- 1The Mind Research Network, Albuquerque, NM, United States
- 2Department of Psychology, Georgia State University, Atlanta, GA, United States
- 3Department of Electrical and Computer Engineering, Georgia Institute of Technology, Atlanta, GA, United States
- 4Tri-Institutional Center for Translational Research in Neuroimaging and Data Science (TReNDS), Georgia State University, Georgia Institute of Technology, Emory University, Atlanta, GA, United States
- 5Department of Computer Science, Georgia State University, Atlanta, GA, United States
- 6Department of Psychology, University of New Mexico, Albuquerque, NM, United States
Previous work in incarcerated boys and adult men and women suggest that individuals scoring high on psychopathic traits show altered resting-state limbic/paralimbic, and default mode functional network properties. However, it is unclear whether similar results extend to high-risk adolescent girls with elevated psychopathic traits. This study examined whether psychopathic traits [assessed via the Hare Psychopathy Checklist: Youth Version (PCL:YV)] were associated with altered inter-network connectivity, intra-network connectivity (i.e., functional coherence within a network), and amplitude of low-frequency fluctuations (ALFFs) across resting-state networks among high-risk incarcerated adolescent girls (n = 40). Resting-state networks were identified by applying group independent component analysis (ICA) to resting-state fMRI scans, and a priori regions of interest included limbic, paralimbic, and default mode network components. We tested the association of psychopathic traits (PCL:YV Factor 1 measuring affective/interpersonal traits and PCL:YV Factor 2 assessing antisocial/lifestyle traits) to these three resting-state measures. PCL:YV Factor 1 scores were associated with increased low-frequency and decreased high-frequency fluctuations in components corresponding to the default mode network, as well as increased intra-network FNC in components corresponding to cognitive control networks. PCL:YV Factor 2 scores were associated with increased low-frequency fluctuations in sensorimotor networks and decreased high-frequency fluctuations in default mode, sensorimotor, and visual networks. Consistent with previous analyses in incarcerated adult women, our results suggest that psychopathic traits among incarcerated adolescent girls are associated with altered intra-network ALFFs—primarily that of increased low-frequency and decreased high-frequency fluctuations—and connectivity across multiple networks including paralimbic regions. These results suggest stable neurobiological correlates of psychopathic traits among women across development.
1. Introduction
The construct of psychopathy is characterized as an array of traits, including callousness, impulsivity, poor decision-making, and a lack of empathy. These traits, alone, and in combination, have been found to be associated with poor interpersonal relationship success and treatment outcomes, and increased rates of substance use and rearrest (Taylor and Lang, 2006; Reidy et al., 2013; Mooney et al., 2019; Sohn et al., 2020; Allen et al., 2022a; Edwards et al., 2023). The societal cost of psychopathy to taxpayers is estimated to be nearly $460 billion per year, with $56.7 billion being accounted for by juveniles (Anderson, 1999; Caldwell et al., 2006; Kiehl and Hoffman, 2011; Cope et al., 2014; Reidy and Holland, 2018). Successful interventions for altering antisocial trajectories depend on gaining a better understanding of the underpinnings of such traits (Caldwell et al., 2006, 2007; Caldwell, 2011).
Research has identified a variety of causes and contributing conditions for psychopathic traits. Some of these include parenting style, childhood trauma, environmental exposures, and genetic make-up (Fergusson et al., 2008; Krischer and Sevecke, 2008; Wright et al., 2008; Marcus et al., 2010; Waller et al., 2012; Beckley et al., 2018; Sampson and Winter, 2018; Reuben et al., 2019). Brain imaging has shown that these traits are associated with altered functioning, primarily in limbic and paralimbic regions (e.g., insulae, temporal poles, posterior and anterior cingulate cortex, ventral striatum, and amygdalae) but also across the default mode network more generally [DM: e.g., precuneus and medial prefrontal cortex (mPFC): Chen et al., 2015; Cohn et al., 2015; Thijssen and Kiehl, 2017; Dugré and Potvin, 2021; Thijssen et al., 2021; Umbach and Tottenham, 2021; Werhahn et al., 2021; Winters et al., 2021]. While the literature investigating the relationship between functional connectivity and antisocial traits in adolescents is growing, there is an absence of research focusing specifically on high-risk adolescent girls with established poor behavioral outcomes (i.e., arrests and convictions). Prior studies have focused mainly on boys, leaving potential sex-specific developmental differences corresponding to psychopathic traits unexplored. With rates of incarceration of adolescent girls declining more slowly than those of boys (U.S. Department of Justice, Office of Justice Programs, 2021), and rates of incarceration of girls generally increasing on a global scale (Reynolds, 2008; Harmon and Boppre, 2018), the impetus to fill this gap is evident.
Because of the relatively sparse literature concerning resting-state alterations in adolescent girls relating to psychopathic traits, it is unclear whether alterations are stable from adolescence to adulthood, or rather, present differently in younger samples. Our research group recently explored resting-state alterations in adult women scoring high on psychopathic traits. We found that interpersonal/affective psychopathic traits (e.g., deficient empathy, a lack of remorse, and manipulativeness) were associated with increased amplitude of low-frequency fluctuations (ALFFs) in executive control and attentional networks, decreased high-frequency ALFFs in executive control and visual networks, and decreased intra-network connectivity in the default mode network. Lifestyle/antisocial psychopathic traits were associated with decreased high-frequency ALFFs in executive control and default mode networks, and both increased and decreased intra-network functional connectivity in visual networks (Allen et al., 2022b), diverging from similar analyses conducted in adult men scoring high on psychopathic traits which found no effects for the same measure (Espinoza et al., 2018). These results suggest potentially sex-specific neurobiological correlates of high-risk phenotypes, primarily occurring across regions and networks involved in socioemotional processing. Identifying stable or divergent neurobiological alterations in adolescent girls compared to those in adult women may inform our understanding of possible intervention targets designed to reduce psychopathic traits.
The relationship of psychopathic traits to other resting-state activational measures in incarcerated adolescent girls, including ALFFs, has been left unexplored. Due to their demonstrated association with functional connectivity more generally, and psychiatric disorders and behavioral characteristics, ALFFs may be a useful mode of investigation in relation to psychopathic traits (Guo et al., 2013; Yue et al., 2015; Wielaard et al., 2018; Eggart et al., 2019; Weightman et al., 2019; Zamani Esfahlani et al., 2020; Allen et al., 2022b; Gazula et al., 2022). By assessing the relationship of psychopathic traits to measures previously examined in the literature—such as altered inter-network and intra-network functional connectivity—as well as those unexamined in the literature (ALFFs), a more thorough picture of how these traits relate to altered functional brain connectivity on a local and global scale in relation to adolescent girls can be offered.
Here we examine resting-state metrics and their relationships to psychopathic traits [assessed via the Hare Psychopathy Checklist: Youth Version (PCL:YV)] (Forth et al., 2003) in a sample of incarcerated adolescent girls (n = 40). Resting networks were assessed using three different metrics [static functional network connectivity (sFNC: inter-network connectivity), ALFFs, and intra-network connectivity], to comprehensively evaluate local and global associations with psychopathic traits in incarcerated adolescent girls. We hypothesized that abnormalities in functional network connectivity related to psychopathic traits would occur primarily in limbic, paralimbic, and default mode network related regions of the brain (i.e., temporal poles, amygdalae, caudate/putamen, orbitofrontal cortex, dorsomedial prefrontal cortex, posterior cingulate cortex, and precunei). These regions span multiple cognitive domains, are involved in higher-order cognitive processes, such as emotion regulation, and are consistent with previously published studies in adolescent boys and adult men and women (Kiehl, 2006; Cope et al., 2014; Fairchild et al., 2014; Chen et al., 2015; Cohn et al., 2015; Philippi et al., 2015; Thijssen and Kiehl, 2017; Lindner et al., 2018; Dugré and Potvin, 2021; Thijssen et al., 2021; Umbach and Tottenham, 2021; Werhahn et al., 2021; Winters et al., 2021; Allen et al., 2022b). Parallel to analyses by our research group in incarcerated adult women and adolescent boys scoring high on psychopathic traits, this study serves an important role in assessing whether psychopathy-related neural alterations are consistent from adolescence to adulthood, or rather, present differently in younger samples of women.
2. Methods
2.1. Participants
Participants included adolescent girls from the National Institute of Mental Health (NIMH)-funded SouthWest Advanced Neuroimaging Cohort, Youth sample (SWANC-Y), at a maximum-security juvenile correctional facility in New Mexico, collected between June 2007 and March 2011. With an initial sample of n = 78, exclusions included participants that did not have a resting-state scan (n = 27), or PCL:YV administered (n = 7), and poor brain masks during scan (n = 4), leaving complete data sets from 40 incarcerated adolescent girls, ranging from 14 to 20 years of age.1 The average age of participants was 17.4 years (SD = 1.0: see Table 1). Using National Institutes of Health racial and ethnic classification, 45.0% of the sample self-identified as white, 7.5% as black/African American, 25.0% as American Indian or Alaskan Native, 22.5% as multiracial/other, and, ethnically, 60% as Hispanic or Latina. 97.5% participants were right-handed.
Participants provided written informed consent (if ≥18 years or age) or written informed assent and parent/guardian written informed consent (if < 18 years of age), in protocols approved by the Institutional Review Board of the University of New Mexico and were paid at a rate commensurate with institution compensation for work assignments at the correctional facility.
2.2. Assessments and measures
2.2.1. Psychopathic traits
Psychopathic traits were assessed using the Psychopathy Checklist: Youth Version (PCL:YV; Forth et al., 2003). The assessment includes a semi-structured interview covering individuals' school, family, work, and antisocial histories, as well as their interpersonal and emotional skills and a review of institutional records. Individuals are scored from zero to two on 20 different items that measure traits and behavioral characteristics of psychopathy, with total scores ranging from zero to 40 (see Kosson et al., 2002, 2013 for further assessment information). Interviews were conducted by trained researchers and videotaped for reliability assessment. Consistent with the literature (Thijssen and Kiehl, 2017), and in addition to PCL:YV Total scores, we examined a two-factor model of psychopathic traits (Harpur et al., 1989; Hare, 2003; Kennealy et al., 2007). The two-factor model of psychopathic traits was originally constructed via factor analysis (Harpur et al., 1989; Hare, 1991). Two correlated overarching factors that held explanatory value for the underlying individual items. Subsequent confirmatory factor analyses suggest each of these factors can be further explained by two underlying facets (Vitacco et al., 2005; Kosson et al., 2013). Specifically, Factor 1 is composed of interpersonal and affective facets (e.g., grandiosity and a lack of empathy), whereas Factor 2 is composed of antisocial and developmental facets (e.g., impulsivity and early behavioral problems). While the number of factors to extract for the PCL:YV is an open debate (Kosson et al., 2013), we chose to focus on the two-factor model for a more direct comparison to previous analyses in adult women and adolescent boy samples (see Thijssen and Kiehl, 2017; Allen et al., 2022b). An analysis of all PCL:YV items within this sample suggest high internal reliability (Cronbach's alpha = 0.82).
