type markers. They target specific populations of neurons, which may differ from those neurons targeted by other methods. The size of these potential selection biases again remains relatively uncharacterized. Fluorescent techniques are popular because they readily integrate both into genetic and physiological approaches.
Immunostaining has advanced to be a leading staining technique. Immunostaining uses antibodies to target neuronal molecular markers, which can be labeled with fluorescent or chromogenic tags for imaging (Chen et al., 2010; Tanapat, 2013). A common approach relies on biotin variants, such as biocytin or neurobiotin, being conjugated to an antibody (Swietek et al., 2016). A complex of biocytin and its binding partner, avidin, are tagged with a fluorescent or colored label that can then be imaged. The avidin-biotin complex allows imaging through light, fluorescent, or EM microscopy depending on the label. Common fluorescent dyes used with immunostaining include Alexa Fluor (AF) (Carter and Shieh, 2015). Immunostaining targets neurons based on particular molecular markers which allows a broad range of targets. Immunostaining is particularly popular as it readily integrates into the modern molecular approaches.
Finally, neurons can be directly injected (Vaney, 2002; Elston, 2003). Direct injection allows neurons to be labeled in vivo or in slice samples and later imaged in a fixed preparation, meaning electrophysiology can be related to morphology. It is common to directly inject fluorescent dyes such as Lucifer Yellow (Hanani, 2012) or biotin variants such as biocytin or neurobiotin (Klenowski et al., 2017).
Each method comes with idiosyncrasies and methodological steps that can vary across laboratories. For instance, in immunostaining the antibody concentration, length of incubation time, and accessibility to the antigen all must be balanced to produce a good result (Paavilainen et al., 2010; Carter and Shieh, 2015). All these factors may vary from lab to lab and are a known source of variability. For example, it has been shown that hippocampal CA1 neurons measured in rats housed in different labs are not consistent in terms of their morphometry (Scorcioni et al., 2004). Tripathy et al. (2015) have shown similar biases in electrophysiology (Tripathy et al., 2015; Tebaykin et al., 2017). Understanding the effects of staining is thus crucial for the interpretation of downstream analyses.
Each method also targets different neurons and operates through different biochemical processes such that, even if performed within the same lab, morphology measurements can differ by staining method. For instance, during dehydration it is well-known that incubation with different dyes can affect tissue shrinkage which in turn can affect morphology (Grace and Llinás, 1985). Neurobiotin staining is known to affect both electrophysiology and morphology (Xi and Xu, 1996). In comparing morphology obtained by Golgi-Cox staining and neurobiotin electroporation, it has been shown that neurobiotin-filling revealed significantly larger dendritic arbors and different spine densities compared to GolgiCox-stained neurons (Klenowski et al., 2017). Despite these known issues, there are few systematic studies that examine the size and nature of these biases across the many methods used to quantify morphology.
Large databases of neuron morphologies (Ascoli, 2006) collect data from many labs, each employing different methods. This allows the comparison of data across distinct staining methods. While many experimental aspects of neuron quantification will differ, the staining method is a central experimental choice. As such, it is important to ask what large databases can tell us about the biases induced by staining methods.
Here we quantify the variation in measured neuron morphology related to the staining or the fluorescent labeling method used, though we will refer to both of these as staining method. We analyze rodent data that has been uploaded by various labs to the public morphology repository neuromorpho.org (Ascoli, 2006). We group them based on the biological attributes and the staining methods. By matching on biological attributes and comparing the morphometry of each group we identify the variation that can be explained by different staining methods.
We used dendrite morphologies submitted to neuromorpho.org (version 7.4), a publicly available database of morphology. We performed a careful search of neuromoropho.org to identify populations of neurons that allow for an appropriate study of the effect of staining method. We describe the search criteria used below.
To ensure that dendrites were traced completely, we filtered out neurons in the database whose physical integrity of their dendritic reconstructions was labeled as incomplete. We analyzed neurons that are extracted from a healthy animal, by considering only the neurons whose experimental condition were labeled as control. These steps prevent our analysis from including unwanted effects due to poor reconstruction and experimental condition.
We identified populations of neurons sampled from a specific species, age, gender distribution, region, laminar location obtained with a least two staining methods. To do this we restricted our analysis to the neurons from rat to mouse. We grouped neurons into three age classes: young (more than a month and < 2 months), young adult (between 2 and 6 months) and adult (more than 6 months). To match the gender distribution, we grouped the neurons into three classes: male, female, and male/female. The latter class was used when labs deposited the equal number of neurons from male and female in the same experiment. We matched the cell types as follows. First, we grouped the neurons into two primary cell types: principal cell and interneurons. Then we grouped each primary cell types into secondary cell type (including pyramidal, granule, mitral, GABAergic). Finally, we grouped them into territory cell type (including Aspiny, spiny, adult-born, newborn) if such information was provided. Similarly, we matched the brain regions as follows. We first grouped them by primary brain regions (neocortex, cerebellum, hippocampus, main olfactory bulb, retina, amygdala, brainstem, entorhinal cortex, spinal cord, protocerebrum). Then we grouped each primary brain regions into secondary brain regions (including primary somatosensory, somatosensory, primary visual, CA1, CA, CA3, dentate gyrus, striatum, anterior cingulate, prelimbic, thalamus, hypothalamus, basolateral amygdala). Then, if each region has a laminar structure (for example somatosensory cortex), we grouped the neurons by their layer [laminar structure contains six distinctive layers (1–6) and three shared layers (2–3, 3–4, 5–6)]. Similarly if the regions had sagittal structure (left, right), or coronal structure (occipital, medial, prefrontal, frontal) or ventral/dorsal structure then we grouped them accordingly. Brain region definitions and nomenclature are taken from the Allen Institute for Brain Science mouse brain atlas, for both the mouse and rat data (Jones et al., 2009). We use it to normalize neuron assignment at the coarse layer (e.g., CA1 = subregion of hippocampus), as the fine structure of the neuron locations is typically not reported. We omitted neurons for which at least one of the above labels was not reported in the database. Using these criteria we grouped the neurons into classes.
If there were at least two different staining methods in a matched group (same brain region, gender distribution, age, species, cell-type) and each staining method has at least five samples in the set, the group was chosen for comparison. In this way we identified 22 matched sets of neurons sourced from more than 60 papers (Figure 1, Tables 1, 2).
Figure 1. The spatial distribution of the 22 groups analyzed are from multiple brain regions in rodents. The number of comparison groups in each region are listed in parentheses.
