- Department of Radiology and Biomedical Imaging, The Brain Observatory, University of California, San Diego, CA, USA
One of the major issues hindering a comprehensive connectivity model for the human brain is the difficulty in linking Magnetic Resonance Imaging (MRI) measurements to anatomical evidence produced by histological methods. In vivo and postmortem neuroimaging methodologies are still largely incompatible in terms of sample size, scale, and resolution. To help bridge the hiatus between different approaches we have established a program that characterizes the brain of individual subjects, combining MRI with postmortem neuroanatomy. The direct correlation of MRI and histological features is possible, because registered images from different modalities represent the same regions in the same brain. Comparisons are also facilitated by large-scale, digital microscopy techniques that afford images of the whole-brain sections at cellular resolution. The goal is to create a neuroimaging catalog representative of discrete age groups and specific neurological conditions. Individually, the datasets allow for investigating the relationship between different modalities; combined, they provide sufficient predictive power to inform analyses and interpretations made in the context of non-invasive studies of brain connectivity and disease.
Introduction
For centuries, the human brain remained impervious to direct observation and experimentation (Penfield, 1958), but with the advent of Magnetic Resonance Imaging (MRI) this situation changed very quickly. The technique supported the fastest and most significant progress toward understanding the functional architecture of the human brain; after three decades of MRI, thousands of individuals of all ages, conditions, and denominations have been scanned, leading to the emergence of comprehensive models of brain structure and function. Functional imaging studies recently broadened their focus from measuring local phenomena associated with specific perceptual and cognitive tasks, to identifying long-range networks involved in the orchestration of neural activity (Sporns et al., 2005). These networks are revealed statistically using resting-state functional MRI (R-fMRI) based on the time course of slow fluctuations in the BOLD signal when the subject is not engaged in any particular task and when the brain is at “rest” (De Luca et al., 2006). Whether R-fMRI-derived models depend on hard-wired anatomical connectivity is an open question, so this technique is often combined with diffusion tensor imaging (DTI) to show major fiber tracts in the deep white matter (Pierpaoli et al., 1996; Bandettini, 2009). These non-invasive neuroimaging modalities are the most widely utilized methodologies for mapping brain circuitry at the system level, and thus also the primary tools of the Human Connectome Project (HCP; Akil et al., 2011).
The problem with images of the brain acquired in vivo with MRI and DTI is that they are limited in both resolution and contrast. The data needs be averaged across many subjects who represent broad demographic or clinical groups in order to extract significant measures. In fact, even the quantification of biomarkers that are crucial clinically for the individual patient is contingent on population-based atlases that only contain very rudimentary anatomical information. In practice, it is not possible with MRI to localize the exact borders of neighboring neuroanatomical structures and identify the underlying histological features on which radiologic images depend. The latter can only be determined postmortem.
Given the undeniable importance of validating MRI, why have neuroanatomical and histological studies have only played a small, tangential role in human brain mapping and why does the HCP, despite the large investment by the National Institute for Health (NIH), not formally include a parallel histological program? The constraints are not conceptual; everyone agrees it would be very useful to corroborate non-invasive measures with baseline information on the actual properties of brain tissue. However, DTI-based models of fiber tract orientation in the human brain are currently validated only on the basis of classic atlases (Dejerine, 1895; Flechsig, 1901; Ludwig and Klinger, 1956; Yakovlev and Lecours, 1967). These contain anachronistically zealous, yet subjectively construed illustrations and were derived from the dissection of a few undocumented brain specimens, so the level of comparison is severely limited.
The challenges in creating a comprehensive histological model of the human brain that matches the scope and usability of MRI population-based templates, including the proposed Connectome, are primarily logistical. To begin with, any study that aims at examining cellular-level features in a brain depends on the expiration of the subject, and consequently neuroanatomical studies have been confined to those few cases where autopsies were prescribed because of clinical or legal concerns or to individuals who enrolled in tissue donation programs. Because in the first scenario the rate of autopsy has universally been decreasing (Kretzschmar, 2009), the enhancement of brain donation programs (via incentives, outreach, and education) represents the only realistic future opportunity of supporting a large-scale, neuro-histological mapping effort.
