- 1S. Anna Institute and Research in Advanced Neurorehabilitation (RAN) Crotone, Crotone, Italy
- 2Neuroimaging Unit, IBFM-CNR, Catanzaro, Italy
- 3Neuroscience Research Centre, University Magna Græcia, Catanzaro, Italy
Background: Technology-supported rehabilitation is emerging as a solution to support therapists in providing a high-intensity, repetitive and task-specific treatment, aimed at improving stroke recovery. End-effector robotic devices are known to positively affect the recovery of arm functions, however there is a lack of evidence regarding exoskeletons. This paper evaluates the impact of cerebral lesion load on the response to a validated robotic-assisted rehabilitation protocol.
Methods: Fourteen hemiparetic patients were assessed in a within-subject design (age 66.9 ± 11.3 years; 10 men and 4 women). Patients, in post-acute phase, underwent 7 weeks of bilateral arm training assisted by an exoskeleton robot combined with a conventional treatment (consisting of simple physical activity together with occupational therapy). Clinical and neuroimaging evaluations were performed immediately before and after rehabilitation treatments. Fugl-Meyer (FM) and Motricity Index (MI) were selected to measure primary outcomes, i.e., motor function and strength. Functional independance measure (FIM) and Barthel Index were selected to measure secondary outcomes, i.e., daily living activities. Voxel-based lesion symptom mapping (VLSM) was used to determine the degree of cerebral lesions associated with motor recovery.
Results: Robot-assisted rehabilitation was effective in improving upper limb motor function recovery, considering both primary and secondary outcomes. VLSM detected that lesion load in the superior region of the corona radiata, internal capsule and putamen were significantly associated with recovery of the upper limb as defined by the FM scores (p-level < 0.01).
Conclusions: The probability of functional recovery from stroke by means of exoskeleton robotic rehabilitation relies on the integrity of specific subcortical regions involved in the primary motor pathway. This is consistent with previous evidence obtained with conventional neurorehabilitation approaches.
Introduction
Several systematic and meta-analytic reviews have confirmed that robotic-assisted devices elicit robust motor recovery in patients with stroke, mainly in relation to the upper limb intervention (Masiero et al., 2007; Bertani et al., 2017; Lo et al., 2017). Early research on robotic therapy for the upper limb was based on end-effector robots, which hold the patient’s hand or forearm at one point and generate forces at the interface. Recently this field of study has shifted towards an exoskeleton device, which overcomes many of the inherent limitations of end-effector robots (Pignolo, 2009; Lo and Xie, 2012). Compared to conventional therapy, exoskeletons have the potential to provide intensive rehabilitation consistently for a longer duration and irrespective of the skills and fatigue level of the therapist (Huang and Krakauer, 2009; Lo and Xie, 2012).
The Automatic Recovery Arm Motility Integrated System (ARAMIS) is a concept robot and prototype for the neurorehabilitation of the paretic upper limb developed at the Institute S. Anna—Crotone, Italy. ARAMIS was designed with two computer-controlled, symmetric and interacting exoskeletons, which compensate for the inadequate strength and accuracy of the paretic arm movements and the effect of gravity during rehabilitation. The basic idea is to exploit proprioceptive inputs using passive, repetitive, interactive, high-intensive bilateral movement training, which has been demonstrated to enhance motor recovery in stroke patients (Stinear et al., 2008; Choo et al., 2015; Saleh et al., 2017; Gandolfi et al., 2018). This device has been widely validated (Colizzi et al., 2009; Dolce et al., 2009; Pignolo et al., 2012) with respect to conventional neurorehabilitation approaches, demonstrating high degree of upper limb recovery as assessed by the Fugl-Meyer (FM) scale (Fugl-Meyer et al., 1975). The FM is a performance-based impairment index designed to assess motor functioning, balance, sensation and joint functioning in patients with post-stroke hemiplegia. Overall, FM together with the Modified Ashworth Scale (MAS) and functional independance measure (FIM) (Keith et al., 1987), are the most reliable clinical scales employed to unravel motor recovering after robotic-treatment in stroke patients (Bertani et al., 2017).
Despite this large amount of evidence confirming the effectiveness and robustness of robotic-assisted rehabilitation in promoting motor recovery the underlying pathophysiology is still unclear. In fact, some biomarkers have been effectively demonstrated to predict therapeutic response or recovery following stroke (Burke Quinlan et al., 2015). Generally, research has focused on the neural substrate of motor recovery obtained with a conventional neurorehabilitation approach (Shelton and Reding, 2001; Murphy and Corbett, 2009; Carrera and Tononi, 2014; Choo et al., 2015; Lee et al., 2017; Siegel et al., 2018), whereas little attention has been paid to robotic-assisted therapy with exoskeleton devices (Formaggio et al., 2013; Fan et al., 2016; Gandolfi et al., 2018). Overall, preservation of the corticospinal tract is considered as a hallmark for good recovery of impaired motor function in patients with brain injury (Hendricks et al., 2002; Swayne et al., 2008; Stinear, 2010). Within this pathway there are several critical hubs that have been associated with functional recovery after conventional therapy. Mounting evidence from functional magnetic resonance imaging (fMRI), diffusion tensor imaging (DTI) as well as resting-state functional connectivity studies have demonstrated that the increased activity in ipsilateral primary motor cortex and the morphological integrity of the posterior limb of the capsula interna predict the positive clinical outcome (Shelton and Reding, 2001; Stinear et al., 2012; Stinear and Ward, 2013; Favre et al., 2014; Rehme et al., 2015). Moreover, lesions in the globus pallidus, and putamen (together with corona radiata, internal capsule) were also associated with poor recovery (Lee et al., 2017).
This study, thus, assesses the effects of lesion location on the response to rehabilitation training obtained with an exoskeleton robot device. The aim is to expand knowledge of the neural basis of stroke rehabilitation and the prognosis of upper limb disorders.