In these 22 matched groups of neurons are 19 distinct staining methods, labeled by neuromorpho.org. We grouped these into three types: staining with heavy metals, genetic markers, and immunostaining and direct injection. In the heavy metals group: Golgi, and Golgi-Cox. In the genetic markers group: green fluorescent protein, red fluorescent protein, enhanced green fluorescent protein, and Tag red fluorescent protein. In the immunostaining group: immunostaining, horseradish peroxidase, neurobiotin, biocytin, biocytin & betaIV-spectrin, Alexa Fluor 488, Alexa 647-dextrane, Alexa Fluor 594, OGB-1, biotinylated dextran amine, lucifer yellow, green fluorescent protein, Alexa Fluor 488, immunostaining, green fluorescent protein, immunostaining.
To compare neuron morphologies we need to quantify them. The morphology of a neuron is described by a set of points each with a coordinate, diameter and index of its parent point. And a set of edges connecting parent points to their children (Stockley et al., 1993). We used six features to measure the effect of staining. Four features are defined in previous publications and are parts of the L-measure (Scorcioni et al., 2008). Two features are unique to this paper. We classed each feature as either global or local.
Three global features are used. First, the number of branching points in the neuron, or how many times the morphology branched. This feature is defined previously in L-measure. Second, the total length of the dendritic tree. This feature is defined previously in L-measure. Third, the global angle. This measures the angle between the dendritic segment and the vector pointing toward the soma. It provides a measure of how much dendrites point away from the soma (Figure 2). This feature is defined in this paper for the first time.
Figure 2. Sample neuron morphologies and features. (A) Sample morphologies from two groups of neurons, comparing two distinct staining methods. (B) Geometrical features of neuron morphology. Local angle represents the angle between adjacent edges not at a branch point. The global angle represents the angle between an edge and the vector pointing toward the soma. The branch angle represents the angle between two edges that branch from a common node. (C) Histograms for the two sample groups of the six morphological features used in analysis.
Three local features are used. First, the branching angle. This is the angle between two edges that branch from a common parent. This feature is defined previously in L-measure. Second, the length of segments. This is the length of dendrite between two consecutive branching nodes. This feature is defined previously in L-measure. Third, the local angle. The local angle measures the angles between the vector connecting the node to its parent and the vector connecting the node to its child. We only consider nodes that have one child. This measures how straight the neurites of the neurons are. This feature is defined for the first time.
For features that are measured per dendrite segment (e.g., branching angle, global angle, local angle), data are pooled over all neurons in the group. In order to avoid artifacts due to software reconstruction of the neuron, we resampled the morphology such that the distance between each consecutive node was equal. To do this we preserved the terminals and branching nodes and selected one node every 10 micrometers (but we suppress the last point if it is within 10 micrometers of the terminal or branch node). This way we obtain a normalized representation that can be compared.
We tested for an effect of the staining method on each morphological feature, above effects explained through biological attributes. Our morphological features are generally continuous valued, while neuron classes are categorical. Further, the morphological features generally follow a non-Gaussian distribution (Figure 2). This requires using non-parametric tests. We thus used the Wilcoxon rank-sum test. That is, for each group , we tested:
for all , where μ1/2 represents the median, S the staining method, and M the morphological feature. The hypothesis that no overall effect exists for a given morphological feature is
for the N levels in B. To correct for multiple testing we used Bonferroni correction.
The differences in morphology between staining methods can also be quantified over groups by considering the difference in means:
which summarizes the average difference in morphological feature Mj. This corresponds to the average treatment effect in the causal inference literature (Pearl, 2009), although we can not (and do not) make claims about causality here. A null distribution for each βj is generated by repeated permutation of staining label, allowing us to determine significance levels.
We first asked if neurons obtained by distinct staining methods are distinguishable. Within each group, we compared the distribution of each morphological feature between a pair of staining methods (Figure 2). To do this we tested the hypothesis that the reconstructed morphologies are statistically similar within each group. We observed that, for each pairwise comparison between two staining methods, there is at least one group which shows significant differences in at least one morphological feature (Figure 3, Wilcoxon rank-sum test, p < 0.05, corrected). In fact, for most of the pairwise comparisons between staining methods we observed a large proportion of highly significant differences (Figure 3, Wilcoxon rank-sum test, p < 0.001, corrected). This suggests that morphologies obtained by different staining methods seldom agree with each other.
Figure 3. Proportion of significant differences between pairs of staining methods over all groups and morphological features. Zero means there is not any difference between the staining methods among the groups and one means all the groups are different. Computed using the Wilcoxon rank-sum test, corrected (Equation 2). Significant means p < 0.05, highly significant means p < 0.001.
We wondered if the biases in neuronal morphology between staining methods of the same type (e.g., Golgi vs. Golgi-Cox) were less than the biases in morphology between methods of a different type (e.g., Golgi vs. GFP). In fact comparisons between staining methods of the same class showed just as high a proportion of statistically significant differences as comparisons between methods of a different class: in both within-class and between-class comparisons 90% of tests performed were statistically significant (Figure 3). Thus, even morphologies obtained by similar methods can show strong biases due to experimental choices.
Given this preponderance of variability related to the staining method, we sought to understand which morphological features in particular had the strongest biases. In order to examine this we computed the average difference in each feature between each pair of staining methods, averaged over all groups for that comparison. This analysis shows that in general the total length, number of branches, and the length of segments show the strongest biases related to the staining method (Figure 4). Using a permutation test to determine the statistical significance of the average effect, we observed that 76% of average effects within these three features were significant. While features related to angles of the dendritic tree show weaker effects—only 32% of average effects in these features were significant. This suggests that features related to the coverage of the dendritic tree are most affected by the choice of staining method.
Figure 4. Pairwise average effect sizes for six morphological features. Upper right entries show statistical significance of differences (Equation 3). White squares represent no comparison, light gray squares represent a non-significant difference, gray squares represent a significant difference (p < 0.05), and black squares represent a highly significant difference (p < 0.001). To compute the significance level the average absolute difference in morphological features is compared with a null-distribution generated through permutation. Lower left entries show average difference in each feature between the two corresponding methods.
