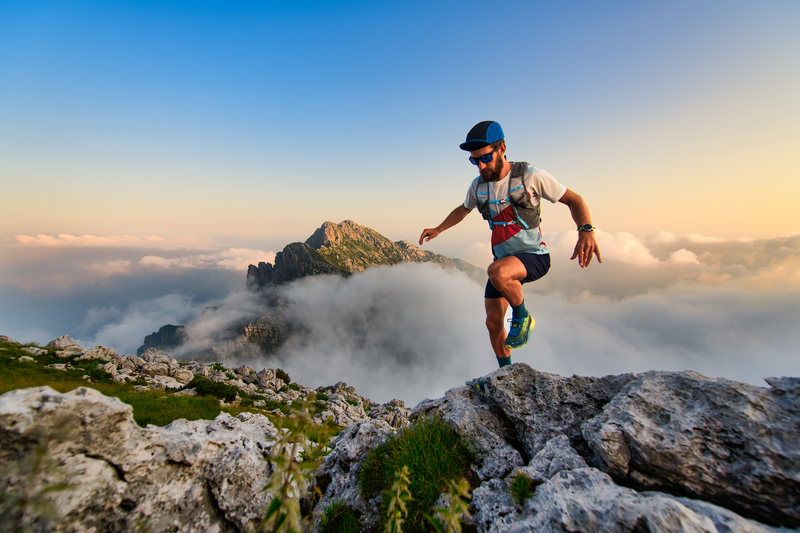
94% of researchers rate our articles as excellent or good
Learn more about the work of our research integrity team to safeguard the quality of each article we publish.
Find out more
BRIEF RESEARCH REPORT article
Front. Hum. Neurosci. , 13 March 2025
Sec. Brain Imaging and Stimulation
Volume 19 - 2025 | https://doi.org/10.3389/fnhum.2025.1514087
No prior work has directly compared the impacts of transcranial photobiomodulation (tPBM) and transcranial magnetic stimulation (TMS) on the human brain. This within-subjects pilot study compares the effects of tPBM and TMS of human somatomotor cortex on brain structural and functional connectivity. Eight healthy participants underwent four lab visits each, each visit consisting of a pre-stimulation MRI, stimulation or sham, and a post-stimulation MRI, respectively. Stimulation and sham sessions were counterbalanced across subjects. Collected measures included structural MRI data, functional MRI data from a finger-tapping task, resting state functional connectivity, and structural connectivity. Analyses indicated increased activation of the left somatomotor region during a right-hand finger-tapping task following both tPBM and TMS. Additionally, trending increases in left-lateralized functional and structural connectivity from M1 to thalamus were observed after tPBM, but not TMS. Thus, tPBM may be superior to TMS at inducing changes in connected nodes in the somatomotor cortex, although further research is warranted to explore the potential therapeutic benefits and clinical utility of tPBM.
Pharmacological treatment of neurological disorders (e.g., Parkinson’s Disease; PD) and psychiatric disorders (e.g., Major Depressive Disorder; MDD) has had limited success and numerous undesirable side effects (Borovac, 2016; Ferguson, 2001). As such, there is a recent push to identify non-pharmacological treatments that target relevant brain regions and circuits using neuromodulation techniques such as deep brain stimulation (DBS) and transcranial magnetic stimulation (TMS). Though both techniques have had success at treating both MDD and PD, each has notable limitations that decrease its clinical utility. For example, the implementation of electrodes for DBS require risky surgery (Kantzanou et al., 2021), and the implanted pulse generators have a limited lifespan due to battery failure (Sarica et al., 2021). Meanwhile, TMS may afford greater specificity of treatment than pharmaceuticals without the invasive surgery of DBS (Hallett, 2007; Khedr et al., 2005; Lefaucheur, 2019; Schiena et al., 2021); however, TMS is limited to stimulating only the cortical surface due to rapid attenuation of the electric field as it nears the center of the brain (Deng et al., 2013; Hardwick et al., 2014). Sophia A Bibb novel method of brain stimulation, known as transcranial photobiomodulation (tPBM), may help to address these shortcomings.
Transcranial photobiomodulation involves the use of light from the visible and near infrared (NIR) spectrum at a low power density on soft tissue for therapeutic effect (Cardoso et al., 2022; Fekri et al., 2019; Zomorrodi et al., 2019). In the context of neuromodulation, tPBM is applied to the scalp and penetrates through the bone up to ∼4 cm below the cortical surface (Tedford et al., 2015), which is deeper than TMS is able to penetrate, even when using the largest TMS coils available (Deng et al., 2013). The ability to penetrate to deeper brain structures may allow clinicians to target nodes that are inaccessible using TMS, which may in turn improve clinical outcomes of treatment (Fitzsimmons et al., 2024; Reddy et al., 2023).
Prior research investigating the impact of tPBM on brain function has demonstrated that use of 600–1,070 nm wavelength tPBM improves both locomotive and cognitive behavior in both human and animal models (Fekri et al., 2019; Figueiro Longo et al., 2020; Johnstone et al., 2021; Wang et al., 2019). Clinically relevant findings in humans include improvement of cognitive function in individuals with Alzheimer’s disease (Johnstone et al., 2016) and general increase in positive affect following treatment (Barrett and Gonzalez-Lima, 2013). Although tPBM’s precise mechanisms of action are not yet clear, researchers hypothesize that the observed functional changes in brain activity are the result of either direct stimulation of distressed neurons or indirect stimulation of neurons via increased ATP production in neuronal mitochondria (Chung et al., 2012; Wang et al., 2017).