Assuming that the necessity of enrolling a large enough number of brain donors has been addressed, noting that the benefits of such donor program cannot be expected to roll out in the short-term, what pre-mortem information should characterize the specimen and how should the brain be processed in order to create images that can complement and validate MRI studies? Because the main goal is to bridge the gap between non-invasive neuroimaging and histology it would be useful to have access to radiologic images acquired in vivo for each case that comes to autopsy. When comparisons are made between images from different modalities in the context of the same subject all the information necessary to localize and quantify microscopic features underlying the patterns revealed in MRI images is available. Furthermore, the correspondence between images acquired in vivo and those from histopathology is enormously improved if the latter cover the whole brain, just like MRI. These methodological prerogatives are the basis for a novel neuroimaging resource that will be used to cross-reference different modalities and establish fundamental properties of brain structure at multiple scales.
The approach is exemplified by the multimodal examination of the brain of H.T., one of our program participants who passed away in May 2009. Her neuroimaging profile was created using a palette of multiple modalities that provided correlated images of the brain at different levels of resolution. The study that is described in this communication was approved by the University of California's Institutional Review Board.
Case Description
H.T. was a woman of Caucasian descent born in Rochester, NY in 1920. She had 15 years of education which included two years of college. A smoker for 50 years, her only major medical concern was hypertension and she took medication to lower her blood pressure. She died of heart failure. During the last year of her life she experienced a slight decline in memory and cognitive proficiency (it should be noted that these phenomena were observed by her closest relatives; H.T. never recognized or admitted to having any impairment). The examination of the clinical scans by a trained neuroradiologist did not report major abnormalities in the brain of H.T. with the exception of several small lacunar infarcts.
Multi-Scale Neuroimaging
H.T. died of cardiac arrest at the age of 88. Because she wore a pacemaker at the time of her enrollment in our study (thus precluding her from undergoing MRI scanning while she was living), multiple scans of the brain in situ were acquired postmortem. Images of the brain were acquired on an “HDX” Twinspeed EXCITE 1.5T scanner (Milwaukee, WI.) using an eight-channel phased-array head coil. Scan parameters were selected to allow robust reconstruction of the brain's cortical surface using FreeSurfer Software and automated segmentation of cortex and subcortical structures (Fischl et al., 2002, 2004; Figure 1A). DTI imaging consisted in the collection of 51 diffusion gradient directions (Figure 2A).
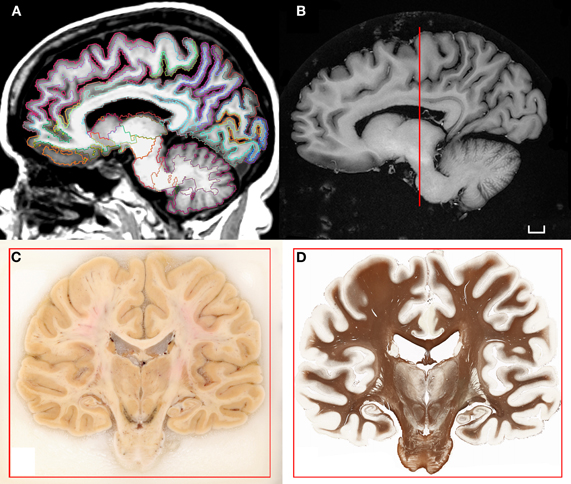
Figure 1. (A) T1-weighted scan of the brain of H.T. acquired in situ 3D IR-SPGR, matrix size: 256 × 256, voxel size of 0.9375 × 0.9375, slice thickness = 1.2 mm. The volume underwent the automated labeling of subcortical structures and cortical gyri that is part of the morphometry pipeline included in the software Freesurfer (Fischl et al., 2002, 2004). (B) High-resolution T1-weighted scan of the formalin-fixed specimen imaged ex situ. The brain was scanned in a dedicated chamber filled with phosphate buffer (matrix size: 512 × 512, pixel size: 0.5/0.5, slice thickness: 1.2 mm). Multiple acquisitions were repeated in order to increase the signal-to-noise ratio. (C) Coronal blockface image from the same brain at the level of the posterior hippocampus. (D) Corresponding histological slice stained for myelinated fibers [protocol modified from Gallyas (1979); Annese et al. (2004)]. Scale bar: 1 cm.