Materials and Methods
Subjects
We enrolled patients who met the criteria for a first attack of sub-cortical ischemic stroke recruited at the Sant’Anna Rehabilitation Center. From an initial cohort of 108 subacute hemiplegic patients, we enrolled only those who fulfilled the following criteria: (i) unilateral stroke, (ii) ability to follow verbal instructions, and (iii) right-handed patients. Exclusion criteria were (1) bilateral impairment; severe sensory deficits in the paretic upper limb; (2) pregnancy, epilepsy, aphasia, cognitive impairment (Mini Mental State Evaluation, MMSE < 24) or behavioral dysfunction that would influence the patient’s ability to comprehend or participate in the treatment; (3) botulinum toxin injections or other medication influencing the function of the upper-limb; (4) inability to provide informed consent and (5) and/or pacemakers or other metallic implants incompatible with the 3T MRI scanner. From the initial cohort, 34 patients were selected for the rehabilitation program (Figure 1).
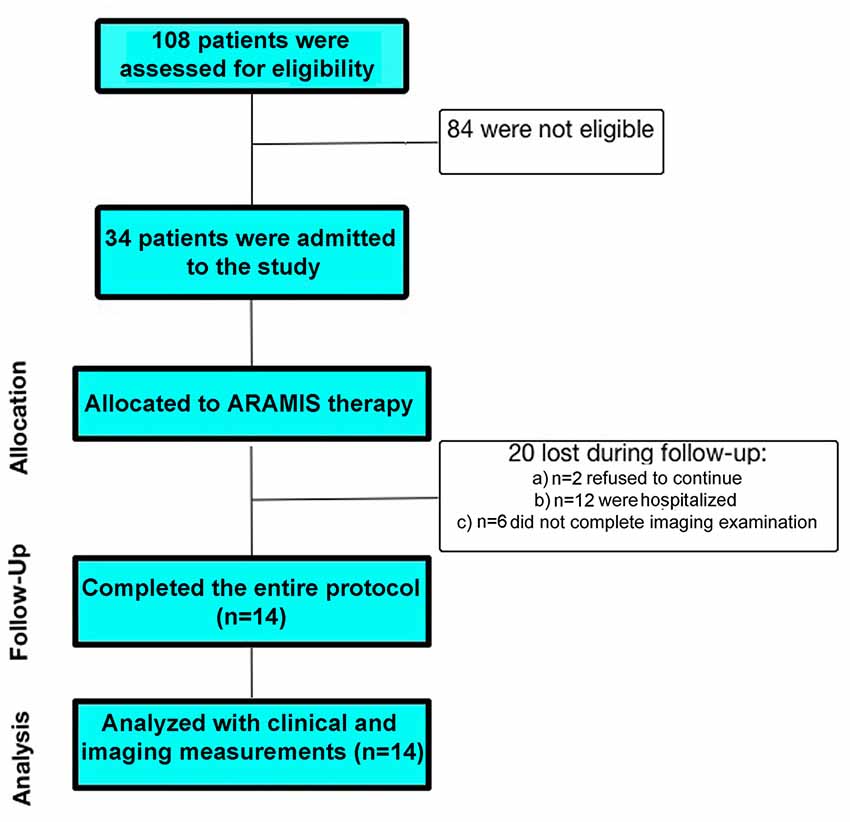
Figure 1. Flow diagram of participant recruitment and participation in the study: stroke patients participated in an individualized robotic-assisted neurorehabilitation program (ARAMIS system).
All the participants gave written informed consent. The study was approved by the Ethical Committee of the University “Magna Graecia” of Catanzaro, according to the Helsinki Declaration.
All patients completed an extensive series of clinical tests that were administered by an experienced physician who was blind to any other result. The degree of disability during daily living activities was assessed with the Barthel Index (Collin et al., 1988) and the motor strength of the upper-limb was assessed with the Motricity Index (MI; Collin and Wade, 1990). Patients’ synergistic motor control of the paretic arm was assessed with the upper extremity (UE) section of the FM (FM-UE; Lindmark, 1988). Further measures included the FIM and the Trunk Control Test (TCT; Franchignoni et al., 1997).
ARAMIS Hard/Software Structure
The robotic-assisted rehabilitation performed through the ARAMIS device has been described elsewhere (Colizzi et al., 2009; Dolce et al., 2009; Pignolo et al., 2012). The ARAMIS framework is a fully integrated set of software that enables the therapist to program and manage the rehabilitation procedures. Briefly, the robotic platform includes two fully-motorized 6 DOF symmetric exoskeletons (Figure 2). Kinematic and dynamic data are jointly continuously acquired and stored by the control system, which evaluates the weight torque and compensates for it by controlling each upper limb posture and the strength delivered by the patient to the exoskeleton. Movements are, therefore, supported by a drive motor, which adjusts its strength on a step-by-step basis.
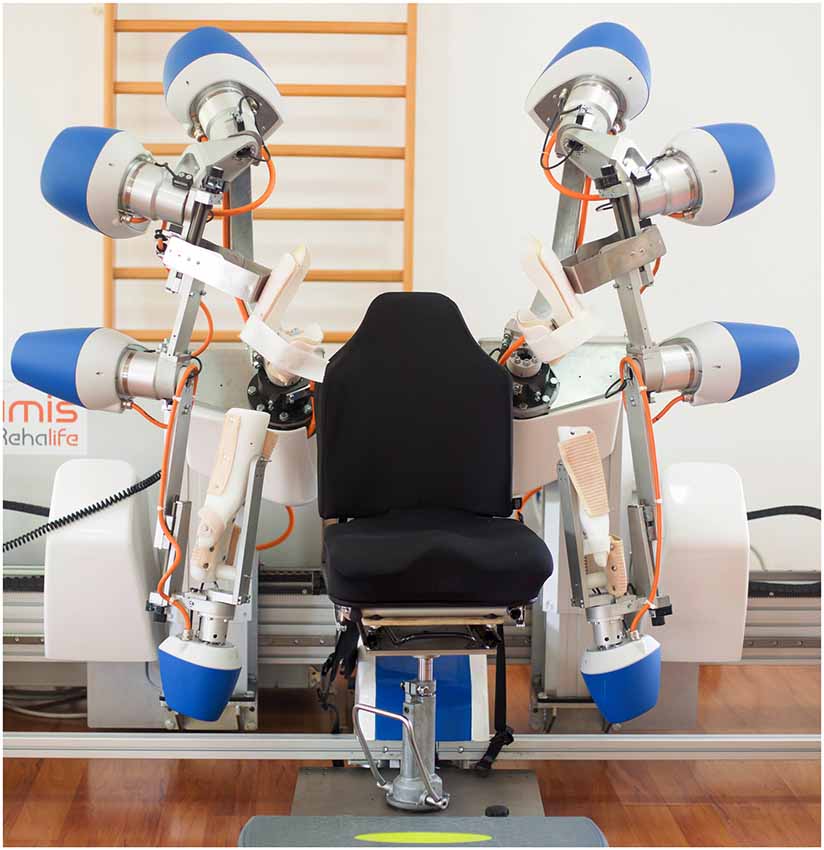
Figure 2. The robotic-assisted device called Automatic Recovery Arm Motility Integrated System (ARAMIS).