While extant research has characterized the effects of tPBM on neuronal function, no prior studies have directly compared the effects of tPBM and TMS on functional and structural connectivity between stimulated regions and connected nodes. Here, we use a multi-wavelength tPBM system to investigate the effects of 1,064 nm laser treatment on brain function and connectivity in the primary motor (M1) and primary somatosensory (S1) cortices (henceforth referenced together as the somatomotor cortex) as compared to high-frequency TMS. In this initial stage to establish proof of concept, we focused our treatment on the somatomotor cortex for two reasons: first, because motor deficits are prevalent in/comorbid to both neurological and psychiatric disorders (e.g., PD and MDD); second, because somatomotor cortex is an optimal target to compare the effects of both tPBM and TMS because of its proximity to the cortical surface. We asked two questions in this study. First, can tPBM alter BOLD signal in the somatomotor cortex during a finger-tapping task, and how do the effects of this stimulation compare to functional changes elicited by TMS? We investigated this question using functional task MRI. Second, how does tPBM treatment impact efferent motor pathways and connected regions? We explored this question by assessing functional and structural connectivity before and after stimulation. All exploratory analyses were conducted with the goal of identifying short-term effects of tPBM stimulation (compared to TMS and sham) within subjects.
Ten healthy volunteers were recruited from the Ohio State University campus. Participants were required to be 18–35 years of age, native English-speakers, report normal or corrected-to-normal vision (including visual acuity, binocular depth perception, and color vision), report normal audition, no history of neurological or psychiatric diseases, and have a primary care practitioner (required by the Center for Cognitive and Behavioral Brain Imaging center). Further, participants were required to have no contraindications for MRI or TMS, no pregnancy, and not be taking psychoactive substances or medications that induce light-sensitivity. Participants were informed of any risks and provided informed consent prior to participation. Monetary compensation was provided for voluntary participation in the study. The protocol was approved by the Institutional Review Board (protocol number: IRB- 2022H0044). Two participants failed to complete all study sessions, resulting in a final sample of eight healthy participants (two female; M = 23.23 years, SD = 2.96 years).
Each participant underwent four neuromodulation visits, wherein they received one of the following treatments: tPBM, sham tPBM, TMS, or sham TMS, with order counterbalanced across subject (Table 1). We included sham stimulation to control for the effects of experience and task-learning on collected brain measures. Days between adjacent lab visits varied from two to 64 days (M = 13 days; SD = 15 days). For both tPBM and sham tPBM, participants were comfortably seated facing away from the tPBM system and were instructed to keep still during stimulation. Both the participants and experimenters wore dark safety goggles during both sham and active sessions. The tPBM system (shown in Figure 1) consisted of a 1,064 nm (Zhao et al., 2022) continuous wave laser (EVO FX Tri-wavelength Class-4 Therapy Laser). The circular laser beam was positioned over the vertex of the cranium according to the 10–20 international system. Importantly, the vertex was used as the point of stimulation in order to balance the headset, as participants found it uncomfortable to wear if the laser were positioned directly over the somatomotor region; we felt comfortable doing this because previous work has demonstrated that tPBM stimulation has broad focality, making precision of application less essential for tPBM than for TMS (Wang et al., 2019). Furthermore, prior work from our group simulating the optical scattering of the tPBM laser using Monte Carlo eXtreme from MATLAB (Fang and Yan, 2019) confirmed that the bihemispheric somatomotor regions, including the right hand area of M1, fell within the range of stimulation when the laser was applied at the vertex. The laser was held in place by a modified OpenBCI 3D printed headset with a strap under the participant’s chin. Active tPBM sessions consisted of laser output of 0.6 W for 11 min for a total dosage of 396 J. Sham tPBM sessions consisted of 0.1 W for 11 min, and black electrical tape placed over the beam to assist in blocking the laser.
Table 1. Counterbalanced order of stimulation vs. sham sessions for transcranial photobiomodulation (tPBM) and transcranial magnetic stimulation (TMS) for all participants.
Figure 1. Transcranial photobiomodulation (tPBM) system and setup. (Left) Laser system control console. (Right) Participant with headset, with laser situated over the vertex of the head (Cz).
Transcranial magnetic stimulation was delivered using a MagPro X100 stimulator with a Cool B6 Coil. Participants first underwent the motor cortex mapping and motor threshold determination. Single pulses of biphasic waves, starting at 30% of maximum stimulator output, were provided to a point corresponding to C3 on the participant’s head, 45° to the midsagittal line. Stimulation was titrated until 50% of trials elicited a visible contraction of the first dorsal interosseus muscles of the hand, and the pulse amplitude and location of the coil were recorded for each participant (Badran et al., 2019). The coil contained an active stimulation face, where the electromagnetic field could pass freely to the subject’s cortex, and an opposing, shielded face, with an insert that blocked the electromagnetic field during sham sessions. The faces were not marked for the subject, so the subject remained blinded to the condition. During TMS sessions, biphasic stimulation was provided to this spot using the excitatory intermittent theta burst protocol (Oberman et al., 2011) at 80% of the participant’s resting motor threshold for 600 pulses. Sham TMS consisted of the same protocol, but with the shielded face of the coil applied to the participant’s head.
Magnetic resonance imaging scans were conducted twice per visit, both pre- and post-stimulation or sham. Each MRI session lasted approximately 1 h, including all tasks and time getting the participant comfortably in and out of the scanner. Participants were scanned using Siemens 3T Magnetom Prisma with a 32-channel head coil. First, a high resolution (TE/TR = 2.9/2,300 ms, voxel size = 1.0 × 1.0 × 1.0 mm3) T1 weighted 3D magnetization-prepared rapid acquisition with gradient echo (MPRAGE) was collected. Next, 10 min of DWI data (to compute structural connectivity) were acquired using echo planar imaging (EPI; 78 slices, voxel size 2.0 × 2.0 × 20 mm3, 256 mm field of view, bandwidth of 1,698 Hz/Px, TR = 3,400 ms) with a multiband factor of two with b-values of 2,000 s/mm2, and one b = 0 volume. Diffusion weighting was isotropically distributed along 96 directions. Then, 10 min of resting state fMRI data (to define functional connectivity) was collected using echo planar imaging on all participants while awake for approximately 10 min (TE/TR = 28/2,000 ms, voxel size = 2.0 × 2.0 × 2.0 mm3).