2.2.2. IQ
Participants' IQs were estimated from the Wechsler Intelligence Scale for Children-Fourth Edition (WISC-IV; Wechsler, 2003; Sattler and Dumont, 2004) for those younger than 16 years of age and from the Vocabulary and Matrix Reasoning subtests of the Wechsler Adult Intelligence Scale (WAIS-III; Wechsler, 1997; Ryan and Ward, 1999) for those older than 16 years of age. The mean full-scale IQ estimate in this sample was 98.1 (SD = 11.3: see Table 1); IQ scores were unavailable for eight participants and were subsequently mean replaced for inclusion in imaging analyses.
2.2.3. Diagnosis of psychiatric disorders
To assess whether or not participants met criteria for various forms of psychopathology, including mood disorders, anxiety disorders, post-traumatic stress disorder (PTSD), and attention-deficit/hyperactivity disorder (ADHD), we utilized the Kiddie Schedule for Affective Disorders and Schizophrenia (KSADS; scoring and criteria explained in Kaufman et al., 1997). Categorization of potential mood disorders included major depressive disorder (with and without psychotic features), melancholic depression, dysthymia, adjustment disorder with depressed mood, depressive disorder NOS, schizoaffective disorder (depressed and manic types), mania, hypomania, cyclothymia, or bipolar disorder NOS. Anxiety disorders included obsessive compulsive disorder, generalized anxiety disorder, acute stress disorder, panic disorder (with and without agoraphobia), separation anxiety, phobias (i.e., social phobia and/or specific phobias), agoraphobia, or an anxiety disorder not otherwise specified (NOS). Based on this criteria, and out of the 39 participants that were administered the KSADS, 15 participants met criteria for any mood disorder, five participants met criteria for any anxiety disorder, six met criteria for ADHD, and 11 participants met criteria for PTSD (see Table 1).
2.2.4. Substance use
For descriptive purposes, and similarly to other published methods (Cope et al., 2014; Edwards et al., 2023), we assessed substance use history using the KSADS, summing the total number of substances (alcohol, cannabis, sedatives/hypnotics/anxiolytics, cocaine, opioids, hallucinogens, stimulants, and solvents/inhalants/other) for which an individual met the lifetime dependence diagnostic criteria was calculated [substance dependence (SUD); theoretical range: 0–8, M = 2.6, SD = 1.6]. A dimensional score for SUD was used to provide a more meaningful and representative measure of substance use for the sample, as ~92% of participants met criteria for at least one SUD (see Table 1).
2.3. Imaging parameters
Resting-state functional magnetic resonance images were collected at the correctional facility where participants were housed, using the Mind Research Network's mobile Siemens 1.5T Avanto with advanced SQ gradients (max slew rate 200T/m/s, 346T/m/s vector summation, rise time 200 μs) equipped with a 12-element head coil. The EPI gradient echo pulse sequence (TR = 2,000 ms, TE = 39 ms, flip angle = 75, FOV = 24 × 24 cm, 64 × 64 matrix, 3.75 × 3.75 mm in-plane resolution, 4 mm slice thickness, 1 mm gap, 27 slices) effectively covered the entire brain (150 mm) in 2.0 s. Head motion was minimized using padding and restraint. The participants were asked to lay still, look at the fixation cross, and keep eyes open during the 5-min rsfMRI scanning.
2.4. EPI preprocessing
Data were preprocessed using statistical parametric mapping (SPM12) (Friston et al., 1994) (http://www.fil.ion.ucl.ac.uk/spm) including image reorientation, realignment [motion estimation using INRialign (Freire and Mangin, 2001)], and spatial normalization to the Montreal Neurological Institute standard space at a resolution of a 3 × 3 × 3 mm3. A full width half maximum Gaussian kernel of 6 mm was then used for spatial smoothing. Framewise displacement (FD) was used to assess motion quality control. For FD, the translation and rotation parameters were computed as the mean of the sums of the absolute translation and rotation frame displacements. Following the removal of participants with scans resulting in inadequate brain masks (i.e., those missing large areas of brain), all participants demonstrated a mean FD < 0.3 mm, and therefore, none were removed due to excessive motion (Stout et al., 2021). Additionally, ArtRepair was used to remove noise spikes larger than 4% of the global signal (Mazaika et al., 2007), further addressing the management of subject motion.
2.5. Independent component analysis
We applied group ICA (gICA) on the preprocessed rsfMRI data using the Group ICA of fMRI Toolbox (GIFT: http://trendscenter.org/software/gift) (Calhoun et al., 2001). The rsfMRI data was compressed using two stages of principal component analysis (PCA) (Rachakonda et al., 2016). Consistent with previously published studies, in the first step of data reduction, we retained 100 principal components (PCs), and 75 independent components (ICs) for group data reduction, (Kiviniemi et al., 2009; Smith et al., 2009; Ystad et al., 2010; Abou Elseoud et al., 2011; Allen et al., 2011a; Erhardt et al., 2011). High-model order ICA (i.e., 75 components) yields more refined components that correspond more closely to known functional and anatomical segmentations in comparison to low-model order ICA (i.e., 25 or 50 components) (Allen et al., 2011a; Hu et al., 2020). Participant specific spatial maps and their corresponding time-courses were obtained using gICA. Out of the 75 ICs that were estimated, 39 components were identified as components of RSNs by evaluating whether peak network activation occurred in gray matter and whether the peak ALFFs occurred in the low-frequency power portion of the spectra of components (see Figure 1 for whole-brain component solution: Meda et al., 2008; Robinson et al., 2009; Allen et al., 2011b). The other 36 components were excluded, as they appeared to be related to motion artifacts, the ventricular system, or cerebrospinal fluid, spatial maps including white matter, or having irregular time-course spectra power or low stability (Allen et al., 2011b). The reliability and stability of these extracted networks were evaluated via ICASSO (Himberg and Hyvarinen, 2003), a process that iteratively re-runs component estimations with alternatively bootstrapped datasets. This analysis suggested high stability across the selected 39 components (mean stability index = 0.94), well above the threshold of 0.80 established in the literature (Ma et al., 2011). Within GIFT, the time-courses of the RSNs underwent despiking and bandpass filtering with (0.01–0.15) Hz cutoffs. From the 39 extracted components, 10 components of interest were selected for the primary analyses based on relevant literature (see Figure 2 for a priori components of interest: Kiehl, 2006; Cope et al., 2014; Fairchild et al., 2014; Chen et al., 2015; Cohn et al., 2015; Philippi et al., 2015; Thijssen and Kiehl, 2017; Lindner et al., 2018; Dugré and Potvin, 2021; Thijssen et al., 2021; Umbach and Tottenham, 2021; Werhahn et al., 2021; Winters et al., 2021; Allen et al., 2022b).
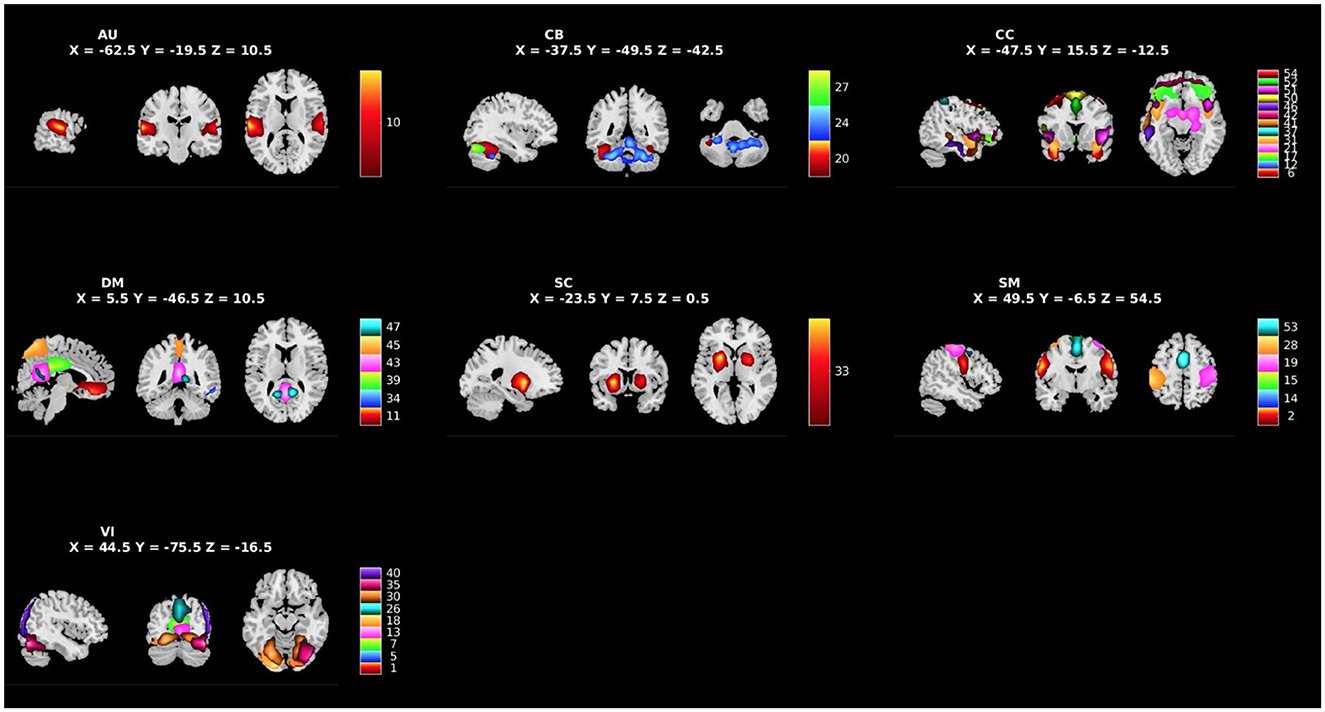
Figure 1. Spatial maps of the 39 independent components identified as RSNs categorized by domain [auditory (AU), cerebellar (CB), cognitive control (CC), default mode (DM), subcortical (SC), sensorimotor (SM), and visual (VI)] and component number.
