- Experimental Therapeutics and Pathophysiology Branch, National Institute of Mental Health, National Institutes of Health, Bethesda, MD, United States
Functional magnetic resonance imaging (fMRI) is a non-invasive technique that can be used to examine neural responses with and without the use of a functional task. Indeed, fMRI has been used in clinical trials and pharmacological research studies. In mental health, it has been used to identify brain areas linked to specific symptoms but also has the potential to help identify possible treatment targets. Despite fMRI's many advantages, such findings are rarely the primary outcome measure in clinical trials or research studies. This article reviews fMRI studies in depression that sought to assess the efficacy and mechanism of action of compounds with antidepressant effects. Our search results focused on selective serotonin reuptake inhibitors (SSRIs), the most commonly prescribed treatments for depression and ketamine, a fast-acting antidepressant treatment. Normalization of amygdala hyperactivity in response to negative emotional stimuli was found to underlie successful treatment response to SSRIs as well as ketamine, indicating a potential common pathway for both conventional and fast-acting antidepressants. Ketamine's rapid antidepressant effects make it a particularly useful compound for studying depression with fMRI; its effects on brain activity and connectivity trended toward normalizing the increases and decreases in brain activity and connectivity associated with depression. These findings highlight the considerable promise of fMRI as a tool for identifying treatment targets in depression. However, additional studies with improved methodology and study design are needed before fMRI findings can be translated into meaningful clinical trial outcomes.
1. Introduction
Functional magnetic resonance imaging (fMRI) is a commonly-used, non-invasive technique used to study the living human brain. fMRI refers to the acquisition of data modulated with extrinsic (e.g., task stimulus) stimuli or allowed to follow intrinsic fluctuations (e.g., thought). It is typically conducted using blood oxygen level dependent (BOLD) sensitivity methods—such as resting-state and task-based MRI.
In pharmacological research, fMRI is mainly used to help develop and validate markers associated with brain dysfunction in specific disorders (Sadraee et al., 2021). Examining these fMRI markers as potential treatment targets can improve our understanding of the impact that certain compounds have on brain function and structure (Carmichael et al., 2018). In clinical trials, fMRI can be used in the earlier stages of drug development to help detect whether the compound under investigation causes central nervous system (CNS) changes in brain regions relevant to the study population. In that respect, fMRI could be a useful tool for the NIMH-proposed “fast-fail” approach where pharmacological compounds are assessed based on their efficacy to modulate the function of specific treatment targets. This approach aims to speed up drug development by quickly discarding compounds that fail to demonstrate efficacy in affecting biomarkers relevant to the disorders they are investigated for (From Discovery to Cure, 2010). In later-stage clinical trials, fMRI can help detect the normalization of a disease-related signal at a treatment-relevant dose (Carmichael et al., 2018; Sadraee et al., 2021).
fMRI is the primary tool used in research studies of both patients and healthy volunteers that seek to examine brain function and evaluate drug action in mental health. A great example of the efficacy of fMRI as a primary outcome measure in clinical trials that aims to quickly and reliably investigate pharmacological compounds in mental health disorders is the study by Krystal et al. In this study, fMRI signal changes in the VS (Ventral Striatum) during reward anticipation, were successfully used as a primary outcome measure to assess the efficacy of a κ-opioid receptor in a population with anhedonia and mood or anxiety disorder (Krystal et al., 2020). Despite its many advantages, fMRI is not commonly included as a primary outcome measure in clinical trials, even in those investigating mental health disorders. However, in depression, for example, clinical trials still rely primarily on scales and questionnaires that measure symptom changes before and after the administration of specific compounds with antidepressant action to assess drug efficacy. Such instruments, although important in disease diagnosis and in the identification of symptom changes before and after treatment, offer no insight as to the mechanisms of action of the antidepressant compounds under investigation or the brain areas targeted by these compounds.
In this context, fMRI offers an objective research tool that, if used effectively, could enable not only the characterization of brain-related deficits associated with depression and other mental health disorders but also help identify drug targets and compare brain activity between different drug conditions (Tracey and Wise, 2001; Wise and Tracey, 2006; Carmichael et al., 2018). Such information is critical for determining the specificity of targets that could be investigated as potential biomarkers for these disorders as well as their treatment. Despite these advantages and the technological progress of the field, fMRI research findings still do not easily translate to results with immediate clinical value.
This review seeks to understand the use of fMRI in pharmacological clinical trials and research studies in depression. It focuses on compounds with antidepressant action—mainly selective serotonin reuptake inhibitors (SSRIs) and the glutamatergic modulator ketamine—to identify the brain areas and networks targeted by these compounds that could serve as potential biomarkers of antidepressant response. The results presented here can be used to consider fMRI as a primary outcome measure for clinical trials and experimental research studies. Broader lessons regarding what has been learned from the use of fMRI in clinical trials and research studies are also discussed, and issues that could impede its use as a primary outcome measure in clinical trials are addressed. Finally, future directions that could further enhance the role of the technique in pharmacological studies are proposed.
2. Methodology
2.1. Study selection
Two clinical trial databases were searched to identify clinical trials of pharmacological compounds that target depression and have used fMRI: clinicaltrials.gov (clinicaltrials.gov) and the ISRCTN database (https://www.isrctn.com). Search terms included: “depression,” “completed,” “adult,” “interventional,” “with results,” and “imaging”. Our search terms were kept broad to be maximally inclusive, although this could result in a high exclusion rate. These search criteria were selected to identify completed studies with published results that have used imaging and pharmacological interventions in adult populations. Identified studies were assessed for their relevance in depression. An additional search was then conducted using the trial number for each study, in order to ensure that publications directly related to these trials but not listed in the clinical trials databases were also examined. All trials conducted using pharmacological compounds with antidepressant action that used fMRI (resting-state and/or task-based) to assess their efficacy and/or use in depression were included in this review. This selection process is outlined in Supplementary Data Sheet S1.
As a second-level search, the antidepressants used in the clinical trials identified in the first search were entered as search terms in PubMed (https://pubmed.ncbi.nlm.nih.gov). This was done to identify research studies relevant to depression that used fMRI (resting-state and/or task-based) to examine the effects of these compounds on brain activity and connectivity in healthy volunteers or patient groups but not in a clinical trial setting. Research studies that used compounds with antidepressant action solely as models for mental health disorders (for example, ketamine as a model for psychosis) without assessing the efficacy of these compounds in treating depression were excluded.
Trial selection and reporting of relevant studies followed the PRISMA guidelines for Systematic Review, as indicated in Supplementary Data Sheet S1. All publications were assessed for eligibility by the authors. The NbN3 nomenclature was followed to describe compounds with antidepressant action (Frazer and Blier, 2016).
2.2. Study classification
All studies fulfilling the search and selection criteria are presented in Tables 1, 2. Table 1 includes publications from studies selected using the clinical trial databases, and Table 2 includes research studies that investigated antidepressants identified from the clinical trials search. Studies were classified based on whether they used task-based or resting-state fMRI. A brief description of the recruited sample, methodology, main findings, and conclusions from each study are also included in the tables. Sample size and age, functional imaging technique, as well as the mechanisms of action of the compounds investigated in all included studies are summarized in Figure 1.
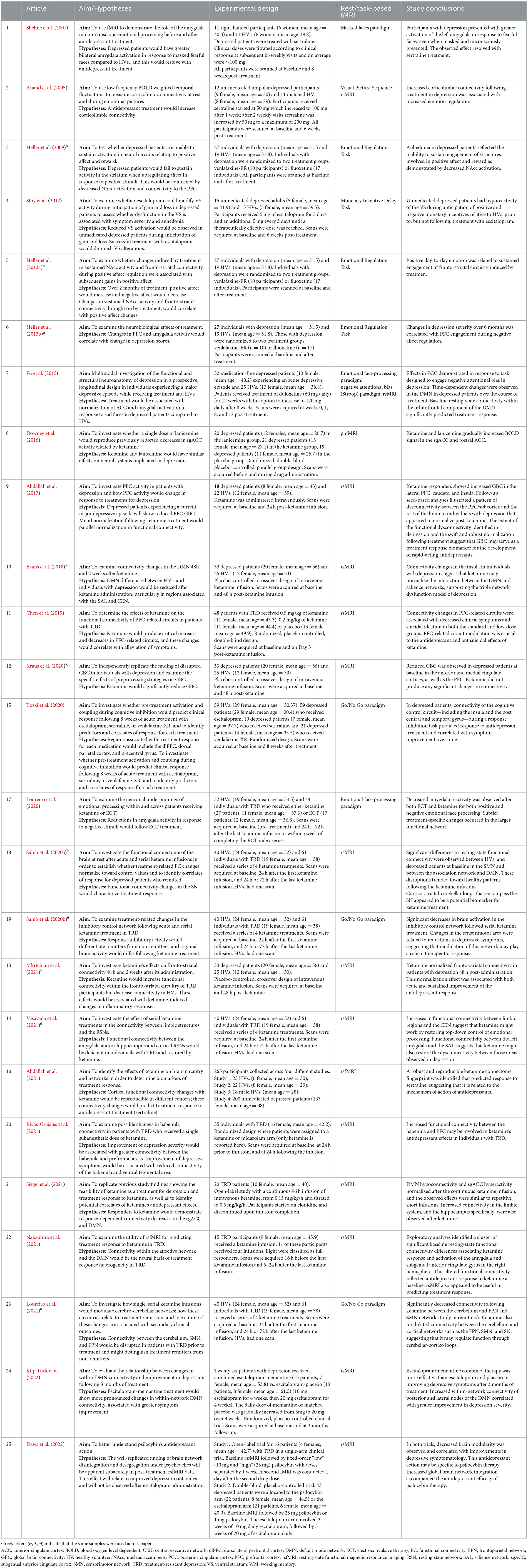
Table 1. Clinical trials investigating compounds with antidepressant action. Articles are listed by date of publication.