Task fMRI scans (EPI sequence, TR = 1,000 ms, TE = 28 ms, voxel size = 2.0 × 2.0 × 3.0 mm3) consisted of three 3.72 min runs of a block-design finger-tapping task per MRI session (Khoury et al., 2019). Each run of the motor task was comprised of four 18 s blocks of rest and four 18 s blocks of motor action, for a total of eight blocks per run. Rest was cued by presentation of a fixation cross, wherein participants were instructed to remain still and move as little as possible. Motor action was cued by a line drawing of a hand, whereafter participants were instructed to tap either the second or third digit of their right hand on a button box (following the finger indicated on the picture) at a frequency of 1 Hz. The output from the button box was monitored to ensure that the participant remained awake and complied with the task directions. A total of six finger-tapping task runs were collected per visit—three runs pre-stimulation, and three runs post-stimulation.
All structural (MPRAGE) images were preprocessed using the recon-all pipeline in FreeSurfer (FreeSurfer Wiki, 2024c) with default parameters. This pipeline includes intensity correction, skull stripping, surface co-registration, spatial smoothing, segmentation of white matter and subcortical areas, and parcellation of the cortex. All outputs were visually inspected to ensure successful reconstruction. The reconstructed images were used for multimodal registration within subject and across sessions, as well as to the group average template.
First, we examined whether tPBM would increase BOLD response during a finger-tapping task (Khoury et al., 2019) and how any observed change compared to that induced by TMS. Task data preprocessing was conducted in FreeSurfer using FS-Fast and included (a) motion correction, where timepoints with greater than 1 mm total vector moment between TRs were identified and regressed out as framewise nuisance regressors, (b) distortion correction, and (c) registration to anatomical space (using FreeSurfer’s bbregister) (Greve and Fischl, 2009). For each subject, the neural activation of motor activity (β estimates and contrast maps) were computed in FreeSurfer (using FS-Fast) using a first-level general linear model (GLM) analysis. Data were detrended and smoothed (FWHM = 3 mm kernel) with a regressor entered for the contrast of interest (finger tapping) as well as six motion parameters as nuisance regressors. A block design with a standard boxcar function (events on/off) convolved with a canonical hemodynamic response function was used. The resulting contrast maps for finger tapping over baseline were used for the probabilistic maps of activation. Individual contrast maps were registered from the subject’s native space (FreeSurfer Wiki, 2024a), and then projected onto the surface of each hemisphere and then to FreeSurfer’s FSAverage space. Contrast maps were thresholded and binarized for each subject to show any voxels where the effect of stimulation was 1.3x the effect of sham stimulation, and then concatenated across subjects to create a probabilistic map of activation, with the value of voxel corresponding to the number of subjects with significant motor activation.
We further explored the effect of stimulation within each individual’s somatomotor cortex. Subject-specific functional regions of interest (fROIs) were defined in MATLAB using the Group-Constrained Subject-Specific method (Fedorenko et al., 2010). Specifically, we extracted the top 10% of activated voxels (across pre- and post-stimulation runs) within the somatomotor cortex (precentral and postcentral gyri) for the automated Desikan/Killiany segmentation (Desikan et al., 2006) in individual subject space. The percent signal change (PSC) in identified fROIs was then computed in MATLAB for the three pre-stimulation motor task runs and three post-stimulation runs using the absolute value of first-level β estimates for the motor blocks divided by the baseline (intercept) for post- and pre- runs for each session (tPBM stimulation, tPBM sham, TMS, TMS sham). The effect of stimulation on motor activation was defined as the absolute change in PSC from pre- to post-stimulation minus the change in PSC from pre- to post-sham stimulation:
One-sample t-tests were conducted in MATLAB to assess whether normalized PSC was significantly different from zero for both tPBM and TMS. Paired t-tests were then conducted to assess whether PSC due to stimulation differed within subjects between tPBM and TMS.
Structural connectivity describes how physically connected two brain regions are by neuronal white matter tracts (Warbrick et al., 2017). Similarly to functional connectivity, alterations in white-matter have been observed in both MDD and PD (Droby et al., 2022; Liberg and Rahm, 2015); thus, we examined the absolute and relative effects of tPBM and TMS on structural connectivity of the somatomotor cortex to connected nodes. Fractional anisotropy (FA) is the measurement of the directionality of water diffusion along axons and provides an approximation of structural integrity of white matter tracts connecting two regions (Elton et al., 2023). FA image preprocessing was conducted using FDT (FMRIB’s Diffusion Toolbox), which is a part of FSL (FMRIB’s Software Library)1 (Behrens et al., 2003; Behrens et al., 2007). Preprocessing included an eddy current correction (eddycorrect from FDT) and brain extraction (BET from FDT). The images were then eroded and the end slices coded to zero in order to remove potential outliers. The images were then non-linearly registered to a standard template (Jenkinson et al., 2012) and thresholded at 0.2. Participant images were then concatenated into a single file for group analysis. A repeated measures ANOVA was used to calculate group statistics using threshold free cluster enhancement (TFCE) with a standard space white matter skeleton used to mask FA images.
To perform individual-subject region-to-region connectivity analyses (i.e., quantitative tractography), automated cortical parcellations were registered to each participant’s diffusion images. Image preprocessing was again conducted using FDT and included an eddy current correction (eddycorrect from FDT) and brain extraction (BET from FDT). Motor regions were used as seed regions for the analysis, while the thalamus and striatal regions were chosen as target regions. Principal diffusion directions were calculated for each voxel and probabilistic tractography was carried out using FDT with 10,000 streamline samples in each seed voxel to create a connectivity distribution to each of the target regions, while avoiding the ventricles as defined by a mask. Thus, every voxel within the motor seed region was described by a vector of connection probabilities to the target regions; we averaged these connection probabilities across voxels of the motor cortex per subject and then calculated the effect of stimulation on structural connectivity as above for functional connectivity. Inset of Figure 3 shows an example participant’s FDT_paths file, thresholded at 10% of maximum streamlines, as visualization of the tracts connecting motor to subcortex. While we had an a priori αlevel of 0.05, we found no differences in FA from pre- to post-tPBM stimulation at this level. Therefore, Figure 3 shows a cluster-corrected group map instead thresholded at p < 0.07.
