- 1Department of Neuropsychiatry, Graduate School of Medicine and Pharmaceutical Sciences, University of Toyama, Toyama, Japan
- 2Research Center for Idling Brain Science, University of Toyama, Toyama, Japan
- 3Center for Clinical Training, Toyama University Hospital, Toyama, Japan
- 4Uozu Shinkei Sanatorium, Toyama, Japan
- 5Itoigawa Clinic, Niigata, Japan
- 6Ariwawabashi Hospital, Toyama, Japan
Background and objectives: Several studies have reported on the resting-state electroencephalogram (EEG) power in patients with schizophrenia, with a decrease in α (especially α2) and an increase in δ and β1 power compared with healthy control; however, reports on at-risk mental states (ARMS) are few. In this study, we measured the resting-state EEG power in ARMS, and investigated its features and the relationship between the power of the frequency bands and their diagnostic outcomes.
Methods: Patients with ARMS who were not on any psychotropic medication and met the Comprehensive Assessment of At-Risk Mental State criteria were included. Patients who developed psychotic disorders were labeled as the ARMS-P group, while patients with ARMS who were followed up prospectively for more than 2 years and did not develop psychotic disorders were classified as the ARMS-NP group. EEGs were measured in the resting state, and frequencies were analyzed using standardized low-resolution brain electromagnetic tomography (sLORETA). Seven bands (δ, θ, α1, α2, β1–3) underwent analysis. The sLORETA values (current source density [CSD]) were compared between the ARMS-P and ARMS-NP groups. Clinical symptoms were assessed at the time of EEG measurements using the Positive and Negative Syndrome Scale (PANSS).
Results: Of the 39 patients included (25 males, 14 females, 18.8 ± 4.5 years old), eight developed psychotic disorders (ARMS-P). The ARMS-P group exhibited significantly higher CSD in the β1 power within areas of the left middle frontal gyrus (MFG) compared with the ARMS-NP group (best match: X = −35, Y = 25, Z = 50 [MNI coordinates], Area 8, CSD = 2.33, p < 0.05). There was a significant positive correlation between the β1/α ratio of the CSD at left MFG and the Somatic concern score measured by the PANSS.
Discussion: Increased β1 power was observed in the resting EEG before the onset of psychosis and correlated with a symptom. This suggests that resting EEG power may be a useful marker for predicting future conversion to psychosis and clinical symptoms in patients with ARMS.
1 Introduction
A high-risk psychosis strategy aims to improve early detection of psychosis and provide earlier and more efficient treatment (McGorry et al., 2008; Riecher-Rossler et al., 2006). An at-risk mental state (ARMS) for psychosis defined using semi-structured clinical interviews includes attenuated psychotic symptoms, brief limited psychotic episodes, and genetic risk and social decline (Yung et al., 1998). However, not all patients identified with ARMS using these criteria develop psychosis. A recent meta-analysis reported a transition-to-psychosis rate of only 23% (Salazar de Pablo et al., 2021). Thus, the current criteria result in several false positives. Differentiating between patients with ARMS who will transition to psychosis (ARMS-P) and those who will not develop psychosis (ARMS-NP) is crucial to avoid imposing unnecessary burdens on patients.
Electroencephalography (EEG) is a noninvasive, convenient and economical electrophysiological measurement technique that explores neuronal activity by placing electrodes on the scalp (Schoenberg, 2020). EEG has a high temporal resolution on the same millisecond scale as brain neural activity, enabling accurate acquisition of neural activity and characterization of functional changes during dynamic cerebral activity (Schoenberg, 2020). EEGs are available in large healthcare facilities and are widely used to reveal the etiology of various neuropsychiatric disorders.
Patients with ARMS-P are more severely impaired in certain neurological domains compared to patients with ARMS-NP; therefore, biomarker studies have been performed to identify patients with ARMS-P using several measurement tools. As for EEG, evoked potentials containing event-related potentials, particularly mismatch negativity, have been reported to be able to detect patients with ARMS-P (Bodatsch et al., 2015; Erickson et al., 2016). Traditional resting EEG findings have also been reported because they are easy to measure and important for understanding the functional characteristics of the idling human brain. The EEG raw signal can be decomposed into five oscillatory rhythms or broad frequency bands, namely δ (0.5–4.0 Hz), θ (4–8 Hz), α (8–13 Hz), β (13–30 Hz), and γ (30–100 Hz) bands (Babiloni et al., 2020). Previous studies for the power spectrum in schizophrenia generally reported increased δ (Guntekin and Basar, 2016; Newson and Thiagarajan, 2018) and decreased α (Newson and Thiagarajan, 2018; Murphy and Ongur, 2019; Begre et al., 2003; Pascual-Marqui et al., 1999), while inconsistent findings have been also reported in studies investigating abnormalities of the resting θ, β and γ band power (Newson and Thiagarajan, 2018; Perrottelli et al., 2021; Reilly et al., 2018). Some studies subdivided frequency bands into lower and higher frequency components, namely α1, β2 (Newson and Thiagarajan, 2018). Narrower frequency bands can reduce the risk of offsetting or undetected frequency effects (Klimesch et al., 1997; Li et al., 2020). By this approach, the resting-state EEG in patients with schizophrenia was reported with a decrease in α (especially α2) and increase in β1 power (Vignapiano et al., 2019). As for ARMS, within the authors’ knowledge, four papers have examined whether EEG power predicts future transition to psychosis. Zimmermann et al. (2010) showed difference between ARMS-P and ARMS-NP by combination with negative symptom, the δ, θ, β1, and β2 power, however, they failed that negative symptom or EEG spectral power alone predicted transition to psychosis (Zimmermann et al., 2010). Sollychin et al. (2019) tried to reveal increased frontal slow wave activity and functioning shows any value in the prediction of transition to psychosis, however, there was no difference between the ARMS-P and the ARMS-NP groups, and no change in slow frequency power following transition to psychosis (Sollychin et al., 2019). Ramyead et al. (2016) suggested that current source density (CSD, which will be mentioned later) measurements extracted from clinical resting state EEG can help to improve the prediction of psychosis on a single-subject level by using machine-learning (Ramyead et al., 2016). They also showed ARMS-P patients showed higher γ activity in the medial prefrontal cortex compared to healthy control and ARMS-P patients lagged phase synchronization of β oscillations decreased compared to ARMS-NP and healthy control (Ramyead et al., 2015). Although their suggestions were evocative, the results were varied and further evidence demanded. Thus, it remains elusive whether ARMS patients have abnormal resting-state EEG power and whether, if present, it is associated with their clinical characteristics (e.g., symptom severity, later psychosis onset).