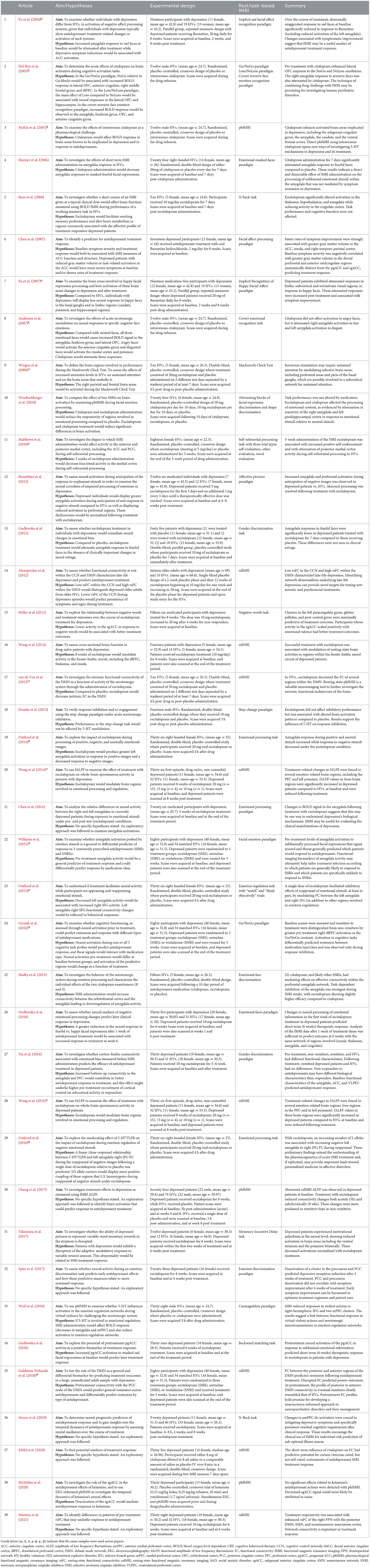
Table 2. Research studies investigating compounds with antidepressant action. Articles are listed by date of publication.
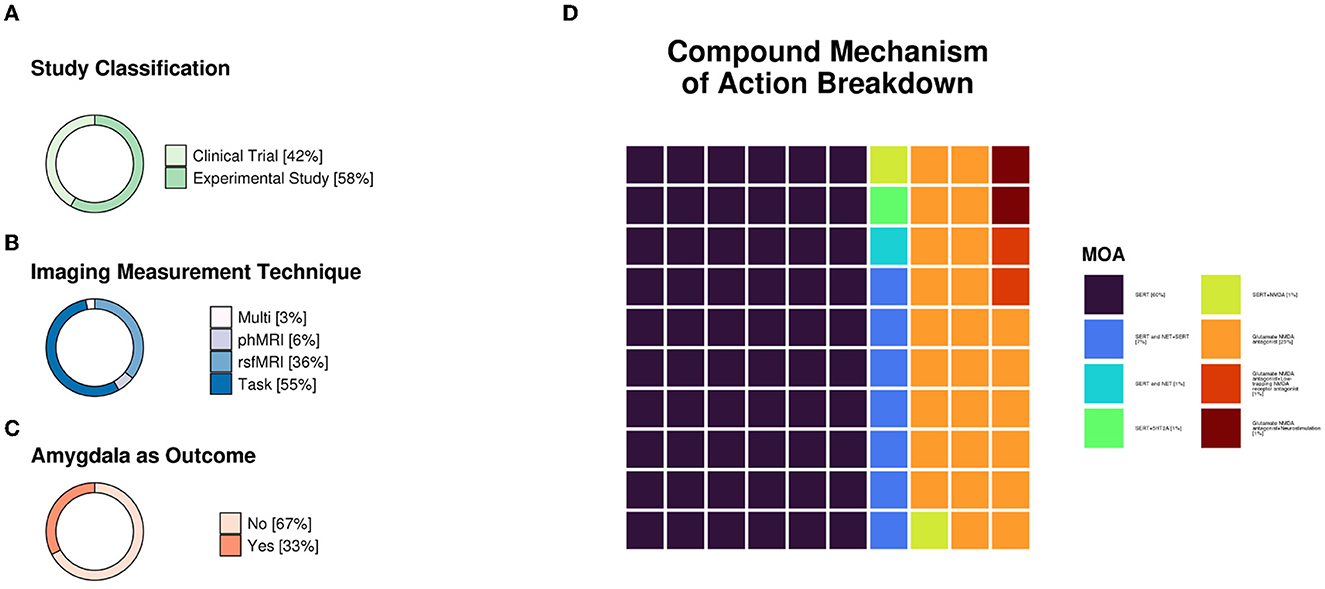
Figure 1. (A) Study classification distributions (n = 28 clinical trials and n = 39 experimental studies). (B) Imaging measurement technique distributions. Pharmacological functional magnetic resonance imaging (phfMRI), n = 4; resting-state functional MRI (rsfMRI), n = 24; task = data collected during stimulus presentation and/or response, n = 37; multi = two or more techniques used in one study (e.g., rsfMRI and task), n = 2. (C) Was the amygdala an outcome reported in the study (n = 22 ‘yes' and n = 45 ‘no'). (D) Compound mechanism of action breakdown. SERT, n = 40; SERT and NET+SERT, n = 5; SERT and NET, n = 1; SERT+H5T2A, n = 1; SERT+NMDA, n = 1; MDA antagonist, n = 17; NMDA antagonist+low-trapping NMDA receptor antagonist, n = 1; NMDA antagonist+neurostimulation, n = 1. Mechanisms of action were primarily referenced from Neuroscience Based Nomenclature (“NbN”; https://nbn2r.com/, https://www.sagepub.com/sites/default/files/nbn_glossary.pdf). “+” indicates that antidepressants with differing mechanisms of action were used in the study, whereas “and” indicates that a given compound has multiple theorized mechanisms of action. If multiple studies were reported in one paper, each study was counted separately. SERT, serotonin transporter; NET, norepinephrine transporter; 5-HT2, 5-hydroxytryptamine 2; NMDA, N-methyl-D-aspartate.
3. Results
The clinicaltrials.gov database identified 378 studies, 14 of which fulfilled our inclusion criteria. The ISRCTN Registry search identified 60 studies, 11 of which fulfilled our inclusion criteria. The 25 studies from the two clinical trial databases are summarized in Table 1. These studies included patients with depression, including bipolar depression and treatment-resistant depression (TRD), as well as healthy volunteers, depending on their aims and study design. When compounds with antidepressant action were entered as search terms, 39 additional experimental research studies that were not registered as clinical trials were identified that were relevant to this review (see Table 2). The ratio of clinical trials to research studies, as well as the imaging techniques used in each study, are presented in Figures 1A, B. The sample size and biological sex balance of all included studies are presented in Figure 2.
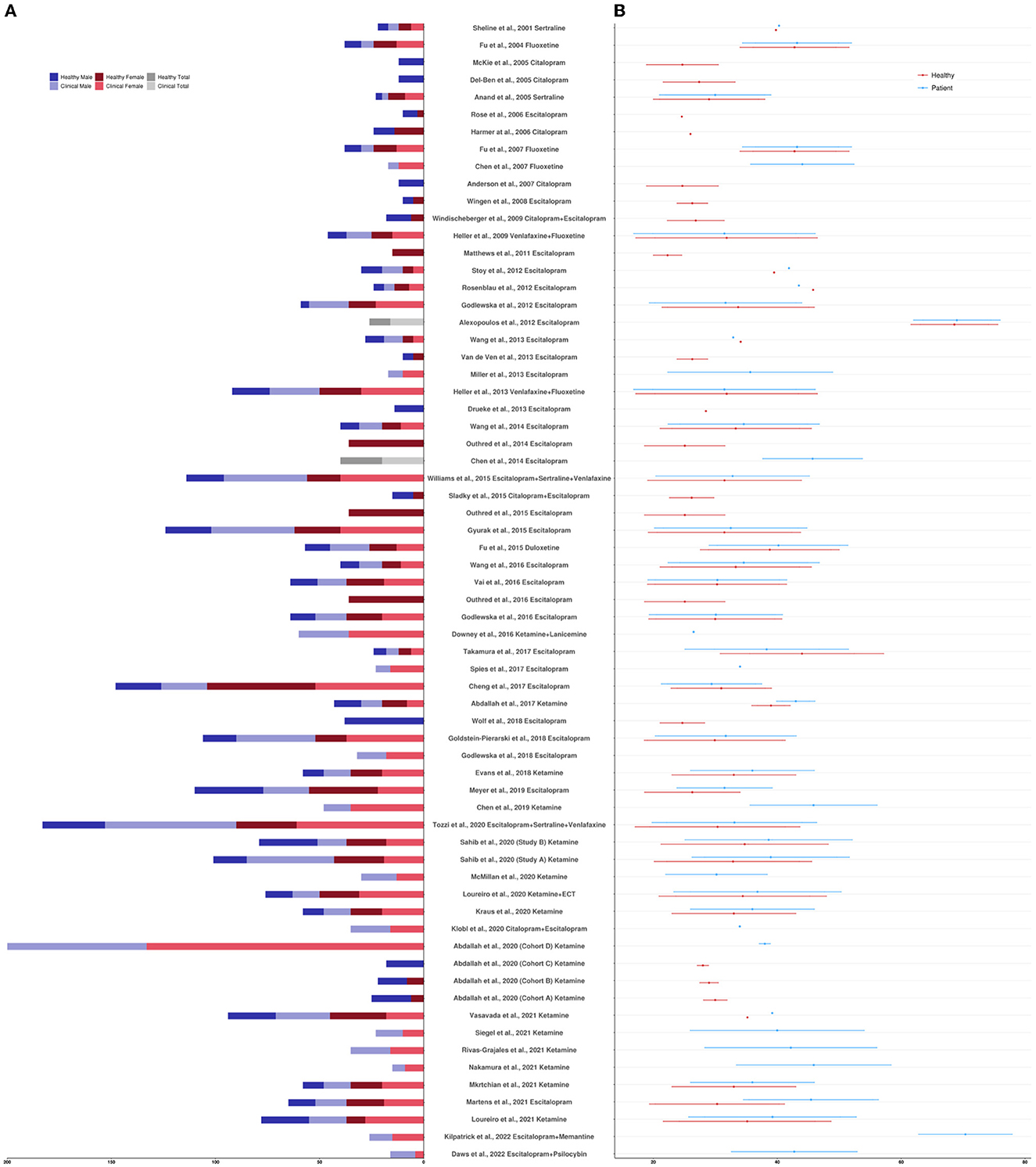
Figure 2. (A) Sample size reported per study by clinical designation and biological sex. (B) Sample age mean and standard deviation reported per study by clinical designation. If sample age was divided by treatment response or treatment arm, values were averaged; if sample age was not reported but an age recruitment range was provided, values were averaged. If multiple studies were reported in one paper, each study was counted separately.