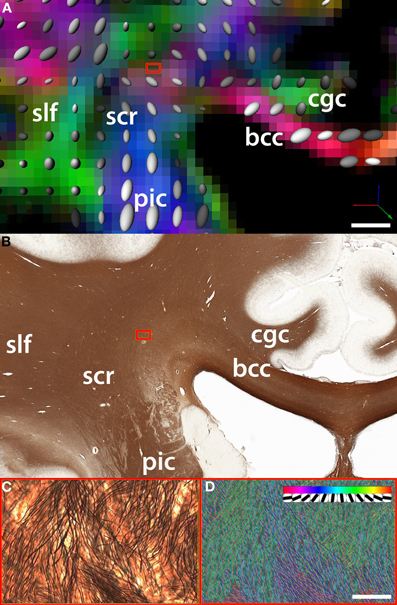
Figure 2. Comparison between (A) fiber orientation maps derived from DTI and (B) the underlying myelo-architecture in the region of intersection of the fibers belonging to the corpus callosum (cc) which travel along the coronal plane (parallel to the plane of the figure) and the superior corona radiata (scr). In this example, the region of intersection between cc fibers and the scr is an area that shows significant crossing over of fibers of small caliber (blue box). (C) One DTI voxel-wide selection from silver-stained tissue slice (magnification = 20×). (D) The density and orientation of single white matter fibers is quantified using a template matching algorithm [Bartsch et al. (2011)]. The insert in panel D shows the discrete orientations of the templates used to classify fiber bundles. DTI acquisition parameters: image matrix size = 96 × 96, B0 = 1000, 51 directions, FOV = 24 cm, 47 axial slices, slice thickness = 2.5 mm. Fiber track definition derived from the atlas of Oishi and colleagues (2011); bcc: body of the corpus callosum; cgc: cingulum; slf: superior longitudinal fasciculus. Scale bars: Panel B = 5 mm; Panel C = 100 microns.
After the scans of the brain in situ were completed the brain was extracted at autopsy and suspended by the basilar artery in fixative solution (4% paraformaldehyde phosphate-buffered solution) at 4°C. After six weeks of fixation in formaldehyde, a second series of scans were performed ex situ (Figure 1B). The protocol lasted approximately 36 h, using the same sequences that were run in the previous session but with multiple acquisitions (4–32 NEX) in order to improve the signal-to-noise ratio in the fixed tissue and increase resolution.
Fixation was protracted for 10 weeks after the second MRI session and the tissue was subsequently cryo-protected by immersion in 30% sucrose. Following the removal of superficial blood vessels and pial membranes, the whole specimen was embedded in a block of 10% gelatin and frozen in a bath of chilled isopentane (−40°C). The gelatin-brain block was attached to a custom-engineered freezing stage and sectioned on a large motorized sliding microtome (Leica Microsystems Inc., Bannockburn, IL) during an uninterrupted cutting procedure. The tissue was cut at an interval of 70 microns. Digital images of the cut surface were acquired before each tissue slice was collected using a digital, single-lens reflex camera (Nikon D700; Nikon Inc., Melville, NY) that was mounted directly in line with the microtome stage. Image acquisition during the sectioning procedure produced an unabridged series of tomographic anatomical images through the brain (Figure 1C). These data contain excellent tissue contrast, ideal for anatomical delineations and the stack is the basis for the correct alignment and 3-D reconstruction of corresponding tissue slices.
Two adjacent, regularly spaced series of giant histological slices were stained using Thionin (Nissl staining) and a sensitive silver impregnation technique based on the original Gallyas (1979) protocol for myelinated fibers (Annese et al., 2004; Figure 1D). Tissue slices were mounted on large glass slides (5 × 7 inches) and the tissue was imaged on custom-engineered large-format microscope scanners. The core digitizing unit is composed of a computer-controlled microscope, a linear-encoded motorized stage, a line scanner camera, and storage server (Annese et al., 2009). The images representing the entire giant tissue slice are typically composed of 40,000 image tiles that are acquired systematically at 20× magnification (resolution: ∼0.4 μm/pixel). Image tiles are stitched into pyramidal “virtual” slices that allow for fast exploratory viewing and the topographic localization of microscopic features (Mikula et al., 2007; Weinstein et al., 2009). The images are very large (dimensions in pixels are: 334,500 × 266,200; file size on disk 250–400 GB) nevertheless, as JPG2000 compressed pyramidal files (size reduced to 15 GB), the images can be examined through increasing magnification levels while a fixed number of pixels spanning progressively smaller fields of view are shown. This technology, combined with the possibility of surveying slices at multiple locations in the 3D model of the brain, is the key to the proper comparison of histological and neuroimaging data sets.