Low-resolution electromagnetic tomography (LORETA) provides three-dimensional images of brain electrical activity, which is the so-called CSD (Pascual-Marqui et al., 1999). The strength of LORETA includes the better representation of a widely distributed or multiple-oriented activity, which is difficult to model with a dipole procedure. It is a simple method and produces less demand on patients than other functional brain measures, such as functional magnetic resonance imaging. EEG recording CSD measures have remarkable advantages over scalp potentials in terms of topographic patterns, strength of activation, and more reliable spatial localization of neuroelectric activity during resting EEG, as well as during cognitive tasks, by elucidating subtle differences across and within several neuropsychiatric conditions, such as schizophrenia (Kamarajan et al., 2015).
When investigating resting EEG, we must consider the weakness of its sensitivity to the effects of psychotropic drugs, such as benzodiazepines and antipsychotics (Mucci et al., 2006). It is important to conduct resting EEG studies in drug-free patients to identify reliable biomarkers.
In this study, we measured the resting-state EEG power using LORETA in psychotropic medication-free patients with ARMS, and investigated its features and the relationship between the power of the frequency bands and their outcomes. The relationship between resting EEG signals, clinical symptoms, and cognitive and social functioning in patients with ARMS was also investigated. We predicted that the frequency bands characteristic reported in schizophrenia would be found in patients with ARMS-P but not in patients with ARMS-NP.
2 Materials and methods
2.1 Participants
Patients with ARMS were recruited from the University of Toyama Hospital or the Toyama Prefectural Mental Health Centre (Mizuno et al., 2009). None of the patients took psychotropic medications, including anti-psychotics, anti-depressant, or benzodiazepines within 2 weeks before the EEG recording. Diagnoses were made by experienced psychiatrists based on the Comprehensive Assessment of At-Risk Mental State (Yung et al., 1996). Psychiatric and treatment histories were collected from the participants, and their families and medical records. Physical examination and standard laboratory tests confirmed that the eligible patients were physically healthy. Exclusion criteria included the following: patients with a history of substance abuse or dependence, seizure, and head injury.
Individuals with ARMS were further subgrouped based on clinical outcomes during the follow-up period as described in previous reports (Fusar-Poli et al., 2012). Conversion to psychosis was defined according to the psychotic disorder criteria in the Comprehensive Assessment of At-Risk Mental State: (i) hallucinations, unusual thoughts, and suspiciousness exceed defined severities, or delusion with strong conviction, or conceptual disorganization exceeds moderate level, (ii) frequency of symptoms is at least several times a week, and (iii) the episode is longer than 1 week (Yung et al., 2005).
Experienced psychiatrists administered the Positive and Negative Syndrome Scale (PANSS) (Kay et al., 1987). The Japanese Adult Reading Test (JART) (Matsuoka et al., 2006) was used to estimate premorbid IQ. The Brief Assessment of Cognition in Schizophrenia (BACS) (Keefe et al., 2004; Kaneda et al., 2007), Schizophrenia Cognition Rating Scale (SCoRS) (Keefe et al., 2006; Kaneda et al., 2011; Higuchi et al., 2017), and modified Global Assessment of Functioning (mGAF) (Hall, 1995) were used to evaluate cognitive and social functions. The BACS score was standardized using z-scores based on the mean scores of healthy Japanese controls (Kaneda et al., 2007).
This study was conducted in accordance with the principles of the Declaration of Helsinki and approved by the Committee on Medical Ethics of Toyama University (no. I2013006) on February 5, 2014. Written informed consent was obtained from all participants after a full explanation of the purpose and procedure of the study was provided. Written consent was obtained from the parents or guardians of participants under 20 years of age.
2.2 EEG recording
EEGs were recorded at baseline, based on previous reports from our laboratory (Higuchi et al., 2008; Sumiyoshi et al., 2009; Miyanishi et al., 2013). Briefly, a 32-channel DC amplifier (EEG-2100 version 2.22 J, Nihon Kohden Corp., Tokyo, Japan) was used according to the international 10–20 system. Recordings were performed using electro cap (Electro cap Inc., Eaton, OH) in a sound-attenuated room. The data were collected at a sampling rate of 500 Hz. All electrodes were referred to as the average amplitude of the ear electrodes (bandwidth, 0.53–120 Hz, 60 Hz notch filter). Electrode impedance was < 10 k-ohm. Patients were assessed using the Epworth Sleepiness Scale (Johns, 1991) and were required to lay on a bed for 5 min with their eyes closed in a silent and dark room during EEG recording. From the resting-EEG, 2-second, 20-epoch EEGs without large artifacts, such as blinking, were extracted manually.
2.3 Standardized LORETA (sLORETA) analysis
The sLORETA images were obtained by estimating the CSD distribution for epochs of brain electrical activity on a dense grid of 6239 voxels at 5-mm spatial resolution applied to the digitized Talairach and Tournoux (1988), based on the established method (Pascual-Marqui et al., 1999). The sLORETA makes use of the three-shell spherical head model registered in the Talairach atlas, which is available as a digitized magnetic resonance imaging from the Brain Imaging Centre, Montreal Neurologic Institute. The registration between spherical and realistic head geometries uses EEG electrode coordinates (Towle et al., 1993). The solution space was restricted to the cortical gray matter and hippocampus, as determined by the corresponding digitized Probability Atlas, which is also available from the Brain Imaging Center.
Cross-spectra of the EEG epochs for each subject in each condition were computed using the sLORETA software in eight frequency bands: δ, 1.5–6.0 Hz; θ, 6.5–8.0 Hz; α1, 8.5–10.0 Hz; α2, 10.5–12.0 Hz; β1, 12.5–18.0 Hz; β2, 18.5–21.0 Hz; and β3, 21.5–30.0 Hz. The sLORETA computed the cortical distribution of the CSD of neuronal oscillations in 6239 voxels from the averaged cross-spectra in each band for each subject under each condition. The CSD data were normalized subject-wise; the CSD at each voxel was normalized with the power density averaged across all frequencies and all 6239 voxels (Babiloni et al., 2007). Subsequently, subtracted CSD were compared between the ARMS-P and -NP groups voxel-by-voxel in each band using the t-statistical non-parametric mapping implemented in sLORETA, and t-values were obtained.