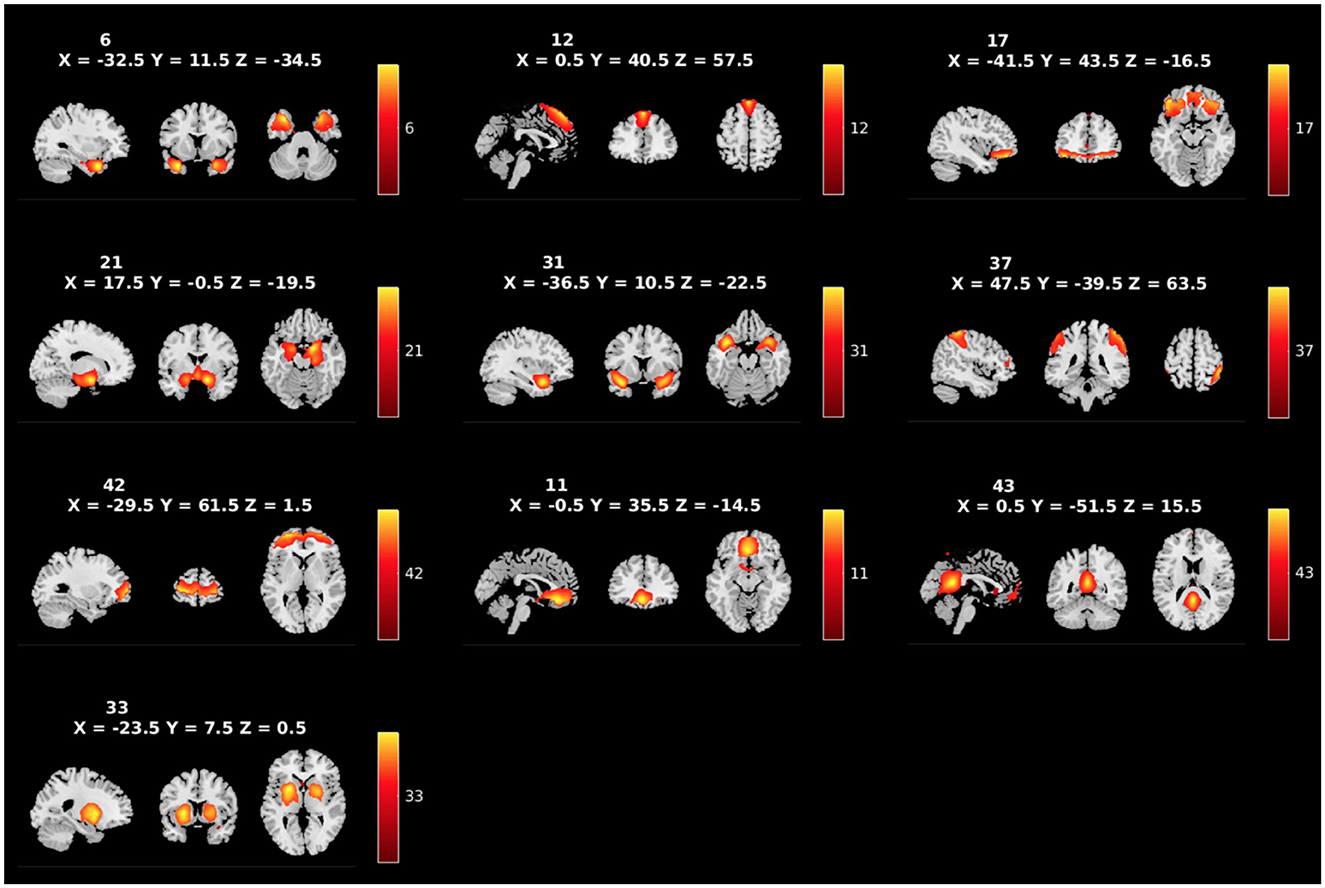
Figure 2. Spatial maps of the 10 RSNs identified as a priori networks of interest, labeled by component number. Broadly, the networks of interest include the temporal pole (ICs 6 and 31), the dmPFC (IC12), the pars orbitalis (IC17), the amygdalae (IC21), the precuneus (IC37), the aPFC (IC42), the OFC (IC11), the PCC (IC43), and the caudate/putamen (IC33).
2.6. Resting-state measures
In order to assess various types of resting-state functional connectivity and activational measures, using the GIFT toolbox and its suggested default parameters (http://trendscenter.org/software/gift) (Calhoun et al., 2001), we calculated the sFNC between the selected RSNs as pairwise correlations between the RSNs' time-courses for each individual (inter-network connectivity), pairwise correlations between individual voxels within the RSN to the overall RSN's time-course (intra-network connectivity), and the Fourier transform of individual RSN time-courses (ALFFs: decompositions of the time-course into the frequencies of activation and their amplitudes).
2.7. Statistical analyses
We performed regression analysis to identify associations between sFNC values (inter-network connectivity), spatial maps (intra-network connectivity), and ALFFs with psychopathy measures: PCL:YV Factor 1, Factor 2, and Total scores, which were included as continuous variables. The analyses were corrected for “nuisance” covariates (age at scan and IQ). Univariate associations between psychopathic traits and a priori networks of interest were first examined. For these region of interest analyses, we report both uncorrected and false discovery rate (FDR) corrected results, at an alpha level of 0.05 (Genovese et al., 2002; Thijssen and Kiehl, 2017). Additionally, we performed exploratory whole-brain analyses (i.e., tested all extracted components rather than solely a priori networks of interest) using FDR multiple comparison correction.
3. Results
3.1. Psychopathic traits
The PCL:YV total scores for this sample ranged from 11.0 to 36.0 (M = 23.0, SD = 6.1), the PCL:YV Factor 1 scores ranged from 1.0 to 13.0 (M = 7.2, SD = 3.3), and the PCL:YV Factor 2 scores ranged from 6.0 to 19.0 (M = 14.4, SD = 3.0; see Table 1 for descriptive statistics and Supplementary Table S1 for correlations with other psychiatric data).
3.2. Group independent component analysis and a priori component selection
Figure 1 shows the spatial maps of the 39 RSNs across the whole brain. The 39 RSNs listed in Supplementary Table S2 were grouped into seven domains: auditory (AU), cerebellar (CB), cognitive control (CC), default mode network (DM), subcortical (SC), sensorimotor (SM), and visual (VI) based on their peak coordinate, functional properties, the automatic labeling tool in GIFT (see Supplementary Figure S1 for average sFNC across all components and domains, suggesting high intra-domain sFNC: Du et al., 2020; Salman et al., 2022), and confirmed by visual inspection. From these 39 components, 10 components were selected as a priori candidates for analysis (see Figure 2: Philippi et al., 2015; Thijssen and Kiehl, 2017; Espinoza et al., 2018; Lindner et al., 2018; Allen et al., 2022b).
3.3. Time-course power spectra
3.3.1. PCL:YV Factor 1 scores
PCL:YV Factor 1 scores were associated with increased ALFF at low-frequency bands (0–0.05 Hz) in the aPFC (IC 42, CC) and PCC (IC43, DM), decreased ALFF at mid/high-frequency bands (0.10–0.20 Hz) in the dmPFC (IC12, CC), aPFC (IC 42, CC), and PCC (IC43, DM), and increased ALFF at high-frequency bands (0.20–0.25 Hz) in the precuneus (IC37, CC: see Figure 3A). Effects of increased ALFF at low-frequency bands (0–0.05 Hz) and decreased ALFF at mid-frequency bands (0.10–0.15 Hz) in the PCC (IC43, DM) remain following FDR correction and also emerge in the exploratory whole-brain analysis (see Figures 3B, C).
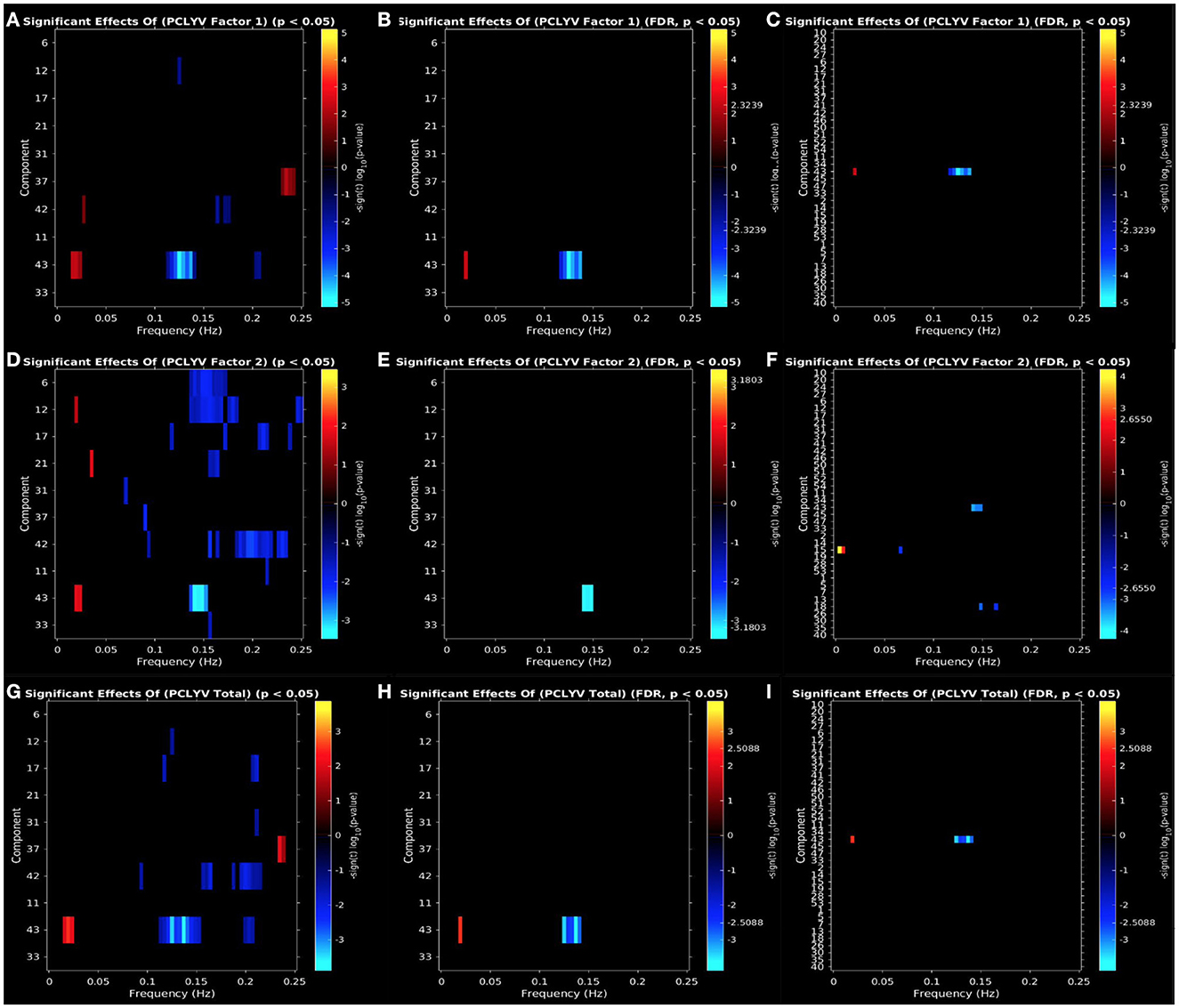
Figure 3. Univariate associations between psychopathic traits [(A–C): PCL:YV Factor 1, (D–F): PCL:YV Factor 2, (G–I): PCL:YV Total] and power spectra (ALFFs) of the significant components. Panel depicts the significance and direction of effects as a function of frequency for the significant components, displayed as–sign(t) log10(p), at an (A, D, G) uncorrected threshold of p < 0.05 and (B, E, H) FDR corrected p < 0.05 for a priori networks of interest, and (C, F, I) FDR corrected p < 0.05 for the exploratory whole-brain analysis.
