- 1The Mind Research Network, Albuquerque, NM, United States
- 2Department of Psychology, University of New Mexico, Albuquerque, NM, United States
- 3Department of Electrical and Computer Engineering, Georgia Institute of Technology, Atlanta, GA, United States
- 4Tri-Institutional Center for Translational Research in Neuroimaging and Data Science (TReNDS), Georgia Institute of Technology, Georgia State University, Emory University, Atlanta, GA, United States
- 5Department of Computer Science, Georgia State University, Atlanta, GA, United States
Previous work in incarcerated men suggests that individuals scoring high on psychopathy exhibit aberrant resting-state paralimbic functional network connectivity (FNC). However, it is unclear whether similar results extend to women scoring high on psychopathy. This study examined whether psychopathic traits [assessed via the Hare Psychopathy Checklist – Revised (PCL-R)] were associated with aberrant inter-network connectivity, intra-network connectivity (i.e., functional coherence within a network), and amplitude of fluctuations across limbic and surrounding paralimbic regions among incarcerated women (n = 297). Resting-state networks were identified by applying group Independent Component Analysis to resting-state fMRI scans. We tested the association of psychopathic traits (PCL-R Factor 1 measuring interpersonal/affective psychopathic traits and PCL-R Factor 2 assessing lifestyle/antisocial psychopathic traits) to the three FNC measures. PCL-R Factor 1 scores were associated with increased low-frequency fluctuations in executive control and attentional networks, decreased high-frequency fluctuations in executive control and visual networks, and decreased intra-network FNC in default mode network. PCL-R Factor 2 scores were associated with decreased high-frequency fluctuations and default mode networks, and both increased and decreased intra-network functional connectivity in visual networks. Similar to previous analyses in incarcerated men, our results suggest that psychopathic traits among incarcerated women are associated with aberrant intra-network amplitude fluctuations and connectivity across multiple networks including limbic and surrounding paralimbic regions.
Introduction
Individuals scoring high on psychopathy are characterized by a constellation of traits including impulsivity, poor decision making, callousness, and a lack of empathy (Hare, 2003). An individual with psychopathy is 20–25 times more likely to be arrested than a non-psychopathic individual, and once released, is four to eight times as likely to recidivate violently 1-year post-release (Hemphill et al., 1998; Kiehl and Hoffman, 2011). Relatedly, the social costs due to elevated criminal activity that can be attributed to individuals with psychopathy are estimated to be nearly $460 billion per year (Anderson, 1999; Kiehl and Hoffman, 2011). As such, great efforts have been made to understand the sociological, psychological, and neurobiological origins of the psychopathic phenotype.
Most theories suggest that individuals scoring high on psychopathy exhibit deficits in limbic (e.g., amygdala, cingulate gyrus, and parahippocampal gyrus) and surrounding paralimbic brain regions (e.g., orbitofrontal cortex, insula, and temporal pole) (Kiehl, 2006; Anderson and Kiehl, 2012; though see Blair, 2006). Resting-state functional analyses suggest broadly distributed psychopathy-related aberrations in inter-network functional network connectivity (FNC). These aberrances span across multiple networks but primarily occur in networks associated with executive control, decision making, salience detection, and motor control (Tang et al., 2013; Contreras-Rodríguez et al., 2015; Del Casale et al., 2015; Philippi et al., 2015; Leutgeb et al., 2016; Korponay et al., 2017; Espinoza et al., 2018; Dotterer et al., 2020).
Psychometric analyses generally support dividing psychopathic traits, assessed via the Hare Psychopathy Checklist – Revised (PCL-R; Hare, 2003), into two clusters or factors (Harpur et al., 1989; Hare and Neumann, 2010). Factor 1 contains items related to interpersonal and affective traits, while Factor 2 assesses impulsive, life-course developmental, and antisocial traits. Several studies have found interpersonal and affective traits to be associated with localized disruption between the DMN and central executive network (CEN) (Espinoza et al., 2018; Dotterer et al., 2020). Lifestyle/behavioral and antisocial/developmental psychopathic traits, on the other hand, are associated with resting-state correlates ranging from subcortical structures to sensorimotor networks (SEN), DMNs, and visual networks (VIS) (Korponay et al., 2017). Overall, these findings suggest that specific psychopathic traits may associate differentially with resting-state measures.
The bulk of these previous studies have been conducted on entirely male samples, leaving open the question of sex-specific differences in the neurobiological correlates of psychopathy (Verona and Vitale, 2018). Women scoring high on psychopathy are characterized by similar neurobiological deficits as men scoring high on psychopathy (Carré et al., 2013; Cope et al., 2014; Harenski et al., 2014a; Crooks et al., 2019; Maurer et al., 2022), but unique gender differences have also been observed. For example, while men scoring high on psychopathy are characterized by response perseveration deficits, women scoring high on psychopathy are not (Vitale and Newman, 2001b). As such, women scoring high on psychopathy may be characterized by unique FNC patterns compared to men scoring high on psychopathy. Despite advances in our understanding of the relationship between psychopathy and inter-network connectivity of RSNs, research including analyses of amplitude of fluctuations (AFs)—that is, the spectral power of RSN activational profiles—and intra-network connectivity in their relationship to antisocial traits are scant. Furthermore, these studies are largely group comparison based rather dimensionality based (Liu et al., 2014; Xu et al., 2014; Cao et al., 2018). Intra-network high-frequency AFs are believed to contribute to higher-order cognitive processes, and thus, may also differ dimensionally with psychopathic traits (Baria et al., 2011; Craig et al., 2018). These limitations of scope and study obscure functional aberrances associated with psychopathy that may occur on a local RSN specific level rather than an inter-RSN level, as well as potential dimensional correlates associated with psychopathic traits of interest that may be otherwise lost via traditional group comparisons.
Here we examine resting-state measures and their relationships to psychopathic traits (assessed via the PCL-R) (Hare, 2003) in a large sample of incarcerated women (n = 297). Functional connectivity was assessed using three different measures [static functional network connectivity (sFNC: inter-network connectivity), AFs, and intra-network connectivity], to comprehensively evaluate the functional characteristics of RSNs and their associations with psychopathic traits in women. We hypothesized that the majority of aberrant functional connectivity measures related to psychopathy would occur in limbic and paralimbic regions of the brain (Kiehl, 2006).
Methods
Participants
Participants included 308 adult female offenders recruited from a medium- and maximum-security correctional facility who previously participated as part of NIH-funded research and treatment studies (R01 DA020870, R01 DA026964, and R01 MH085010). While all offenders within the correctional facility were offered the opportunity to participate in the current study, the final sample included participants who completed the relevant clinical assessments and resting-state functional MRI scans and met further inclusion criteria. Inclusion criteria included fluency in English at or above a fourth-grade reading level; estimated IQ over 70 (n = 4 excluded); and no presence of psychotic disorder (schizoaffective disorder, n = 1; delusional disorder, n = 1, excluded). An additional n = 5 participants were excluded for MRI-related reasons, excessive head motion (i.e., mean framewise displacement values > three standard deviations above the mean or comprising more than 10% of their total volume, n = 3; Power et al., 2014), large susceptibility artifacts (n = 1), or an incomplete resting-state scan (n = 1). In total, 11 (3.57%) participants were excluded from the study, leaving a final sample of 297 adult female offenders.
Participants were between the ages of 21 and 57 (average age = 34.6 years, SD = 7.5 years) at the time of their scan and ~10% were left-handed. Based on NIH racial and ethnic classification, 78.5% of the sample self-identified as White, 9.1% as Black/African American, 9.1% as American Indian or Alaskan Native, 3.4% as mixed/other, and 56.2% as Hispanic. Participants' demographics and PCL-R scores are shown in Table 1. Participants provided written informed consent in protocols approved by the institutional review board of the University of New Mexico and by the Independent Review (E&I) Services for the Mind Research Network and were paid at a rate commensurate with institution compensation for work assignments at their facility.