Predictably, most of the included studies investigated SSRIs, which are the most commonly prescribed treatments for depression (Figure 1C). Compounds with a glutamatergic mechanism of action, mainly ketamine, were the second most frequently identified class of medication with antidepressant action. The SSRI studies mainly focused on the mechanism of action of these compounds as treatments for depression and often reported outcomes that involved the amygdala. The amygdala was a common target area for all investigational compounds for depression, with 67% of the clinical trials and research studies included in this review reporting outcomes in this region (Figure 1C).
3.1. SSRIs
3.1.1. The amygdala
Twenty-two of the 48 studies that investigated antidepressants with serotonergic action and reported findings in the amygdala used task-based fMRI involving discrimination of emotional faces. These studies found increased amygdala activation at baseline in response to negative stimuli in individuals with depression vs. healthy volunteers [as an example, see (Godlewska et al., 2012). Administration of SSRIs, mainly sertraline and escitalopram, decreased amygdala activation in response to fearful faces (Sheline et al., 2001; Godlewska et al., 2012, 2016), sad faces (Fu et al., 2004), and negative pictures (Rosenblau et al., 2012), and this decrease positively correlated with reduced depressive symptoms six to eight weeks post-treatment. This trend toward normalization of amygdala activation in response to SSRIs was observed as early as seven days after treatment, when no change in depressive symptoms had yet been observed (Godlewska et al., 2012). Baseline amygdala activation (Williams et al., 2015), as well as amygdala connectivity with the anterior cingulate cortex (ACC) and ventrolateral prefrontal cortex (VLPFC) (Godlewska et al., 2018) also predicted treatment response to specific SSRIs.
In contrast to studies of individuals with depression—which, with few exceptions, have targeted the amygdala—studies of healthy volunteers are much more exploratory in their approach. One pharmacological fMRI (phfMRI) study in healthy volunteers found that activity in the caudate, subgenual and anterior cingulate, hippocampus, amygdala, and frontal cortex increased after a single citalopram infusion compared to placebo (McKie et al., 2005). Other studies that assessed amygdala activity at peak blood concentration levels for various SSRIs found that reduced activation was observed in response to negative stimuli in healthy volunteers (Del-Ben et al., 2005; Anderson et al., 2007; Outhred et al., 2015, 2016). Finally, when healthy volunteers received compounds with a serotonergic mechanism of action for 7-10 days, reduced amygdala and parahippocampal activation was observed in response to emotional stimuli (Harmer et al., 2006; Windischberger et al., 2010).
3.1.2. The anterior cingulate cortex (ACC) and striatal areas
Four studies examined the effects of SSRIs on the activation and connectivity of the ACC in depression. These studies found that baseline activity and connectivity in the ACC in response to emotional and negative stimuli successfully predicted response to treatments for depression, highlighting the potential importance of studying the ACC as a brain area that could predict treatment response (Chen et al., 2007; Miller et al., 2013; Godlewska et al., 2016, 2018).
When activations in the striatum were examined, unmedicated patients with depression demonstrated hypoactivity in the VS during the anticipation of positive and negative monetary rewards (Stoy et al., 2012). This hypoactivation was reversed after 6 weeks of treatment with escitalopram.
3.1.3. The default mode network (DMN) and connectivity between other brain areas
Individuals with depression showed increased DMN connectivity that reduced in response to antidepressant treatment, a decrease that correlated with symptom improvement (Alexopoulos et al., 2012; Goldstein-Piekarski et al., 2018; Kilpatrick et al., 2022). Psilocybin, an agent with serotonergic action, also produced disorganization of the DMN along with other brain networks, a finding that correlated with improved depression outcomes (Daws et al., 2022). Finally, both acute and longer-term (three weeks) treatment with escitalopram reduced DMN connectivity in healthy volunteers, a finding that is in line with the effects that the drug produces in depression (Matthews et al., 2010; van de Ven et al., 2013).
Concerning connectivity between other brain areas and networks, SSRIs increased corticolimbic connectivity in patients with depression, 6 weeks post-treatment (Anand et al., 2005), while frontostriatal connectivity changes induced by antidepressant treatment correlated with positive day-to-day emotion during an emotional processing task (Heller et al., 2013a).
3.2. Antidepressants with glutamatergic action
Studies investigating the role of glutamatergic agents have mainly focused on the effects of the N-methyl-D-aspartate (NMDA) glutamatergic modulator ketamine as an antidepressant treatment.
The amygdala Loureiro et al. (2020) showed that both ketamine and electroconvulsive therapy decreased amygdala reactivity to positive and negative emotional face processing. Additionally, in an emotional judgement task analysis, the researchers found that, at 24 h post-administration, ketamine decreased activation of the amygdala along with the insula, ACC, and ventral tegmental areas (Sterpenich et al., 2019).
3.2.1. The anterior cingulate cortex and striatal areas
Compared to baseline, ketamine enhanced neural response to positive emotion in the right caudate of individuals with depression undergoing an emotional processing task (Murrough et al., 2015). Ketamine also decreased activation in the ACC and ventral tegmental areas during an emotional judgement task (Sterpenich et al., 2019). Finally, in a study by Downey et al., it was shown that ketamine and lanicemine, both increased activation of the sgACC and rostral ACC.
3.2.2. The default mode network (DMN) and connectivity between other brain areas
A single ketamine infusion normalized global brain connectivity in individuals with depression (Abdallah et al., 2017), though this finding was not replicated by Kraus and colleagues (Kraus et al., 2020). Normalization of the connectivity between the insula and the DMN (Evans et al., 2018), the subgenual ACC (sgACC) and the DMN (Nakamura et al., 2021; Siegel et al., 2021), the habenula and occipital and temporal areas (Rivas-Grajales et al., 2021), as well as fronto-striatal connectivity (Mkrtchian et al., 2021) was also observed 24 and 48 h after a single ketamine infusion. When the effects of 4 weeks of ketamine treatment on brain connectivity were examined, decreased functional connectivity between the cerebellum and the salience network was observed in individuals with depression (Vasavada et al., 2021); increased functional connectivity between the cerebellum and the striatum prior to treatment predicted remission (Sahib et al., 2020a). 4 weeks of ketamine treatment (Vasavada et al., 2021) also significantly increased functional connectivity between limbic regions and the central executive network while decreasing connectivity between the left amygdala and the salience network (Siegel et al., 2021).
3.3. Other compounds with antidepressant action
In one study of the serotonin-norepinephrine reuptake inhibitor (SNRI) duloxetine, unmedicated individuals with depression who received 12 weeks of duloxetine showed normalized activation in the amygdala and ACC, a finding that was linked to symptom reduction (Fu et al., 2015). Studies of venlafaxine, another SNRI, found that venlafaxine had no effect on amygdala activation after 2 months (Heller et al., 2013a) and 6 months of treatment (Heller et al., 2013b), and that amygdala activity at baseline did not correlate with symptom changes and negative affect regulation (Heller et al., 2009).
4. Discussion
This review sought to examine the use of fMRI as an outcome measure in clinical trials and research studies that focus on depression. Most of the studies seeking to understand the changes in brain activity and connectivity that accompany successful antidepressant treatment and predict treatment response have, to date, focused on SSRIs and, to a lesser extent, ketamine. Our discussion will focus on the amygdala and on ketamine as two different avenues for assessing what the field has learned from the use of fMRI in clinical trials and research studies in depression.