Significant voxels were counted in each brain region as defined by the sLORETA. The results showed that all voxels with significant differences were contained in the left middle frontal gyrus (MFG). With reference to this, we calculated the average CSD containing the following brain regions of interest: significant 3-voxels ([−35, 25, 50], [−35, 25, 45], and [−35, 20, 50]), left MFG, left Brodmann area 8, and left frontal lobe. In the correlation analysis, we used these CSDs divided by the average CSD of the occipital α band, which is the basic rhythm.
2.4 Data analysis
Statistical analyses were performed using the Statistical Package for Social Sciences version 25 (SPSS Japan Inc.) and Jamovi Software version 2.3.18.0.1 Demographic and clinical data (age, JART, PANSS, BACS, SCoRS, and mGAF scores) were assessed using independent t-tests. Age had skewed distributions, and the nonparametric Mann-Whitney U (for two-group comparisons) test was used to compare group differences. Sex was analyzed using the Chi-square test. Comparisons between the ARMS-P and -NP groups in LORETA source imaging were conducted using voxel-by-voxel unpaired t-statistics after logarithmic transformation of the data. The Holmes’ non-parametric correction was applied for multiple comparisons (Holmes et al., 1996). The relationships between CSD and PANSS, BACS, SCoRS, and mGAF were analyzed using Spearman’s rank correlations because CSD had skewed distributions. The Benjamini–Hochberg false discovery rate procedure was used for post-hoc analysis (Benjamini et al., 2001). Significance was set at a value of p-value of less than 0.05 (two-tailed) for CSD analysis and false discovery rate adjusted p-value (= q) for correlation analysis.
3 Results
3.1 Patient demographics
The demographic and clinical data of the participants at baseline are shown in Table 1. Briefly, this study included participants with an average age of 18.8 ± 4.5 years old, 64% male and estimated premorbid IQs measured by the JART were over 70. During the follow-up period of the 39 ARMS patients, eight (20.5%) developed psychosis (ARMS-P) with the definitive diagnoses of schizophrenia (n = 7) and depression with psychotic symptoms (n = 1). Thirty-one patients did not develop psychosis during the 2-year follow-up period and were classified as ARMS-NP. Age, sex ratios, estimated premorbid IQ measured by the JART, and PANSS scores did not differ significantly between the ARMS-P and -NP groups. Regarding cognitive function, the ARMS-P indicated over one standard deviation lower on a BACS composite score. The impairment of BACS in the ARMS-NP group was milder, and the difference from the ARMS-P group was at the trend level (p = 0.06). The SCoRS score was high in the current dataset, particularly for the ARMS-P group, and there was a significant difference between the ARMS-P and -NP groups (p = 0.006). Social functioning, measured using the mGAF, was approximately 40 points, and no significant differences were found between the groups.
3.2 Brain CSD activity
The sLORETA images for each frequency band are shown in Figure 1. The average CSDs of the ARMS-P and -NP groups for each voxel are plotted in Figure 2. The mean CSDs of the ARMS-P group seem larger in the α2 (especially in the caudal area) and β1 (rostral area) bands. On the other hand, the CSDs of the α1 band in ARMS-P looks smaller especially in the caudal area. Table 2 shows the brain areas with the five largest differences in CSD for each frequency band. Statistical analysis revealed that the ARMS-P group had significantly higher CSDs for β1 at 3 regions within the left MFG compared to the ARMS-NP group. In Supplementary Figure 1, the t-values of each voxel calculated using the sLORETA software and significant voxels (indicated by arrows) are plotted.
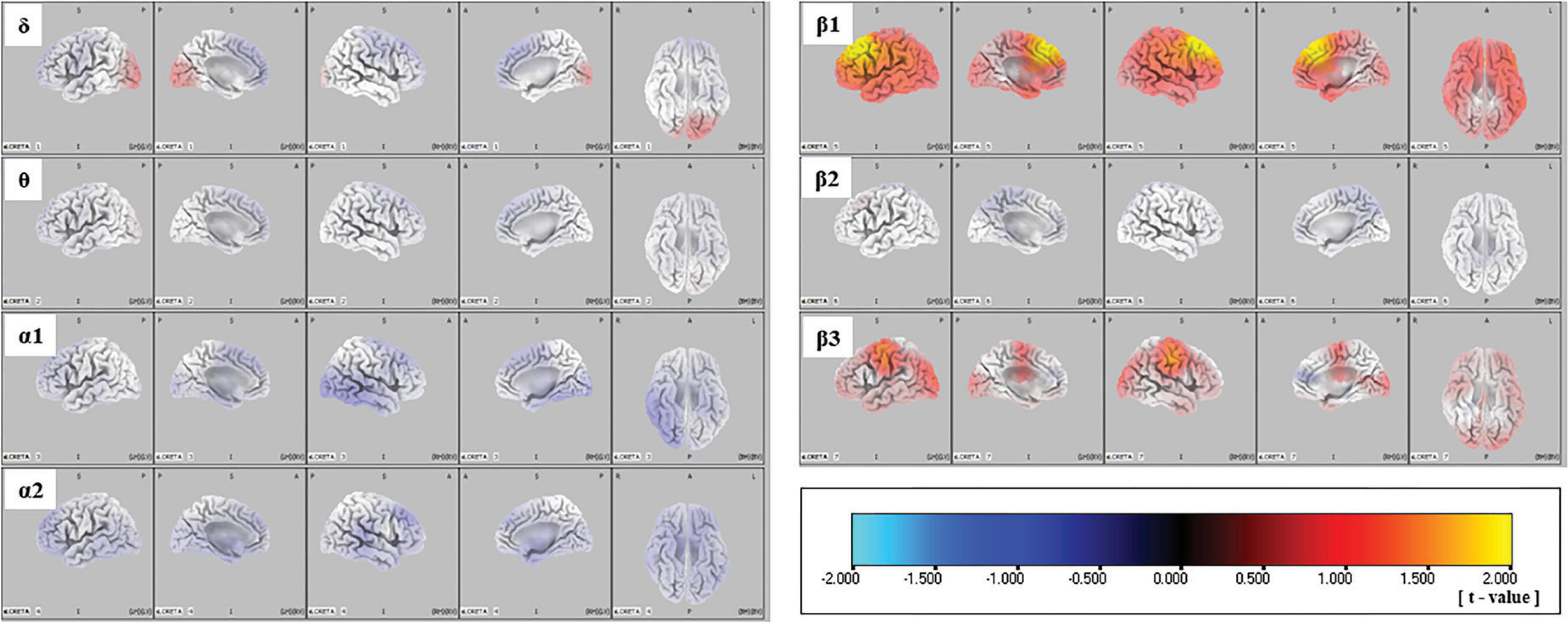
Figure 1. Comparison of source imaging in the ARMS-P and -NP groups, as revealed by SnPM voxel-wise sLORETA comparisons of independent samples. Negative (i.e., lower in ARMS-P group) to positive (i.e., higher in ARMS-P group) t-values are represented by cyan-blue-black-red-yellow. ARMS-NP, at-risk mental state non-psychosis; ARMS-P, at-risk mental state psychosis; sLORETA, standardized low-resolution brain electromagnetic tomography; SnPM, statistical non-parametric mapping.