An omission from these analyses is the possible confounding effect that the rodent strain may have on neuron morphology (Rodriguez et al., 1999; Routh et al., 2009). There is less available data that we could use for matching. Nonetheless, we also analyzed the effect of the staining method on data matched also by strain. This resulted in six comparison groups. In this analysis the staining method biases are of similar significance and size in comparison to the analysis presented above (Supplementary Figures 1, 2). Thus, the biases we observe in morphology due to the staining method do not appear to be explained by reported differences in neuron morphology between rodent strains.
A caveat of the available data for our analysis is that it cannot fully separate the effect of the staining method from other laboratory-fixed effects. The ideal dataset to uniquely identify the effect of the staining method would be to have one lab perform the same experiments but with different staining methods. There are a few instances in which such a comparison was made. However, while the data we have thus exhibits a threat to causal validity (Pearl, 2009), we do have sufficient data to estimate how many neurons would be required to estimate such an artifact. To do this we performed a power analysis of the Wilcoxon rank-sum test through resampling. For most morphological features, we found that an effect size of d = 0.5 required 50 neurons/data points to detect the effect with probability 0.9 (1−β), assuming a type I error rate (α) of 0.05. For features that are defined for each dendrite segment, a single neuron would generally provide this much data. However, to ensure neuron-neuron variability is taken into account, a safer estimate is to assume at least 50 neurons are required. Thus, it seems likely that future versions of neuromorpho.org will soon be able to answer these questions with more precision.
It is important to accurately characterize neuron morphology for a number of reasons. Dendritic morphology determines the computations a neuron can perform, and has a role in circuit function and neurological disease (Agmon-Snir et al., 1998; Elston and Fujita, 2014; Šišková et al., 2014; Yang et al., 2017). Morphology varies by brain region, cell layer, species, and age (Scheibel and Jacobs, 2003; Elston, 2007; Spruston, 2008; Elston and Fujita, 2014). It thus may provide clues as to the function of the region. For instance, Purkinje cells in the cerebellum and pyramidal cells in cortex may provide striking examples of a structure-function relation (Stein and Glickstein, 1992; Körding and König, 2001; Guerguiev et al., 2017). And distinct morphological features affect functional properties differently. For instance, features to do with dendrite diameter may affect electrophysiological properties more than branching angle. Characterizing the morphology specific to brain region, species, etc. is thus important. This is most cleanly identified when the same experimental methodology is used over different brain regions. For instance, Jacobs and Scheibel studied dendritic variation in primate cortical pyramidal cells with the Golgi technique (Scheibel and Jacobs, 2003). Elston and colleagues studied thousands of individually injected cells from multiple cortical areas in a singe hemisphere, replicating the studies in age/sex/hemisphere matched brains within a species and across species (Elston et al., 2001; Elston, 2007). Yet many brain regions and cell types have not been analyzed in this form. Studying variability in morphology by brain region and cell type must typically be performed with data collected from many different methods. Before conclusions can be made, the bias of the methodology must therefore be established.
Here we focused on the correlation of staining methods on measured neuron morphology. We showed a significant difference between neurons that were extracted from the same region, species, gender distribution, and age but with different staining methods. Although this analysis was focused on the staining method, a similar approach could be taken to study the effect of other methodological details such as the reconstruction software or microscopy method. Understanding the source of these artifacts is necessary for us to have an accurate picture of the variation of neurons in the brain.
There are a number of explanations for biases in morphology related to staining methods. First, there may be procedural differences between laboratories, coming from preferences for particular sub-regions or cell types or other preparation details not reported. Indeed previous studies show this is a large source of variability (Scorcioni et al., 2004). Large differences in morphology can exist within a small region, e.g., visuotopic variation within visual cortex and age (Elston, 2003, 2007; Elston and Fujita, 2014). As such, we may expect significant biases to be related to non-staining related signals.
Second, there are methodological biases related to the 3D or planar reconstruction of neuron morphology. To produce a 3D reconstruction of a neuron, we need to fix a direction for the slicing the specimen and choose the thickness of the slices. The staining methods may set a limit on the slice thickness. For instance, when using GFP, neurons are often imaged through confocal microscopy. This sets a bound for the slice thickness, which may affect morphometrics (Rodriguez et al., 2003; Ke et al., 2013). Shrinkage of the neuron during the fixation can also affect measured morphology (Grace and Llinás, 1985). These biases most likely affect the local morphological features, such as angles; the global features, such as total length, are likely less affected. Yet we observed larger biases in global features. This may suggest that biases related to 3D reconstruction are minimal.
Third, there may exist differences caused by other methodological details that happen to be correlated with the staining method, not because the method goes in hand with the staining method itself, but just by chance (or cultural heritage) in the data we analyzed. For example if the objective type used in the microscopy correlates with different staining methods then that would be a potential confound. However, by performing the same comparison over lab groups and brain regions, we mitigate these confounding effects to some extent, and thus better measure differences that are particular to the staining method. But these other explanations can not be ruled out entirely without more controlled comparisons. This is challenging, even with a large database such as neuromorpho.org. Our power analysis demonstrates how much data would be needed to cleanly address these questions. Ultimately, only a clean experiment with a proper random assignment strategy could produce causal certainty.
Our results are consistent with one mechanistic account by which biases are created from the staining method, and not other potential confounds: different molecule size of agents used in different staining methods target different parts of a neuron. If different staining methods capture different parts of the morphology then we would expect strongest artifacts to be observed between features to do with the amount of dendritic tree described, e.g., total length, dimension, number of branches, etc, and smaller artifacts for more local geometric properties like the branching angles. If, alternatively, the biases observed in morphology were due to staining methods/labs targeting different neuronal subpopulations within a given brain region, then we may expect stronger artifacts to also be observed in local parameters such as branching angle. We do not observe strong artifacts in these parameters. A more careful modeling approach that takes generated neuron morphologies and subsamples them according to a staining model may be able to give a more precise account of the type of biases we may expect due to the staining method, and thus this interpretation could be better tested.
The feature set used here is often used as a basis of cell classification (Vasques et al., 2016). In this regard, our results suggest the need to standardize and carefully characterize these artifacts–after all, such biases could have massive effects on the results of clustering methods used for cell type identification. Alternatively, although some features are affected by different staining method, there are some features that are only weakly affected by the method (Figure 4). It would thus be possible to use features that vary most by cell type and least by staining method as the basis of classification or clustering. This should allow combining our findings with those of previous classification approaches to make the procedures robust to the details of the staining method.