3.3.2. PCL:YV Factor 2 scores
PCL:YV Factor 2 scores were associated with increased ALFF at low-frequency bands (0–0.05 Hz) in the dmPFC (IC12, CC), amygdalae (IC21, CC), and PCC (IC43, DM), and decreased ALFF at mid/high-frequency bands (0.10–0.25Hz) in the temporal pole (IC6 and IC31, CC), dmPFC (IC12, CC), pars orbitalis (IC17, CC), amygdalae (IC21, CC), precuneus (IC37, CC), aPFC (IC 42, CC), OFC (IC11, DM), PCC (IC43, DM), and caudate/putamen (IC33, SC: see Figure 3D). Effects of decreased ALFFs at mid-frequency bands in the PCC (IC43, DM) remain following FDR correction and also emerge in the exploratory whole-brain analysis (see Figures 3E, F). Additionally, the whole-brain analysis also suggests a relationship between PCL:YV Factor 2 scores and decreased ALFFs at mid-frequency bands (0.05–0.20 Hz) in the primary motor cortex (IC15, SM) and secondary visual cortex (IC18, VI), and increased ALFFs at low-frequency bands (0–0.01 Hz) in the primary motor cortex (IC15, SM: see Figure 3F).
3.3.3. PCL:YV Total scores
PCL:YV Total scores were associated with increased ALFF at low-frequency bands (0–0.05 Hz) in the PCC (IC43, DM), decreased ALFF at mid/high-frequency bands (0.10–0.25 Hz) in the temporal pole (IC31, CC), dmPFC (IC12, CC), pars orbitalis (IC17, CC), aPFC (IC 42, CC), and PCC (IC43, DM), and increased ALFF at high-frequency bands (0.20–0.25 Hz) in the precuneus (IC37, CC: see Figure 3G). Effects of increased ALFFs in low-frequency and decreased ALFFs at mid-frequency bands in the PCC (IC43, DM) remain following FDR correction and also emerge in the exploratory whole-brain analysis (see Figures 3H, I).
3.4. Intra-network connectivity
3.4.1. PCL:YV Factor 1 scores
PCL:YV Factor 1 scores were associated with altered intra-network connectivity in regions within all a priori networks of interest (see Table 2). Specifically, Factor 1 related increases of intra-network connectivity were found in the middle, and superior temporal gyrus, middle, medial, and inferior frontal gyrus, caudate, insula, parahippocampal gyrus, and lentiform nucleus. Factor 1 related decreases of intra-network connectivity were found in the superior temporal gyrus, parahippocampal gyrus, medial and inferior frontal gyrus, insula, caudate, anterior cingulate, and the precuneus (see Table 2). Effects of increased intra-network connectivity in the medial frontal gyrus relative to the dmPFC (IC12, CC) remain following FDR correction and also emerge in the exploratory whole-brain analysis (see Figure 4A, Table 2). Additionally, the whole-brain analysis also suggests a relationship between PCL:YV Factor 1 scores and increased intra-network connectivity in the middle temporal gyrus relative to the inferior temporal gyrus (see Figure 4B, Table 2).
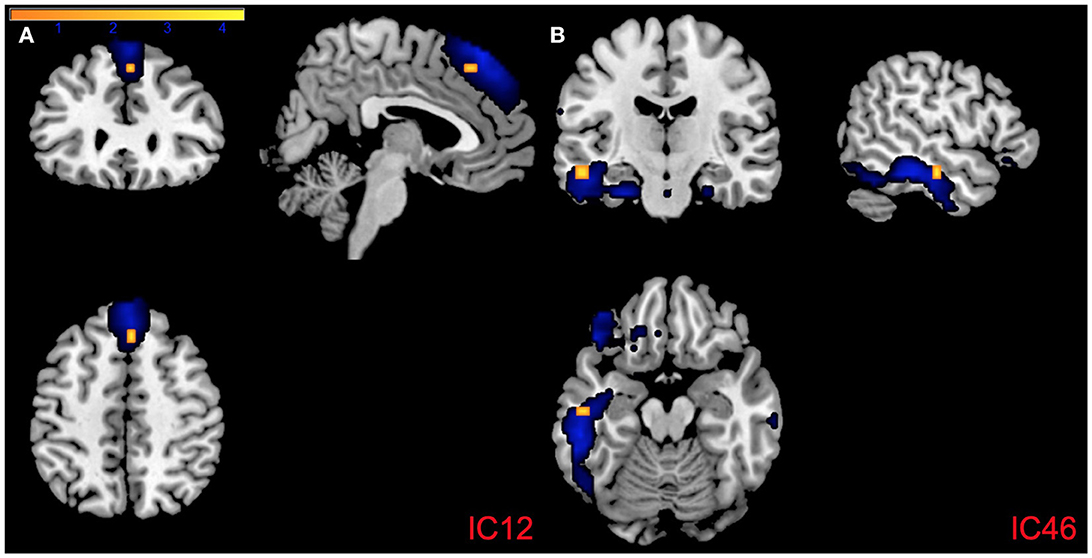
Figure 4. Regions of intra-network connectivity effects for visualization purposes. (A) Association between PCL:YV Factor 1 score and intra-network connectivity within component 12 and (B) component 46, FDR corrected p < 0.05. Blue mapping corresponds to component 12 and 46's spatial maps and orange reflects regions of increased intra-network connectivity, with the color bar indicating T-values ranging from 0 to 4.3.
3.4.2. PCL:YV Factor 2 scores
PCL:YV Factor 2 scores were associated with altered intra-network connectivity in regions within all a priori networks of interest (see Table 2). Specifically, Factor 2 related increases of intra-network connectivity were found in the medial, middle, and inferior frontal gyrus, middle and inferior temporal gyrus, culmen, caudate, parahippocampal gyrus, postcentral gyrus, supramarginal gyrus, uncus, extra-nuclear regions, and the inferior parietal lobule. Factor 2 related decreases of intra-network connectivity were found in the caudate, anterior cingulate, superior and middle temporal gyrus, supramarginal gyrus, postcentral gyrus, temporal pole, parahippocampal gyrus, medial frontal gyrus, and the inferior parietal lobule (see Table 2). After correction for FDR and controlling for age and IQ, neither a priori nor whole brain results emerged for PCL:YV Factor 2 scores.
3.4.3. PCL:YV Total scores
PCL:YV Total scores were associated with altered intra-network connectivity in regions within all a priori networks of interest (see Table 2). Specifically, PCL:YV Total score related increases of intra-network connectivity were found in the middle, inferior, and superior temporal gyrus, superior, inferior, middle, and medial frontal gyrus, extra-nuclear and sub-gyral regions, caudate, lentiform nucleus, and supramarginal gyrus. PCL:YV Total score related decreases of intra-network connectivity were found in the superior temporal gyrus, parahippocampal gyrus, inferior, superior, medial, and middle frontal gyrus, lentiform nucleus, temporal pole, anterior cingulate, postcentral gyrus, and precuneus (see Table 2). After correction for FDR and controlling for age and IQ, neither a priori nor whole brain results emerged for PCL:YV Total scores.
3.5. Inter-network connectivity
PCL:YV Factor 1 scores were associated with decreased sFNC between the pars orbitalis (IC17, CC) and both the precuneus (IC37, CC) and temporal pole (IC6, CC: see Figure 5A). PCL:YV Factor 2 scores were associated with decreased sFNC between the aPFC (IC42, CC) and the amygdalae (IC21, CC), and increased sFNC between the OFC (IC11, DM) and the pars orbitalis (IC17, CC: see Figure 5B). PCL:YV Total scores were associated with decreased sFNC between the pars orbitalis (IC17, CC) and the precuneus (IC37, CC: see Figure 5C). There were no significant a priori nor whole-brain associations between PCL:YV scores and sFNC that survived FDR correction while controlling for age and IQ.