Psychopathy scores
Psychopathic traits were assessed using the PCL-R (Hare, 2003), which has been validated for use among women (Vitale and Newman, 2001a; Vitale et al., 2002). The PCL-R consists of 20 items which are scored on a three-point scale, 0 (does not apply), 1 (applies somewhat), and 2 (definitely applies). It is based on participants' clinical interview and extensive file review conducted by trained research staff. The resulting PCL-R total scores range from 0 to 40. Factor analyses of the 20 PCL-R items have consistently revealed two factors: Factor 1 scores correspond to affective/interpersonal characteristics (e.g., manipulativeness, deficient empathy, and a lack of remorse), whereas Factor 2 scores, correspond to impulsive and irresponsible behavior and early and persistent antisocial behavior (Harpur et al., 1989; Hare and Neumann, 2010). While the two-factor model of psychopathy was originally developed and validated in men (Harpur et al., 1989; Hare and Neumann, 2010), research suggests similar validity in women (Kennealy et al., 2007), including participants included in the current sample (Eisenbarth et al., 2018).
Additional psychosocial data
Mood, anxiety, post-traumatic stress, and substance use disorders
Participants were assessed for past or current presence of a mood disorder, including major depressive disorder, dysthymic disorder or persistent depressive disorder, depressive disorder not otherwise specified (NOS), bipolar disorder, mood disorder due to a general medical condition (GMC), and substance-induced mood disorder using the Structured Clinical Interview for DSM-IV-TR Axis I Disorders (SCID-I/P; First et al., 2002) or Structured Clinical Interview for DSM-5–Research Version (SCID-5-RV; First et al., 2015)1. Likewise, participants were assessed for lifetime presence of an anxiety disorder (i.e., panic disorder, agoraphobia, social phobia or social anxiety disorder, specific phobia, generalized anxiety disorder, anxiety disorder NOS or otherwise specified, anxiety disorder due to a GMC, and substance-induced anxiety disorder). Finally, participants were also assessed for lifetime posttraumatic stress disorder (PTSD). PTSD assessment procedures differed across SCID versions, and when the SCID-I/P was used, participants completed an initial screening form to determine whether the PTSD interview module would be administered in full. When the SCID-5-RV version was used the PTSD interview module was administered to all participants. Presence of any mood, anxiety, or traumatic disorder was coded dichotomously, and participants meeting past or current diagnostic criteria for any one of these disorders were coded as having the respective disorder (see Table 1).
Alcohol and/or substance-related diagnoses were assigned based on diagnostic criteria for the SCID version administered (either the SCID-I/P or the SCID-5-RV). Participants administered the SCID-I/P were assessed for lifetime alcohol and drug abuse or dependence. A lifetime diagnosis of abuse was defined as scoring at threshold on at least one of four abuse criteria for alcohol and/or seven drug categories (i.e., sedatives-hypnotics-anxiolytics, cannabis, stimulants, opioids, cocaine, hallucinogens/PCP, and other). A lifetime diagnosis of dependence was defined as scoring at threshold in at least three of seven dependence criteria. Alternatively, for participants administered the SCID-5-RV, a lifetime alcohol use disorder was obtained if at least two of 11 alcohol criteria were scored at threshold, and lifetime substance use disorder(s) were obtained if at least two of 11 criteria were scored at threshold for eight categories (i.e., sedative-hypnotics-anxiolytics, cannabis, stimulants, opioids, inhalants, PCP, hallucinogens, and other/unknown; see Table 1).
Imaging parameters
Resting-state functional magnetic resonance images were collected on the grounds of the correctional facility where participants were housed using the Mind Research Network's mobile Siemens 1.5 T Avanto with advanced SQ gradients (max slew rate 200 T/m/s, 346 T/m/s vector summation, rise time 200 us) equipped with a 12-element head coil. The EPI gradient echo pulse sequence (TR = 2,000 ms, TE = 39 ms, flip angle = 75, FOV = 24 x 24 cm, 64 x 64 matrix, 3.75 x 3.75 mm in-plane resolution, 4 mm slice thickness, 1 mm gap, 27 slices) effectively covered the entire brain (150 mm) in 2.0 s. Head motion was minimized using padding and restraint. The participants were asked to lay still, look at the fixation cross and keep eyes open during the 5-min rsfMRI scanning. Compliance with instructions was monitored by eye tracking.
EPI preprocessing
Data were preprocessed using statistical parametric mapping (SPM12) (Friston et al., 1994) (http://www.fil.ion.ucl.ac.uk/spm) including image reorientation, realignment [motion estimation using INRialign (Freire and Mangin, 2001)], and spatial normalization to the Montreal Neurological Institute standard space at a resolution of a 3 x 3 x 3 mm3. A full width half maximum Gaussian kernel of 6 mm was then used for spatial smoothing. Framewise displacement (FWD) was used to assess motion quality control. For FWD, the translation and rotation parameters were computed as the mean of the sums of the absolute translation and rotation frame displacements, yielding a single FWD value for each participant.
Independent component analysis
Per Espinoza et al. (2018), we applied gICA on the preprocessed rsfMRI data using the GIFT toolbox (http://trendscenter.org/software/gift) (Calhoun et al., 2001). The rsfMRI data was compressed using two stages of principal component analysis (PCA) (Rachakonda et al., 2016). For the first data reduction step, we retained 100 principal components (PCs), and 75 independent components (ICs) for group data reduction, consistent with previously published studies (Kiviniemi et al., 2009; Smith et al., 2009; Ystad et al., 2010; Allen et al., 2011a; Elseoud et al., 2011; Erhardt et al., 2011). High-model order ICA (i.e., 75 components) results in more refined components corresponding to known anatomical and functional segmentations compared to low-model order ICA (i.e., 25 or 50 components) (Allen et al., 2011a; Hu et al., 2020). Individual specific spatial maps and their time-courses were obtained using gICA. Out of the 75 ICs that were estimated, 48 components were identified as components of RSNs by evaluating whether peak activation occurred in gray matter and whether the peak AFs occurred in the low-frequency power portion of the spectra of components (Meda et al., 2008; Robinson et al., 2009; Allen et al., 2011a). The reliability and stability of these extracted networks were evaluated via ICASSO (Himberg and Hyvärinen, 2003), a process that iteratively re-runs component estimations with differently bootstrapped datasets. This analysis suggested high stability across the 48 components (mean stability index = 0.89), well above the threshold of 0.70 established in the literature (Ma et al., 2011). The other 27 components were excluded, as they appeared to be related to motion artifacts, spatial maps including white matter, the ventricular system, or cerebrospinal fluid, or having irregular time-course spectra power (Allen et al., 2011a,b). Within GIFT, the time-courses of the RSNs underwent despiking and bandpass by filtering with [0.01–0.15] Hz cutoffs.
Functional connectivity measures
In order to assess various types of resting-state functional connectivity measures, we calculated the sFNC between the selected 48 RSNs as pairwise correlations between the RSNs time-courses for each individual (inter-network connectivity), pairwise correlations between individual voxels within the RNSs to the overall RSN's time-course (intra-network connectivity), and the AFs within each RSN.
Statistical analyses
We performed regression analysis to identify associations between individual sFNC values (inter-network connectivity), spatial maps (intra-network connectivity), and AFs with psychopathy measures: PCL-R Factor 1 and Factor 2 as continuous variables (see Supplementary materials for analyses of PCL-R total). The analyses were corrected for “nuisance” covariates (age, IQ2, FWD3). The significance of the univariate psychopathy results for each factor was determined using a false discovery rate (FDR) (Genovese et al., 2002) threshold at p < 0.05.
Results
Psychopathic traits
The PCL-R total scores for this sample ranged from 2.2 to 34.0 (mean = 18.8, SD = 6.2, Cronbach's α = 0.79; see Table 1). PCL-R Factor 1 scores ranged from 0.0 to 13.0 (mean = 4.6, SD = 2.7; see Table 1), and PCL-R Factor 2 scores ranged from 0.0 to 20.0 (mean = 12.2, SD = 3.8; see Table 1).
Group independent component analysis and group level inter-network connectivity
Figure 1 shows the spatial maps of the 48 selected RSNs. The 48 RSNs listed in Table 2 were grouped into eight domains: auditory (AUD), default mode network (DMN), executive control (ECN), salience (SAL), sensorimotor (SEN), subcortical (SBC), attentional (ATT), and visual (VIS) based on their peak coordinate, functional properties, the automatic labeling tool in GIFT, and confirmed by visual inspection (see Figure 2 for the estimated inter-network connectivity between domains and RSNs)4. Consistent with prior literature, the inter-network connectivity in Figure 2 suggests largely positive within domain inter-network connectivity within the DMN, ECN, SAL, ATT, and VIS networks (Espinoza et al., 2018; Du et al., 2020). Similar to analyses in clinical populations, Figure 2 also suggests cases of negative inter-network connectivity within SBC networks (Du et al., 2020).