Each exoskeleton can record (motion capture) the movements of the unaffected arm and the patient is requested to replicate each single movement by the paretic arm in synchronous or asynchronous modalities depending on the exercise typology or training program, with continuous compensation for the paretic arm’s inadequate strength and accuracy. ARAMIS-assisted rehabilitation is possible in three different modalities: (1) asynchronous: the patient wears both exoskeletons and uses the unaffected arm to perform pre-programmed exercises that are replicated by the paretic arm supported by its own exoskeleton; (2) synchronous: the unaffected arm paces the movements to be replicated synchronously and with the same physical characteristics (such as strength, acceleration, range, and speed) by the exoskeleton hosting the paretic arm; (3) active-assisted: when the patient is not able to carry out a movement, the robot supports the arm strength against gravity, thus replicating movements executed by the unaffected arm.
Design and Procedure
We used a within-subject design divided into four main stages. The first stage was based on the recruitment of the patients (see inclusion criteria reported above). Physiotherapists as well as data entry assistants were blinded to all phases of the study. In the second stage, the eligible stroke patients underwent an MRI examination at baseline (T0). In the third stage, participants underwent a rehabilitation program consisting of a validated protocol of exoskeleton-robot assisted activities (Colizzi et al., 2009; Dolce et al., 2009; Pignolo et al., 2012) combined with an additional 4–5 h per week of conventional therapy (Aisen et al., 1997; Volpe et al., 2000), in agreement with Italian norms on the treatment of stroke patients. The conventional activities were carried out by an (blinded) expert therapist and consisted of occupational therapy exercises together with passive/active mobilization of upper and lower limbs, trunk control, standing and ambulation. The robot-assisted rehabilitation programs are summarized in Figure 3 (Colizzi et al., 2009; Dolce et al., 2009; Pignolo et al., 2012). The ARAMIS protocol for rehabilitation included 60-min sessions daily over periods not exceeding 7 weeks. Both single and multiple movements were scheduled. In the first 2–3 weeks of treatment, all subjects performed a series of asynchronous exercises, where the paretic arms repeated each of the exercises 20 times for a total of 200 repetitions per session (Table 1). In the following 2–3 weeks, the asynchronous exercises were gradually reduced to 100 per session and replaced by synchronous exercises (100/session), with the total number remaining unchanged. The rehabilitation sessions in the active-assisted modality began following an adequate motor recruitment of the upper limb where necessary as stipulated in the FM-UE scale modified by Lindmark and Hamrin (1988; total score >70), which continued to the end of scheduled treatment (Figure 3). All patients started rehabilitation periods at the same time.
Finally, at the end of the 7-week training period, participants were given a blinded motor assessment, using the same protocol as at baseline (T1). Twenty patients were removed from the final analysis: (a) two refused to continue; (b) 12 were hospitalized and (c) six patients were removed because they did not complete imaging evaluation. Thus, 14 stroke patients completed the entire protocol.
MRI Data Acquisition
All participants underwent the same MRI scanning protocol immediately before and after rehabilitation. MRI and clinical assessments were performed in the same day. Patients were examined using a 3-Tesla GE MR750 scanner (GE Healthcare, Rahway, NJ, USA). The MRI protocol included whole-brain, three-dimensional, T1-weighted (BRAVO), spoiled gradient recall echo (TE/TR = 3.7/9.2 ms, flip angle 12°, voxel size = 1 × 1 × 1 mm3), T2-weighted images (TR = 3500 ms, TE = 20/85 ms) and fast fluid-attenuated inversion-recovery (FLAIR) axial images (TR = 9500 ms, TE = 100 ms; matrix size 512 × 512, FOV: 24 cm; 36 slices, 4 mm slices, 0 mm gap).
Lesion Identification
Lesion locations and size was performed, using MRIcron software (version 12, Rorden and Brett, 2000). For each patient, we manually outlined the lesion area (volume of interest (VOI) images) on each slice of the FLAIR images. All lesions were traced by a trained image analyst and by an experienced clinical neurologist and neuroradiologist, who were blinded to all clinical data. These specialists worked together to develop a consensus regarding the extent of the lesion in each individual. The FLAIR scan and the corresponding lesion maps were coregistered onto the T1-weight template MRI scan from the Montreal Neurological Institute (Brett et al., 2001; Rorden et al., 2007). Finally, the T1 scan and the VOI images were mapped into stereotaxic space, using the normalization algorithm provided in the SPM81 software, which is exceptionally robust to the presence of lesions (Seghier, 2008). The number of MRI voxels involved in each stroke lesion was calculated. The lesion size was also derived from the lesion mask by multiplying the number of voxels with voxel volume (1 mm3).
Voxel-Based Lesion-Symptom Mapping (VLSM)
To visualize the lesion locations and evaluate the overlap of the lesioned voxels across participants, MRIcron software was used. The relationship between neuroanatomical damage and motor recovery was evaluated using a well-known validated method: Voxel-Based Lesion-Symptom Mapping (VLSM; Bates et al., 2003).