Different Views of the Deep White Matter
The anatomical and DTI data were aligned using tools for automated affine registration included in Amira (Developer Package; Visage Imaging Inc, San Diego, CA). A binary mask based on color segmentation (Annese et al., 2006) was created to separate brain tissue from the surrounding gelatin matrix in the tomographic images acquired during the sectioning procedure. These were reconstructed into a volume and this dataset was registered to the average B0 volume acquired during the DTI protocol. Because the anatomical volume is composed of images that correspond to unique histological sections, it is possible to identify which sections cross any region of interest in the DTI data. The direct, landmark-based comparison of two equivalent coronal images at the level of the posterior hippocampus reveals the histological complexity underlying tensor-based maps of fiber orientation (Figures 2A,B).
This approach permits quantitative correlations between diffusion-based measures of fiber integrity and directionality (derived from fractional anisotropy values) with formal descriptions of axonal architecture. The latter can be produced from high-resolution histological images (Figure 2C) using automated (hence potentially large-scale) image analysis routines (Figure 2D). Quantification at the histological level produces actual values representing main fiber directionality, axonal diameter, and cross-over (Bartsch et al., 2011).
It should be noted that myelination is not the only histological feature that affects MRI signal; gliosis, and perivascular neuropathogenetic phenomena produce visible effects in MRI images of the deep white matter. In order to decipher the relationship between non-invasive imaging and the underlying histology, multiple sequences with different scanning parameters must be correlated with the co-localization of different histopathologic markers of structure and disease. Using specific antibody staining, it is possible to localize neuropathological phenomena that produce visible MRI abnormalities. Each subject-specific data set should be analyzed as a registered stack of anatomical layers that contain overlapping maps of biomarkers. In the context of our methodological pipeline, MRI and DTI are also “stains” in the sense that they show properties of the tissue that are complementary to the features detected by histochemical or immuno-cytological methods.
A better understanding of local white matter architecture at the microscopic level can help defining DTI acquisition and modeling parameters; these may need to be specific to different regions of the white-matter if the goal is to generate accurate whole-brain models of connectivity. In general, the approach demonstrates the possibility of extending animal neuroimaging studies and resources that combine non-invasive and histological mapping (Johnson et al., 2010) to the human brain.
Potential and Future Prospects
The power of our approach lies in the fact that the resolution of the images of H.T.'s brain created with different modalities spans from several millimeters (2.5 mm/voxel in our 3-D DTI data) to a few hundred nanometers (0.3 μm/pixel in the 2-D histological images). The MRI (and DTI) of the brain acquired in situ, containing gray matter (GM), white matter (WM), and cerebrospinal fluid (CSF) and representing the original geometry is necessary because these scans link the microscopic maps created from H.T.'s brain to the very large base of non-invasive structural and functional neuroimaging data that will be collected by the HCP. Furthermore, the neuroimaging sequences that were used to acquire images of the brain of H.T. can be matched to the standard protocols employed by the HCP and other ongoing neuroimaging projects to make direct correlations and inform the development of analysis tools. This way, the growing sample of brain specimens that will be fully characterized at the microscopic level will also be accessible as anatomical reference to large-scale neuroimaging databases. This cross-sectional data will serve as a base line reference for the interpretation of low-resolution images. One could additionally envisage “embedding” MRI data-sets, like that belonging to the brain of H.T., into the processing pipelines of the HCP and other purely non-invasive studies. The output of these pipelines could be evaluated based on corresponding histological images, providing an efficient means to algorithm optimization.
As noted in the previous section, mapping local microscopic features within a 3D model of the whole brain can lead to the formulation of “signature” models that classify neuroanatomical features in specific compartments of the brain. Histological models may help predict individual anatomy (and pathology) from the macroscopic markers that are visible and measurable in non-invasive low-resolution images. In other words, local micro-architectural patterns that can be localized consistently in a large number of subjects may eventually be used to resolve ambiguities in the interpretation of low-resolution data (Leergaard et al., 2010). The systematic characterization and classification of local micro-architectural patterns as exemplified in Figure 2 will be extremely useful for the calibration of the next generation of fiber tracking software and interpretation of the results from diffusion imaging.
Our methodology may not be universally feasible for routine implementation. Aside from equipment and operational costs, the computational requirements for managing massive histological data-sets are considerable. However, depreciation of digital storage will occur sufficiently rapidly to justify the timely implementation of the protocols described above. Many web-based products and initiatives, like Google Maps and the Hubble Telescope, leverage on remote access and interoperability with digital images in the Gigapixel range. The digitization at 20× magnification of whole histological sections of the human brain actually produces images in the Terapixel range; these can nonetheless be shared on the web with the support of appropriate hardware and bandwidth. To demonstrate this functionality, The Brain Observatory developed the infrastructure for a web-based atlas of the human brain that can deliver Terapixel histological images representing whole brain sections at cellular resolution; these are associated with other views of the brain created in 2D and 3D using other neuroimaging modalities (Annese et al., 2012).