Almost half of the SSRI studies exploring the effects of serotonergic compounds in depression (20 out of 44 in our review) focused on the amygdala, a brain area involved in the processing of emotionally valenced stimuli. Research in individuals with depression has consistently observed increased activation in the amygdala during the presentation of fearful and negative stimuli (Drevets et al., 2008). Furthermore, successful treatment for depression has tended to normalize this amygdala hyperactivity, often prior to any detectable symptom changes (Godlewska et al., 2012, 2016; Rosenblau et al., 2012; Vai et al., 2016). Decreased amygdala activity was primarily observed after SSRI administration, although it remains unclear whether this effect is specific to SSRIs or whether it also extends to antidepressant treatments with different mechanisms of action (Williams et al., 2015). In addition, the effect of antidepressant treatment on amygdala activation appears to be specific to the type of stimulus that triggers the activation of that brain area. For instance, amygdala activation increased at baseline in response to fearful/sad faces (Godlewska et al., 2016), a finding in line with depression being characterized by increased emotional response to negative affect (Godlewska et al., 2016) and associated with heightened amygdala response.
The fact that chronic and acute SSRI administration produce similar effects on the amygdala of healthy volunteers and individuals with depression (Harmer et al., 2006; Windischberger et al., 2010; Outhred et al., 2015) offers insight into the antidepressant mechanism of action of those compounds. It also underscores both that the amygdala is a key area for antidepressant response and that fMRI is a useful tool for understanding antidepressant drug action. Interestingly, the anxiolytic drug lorazepam also appears to decrease amygdala activation (Paulus et al., 2005), further highlighting the importance of this area as a potential “hub” for drug action in mental health.
Although the studies summarized here consistently observed decreased amygdala activation following treatment with SSRIs, they did not determine whether antidepressant response was specific to the right or left amygdala or appeared bilaterally. If the amygdala is to be used as an fMRI biomarker to determine treatment response, more precise localization of these effects is required. In addition, although most of the amygdala studies to date have focused on emotional processing, they use different fMRI tasks to examine response. While observation of amygdala activation under different experimental conditions strengthens the idea that the amygdala plays a critical role in antidepressant drug action and highlights fMRI as a powerful tool for drug research, consensus on a single paradigm that could be used to determine drug effects in the amygdala could be useful for studies seeking to establish its validity as a marker of drug efficacy. Finally, it is worth highlighting that although the majority of the task-based fMRI studies for SSRIs have focused on emotional processing tasks, there are other tasks including reward processing (for example, Stoy et al., 2012), response inhibition (see Gyurak et al., 2016) and working memory (for review on the n-back task see Nikolin et al., 2021) that have also been used in addition to emotional processing or in isolation in order to examine different brain processes that are affected in depression. These tasks could help in the identification of novel drug targets as well as contribute to the better understanding of the depression-related brain changes. Finally, when incorporated in to clinical trials, they provide a more holistic characterization of the mechanism of action of different antidepressant treatments.
The NMDA receptor antagonist ketamine is the most widely investigated of the glutamatergic antidepressants. Ketamine is a commonly used anesthetic and, at sub-anesthetic doses, a potent and rapid-acting treatment for depression (Berman et al., 2000). It should be noted, however, that although ketamine's antidepressant efficacy is a rather well-replicated finding (McIntyre et al., 2020)—especially in cases of TRD—research around the neuronal pathways that underlie ketamine's actions and the relevant brain areas involved in antidepressant response to ketamine remain unknown. Indeed, ketamine's antidepressant effects on brain activity and connectivity are complex and difficult to decipher compared to serotonergic agents. fMRI studies that investigated ketamine as an antidepressant focused on drug-induced changes in brain activity and connectivity; in particular, these studies found that ketamine normalized the connectivity changes associated with depression (Abdallah et al., 2017; Evans et al., 2018; Mkrtchian et al., 2021). Each study, however, focused on different networks and/or brain areas implicated in the symptoms of depression and, as a result, few findings have yet been replicated across studies. In addition, most of these studies focused on the 24 h post-ketamine administration timepoint, when the drug's antidepressant effects peak; nevertheless, changes in brain activity and connectivity that precede these effects might also be important markers for ketamine's antidepressant actions. Another key point is that, although some studies of multiple ketamine infusions have been conducted (Sahib et al., 2020a; Vasavada et al., 2021), most findings are drawn from analyses conducted on samples collected after a single infusion. Further research and replication in other cohorts is needed to confirm the ketamine fMRI findings.
Task-based fMRI studies that aim to characterize ketamine's antidepressant mechanism of action have mainly focused on emotional processing and reward tasks and identified decreased amygdala activity after ketamine administration (Siegel et al., 2021). This finding is in line with the antidepressant effects observed for SSRIs and SNRIs, further underscoring the potential role of the amygdala as a biomarker for determining antidepressant drug efficacy with fMRI. Increased striatal activity has also been observed with task-based fMRI post-ketamine administration (Sterpenich et al., 2019). Although few such studies exist, their results are in line with literature reporting decreased striatal response in individuals with depression associated with blunted responses to reward and feedback; the findings are also in line with ketamine's positive effects on anhedonia (Wilkowska et al., 2021).
Despite these intriguing early findings, many questions need to be addressed before fMRI can successfully be used to identify brain areas that could determine successful antidepressant response to ketamine. For instance, are the changes in brain activity and connectivity that occur during acute ketamine administration relevant and necessary for determining antidepressant response to ketamine? Furthermore, given that ketamine is mainly used to treat TRD, a better understanding of the brain changes that characterize TRD is necessary. For example, research suggests that individuals with TRD may have a different brain response to ketamine than those with other forms of depression (Sun et al., 2022), suggesting that different brain areas that might serve as biomarkers to determine treatment response in TRD vs. other forms of depression (Carboni et al., 2021).
Apart from the biophysical considerations, fMRI data quality, acquisition parameter selection, trial type and size, may also affect the detection and reliability of the markers identified. Although a detailed review of these choices is outside the scope of the current discussion (see Bodurka et al., 2007; Noble et al., 2017; Raimondo et al., 2021), it is worth pointing out that fMRI data quality can be well-monitored prospectively, during a study, if appropriate quality control measures are in place (for example Reynolds et al., 2022). Technical developments in fMRI have increased the potential to localize small brain structures. The fundamental requirement of adequate temporal signal-to-noise ratio (tSNR) to detect them is dictated by several variables including field strength, spatial and temporal resolution, and scan length (Murphy et al., 2007) and consensus suggestions for the optimal values of these parameters are still not in place. For resting state scans, optimal scan lengths have been studied and it was shown that 9–12 min of scanning is sufficient to reach a plateau for inter-session reliability (Birn et al., 2013) while five, repeated 5 min sessions of resting state scanning, pooled together, is enough to provide “fair” reliability (Noble et al., 2017) with no major site, scanner manufacturer, or day-of-scan effects found. For task based scans, there has been a recent suggestion that increasing the number of event trial sample size has nearly the same impact as subject sample size on statistical efficiency (Chen et al., 2022). From the above, it is safe to conclude that unsurprisingly the underlying data quality and quantity would have a significant impact on the ability to detect and identify markers. In that respect, current trends toward openly sharing raw data could enable researchers to have more confidence in the origin of their results.
Taken together, the fMRI findings reviewed above suggest that successful antidepressant response is reflected in a normalization of the brain activity and connectivity deficits associated with depression. As a result, areas that present with altered brain fMRI activation in depression could be used as potential treatment targets in clinical trials. Such targeted use of fMRI would require that the changes in brain activity and connectivity that underlie depression and depressive symptoms be consistently observed and well-characterized in individuals with depression.
5. Future directions
fMRI research that aims to understand changes in brain activity and connectivity in individuals with depression as well as healthy volunteers is often obscured by our relatively poor understanding of the long-term effects of antidepressant medication on brain activity and connectivity. A meta-analysis of resting-state studies in depression that took antidepressant treatment into account (Javaheripour et al., 2021) suggested that the depressed brain may not be as well-characterized as previously believed. The effects of natural aging on brain activation and connectivity should also be taken into account, especially in studies where there is a broad age range between participants (Kaufmann et al., 2019).
Because of its rapid antidepressant effects, ketamine may be a particularly useful tool for investigating antidepressant action on brain circuits, thus bypassing some of the confounding factors associated with functional imaging research. Its quick action enables researchers to measure a baseline state with significant symptomatology, the acute drug effects associated with ketamine, and the subsequent symptom reduction that occurs within hours. The ability to acquire successive images of an individual's changing state on such a short timescale increases the likelihood that the measured changes are due to mood improvement from the drug rather than other environmental (e.g., hydration) or longitudinal changes (e.g., lifestyle changes, aging) that can confound imaging findings. Studying the antidepressant mechanisms of ketamine could also improve our understanding of antidepressant drug action in general, given that the pathways and brain areas that mediate antidepressant effects—for example the amygdala (Sheline et al., 2001; Godlewska et al., 2012, 2016; Loureiro et al., 2020) and DMN (Matthews et al., 2010; van de Ven et al., 2013; Nakamura et al., 2021; Siegel et al., 2021)—appear to be shared between different antidepressants. Within that framework of shared pathways between drugs and mood disorders, employing an approach such as the one proposed by the Research Domain Criteria Initiative (RDoC) could be particularly useful. The RDoC framework provides an organizational structure for research that is based on key domains of neurobehavioral functioning that are shared across several mental health disorders (Cuthbert and Insel, 2013). Research planned with these criteria in mind, would lead to a common framework for results reporting which could increase the reproducibility of findings.
Concerning the fMRI modalities, task-based or resting-state, that different studies use to examine the effects of antidepressant treatments, resting-state fMRI, seems to be producing consistent findings, especially in relation to the brain connectivity responses induced by ketamine. However, it should be noted that imaging studies in general and particularly those involving resting-state fMRI, present the challenge of disentangling the antidepressant effects of pharmacological treatments from the neurovascular ones (De Simoni et al., 2013). This could be mitigated by measuring peripheral physiological changes and/or using multi-echo sequences or concurrent EEG monitoring (McMillan et al., 2020). Additionally, there are many different approaches that are currently used to analyze resting-state fMRI data, including looking at brain networks and/or individual regions of interest (ROIs). Different pharmacological studies, employ one or more of these approaches, which would best fit with the study design and hypotheses, however, this limits the replicability of the resting-state fMRI findings. A battery of standardized analyses that could be included to all pharmacology studies that aim to exam drug efficacy, applied along with exploratory approaches, could provide with findings that are replicable and help better characterize and understand drug effects.