The normalized images and lesion masks, along with the measures of motor impairment, were used in the VSLM analysis, implemented in the nonparametric mapping (NPM) software (version 12 December 2012; Chris Rorden, Columbia, SC, USA2) included in the MRIcron software suite. In the first analysis, in order to verify the presence of lesion changes between the two time-points (before and after rehabilitation), the nonparametric Liebermeister statistical analysis for binary data was used (Rorden et al., 2007). In addition, taking into account the non-normal distribution of the data to determine which clinical parameter correlated with motor recovery a nonparametric test (using the permuted Brunner-Munzel rank order statistic) for continuous data within the NPM software was considered (Rorden et al., 2007).
Both the Liebermeister statistical analysis and Brunner-Munzel test were conducted assuming an a priori minimum lesion density threshold. In fact, only voxels that were lesioned in at least 20% of all patients were included in the analysis. This value is based on a conventional threshold regarding the total incidence of lesions in a voxel regardless of behavioral performance (Rorden et al., 2007), which maximizes statistical power, thus reducing the likelihood of error (i.e., unidentified lesions) (Inoue et al., 2014). The color-coded map derived from the nonparametric Brunner-Munzel test with false-discovery rate (FDR) corrections at P ≤ 0.05 was then overlaid onto a standard brain template3 and used to determine significant differences between lesioned areas and motor behavioral scores.
Statistical Analysis
Statistical analyses were performed with Statistica Version 6.04. Assumptions for normality were tested for all continuous variables using the Kolmogorov–Smirnov test. Demographical and clinical variables were normally distributed except for lesion volume. A paired-sample t-test (two-sided) was used to verify any statistical significant changes in motor scales before and after robotic-assisted rehabilitation. A correlation between clinical evaluations was performed using nonparametric r’ Spearman on motor performance defined as delta scores. In fact, clinical changes associated with different motor neurorehabilitation approaches were calculated as the differential between T1 and T0 (Δ) scores. A two-tailed alpha level of <0.05 was used to define significance.
Results
Fourteen stroke patients (age: 66.9 ± 11.3; 28% female) completed all phases of rehabilitation protocol and were, finally, included in the imaging analysis. The median lesion volume related to stroke damages was of 44.6 [range: 7–146] cm3. The average time period between baseline and re-test evaluation was 44.1 ± 13.9 days. The hemiplegic side was properly disturbed among patients (50% left side). After treatment the vast majority of patients showed evident motor recovery: (A) 68.7% improvement in the FM-UE scale (global score: from 63 ± 21 at baseline to 92 ± 27 after treatment; t-value = 6.1; p < 0.0001); (B) 94.7% in the MI scale (t-value = 6.6; p < 0.0001); (C) 65, 6% in the FIM (t-value = 6.5; p < 0.0001); (D) 174% in the Barthel-Index (t-value = 7.6; p < 0.0001) and (E) 150% improvement in the TCT scale (t-value = 4.4; p < 0.009). Motor recovery as assessed by the FM-UE scale, correlated significantly with TCT values (r = 0.86; p-level < 0.001), whilst the Barthel-Index was related to FIM scores (r = 0.66; p-level = 0.01).
Figure 4 shows the lesion-mapping analysis. The overlapping lesions of all strokes’ brains mainly included putamen, internal capsule, anterior thalamic radiation, superior/posterior regions of corona radiata (Figure 4A). Lesion mapping analysis was first performed to evaluate the presence of lesion load between the two time-points, without revealing any significant changes. We then performed Voxel-based lesion symptom mapping (VLSM) analysis with a nonparametric approach to evaluate the relationship between each clinical scale and the lesion load and localization. The superior region of the corona radiata, internal capsule and putamen were significantly associated with recovery as assessed by the FM-UE scale (Figure 4B, p-level < 0.01). No significant relationship was detected between other clinical scales and lesion mapping. In other words, evidence of motor improvements as defined by MI, FIM, Barthel and TCT scales were not related to lesion load.
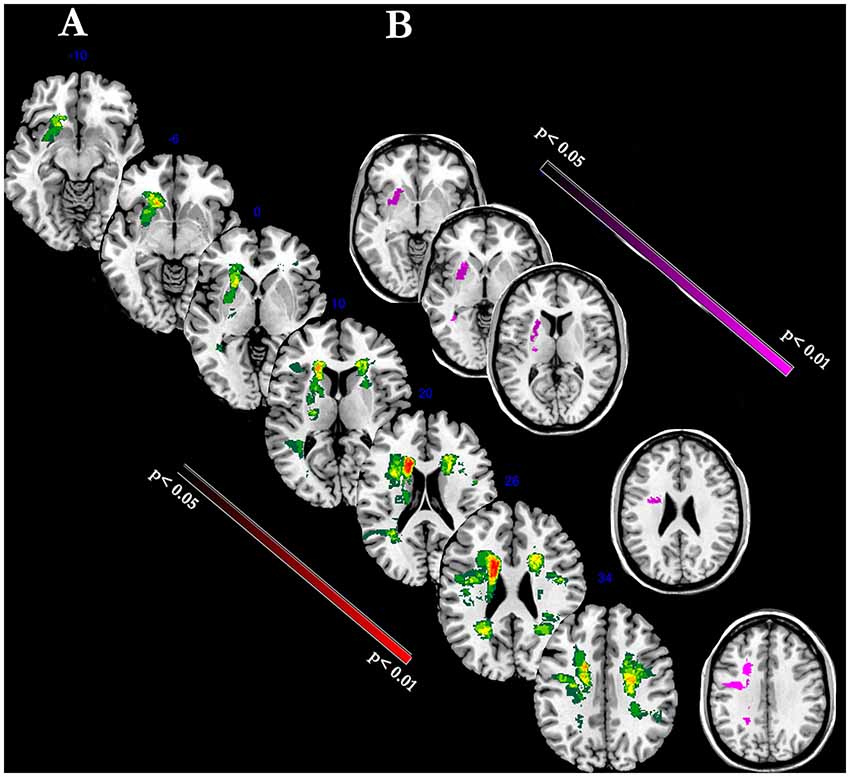
Figure 4. The figure represents voxel-level lesion-mapping analysis performed with voxel-based lesion symptom mapping (VLSM) method implemented in the nonparametric mapping (NPM) software included into the MRIcron software (A). Overlay of FLAIR-dependent MRI lesions detected in all stroke patients (n = 14). The color indicates the frequency of overlapping stroke-related lesions (maximal: red blobs). (B) Regression Analysis: Voxels within the superior region of the corona radiata, internal capsule and putamen were significantly correlated with the degree of motor recovery as assessed by FM-UE scale (violet blobs; P < 0.01).