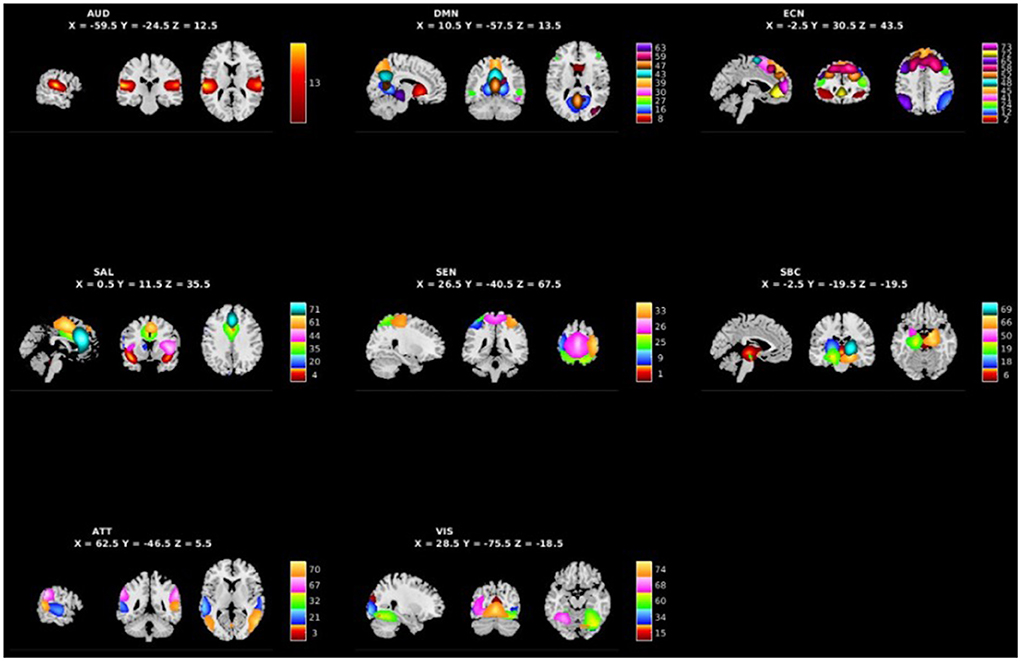
Figure 1. Spatial maps of the 48 independent components identified as RSNs categorized by domain [auditory (AUD), default mode network (DMN), executive control (ECN), salience (SAL), sensorimotor (SEN), subcortical (SBC), attentional (ATT), and visual (VIS)] and component number.
Time-course power spectra
PCL-R factor 1 scores
PCL-R Factor 1 scores were associated with increased AF at low-frequency bands (0–0.05 Hz) in the left middle frontal gyrus (Component 2, ECN), the left superior temporal gyrus (Component 3, ATT), and the right superior frontal gyrus (Component 52, ECN), and decreased AF at high-frequency spectra bands (0.09–0.25 Hz) in the cuneus (Component 15, VIS), left middle frontal gyrus (Component 2, ECN), and the superior frontal gyrus (Component 41, ECN) (see Figures 3, 5, Table 3).
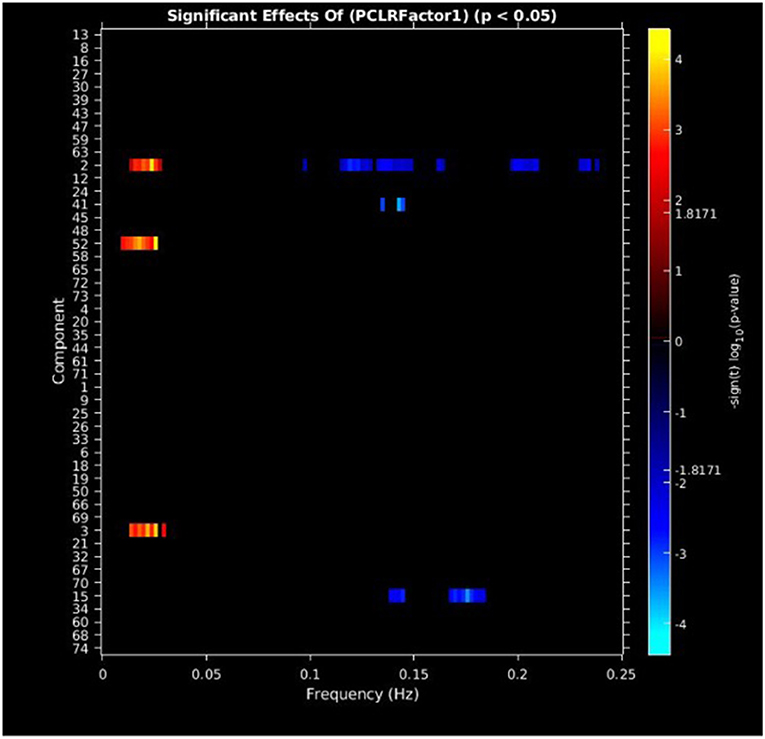
Figure 3. Univariate associations between PCL-R Factor 1 score and power spectra of significant components, predominantly occurring in the ECN. Panel depicts the significance and direction of PCL-R Factor 1 scores as a function of frequency for each significant component, displayed as–sign(t) log10(p), FDR corrected p < 0.05.
PCL-R factor 2 scores
PCL-R Factor 2 scores were associated with decreased AF at high-frequency bands (0.10–0.25 Hz) in the posterior cingulate cortex (Component 47, DMN) and left middle frontal gyrus (Component 2, ECN) (see Figures 4, 5, Table 3).
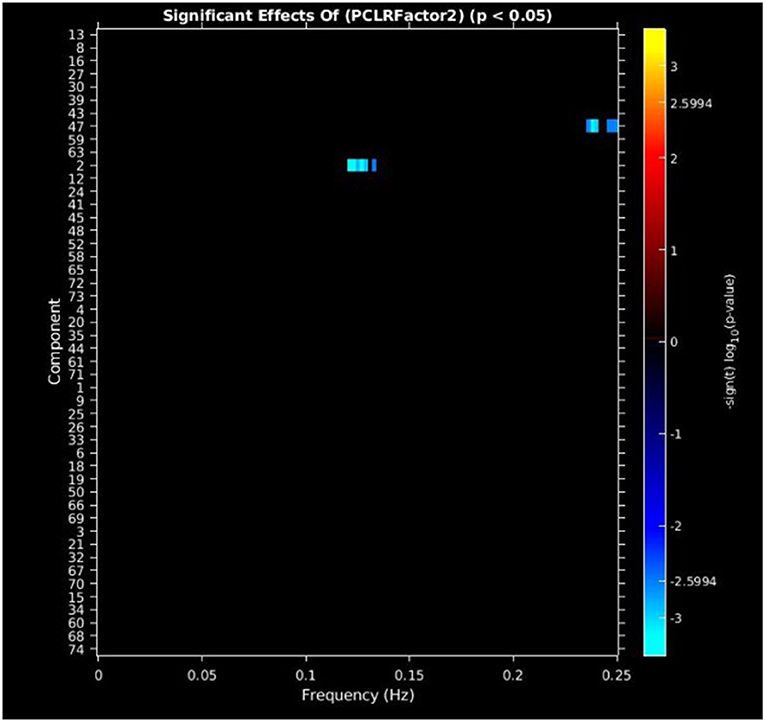
Figure 4. Univariate associations between PCL-R Factor 2 score and power spectra of significant components. Panel depicts the significance and direction of PCL-R Factor 2 scores as a function of frequency for the significant component, displayed as–sign(t) log10(p), FDR corrected p < 0.05.