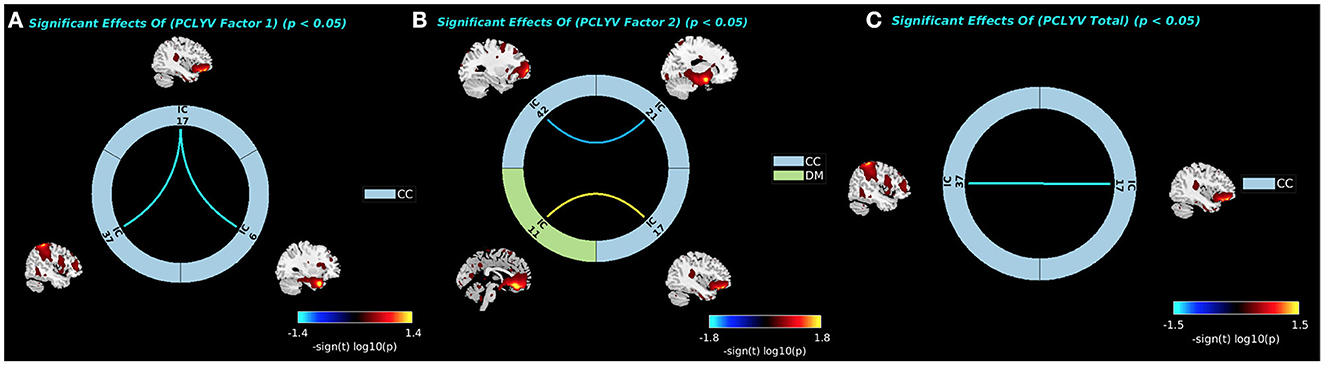
Figure 5. The significant associations between FNC values and PCL:YV Factor 1, Factor 2, and Total scores are shown with connecting curves between networks displayed as –sign(t) log10(p), color bars ranging from −1.4 to 1.4, −1.8 to 1.8, and −1.5 to 1.5, respectively. Red and orange colors correspond to positive correlations; and dark and light blue colors correspond to negative correlations. (A) Factor 1 effects showing negative associations between the pars orbitalis (IC17, CC) and both the precuneus (IC37, CC) and temporal pole (IC6, CC). (B) Factor 2 effects showing negative associations between the aPFC (IC42, CC) and the amygdalae (IC21, CC), and positive associations between the OFC (IC11, DM) and the pars orbitalis (IC17, CC). (C) PCL:YV Total effects showing negative associations between pars orbitalis (IC17, CC) and the precuneus (IC37, CC).
4. Discussion
The purpose of this study was to assess abnormalities in resting-state measures related to psychopathic traits in a sample of high-risk adolescent girls. We found that psychopathic traits (assessed via the PCL:YV) were associated with altered functional connectivity and ALFF during a resting-state fMRI experimental paradigm. Consistent with previous research performed in high-risk adolescent boys and adult men and women, PCL:YV scores were associated with altered ALFFs, and inter-/intra-network connectivity across multiple domains, with the majority of effects occurring in limbic, paralimbic, and default mode network regions (i.e., temporal poles, amygdalae, caudate/putamen, orbitofrontal cortex, dorsomedial prefrontal cortex, posterior cingulate cortex, and precunei: Thijssen and Kiehl, 2017; Allen et al., 2022b).
PCL:YV scores were associated with increased ALFFs in low- and high-frequency bands and reduced ALFFs in mid- frequency bands across regions in the CC, DM, SC, SM, and VI domains, with the most robust effects occurring in the PCC, primary motor cortex, and secondary visual cortex. These regions have been commonly implicated in previously published studies relating psychopathic traits to altered resting-state FNC in incarcerated adolescent boys (Cohn et al., 2015; Thijssen and Kiehl, 2017) and community adolescent boy and girl samples (Dugré and Potvin, 2021; Umbach and Tottenham, 2021; Werhahn et al., 2021; Winters et al., 2021). Likewise, the sparse literature investigating resting-state ALFFs and psychopathic traits in incarcerated adolescents have reported largely consistent findings with those obtained in the current study. Specifically, Thijssen and Kiehl (2017) observed that adolescent males scoring high on PCL:YV Factor 1 and Total scores were characterized by increased low-frequency (0–0.01 Hz), decreased low- to mid-frequency (0.05–0.07 Hz), and increased high-frequency (0.20–0.25 Hz) ALFFs in the DM, aligning with the above results: psychopathy-related increased ALFFs at low-frequency bands (0–0.05 Hz) and decreased ALFF at mid/high-frequency bands (0.10–0.15 Hz) in the PCC, and increased ALFFs at high-frequency bands (0.20–0.25 Hz) in the precuneus (see Figures 3A, G: Thijssen and Kiehl, 2017). Importantly, across both studies, these DM effects are accounted by variance in PCL:YV Factor 1, rather than Factor 2. These regions, more generally, are involved in higher-order cognitive processes, such as emotion regulation, movement and action coding/regulation, and attentional modulation (Leech and Sharp, 2014; Kreiman and Serre, 2020; Bhattacharjee et al., 2021).
Accordingly, research suggests that relatively higher-frequency ALFFs, compared to lower-frequency ALFFs, contribute to cognitive processes of higher-order nature (Baria et al., 2011; Craig et al., 2018; though see Biswal et al., 1995). Consistent with similar findings in incarcerated adult women scoring high on psychopathic traits (Allen et al., 2022b), reduced high-frequency ALFFs (e.g., in the PCC) may relate to previously observed deficits characteristic of youth scoring high on psychopathy, such as error-related processing deficits (Maurer et al., 2016). Successful error-related processing depends on the coordination of several brain regions, including psychopathy-related regions as identified above—the primary motor cortex and PCC (Steele et al., 2014). Furthermore, adolescent girls scoring high on psychopathic traits have been previously characterized by altered BOLD reactivity to stimuli featuring facial expressions in the secondary visual cortex, a region also showcasing altered mid- to high-frequency ALFFs related to psychopathic traits within our sample (Fairchild et al., 2014). Finally, and opposite to the deficit hypothesis, some research suggests that increased low-frequency ALFFs may correspond to refined neural efficiency (Biswal et al., 1995). Given findings suggesting that adolescent girls and adult women scoring high on psychopathic traits do not exhibit the same response perseveration deficits as comparable men (Vitale and Newman, 2001; Vitale et al., 2005), the increase in low-frequency ALFFs in regions implicated in response perseveration (i.e., the PCC), may reflect markers of increased neural efficiency (Ersche et al., 2011; Yang et al., 2011). One mechanism that may be giving rise to the observed ALFF effects is altered brain structure. Given that ALFF has been found to vary based on underlying structural differences (Qing and Gong, 2016), the observed effects (e.g., psychopathy related altered ALFF in the OFC) may be related to previously observed structural deficits in the majority of the present sample (Cope et al., 2014).
We also observed that adolescent girls scoring high on psychopathy were characterized by altered inter- and intra-network connectivity in CC and DM networks, with effects in the PFC, precuneus, temporal poles, amygdalae, dmPFC, and left ITG, with the most robust effects occurring in the latter two regions. Specifically, adolescent girls scoring high on psychopathic traits were characterized by increased intra-network functional connectivity within Component 12 (dmPFC) and Component 46 (left ITG), regions consistent with previous analyses in incarcerated adolescents (Chen et al., 2015; Cohn et al., 2015; Thijssen and Kiehl, 2017) and community adolescents (Dugré and Potvin, 2021; Thijssen et al., 2021; Umbach and Tottenham, 2021; Werhahn et al., 2021; Winters et al., 2021). Likewise, consistent with the present analyses and Thijssen and Kiehl (2017), psychopathy-related intra-network connectivity effects that survived FDR correction were constrained to PCL:YV Factor 1, but did not extend to PCL:YV Factor 2 or Total scores. Due to the important role that prefrontal and temporal regions play in emotional regulation, the altered intra-network effects observed in the dmPFC may indicate delayed—or altered—maturation between these circuits and subcortical regions involved in emotional processing (Rubia, 2013; Chen et al., 2015; Morawetz et al., 2016; Dugré and Potvin, 2022), potentially leading to the higher occurrence of antisocial actions associated with psychopathy.
Broadly, our investigation into the relationship between resting-state neurobiological alterations and psychopathic traits in incarcerated adolescent girls underscores two points. First, our results highlight the importance of considering multiple approaches to estimating resting-state alterations on a local (i.e., ALFFs and intra-network connectivity) and global (inter-network connectivity) scale, as these complimentary neurobiological measures are likely to account for alternative types of variance in explaining behavioral traits (see Thijssen and Kiehl, 2017). Second, our results suggest that altered resting-state functional connectivities associated with psychopathic traits present similarly in incarcerated adolescent girls and incarcerated adult women and adolescent boys (Allen et al., 2022b), potentially identifying stable markers for work seeking to predict subsequent antisocial actions utilizing brain-based metrics (Aharoni et al., 2013, 2022; Allen et al., 2022a). More specifically, psychopathy related paralimbic and default mode network alterations in the form of increased low-frequency ALFFs, decreased mid-frequency ALFFs, and increased high-frequency ALFFs were identified across adolescent and adult samples, suggesting that these neurobiological/trait correlates may be stable across development (Thijssen and Kiehl, 2017; Allen et al., 2022b). While the present work adds to a growing literature showcasing consistency in neurobiological alterations from youth to adulthood, future research stands to further explore how these neurobiological correlates may remain or differ from adolescence to adulthood in longitudinal samples of high-risk women.
4.1. Study limitations and future research
A number of limitations must be considered alongside the results presented. It is worth noting the small sample size (n = 40) compared to other similar analyses (Cohn et al., 2015; Thijssen and Kiehl, 2017; Espinoza et al., 2018; Allen et al., 2022b), potentially casting concerns on the reliability of the results presented. While this shortcoming is likely a side-effect of the low base rate of incarcerated adolescent girls compared to boys in forensic institutions, future studies could consider collaborative efforts that span multiple institutions and samples in order to strengthen the conclusions that can be drawn from the analyses. Additionally, another potential limitation of this study is the length of resting-state scan for the FNC measures being investigated (i.e., static FNC vs. dynamic FNC). While some research suggests that resting-state scans longer than 5-min are necessary to ensure high stability RSNs (Birn et al., 2013), other research finds shorter length scans adequate (Allen et al., 2011a; Espinoza et al., 2018, 2019; Duda et al., 2023). Static FNC, as compared to dynamic FNC, entails a number of assumptions regarding the coherence of the RSN relationships across the 5-min scan, thus, future work should consider exploring variable sliding window dFNC approaches in investigating the stability of resting-state alterations associated with psychopathic traits in incarcerated samples. Likewise, combined analysis of resting-state alterations with task-based scans (such as impulsivity or socioemotional processing tasks) should be considered in future work to not only test the generalizability of neurobiological correlates associated with psychopathic traits, but also corresponding functional and behavioral deficits that may differ during and through development. Thus, more work, and larger samples, are needed to probe the relationships between various functional activity and connectivity measures as they relate to psychopathic traits in incarcerated adolescent women.