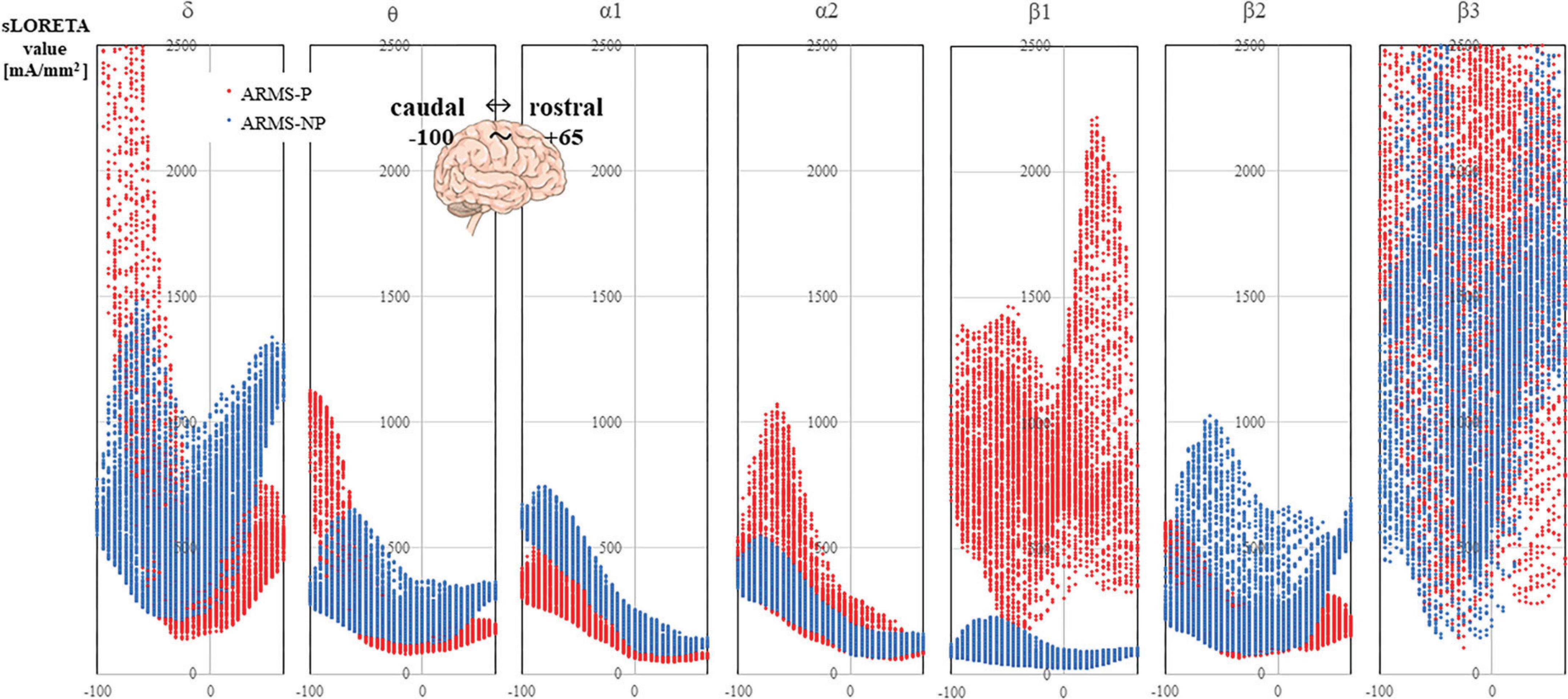
Figure 2. The CSDs of the ARMS-P (red marker) and ARMS-NP (blue marker) groups are plotted. The X-axis of each graph represents the Y-axis of the MNI coordinate, which ranged from –100 to +65. The negative direction is caudal and the positive direction is rostral. ARMS-NP, at-risk mental state non-psychosis; ARMS-P, at-risk mental state psychosis; CSD, current source density; MNI, Montreal Neurological Institute.