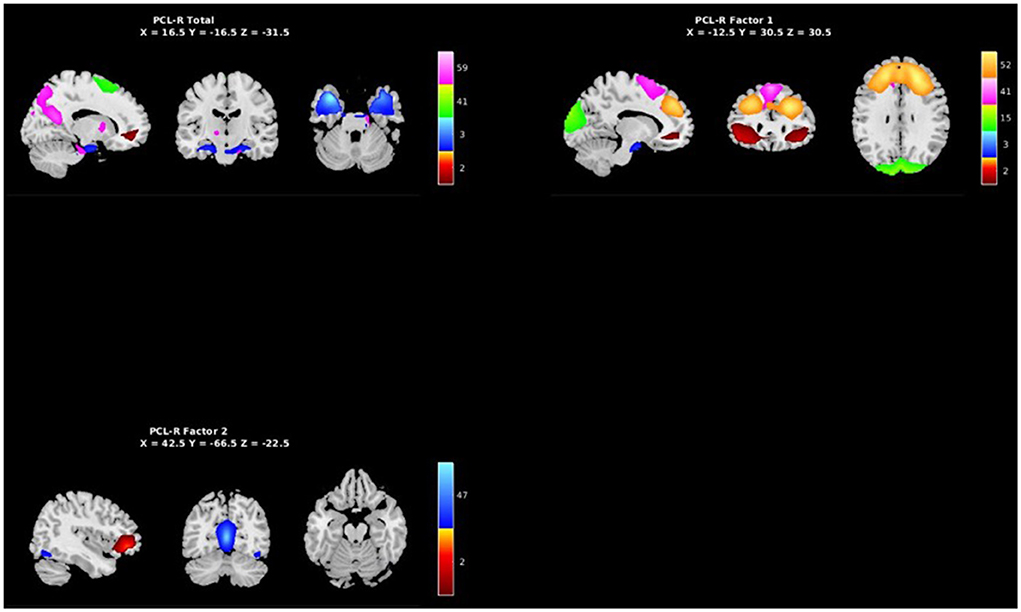
Figure 5. Spatial maps of the 7 independent components identified in Table 3 as exhibiting psychopathy related aberrant AFs, grouped by PCL-R Total effects (see Supplementary materials), PCL-R Factor 1 effects, and PCL-R Factor 2 effects.
Component spatial maps
PCL-R factor 1 scores
PCL-R Factor 1 scores were associated with functional connectivity in a network primarily pertaining to the parahippocampal gyrus (Component 63, DMN), such that higher PCL-R Factor 1 scores were associated with decreased intra-network functional connectivity of the left insula and right thalamus within Component 63 (see Figure 6, Table 4).
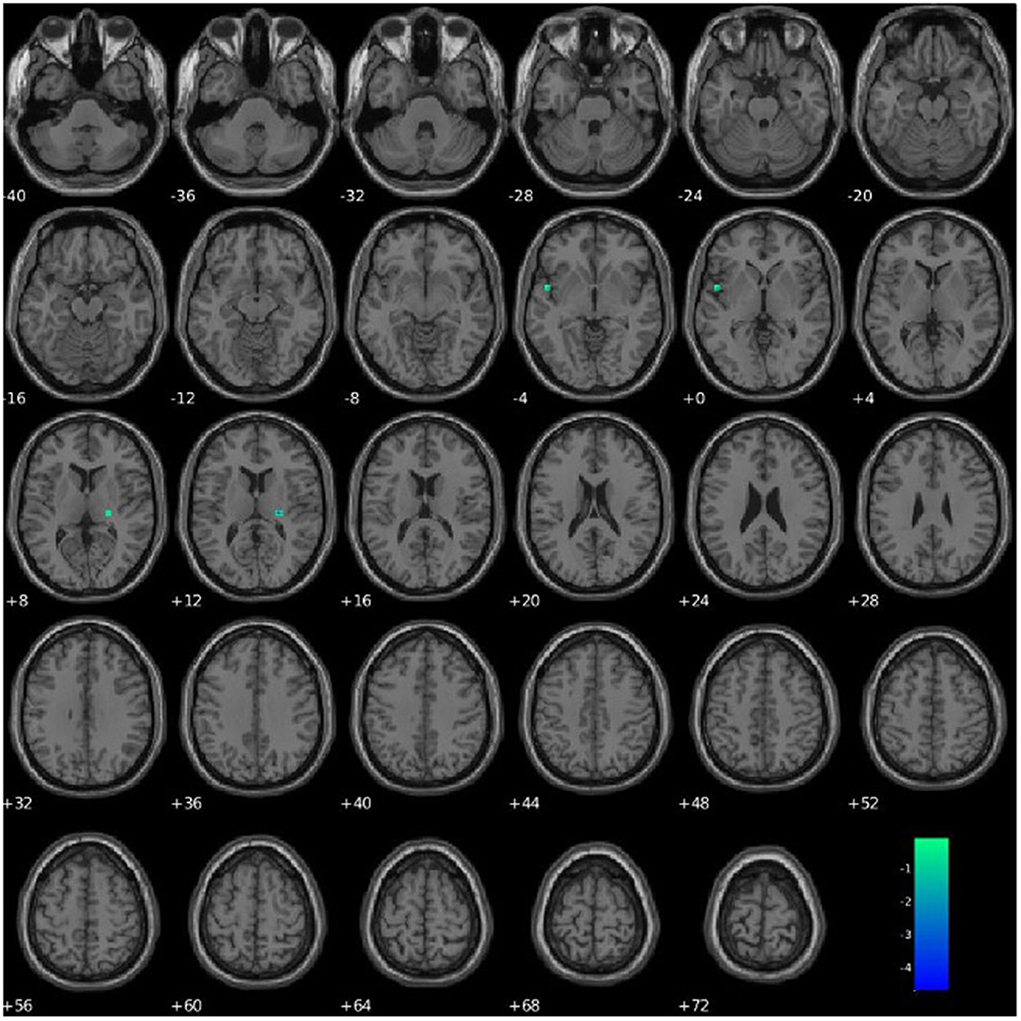
Figure 6. Association between PCL-R Factor 1 score and intra-network connectivity within a network primarily pertaining to the parahippocampal gyrus, FDR corrected p < 0.05.
PCL-R factor 2 scores
PCL-R Factor 2 scores were associated with functional connectivity in the right fusiform gyrus (Component 60, VIS), such that higher PCL-R Factor 2 scores were associated with both increased and decreased intra-network functional connectivity (see Figure 7). PCL-R Factor 2 scores were also associated with functional connectivity in the left lingual gyrus (Component 68, VIS), such that higher PCL-R Factor 2 scores were associated with an increased intra-network functional connectivity (see Figure 7, Table 4).