Task-based fMRI studies use different paradigms to target specific brain areas and to examine drug effects in those regions. However, a large variety of tasks—as well as many variations of the same task—are used to elicit specific responses from participants. This is an excellent way to explore the neural responses that underlie different tasks and different drugs, but a high number of task variations also results in a lack of replicability. A recent review and meta-analysis found that commonly used fMRI tasks do not currently have the test-retest reliability necessary for biomarker discovery or brain-behavior mapping (Elliott et al., 2020). In this context, the field would benefit from developing robust task paradigms that could be implemented in the scanner and parallel the effectiveness of clinically developed rating scales. In addition, traditional tasks have been designed to reduce inter-subject variability for stability in group averages and may thus not reflect changes within an individual with repeated scans. In order to best capture individual dynamics, the use of more ecologically valid tasks has been proposed, including using naturalistic stimuli (Finn et al., 2020).
Another area that could be re-examined in order to enhance the reliability of fMRI measures as well as the quality of fMRI data—and especially task-based fMRI measures—would be a trade-off between the number of participants and the amount of data collected per participant that is needed to make a particular inference (Naselaris et al., 2021). Because of their specialized populations and the additional burden and time required to complete a full trial, clinical studies take a long time to accrue enough participants for imaging trials. As a result, collecting more data from a smaller number of participants might be a promising way to improve the reliability of fMRI (Gordon et al., 2017; Gratton et al., 2018; Elliott et al., 2019), employ better task controls, collect data at many different time points as well as increase the length of data that are collected. Forming complete models from a relatively small number of individuals with dense, high quality fMRI sampling (akin to N-of-1 trials) may provide clinically useful imaging information and generalizable results that may help with research reliability and biomarker development. A smaller study sample could also allow for the better characterization of the study population in terms of symptoms. In clinical populations, comorbidities are a factor that is almost impossible to avoid. Depression for example, is often accompanied by anxiety or trauma-related disorders (Kupfer and Frank, 2003). Although, a mixed sample would add to the ecological validity of the study, it could impact the replicability of the study findings as well as make it difficult to link the brain changes that are observed after the drug administration to a specific disorder. The type of dense sampling that we proposed above, also lends itself favorably to deep phenotyping of individual responses when multiple modalities are acquired—for instance, from genetics, blood, behavioral data, and a variety of imaging modalities [e.g., magnetoencephalography (MEG), MRI, positron emission tomography (PET)] which could lead to more direct links between disorders and drug effects as well as enable the recruitment of more homogenous clinical populations. However, this dense sampling method may be difficult for clinical populations, where the burden that these interventions pose could add to participants' health and mood challenges; implementation of any such methods should thus be carefully considered. Alternatively, or in addition to this approach, large multi-site studies that could assemble large data sets across diverse population (Spellman and Liston, 2020) could also help increase the power and reliability of imaging studies, although they face the challenge of coordinating data collection from multiple sites while ensuring data quality and consistency.
Finally, data sharing between different labs and research centers would also enable the analyses of larger data sets that could increase the reliability of findings as well as help overcome the common problem of small sample sizes that most clinical trials and pharmacology research studies are faced with.
However, several positive steps have already been made in this direction, with studies beginning to employ a standardized and uniform way of organizing imaging data (Gorgolewski et al., 2016; Niso et al., 2018). Such efforts ensure that data are readily available for sharing between different acquisition centers, but also between researchers, making their analysis much easier and more robust and thus more reliable.
6. Conclusions
This review sought to examine the use of fMRI in clinical trials and research studies of compounds that target depression. Much of the work conducted to date has identified the amygdala as a particularly promising target region for antidepressant treatments. Indeed, most studies in this review found a consistent decrease in amygdala activity following antidepressant treatment. This review also highlighted the potential role of ketamine as a tool for studying antidepressant effects with fMRI, given that ketamine administration not only leads to very rapid antidepressant effects but consistently normalizes brain activity and connectivity in depression. These findings highlight the considerable promise of fMRI for studying and developing drugs targeted at mental health conditions in general and depression in particular. Nevertheless, a gap persists between the extensive use of fMRI in research and its systematic and primary use in clinical trials-related research. Efforts to develop more effective fMRI tasks and adapt existing study designs would help harness the advantages of fMRI and improve our ability to translate research findings into clinical trial outcomes.
Author contributions
VK: conceptualization, research, analysis, and writing. JE: conceptualization, analysis, and writing. CP: research, analysis, and writing. CZ: analysis, editing for critical intellectual content, and supervision. All authors contributed to the article and approved the submitted version.
Funding
Funding for this work was provided by the Intramural Research Program at the National Institute of Mental Health, National Institutes of Health (IRP-NIMH-NIH; ZIAMH002857). The work was completed as part of the authors' official duties as Government employees. The views expressed do not necessarily reflect the views of the NIH, the Department of Health and Human Services, or the United States Government.
Acknowledgments
The authors thank the 7SE research unit and staff for their support. Ioline Henter (NIMH) provided invaluable editorial assistance.
Conflict of interest
JE and CZ declared that they were an editorial board member of Frontiers, at the time of submission. This had no impact on the peer review process and the final decision.
The remaining authors declare that the research was conducted in the absence of any commercial or financial relationships that could be construed as a potential conflict of interest.
Publisher's note
All claims expressed in this article are solely those of the authors and do not necessarily represent those of their affiliated organizations, or those of the publisher, the editors and the reviewers. Any product that may be evaluated in this article, or claim that may be made by its manufacturer, is not guaranteed or endorsed by the publisher.
Supplementary material
The Supplementary Material for this article can be found online at: https://www.frontiersin.org/articles/10.3389/fnimg.2023.1110258/full#supplementary-material
References
Abdallah, C. G., Ahn, K. H., Averill, L. A., Nemati, S., Averill, C. L., Fouda, S., et al. (2021). A robust and reproducible connectome fingerprint of ketamine is highly associated with the connectomic signature of antidepressants. Neuropsychopharmacology 46, 478–485. doi: 10.1038/s41386-020-00864-9
Abdallah, C. G., Averill, L. A., Collins, K. A., Geha, P., Schwartz, J., Averill, C., et al. (2017). Ketamine treatment and global brain connectivity in major depression. Neuropsychopharmacology 42, 1210–1219. doi: 10.1038/npp.2016.186
Alexopoulos, G. S., Hoptman, M. J., Kanellopoulos, D., Murphy, C. F., Lim, K. O., Gunning, F. M., et al. (2012). Functional connectivity in the cognitive control network and the default mode network in late-life depression. J. Affect. Disord. 139, 56–65. doi: 10.1016/j.jad.2011.12.002
Anand, A., Li, Y., Wang, Y., Wu, J., Gao, S., Bukhari, L., et al. (2005). Antidepressant effect on connectivity of the mood-regulating circuit: an fMRI study. Neuropsychopharmacology 30, 1334–1344. doi: 10.1038/sj.npp.1300725