Our analysis tells a cautionary tale about the progressively more popular combination of data sets across labs. Fully characterizing neuronal morphology and its relation to function relies on the generation and analysis of vast amounts of data. Across neuroscience, collaborative efforts across institutions are studying morphology (e.g., Churchland, 2017). Amongst the wealth of datasets available, the need for understanding variability due to the data generation process is important for drawing inferences and analyzing data across disparate sources. This problem is becoming widespread in neuroscience where electrophysiological, molecular, and morphological data are now routinely shared.
The datasets analyzed for this study can be found in repository neuromorpho (version 7.4) http://neuromorpho.org. The code for performing the analysis is available at https://github.com/BonsaiNet/Staining-methods-and-morphologies.
RF and KK designed the study. RF and BL performed the analysis. RF, BL, and KK wrote the manuscript.
The authors declare that the research was conducted in the absence of any commercial or financial relationships that could be construed as a potential conflict of interest.
We are grateful to people who helped us to perform this work: David Rolnick for forming the initial formulation; Giorgio Ascoli and Shreejoy Tripathy for reading through the manuscript and providing useful feedback; Samantha Ing-Esteves for valuable ideas and conversation; and the organizers of Neuroinformatics 2018 (http://www.neuroinformatics2018.org/) and CCN 2018 (https://ccneuro.org/2018/default.asp) meetings, where versions of this work were presented. The work of RF was part of his Ph.D. program at Sharif University of Technology under supervision of Dr. Morteza Fotouhi. The authors are grateful for NIH funding (R01MH103910).
The Supplementary Material for this article can be found online at: https://www.frontiersin.org/articles/10.3389/fninf.2019.00036/full#supplementary-material
Agmon-Snir, H., Carr, C. E., and Rinzel, J. (1998). The role of dendrites in auditory coincidence detection. Nature 393, 268–272. doi: 10.1038/30505
Alpar, A., Ueberham, U., Bruckner, M. K., Seeger, G., Arendt, T., and Gartner, U. (2006). Different dendrite and dendritic spine alterations in basal and apical arbors in mutant human amyloid precursor protein transgenic mice. Brain Res. 1099, 189–198. doi: 10.1016/j.brainres.2006.04.109
Anstotz, M., Huang, H., Marchionni, I., Haumann, I., Maccaferri, G., and Lubke, J. H. (2016). Developmental profile, morphology, and synaptic connectivity of Cajal-Retzius cells in the postnatal mouse hippocampus. Cereb. Cortex 26, 855–872. doi: 10.1093/cercor/bhv271
Arisi, G. M., and Garcia-Cairasco, N. (2007). Doublecortin-positive newly born granule cells of hippocampus have abnormal apical dendritic morphology in the pilocarpine model of temporal lobe epilepsy. Brain Res. 1165, 126–134. doi: 10.1016/j.brainres.2007.06.037
Ascoli, G. A. (2006). Mobilizing the base of neuroscience data: the case of neuronal morphologies. Nat. Rev. Neurosci. 7, 318–324. doi: 10.1038/nrn1885
Bannister, N. J., and Larkman, A. U. (1995). Dendritic morphology of CA1 pyramidal neurones from the rat hippocampus: I. Branching patterns. J. Comp. Neurol. 360, 150–160. doi: 10.1002/cne.903600111
Beguin, S., Crepel, V., Aniksztejn, L., Becq, H., Pelosi, B., Pallesi-Pocachard, E., et al. (2013). An epilepsy-related ARX polyalanine expansion modifies glutamatergic neurons excitability and morphology without affecting GABAergic neurons development. Cereb. Cortex 23, 1484–1494. doi: 10.1093/cercor/bhs138
Beining, M., Jungenitz, T., Radic, T., Deller, T., Cuntz, H., Jedlicka, P., et al. (2017). Adult-born dentate granule cells show a critical period of dendritic reorganization and are distinct from developmentally born cells. Brain Struct. Funct. 222, 1427–1446. doi: 10.1007/s00429-016-1285-y
Belnoue, L., Malvaut, S., Ladevèze, E., Abrous, D. N., and Koehl, M. (2016). Plasticity in the olfactory bulb of the maternal mouse is prevented by gestational stress. Sci. Rep. 6:37615. doi: 10.1038/srep37615
Benavides-Piccione, R., Hamzei-Sichani, F., Ballesteros-Yáñez, I., DeFelipe, J., and Yuste, R. (2005). Dendritic size of pyramidal neurons differs among mouse cortical regions. Cereb. Cortex 16, 990–1001. doi: 10.1093/cercor/bhj041
Bergstrom, H. C., Smith, R. F., Mollinedo, N. S., and McDonald, C. G. (2010). Chronic nicotine exposure produces lateralized, age-dependent dendritic remodeling in the rodent basolateral amygdala. Synapse 64, 754–764. doi: 10.1002/syn.20783
Bezchlibnyk, Y. B., Stone, S. S., Hamani, C., and Lozano, A. M. (2017). High frequency stimulation of the infralimbic cortex induces morphological changes in rat hippocampal neurons. Brain Stimul. 10, 315–323. doi: 10.1016/j.brs.2016.11.013
Boillot, M., Lee, C. Y., Allene, C., Leguern, E., Baulac, S., and Rouach, N. (2016). LGI1 acts presynaptically to regulate excitatory synaptic transmission during early postnatal development. Sci. Rep. 6:21769. doi: 10.1038/srep21769
Burton, S. D., and Urban, N. N. (2015). Rapid feedforward inhibition and asynchronous excitation regulate granule cell activity in the mammalian main olfactory bulb. J. Neurosci. 35, 14103–14122. doi: 10.1523/JNEUROSCI.0746-15.2015
Carim-Todd, L., Bath, K. G., Fulgenzi, G., Yanpallewar, S., Jing, D., Barrick, C. A., et al. (2009). Endogenous truncated TrkB.T1 receptor regulates neuronal complexity and TrkB kinase receptor function in vivo. J. Neurosci. 29, 678–685. doi: 10.1523/JNEUROSCI.5060-08.2009
Carnevale, N. T., Tsai, K. Y., Claiborne, B. J., and Brown, T. H. (1997). Comparative electrotonic analysis of three classes of rat hippocampal neurons. J. Neurophysiol. 78, 703–720. doi: 10.1152/jn.1997.78.2.703
Carrel, D., Hernandez, K., Kwon, M., Mau, C., Trivedi, M. P., Brzustowicz, L. M., et al. (2015). Nitric oxide synthase 1 adaptor protein, a protein implicated in schizophrenia, controls radial migration of cortical neurons. Biol. Psychiatry 77, 969–978. doi: 10.1016/j.biopsych.2014.10.016
Carter, M., and Shieh, J. C. (2015). Guide to Research Techniques in Neuroscience. Burlington, MA: Academic Press.