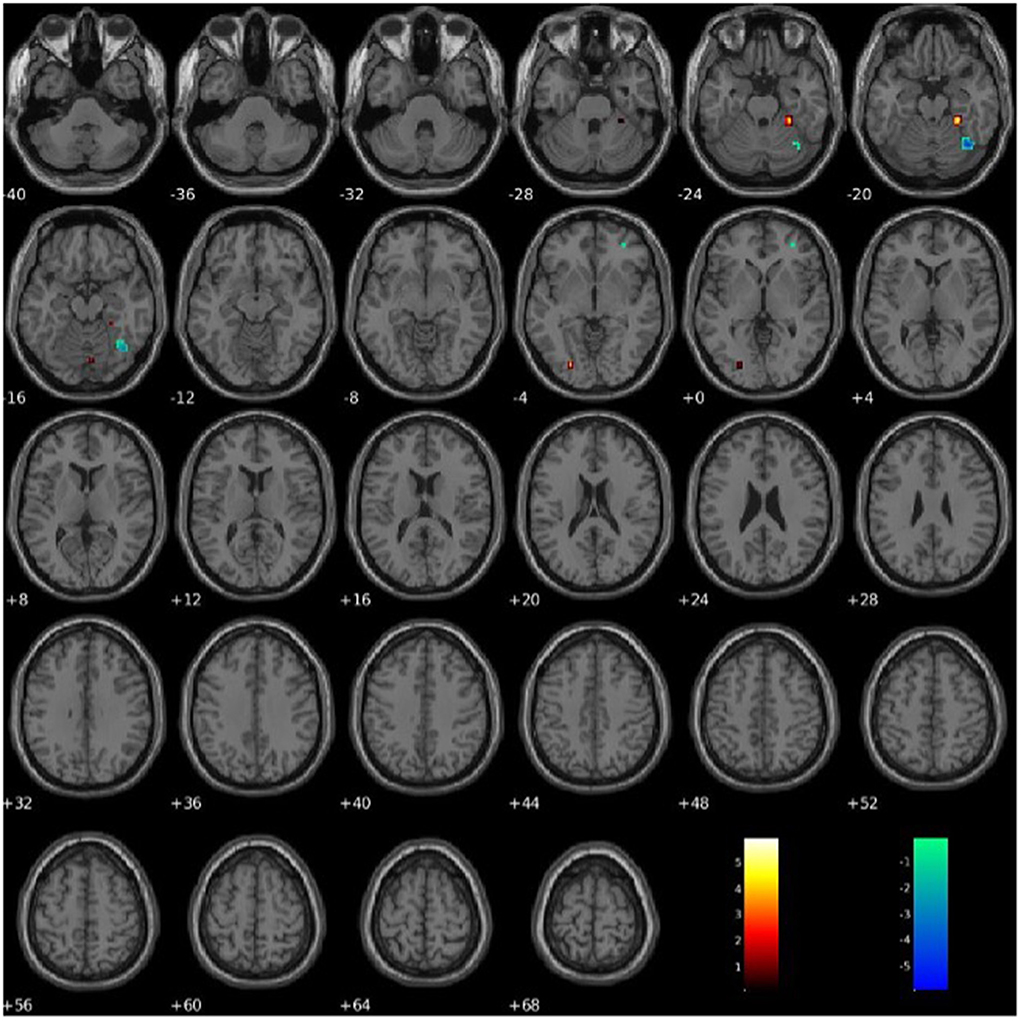
Figure 7. Association between PCL-R Factor 2 score and intra-network connectivity within the lingual gyrus and fusiform gyrus, FDR corrected p < 0.05.
Functional network connectivity
There were no significant associations between PCL-R scores and sFNC that survived FDR correction while controlling for age, IQ, and FWD.
Discussion
Here we report that psychopathic traits (assessed via the PCL-R) were associated with aberrant functional connectivity measures during a resting-state fMRI experimental paradigm in a sample of incarcerated women. Consistent with previous research performed in men and our hypotheses, PCL-R scores were associated with aberrant functional connectivity across multiple domains among incarcerated women.
PCL-R scores were associated with increased amplitude fluctuations (AF) in low-frequency bands and reduced AF in high-frequency bands across regions included within the paralimbic system, including the PCC and superior temporal gyrus, and additional regions, including the superior frontal gyrus, middle frontal gyrus, and cuneus. Compared to low-frequency AFs, high-frequency AFs are believed to contribute to higher-order cognitive processes (Baria et al., 2011; Craig et al., 2018). Reduced high-frequency AFs may relate to some of the previously observed deficits characteristic of women scoring high on psychopathy. For example, successful error-related processing depends on the collaboration of several higher-order brain regions, including the middle/superior frontal gyrus, PCC, and cuneus (Steele et al., 2014). Women scoring high on the PCL-R have been previously characterized by error-related processing deficits, including reduced amplitude of the error-related positivity event-related potential component (Maurer et al., 2016). Furthermore, women scoring high on psychopathy have been previously characterized by reduced reactivity to emotional facial expressions (Eisenbarth et al., 2013); regions implicated in the current study, including the middle frontal gyrus, have been previously associated with processing of emotional faces (Pessoa et al., 2002; Willis et al., 2010).
We also observed that women scoring high on psychopathy were characterized by aberrant intra-network connectivity within regions of the paralimbic system (e.g., parahippocampal cortex) and additional regions, including the fusiform gyrus and lingual gyrus. Women scoring high on psychopathy were characterized by reduced intra-network functional connectivity within Component 63 (parahippocampal gyrus), Component 60 (right fusiform gyrus), and Component 68 (left lingual gyrus), and increased intra-network functional connectivity in Component 60 (right fusiform gyrus). Reduced intra-network functional connectivity in the fusiform and parahippocampal gyrus is consistent with a previously published study with incarcerated women, observing that women scoring higher on the PCL-R were characterized by reduced hemodynamic activity in the fusiform and parahippocampal gyrus during the processing of moral violations (Harenski et al., 2014b). Our finding of PCL-R Factor 2 relating to increases in intra-network functional connectivity of the fusiform and lingual region is also consistent with previously published studies suggesting relationships between impulsivity and intra-visual network functional connectivity (Davis et al., 2013; Pu et al., 2017).
Published studies investigating resting-state AFs and youth psychopathic traits have reported different findings than those obtained in the current study. For example, Thijssen and Kiehl (2017) observed that youth scoring high on the Psychopathy Checklist: Youth Version (PCL:YV; Forth and Kosson, 2003) were largely characterized by decreased AFs in low-frequency bands and increased AFs at high-frequency bands. While differences between studies may have been observed, we believe that the results obtained in the current study may relate to specific deficits previously observed in women scoring high on psychopathy. For example, increased low frequency AFs are believed to relate to improvements within neural efficiency (Biswal et al., 1995). Compared to men scoring high on psychopathy, women scoring high on psychopathy are not characterized by the response perseveration deficits (Vitale and Newman, 2001a). Important to note, response perseveration is associated with dysfunction with several regions implicated in the current study, including the middle frontal gyrus (Yang et al., 2011), superior temporal gyrus (Ersche et al., 2011), and superior frontal gyrus (De Ruiter et al., 2009; Camchong et al., 2011). Therefore, women scoring high on psychopathy may be characterized by increased neural efficiency (observed via higher low-frequency AFs) in several paralimbic brain regions, contributing to improved performance on response perseveration tasks (Vitale et al., 2011). By exhibiting reduced AFs in low-frequency bands, this may relate to youth scoring high on psychopathy exhibiting response preservation deficits (Vitale et al., 2005).
Importantly, and more broadly, our investigation into the relationships between psychopathic traits in women and multiple measurements of rsFNC (inter-network connectivity, intra-network connectivity, and AFs) underscore three points. First, neurobiological aberrances related to psychopathic traits in women may best be accounted for on a local (i.e., intra-network connectivity & AFs) neurobiological level rather than a global level (i.e., inter-network connectivity). Second, while variability in psychopathic traits may be accounted for by the relationships between voxel and RSN time courses (e.g., Thijssen and Kiehl, 2017; Espinoza et al., 2018), additional variability can also be explained by rates and amplitudes of individual RSN activational profiles themselves (i.e., AFs; see Thijssen and Kiehl, 2017). And finally, by exploring both local (i.e., intra-network connectivity and AFs) and global (i.e., inter-network connectivity) rsFNC measures in their relationships to antisocial traits, future research stands to further explore and potentially differentiate how antisocial phenotypes are represented neurobiologically across various demographic groups.
Study limitations
A number of limitations must be considered for the present study. Though all regions identified as being associated with psychopathic traits in the present study were also identified in Espinoza et al. (2018), here we did not find any significant psychopathy related inter-network FNC results. One potential explanation for this result is the size of the sample analyzed. While the present sample is considered large by neuroimaging standards (n = 297), Espinoza et al. (2018) conducted inter-network connectivity analysis in a sample more than three times as large (n = 985). Additionally, because all FNC measures tested in the present analysis were static, there are a number of assumptions being made regarding the relationships between the consistency of network activity across the 5-min resting-state scan. Similarly, because the scans were resting-state rather than task-based, extrapolations of RSN aberrances to specific functional domains are hard to attribute. Finally, while our experimental design utilized 5-min resting-state scans, there is evidence suggesting that longer scans are needed to ensure higher RSN stabilities (e.g., Birn et al., 2013; though see Allen et al., 2011a; Espinoza et al., 2018, 2019; Duda et al., 2022). Thus, more work is needed to probe the relationships between various inter- and intra-network static and dynamic connectivity measures as they relate to psychopathic traits and perhaps longer resting-state and task-based measures in large samples of both men and women.