4.2. Conclusion
Our results suggest that psychopathic traits among incarcerated adolescent girls are associated most robustly with altered intra-network amplitude of low-frequency fluctuations—primarily that of increased low-frequency and decreased mid- to high-frequency fluctuations—and connectivity across multiple networks including paralimbic and default mode network regions, including the PCC. These results, and their relative consistency to similar findings in incarcerated adult women and adolescent boys scoring high on psychopathy (Thijssen and Kiehl, 2017; Allen et al., 2022b), suggest stable neurobiological correlates of psychopathic traits across development. To our knowledge, this is the first study to date on the association of psychopathic traits and intrinsic RSN alterations in incarcerated high-risk adolescent girls.
Data availability statement
The datasets presented in this article are not readily available because of the potential for personal identification of participants in the present sensitive population (incarcerated adolescent girls). Interested parties should contact the corresponding author, KK for the data used in this report. Requests to access the datasets should be directed at: KK, a2tpZWhsQG1ybi5vcmc=.
Ethics statement
The studies involving human participants were reviewed and approved by Institutional Review Board of the University of New Mexico. Participants provided written informed consent (if ≥ 18 years or age) or written informed assent and parent/guardian written informed consent (if < 18 years of age).
Author contributions
CA: data curation, methodology, writing–original draft, formal analysis, and writing–review and editing. JM: data curation, methodology, writing-original draft, and writing-review and editing. AG, BE, NA, CH, KH, and KK: data curation, methodology, and writing-review and editing. EA and VC: methodology and writing-review and editing. All authors contributed to the article and approved the submitted version.
Funding
This material was based upon work supported by the National Institute of Health under Grant Nos. (R01MH071896, R01HD092331, and R01MH123610) and National Science Foundation grant number 2112455.
Conflict of interest
CA, JM, AG, BE, CH, NA, KH, and KK were employed by The Mind Research Network.
The remaining authors declare that the research was conducted in the absence of any commercial or financial relationships that could be construed as a potential conflict of interest.
Publisher's note
All claims expressed in this article are solely those of the authors and do not necessarily represent those of their affiliated organizations, or those of the publisher, the editors and the reviewers. Any product that may be evaluated in this article, or claim that may be made by its manufacturer, is not guaranteed or endorsed by the publisher.
Author disclaimer
The contents of this manuscript are solely the responsibility of the authors and do not necessarily represent the views of the National Institute of Health or the National Science Foundation.
Supplementary material
The Supplementary Material for this article can be found online at: https://www.frontiersin.org/articles/10.3389/fnimg.2023.1216494/full#supplementary-material
Footnotes
1. ^Previous analyses in a subset of the present sample demonstrate demographic similarity to adolescent boys scanned at the same facility in terms of age, IQ, substance dependence, psychopathic traits, and criminal convictions (Ermer et al., 2013; Cope et al., 2014).
References
Abou Elseoud, A., Littow, H., Remes, J., Starck, T., Nikkinen, J., Nissilä, J., et al. (2011). Group-ICA model order highlights patterns of functional brain connectivity. Front. Syst. Neurosci. 5, 37. doi: 10.3389/fnsys.2011.00037
Aharoni, E., Abdulla, S., Allen, C. H., and Nadelhoffer, T. (2022). 6 Ethical implications of neurobiologically informed risk assessment for criminal justice decisions: a case for pragmatism. Neurosci. Philos. 161–194. doi: 10.7551/mitpress/12611.001.0001
Aharoni, E., Vincent, G. M., Harenski, C. L., Calhoun, V. D., Sinnott-Armstrong, W., Gazzaniga, M. S., et al. (2013). Neuroprediction of future rearrest. Proc. Nat. Acad. Sci. 110, 6223–6228. doi: 10.1073/pnas.1219302110
Allen, C. H., Aharoni, E., Gullapalli, A. R., Edwards, B. G., Harenski, C. L., Harenski, K. A., et al. (2022a). Hemodynamic activity in the limbic system predicts reoffending in women. Neuroimage Clin. 36, 103238. doi: 10.1016/j.nicl.2022.103238
Allen, C. H., Maurer, J. M., Edwards, B. G., Gullapalli, A. R., Harenski, C. L., Harenski, K. A., et al. (2022b). Altered resting-state functional connectivity in incarcerated women with elevated psychopathic traits. Front. Neuroimaging 42, 971201. doi: 10.3389/fnimg.2022.971201
Allen, E. A., Erhardt, E. B., Damaraju, E., Gruner, W., Segall, J. M., Silva, R. F., et al. (2011a). A baseline for the multivariate comparison of resting-state networks. Front. Syst. Neurosci. 5, 2. doi: 10.3389/fnsys.2011.00002
Allen, E. A., Liu, J., Kiehl, K. A., Gelernter, J., Pearlson, G. D., Perrone-Bizzozero, N. I., et al. (2011b). Components of cross-frequency modulation in health and disease. Front. Syst. Neurosci. 5, 59. doi: 10.3389/fnsys.2011.00059
Anderson, D. A. (1999). The aggregate burden of crime. J. Law Econ. 42, 611–642. doi: 10.1086/467436
Baria, A. T., Baliki, M. N., Parrish, T., and Apkarian, A. V. (2011). Anatomical and functional assemblies of brain BOLD oscillations. J. Neurosci. 31, 7910–7919.
Beckley, A. L., Caspi, A., Broadbent, J., Harrington, H., Houts, R. M., Poulton, R., et al. (2018). Association of childhood blood lead levels with criminal offending. JAMA Pediatr. 172, 166–173. doi: 10.1001/jamapediatrics.2017.4005
Bhattacharjee, S., Kashyap, R., Abualait, T., Annabel Chen, S. H., Yoo, W. K., Bashir, S., et al. (2021). The role of primary motor cortex: more than movement execution. J. Mot. Behav. 53, 258–274. doi: 10.1080/00222895.2020.1738992
Birn, R. M., Molloy, E. K., Patriat, R., Parker, T., Meier, T. B., Kirk, G. R., et al. (2013). The effect of scan length on the reliability of resting-state fMRI connectivity estimates. Neuroimage. 83, 550–558.
Biswal, B., Zerrin Yetkin, F., Haughton, V. M., and Hyde, J. S. (1995). Functional connectivity in the motor cortex of resting human brain using echo-planar MRI. Magn. Reson. Med. 34, 537–541.
Caldwell, M., Skeem, J., Salekin, R., and Van Rybroek, G. (2006). Treatment response of adolescent offenders with psychopathy features: a 2-year follow-up. Crim. Justice Behav. 33, 571–596. doi: 10.1177/0093854806288176
Caldwell, M. F. (2011). Treatment-related changes in behavioral outcomes of psychopathy facets in adolescent offenders. Law Hum. Behav. 35, 275–287. doi: 10.1007/s10979-010-9239-z
Caldwell, M. F., McCormick, D. J., Umstead, D., and Van Rybroek, G. J. (2007). Evidence of treatment progress and therapeutic outcomes among adolescents with psychopathic features. Crim. Justice Behav. 34, 573–587. doi: 10.1177/0093854806297511
Calhoun, V. D., Adali, T., Pearlson, G. D., and Pekar, J. J. (2001). A method for making group inferences from functional MRI data using independent component analysis. Hum. Brain Mapp. 14, 140–151. doi: 10.1002/hbm.1048
Chen, C., Zhou, J., Liu, C., Witt, K., Zhang, Y., Jing, B., et al. (2015). Regional homogeneity of resting-state brain abnormalities in violent juvenile offenders: a biomarker of brain immaturity? J. Neuropsychiatry Clin. Neurosci. 27, 27–32. doi: 10.1176/appi.neuropsych.13030044
Cohn, M. D., Pape, L. E., Schmaal, L., van den Brink, W., van Wingen, G., Vermeiren, R. R., et al. (2015). Differential relations between juvenile psychopathic traits and resting state network connectivity. Hum. Brain Mapp. 36, 2396–2405. doi: 10.1002/hbm.22779
Cope, L. M., Ermer, E., Nyalakanti, P. K., Calhoun, V. D., and Kiehl, K. A. (2014). Paralimbic gray matter reductions in incarcerated adolescent females with psychopathic traits. J. Abnorm. Child Psychol. 42, 659–668. doi: 10.1007/s10802-013-9810-4
Craig, M. M., Manktelow, A. E., Sahakian, B. J., Menon, D. K., and Stamatakis, E. A. (2018). Spectral diversity in default mode network connectivity reflects behavioral state. J. Cogn. Neurosci. 30, 526–539.
Du, Y., Fu, Z., Sui, J., Gao, S., Xing, Y., Lin, D., et al. (2020). NeuroMark: An automated and adaptive ICA based pipeline to identify reproducible fMRI markers of brain disorders. NeuroImage: Clin. 28, 102375.
Duda, M., Iraji, A., Ford, J. M., Lim, K. O., Mathalon, D. H., Mueller, B. A., et al. (2023). Reliability and clinical utility of spatially constrained estimates of intrinsic functional networks from very short fMRI scans. Hum. Brain Mapp. 44, 2620–2635. doi: 10.1002/hbm.26234
Dugré, J. R., and Potvin, S. (2021). Impaired attentional and socio-affective networks in subjects with antisocial behaviors: a meta-analysis of resting-state functional connectivity studies. Psychol. Med. 51, 1249–1259. doi: 10.1017/S0033291721001525
Dugré, J. R., and Potvin, S. (2022). The origins of evil: from lesions to functional architecture of the antisocial brain. Front. Psychiatry 13, 969206. doi: 10.3389/fpsyt.2022.969206
Edwards, B. G., Gullapalli, A. R., Maurer, J. M., Ulrich, D. M., Harenski, C. L., Thomson, N. D., et al. (2023). Psychopathy and substance use predict recidivism in women: a 7-year prospective study. Personal Disord. 14, 419–428. doi: 10.1037/per0000615
Eggart, M., Lange, A., Binser, M. J., Queri, S., and Müller-Oerlinghausen, B. (2019). Major depressive disorder is associated with impaired interoceptive accuracy: a systematic review. Brain Sci. 9, 131. doi: 10.3390/brainsci9060131
Erhardt, E. B., Rachakonda, S., Bedrick, E. J., Allen, E. A., Adali, T., Calhoun, V. D., et al. (2011). Comparison of multi-subject ICA methods for analysis of fMRI data. Hum. Brain Mapp. 32, 2075–2095. doi: 10.1002/hbm.21170
Ermer, E., Cope, L. M., Nyalakanti, P. K., Calhoun, V. D., and Kiehl, K. A. (2013). Aberrant paralimbic gray matter in incarcerated male adolescents with psychopathic traits. J. Am. Acad. Child Adolesc. Psychiatr. 52, 94–103.