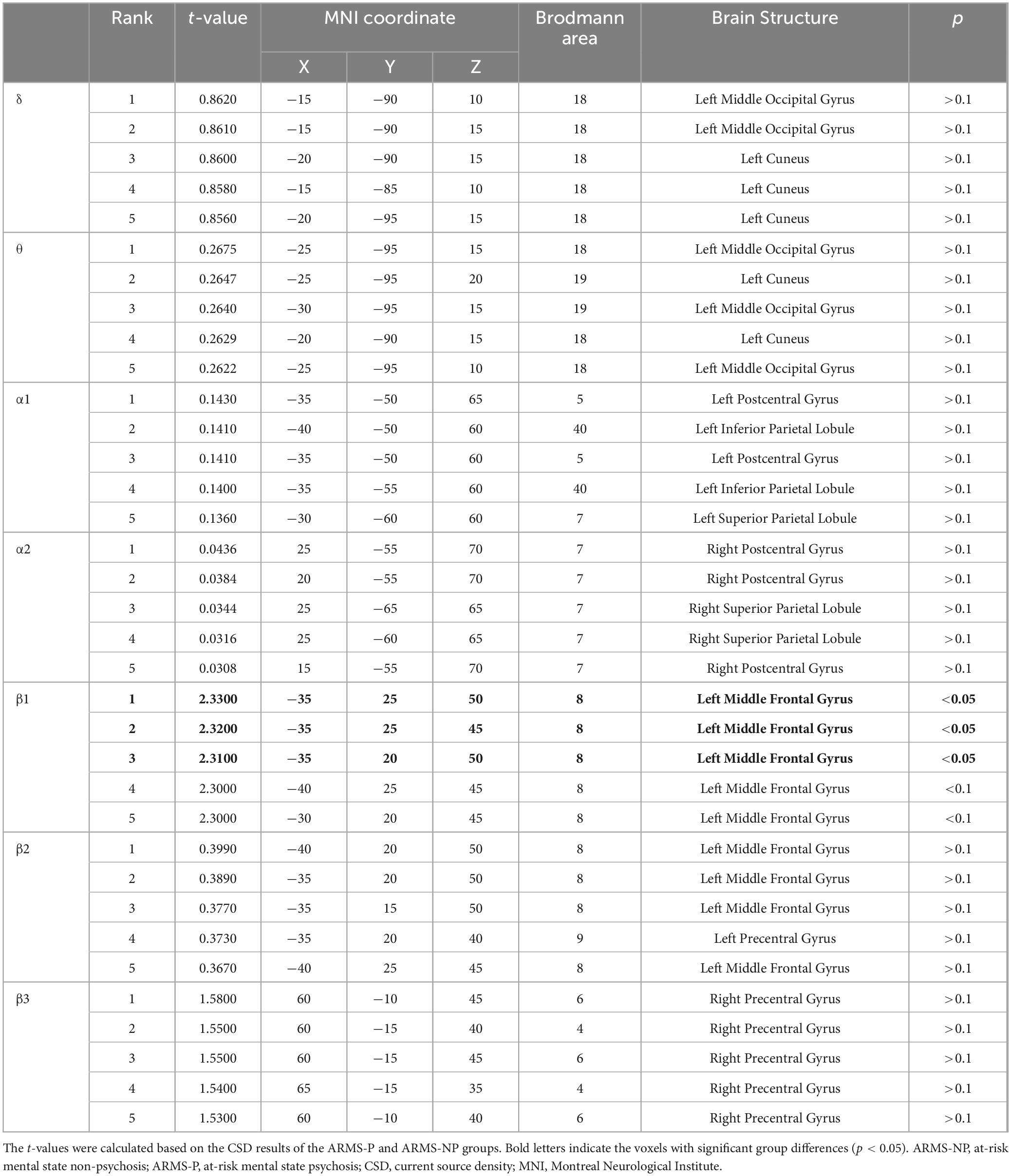
Table 2. MNI coordinates for brain areas showing the largest CSD differences (top five) between the ARMS-P and -NP groups.
3.3 Relationships between psychiatric or cognitive symptoms and CSD activity
Table 3 shows the relationships between the β1/α ratio of the CSD at the left MFG and PANSS in patients with ARMS (n = 39). Statistical analyses by Spearman’s rank correlation revealed that the CSD significantly and positively correlated with the PANSS G1 score (General Psychopathology; G1 = Somatic concern). It was with moderate to large effect sizes (rho = 0.50, R2 = 0.25, p = 0.0017, q = 0.027). When analyzed separately for ARMS-P and ARMS-NP, only ARMS-NP correlated with G1 (rho = 0.53, R2 = 0.28, p = 0.0023, q = 0.037), and it was also a moderate to large effect size. We also investigated the correlations between the cognitive and social functioning of patients and the β1/α ratio of the CSD at the left MFG, but the results showed no significant correlations (Supplementary Table 1). In the other regions of interest, the β1/α CSD values did not relate to clinical data (data not shown).
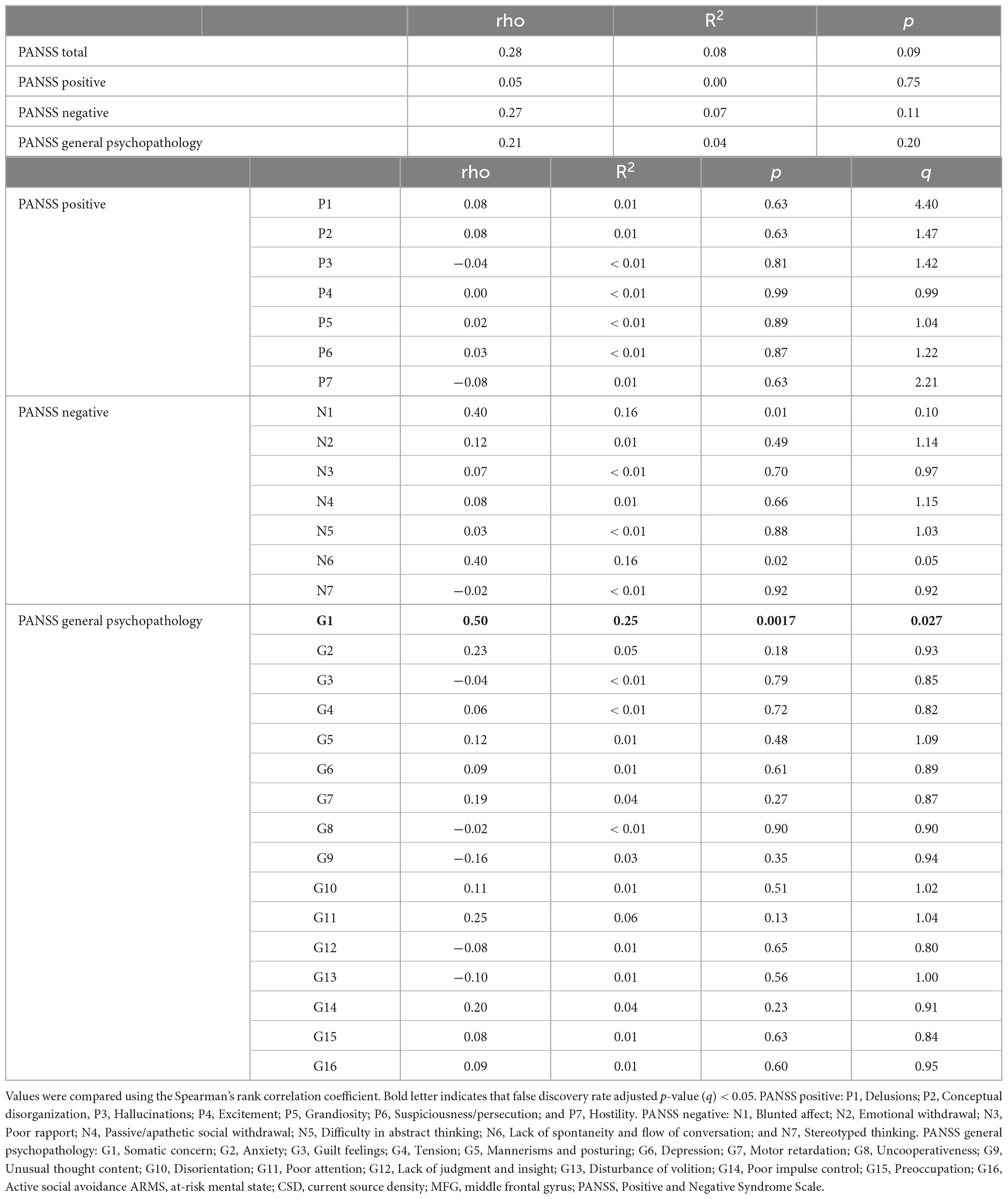
Table 3. Relationships between the β/α ratio of the CSD at the left MFG and PANSS in patients with ARMS.