Discussion
Since the beginning of the 21st century, it has been assumed that motor recovery after stroke is widely influenced by lesion location, specially in the subcortical regions which are involved in the primary or secondary motor systems (Shelton and Reding, 2001). Our lesion mapping study confirms that lesion load mainly affecting the primary motor pathway was related to the degree of upper limb motor recovery induced by an exoskeleton robotic-assisted rehabilitation program.
It is worth noting that in the months immediately following a stroke episode, patients can recover between 40%–70% of initial clinical deficits (Ramsey et al., 2017). This behavioral phenomenon is directly dependent on the functional and structural reorganization of specific brain systems (Murphy and Corbett, 2009), which may occur within a few millimeters of lesion borders and may continue over a wide temporal scale (Siegel et al., 2018). Despite the fact that part of this recovery might be spontaneous, it has widely been demonstrated that intensive, repetitive, variable and rewarding motor activities improve functional outcome (Lo et al., 2010; Turner et al., 2013). However, this kind of protocol is time-consuming for conventional manual therapy and given the growing demand due to new cases, alterative automated procedures are needed. As stated by Lo and Xie (2012), robotic-assisted therapy and, in particular, exoskeletons have the potential to provide intensive rehabilitation consistently for a longer duration, thus enabling more frequent treatment and potentially reducing costs. Generally, it has been demonstrated that robot-assisted rehabilitation approaches have a greater effect (although not significant) on motor recovery with respect to conventional therapy (Lo et al., 2010; Bertani et al., 2017). Exoskeleton-robot assisted therapy would seem to increase this effect (Lo and Xie, 2012). This kind of device can accurately control multiple joints at the same time, thus promoting the interaction between the paretic and unaffected upper limbs, which may ultimately aid a patient to produce more realistic task-based exercises (Dolce et al., 2009; Lo and Xie, 2012; Pignolo et al., 2016). However, the neural basis of this clinical effect has been poorly investigated.
Motor recovery in stroke patients has attracted the interest of the neuroimaging community due to the drawbacks of the conventional treatment characterized by simple active/passive strengthening of the affected upper/lower extremities and occupational activity. Generally, motor recovery is typically associated with widespread, synchronous activity modulations in a spatially distributed, highly integrated network encompassing the corticospinal tract (Shelton and Reding, 2001; Lin et al., 2009), The primary motor cortex together with the putamen and capsula interna are all essential components of the brain network involved in restoring of the motor routines necessary for relearning skilled motor behavior (Doyon et al., 2003). In a recent meta-analysis of 24 studies using fMRI of motor tasks, Favre et al. (2014) affirmed that the activity in ipsilateral primary motor cortex is one of the best predictors of the good motor recovery in chronic stroke patients. Similarly, connectivity (i.e., DTI) as well as lesion mapping studies demonstrated that the disruption of the capsula interna, corona radiata, globus pallidus, and putamen predict the functional recovery (Shelton and Reding, 2001; Rehme et al., 2011; Stinear et al., 2012; Stinear and Ward, 2013; Hannanu et al., 2017; Lee et al., 2017). The pathway along the pedunculopontine nucleus, cerebellum, striatum and the motor cortex contributes to motor initiation, modulation of motor rhythm and postural muscle tone during motor behaviors (Takakusaki, 2013). Thus, injuries to the capsula interna, putamen and corona radiata could be considered as a hallmark of motor recovery in stroke patients, especially in the chronic phase (Nudo et al., 1996; Takakusaki, 2013). The few neuroimaging (fMRI and EEG) studies on robotic-assisted devices have described functional compensatory responses in the primary and secondary motor cortices as a function of the increased motor activities related to haptic feedback and bimanual training (Fan et al., 2016; Saleh et al., 2017; Gandolfi et al., 2018).
We believe that this is the first neuroimaging study evaluating how motor recovery obtained with an exoskeleton robot exploits a specific neural substrate. Overall, our lesion map data largely matches previous pathophysiological evidence (Shelton and Reding, 2001; Lo et al., 2010; Turner et al., 2013). However, in contrast to previous studies, one additional finding is worth discussing. In fact, we found that the clinical relevance of neural damage was better express by FM-UE scores, a scale focused on the motor activity of upper limbs. The FM-UE is recognized as the most sensitive clinical scale for detecting the motor recovery of the upper limb functions and is a reliable measure of the neural changes associated with neurorehabilitation (Lo et al., 2010; Hannanu et al., 2017).
Two important limitations need to be discussed. First, the lack of a useful control group for evaluating the impact of robotic-assisted rehabilitation with respect to conventional treatment. Although we are aware of this limitation, it is important to bear in mind that the effectiveness of upper-extremity rehabilitation highlighted by the ARAMIS device with respect to conventional treatment has previously been demonstrated (Colizzi et al., 2009; Dolce et al., 2009; Pignolo et al., 2012). The main target of this study was to investigate how neural damage might affect the motor recovery induced by rehabilitation treatment primarily focused on the employment of an exoskeleton robotic-assisted system rather than evaluating its effectiveness with respect to other approaches. Second, in our study we cannot disentangle the contribution of occupational therapy with respect to robot-assisted rehabilitation since in Italy the guidelines for stroke unit interventions entail combining advanced robotic-assisted rehabilitation with conventional protocol. However, previous studies have already investigated the difference between robotic-assisted therapies in addition to conventional therapy with respect to conventional therapy alone. In particular, Aisen et al. (1997) and Volpe et al. (2000) demonstrated that the outcome of stroke patients increases as a function of intensity and effectiveness of the motor therapy. This, thus, suggests that a combination of rehabilitation approaches is preferable over a single treatment.