Ersche, K. D., Roiser, J. P., Abbott, S., Craig, K. J., Müller, U., Suckling, J., et al. (2011). Response perseveration in stimulant dependence is associated with striatal dysfunction and can be ameliorated by a D2/3 receptor agonist. Biol. Psychiatry 70, 754–762. doi: 10.1016/j.biopsych.2011.06.033
Espinoza, F. A., Anderson, N. E., Vergara, V. M., Harenski, C. L., Decety, J., Rachakonda, S., et al. (2019). Resting-state fMRI dynamic functional network connectivity and associations with psychopathy traits. NeuroImage: Clin. 24, 101970.
Espinoza, F. A., Vergara, V. M., Reyes, D., Anderson, N. E., Harenski, C. L., Decety, J., et al. (2018). Altered functional network connectivity in psychopathy from a large (N= 985) forensic sample. Hum. Brain Mapp. 39, 2624–2634. doi: 10.1002/hbm.24028
Fairchild, G., Hagan, C. C., Passamonti, L., Walsh, N. D., Goodyer, I. M., Calder, A. J., et al. (2014). Atypical neural responses during face processing in female adolescents with conduct disorder. J. Am. Acad. Child Adolesc. Psychiatry 53, 677–687. doi: 10.1016/j.jaac.2014.02.009
Fergusson, D. M., Boden, J. M., and Horwood, L. J. (2008). Dentine lead levels in childhood and criminal behaviour in late adolescence and early adulthood. J. Epidemiol. Community Health 62, 1045–1050. doi: 10.1136/jech.2007.072827
Forth, A. E., Kosson, D. S., and Hare, R. D. (2003). Hare The Psychopathy Checklist: Youth Version. Toronto, ON: Multi-Health Systems.
Freire, L., and Mangin, J. F. (2001). Motion correction algorithms may create spurious brain activations in the absence of subject motion. Neuroimage 14, 709–722. doi: 10.1006/nimg.2001.0869
Friston, K. J., Holmes, A. P., Worsley, K. J., Poline, J. P., Frith, C. D., Frackowiak, R. S., et al. (1994). Statistical parametric maps in functional imaging: a general linear approach. Hum. Brain Mapp. 2, 189–210. doi: 10.1002/hbm.460020402
Gazula, H., Rootes-Murdy, K., Holla, B., Basodi, S., Zhang, Z., Verner, E., et al. (2022). Federated analysis in COINSTAC reveals functional network connectivity and spectral links to smoking and alcohol consumption in nearly 2,000 adolescent brains. Neuroinformatics 21, 287–301. doi: 10.1007/s12021-022-09604-4
Genovese, C. R., Lazar, N. A., and Nichols, T. (2002). Thresholding of statistical maps in functional neuroimaging using the false discovery rate. Neuroimage. 15, 870–878.
Guo, W. B., Liu, F., Xun, G. L., Hu, M. R., Guo, X. F., Xiao, C. Q., et al. (2013). Reversal alterations of amplitude of low-frequency fluctuations in early and late onset, first-episode, drug-naive depression. Prog. Neuropsychopharmacol. Biol. Psychiatry 40, 153–159. doi: 10.1016/j.pnpbp.2012.08.014
Hare, R. D. (1991). Manual for the Revised Psychopathy Checklist, 1st Edn. Toronto, ON: Multi-Health Systems.
Hare, R. D. (2003). Manual for the Hare Psychopathy Checklist-Revised. Toronto, ON: Multi-Health Systems.
Harmon, M. G., and Boppre, B. (2018). Women of color and the war on crime: an explanation for the rise in Black female imprisonment. J. Ethn. Crim. Justice 16, 309–332. doi: 10.1080/15377938.2015.1052173
Harpur, T. J., Hare, R. D., and Hakstian, A. R. (1989). Two-factor conceptualization of psychopathy: construct validity and assessment implications. Psychol. Assess. 1, 6. doi: 10.1037/1040-3590.1.1.6
Himberg, J., and Hyvarinen, A. (2003). “ICASSO: software for investigating the reliability of ICA estimates by clustering and visualization,” in 2003 IEEE XIII Workshop on Neural Networks for Signal Processing (IEEE cat. No. 03TH8718) (IEEE), 259–268. doi: 10.1109/NNSP.2003.1318025
Hu, G., Waters, A. B., Aslan, S., Frederick, B., Cong, F., Nickerson, L. D., et al. (2020). Snowball ICA: a model order free independent component analysis strategy for functional magnetic resonance imaging data. Front. Neurosci. 14, 569657. doi: 10.3389/fnins.2020.569657
Kaufman, J., Birmaher, B., Brent, D., Rao, U. M. A., Flynn, C., Moreci, P., et al. (1997). Schedule for affective disorders and schizophrenia for school-age children-present and lifetime version (K-SADS-PL): initial reliability and validity data. J. Am. Acad. Child Adolesc. Psychiatry 36, 980–988. doi: 10.1097/00004583-199707000-00021
Kennealy, P. J., Hicks, B. M., and Patrick, C. J. (2007). Validity of factors of the psychopathy checklist—revised in female prisoners: discriminant relations with antisocial behavior, substance abuse, and personality. Assessment 14, 323–340. doi: 10.1177/1073191107305882
Kiehl, K. A. (2006). A cognitive neuroscience perspective on psychopathy: evidence for paralimbic system dysfunction. Psychiatry Res. 142, 107–128. doi: 10.1016/j.psychres.2005.09.013
Kiehl, K. A., and Hoffman, M. B. (2011). The criminal psychopath: history, neuroscience, treatment, and economics. Jurimetrics 51, 355.
Kiviniemi, V., Starck, T., Remes, J., Long, X., Nikkinen, J., Haapea, M., et al. (2009). Functional segmentation of the brain cortex using high model order group PICA. Hum. Brain Mapp. 30, 3865–3886. doi: 10.1002/hbm.20813
Kosson, D. S., Cyterski, T. D., Steuerwald, B. L., Neumann, C. S., and Walker-Matthews, S. (2002). The reliability and validity of the psychopathy checklist: youth version (PCL: YV) in nonincarcerated adolescent males. Psychol. Assess. 14, 97. doi: 10.1037/1040-3590.14.1.97
Kosson, D. S., Neumann, C. S., Forth, A. E., Salekin, R. T., Hare, R. D., Krischer, M. K., et al. (2013). Factor structure of the hare psychopathy checklist: youth version (PCL: YV) in adolescent females. Psychol. Assess. 25, 71. doi: 10.1037/a0028986
Kreiman, G., and Serre, T. (2020). Beyond the feedforward sweep: feedback computations in the visual cortex. Ann. N. Y. Acad. Sci. 1464, 222–241. doi: 10.1111/nyas.14320
Krischer, M. K., and Sevecke, K. (2008). Early traumatization and psychopathy in female and male juvenile offenders. Int. J. Law Psychiatry 31, 253–262. doi: 10.1016/j.ijlp.2008.04.008
Leech, R., and Sharp, D. J. (2014). The role of the posterior cingulate cortex in cognition and disease. Brain 137, 12–32. doi: 10.1093/brain/awt162
Lindner, P., Flodin, P., Budhiraja, M., Savic, I., Jokinen, J., Tiihonen, J., et al. (2018). Associations of psychopathic traits with local and global brain network topology in young adult women. Biol. Psychiatry 3, 1003–1012. doi: 10.1016/j.bpsc.2018.04.010
Ma, S., Correa, N. M., Li, X. L., Eichele, T., Calhoun, V. D., and Adali, T. (2011). Automatic identification of functional clusters in FMRI data using spatial dependence. IEEE Trans. Biomed. Eng. 58, 3406–3417.