Functional connectivity describes the extent to which two brain regions coactivate and is thought to reflect both direct and indirect connections between related brain regions (Bullmore and Sporns, 2009; Logothetis, 2003). We examined how tPBM compares to TMS in modulating the brain’s functional connectivity at rest (i.e., resting state functional connectivity; rsFC), as disturbances in typical rsFC networks have been seen in both MDD and PD (de Kwaasteniet et al., 2013; Petersen et al., 2018; Wang et al., 2017). RsFC data preprocessing was conducted using FreeSurfer’s FS-Fast (FreeSurfer Wiki, 2024b) and included motion correction, smoothing gray matter with a 3 mm FWHM kernel, linear interpolation for spikes greater than 0.5 mm, and bandpass filtering (0.0009–0.08 Hz). The functional connectivity changes were then explored on an individual subject-level within the following regions of interest (ROIs), defined from the Desikan-Killiany automated segmentation (Desikan et al., 2006) in subject space: motor cortex, thalamus, and striatum (caudate and putamen). Correlation analyses were run for signal extracted from the motor cortex to those of the thalamus and striatum. Time courses were averaged within each region of interest, then the Pearson product correlations between the time series were computed. The r values were then Fisher z-transformed and the effect of stimulation on connectivity was defined using the Fisher correlations as the difference from pre- to post-stimulation over difference from pre- to post-sham stimulation.
Analyses for all MRI modalities (i.e., task-based fMRI, structural connectivity, and resting state functional connectivity) were calculated based on changes in activation or connectivity after neuromodulation, normalized by changes after sham for both tPBM and TMS.
We first evaluated whether tPBM and TMS induced detectable changes in BOLD response to a finger-tapping motor task in each participant’s bilateral somatomotor fROIs. tPBM stimulation of bi-hemispheric somatomotor cortex elicited changes in somatomotor activation in the left hemisphere (LH) only, controlling for changes observed in the sham sessions (see Figure 2A for probabilistic atlas of activation across participants). Stimulation of similar loci was observed in the TMS group. Extraction of subject-specific fROIs revealed that both tPBM and TMS evoked increased motor activation in the LH somatomotor fROI only [Figure 2B; tPBM: LH: t(7) = 2.26; p = 0.058; Hedge’s g = 0.710; RH: t(7) = 0.59; p = 0.57; TMS: LH: t(7) = 3.37; p = 0.012, Hedge’s g = 1.05; RH: t(7) = −0.36; p = 0.72]. There were no differences between tPBM and TMS activation in LH and RH somatomotor region fROIs [LH: t(7) = 1.480; p = 0.184; RH: t(7) = 1.147; p = 0.290].
Figure 2. Effects of stimulation on fMRI activation. (A) Probabilistic map across subjects of changes in somatomotor activation after stimulation, thresholded to show effects in over 4/8 subjects. Black outline indicates somatomotor region of interest (ROI). (B) Individual subject changes in activation (percent signal change; PSC) of subject-specific somatomotor ROIs after stimulation, normalized by sham changes. Individual-subject data points indicated via open circles, with standard error in black lines.
We next evaluated changes in structural connectivity, starting with whole-brain voxelwise FA within white matter, to assess whether tPBM and TMS impacted the integrity of white matter connections between brain regions. Following tPBM stimulation, we found trending increase in FA within LH thalamic pathways only (around the anterior thalamic radiations and portions of the corticospinal tract and uncinate fasciculus; whole brain cluster correction, p < 0.07; Figure 3A). We found no differences between tPBM and TMS within subjects (no clusters surviving whole brain cluster correction, even at p < 0.1). Likewise, quantitative (probabilistic) tractography revealed a trending increase in connectivity between the LH M1 and thalamus following tPBM stimulation [LH: t(7) = 1.94; p = 0.08; RH: t(7) = 0.64; p = 0.54; Figure 3B], while TMS did not induce connectivity changes in LH [t(7) = 1.19; p = 0.28] or RH [t(7) = 1.79; p = 0.12]. There were no differences between tPBM and TMS’ effects on the LH [t(7) = −1.86, p = 0.11] or RH [t(7) = −0.59, p = 0.58] somatomotor cortices.
Figure 3. Effects of stimulation on structural and functional connectivity. (A) Changes in white matter FA after transcranial photobiomodulation (tPBM) stimulation, normalized by changes during sham session; cluster corrected group map at p < 0.07. (B) Changes in probabilistic tractography performed per subject to map motor to thalamic white matter connections [illustrated on representative subject in inset, with arrows indicating motor and thalamic region of interest (ROIs)]. Bar plots show changes after tPBM and transcranial magnetic stimulation (TMS) on left hemisphere (LH) motor-thalamic structural connectivity. (C) Changes in stimulation on LH motor-thalamic functional connectivity. Individual-subject data points indicated via open circles, with standard error in black lines.
Functional connectivity analyses investigating the impact of stimulation on coactivation of related brain regions revealed similar results, with significant increase in LH M1-thalamus connectivity [t(7) = 2.35; p = 0.0432] but not in RH [t(7) = 0.78; p = 0.46; Figure 3C] following tPBM. TMS did not induce any significant changes in connectivity in LH [t(7) = 0.11; p = 0.92] or RH [t(7) = 0.48; p = 0.64]. There were also no differences between tPBM and TMS’ effects on functional connectivity [LH: t(7) = 1.84, p = 0.11; RH: t(7) = −0.93, p = 0.38].
The purpose of this pilot study was to examine the functional and structural impacts of tPBM on somatomotor cortex and connected regions when compared to high-frequency TMS and sham using functional and structural MRI. Our preliminary data showed a significant increase in left-hemispheric somatomotor cortex activation during the finger-tapping task for both tPBM and TMS. We also observed trending increases in both structural and functional connectivity between left-hemispheric somatomotor regions and striatal/thalamic pathways following tPBM only.