The development of robust quantitative methods for registration and analysis combined with the availability of adequate computational resources for handling very large data-sets will make comparisons between neuroimaging data and the underlying microscopic features increasingly effective. Furthermore, as the number of single-subject neuroimaging studies like the one involving H.T, where we combined in vivo and postmortem modalities and for whom we were able to compute maps that span multiple spatial scales, increases the predictive power of the digital neuroanatomical catalog will become stronger, representing a useful resource for data mining, cross-modality validations, and algorithm development.
The example provided in this article should support the notion of multi-modal, single-subject paradigm as a complement to MRI data obtained in the context of large-scale neuroimaging initiatives such as the HCP (Marcus et al., 2011). However, a note of caution is due before the concluding remarks. Histological images only contain a final, “freeze-frame” picture of the brain's microanatomy; whereas we know that the architecture of neural circuits, from the system level to local dendritic properties, changes with maturation, behavior, and disease. Secondly, histology should not be considered unquestioningly as the “gold standard” for neuroimaging. Postmortem methods produce well-known artifacts and admittedly it is extremely difficult to produce consistent results on a large scale; nevertheless, the implementation of “industrial” and carefully controlled protocols for tissue processing can greatly increase the quality and reproducibility of microscopic data. Common standards are needed for histological processing, as well as for the acquisition, storage, and sharing of digital histological files. The importance of incorporating maps of microscopic anatomy into the HCP and other neuroimaging studies ought to justify these efforts and promote substantial investments that will provide solutions to the logistic and technical challenges that still hinder postmortem studies of the human brain (Crick and Jones, 1993). Given that modern clinical strategies for prevention and treatment of neurological disease depend largely on knowledge acquired via non-invasive MR-based tools and thus from the indirect observation of developmental and neuropathogenetic phenomena, it is crucial at this point in time to support a new phase of validation studies based on rigorously prepared histological material. These studies ought to be commensurate both in scale and funding to the ambitious neuroimaging initiatives like the HCP. After all, truth is in the brain of the beholder.
Conflict of Interest Statement
The author declares that the research was conducted in the absence of any commercial or financial relationships that could be construed as a potential conflict of interest.
Acknowledgments
The author would like to acknowledge the contribution of all the research staff of The Brain Observatory. In particular Mr. Paul Maechler and Dr. Hauke Bartsch had a primary role in the generation of the data and results that were discussed in this communication. Jacopo Annese would like to thank Miss Colleen Sheh for the assistance in generating the illustrations and Miss Chris Ha and Dr. Justin Feinstein for their useful comments on the manuscripts.
The work was supported by two research grants from the National Eye Institute, R01 EY018359–02 and ARRA R01 EY018359–02S1 (Jacopo Annese Principal Investigator) and a research grant from the National Institute for Mental Health R01MH084756 (Jacopo Annese Principal Investigator).
References
Akil, H., Martone, M. E., and van Essen, D. C. (2011). Challenges and opportunities in mining neuroscience data. Science 331, 708–712.
Annese, J. (2010). “Deconstructing Henry. The Neuroanatomy and Neuroinformatics of the Brain of the Amnesic Patient H.M. Program No. 397.18,” Neuroscience Meeting Planner. 40th Annual Meeting of the Society for Neuroscience. (San Diego, CA). [Online].
Annese, J., Schenker, M. N., Ha, C., Maechler, P., Sheh, C., and Bartsch, H. (2012). A public atlas of the human brain. Web-resource. http://thebrainobservatory.ucsd.edu/content/public-brain-atlas
Annese, J., Pitiot, A., and Toga, A. W. (2004). A myelo-architectonic method for the structural classification of cortical areas. Neuroimage 21, 15–26.
Annese, J., Schenker, M. N., and Candido, C. (2009). “Anatomical mapping and digital pathology of the human brain by Giant Whole Slide Imaging (GWSI),” Program No. 104.5. Neuroscience Meeting Planner. 39th Annual Meeting of the Society for Neuroscience. (Chicago, IL). [Online].
Annese, J., Sforza, D. M., Dubach, M., Bowden, D., and Toga, A. W. (2006). Postmortem high resolution 3-dimensional imaging of the primate brain. Blockface imaging of perfusion stained tissue. Neuroimage 30, 61–69.