Conclusion
This study contributes to the current literature by examining whole brain inter- and intra-network connectivity and AFs across RSNs and their relationship to psychopathic traits in women. We showed that psychopathy is associated with increased low-frequency, decreased high-frequency AFs, and both increased and decreased intra-network connectivity across four brain domains (DMN, ECN, VIS, and ATT). Similar to previous analyses in incarcerated men, our results suggest that psychopathic traits among incarcerated women are associated with aberrant intra-network AFs and connectivity across multiple networks associated with executive control, decision making, salience detection, and motor control. Our results showcase aberrant intra-network FNC rather than aberrant inter-network FNC underlying psychopathic traits in women, suggesting the potential for sex-specific neurobiological psychopathy related phenotypes. To our knowledge, this represents the largest study to date on the association of psychopathic traits and intrinsic RSN aberrances in incarcerated women.
Data availability statement
The datasets presented in this article are not readily available because of ethical and privacy restrictions. Requests to access the datasets should be directed to KK, kkiehl@mrn.org.
Ethics statement
The studies involving human participants were reviewed and approved by University of New Mexico IRB and the Independent Review (E&I) Services for the Mind Research Network. The patients/participants provided their written informed consent to participate in this study.
Author contributions
CA performed the statistical analysis and wrote the first draft of the manuscript. JM wrote sections of the manuscript. All authors contributed to the conception, design of the study, manuscript revision, read, and approved the submitted version.
Funding
This material is based upon work supported by the National Institute on Drug Abuse (NIDA; R01 DA020870 and R01 DA026964) and National Institute of Mental Health (NIMH; R01 MH085010).
Conflict of interest
Authors CA, JM, BE, AG, CH, KH, and KK were employed by The Mind Research Network.
The remaining author declares that the research was conducted in the absence of any commercial or financial relationships that could be construed as a potential conflict of interest.
Publisher's note
All claims expressed in this article are solely those of the authors and do not necessarily represent those of their affiliated organizations, or those of the publisher, the editors and the reviewers. Any product that may be evaluated in this article, or claim that may be made by its manufacturer, is not guaranteed or endorsed by the publisher.
Author disclaimer
The contents of this manuscript are solely the responsibility of the authors and do not necessarily represent the views of the National Institute on Drug Abuse nor the National Institute of Mental Health.
Supplementary material
The Supplementary Material for this article can be found online at: https://www.frontiersin.org/articles/10.3389/fnimg.2022.971201/full#supplementary-material
Footnotes
1. ^One participant in the sample was administered the SCID-5-RV, whereas the rest were administered the SCID-I/P.
2. ^As estimated by the vocabulary and matrix reasoning subscales of the Wechsler Adult Intelligence Scale; see Ryan and Ward, 1999.
3. ^Note: The inclusion or exclusion of FWD in analyses did not alter significant findings for any present analyses.
4. ^While the automatic labels and correlations to networks provided by the GIFT toolbox (http://trendscenter.org/software/gift) (Calhoun et al., 2001) were helpful in the initial network classification and description, these network labels differ from those commonly utilized in relevant literature (i.e., Espinoza et al., 2018). Thus, a combination of the correlational outputs/labeling, the peak coordinates, and functional properties commonly ascribed to the regions were used to name regions and assign those regions to relevant domains. The assignment of regions to a specific domain was performed independently by three authors and the final domain classification was based upon unanimous agreements.
References
Allen, E. A., Erhardt, E. B., Damaraju, E., Gruner, W., Segall, J. M., Silva, R. F., et al. (2011a). A baseline for the multivariate comparison of resting-state networks. Front. Syst. Neurosci. 5, 2. doi: 10.3389/fnsys.2011.00002
Allen, E. A., Liu, J., Kiehl, K. A., Gelernter, J., Pearlson, G. D., Perrone-Bizzozero, N. I., et al. (2011b). Components of cross-frequency modulation in health and disease. Front. Syst. Neurosci. 5, 59. doi: 10.3389/fnsys.2011.00059
Anderson, D. A. (1999). The aggregate burden of crime. J. Law Econ. 42, 611–642. doi: 10.1086/467436
Anderson, N. E., Kiehl, K. A. (2012). The psychopath magnetized: insights from brain imaging. Trends Cogn. Sci. 16, 52–60. doi: 10.1016/j.tics.2011.11.008
Baria, A. T., Baliki, M. N., Parrish, T., Apkarian, A. V. (2011). Anatomical and functional assemblies of brain BOLD oscillations. J. Neurosci. 31, 7910–7919. doi: 10.1523/JNEUROSCI.1296-11.2011
Birn, R. M., Molloy, E. K., Patriat, R., Parker, T., Meier, T. B., Kirk, G. R., et al. (2013). The effect of scan length on the reliability of resting-state fMRI connectivity estimates. Neuroimage 83, 550–558. doi: 10.1016/j.neuroimage.2013.05.099
Biswal, B., Zerrin Yetkin, F., Haughton, V. M., Hyde, J. S. (1995). Functional connectivity in the motor cortex of resting human brain using echo-planar MRI. Mag. Reson. Med. 34, 537–541. doi: 10.1002/mrm.1910340409
Blair, R. J. R. (2006). “Subcortical brain systems in psychopathy: the amygdala and associated structures,” in Handbook of Psychopathy, ed C. J. Patrick (New York, NY: The Guilford Press), 296–312.
Calhoun, V. D., Adali, T., Pearlson, G. D., Pekar, J. J. (2001). A method for making group inferences from functional MRI data using independent component analysis. Hum. Brain Mapp. 14, 140–151. doi: 10.1002/hbm.1048
Camchong, J., MacDonald III, A. W., Nelson, B., Bell, C., Mueller, B. A., et al. (2011). Frontal hyperconnectivity related to discounting and reversal learning in cocaine subjects. Biol. Psychiatry 69, 1117–1123. doi: 10.1016/j.biopsych.2011.01.008
Cao, W., Sun, X., Dong, D., Yao, S., Huang, B. (2018). Sex differences in spontaneous brain activity in adolescents with conduct disorder. Front. Psychol. 30, 1598. doi: 10.3389/fpsyg.2018.01598
Carré, J. M., Hyde, L. W., Neumann, C. S., Viding, E., Hariri, A. R. (2013). The neural signatures of distinct psychopathic traits. Soc. Neurosci. 8, 122–135. doi: 10.1080/17470919.2012.703623
Contreras-Rodríguez, O., Pujol, J., Batalla, I., Harrison, B. J., Soriano-Mas, C., Deus, J., et al. (2015). Functional connectivity bias in the prefrontal cortex of psychopaths. Biol. Psychiatry 78, 647–655. doi: 10.1016/j.biopsych.2014.03.007
Cope, L. M., Ermer, E., Nyalakanti, P. K., Calhoun, V. D., Kiehl, K. A. (2014). Paralimbic gray matter reductions in incarcerated adolescent females with psychopathic traits. J. Abnorm. Child Psychol. 42, 659–668. doi: 10.1007/s10802-013-9810-4
Craig, M. M., Manktelow, A. E., Sahakian, B. J., Menon, D. K., Stamatakis, E. A. (2018). Spectral diversity in default mode network connectivity reflects behavioral state. J. Cogn. Neurosci. 30, 526–539. doi: 10.1162/jocn_a_01213
Crooks, D., Anderson, N. E., Widdows, M., Petseva, N., Decety, J., Pluto, C., et al. (2019). The relationship between cavum septum pellucidum and psychopathic traits in female offenders. Behav. Brain Res. 359, 967–972. doi: 10.1016/j.bbr.2018.06.011
Davis, F. C., Knodt, A. R., Sporns, O., Lahey, B. B., Zald, D. H., Brigidi, B. D., et al. (2013). Impulsivity and the modular organization of resting-state neural networks. Cereb. Cortex 23, 1444–1452. doi: 10.1093/cercor/bhs126