Our findings of increased BOLD activity in LH somatomotor cortex following stimulation align with prior literature (Khedr et al., 2005; Kim and Shin, 2014; Saltmarche et al., 2017) and provide evidence that both tPBM and TMS may have a modulatory effect on BOLD activation in response to a motor task. Based on prior work, we hypothesize that areas that tPBM increased blood flow to the somatomotor cortex during the finger-tapping task by means stimulating mitochondrial respiration, resulting in increased production of the vasodilator nitrous oxide, one of the biproducts of mitochondrial respiration (Poyton and Hendrickson, 2016; Saucedo et al., 2021). While we did not collect a behavioral measure of motor task performance (e.g., speed of tapping), future work may include fMRI analyses of pre- and post-stimulation effortful motor tasks to assess change in behavioral motor function.
As aforementioned, tPBM at the vertex stimulated both left and right somatomotor cortices; however, trending change was only observed in LH somatomotor cortex. This may suggest that cortical areas active before and after stimulation, as in the finger-tapping task, may be primed for change after stimulation—though further research is needed. Additionally, our trending increases in functional and structural connectivity suggest tPBM might surpass TMS in inducing structural changes in connected nodes, a finding that is tentatively supported by extant research (Chao, 2019; Urquhart et al., 2020). However, structural changes following TMS have also been reported (Beynel et al., 2020; Schiena et al., 2021), and our findings did not reach statistical significance. Furthermore, we found no statistical difference between tPBM and TMS in subcortical connectivity. Our null findings may stem from insufficient power rather than TMS inefficacy, warranting further investigation into their differential effects on connectivity.
Indeed, one limitation of this study is the small, predominantly male sample. Although the within-subjects design enhances statistical power, there is high individual variability in tPBM and TMS effects (Dole et al., 2023; Wassermann et al., 2008); furthermore, it is unclear whether sex impacts the results of TMS and tPBM stimulation, and due to sample size, the present study is underpowered to test sex differences. Larger samples may yield stronger effect sizes and allow for investigation of sex differences in stimulation effects. The sample’s average age is also relevant, as neuroplasticity and oxidative stress change across the lifespan, potentially altering tPBM and TMS effects on brain connectivity (Chan et al., 2019; Gutiérrez-Menéndez et al., 2022; Saucedo et al., 2021). Future studies should seek to compare younger and older healthy populations. Additionally, baseline differences in connectivity and stress levels between healthy individuals and those with PDD or MDD may influence responses to tPBM (Cardoso et al., 2022; Petersen et al., 2018). Clinical samples are needed to determine whether these preliminary findings generalize to patient populations.
It is also worth noting that we observed differences between subjects in the direction and extent of change in somatomotor activation following stimulation. While we address this by normalizing every individual’s post-neuromodulation data by sham, future work investigating the impact of tPBM and TMS on neural circuitry, including the effect of stimulation on neuroinflammation, mitochondrial function, and synaptic plasticity, may help to explain the directionality and causal mechanisms behind the physiological changes that occur following tPBM and TMS in human participants (Saucedo et al., 2021; Zong et al., 2020). Furthermore, because of our small sample size, we lacked sufficient power to investigate whether there were any order effects (i.e., long-term effects of the first treatment that might have impacted subsequent treatments). Alhough we attempted to control for order effects by counterbalancing treatment order, future work specifically investigating the long-term effects of tPBM and TMS is warranted.
With regards to the tPBM stimulation, further investigation is needed to examine whether different infrared wavelengths and irradiation levels of tPBM can sufficiently target deeper brain structures, such as the ventral and dorsal striatum, which cannot be stimulated using non-invasive techniques like TMS. Finally, it is important to consider the focality of tPBM stimulation. Due to optical scattering of light as the laser passes through brain tissue, tPBM stimulates a large area of the brain with broad focality (Boas et al., 2002; Huang et al., 2021; Yuan et al., 2020). While this quality is useful for stimulating multiple related brain regions at once (i.e., somatomotor cortex) for maximum therapeutic effect, it is also important to consider the influence of off-target effects of other brain regions on observed effects on brain function.
In conclusion, the present work highlights the potential utility of tPBM to stimulate neuronal activity and increase functional and structural connectivity of the targeted area to connected nodes. In the context of clinical work, tPBM shows promise as a tool to induce neuroplasticity in diseased populations, though further work in diseased samples is necessary to corroborate our tentative findings. As the first study to directly compare tPBM and TMS using MRI, the present work serves as a useful pilot study, paving the way for future investigation of the clinical utility of tPBM for motor deficits in humans.
The datasets for this article are not publicly available due to concerns regarding participant anonymity. Requests to access the datasets should be directed to the corresponding author.
The studies involving humans were approved by The Ohio State University Institutional Review Board. The studies were conducted in accordance with the local legislation and institutional requirements. The participants provided their written informed consent to participate in this study.
SB: Formal Analysis, Visualization, Writing – original draft, Writing – review and editing. EY: Data curation, Writing – review and editing. MM: Data curation, Formal Analysis, Visualization, Writing – review and editing. JL: Data curation, Investigation, Methodology, Writing – review and editing. PR: Data curation, Project administration, Writing – review and editing. KR: Investigation, Methodology, Supervision, Writing – review and editing. KP: Conceptualization, Funding acquisition, Investigation, Methodology, Resources, Supervision, Writing – review and editing. SK: Conceptualization, Funding acquisition, Methodology, Resources, Supervision, Writing – review and editing. ZS: Conceptualization, Formal Analysis, Funding acquisition, Investigation, Methodology, Supervision, Validation, Visualization, Writing – review and editing.
The author(s) declare that financial support was received for the research and/ or publication of this article. This work was supported by The Ohio State University President Catalyst Grant to Krishna, Saygin, and Phan.
We would like to acknowledge the support that this work received from the Center for Cognitive and Behavioral Brain Imaging (CCBBI) and Ohio Supercomputer Center (OSC).