Anderson, I. M., Del-Ben, C. M., McKie, S., Richardson, P., Williams, S. R., Elliott, R., et al. (2007). Citalopram modulation of neuronal responses to aversive face emotions: a functional MRI study. NeuroReport 18, 1351–1355. doi: 10.1097/WNR.0b013e3282742115
Berman, R. M., Cappiello, A., Anand, A., Oren, D. A., Heninger, G. R., Charney, D. S., et al. (2000). Antidepressant effects of ketamine in depressed patients. Biol. Psychiatry 47, 351–354. doi: 10.1016/S0006-3223(99)00230-9
Birn, R. M., Molloy, E. K., Patriat, R., Parker, T., Meier, T. B., Kirk, G. R., et al. (2013). The effect of scan length on the reliability of resting-state fMRI connectivity estimates. Neuroimage 83, 550–558. doi: 10.1016/j.neuroimage.2013.05.099
Bodurka, J., Ye, F., Petridou, N., Murphy, K., and Bandettini, P. A. (2007). Mapping the MRI voxel volume in which thermal noise matches physiological noise–implications for fMRI. Neuroimage 34, 542–549. doi: 10.1016/j.neuroimage.2006.09.039
Carboni, E., Carta, A. R., Carboni, E., and Novelli, A. (2021). Repurposing ketamine in depression and related disorders: can this enigmatic drug achieve success? Front. Neurosci. 15, 657714. doi: 10.3389/fnins.2021.657714
Carmichael, O., Schwarz, A. J., Chatham, C. H., Scott, D., Turner, J. A., Upadhyay, J., et al. (2018). The role of fMRI in drug development. Drug Discov. Today 23, 333–348. doi: 10.1016/j.drudis.2017.11.012
Chen, C-. H., Ridler, K., Suckling, J., Williams, S., Fu, C. H. Y., Merlo-Pich, E., et al. (2007). Brain imaging correlates of depressive symptom severity and predictors of symptom improvement after antidepressant treatment. Biol. Psychiatry 62, 407–414. doi: 10.1016/j.biopsych.2006.09.018
Chen, G., Pine, D. S., Brotman, M. A., Smith, A. R., Cox, R. W., Taylor, P. A., et al. (2022). Hyperbolic trade-off: the importance of balancing trial and subject sample sizes in neuroimaging. NeuroImage. 247, 118786. doi: 10.1016/j.neuroimage.2021.118786
Chen, M-. H., Lin, W-. C., Tu, P-. C., Li, C-. T., Bai, Y-. M., Tsai, S-. J., et al. (2019). Antidepressant and antisuicidal effects of ketamine on the functional connectivity of prefrontal cortex-related circuits in treatment-resistant depression: a double-blind, placebo-controlled, randomized, longitudinal resting fMRI study. J. Affect. Disord. 259, 15–20. doi: 10.1016/j.jad.2019.08.022
Chen, Y-. T., Huang, M-. W., Hung, I. C., Lane, H-. Y., and Hou, C-. J. (2014). Right and left amygdalae activation in patients with major depression receiving antidepressant treatment, as revealed by fMRI. Behav. Brain Funct. 10, 36. doi: 10.1186/1744-9081-10-36
Cheng, Y., Xu, J., Arnone, D., Nie, B., Yu, H., Jiang, H., et al. (2017). Resting-state brain alteration after a single dose of SSRI administration predicts 8-week remission of patients with major depressive disorder. Psychol. Medicine. 47, 438–450. doi: 10.1017/S0033291716002440
Cuthbert, B. N., and Insel, T. R. (2013). Toward the future of psychiatric diagnosis: the seven pillars of RDoC. BMC Med. 1, 126. doi: 10.1186/1741-7015-11-126
Daws, R. E., Timmermann, C., Giribaldi, B., Sexton, J. D., Wall, M. B., Erritzoe, D., et al. (2022). Increased global integration in the brain after psilocybin therapy for depression. Nat. Med. 28, 844–851. doi: 10.1038/s41591-022-01744-z
De Simoni, Schwarz, S., O'Daly, A. J., Marquand, O. G., Brittain, A. F., Gonzales, C., et al. (2013). Test-retest reliability of the BOLD pharmacological MRI response to ketamine in healthy volunteers. Neuroimage 64, 75–90. doi: 10.1016/j.neuroimage.2012.09.037
Del-Ben, C. M., Deakin, J. F. W., McKie, S., Delvai, N. A., Williams, S. R., Elliott, R., et al. (2005). The effect of citalopram pretreatment on neuronal responses to neuropsychological tasks in normal volunteers: an fMRI study. Neuropsychopharmacology 30, 1724–1734. doi: 10.1038/sj.npp.1300728
Downey, D., Dutta, A., McKie, S., Dawson, G. R., Dourish, C. T., Craig, K., et al. (2016). Comparing the actions of lanicemine and ketamine in depression: key role of the anterior cingulate. Eur. Neuropsychopharmacol. 26, 994–1003. doi: 10.1016/j.euroneuro.2016.03.006
Drevets, W. C., Savitz, J., and Trimble, M. (2008). The subgenual anterior cingulate cortex in mood disorders. CNS Spectr. 13, 663–681. doi: 10.1017/S1092852900013754
Drueke, B., Schlaegel, S. M. A., Seifert, A., Moeller, O., Gründer, G., Gauggel, S., et al. (2013). The role of 5-HT in response inhibition and re-engagement. Eur Neuropsychopharmacol. 23, 830–841. doi: 10.1016/j.euroneuro.2013.05.005
Elliott, M. L., Knodt, A. R., Cooke, M., Kim, M. J., Melzer, T. R., Keenan, R., et al. (2019). General functional connectivity: shared features of resting-state and task fMRI drive reliable and heritable individual differences in functional brain networks. NeuroImage 189, 516–532. doi: 10.1016/j.neuroimage.2019.01.068
Elliott, M. L., Knodt, A. R., Ireland, D., Morris, M. L., Poulton, R., Ramrakha, S., et al. (2020). What is the test-retest reliability of common task-functional MRI measures? New empirical evidence and a meta-analysis. Psychol. Sci. 31, 792–806. doi: 10.1177/0956797620916786
Evans, J. W., Szczepanik, J. E., Brutsche, N., Park, L. T., Nugent, A. C., Zarate, C. A. Jr., et al. (2018). Default mode connectivity in major depressive disorder measured up to 10 days after ketamine administration. Biol. Psychiatry 84, 582–590. doi: 10.1016/j.biopsych.2018.01.027
Finn, E. S., Glerean, E., Khojandi, A. Y., Nielson, D., Molfese, P. J., Handwerker, D. A., et al. (2020). Idiosynchrony: from shared responses to individual differences during naturalistic neuroimaging. NeuroImage 215, 116828. doi: 10.1016/j.neuroimage.2020.116828
Frazer, A., and Blier, P. (2016). A neuroscience-based nomenclature (NbN) for psychotropic agents. Int. J. Neuropsychopharmacol. 19, pyw066. doi: 10.1093/ijnp/pyw066
Fu, C. H. Y., Costafreda, S. G., Sankar, A., Adams, T. M., Rasenick, M. M., Liu, P., et al. (2015). Multimodal functional and structural neuroimaging investigation of major depressive disorder following treatment with duloxetine. BMC Psychiatry 15, 82. doi: 10.1186/s12888-015-0457-2
Fu, C. H. Y., Williams, S. C. R., Brammer, M. J., Suckling, J., Kim, J., Cleare, A. J., et al. (2007). Neural responses to happy facial expressions in major depression following antidepressant treatment. Am. J. Psychiatry 164, 599–607. doi: 10.1176/ajp.2007.164.4.599
Fu, C. H. Y., Williams, S. C. R., Cleare, A. J., Brammer, M. J., Walsh, N. D., Kim, J., et al. (2004). Attenuation of the neural response to sad faces in major depression by antidepressant treatment: a prospective, event-related functional magnetic resonance imaging study. Arch. Gen. Psychiatry 61, 877–889. doi: 10.1001/archpsyc.61.9.877
Godlewska, B. R., Browning, M., Norbury, R., Cowen, P. J., and Harmer, C. J. (2016). Early changes in emotional processing as a marker of clinical response to SSRI treatment in depression. Transl. Psychiatry 6, e957. doi: 10.1038/tp.2016.130
Godlewska, B. R., Browning, M., Norbury, R., Igoumenou, A., Cowen, P. J., Harmer, C. J., et al. (2018). Predicting treatment response in depression: the role of anterior cingulate cortex. Int. J. Neuropsychopharmacol. 21, 988–996. doi: 10.1093/ijnp/pyy069
Godlewska, B. R., Norbury, R., Selvaraj, S., Cowen, P. J., and Harmer, C. J. (2012). Short-term SSRI treatment normalises amygdala hyperactivity in depressed patients. Psychol. Med. 42, 2609–2617. doi: 10.1017/S0033291712000591
Goldstein-Piekarski, A. N., Staveland, B. R., Ball, T. M., Yesavage, J., Korgaonkar, M. S., Williams, L. M., et al. (2018). Intrinsic functional connectivity predicts remission on antidepressants: a randomized controlled trial to identify clinically applicable imaging biomarkers. Transl. Psychiatry 8, 57. doi: 10.1038/s41398-018-0100-3
Gordon, E. M., Laumann, T. O., Gilmore, A. W., Newbold, D. J., Greene, D. J., Berg, J. J., et al. (2017). Precision functional mapping of individual human brains. Neuron 95, 791–807. doi: 10.1016/j.neuron.2017.07.011
Gorgolewski, K. J., Auer, T., Calhoun, V. D., Craddock, R. C., Das, S., Duff, E. P., et al. (2016). The brain imaging data structure, a format for organizing and describing outputs of neuroimaging experiments. Sci. Data 3, 160044. doi: 10.1038/sdata.2016.44
Gratton, C., Laumann, T. O., Nielsen, A. N., Greene, D. J., Gordon, E. M., Gilmore, A. W., et al. (2018). Functional brain networks are dominated by stable group and individual factors, not cognitive or daily variation. Neuron 98, 439–452. doi: 10.1016/j.neuron.2018.03.035
Gyurak, A., Patenaude, B., Korgaonkar, M. S., Grieve, S. M., Williams, L. M., Etkin, A., et al. (2016). Frontoparietal activation during response inhibition predicts remission to antidepressants in patients with major depression. Biol. Psychiatry 79, 274–281. doi: 10.1016/j.biopsych.2015.02.037