4 Discussion
Our current resting-EEG investigation revealed that the ARMS-P group had a significantly higher β1 power within areas of the left MFG compared with the ARMS-NP group. The advantage of our study was a simple and well understandable finding that β1 power alone predicts the transition to psychosis. This finding suggests that the features of the resting EEG that are impaired during psychosis exist prior to the onset of psychosis as a trait marker. The CSD of the left MFG was positively correlated with psychiatric symptoms, suggesting a relationship between the clinical phenotype and underlying neuropsychological mechanisms. These findings suggest that the resting EEG power may be a useful marker for predicting future diagnoses and clinical symptoms in patients with ARMS.
As for schizophrenia, abnormalities of β power manifest as increased activity in the resting-state EEG (Venables et al., 2009; Narayanan et al., 2014; Garakh et al., 2015). However, some studies found no differences in the resting β power between patients with schizophrenia and controls (Renaldi et al., 2019; Harris et al., 2006; Yeragani et al., 2006; Clementz et al., 1994). To the best of our knowledge, no study has reported that resting β power is reduced in schizophrenia. The β oscillation is thought to be associated with sensorimotor functions (Hari and Salmelin, 1997; Pfurtscheller and Lopes da Silva, 1999), the vigilant and excited state of the brain (Li et al., 2020; Pfurtscheller and Lopes da Silva, 1999; Al-Ezzi et al., 2020), and cognitive processes, such as the working memory and top-down regulation of attention (Brinkman et al., 2014; Spitzer and Haegens, 2017; Engel et al., 2001; Miskovic et al., 2010). Some studies found correlations between the β power and clinical symptoms, such as worse negative symptoms (Zimmermann et al., 2010; Gschwandtner et al., 2009) and decreased insight (Arikan et al., 2018). The findings of increased β power in the ARMS-P group in the present study are consistent with findings in previous studies of schizophrenia, suggesting that they share the same abnormalities in brain function, which were detected in schizophrenia, compared with the ARMS-NP group. In the current study, a somatic concern measured by PANSS positively correlated with the CSD of the β1-band. The somatic concern itself does not differ between ARMS-P and ARMS-NP (data not shown), and this finding might relate to biological background as a clinical high-risk condition for psychiatric disorders rather than a trait of psychosis. Somatic concerns refer to experiences of somatic symptoms that may be caused by an underlying somatic background, but can also occur in patients with anxiety disorder, depression, stress-related disorder, or psychosomatic symptoms (Tolmunen et al., 2014; Sikharulidze et al., 2017). The frontal lobe has functions such as motor function, thinking, decision-making, emotional control, memory retention, behavioral control, in particular the MFG is known to be involved in reorienting attention(Shulman et al., 2009; Japee et al., 2015). Also, the β band activity, particularly in lower β activity range (β1), is described to link to active cognitive engagement and vigilance, indicating heightened alertness and mental effort during problem-solving and decision-making tasks (Ray and Cole, 1985). ARMS subjects with high β1 power at MFG might have abnormal switching of attention to the somatic concern. Recently, a report indicated that patients with anxiety disorder exhibited a significant increase in the β rhythm and decrease in the α1 rhythm of the power spectral density (Shen et al., 2022). According to that study, although the α1 rhythms also had statistically significant differences in the power spectral density analysis, the diagnostic classification accuracy of the α1 rhythms (74%) was lower compared to the β rhythms (96%). That finding corresponded with the present study, which showed that β abnormality was more severe than α abnormality and a correlation existed between a high β/α ratio and severe anxiety-related symptoms. One possible explanation for this finding may be increased worry or internal cognitive processing of anxiety in the form of divergent, negatively biased mind wandering during nonspecific information processing (Schoenberg, 2020). Thus, significant changes in the rhythm might help explain the neural mechanism of ARMS and provide basic theoretical support for biomarker identification. Compared with previous studies discussing the differences between ARMS-P and ARMS-NP, our study partially agreed with the study by Zimmermann et al. (2010) who found differences in β1 power, within combination of other frequency bands (Zimmermann et al., 2010), and with the study of Sollychin et al. (2019) who found no difference in the slow wave activity (Sollychin et al., 2019). Ramyead et al. (2015), employed LORETA for quantitative EEG similar to us, also found differences in β oscillations for phase synchronization (Ramyead et al., 2015). Thus, the β-power might be a key frequency in predicting psychotic transitions in ARMS, as some studies found findings related to the β band as we showed. However, the consistent results were partial, and the four previous studies performed comparison in only a small number of ARMS-Ps (n = 7–23), suggesting that more detailed studies with more big data accumulation were needed.
In Figure 2, the mean CSDs of the ARMS-P group seemed larger in the α2 but smaller in the α1 compared with the ARMS-NP group. However, we failed to detect significant group difference related to α activity, probably due to the small sample size; hence, further investigation is needed. The α amplitude in the posterior electrodes increases in relaxed situations when the eyes are closed (Treder et al., 2011) and is thought to reflect an idling state of the brain (Bazanova and Vernon, 2014). This notion may be in line with increased β power, which reflects the vigilant and excited state of the brain (Li et al., 2020; Pfurtscheller and Lopes da Silva, 1999; Al-Ezzi et al., 2020), in patients with ARMS-P. It is well-known that the amplitude of the α frequency band is related to the synchrony of the underlying neuro-electrical source and a reduction in α wave amplitude is often labeled as desynchronization (Pfurtscheller and Lopes da Silva, 1999). Hence, the amplitude of the α rhythm is an index of cortical inactivity that is thought to be, in part, generated by the thalamus (Bazanova and Vernon, 2014). As for schizophrenia, many studies have shown that a reduction in α, particularly of α2, is thought to reflect reduced brain function (Galderisi et al., 1994; Sponheim et al., 1994; Boutros et al., 2008; Begic et al., 2011). A finding of negative correlation between α1 spectral amplitude and the disorganization factor measured by PANSS in schizophrenia (Vignapiano et al., 2019) may also support such mechanism.