The authors declare that the research was conducted in the absence of any commercial or financial relationships that could be construed as a potential conflict of interest.
The authors declare that no Generative AI was used in the creation of this manuscript.
All claims expressed in this article are solely those of the authors and do not necessarily represent those of their affiliated organizations, or those of the publisher, the editors and the reviewers. Any product that may be evaluated in this article, or claim that may be made by its manufacturer, is not guaranteed or endorsed by the publisher.
Badran, B., Ly, M., DeVries, W., Glusman, C., Willis, A., Pridmore, S., et al. (2019). Are EMG and visual observation comparable in determining resting motor threshold? A reexamination after twenty years. Brain Stimul. 12, 364–366. doi: 10.1016/j.brs.2018.11.003
Barrett, D., and Gonzalez-Lima, F. (2013). Transcranial infrared laser stimulation produces beneficial cognitive and emotional effects in humans. Neuroscience 230, 13–23. doi: 10.1016/j.neuroscience.2012.11.016
Behrens, T., Berg, H., Jbabdi, S., Rushworth, M., and Woolrich, M. (2007). Probabilistic diffusion tractography with multiple fibre orientations: What can we gain? Neuroimage 34, 144–155.
Behrens, T., Woolrich, M., Jenkinson, M., Johansen-Berg, H., Nunes, R., Clare, S., et al. (2003). Characterization and propagation of uncertainty in diffusion-weighted MR imaging. Magn. Reson. Med. 50, 1077–1088. doi: 10.1002/mrm.10609
Beynel, L., Powers, J., and Appelbaum, L. (2020). Effects of repetitive transcranial magnetic stimulation on resting-state connectivity: A systematic review. NeuroImage 211:116596.
Boas, D., Culver, J., Stott, J., and Dunn, A. (2002). Three dimensional Monte Carlo code for photon migration through complex heterogeneous media including the adult human head. Opt. Express 10, 159–170. doi: 10.1364/oe.10.000159
Borovac, J. (2016). Side effects of a dopamine agonist therapy for Parkinson’s disease: A mini-review of clinical pharmacology. Yale J. Biol. Med. 89, 37–47.
Bullmore, E., and Sporns, O. (2009). Complex brain networks: Graph theoretical analysis of structural and functional systems. Nat. Rev. Neurosci. 10, 186–198.
Cardoso, F. S., Serra, F. T., Coimbra, N. C., Gonzalez-Lima, F., and Gomes da Silva, S. (2022). Transcranial photobiomodulation changes neuronal morphology in the cerebral cortex of rats. Neurosci. Lett. 781:136681. doi: 10.1016/j.neulet.2022.136681
Chan, A., Lee, T., Yeung, M., and Hamblin, M. (2019). Photobiomodulation improves the frontal cognitive function of older adults. Int. J. Geriatric Psychiatry 34, 369–377.
Chao, L. (2019). Effects of home photobiomodulation treatments on cognitive and behavioral function, cerebral perfusion, and resting-state functional connectivity in patients with dementia: A pilot trial. Photobiomodul. Photomed. Laser Surg. 37, 133–141. doi: 10.1089/photob.2018.4555
Chung, H., Dai, T., Sharma, S., Huang, Y., Carroll, J., and Hamblin, M. (2012). The nuts and bolts of low-level laser (Light) therapy. Ann. Biomed. Eng. 40, 516–533. doi: 10.1007/s10439-011-0454-7
de Kwaasteniet, B., Ruhe, E., Caan, M., Rive, M., Olabarriaga, S., Groefsema, M., et al. (2013). Relation between structural and functional connectivity in major depressive disorder. Biol. Psychiatry 74, 40–47.
Deng, Z., Lisanby, S., and Peterchev, A. (2013). Electric field depth–focality tradeoff in transcranial magnetic stimulation: Simulation comparison of 50 coil designs. Brain Stimul. 6, 1–13.
Desikan, R., Ségonne, F., Fischl, B., Quinn, B., Dickerson, B., Blacker, D., et al. (2006). An automated labeling system for subdividing the human cerebral cortex on MRI scans into gyral based regions of interest. Neuroimage 31, 968–980. doi: 10.1016/j.neuroimage.2006.01.021
Dole, M., Auboiroux, V., Langar, L., and Mitrofanis, J. A. (2023). systematic review of the effects of transcranial photobiomodulation on brain activity in humans. Rev. Neurosci. 34, 671–693. doi: 10.1515/revneuro-2023-0003
Droby, A., Nosatzki, S., Edry, Y., Thaler, A., Giladi, N., Mirelman, A., et al. (2022). The interplay between structural and functional connectivity in early stage Parkinson’s disease patients. J. Neurol. Sci. 442:120452.
Elton, A., Allen, J., Yorke, M., Khan, F., Xu, P., and Boettiger, C. (2023). Sex moderates family history of alcohol use disorder and childhood maltreatment effects on an fMRI stop-signal task. Hum. Brain Mapp. 44, 2436–2450. doi: 10.1002/hbm.26221
Fang, Q., and Yan, S. (2019). Graphics processing unit-accelerated mesh-based Monte Carlo photon transport simulations. J. Biomed. Opt. 24:115002. doi: 10.1117/1.JBO.24.11.115002
Fedorenko, E., Hsieh, P., Nieto-Castañón, A., Whitfield-Gabrieli, S., and Kanwisher, N. (2010). New method for fMRI investigations of language: Defining ROIs functionally in individual subjects. J. Neurophysiol. 104, 1177–1194.
Fekri, A., Jahan, A., Moghadam Salimi, M., and Oskouei, A. (2019). Short-term effects of transcranial near-infrared photobiomodulation on motor performance in healthy human subjects: An experimental singleblind randomized clinical trial. J Lasers Med Sci. 10, 317–323. doi: 10.15171/jlms.2019.51
Ferguson, J. M. (2001). SSRI antidepressant medications: Adverse effects and tolerability. Prim Care Companion J. Clin. Psychiatry 3, 22–27.