Taking into account the fact that our data are similar to findings reported in previous studies regarding the impact of stroke lesions on motor recovery induced by conventional approaches (Turner et al., 2013), all this evidence supports a new hypothesis. In fact, regardless of the neurorehabilitation approaches (conventional vs. robotic-assisted) brain lesions affecting motor recovery are mainly localized in the capsula interna, corona radiata and putamen (Shelton and Reding, 2001). Different kinds of rehabilitation approaches, instead, could be useful to understand which neural networks, subserving functional compensatory responses or strategies, could be exploited to bypass the lesions (Fan et al., 2016; Hannanu et al., 2017). Further fMRI studies investigating the different neural pathways engaged after conventional and robotic-assisted rehabilitation programs are needed. In fact, it has been proposed that the compensatory overactivity of the ipsilateral motor cortex (Favre et al., 2014) is not sufficient to support functional recovery (Ganguly et al., 2009). For this reason, we believe that exoskeleton-robot assisted therapy could exploit different neural networks subserving haptic feedback (Turner et al., 2013; Lo and Xie, 2012).
Overall, as stated by Langhorne et al. (2009), recovery from a stroke event is a complex process that occurs through a potentiation and extension of residual brain areas where lesion location rather lesion volume is more effective in influencing motor recovery. Our data could be useful for monitoring and planning rehabilitation strategies for motor recovery in stroke patients and to highlight the reliability of exoskeleton-robot assisted therapy in addition to other approaches.
Author Contributions
All authors gave substantial contribution to conception and experimental design, as well as data analysis, interpretation and drafting of the manuscript. The submission of this article has been approved by all authors and by the institution where the work was carried out.
Funding
This study was supported by MIUR (Ministero Universita’ e Ricerca Italiana; PON01-01180-NEUROSTAR).
Conflict of Interest Statement
The authors declare that the research was conducted in the absence of any commercial or financial relationships that could be construed as a potential conflict of interest.
Acknowledgments
This study was made in memory of Herr Professor Giuliano Dolce.
Footnotes
- ^ http://www.fil.ion.ucl.ac.uk/spm/software/spm8
- ^ http://www.cabiatl.com/mricro/npm/
- ^ http://www.bic.mni.mcgill.ca/ServicesAtlases/Colin27
- ^ www.statsoft.com
References
Aisen, M. L., Krebs, H. I., Hogan, N., McDowel, F., and Volpe, B. T. (1997). The effect of robot-assisted therapy and rehabilitative training on motor recovery following stroke. Arch. Neurol. 54, 443–446. doi: 10.1001/archneur.1997.00550160075019
Bates, E., Wilson, S. M., Saygin, A. P., Dick, F., Sereno, M. I., Knight, R. T., et al. (2003). Voxel-based lesion- symptom mapping. Nat. Neurosci. 6, 448–450. doi: 10.1038/nn1050
Bertani, R., Melegari, C., De Cola, M. C., Bramanti, A., Bramanti, P., and Calabrò, R. S. (2017). Effects of robot-assisted upper limb rehabilitation in stroke patients: a systematic review with meta-analysis. Neurol. Sci. 38, 1561–1569. doi: 10.1007/s10072-017-2995-5
Brett, M., Leff, A. P., Rorden, C., and Ashburner, J. (2001). Spatial normalization of brain images with focal lesions using cost function masking. Neuroimage 14, 486–500. doi: 10.1006/nimg.2001.0845
Burke Quinlan, E., Dodakian, L., See, J., McKenzie, A., Le, V., Wojnowicz, M., et al. (2015). Neural function, injury, and stroke subtype predict treatment gains after stroke. Ann. Neurol. 77, 132–145. doi: 10.1002/ana.24309
Carrera, E., and Tononi, G. (2014). Diaschisis: past, present, future. Brain 137, 2408–2422. doi: 10.1093/brain/awu101
Choo, P. L., Gallagher, H. L., Morris, J., Pomeroy, V. M., and van Wijck, F. (2015). Correlations between arm motor behavior and brain function following bilateral arm training after stroke: a systematic review. Brain Behav. 5:e00411. doi: 10.1002/brb3.411
Colizzi, L., Lidonnici, A., and Pignolo, L. (2009). The ARAMIS project: a concept robot and technical design. J. Rehab. Med. 41, 1011–1101. doi: 10.2340/16501977-0407
Collin, C., and Wade, D. (1990). Assessing motor impairment after stroke: a pilot reliability study. J. Neurol. Neurosurg. Psychiatry 53, 576–579. doi: 10.1136/jnnp.53.7.576
Collin, C., Wade, D. T., Davies, S., and Horne, V. (1988). The Barthel ADL Index: a reliability study. Int. Disabil. Stud. 10, 61–63. doi: 10.3109/09638288809164103
Dolce, G., Lucca, L. F., and Pignolo, L. (2009). Robot-assisted rehabilitation of the paretic upper limb: rationale of the ARAMIS project. J. Rehabil. Med. 41, 1007–1101. doi: 10.2340/16501977-0406
Doyon, J., Penhune, V., and Ungerleider, L. G. (2003). Distinct contribution of the cortico-striatal and cortico-cerebellar systems to motor skill learning. Neuropsychologia 41, 252–262. doi: 10.1016/s0028-3932(02)00158-6
Fan, Y. T., Lin, K. C., Liu, H. L., Wu, C. Y., Wai, Y. Y., and Lee, T. H. (2016). Neural correlates of motor recovery after robot-assisted stroke rehabilitation: a case series study. Neurocase 22, 416–425. doi: 10.1080/13554794.2016.1215469
Favre, I., Zeffiro, T. A., Detante, O., Krainik, A., Hommel, M., and Jaillard, A. (2014). Upper limb recovery after stroke is associated with ipsilesional primary motor cortical activity: a meta-analysis. Stroke 45, 1077–1083. doi: 10.1161/STROKEAHA.113.003168
Formaggio, E., Storti, S. F., Boscolo Galazzo, I., Gandolfi, M., Geroin, C., Smania, N., et al. (2013). Modulation of event-related desynchronization in robot-assisted hand performance: brain oscillatory changes in active, passive and imagined movements. J. Neuroeng. Rehabil. 10:24. doi: 10.1186/1743-0003-10-24
Franchignoni, F. P., Tesio, L., Ricupero, C., and Martino, M. T. (1997). Trunk control test as an early predictor of stroke rehabilitation outcome. Stroke 28, 1382–1385. doi: 10.1161/01.str.28.7.1382
Fugl-Meyer, A. R., Jääskö, L., Leyman, I., Olsson, S., and Steglind, S. (1975). The post-stroke hemiplegic patient. Scand. J. Rehabil. Med. 7, 13–31.