Cazorla, M., Shegda, M., Ramesh, B., Harrison, N. L., and Kellendonk, C. (2012). Striatal D2 receptors regulate dendritic morphology of medium spiny neurons via Kir2 channels. J. Neurosci. 32, 2398–2409. doi: 10.1523/JNEUROSCI.6056-11.2012
Chen, J. R., Wang, B. N., Tseng, G. F., Wang, Y. J., Huang, Y. S., and Wang, T. J. (2014). Morphological changes of cortical pyramidal neurons in hepatic encephalopathy. BMC Neurosci 15:15. doi: 10.1186/1471-2202-15-15
Chen, X., Cho, D.-B., and Yang, P.-C. (2010). Double staining immunohistochemistry. Nat. Am. J. Med. Sci. 2:241. doi: 10.4297/najms.2010.2241
Chen, Y. P., and Chiao, C. C. (2014). Spatial distribution of excitatory synapses on the dendrites of ganglion cells in the mouse retina. PLoS ONE 9:e86159. doi: 10.1371/journal.pone.0086159
Churchland (2017). An international laboratory for systems and computational neuroscience. Neuron 96, 1213–1218. doi: 10.1016/j.neuron.2017.12.013
Cohen, L., Koffman, N., Meiri, H., Yarom, Y., Lampl, I., and Mizrahi, A. (2013). Time-lapse electrical recordings of single neurons from the mouse neocortex. Proc. Natl. Acad. Sci. U.S.A. 110, 5665–5670. doi: 10.1073/pnas.1214434110
Donohue, D. E., and Ascoli, G. A. (2011). Automated reconstruction of neuronal morphology: an overview. Brain Res. Rev. 67, 94–102. doi: 10.1016/j.brainresrev.2010.11.003
Dougherty, K. A., Islam, T., and Johnston, D. (2012). Intrinsic excitability of CA1 pyramidal neurones from the rat dorsal and ventral hippocampus. J. Physiol. 590, 5707–5722. doi: 10.1113/jphysiol.2012.242693
Druckmann, S., Feng, L., Lee, B., Yook, C., Zhao, T., Magee, J. C., and Kim, J. (2014). Structured synaptic connectivity between hippocampal regions. Neuron 81, 629–640. doi: 10.1016/j.neuron.2013.11.026
D'Souza, R. D., Meier, A. M., Bista, P., Wang, Q., and Burkhalter, A. (2016). Recruitment of inhibition and excitation across mouse visual cortex depends on the hierarchy of interconnecting areas. Elife 5:e19332. doi: 10.7554/eLife.19332
Elston, G. N. (2003). Cortex, cognition and the cell: new insights into the pyramidal neuron and prefrontal function. Cereb. Cortex 13, 1124–1138. doi: 10.1093/cercor/bhg093
Elston, G. N. (2007). Specialization of the neocortical pyramidal cell during primate evolution. Evol. Nervous Syst. 4, 191–242. doi: 10.1016/B0-12-370878-8/00164-6
Elston, G. N., Benavides-Piccione, R., and DeFelipe, J. (2001). The pyramidal cell in cognition: a comparative study in human and monkey. J. Neurosci. 21:RC163. doi: 10.1523/JNEUROSCI.21-17-j0002.2001
Elston, G. N., and Fujita, I. (2014). Pyramidal cell development: postnatal spinogenesis, dendritic growth, axon growth, and electrophysiology. Front. Neuroanat. 8:78. doi: 10.3389/fnana.2014.00078
Elston, G. N., Tweedale, R., and Rosa, M. G. (1999). Cortical integration in the visual system of the macaque monkey: large-scale morphological differences in the pyramidal neurons in the occipital, parietal and temporal lobes. Proc. R. Soc. Lond. B Biol. Sci. 266, 1367–1374. doi: 10.1098/rspb.1999.0789
Fukunaga, I., Berning, M., Kollo, M., Schmaltz, A., and Schaefer, A. T. (2012). Two distinct channels of olfactory bulb output. Neuron 75, 320–329. doi: 10.1016/j.neuron.2012.05.017
Golding, N. L., Mickus, T. J., Katz, Y., Kath, W. L., and Spruston, N. (2005). Factors mediating powerful voltage attenuation along CA1 pyramidal neuron dendrites. J. Physiol. 568, 69–82. doi: 10.1113/jphysiol.2005.086793
Grace, A. A., and Llinás, R. (1985). Morphological artifacts induced in intracellularly stained neurons by dehydration: circumvention using rapid dimethyl sulfoxide clearing. Neuroscience 16, 461–475. doi: 10.1016/0306-4522(85)90018-1
Groen, M. R., Paulsen, O., Perez-Garci, E., Nevian, T., Wortel, J., Dekker, M. P., et al. (2014). Development of dendritic tonic GABAergic inhibition regulates excitability and plasticity in CA1 pyramidal neurons. J. Neurophysiol. 112, 287–299. doi: 10.1152/jn.00066.2014
Guerguiev, J., Lillicrap, T. P., and Richards, B. A. (2017). Towards deep learning with segregated dendrites. Elife 6:e22901. doi: 10.7554/eLife.22901
Hamada, M. S., Goethals, S., de Vries, S. I., Brette, R., and Kole, M. H. (2016). Covariation of axon initial segment location and dendritic tree normalizes the somatic action potential. Proc. Natl. Acad. Sci. U.S.A. 113, 14841–14846. doi: 10.1073/pnas.1607548113
Hanani, M. (2012). Lucifer yellow - an angel rather than the devil. J. Cell. Mol. Med. 16, 22–31. doi: 10.1111/j.1582-4934.2011.01378.x
Henckens, M. J., van der Marel, K., van der Toorn, A., Pillai, A. G., Fernandez, G., Dijkhuizen, R. M., et al. (2015). Stress-induced alterations in large-scale functional networks of the rodent brain. Neuroimage 105, 312–322. doi: 10.1016/j.neuroimage.2014.10.037
Hoffmann, J. H., Meyer, H. S., Schmitt, A. C., Straehle, J., Weitbrecht, T., Sakmann, B., et al. (2015). Synaptic conductance estimates of the connection between local inhibitor interneurons and pyramidal neurons in layer 2/3 of a cortical column. Cereb. Cortex 25, 4415–4429. doi: 10.1093/cercor/bhv039
Jacobs, B., Schall, M., Prather, M., Kapler, E., Driscoll, L., Baca, S., et al. (2001). Regional dendritic and spine variation in human cerebral cortex: a quantitative golgi study. Cereb. Cortex 11, 558–571. doi: 10.1093/cercor/11.6.558