Marcus, D. K., Fulton, J. J., and Clarke, E. J. (2010). Lead and conduct problems: a meta-analysis. J. Clin. Child Adolesc. Psychol. 39, 234–241. doi: 10.1080/15374411003591455
Maurer, J. M., Steele, V. R., Edwards, B. G., Bernat, E. M., Calhoun, V. D., Kiehl, K. A., et al. (2016). Dysfunctional error-related processing in female psychopathy. Soc. Cogn. Affect. Neurosci. 11, 1059–1068. doi: 10.1093/scan/nsv070
Mazaika, P., Whitfield-Gabrieli, S., Reiss, A., and Glover, G. (2007). Artifact repair for fMRI data from high motion clinical subjects. Hum. Brain Mapp. 47, 70238–1. Available online at: https://cibsr.stanford.edu/content/dam/sm/cibsr/documents/tools/methods/artrepair-software/ArtRepairHBM2007.pdf
Meda, S. A., Giuliani, N. R., Calhoun, V. D., Jagannathan, K., Schretlen, D. J., Pulver, A., et al. (2008). A large scale (N5400) investigation of gray matter differences in schizophrenia using optimized voxel-based morphometry. Schizophr. Res. 101, 95–105. doi: 10.1016/j.schres.2008.02.007
Mooney, R., Ireland, J. L., and Lewis, M. (2019). Understanding interpersonal relationships and psychopathy. J. Forensic Psychiatry Psychol. 30, 658–685. doi: 10.1080/14789949.2019.1615102
Morawetz, C., Bode, S., Baudewig, J., Jacobs, A. M., and Heekeren, H. R. (2016). Neural representation of emotion regulation goals. Hum. Brain Mapp. 37, 600–620. doi: 10.1002/hbm.23053
Philippi, C. L., Pujara, M. S., Motzkin, J. C., Newman, J., Kiehl, K. A., Koenigs, M., et al. (2015). Altered resting-state functional connectivity in cortical networks in psychopathy. J. Neurosci. 35, 6068–6078. doi: 10.1523/JNEUROSCI.5010-14.2015
Qing, Z., and Gong, G. (2016). Size matters to function: brain volume correlates with intrinsic brain activity across healthy individuals. Neuroimage 139, 271–278. doi: 10.1016/j.neuroimage.2016.06.046
Rachakonda, S., Silva, R. F., Liu, J., and Calhoun, V. D. (2016). Memory efficient PCA methods for large group ICA. Front. Neurosci. 10, 17. doi: 10.3389/fnins.2016.00017
Reidy, D. E., and Holland, K. M. (2018). “Psychopathy: an obscure public health issue,” in Routledge International Handbook of Psychopathy and Crime (London: Routledge), 635–644. doi: 10.4324/9781315111476-40
Reidy, D. E., Kearns, M. C., and DeGue, S. (2013). Reducing psychopathic violence: a review of the treatment literature. Aggress. Violent Behav. 18, 527–538. doi: 10.1016/j.avb.2013.07.008
Reuben, A., Schaefer, J. D., Moffitt, T. E., Broadbent, J., Harrington, H., Houts, R. M., et al. (2019). Association of childhood lead exposure with adult personality traits and lifelong mental health. JAMA Psychiatry 76, 418–425. doi: 10.1001/jamapsychiatry.2018.4192
Reynolds, M. (2008). The war on drugs, prison building, and globalization: catalysts for the global incarceration of women. NWSA J. 20, 72–95. Available online at: https://www.semanticscholar.org/paper/The-War-on-Drugs%2C-Prison-Building%2C-and-Catalysts-of-Reynolds/3dd087873bb996bc1b185a7583121128514401d7
Robinson, S., Basso, G., Soldati, N., Sailer, U., Jovicich, J., Bruzzone, L., et al. (2009). A resting state network in the motor control circuit of the basal ganglia. BMC Neurosci. 10, 137. doi: 10.1186/1471-2202-10-137
Rubia, K. (2013). Functional brain imaging across development. Eur. Child Adolesc. Psychiatry 22, 719–731. doi: 10.1007/s00787-012-0291-8
Ryan, J. J., and Ward, L. C. (1999). Validity, reliability, and standard errors of measurement for two seven-subtest short forms of the Wechsler Adult Intelligence Scale—III. Psychol. Assess. 11, 207. doi: 10.1037/1040-3590.11.2.207
Salman, M. S., Wager, T. D., Damaraju, E., Abrol, A., Vergara, V. M., Fu, Z., et al. (2022). An approach to automatically label and order brain activity/component maps. Brain Connect. 12, 85–95.
Sampson, R. J., and Winter, A. S. (2018). Poisoned development: assessing childhood lead exposure as a cause of crime in a birth cohort followed through adolescence. Criminology 56, 269–301. doi: 10.1111/1745-9125.12171
Sattler, J. M., and Dumont, R. (2004). Assessment of Children: WISC-IV and WPPSI-III Supplement. La Mesa, CA: Jerome M. Sattler, Publisher.
Smith, S. M., Fox, P. T., Miller, K. L., Glahn, D. C., Fox, P. M., Mackay, C. E., et al. (2009). Correspondence of the brain's functional architecture during activation and rest. Proc. Nat. Acad. Sci. 106, 13040–13045. doi: 10.1073/pnas.0905267106
Sohn, J. S., Raine, A., and Lee, S. J. (2020). The utility of the psychopathy checklist-revised (PCL-R) facet and item scores in predicting violent recidivism. Aggress. Behav. 46, 508–515. doi: 10.1002/ab.21922
Steele, V. R., Claus, E. D., Aharoni, E., Harenski, C., Calhoun, V. D., Pearlson, G., et al. (2014). A large scale (N = 102) functional neuroimaging study of error processing in a Go/NoGo task. Behav. Brain Res. 268, 127–138.
Stout, D. M., Harlé, K. M., Norman, S. B., Simmons, A. N., and Spadoni, A. D. (2021). Resting-state connectivity subtype of comorbid PTSD and alcohol use disorder moderates improvement from integrated prolonged exposure therapy in Veterans. Psychol. Med. 53, 332–341. doi: 10.1017/S0033291721001513
Taylor, J., and Lang, A. R. (2006). Psychopathy and Substance Use Disorders. Available online at: https://psycnet.apa.org/record/2006-01001-025
Thijssen, S., Collins, P. F., Weiss, H., and Luciana, M. (2021). The longitudinal association between externalizing behavior and frontoamygdalar resting-state functional connectivity in late adolescence and young adulthood. J. Child Psychol. Psychiatry 62, 857–867. doi: 10.1111/jcpp.13330
Thijssen, S., and Kiehl, K. A. (2017). Functional connectivity in incarcerated male adolescents with psychopathic traits. Psychiatr Res. Neuroimaging 265, 35–44. doi: 10.1016/j.pscychresns.2017.05.005
U.S. Department of Justice Office of Justice Programs. (2021). Juvenile Justice Statistics, National Report Series Bulletin: Juvenile Arrests, 2019. Washington, DC: U.S. Department of Justice.
Umbach, R. H., and Tottenham, N. (2021). Callous-unemotional traits and reduced default mode network connectivity within a community sample of children. Dev. Psychopathol. 33, 1170–1183. doi: 10.1017/S0954579420000401
Vitacco, M. J., Rogers, R., Neumann, C. S., Harrison, K. S., and Vincent, G. (2005). A comparison of factor models on the PCL-R with mentally disordered offenders: the development of a four-factor model. Crim. Justice Behav. 32, 526–545. doi: 10.1177/0093854805278414
Vitale, J. E., and Newman, J. P. (2001). Response perseveration in psychopathic women. J. Abnorm. Psychol. 110, 644. doi: 10.1037/0021-843X.110.4.644
Vitale, J. E., Newman, J. P., Bates, J. E., Goodnight, J., Dodge, K. A., Pettit, G. S., et al. (2005). Deficient behavioral inhibition and anomalous selective attention in a community sample of adolescents with psychopathic traits and low-anxiety traits. J. Abnorm. Child Psychol. 33, 461–470. doi: 10.1007/s10802-005-5727-X
Waller, R., Gardner, F., Hyde, L. W., Shaw, D. S., Dishion, T. J., Wilson, M. N., et al. (2012). Do harsh and positive parenting predict parent reports of deceitful-callous behavior in early childhood? J. Child Psychol. Psychiatry 53, 946–953. doi: 10.1111/j.1469-7610.2012.02550.x
Wechsler, D. (2003). Wechlser Intelligence Scale for Children. 4. San Antonio, TX: Psychological Corporation; 2003. doi: 10.1037/t15174-000
Weightman, M. J., Knight, M. J., and Baune, B. T. (2019). A systematic review of the impact of social cognitive deficits on psychosocial functioning in major depressive disorder and opportunities for therapeutic intervention. Psychiatry Res. 274, 195–212. doi: 10.1016/j.psychres.2019.02.035
Werhahn, J. E., Mohl, S., Willinger, D., Smigielski, L., Roth, A., Hofstetter, C., et al. (2021). Aggression subtypes relate to distinct resting state functional connectivity in children and adolescents with disruptive behavior. Eur. Child Adolesc. Psychiatry 30, 1237–1249. doi: 10.1007/s00787-020-01601-9
Wielaard, I., Hoyer, M., Rhebergen, D., Stek, M. L., and Comijs, H. C. (2018). Childhood abuse and late-life depression: mediating effects of psychosocial factors for early-and late-onset depression. Int. J. Geriatr. Psychiatry 33, 537–545. doi: 10.1002/gps.4828
Winters, D. E., Sakai, J. T., and Carter, R. M. (2021). Resting-state network topology characterizing callous-unemotional traits in adolescence. Neuroimage Clin. 32, 102878. doi: 10.1016/j.nicl.2021.102878
Wright, J. P., Dietrich, K. N., Ris, M. D., Hornung, R. W., Wessel, S. D., Lanphear, B. P., et al. (2008). Association of prenatal and childhood blood lead concentrations with criminal arrests in early adulthood. PLoS Med. 5, e101. doi: 10.1371/journal.pmed.0050101
Yang, Y., Raine, A., Colletti, P., Toga, A. W., and Narr, K. L. (2011). Abnormal structural correlates of response perseveration in individuals with psychopathy. J. Neuropsychiatry Clin. Neurosci. 23, 107–110. doi: 10.1176/appi.neuropsych.23.1.107
Ystad, M., Eichele, T., Lundervold, A. J., and Lundervold, A. (2010). Subcortical functional connectivity and verbal episodic memory in healthy elderly—a resting state fMRI study. Neuroimage 52, 379–388. doi: 10.1016/j.neuroimage.2010.03.062
Yue, Y., Jia, X., Hou, Z., Zang, Y., and Yuan, Y. (2015). Frequency-dependent amplitude alterations of resting-state spontaneous fluctuations in late-onset depression. Biomed. Res. Int. 2015, 505479. doi: 10.1155/2015/505479
Keywords: psychopathic traits, functional connectivity, intra-network connectivity, spectra, ALFF, antisocial
Citation: Allen CH, Maurer JM, Gullapalli AR, Edwards BG, Aharoni E, Harenski CL, Anderson NE, Harenski KA, Calhoun VD and Kiehl KA (2023) Psychopathic traits and altered resting-state functional connectivity in incarcerated adolescent girls. Front. Neuroimaging 2:1216494. doi: 10.3389/fnimg.2023.1216494
Received: 03 May 2023; Accepted: 19 July 2023;
Published: 04 August 2023.
Edited by:
Frederic Sampedro, Sant Pau Institute for Biomedical Research, SpainReviewed by:
Ning Tu, Renmin Hospital of Wuhan University, ChinaZonglei Zhen, Beijing Normal University, China
Copyright © 2023 Allen, Maurer, Gullapalli, Edwards, Aharoni, Harenski, Anderson, Harenski, Calhoun and Kiehl. This is an open-access article distributed under the terms of the Creative Commons Attribution License (CC BY). The use, distribution or reproduction in other forums is permitted, provided the original author(s) and the copyright owner(s) are credited and that the original publication in this journal is cited, in accordance with accepted academic practice. No use, distribution or reproduction is permitted which does not comply with these terms.
*Correspondence: Corey H. Allen, Y2FsbGVuQG1ybi5vcmc=; Kent A. Kiehl, a2tpZWhsQG1ybi5vcmc=