Gandolfi, M., Formaggio, E., Geroin, C., Storti, S. F., Boscolo Galazzo, I., Bortolami, M., et al. (2018). Quantification of upper limb motor recovery and EEG power changes after robot-assisted bilateral arm training in chronic stroke patients: a prospective pilot study. Neural Plast. 2018:8105480. doi: 10.1155/2018/8105480
Ganguly, K., Secundo, L., Ranade, G., Orsborn, A., Chang, E. F., Dimitrov, D. F., et al. (2009). Cortical representation of ipsilateral arm movements in monkey and man. J. Neurosci. 29, 12948–12956. doi: 10.1523/JNEUROSCI.2471-09.2009
Hannanu, F. F., Zeffiro, T. A., Lamalle, L., Heck, O., Renard, F., Thuriot, A., et al. (2017). Parietal operculum and motor cortex activities predict motor recovery in moderate to severe stroke. Neuroimage Clin. 14, 518–529. doi: 10.1016/j.nicl.2017.01.023
Hendricks, H. T., Zwarts, M. J., Plat, E. F., and van Limbeek, L. J. (2002). Systematic review for the early prediction of motor and functional outcome after stroke by using motor-evoked potentials. Arch. Phys. Med. Rehabil. 83, 1303–1308. doi: 10.1053/apmr.2002.34284
Huang, V. S., and Krakauer, J. W. (2009). Robotic neurorehabilitation: a computational motor learning perspective. J. Neuroeng. Rehabil. 6:5. doi: 10.1186/1743-0003-6-5
Inoue, K., Madhyastha, T., Rudrauf, D., Mehta, S., and Grabowski, T. (2014). What affects detectability of lesion-deficit relationships in lesion studies? Neuroimage Clin. 6, 388–397. doi: 10.1016/j.nicl.2014.10.002
Keith, R. A., Granger, C. V., Hamilton, B. B., and Sherwin, F. S. (1987). The functional independence measure: a new tool for rehabilitation. Adv. Clin. Rehabil. 1, 6–18.
Langhorne, P., Coupar, F., and Pollock, A. (2009). Motor recovery after stroke: a systematic review. Lancet Neurol. 8, 741–754. doi: 10.1016/s1474-4422(09)70150-4
Lee, K. B., Kim, J. S., Hong, B. Y., Sul, B., Song, S., Sung, W. J., et al. (2017). Brain lesions affecting gait recovery in stroke patients. Brain Behav. 7:e00868. doi: 10.1002/brb3.868
Lin, F. H., Agnew, J. A., Belliveau, J. W., and Zeffiro, T. A. (2009). Functional and effective connectivity of visuomotor control systems demonstrated using generalized partial least squares and structural equation modeling. Hum. Brain Mapp. 30, 2232–2251. doi: 10.1002/hbm.20664
Lindmark, B., and Hamrin, E. (1988). Evaluation of functional capacity after stroke as a basis for active intervention. Scand. J. Rehabil. Med. 20, 111–122. doi: 10.1111/j.1471-6712.1988.tb00357.x
Lo, A. C., Guarino, P. D., Richards, L. G., Haselkorn, J. K., Wittenberg, G. F., Federman, D. G., et al. (2010). Robot-assisted therapy for long-term upper-limb impairment after stroke. N. Engl. J. Med. 362, 1772–1783. doi: 10.1056/NEJMoa0911341
Lo, K., Stephenson, M., and Lockwood, C. (2017). Effectiveness of robotic assisted rehabilitation for mobility and functional ability in adult stroke patients: a systematic review protocol. JBI Database System. Rev. Implement. Rep. 15, 39–48. doi: 10.11124/JBISRIR-2016-002957
Lo, H. S., and Xie, S. Q. (2012). Exoskeleton robots for upper-limb rehabilitation: state of the art and future prospects. Med. Eng. Phys. 34, 261–268. doi: 10.1016/j.medengphy.2011.10.004
Masiero, S., Celia, A., Rosati, G., and Armani, M. (2007). Robotic-assisted rehabilitation of the upper limb after acute stroke. Arch. Phys. Med. Rehabil. 88, 142–149. doi: 10.1016/j.apmr.2006.10.032
Murphy, T. H., and Corbett, D. (2009). Plasticity during stroke recovery: from synapse to behavior. Nat. Rev. Neurosci. 10, 861–872. doi: 10.1038/nrn2735
Nudo, R. J., Wise, B. M., Sifuentes, F., and Milliken, G. W. (1996). Neural substrates for the effects of rehabilitative training on motor recovery after ischemic infarct. Science 272, 1791–1794. doi: 10.1126/science.272.5269.1791
Pignolo, L. (2009). Robotics in neurorehabilitation. J. Rehabil. Med. 41, 955–960. doi: 10.2340/16501977-0434
Pignolo, L., Lucca, L. F., Basta, G., Serra, S., Pugliese, M. E., Sannita, W. G., et al. (2016). A new treatment in the rehabilitation of the paretic upper limb after stroke: the ARAMIS prototype and treatment protocol. Ann. Ist. Super. Sanita 52, 301–308. doi: 10.4415/ANN_16_02_25
Pignolo, L., Rogano, S., Quintieri, M., Leto, E., and Dolce, G. (2012). Decreasing incidence of paroxysmal sympathetic hyperactivity syndrome in the vegetative state. J. Rehabil. Med. 44, 502–504. doi: 10.2340/16501977-0981