While there is consensus on the EEG power spectrum findings in schizophrenia, there are also some contradictory findings. This is probably due to the heterogeneity of schizophrenia, which includes interindividual variability that has not yet been sufficiently resolved (Strik et al., 2017). Moreover, in contrast to this study, some research have revealed that resting EEG power did not differ between ARMS-P and -NP (Zimmermann et al., 2010; Lavoie et al., 2012; Ranlund et al., 2014). There are possible reasons why the findings in the ARMS-P group in this study were not consistent with these previous ARMS findings, such as high heterogeneity of ARMS-P individuals, rather mild EEG abnormalities in ARMS than in established schizophrenia (Bazanova and Vernon, 2014), and potential influence of antipsychotic and other medications (Treder et al., 2011). Nevertheless, a report by Zimmermann et al. (Brinkman et al., 2014) might partly support our findings in showing that resting-state EEG could be predictive of psychosis onset in combination with negative symptoms.
A major limitation of this study was the lack of healthy comparison subjects, where we used the ARMS-NP group as a control group for the ARMS-P group. Further, the number of participants was relatively small, which may have limited the generalizability and statistical power of the results. Finally, the ARMS-P group included a patient who was later definitively diagnosed with depression; this may increase the heterogeneity of our ARMS-P group.
In conclusion, the present study suggested the ability of β1 power to predict the development of psychosis in vulnerable subjects. Our observation also confirmed that the β/α ratio was associated with clinical symptoms related to physical concern. For a biomarker to be actionable, it must be clinically predictive at the individual level and viable in the clinical setting (Abi-Dargham et al., 2023). Therefore, it is important that findings that can be used as biomarkers were detected in the resting EEG, which can be easily measured.
Data availability statement
The raw data supporting the conclusions of this article will be made available by the authors, without undue reservation.
Ethics statement
The studies involving humans were approved by the Committee on Medical Ethics of the University of Toyama. The studies were conducted in accordance with the local legislation and institutional requirements. Written informed consent for participation in this study was provided by the participants’ legal guardians/next of kin.
Author contributions
YH: Writing – review and editing, Writing – original draft, Visualization, Validation, Software, Resources, Project administration, Methodology, Investigation, Funding acquisition, Formal analysis, Data curation, Conceptualization. SO: Writing – review and editing, Visualization, Software, Project administration, Investigation, Formal analysis, Data curation. TaT: Writing – review and editing, Supervision, Project administration, Investigation, Data curation. MS: Writing – review and editing, Supervision, Resources, Project administration, Methodology, Investigation, Funding acquisition, Conceptualization. TsT: Writing – review and editing, Writing – original draft, Validation, Supervision, Resources, Project administration, Methodology, Investigation, Funding acquisition, Data curation, Conceptualization.
Funding
The author(s) declare financial support was received for the research, authorship, and/or publication of this article. This study was supported by the Japan Society for the Promotion of Science KAKENHI (grant numbers 18K07550, 26461739, 20H03598, 22K07554, 23K07031, and 21K11284). This study was also supported by a grant from SENSHIN Medical Research Foundation. The funding sources were not involved in the study design, data collection, data analyses, interpretation of results, writing of the report, or decision to submit the article for publication.
Acknowledgments
We would like to thank all participants involved in this study. We appreciate Shimako Nishiyama, Yuko Mizukami and Yukiko Akasaki for the clinical assessment and supporting the subjects. We also thank Suguru Nakajima for EEG recording. We would like to thank Editage (www.editage.com) for the English language editing.
Conflict of interest
The authors declare that the research was conducted in the absence of any commercial or financial relationships that could be construed as a potential conflict of interest.
Publisher’s note
All claims expressed in this article are solely those of the authors and do not necessarily represent those of their affiliated organizations, or those of the publisher, the editors and the reviewers. Any product that may be evaluated in this article, or claim that may be made by its manufacturer, is not guaranteed or endorsed by the publisher.
Supplementary material
The Supplementary Material for this article can be found online at: https://www.frontiersin.org/articles/10.3389/fnhum.2024.1449820/full#supplementary-material
Abbreviations
ARMS, At-risk mental state; BACS, Brief Assessment of Cognition in Schizophrenia; CSD, Current source density; EEG, Electroencephalography; JART, The Japanese Adult Reading Test; MFG, Middle frontal gyrus; LORETA, Low-resolution electromagnetic tomography; mGAF, Modified Global Assessment of Functioning; PANSS, Positive and Negative Syndrome Scale; SCoRS, Schizophrenia Cognition Rating Scale sLORETA, Standardized low-resolution electromagnetic tomography.
Footnotes
References
Abi-Dargham, A., Moeller, S., Ali, F., DeLorenzo, C., Domschke, K., Horga, G., et al. (2023). Candidate biomarkers in psychiatric disorders: State of the field. World Psychiatry 22, 236–262. doi: 10.1002/wps.21078
Al-Ezzi, A., Kamel, N., Faye, I., and Gunaseli, E. (2020). Review of EEG, ERP, and brain connectivity estimators as predictive biomarkers of social anxiety disorder. Front. Psychol. 11:730. doi: 10.3389/fpsyg.2020.00730
Arikan, M., Metin, B., Metin, S., Tulay, E., and Tarhan, N. (2018). High frequencies in QEEG are related to the level of insight in patients with schizophrenia. Clin. EEG Neurosci. 49, 316–320. doi: 10.1177/1550059418785489
Babiloni, C., Barry, R., Basar, E., Blinowska, K., Cichocki, A., Drinkenburg, W., et al. (2020). International federation of clinical neurophysiology (IFCN) - EEG research workgroup: Recommendations on frequency and topographic analysis of resting state EEG rhythms. Part 1: Applications in clinical research studies. Clin. Neurophysiol. 131, 285–307. doi: 10.1016/j.clinph.2019.06.234
Babiloni, C., Squitti, R., Del Percio, C., Cassetta, E., Ventriglia, M., Ferreri, F., et al. (2007). Free copper and resting temporal EEG rhythms correlate across healthy, mild cognitive impairment, and Alzheimer’s disease subjects. Clin. Neurophysiol. 118, 1244–1260. doi: 10.1016/j.clinph.2007.03.016
Bazanova, O., and Vernon, D. (2014). Interpreting EEG alpha activity. Neurosci. Biobehav. Rev. 44, 94–110. doi: 10.1016/j.neubiorev.2013.05.007
Begic, D., Popovic-Knapic, V., Grubisin, J., Kosanovic-Rajacic, B., Filipcic, I., Telarovic, I., et al. (2011). Quantitative electroencephalography in schizophrenia and depression. Psychiatr. Danub. 23, 355–362.