Harmer, C. J., Mackay, C. E., Reid, C. B., Cowen, P. J., and Goodwin, G. M. (2006). Antidepressant drug treatment modifies the neural processing of non-conscious threat cues. Biol. Psychiatry 59, 816–820. doi: 10.1016/j.biopsych.2005.10.015
Heller, A. S., Johnstone, T., Light, S. N., Peterson, M. J., Kolden, G. G., Kalin, N. H., et al. (2013a). Relationships between changes in sustained fronto-striatal connectivity and positive affect in major depression resulting from antidepressant treatment. Am. J. Psychiatry 170, 197–206. doi: 10.1176/appi.ajp.2012.12010014
Heller, A. S., Johnstone, T., Peterson, M. J., Kolden, G. G., Kalin, N. H., Davidson, R. J., et al. (2013b). Increased prefrontal cortex activity during negative emotion regulation as a predictor of depression symptom severity trajectory over 6 months. JAMA Psychiatry 70, 1181–1189. doi: 10.1001/jamapsychiatry.2013.2430
Heller, A. S., Johnstone, T., Shackman, A. J., Light, S. N., Peterson, M. J., Kolden, G. G., et al. (2009). Reduced capacity to sustain positive emotion in major depression reflects diminished maintenance of fronto-striatal brain activation. Proc Natl. Acad. Sci. USA 106, 22445–22450. doi: 10.1073/pnas.0910651106
Javaheripour, N., Li, M., Chand, T., Krug, A., Kircher, T., Dannlowski, U., et al. (2021). Altered resting-state functional connectome in major depressive disorder: a mega-analysis from the PsyMRI consortium. Transl. Psychiatry 11, 511. doi: 10.1038/s41398-021-01619-w
Kaufmann, T., van der Meer, D., Doan, N. T., Schwarz, E., Lund, M. J., Agartz, I., et al. (2019). Common brain disorders are associated with heritable patterns of apparent aging of the brain. Nat. Neurosci. 22, 1617–1623. doi: 10.1038/s41593-019-0471-7
Kilpatrick, L. A., Krause-Sorio, B., Siddarth, P., Narr, K.L., and Lavretsky, H. (2022). Default mode network connectivity and treatment response in geriatric depression. Brain Behav. 12, e2475. doi: 10.1002/brb3.2475
Klöbl, M., Gryglewski, G., Rischka, L., Godbersen, G. M., Unterholzner, J., Reed, M. B., et al. (2020). Predicting antidepressant citalopram treatment response via changes in brain functional connectivity after acute intravenous challenge. Front. Comput. Neurosci. 14, 554186. doi: 10.3389/fncom.2020.554186
Kraus, C., Mkrtchian, A., Kadriu, B., Nugent, A. C., Zarate, C. A. Jr., and Evans, J.W. (2020). Evaluating global brain connectivity as an imaging marker for depression: influence of preprocessing strategies and placebo-controlled ketamine treatment. Neuropsychopharmacology 45, 982–989. doi: 10.1038/s41386-020-0624-0
Krystal, A. D., Pizzagalli, D. A., Smoski, M., Mathew, S. J., Nurnberger, J. Jr., Lisanby, S. H., et al. (2020). A randomized proof-of-mechanism trial applying the ‘fast-fail' approach to evaluating κ-opioid antagonism as a treatment for anhedonia. Nat. Med. 26, 760–768. doi: 10.1038/s41591-020-0806-7
Kupfer, D. J., and Frank, E. (2003). Comorbidity in depression: Comorbidity in depression. Acta Psychiatr. Scand. 108, 57–60. doi: 10.1034/j.1600-0447.108.s418.12.x
Loureiro, J. R. A., Leaver, A., Vasavada, M., Sahib, A. K., Kubicki, A., Joshi, S., et al. (2020). Modulation of amygdala reactivity following rapidly acting interventions for major depression. Hum. Brain Mapp. 41, 1699–1710. doi: 10.1002/hbm.24895
Loureiro, J. R. A., Sahib, A. K., Vasavada, M., Leaver, A., Kubicki, A., Wade, B., et al. (2021). Ketamine's modulation of cerebro-cerebellar circuitry during response inhibition in major depression. NeuroImage: Clin. 32, 102792. doi: 10.1016/j.nicl.2021.102792
Martens, M. A. G., Filippini, N., Harmer, C. J., and Godlewska, B. R. (2021). Resting state functional connectivity patterns as biomarkers of treatment response to escitalopram in patients with major depressive disorder. Psychopharmacology 239, 3447–3460. doi: 10.1007/s00213-021-05915-7
Matthews, S. C., Simmons, A. N., Strigo, I. A., Arce, E., Stein, M. B., Paulus, M. P., et al. (2010). Escitalopram attenuates posterior cingulate activity during self-evaluation in healthy volunteers. Psychiatry Res. 182, 81–87. doi: 10.1016/j.pscychresns.2010.02.003
McIntyre, R. S., Carvalho, I. P., Lui, L. M. W., Majeed, A., Masand, P. S., Gill, H., et al. (2020). The effect of intravenous, intranasal, and oral ketamine in mood disorders: a meta-analysis. J. Affect. Disord. 276, 576–584. doi: 10.1016/j.jad.2020.06.050
McKie, S., Del-Ben, C., Elliott, R., Williams, S., del Vai, N., Anderson, I., et al. (2005). Neuronal effects of acute citalopram detected by pharmacoMRI. Psychopharmacology 180, 680–686. doi: 10.1007/s00213-005-2270-y
McMillan, R., Sumner, R., Forsyth, A., Campbell, D., Malpas, G., Maxwell, E., et al. (2020). Simultaneous EEG/fMRI recorded during ketamine infusion in patients with major depressive disorder. Prog. Neuropsychopharmacol. Biol. Psychiatry 99, 109838. doi: 10.1016/j.pnpbp.2019.109838
Meyer, B. M., Rabl, U., Huemer, J., Bartova, L., Kalcher, K., Provenzano, J., et al. (2019). Prefrontal networks dynamically related to recovery from major depressive disorder: a longitudinal pharmacological fMRI study. Transl. Psychiatry 9, 64. doi: 10.1038/s41398-019-0395-8
Miller, J. M., Schneck, N., Siegle, G. J., Chen, Y., Ogden, R. T., Kikuchi, T., et al. (2013). fMRI response to negative words and SSRI treatment outcome in major depressive disorder: a preliminary study. Psychiatry Res 214, 296–305. doi: 10.1016/j.pscychresns.2013.08.001
Mkrtchian, A., Evans, J. W., Kraus, C., Yuan, P., Kadriu, B., Nugent, A. C., et al. (2021). Ketamine modulates fronto-striatal circuitry in depressed and healthy individuals. Mol. Psychiatry 26, 3292–3301. doi: 10.1038/s41380-020-00878-1
Murphy, K., Bodurka, J., and Bandettini, P. A. (2007). How long to scan? The relationship between fMRI temporal signal to noise ratio and necessary scan duration. Neuroimage 34, 565–574. doi: 10.1016/j.neuroimage.2006.09.032
Murrough, J. W., Collins, K. A., Fields, J., DeWilde, K. E., Phillips, M. L., Mathew, S. J., et al. (2015). Regulation of neural responses to emotion perception by ketamine in individuals with treatment-resistant major depressive disorder. Transl. Psychiatry 5, e509–e509. doi: 10.1038/tp.2015.10
Nakamura, T., Tomita, M., Horikawa, N., Ishibashi, M., Uematsu, K., Hiraki, T., et al. (2021). Functional connectivity between the amygdala and subgenual cingulate gyrus predicts the antidepressant effects of ketamine in patients with treatment-resistant depression. Neuropsychopharmacol. Rep. 41, 168–178. doi: 10.1002/npr2.12165
Naselaris, T., Allen, E., and Kay, K. (2021). Extensive sampling for complete models of individual brains. Curr. Opin. Behav. Sci. 40, 45–51. doi: 10.1016/j.cobeha.2020.12.008
Nikolin, S., Tan, Y. Y., Schwaab, A., Moffa, A., Loo, C. K., and Martin, D. (2021). An investigation of working memory deficits in depression using the n-back task: A systematic review and meta-analysis. J Affect Disord. 284, 1–8. doi: 10.1016/j.jad.2021.01.084
Niso, G., Gorgolewski, K. J., Bock, E., Brooks, T. L., Flandin, G., Gramfort, A., et al. (2018). MEG-BIDS, the brain imaging data structure extended to magnetoencephalography. Sci. Data 5, 180110. doi: 10.1038/sdata.2018.110
Noble, S., Spann, M. N.„ Tokoglu, F., Shen, X., Constable, R. T., and Dustin, S. (2017). Influences on the test-retest reliability of functional connectivity MRI and its relationship with behavioral utility. Cereb. Cortex 27, 5415–5429. doi: 10.1093/cercor/bhx230
Outhred, T., Das, P., Dobson-Stone, C., Felmingham, K. L., Bryant, R. A., Nathan, P. J., et al. (2016). Impact of 5-HTTLPR on SSRI serotonin transporter blockade during emotion regulation: a preliminary fMRI study. J. Affect. Disord. 196, 11–19. doi: 10.1016/j.jad.2016.02.019
Outhred, T., Das, P., Felmingham, K., Bryant, R., Nathan, P., Malhi, G., et al. (2014). Impact of acute administration of escitalopram on the processing of emotional and neutral images: a randomized crossover fMRI study of healthy women. J. Psychiatry Neurosci. 39, 267–275. doi: 10.1503/jpn.130118
Outhred, T., Das, P., Felmingham, K. L., Bryant, R. A., Nathan, P. J., Malhi, G. S., et al. (2015). Facilitation of emotion regulation with a single dose of escitalopram: a randomized fMRI study. Psychiatry Res. 233, 451–457. doi: 10.1016/j.pscychresns.2015.07.018
Paulus, M. P., Feinstein, J. S., Castillo, G., Simmons, A. N., and Stein, M. B. (2005). Dose-dependent decrease of activation in bilateral amygdala and insula by lorazepam during emotion processing. Arch. Gen. Psychiatry 62, 282–288. doi: 10.1001/archpsyc.62.3.282
Raimondo, L., Knapen, T., Oliveira, Ĺ. A. F., Yu, X., Dumoulin, S. O., van der Zwaag, W., et al. (2021). A line through the brain: implementation of human line-scanning at 7T for ultra-high spatiotemporal resolution fMRI. J. Cereb. Blood Flow. Metab. 41, 2831–2843. doi: 10.1177/0271678X211037266