Jiang, X., Shen, S., Cadwell, C. R., Berens, P., Sinz, F., Ecker, A. S., et al. (2015). Principles of connectivity among morphologically defined cell types in adult neocortex. Science 350:aac9462. doi: 10.1126/science.aac9462
Jones, A. R., Overly, C. C., and Sunkin, S. M. (2009). The allen brain atlas: 5 years and beyond. Nat. Rev. Neurosci. 10:821. doi: 10.1038/nrn2722
Ke, M. T., Fujimoto, S., and Imai, T. (2013). SeeDB: a simple and morphology-preserving optical clearing agent for neuronal circuit reconstruction. Nat. Neurosci. 16, 1154–1161. doi: 10.1038/nn.3447
Klenowski, P., Wright, S., Mu, E., Noakes, P., Lavidis, N., Bartlett, S., et al. (2017). Investigating methodological differences in the assessment of dendritic morphology of basolateral amygdala principal Neurons—A comparison of Golgi–Cox and neurobiotin electroporation techniques. Brain Sci. 7:165. doi: 10.3390/brainsci7120165
Kole, M. H. (2011). First node of Ranvier facilitates high-frequency burst encoding. Neuron 71, 671–682. doi: 10.1016/j.neuron.2011.06.024
Kole, M. H., Brauer, A. U., and Stuart, G. J. (2007). Inherited cortical HCN1 channel loss amplifies dendritic calcium electrogenesis and burst firing in a rat absence epilepsy model. J. Physiol. 578, 507–525. doi: 10.1113/jphysiol.2006.122028
Kole, M. H., Costoli, T., Koolhaas, J. M., and Fuchs, E. (2004). Bidirectional shift in the cornu ammonis 3 pyramidal dendritic organization following brief stress. Neuroscience 125, 337–347. doi: 10.1016/j.neuroscience.2004.02.014
Kole, M. H., Hallermann, S., and Stuart, G. J. (2006). Single Ih channels in pyramidal neuron dendrites: properties, distribution, and impact on action potential output. J. Neurosci. 26, 1677–1687. doi: 10.1523/JNEUROSCI.3664-05.2006
Körding, K. P., and König, P. (2001). Supervised and unsupervised learning with two sites of synaptic integration. J. Comput. Neurosci. 11, 207–215. doi: 10.1023/A:1013776130161
Koyama, Y. (2013). The unending fascination with the golgi method. OA Anatomy 1:24. doi: 10.13172/2052-7829-1-3-848
Krishnaswamy, A., Yamagata, M., Duan, X., Hong, Y. K., and Sanes, J. R. (2015). Sidekick 2 directs formation of a retinal circuit that detects differential motion. Nature 524, 466–470. doi: 10.1038/nature14682
Lee, S. H., Marchionni, I., Bezaire, M., Varga, C., Danielson, N., Lovett-Barron, M., et al. (2014). Parvalbumin-positive basket cells differentiate among hippocampal pyramidal cells. Neuron 82, 1129–1144. doi: 10.1016/j.neuron.2014.03.034
Longordo, F., To, M. S., Ikeda, K., and Stuart, G. J. (2013). Sublinear integration underlies binocular processing in primary visual cortex. Nat. Neurosci. 16, 714–723. doi: 10.1038/nn.3394
Malik, R., Dougherty, K. A., Parikh, K., Byrne, C., and Johnston, D. (2016). Mapping the electrophysiological and morphological properties of CA1 pyramidal neurons along the longitudinal hippocampal axis. Hippocampus 26, 341–361. doi: 10.1002/hipo.22526
Marshall, J., Molloy, R., Moss, G. W., Howe, J. R., and Hughes, T. E. (1995). The jellyfish green fluorescent protein: a new tool for studying ion channel expression and function. Neuron 14, 211–215. doi: 10.1016/0896-6273(95)90279-1
Martone, M. E., Zhang, S., Gupta, A., Qian, X., He, H., Price, D. L., et al. (2003). The cell-centered database: a database for multiscale structural and protein localization data from light and electron microscopy. Neuroinformatics 1, 379–395. doi: 10.1385/NI:1:4:379
Megias, M., Emri, Z., Freund, T. F., and Gulyas, A. I. (2001). Total number and distribution of inhibitory and excitatory synapses on hippocampal CA1 pyramidal cells. Neuroscience 102, 527–540. doi: 10.1016/S0306-4522(00)00496-6
Mendez, P., Pazienti, A., Szabo, G., and Bacci, A. (2012). Direct alteration of a specific inhibitory circuit of the hippocampus by antidepressants. J. Neurosci. 32, 16616–16628. doi: 10.1523/JNEUROSCI.1720-12.2012
Michaelsen, K., Murk, K., Zagrebelsky, M., Dreznjak, A., Jockusch, B. M., Rothkegel, M., et al. (2010). Fine-tuning of neuronal architecture requires two profilin isoforms. Proc. Natl. Acad. Sci. U.S.A. 107, 15780–15785. doi: 10.1073/pnas.1004406107
Mulholland, P. J., Spencer, K. B., Hu, W., Kroener, S., and Chandler, L. J. (2015). Neuroplasticity of A-type potassium channel complexes induced by chronic alcohol exposure enhances dendritic calcium transients in hippocampus. Psychopharmacology 232, 1995–2006. doi: 10.1007/s00213-014-3835-4
Nato, G., Caramello, A., Trova, S., Avataneo, V., Rolando, C., Taylor, V., et al. (2015). Striatal astrocytes produce neuroblasts in an excitotoxic model of Huntington's disease. Development 142, 840–845. doi: 10.1242/dev.116657
Paavilainen, L., Edvinsson, A., Asplund, A., Hober, S., Kampf, C., Pontén, F., et al. (2010). The impact of tissue fixatives on morphology and antibody-based protein profiling in tissues and cells. J. Histochem. Cytochem. 58, 237–246. doi: 10.1369/jhc.2009.954321
Padival, M. A., Blume, S. R., and Rosenkranz, J. A. (2013). Repeated restraint stress exerts different impact on structure of neurons in the lateral and basal nuclei of the amygdala. Neuroscience 246, 230–242. doi: 10.1016/j.neuroscience.2013.04.061
Parekh, R., and Ascoli, G. A. (2013). Neuronal morphology goes digital: a research hub for cellular and system neuroscience. Neuron 77, 1017–1038. doi: 10.1016/j.neuron.2013.03.008