Figueiro Longo, M., Tan, C., Chan, S., Welt, J., Avesta, A., Ratai, E., et al. (2020). Effect of transcranial low-level light therapy vs sham therapy among patients with moderate traumatic brain injury. JAMA Netw. Open 3:e2017337. doi: 10.1001/jamanetworkopen.2020.17337
Fitzsimmons, S., Postma, T., Campen, A., Vriend, C., Batelaan, N., Oppen, P., et al. (2024). Transcranial magnetic stimulation–induced plasticity improving cognitive control in obsessive-compulsive disorder, part I: Clinical and neuroimaging outcomes from a randomized. Trial. Biol. Psychiatry Online ahead of print. doi: 10.1016/j.biopsych.2024.06.029
FreeSurfer Wiki (2024a). FreeSurfer FSAverage. Available online at: https://surfer.nmr.mgh.harvard.edu/fswiki/FsAverage (Accessed October 17, 2024).
FreeSurfer Wiki (2024b). FreeSurfer FSFast. Available online at: https://surfer.nmr.mgh.harvard.edu/fswiki/FsFastAnlysisBySteps (Accessed April 24, 2024).
FreeSurfer Wiki (2024c). FreeSurfer Recon-all. Available online at: https://surfer.nmr.mgh.harvard.edu/fswiki/recon-all/ (Accessed May 18, 2024).
Greve, D., and Fischl, B. (2009). Accurate and robust brain image alignment using boundary-based registration. Neuroimage 48, 63–72. doi: 10.1016/j.neuroimage.2009.06.060
Gutiérrez-Menéndez, A., Martínez, J., Méndez, M., and Arias, J. (2022). No effects of photobiomodulation on prefrontal cortex and hippocampal cytochrome C oxidase activity and expression of c-Fos protein of young male and female rats. Front. Neurosci. 16:897225. doi: 10.3389/fnins.2022.897225
Hardwick, R., Lesage, E., and Miall, R. (2014). Cerebellar transcranial magnetic stimulation: The role of coil geometry and tissue depth. Brain Stimul. 7, 643–649. doi: 10.1016/j.brs.2014.04.009
Huang, L., Kao, T., Sung, K., and Abraham, J. (2021). Simulation study on the optimization of photon energy delivered to the prefrontal cortex in low-level-light therapy using red to near-infrared light. IEEE J. Selected Top. Quantum Electronics 27, 1–10.
Jenkinson, M., Beckmann, C., Behrens, T., Woolrich, M., and Smith, S. M. (2012). FSL. Neuroimage 62, 782–790.
Johnstone, D., Hamilton, C., Gordon, L., Moro, C., Torres, N., Nicklason, F., et al. (2021). Exploring the use of intracranial and extracranial (Remote) photobiomodulation devices in Parkinson’s disease: A comparison of direct and indirect systemic stimulations. J. Alzheimers Dis. 83, 1399–1413. doi: 10.3233/JAD-210052
Johnstone, D., Moro, C., Stone, J., Benabid, A., and Mitrofanis, J. (2016). Turning on lights to stop neurodegeneration: The potential of near infrared light therapy in Alzheimer’s and Parkinson’s disease. Front. Neurosci. 9:500. doi: 10.3389/fnins.2015.00500
Kantzanou, M., Korfias, S., Panourias, I., Sakas, D., and Karalexi, M. (2021). Deep brain stimulation-related surgical site infections: A systematic review and meta-analysis. Neuromodulation 24, 197–211. doi: 10.1111/ner.13354
Khedr, E., Ahmed, M., Fathy, N., and Rothwell, J. (2005). Therapeutic trial of repetitive transcranial magnetic stimulation after acute ischemic stroke. Neurology 65, 466–468.
Khoury, H., Mitrofanis, J., and Henderson, L. (2019). Exploring the effects of near infrared light on resting and evoked brain activity in humans using magnetic resonance imaging. Neuroscience 422, 161–171. doi: 10.1016/j.neuroscience.2019.10.037
Kim, Y., and Shin, S. (2014). Comparison of effects of transcranial magnetic stimulation on primary motor cortex and supplementary motor area in motor skill learning (randomized, cross over study). Front. Hum. Neurosci. 8:937. doi: 10.3389/fnhum.2014.00937
Lefaucheur, J. (2019). “Transcranial magnetic stimulation,” in Handbook of Clinical Neurology, eds K. Levin and P. Chauvel (Amsterdam: Elsevier).
Liberg, B., and Rahm, C. (2015). The functional anatomy of psychomotor disturbances in major depressive disorder. Front. Psychiatry 6:34. doi: 10.3389/fpsyt.2015.00034/full
Logothetis, N. (2003). The underpinnings of the BOLD functional magnetic resonance imaging signal. J. Neurosci. 23, 3963–3971.
Oberman, L., Edwards, D., Eldaief, M., and Pascual-Leone, A. (2011). Safety of theta burst transcranial magnetic stimulation: A systematic review of the literature. J. Clin. Neurophysiol. 28, 67–74.
Petersen, K., Van Wouwe, N., Stark, A., Lin, Y., Kang, H., Trujillo-Diaz, P., et al. (2018). Ventral striatal network connectivity reflects reward learning and behavior in patients with Parkinson’s disease. Hum. Brain. Mapp. 39, 509–521. doi: 10.1002/hbm.23860
Poyton, R., and Hendrickson, M. (2016). “Molecular basis for photobiomodulation: Light-induced nitric oxide synthesis by cytochrome c oxidase in low-level laser therapy,” in Handbook of Low-Level Laser Therapy, eds M. R. Hamblin, T. Agrawal, and M. de Sousa (Boca Raton, FL: CRC Press), 201–220.