Reynolds, R. C., Taylor, P. A., and Glen, D. R. (2022). Quality control practices in FMRI analysis: Philosophy, methods and examples using AFNI. Front Neurosci. 16, 1073800. doi: 10.3389/fnins.2022.1073800
Rivas-Grajales, A. M., Salas, R., Robinson, M. E., Qi, K., Murrough, J. W., Mathew, S. J., et al. (2021). Habenula connectivity and intravenous ketamine in treatment-resistant depression. Int. J. Neuropsychopharmacol. 24, 383–391. doi: 10.1093/ijnp/pyaa089
Rose, E. J., Simonotto, E., Spencer, E. P., and Ebmeier, K. P. (2006). The effects of escitalopram on working memory and brain activity in healthy adults during performance of the n-back task. Psychopharmacology 185, 339–347. doi: 10.1007/s00213-006-0334-2
Rosenblau, G., Sterzer, P., Stoy, M., Park, S., Friedel, E., Heinz, A., et al. (2012). Functional neuroanatomy of emotion processing in major depressive disorder is altered after successful antidepressant therapy. J. Psychopharmacol. 26, 1424–1433. doi: 10.1177/0269881112450779
Sadraee, A., Paulus, M., and Ekhtiari, H. (2021). fMRI as an outcome measure in clinical trials: a systematic review in clinicaltrials.gov. Brain Behav. 11, e02089. doi: 10.1002/brb3.2089
Sahib, A. K., Loureiro, J. R., Vasavada, M., Anderson, C., Kubicki, A., Wade, B., et al. (2020a). Modulation of the functional connectome in major depressive disorder by ketamine therapy. Psychol. Med. 3, 560. doi: 10.1017/S0033291720004560
Sahib, A. K., Loureiro, J. R. A., Vasavada, M. M., Kubicki, A., Wade, B., Joshi, S. H., et al. (2020b). Modulation of inhibitory control networks relate to clinical response following ketamine therapy in major depression. Transl. Psychiatry 10, 260. doi: 10.1038/s41398-020-00947-7
Sheline, Y. I., Barch, D. M., Donnelly, J. M., Ollinger, J. M., Snyder, A. Z., Mintun, M. A., et al. (2001). Increased amygdala response to masked emotional faces in depressed subjects resolves with antidepressant treatment: an fMRI study. Biol. Psychiatry 50, 651–658. doi: 10.1016/S0006-3223(01)01263-X
Siegel, J. S., Palanca, B. J. A., Ances, B. M., Kharasch, E. D., Schweiger, J. A., Yingling, M. D., et al. (2021). Prolonged ketamine infusion modulates limbic connectivity and induces sustained remission of treatment-resistant depression. Psychopharmacology 238, 1157–1169. doi: 10.1007/s00213-021-05762-6
Sladky, R., Spies, M., Hoffmann, A., Kranz, G., Hummer, A., Gryglewski, G., et al. (2015). (S)-citalopram influences amygdala modulation in healthy subjects: a randomized placebo-controlled double-blind fMRI study using dynamic causal modeling. NeuroImage 108, 243–250. doi: 10.1016/j.neuroimage.2014.12.044
Spellman, T., and Liston, C. (2020). Toward circuit mechanisms of pathophysiology in depression. Am. J. Psychiatry 177, 381–390. doi: 10.1176/appi.ajp.2020.20030280
Spies, M., Kraus, C., Geissberger, N., Auer, B., Klöbl, M., Tik, M., et al. (2017). Default mode network deactivation during emotion processing predicts early antidepressant response. Transl. Psychiatry 7, e1008–e1008. doi: 10.1038/tp.2016.265
Sterpenich, V., Vidal, S., Hofmeister, J., Michalopoulos, G., Bancila, V., Warrot, D., et al. (2019). Increased reactivity of the mesolimbic reward system after ketamine injection in patients with treatment-resistant major depressive disorder. Anesthesiology 130, 923–935. doi: 10.1097/ALN.0000000000002667
Stoy, M., Schlagenhauf, F., Sterzer, P., Bermpohl, F., Hägele, C., Suchotzki, K., et al. (2012). Hyporeactivity of ventral striatum toward incentive stimuli in unmedicated depressed patients normalizes after treatment with escitalopram. J. Psychopharmacol. 26, 677–688. doi: 10.1177/0269881111416686
Sun, J., Ma, Y., Chen, L., Wang, Z., Guo, C., Luo, Y., et al. (2022). Altered brain function in treatment-resistant and non-treatment-resistant depression patients: a resting-state functional magnetic resonance imaging study. Front. Psychiatry 13, 904139. doi: 10.3389/fpsyt.2022.904139
Takamura, M., Okamoto, Y., Okada, G., Toki, S., Yamamoto, T., Ichikawa, N., et al. (2017). Patients with major depressive disorder exhibit reduced reward size coding in the striatum. Prog Neuropsychopharmacol. Biol. Psychiatry 79, 317–323. doi: 10.1016/j.pnpbp.2017.07.006
Tozzi, L., Goldstein-Piekarski, A. N., Korgaonkar, M. S., and Williams, L. M. (2020). Connectivity of the cognitive control network during response inhibition as a predictive and response biomarker in major depression: evidence from a randomized clinical trial. Biol. Psychiatry 87, 462–472. doi: 10.1016/j.biopsych.2019.08.005
Tracey, I., and Wise, R. G. (2001). Pharmacological fMRI: a new tool for drug development in humans. J. Pharm. Pract. 14, 368–375. doi: 10.1106/QACM-FBX4-90UK-FRW6
Vai, B., Bulgarelli, C., Godlewska, B. R., Cowen, P. J., Benedetti, F., Harmer, C. J., et al. (2016). Fronto-limbic effective connectivity as possible predictor of antidepressant response to SSRI administration. Eur. Neuropsychopharmacol. 26, 2000–2010. doi: 10.1016/j.euroneuro.2016.09.640
van de Ven, V., Wingen, M., Kuypers, K. P. C., Ramaekers, J. G., and Formisano, E. (2013). Escitalopram decreases cross-regional functional connectivity within the default-mode network. PLoS ONE 8, e68355. doi: 10.1371/journal.pone.0068355
Vasavada, M. M., Loureiro, J., Kubicki, A., Sahib, A., Wade, B., Hellemann, G., et al. (2021). Effects of serial ketamine infusions on corticolimbic functional connectivity in major depression. Biol. Psychiatry Cogn. Neurosci. Neuroimag. 6, 735–744. doi: 10.1016/j.bpsc.2020.06.015
Wang, J. J., Chen, X., Sah, S. K., Zeng, C., Li, Y. M., Li, N., et al. (2016). Amplitude of low-frequency fluctuation (ALFF) and fractional ALFF in migraine patients: a resting-state functional MRI study. Clin. Radiol. 71, 558–564. doi: 10.1016/j.crad.2016.03.004
Wang, L., Li, K., Zhang, Q., Zeng, Y., Dai, W., Su, Y., et al. (2014). Short-term effects of escitalopram on regional brain function in first-episode drug-naive patients with major depressive disorder assessed by resting-state functional magnetic resonance imaging. Psychol. Med. 44, 1417–1426. doi: 10.1017/S0033291713002031
Wilkowska, A., Wiglusz, M. S., Gałuszko-Wegielnik, M., Włodarczyk, A., and Cubała, W. J. (2021). Antianhedonic effect of repeated ketamine infusions in patients with treatment resistant depression. Front. Psychiatry 12, 704330. doi: 10.3389/fpsyt.2021.704330
Williams, L. M., Korgaonkar, M. S., Song, Y. C., Paton, R., Eagles, S., Goldstein-Piekarski, A., et al. (2015). Amygdala reactivity to emotional faces in the prediction of general and medication-specific responses to antidepressant treatment in the randomized iSPOT-D trial. Neuropsychopharmacology 40, 2398–2408. doi: 10.1038/npp.2015.89
Windischberger, C., Lanzenberger, R., Holik, A., Spindelegger, C., Stein, P., Moser, U., et al. (2010). Area-specific modulation of neural activation comparing escitalopram and citalopram revealed by pharmaco-fMRI: a randomized cross-over study. NeuroImage 49, 1161–1170. doi: 10.1016/j.neuroimage.2009.10.013
Wingen, M., Kuypers, K. P. C., van de Ven, V., Formisano, E., and Ramaekers, J. G. (2008). Sustained attention and serotonin: a pharmaco-fMRI study. Hum. Psychopharmacol. 23, 221–230. doi: 10.1002/hup.923
Wise, R. G., and Tracey, I. (2006). The role of fMRI in drug discovery. J. Magn. Reson. Imaging 23, 862–876. doi: 10.1002/jmri.20584
Keywords: functional magnetic resonance imaging (fMRI), depression, clinical trial, selective serotonin reuptake inhibitor (SSRI), ketamine, amygdala
Citation: Kotoula V, Evans JW, Punturieri CE and Zarate CA Jr (2023) Review: The use of functional magnetic resonance imaging (fMRI) in clinical trials and experimental research studies for depression. Front. Neuroimaging 2:1110258. doi: 10.3389/fnimg.2023.1110258
Received: 28 November 2022; Accepted: 12 June 2023;
Published: 27 June 2023.
Edited by:
Xiao Liu, The Pennsylvania State University (PSU), United StatesReviewed by:
Katherine L. Narr, University of California, Los Angeles, United StatesSara Costi, University of Oxford, United Kingdom
Copyright © 2023 Kotoula, Evans, Punturieri and Zarate. This is an open-access article distributed under the terms of the Creative Commons Attribution License (CC BY). The use, distribution or reproduction in other forums is permitted, provided the original author(s) and the copyright owner(s) are credited and that the original publication in this journal is cited, in accordance with accepted academic practice. No use, distribution or reproduction is permitted which does not comply with these terms.
*Correspondence: Vasileia Kotoula, dmFzaWxlaWEua290b3VsYUBuaWguZ292
†These authors have contributed equally to this work