Parekh, R., and Ascoli, G. A. (2015). Quantitative investigations of axonal and dendritic arbors: development, structure, function, and pathology. Neuroscientist 21, 241–254. doi: 10.1177/1073858414540216
Pearl, J. (2009). Causality. New York, NY: Cambridge University Press. doi: 10.1017/CBO9780511803161
Platschek, S., Cuntz, H., Vuksic, M., Deller, T., and Jedlicka, P. (2016). A general homeostatic principle following lesion induced dendritic remodeling. Acta Neuropathol. Commun. 4:19. doi: 10.1186/s40478-016-0285-8
Poria, D., and Dhingra, N. K. (2015). Spontaneous oscillatory activity in rd1 mouse retina is transferred from ON pathway to OFF pathway via glycinergic synapse. J. Neurophysiol. 113, 420–425. doi: 10.1152/jn.00702.2014
Pyapali, G. K., Sik, A., Penttonen, M., Buzsaki, G., and Turner, D. A. (1998). Dendritic properties of hippocampal CA1 pyramidal neurons in the rat: intracellular staining in vivo and in vitro. J. Comp. Neurol. 391, 335–352. doi: 10.1002/(SICI)1096-9861(19980216)391:3<335::AID-CNE4>3.0.CO;2-2
Qin, L., Jing, D., Parauda, S., Carmel, J., Ratan, R. R., Lee, F. S., et al. (2014). An adaptive role for BDNF Val66Met polymorphism in motor recovery in chronic stroke. J. Neurosci. 34, 2493–2502. doi: 10.1523/JNEUROSCI.4140-13.2014
Quast, K. B., Ung, K., Froudarakis, E., Huang, L., Herman, I., Addison, A. P., et al. (2017). Developmental broadening of inhibitory sensory maps. Nat. Neurosci. 20, 189–199. doi: 10.1038/nn.4467
Revest, J. M., Dupret, D., Koehl, M., Funk-Reiter, C., Grosjean, N., Piazza, P. V., et al. (2009). Adult hippocampal neurogenesis is involved in anxiety-related behaviors. Mol. Psychiatry 14, 959–967. doi: 10.1038/mp.2009.15
Rihn, L. L., and Claiborne, B. J. (1990). Dendritic growth and regression in rat dentate granule cells during late postnatal development. Brain Res. Dev. Brain Res. 54, 115–124. doi: 10.1016/0165-3806(90)90071-6
Rodriguez, A., Ehlenberger, D., Kelliher, K., Einstein, M., Henderson, S. C., Morrison, J. H., et al. (2003). Automated reconstruction of three-dimensional neuronal morphology from laser scanning microscopy images. Methods 30, 94–105. doi: 10.1016/S1046-2023(03)00011-2
Rodriguez, I., Feinstein, P., and Mombaerts, P. (1999). Variable patterns of axonal projections of sensory neurons in the mouse vomeronasal system. Cell 97, 199–208. doi: 10.1016/S0092-8674(00)80730-8
Routh, B. N., Johnston, D., Harris, K., and Chitwood, R. A. (2009). Anatomical and electrophysiological comparison of ca1 pyramidal neurons of the rat and mouse. J. Neurophysiol. 102, 2288–2302. doi: 10.1152/jn.00082.2009
Sailor, K. A., Valley, M. T., Wiechert, M. T., Riecke, H., Sun, G. J., Adams, W., et al. (2016). Persistent structural plasticity optimizes sensory information processing in the olfactory bulb. Neuron 91, 384–396. doi: 10.1016/j.neuron.2016.06.004
Scheibel, A. B., and Jacobs, B. (2003). “Regional dendritic variation in primate cortical pyramidal cells,” in Cortical Areas, eds A. Schuez and R. Miller (London: CRC Press), 123–144.
Scorcioni, R., Lazarewicz, M. T., and Ascoli, G. A. (2004). Quantitative morphometry of hippocampal pyramidal cells: differences between anatomical classes and reconstructing laboratories. J. Comp. Neurol. 473, 177–193. doi: 10.1002/cne.20067
Scorcioni, R., Polavaram, S., and Ascoli, G. A. (2008). L-measure: a web-accessible tool for the analysis, comparison and search of digital reconstructions of neuronal morphologies. Nat. Protoc. 3:866. doi: 10.1038/nprot.2008.51
Scorza, C. A., Araujo, B. H., Leite, L. A., Torres, L. B., Otalora, L. F., Oliveira, M. S., et al. (2011). Morphological and electrophysiological properties of pyramidal-like neurons in the stratum oriens of Cornu ammonis 1 and Cornu ammonis 2 area of Proechimys. Neuroscience 177, 252–268. doi: 10.1016/j.neuroscience.2010.12.054
Šišková, Z., Justus, D., Kaneko, H., Friedrichs, D., Henneberg, N., Beutel, T., et al. (2014). Dendritic structural degeneration is functionally linked to cellular hyperexcitability in a mouse model of Alzheimer's disease. Neuron 84, 1023–1033. doi: 10.1016/j.neuron.2014.10.024
Soares-Cunha, C., Coimbra, B., Borges, S., Carvalho, M. M., Rodrigues, A. J., and Sousa, N. (2014). The motivational drive to natural rewards is modulated by prenatal glucocorticoid exposure. Transl. Psychiatry 4:e397. doi: 10.1038/tp.2014.45
Spruston, N. (2008). Pyramidal neurons: dendritic structure and synaptic integration. Nat. Rev. Neurosci. 9:206. doi: 10.1038/nrn2286
Staffend, N. A., and Meisel, R. L. (2011). DiOlistic labeling in fixed brain slices: phenotype, morphology, and dendritic spines. Curr. Protoc. Neurosci. Chapter 2:Unit 2.13. doi: 10.1002/0471142301.ns0213s55
Stein, J. F., and Glickstein, M. (1992). Role of the cerebellum in visual guidance of movement. Physiol. Rev. 72, 967–1017. doi: 10.1152/physrev.1992.72.4.967
Ster, J., Steuble, M., Orlando, C., Diep, T. M., Akhmedov, A., Raineteau, O., et al. (2014). Calsyntenin-1 regulates targeting of dendritic NMDA receptors and dendritic spine maturation in CA1 hippocampal pyramidal cells during postnatal development. J. Neurosci. 34, 8716–8727. doi: 10.1523/JNEUROSCI.0144-14.2014