Ramsey, L. E., Siegel, J. S., Lang, C. E., Strube, M., Shulman, G. L., and Corbetta, M. (2017). Behavioural clusters and predictors of performance during recovery from stroke. Nat. Hum. Behav. 1:0038. doi: 10.1038/s41562-016-0038
Rehme, A. K., Fink, G. R., von Cramon, D. Y., and Grefkes, C. (2011). The role of the contralesional motor cortex for motor recovery in the early days after stroke assessed with longitudinal FMRI. Cereb. Cortex 21, 756–768. doi: 10.1093/cercor/bhQ150
Rehme, A. K., Volz, L. J., Feis, D. L., Eickhoff, S. B., Fink, G. R., and Grefkes, C. (2015). Individual prediction of chronic motor outcome in the acute post-stroke stage: behavioral parameters versus functional imaging. Hum. Brain Mapp. 36, 4553–4565. doi: 10.1002/hbm.22936
Rorden, C., Karnath, H. O., and Bonilha, L. (2007). Improving lesion-symptom mapping. J. Cogn. Neurosci. 19, 1081–1088. doi: 10.1162/jocn.2007.19.7.1081
Rorden, C., and Brett, M. (2000). Stereotaxic display of brain visions. Behav. Neurol. 12, 191–200. doi: 10.1155/2000/421719
Saleh, S., Fluet, G., Qiu, Q., Merians, A., Adamovich, S. V., and Tunik, E. (2017). Neural patterns of reorganization after intensive robot-assisted virtual reality therapy and repetitive task practice in patients with chronic stroke. Front. Neurol. 8:452. doi: 10.3389/fneur.2017.00452
Seghier, M. L. (2008). Laterality index in functional MRI: methodological issues. Magn. Reson. Imaging 26, 594–601. doi: 10.1016/j.mri.2007.10.010
Shelton, F. N., and Reding, M. J. (2001). Effect of lesion location on upper limb motor recovery after stroke. Stroke 32, 107–112. doi: 10.1161/01.str.32.1.107
Siegel, J. S., Seitzman, B. A., Ramsey, L. E., Ortega, M., Gordon, E. M., Dosenbach, N. U. F., et al. (2018). Re-emergence of modular brain networks in stroke recovery. Cortex 101, 44–59. doi: 10.1016/j.cortex.2017.12.019
Stinear, C. (2010). Prediction of recovery of motor function after stroke. Lancet Neurol. 9, 1228–1232. doi: 10.1016/s1474-4422(10)70247-7
Stinear, C. M., Barber, P. A., Coxon, J. P., Fleming, M. K., and Byblow, W. D. (2008). Priming the motor system enhances the effects of upper limb therapy in chronic stroke. Brain 131, 1381–1390. doi: 10.1093/brain/awn051
Stinear, C. M., Barber, P. A., Petoe, M., Anwar, S., and Byblow, W. D. (2012). The PREP algorithm predicts potential for upper limb recovery after stroke. Brain 135, 2527–2535. doi: 10.1093/brain/aws146
Stinear, C. M., and Ward, N. S. (2013). How useful is imaging in predicting outcomes in stroke rehabilitation? Int. J. Stroke 8, 33–37. doi: 10.1111/j.1747-4949.2012.00970.x
Swayne, O. B., Rothwell, J. C., Ward, N. S., and Greenwood, R. J. (2008). Stages of motor output reorganization after hemispheric stroke suggested by longitudinal studies of cortical physiology. Cereb. Cortex 18, 1909–1922. doi: 10.1093/cercor/bhm218
Takakusaki, K. (2013). Neurophysiology of gait: from the spinal cord to the frontal lobe. Mov. Disord. 28, 1483–1491. doi: 10.1002/mds.25669
Turner, D. L., Ramos-Murguialday, A., Birbaumer, N., Hoffmann, U., and Luft, A. (2013). Neurophysiology of robot-mediated training and therapy: a perspective for future use in clinical populations. Front. Neurol. 4:184. doi: 10.3389/fneur.2013.00184
Keywords: stroke, neurorehabilitation, exoskeleton, upper limb, voxel-based lesion symptom mapping
Citation: Cerasa A, Pignolo L, Gramigna V, Serra S, Olivadese G, Rocca F, Perrotta P, Dolce G, Quattrone A and Tonin P (2018) Exoskeleton-Robot Assisted Therapy in Stroke Patients: A Lesion Mapping Study. Front. Neuroinform. 12:44. doi: 10.3389/fninf.2018.00044
Received: 29 March 2018; Accepted: 21 June 2018;
Published: 17 July 2018.
Edited by:
Antonio Fernández-Caballero, Universidad de Castilla-La Mancha, SpainReviewed by:
Antonia Tzemanaki, University of the West of England, United KingdomYi-Ning Wu, University of Massachusetts Lowell, United States
Copyright © 2018 Cerasa, Pignolo, Gramigna, Serra, Olivadese, Rocca, Perrotta, Dolce, Quattrone and Tonin. This is an open-access article distributed under the terms of the Creative Commons Attribution License (CC BY). The use, distribution or reproduction in other forums is permitted, provided the original author(s) and the copyright owner(s) are credited and that the original publication in this journal is cited, in accordance with accepted academic practice. No use, distribution or reproduction is permitted which does not comply with these terms.
*Correspondence: Antonio Cerasa, antonio.cerasa76@gmail.com
Paolo Tonin, patonin18@gmail.com
† These authors have contributed equally to this work.