De Ruiter, M. B., Veltman, D. J., Goudriaan, A. E., Oosterlaan, J., Sjoerds, Z., Van Den Brink, W. (2009). Response perseveration and ventral prefrontal sensitivity to reward and punishment in male problem gamblers and smokers. Neuropsychopharmacology 34, 1027–1038. doi: 10.1038/npp.2008.175
Del Casale, A., Kotzalidis, G. D., Rapinesi, C., Di Pietro, S., Alessi, M. C., Di Cesare, G., et al. (2015). Functional neuroimaging in psychopathy. Neuropsychobiology 72, 97–117. doi: 10.1159/000441189
Dotterer, H. L., Hyde, L. W., Shaw, D. S., Rodgers, E. L., Forbes, E. E., Beltz, A. M. (2020). Connections that characterize callousness: affective features of psychopathy are associated with personalized patterns of resting-state network connectivity. NeuroImage Clin. 28, 102402. doi: 10.1016/j.nicl.2020.102402
Du, Y., Fu, Z., Sui, J., Gao, S., Xing, Y., Lin, D., et al. (2020). NeuroMark: an automated and adaptive ICA based pipeline to identify reproducible fMRI markers of brain disorders. NeuroImage Clin. 28, 102375. doi: 10.1016/j.nicl.2020.102375
Duda, M., Iraji, A., Ford, J. M., Lim, K. O., Mathalon, D. H., Mueller, B. A., et al. (2022). Spatially constrained ICA enables robust detection of schizophrenia from very short resting-state fMRI data. medRxiv [Preprint]. doi: 10.1101/2022.03.17.22271783
Eisenbarth, H., Angrilli, A., Calogero, A., Harper, J., Olson, L. A., Bernat, E. (2013). Reduced negative affect response in female psychopaths. Biol. Psychol. 94, 310–318. doi: 10.1016/j.biopsycho.2013.07.007
Eisenbarth, H., Krammer, S., Edwards, B. G., Kiehl, K. A., Neumann, C. S. (2018). Structural analysis of the PCL-R and relationship to BIG FIVE personality traits and parenting characteristics in an Hispanic female offender sample. Pers. Individ. Differ. 129, 59–65. doi: 10.1016/j.paid.2018.03.015
Elseoud, A. A., Littow, H., Remes, J., Starck, T., Nikkinen, J., Nissilä, J., et al. (2011). Group-ICA model order highlights patterns of functional brain connectivity. Front. Syst. Neurosci. 5, 37. doi: 10.3389/fnsys.2011.00037
Erhardt, E. B., Rachakonda, S., Bedrick, E. J., Allen, E. A., Adali, T., Calhoun, V. D. (2011). Comparison of multi-subject ICA methods for analysis of fMRI data. Hum. Brain Mapp. 32, 2075–2095. doi: 10.1002/hbm.21170
Ersche, K. D., Roiser, J. P., Abbott, S., Craig, K. J., Müller, U., Suckling, J., et al. (2011). Response perseveration in stimulant dependence is associated with striatal dysfunction and can be ameliorated by a D2/3 receptor agonist. Biol. Psychiatry 70, 754–762. doi: 10.1016/j.biopsych.2011.06.033
Espinoza, F. A., Anderson, N. E., Vergara, V. M., Harenski, C. L., Decety, J., Rachakonda, S., et al. (2019). Resting-state fMRI dynamic functional network connectivity and associations with psychopathy traits. NeuroImage Clin. 24, 101970. doi: 10.1016/j.nicl.2019.101970
Espinoza, F. A., Vergara, V. M., Reyes, D., Anderson, N. E., Harenski, C. L., Decety, J., et al. (2018). Aberrant functional network connectivity in psychopathy from a large (N = 985) forensic sample. Hum. Brain Mapp. 39, 2624–2634. doi: 10.1002/hbm.24028
First, M. B., Spitzer, R. L., Gibbon, M., Williams, J. B. W. (2002). Structured Clinical Interview for DSM-IV-TR Axis I Disorders, Research Version, Patient Edition. (SCID-I/P). New York, NY: Biometrics Research, New York State Psychiatric Institute.
First, M. B., Williams, J. B. W., Karg, R. S., Spitzer, R. L. (2015). Structured Clinical Interview for DSM-5: Research Version. Arlington, VA: American Psychiatric Association.
Forth, A. E., Kosson, D. S. (2003). Hare Psychopathy Checklist: Youth Version. Toronto: Multi-Health Systems, 1–52.
Freire, L., Mangin, J. F. (2001). Motion correction algorithms may create spurious brain activations in the absence of subject motion. Neuroimage. 14, 709–722. doi: 10.1006/nimg.2001.0869
Friston, K. J., Holmes, A. P., Worsley, K. J., Poline, J. P., Frith, C. D., Frackowiak, R. S. (1994). Statistical parametric maps in functional imaging: a general linear approach. Hum. Brain Mapp. 2, 189–210. doi: 10.1002/hbm.460020402
Genovese, C. R., Lazar, N. A., Nichols, T. (2002). Thresholding of statistical maps in functional neuroimaging using the false discovery rate. NeuroImage 15, 870–878. doi: 10.1006/nimg.2001.1037
Hare, R. D. (2003). Manual for the Hare Psychopathy Checklist-Revised. Toronto, ON: Multi-Health Systems.
Hare, R. D., Neumann, C. S. (2010). The role of antisociality in the psychopathy construct: comment on Skeem and Cooke. Psychol Assess. 22:446–54. doi: 10.1037/a0013635
Harenski, C. L., Edwards, B. G., Harenski, K. A., Kiehl, K. A. (2014a). Neural correlates of moral and non-moral emotion in female psychopathy. Front. Hum. Neurosci. 8, 741. doi: 10.3389/fnhum.2014.00741
Harenski, C. L., Harenski, K. A., Kiehl, K. A. (2014b). Neural processing of moral violations among incarcerated adolescents with psychopathic traits. Dev. Cogn. Neurosci. 10, 181–189. doi: 10.1016/j.dcn.2014.09.002
Harpur, T. J., Hare, R. D., Hakstian, A. R. (1989). Two-factor conceptualization of psychopathy: construct validity and assessment implications. Psychol. Assessment A J. Consult. Clin. Psychol. 1, 6. doi: 10.1037/1040-3590.1.1.6
Hemphill, J. F., Hare, R. D., Wong, S. (1998). Psychopathy and recidivism: a review. Legal Criminol. Psychol. 3, 139–170. doi: 10.1111/j.2044-8333.1998.tb00355.x
Himberg, J., Hyvärinen, A. (2003). “Icasso: software for investigating the reliability of ICA estimates by clustering and visualization,” in 2003 IEEE XIII Workshop on Neural Networks for Signal Processing (IEEE Cat. No. 03TH8718), 259–268.
Hu, G., Waters, A. B., Aslan, S., Frederick, B., Cong, F., Nickerson, L. D. (2020). Snowball ICA: a model order free independent component analysis strategy for functional magnetic resonance imaging data. Front. Neurosci. 14, 569657. doi: 10.3389/fnins.2020.569657
Kennealy, P. J., Hicks, B. M., Patrick, C. J. (2007). Validity of factors of the psychopathy checklist—revised in female prisoners: discriminant relations with antisocial behavior, substance abuse, and personality. Assessment 14, 323–340. doi: 10.1177/1073191107305882
Kiehl, K. A. (2006). A cognitive neuroscience perspective on psychopathy: evidence for paralimbic system dysfunction. Psychiatry Res. 142, 107–128. doi: 10.1016/j.psychres.2005.09.013
Kiehl, K. A., Hoffman, M. B. (2011). The criminal psychopath: history, neuroscience, treatment, and economics. Jurimetrics 51, 355.
Kiviniemi, V., Starck, T., Remes, J., Long, X., Nikkinen, J., Haapea, M., et al. (2009). Functional segmentation of the brain cortex using high model order group PICA. Human Brain Mapp. 30, 3865–3886. doi: 10.1002/hbm.20813
Korponay, C., Pujara, M., Deming, P., Philippi, C., Decety, J., Kosson, D. S., et al. (2017). Impulsive-antisocial psychopathic traits linked to increased volume and functional connectivity within prefrontal cortex. Soc. Cogn. Affect Neurosci. 12, 1169–1178. doi: 10.1093/scan/nsx042
Leutgeb, V., Wabnegger, A., Leitner, M., Zussner, T., Scharmüller, W., Klug, D., et al. (2016). Altered cerebellar-amygdala connectivity in violent offenders: a resting-state fMRI study. Neurosci. Lett. 610, 160–164. doi: 10.1016/j.neulet.2015.10.063
Liu, H., Liao, J., Jiang, W., Wang, W. (2014). Changes in low-frequency fluctuations in patients with antisocial personality disorder revealed by resting-state functional MRI. PLoS ONE 9, e89790. doi: 10.1371/journal.pone.0089790
Ma, S., Correa, N. M., Li, X. L., Eichele, T., Calhoun, V. D., Adali, T. (2011). Automatic identification of functional clusters in FMRI data using spatial dependence. IEEE Trans. Biomed. Eng. 58, 3406–3417. doi: 10.1109/TBME.2011.2167149
Maurer, J. M., Paul, S., Edwards, B. G., Anderson, N. E., Nyalakanti, P. K., Harenski, C. L., et al. (2022). Reduced structural integrity of the uncinate fasciculus in incarcerated women scoring high on psychopathy. Brain Imaging Behav. doi: 10.1007/s11682-022-00684-z. [Epub ahead of print].