Stockley, E. W., Cole, H. M., Brown, A. D., and Wheal, H. V. (1993). A system for quantitative morphological measurement and electrotonic modelling of neurons: three-dimensional reconstruction. J. Neurosci. Methods 47, 39–51. doi: 10.1016/0165-0270(93)90020-R
Sumbul, U., Song, S., McCulloch, K., Becker, M., Lin, B., Sanes, J. R., et al. (2014). A genetic and computational approach to structurally classify neuronal types. Nat. Commun. 5:3512. doi: 10.1038/ncomms4512
Suo, L., Lu, H., Ying, G., Capecchi, M. R., and Wu, Q. (2012). Protocadherin clusters and cell adhesion kinase regulate dendrite complexity through Rho GTPase. J. Mol. Cell Biol. 4, 362–376. doi: 10.1093/jmcb/mjs034
Swietek, B., Gupta, A., Proddutur, A., and Santhakumar, V. (2016). Immunostaining of biocytin-filled and processed sections for neurochemical markers. J. Vis. Exp. 18, 1–9. doi: 10.3791/54880
Tapia, J. C., Kasthuri, N., Hayworth, K., Schalek, R., Lichtman, J. W., Smith, S. J., et al. (2012). High contrast en bloc staining of neuronal tissue for field emission scanning electron microscopy. Nat. Protoc. 7:193. doi: 10.1038/nprot.2011.439
Tebaykin, D., Tripathy, S. J., Binnion, N., Li, B., Gerkin, R. C., and Pavlidis, P. (2017). Modeling sources of interlaboratory variability in electrophysiological properties of mammalian neurons. J. Neurophysiol. 119, 1329–1339. doi: 10.1152/jn.00604.2017
Travis, K., Ford, K., and Jacobs, B. (2005). Regional dendritic variation in neonatal human cortex: a quantitative golgi study. Dev. Neurosci. 27, 277–287. doi: 10.1159/000086707
Tripathy, S. J., Burton, S. D., Geramita, M., Gerkin, R. C., and Urban, N. N. (2015). Brain-wide analysis of electrophysiological diversity yields novel categorization of mammalian neuron types. J. Neurophysiol. 113, 3474–3489. doi: 10.1152/jn.00237.2015
Tyan, L., Chamberland, S., Magnin, E., Camire, O., Francavilla, R., David, L. S., et al. (2014). Dendritic inhibition provided by interneuron-specific cells controls the firing rate and timing of the hippocampal feedback inhibitory circuitry. J. Neurosci. 34, 4534–4547. doi: 10.1523/JNEUROSCI.3813-13.2014
Vaney, D. I., Azmitia, E. C, DeFelipe, J., Jones, E. G., Rakic, P., Ribak, C.E., et al. (2002). “Retinal neurons: cell types and coupled networks,” in Progress in Brain Research, 136, eds E. C. Azmitia, J. DeFelipe, E. G. Jones, P. Rakic, and C. E. Ribak (Elsevier), 239–254. doi: 10.1016/S0079-6123(02)36020-5
Vannini, E., Restani, L., Pietrasanta, M., Panarese, A., Mazzoni, A., Rossetto, O., et al. (2016). Altered sensory processing and dendritic remodeling in hyperexcitable visual cortical networks. Brain Struct. Funct. 221, 2919–2936. doi: 10.1007/s00429-015-1080-1
Vasques, X., Vanel, L., Villette, G., and Cif, L. (2016). Morphological neuron classification using machine learning. Front. Neuroanat. 10:102. doi: 10.3389/fnana.2016.00102
Watson, M. L. (1958). Staining of tissue sections for electron microscopy with heavy metals. J. Cell Biol. 4, 475–478. doi: 10.1083/jcb.4.4.475
Winkle, C. C., Olsen, R. H., Kim, H., Moy, S. S., Song, J., and Gupton, S. L. (2016). Trim9 deletion alters the morphogenesis of developing and adult-born hippocampal neurons and impairs spatial learning and memory. J. Neurosci. 36, 4940–4958. doi: 10.1523/JNEUROSCI.3876-15.2016
Xi, X. Z., and Xu, Z. C. (1996). The effect of neurobiotin on membrane properties and morphology of intracellularly labeled neurons. J. Neurosci. Methods 65, 27–32. doi: 10.1016/0165-0270(95)00140-9
Yang, S.-A., Yoon, J., Kim, K., and Park, Y. (2017). Measurements of morphological and biophysical alterations in individual neuron cells associated with early neurotoxic effects in Parkinson's disease. Cytometry A 91, 510–518. doi: 10.1002/cyto.a.23110
Keywords: dendritic morphology, staining method, rodent neuroanatomy, neuroinformatics, golgi method, immunostaining, fluorescence microscopy
Citation: Farhoodi R, Lansdell BJ and Kording KP (2019) Quantifying How Staining Methods Bias Measurements of Neuron Morphologies. Front. Neuroinform. 13:36. doi: 10.3389/fninf.2019.00036
Received: 01 September 2018; Accepted: 25 April 2019;
Published: 21 May 2019.
Edited by:
Arjen van Ooyen, VU University Amsterdam, NetherlandsReviewed by:
Guy Elston, University of the Sunshine Coast, AustraliaCopyright © 2019 Farhoodi, Lansdell and Kording. This is an open-access article distributed under the terms of the Creative Commons Attribution License (CC BY). The use, distribution or reproduction in other forums is permitted, provided the original author(s) and the copyright owner(s) are credited and that the original publication in this journal is cited, in accordance with accepted academic practice. No use, distribution or reproduction is permitted which does not comply with these terms.
*Correspondence: Roozbeh Farhoodi, cm9vemJlaGZhcmhvb2RpQGdtYWlsLmNvbQ==
†These authors share first authorship
Disclaimer: All claims expressed in this article are solely those of the authors and do not necessarily represent those of their affiliated organizations, or those of the publisher, the editors and the reviewers. Any product that may be evaluated in this article or claim that may be made by its manufacturer is not guaranteed or endorsed by the publisher.
Research integrity at Frontiers
Learn more about the work of our research integrity team to safeguard the quality of each article we publish.