Reddy, S., Shreekantiah, U., Goyal, N., and Roy, C. (2023). Brain activation alterations with adjunctive deep transcranial magnetic stimulation in obsessive-compulsive disorder: An fMRI study. CNS Spectrums 28, 361–366. doi: 10.1017/S1092852922000803
Saltmarche, A., Naeser, M., Ho, K., Hamblin, M., and Lim, L. (2017). Significant improvement in cognition in mild to moderately severe dementia cases treated with transcranial plus intranasal photobiomodulation: Case series report. Photomed. Laser Surg. 35, 432–441. doi: 10.1089/pho.2016.4227
Sarica, C., Iorio-Morin, C., Aguirre-Padilla, D., Najjar, A., Paff, M., Fomenko, A., et al. (2021). Implantable pulse generators for deep brain stimulation: Challenges, complications, and strategies for practicality and longevity. Front. Hum. Neurosci. 15:708481. doi: 10.3389/fnhum.2021.708481
Saucedo, C., Courtois, E., Wade, Z., Kelley, M., Kheradbin, N., Barrett, D., et al. (2021). Transcranial laser stimulation: Mitochondrial and cerebrovascular effects in younger and older healthy adults. Brain Stimulation 14, 440–449. doi: 10.1016/j.brs.2021.02.011
Schiena, G., Franco, G., Boscutti, A., Delvecchio, G., Maggioni, E., and Brambilla, P. (2021). Connectivity changes in major depressive disorder after rTMS: A review of functional and structural connectivity data. Epidemiol. Psychiatr. Sci. 30:e59. doi: 10.1186/s13063-024-08606-8
Tedford, C., DeLapp, S., Jacques, S., and Anders, J. (2015). Quantitative analysis of transcranial and intraparenchymal light penetration in human cadaver brain tissue. Lasers Surg Med. 47, 312–322.
Urquhart, E., Wanniarachchi, H., Wang, X., Gonzalez-Lima, F., Alexandrakis, G., and Liu, H. (2020). Transcranial photobiomodulation-induced changes in human brain functional connectivity and network metrics mapped by whole-head functional near-infrared spectroscopy in vivo. Biomed. Opt. Express 11, 5783–5799. doi: 10.1364/BOE.402047
Wang, X., Dmochowski, J., Zeng, L., Kallioniemi, E., Husain, M., Gonzalez-Lima, F., et al. (2019). Transcranial photobiomodulation with 1064-nm laser modulates brain electroencephalogram rhythms. Neurophoton 6:025013. doi: 10.1117/1.NPh.6.2.025013
Wang, X., Tian, F., Reddy, D., Nalawade, S., Barrett, D., Gonzalez-Lima, F., et al. (2017). Up-regulation of cerebral cytochrome-c-oxidase and hemodynamics by transcranial infrared laser stimulation: A broadband near-infrared spectroscopy study. J. Cereb. Blood Flow Metab. 37, 3789–3802. doi: 10.1177/0271678X17691783
Warbrick, T., Rosenberg, J., and Shah, N. (2017). The relationship between BOLD fMRI response and the underlying white matter as measured by fractional anisotropy (FA): A systematic review. NeuroImage 153, 369–381. doi: 10.1016/j.neuroimage.2016.12.075
Wassermann, E., Epstein, C., Ziemann, U., Walsh, V., Paus, T., and Lisanby, S. (eds) (2008). “Inter- and intra-individual variation in the response to TMS,” in Oxford Handbook of Transcranial Stimulation, 1st Edn, (Oxford: OUP Oxford), 401–408.
Yuan, Y., Cassano, P., Pias, M., and Fang, Q. (2020). Transcranial photobiomodulation with near-infrared light from childhood to elderliness: Simulation of dosimetry. Neurophotonics 7:015009. doi: 10.1117/1.NPh.7.1.015009
Zhao, C., Li, D., Kong, Y., Liu, H., Hu, Y., Niu, H., et al. (2022). Transcranial photobiomodulation enhances visual working memory capacity in humans. Sci. Adv. 8:eabq3211. doi: 10.1126/sciadv.abq3211
Zomorrodi, R., Loheswaran, G., Pushparaj, A., and Lim, L. (2019). Pulsed near infrared transcranial and intranasal photobiomodulation significantly modulates neural oscillations: A pilot exploratory study. Sci Rep. 9:6309. doi: 10.1038/s41598-019-42693-x
Keywords: photobiomodulation, transcranial magnetic stimulation, functional magnetic resonance imaging, fractional anisotropy, resting-state connectivity
Citation: Bibb SA, Yu EJ, Molloy MF, LaRocco J, Resnick P, Reeves K, Phan KL, Krishna S and Saygin ZM (2025) Pilot study comparing effects of infrared neuromodulation and transcranial magnetic stimulation using magnetic resonance imaging. Front. Hum. Neurosci. 19:1514087. doi: 10.3389/fnhum.2025.1514087
Received: 20 October 2024; Accepted: 24 February 2025;
Published: 13 March 2025.
Edited by:
Julia A. Scott, Santa Clara University, United StatesReviewed by:
Craig David Workman, University of Iowa Hospitals and Clinics, United StatesCopyright © 2025 Bibb, Yu, Molloy, LaRocco, Resnick, Reeves, Phan, Krishna and Saygin. This is an open-access article distributed under the terms of the Creative Commons Attribution License (CC BY). The use, distribution or reproduction in other forums is permitted, provided the original author(s) and the copyright owner(s) are credited and that the original publication in this journal is cited, in accordance with accepted academic practice. No use, distribution or reproduction is permitted which does not comply with these terms.
*Correspondence: Zeynep M. Saygin, c2F5Z2luLjNAb3N1LmVkdQ==; Sophia A. Bibb, c29waGlhLmJpYmJAb3N1bWMuZWR1; Sanjay Krishna, a3Jpc2huYS41M0Bvc3UuZWR1
Disclaimer: All claims expressed in this article are solely those of the authors and do not necessarily represent those of their affiliated organizations, or those of the publisher, the editors and the reviewers. Any product that may be evaluated in this article or claim that may be made by its manufacturer is not guaranteed or endorsed by the publisher.
Research integrity at Frontiers
Learn more about the work of our research integrity team to safeguard the quality of each article we publish.