Maurer, J. M., Steele, V. R., Cope, L. M., Vincent, G. M., Stephen, J. M., Calhoun, V. D., et al. (2016). Dysfunctional error-related processing in incarcerated youth with elevated psychopathic traits. Dev. Cogn. Neurosci. 19, 70–77. doi: 10.1016/j.dcn.2016.02.006
Meda, S. A., Giuliani, N. R., Calhoun, V. D., Jagannathan, K., Schretlen, D. J., Pulver, A., et al. (2008). A large scale (N5400) investigation of gray matter differences in schizophrenia using optimized voxel-based morphometry. Schizophr. Res. 101, 95–105. doi: 10.1016/j.schres.2008.02.007
Pessoa, L., McKenna, M., Gutierrez, E., Ungerleider, L. G. (2002). Neural processing of emotional faces requires attention. Proc. Natl. Acad. Sci. U.S.A. 99, 11458–11463. doi: 10.1073/pnas.172403899
Philippi, C. L., Pujara, M. S., Motzkin, J. C., Newman, J., Kiehl, K. A., Koenigs, M. (2015). Altered resting-state functional connectivity in cortical networks in psychopathy. J. Neurosci. 35, 6068–6078. doi: 10.1523/JNEUROSCI.5010-14.2015
Power, J. D., Mitra, A., Laumann, T. O., Snyder, A. Z., Schlaggar, B. L., Petersen, S. E. (2014). Methods to detect, characterize, and remove motion artifact in resting state fMRI. Neuroimage 84, 320–341. doi: 10.1016/j.neuroimage.2013.08.048
Pu, W., Luo, Q., Jiang, Y., Gao, Y., Ming, Q., Yao, S. (2017). Alterations of brain functional architecture associated with psychopathic traits in male adolescents with conduct disorder. Sci. Rep. 7, 1–11. doi: 10.1038/s41598-017-11775-z
Rachakonda, S., Silva, R. F., Liu, J., Calhoun, V. D. (2016). Memory efficient PCA methods for large group ICA. Front. Neurosci. 10, 17. doi: 10.3389/fnins.2016.00017
Robinson, S., Basso, G., Soldati, N., Sailer, U., Jovicich, J., Bruzzone, L., et al. (2009). A resting state network in the motor control circuit of the basal ganglia. BMC Neurosci. 10, 137. doi: 10.1186/1471-2202-10-137
Ryan, J. J., Ward, L. C. (1999). Validity, reliability, and standard errors of measurement for two seven-subtest short forms of the Wechsler Adult Intelligence Scale—III. Psychol. Assess. 11, 207. doi: 10.1037/1040-3590.11.2.207
Smith, S. M., Fox, P. T., Miller, K. L., Glahn, D. C., Fox, P. M., Mackay, C. E., et al. (2009). Correspondence of the brain's functional architecture during activation and rest. PNAS. 106, 13040–13045. doi: 10.1073/pnas.0905267106
Steele, V. R., Claus, E. D., Aharoni, E., Harenski, C., Calhoun, V. D., Pearlson, G., et al. (2014). A large scale (N = 102) functional neuroimaging study of error processing in a Go/NoGo task. Behav. Brain Res. 268, 127–138. doi: 10.1016/j.bbr.2014.04.001
Tang, Y., Jiang, W., Liao, J., Wang, W., Luo, A. (2013). Identifying individuals with antisocial personality disorder using resting-state FMRI. PLoS ONE 8, e60652. doi: 10.1371/journal.pone.0060652
Thijssen, S., Kiehl, K. A. (2017). Functional connectivity in incarcerated male adolescents with psychopathic traits. Psychiatry Res. Neuroimaging 265, 35–44. doi: 10.1016/j.pscychresns.2017.05.005
Verona, E., Vitale, J. (2018). “Psychopathy in women: assessment, manifestations, and etiology,” in Handbook of Psychopathy, ed C. J. Patrick (New York, NY: The Guilford Press), 509–528.
Vitale, J. E., Maccoon, D. G., Newman, J. P. (2011). Emotion facilitation and passive avoidance learning in psychopathic female offenders. Crim. Justice. Behav. 38, 641–658. doi: 10.1177/0093854811403590
Vitale, J. E., Newman, J. P. (2001a). Using the psychopathy checklist—revised with female samples: reliability, validity, and implications for clinical utility. Clin. Psychol. Sci. Pract. 8, 117. doi: 10.1093/clipsy.8.1.117
Vitale, J. E., Newman, J. P. (2001b). Response perseveration in psychopathic women. J. Abnorm. Psychol. 110, 644–647. doi: 10.1037//0021-843x.110.4.644
Vitale, J. E., Newman, J. P., Bates, J. E., Goodnight, J., Dodge, K. A., Pettit, G. S. (2005). Deficient behavioral inhibition and anomalous selective attention in a community sample of adolescents with psychopathic traits and low-anxiety traits. J. Abnorm. Child Psychol. 33, 461–470. doi: 10.1007/s10802-005-5727-X
Vitale, J. E., Smith, S. S., Brinkley, C. A., Newman, J. P. (2002). The reliability and validity of the psychopathy checklist–revised in a sample of female offenders. Crim. Justice Behav. 29, 202–231. doi: 10.1177/0093854802029002005
Willis, M. L., Palermo, R., Burke, D., McGrillen, K., Miller, L. (2010). Orbitofrontal cortex lesions result in abnormal social judgements to emotional faces. Neuropsychologia 48, 2182–2187. doi: 10.1016/j.neuropsychologia.2010.04.010
Xu, T., Cullen, K. R., Houri, A., Lim, K. O., Schulz, S. C., Parhi, K. K. (2014). “Classification of borderline personality disorder based on spectral power of resting-state fMRI,” in 2014 36th Annual International Conference of the IEEE Engineering in Medicine and Biology Society. IEEE, 5036–5039.
Yang, Y., Raine, A., Colletti, P., Toga, A. W., Narr, K. L. (2011). Abnormal structural correlates of response perseveration in individuals with psychopathy. J Neuropsychiatry Clin. Neurosci. 23, 107–110. doi: 10.1176/appi.neuropsych.23.1.107
Keywords: psychopathy, functional connectivity, intra-network connectivity, spectra, antisocial
Citation: Allen CH, Maurer JM, Edwards BG, Gullapalli AR, Harenski CL, Harenski KA, Calhoun VD and Kiehl KA (2022) Aberrant resting-state functional connectivity in incarcerated women with elevated psychopathic traits. Front. Neuroimaging 1:971201. doi: 10.3389/fnimg.2022.971201
Received: 16 June 2022; Accepted: 20 September 2022;
Published: 04 October 2022.
Edited by:
Filippo Cieri, Neurological Institute, United StatesReviewed by:
Carissa Philippi, University of Missouri–St. Louis, United StatesLena Kaethe Linda Oestreich, The University of Queensland, Australia
Stefano Ferracuti, Sapienza University of Rome, Italy
Zonglei Zhen, Beijing Normal University, China
Copyright © 2022 Allen, Maurer, Edwards, Gullapalli, Harenski, Harenski, Calhoun and Kiehl. This is an open-access article distributed under the terms of the Creative Commons Attribution License (CC BY). The use, distribution or reproduction in other forums is permitted, provided the original author(s) and the copyright owner(s) are credited and that the original publication in this journal is cited, in accordance with accepted academic practice. No use, distribution or reproduction is permitted which does not comply with these terms.
*Correspondence: Corey H. Allen, callen@mrn.org; Kent A. Kiehl, kkiehl@unm.edu