Bartsch, H., Maechler, P., and Annese, J. (2011). “Classification of NeuroAIDS-related white matter abnormalities using polarized light microscopy and histological staining in relation to Magnetic Resonance Imaging (MRI),” Program no. 782.01. Neuroscience Meeting Planner. 41st Annual Meeting of the Society for Neuroscience. (Washington, DC). [Online].
De Luca, M., Beckmann, C. F., de Stefano, N., Matthews, P. M., and Smith, S. M. (2006). fMRI resting state networks define distinct modes of long-distance interactions in the human brain. Neuroimage 29, 1359–1367.
Fischl, B., Destrieux, C., Halgren, E., Segonne, F., Salat, D. H., Busa, E., Seidman, L. J., Goldstein, J., Kennedy, D., Caviness, V., Makris, N., Rosen, B., and Dale, A. M. (2004). Automatic parcellation of the human cerebral cortex. Cereb. Cortex 14, 721–730.
Fischl, B., Salat, D., Albert, M., van der Kouwe, A., Killiany, R., Kennedy, D., Montillo, A., Makris, N., Rosen, B., and Dale, A. M. (2002). Whole brain segmentation: automated labeling of neuroanatomical structures in the human brain. Neuron 33, 341–355.
Flechsig, P. (1901). Developmental (myelogenetic) localisation of the cerebral cortex in the human subject. Lancet 2, 1027–1029.
Gallyas, F. (1979). Silver staining of myelin by means of physical development. Neurol. Res. 1, 203–209.
Johnson, G. A., Badea, A., Brandenburg, J., Cofer, G., Fubara, B., Liu, S., and Nissanov, J. (2010). Waxholm space: an image-based reference for coordinating mouse brain research. Neuroimage 53, 365–372.
Kretzschmar, H. (2009). Brain banking: opportunities, challenges and meaning for the future. Nat. Rev. Neurosci. 10, 70–77.
Leergaard, T. B., White, N. S., de Crespigny, A., Bolstad, I., D'Arceuil, H., Bjaalie, J. G., and Dale, A. M. (2010). Quantitative histological validation of diffusion MRI fiber orientation distributions in the rat brain. PloS One 5:8595. doi: 10.1371/journal.pone.0008595
Ludwig, E., and Klinger, J. (1956). Atlas Cerebris Humani: The Inner Structure of the Brain. Basel, Switzerland: Little, Brown and Company.
Marcus, D. S., Harwell, J., Olsen, T., Hodge, M., Glasser, M. F., Prior, F., Jenkinson, M., Laumann, T., Curtiss, S. W., and van Essen, D. C. (2011). Informatics and data mining tools and strategies for the human connectome project. Front. Neuroinform. 5:4. doi: 10.3389/fninf.2011.00004
Mikula, S., Trotts, I., Stone, J. M., and Jones, E. G. (2007). Internet-enabled high-resolution brain mapping and virtual microscopy. Neuroimage 35, 9–15.
Oishi, K., Faria, A. V., van Zijl, P. C. M., and Mori, S. (2011). MRI Atlas of Human White Matter, 2nd Edn. London, UK: Academic Press.
Pierpaoli, C., Jezzard, P., Basser, P. J., Barnett, A., and Di Chiro, G. (1996). Diffusion tensor MR imaging of the human brain. Radiology 201, 637–648.
Sporns, O., Tononi, G., and Kötter, R. (2005). The human connectome: a structural description of the human brain. PLoS Comput. Biol. 1:e42. doi: 10.1371/journal.pcbi.0010042
Keywords: histology, MRI, DTI, fibers, pathology, connectivity, human, brain
Citation: Annese J (2012) The importance of combining MRI and large-scale digital histology in neuroimaging studies of brain connectivity and disease. Front. Neuroinform. 6:13. doi: 10.3389/fninf.2012.00013
Received: 01 April 2011; Accepted: 31 March 2012;
Published online: 24 April 2012.
Edited by:
Olaf Sporns, Indiana University, USAReviewed by:
Trygve B. Leergaard, University of Oslo, NorwayKatrin Amunts, RWTH Aachen University, Germany
Copyright: © 2012 Annese. This is an open-access article distributed under the terms of the Creative Commons Attribution Non Commercial License, which permits non-commercial use, distribution, and reproduction in other forums, provided the original authors and source are credited.
*Correspondence: Jacopo Annese, Department of Radiology and Biomedical Imaging, The Brain Observatory, 3510 Dunhill Street, San Diego, CA 92121, USA. e-mail: jannese@ucsd.edu