Begre, S., Federspiel, A., Kiefer, C., Schroth, G., Dierks, T., and Strik, W. (2003). Reduced hippocampal anisotropy related to anteriorization of alpha EEG in schizophrenia. Neuroreport 14, 739–742. doi: 10.1097/00001756-200304150-00016
Benjamini, Y., Drai, D., Elmer, G., Kafkafi, N., and Golani, I. (2001). Controlling the false discovery rate in behavior genetics research. Behav. Brain Res. 125, 279–284. doi: 10.1016/s0166-4328(01)00297-2
Bodatsch, M., Brockhaus-Dumke, A., Klosterkotter, J., and Ruhrmann, S. (2015). Forecasting psychosis by event-related potentials-systematic review and specific meta-analysis. Biol. Psychiatry 77, 951–958. doi: 10.1016/j.biopsych.2014.09.025
Boutros, N., Arfken, C., Galderisi, S., Warrick, J., Pratt, G., and Iacono, W. (2008). The status of spectral EEG abnormality as a diagnostic test for schizophrenia. Schizophr. Res. 99, 225–237. doi: 10.1016/j.schres.2007.11.020
Brinkman, L., Stolk, A., Dijkerman, H., de Lange, F., and Toni, I. (2014). Distinct roles for alpha- and beta-band oscillations during mental simulation of goal-directed actions. J. Neurosci. 34, 14783–14792. doi: 10.1523/JNEUROSCI.2039-14.2014
Clementz, B., Sponheim, S., Iacono, W., and Beiser, M. (1994). Resting EEG in first-episode schizophrenia patients, bipolar psychosis patients, and their first-degree relatives. Psychophysiology 31, 486–494. doi: 10.1111/j.1469-8986.1994.tb01052.x
Engel, A., Fries, P., and Singer, W. (2001). Dynamic predictions: Oscillations and synchrony in top-down processing. Nat. Rev. Neurosci. 2, 704–716. doi: 10.1038/35094565
Erickson, M., Ruffle, A., and Gold, J. M. (2016). A meta-analysis of mismatch negativity in schizophrenia: From clinical risk to disease specificity and progression. Biol. Psychiatry 79, 980–987. doi: 10.1016/j.biopsych.2015.08.025
Fusar-Poli, P., Bonoldi, I., Yung, A., Borgwardt, S., Kempton, M., Valmaggia, L., et al. (2012). Predicting psychosis: Meta-analysis of transition outcomes in individuals at high clinical risk. Arch. Gen. Psychiatry 69, 220–229. doi: 10.1001/archgenpsychiatry.2011.1472
Galderisi, S., Maj, M., Mucci, A., Bucci, P., and Kemali, D. (1994). QEEG alpha 1 changes after a single dose of high-potency neuroleptics as a predictor of short-term response to treatment in schizophrenic patients. Biol. Psychiatry 35, 367–374. doi: 10.1016/0006-3223(94)90002-7
Garakh, Z., Zaytseva, Y., Kapranova, A., Fiala, O., Horacek, J., Shmukler, A., et al. (2015). EEG correlates of a mental arithmetic task in patients with first episode schizophrenia and schizoaffective disorder. Clin. Neurophysiol. 126, 2090–2098. doi: 10.1016/j.clinph.2014.12.031
Gschwandtner, U., Zimmermann, R., Pflueger, M., Riecher-Rossler, A., and Fuhr, P. (2009). Negative symptoms in neuroleptic-naive patients with first-episode psychosis correlate with QEEG parameters. Schizophr. Res. 115, 231–236. doi: 10.1016/j.schres.2009.06.013
Guntekin, B., and Basar, E. (2016). Review of evoked and event-related delta responses in the human brain. Int. J. Psychophysiol. 103, 43–52. doi: 10.1016/j.ijpsycho.2015.02.001
Hall, R. (1995). Global assessment of functioning. A modified scale. Psychosomatics 36, 267–275. doi: 10.1016/S0033-3182(95)71666-8
Hari, R., and Salmelin, R. (1997). Human cortical oscillations: A neuromagnetic view through the skull. Trends Neurosci. 20, 44–49. doi: 10.1016/S0166-2236(96)10065-5
Harris, A., Melkonian, D., Williams, L., and Gordon, E. (2006). Dynamic spectral analysis findings in first episode and chronic schizophrenia. Int. J. Neurosci. 116, 223–246. doi: 10.1080/00207450500402977
Higuchi, Y., Sumiyoshi, T., Kawasaki, Y., Matsui, M., Arai, H., and Kurachi, M. (2008). Electrophysiological basis for the ability of olanzapine to improve verbal memory and functional outcome in patients with schizophrenia: A LORETA analysis of P300. Schizophr. Res. 101, 320–330. doi: 10.1016/j.schres.2008.01.020
Higuchi, Y., Sumiyoshi, T., Seo, T., Suga, M., Takahashi, T., Nishiyama, S., et al. (2017). Associations between daily living skills, cognition, and real-world functioning across stages of schizophrenia; a study with the Schizophrenia Cognition Rating Scale Japanese version. Schizophr. Res. Cogn. 7, 13–18. doi: 10.1016/j.scog.2017.01.001
Holmes, A., Blair, R., Watson, J., and Ford, I. (1996). Nonparametric analysis of statistic images from functional mapping experiments. J. Cereb. Blood Flow Metab. 16, 7–22. doi: 10.1097/00004647-199601000-00002
Japee, S., Holiday, K., Satyshur, M., Mukai, I., and Ungerleider, L. G. A. (2015). role of right middle frontal gyrus in reorienting of attention: A case study. Front. Syst. Neurosci. 9:23. doi: 10.3389/fnsys.2015.00023
Johns, M. W. (1991). A new method for measuring daytime sleepiness: The Epworth sleepiness scale. Sleep 14, 540–545. doi: 10.1093/sleep/14.6.540
Kamarajan, C., Pandey, A., Chorlian, D., and Porjesz, B. (2015). The use of current source density as electrophysiological correlates in neuropsychiatric disorders: A review of human studies. Int. J. Psychophysiol. 97, 310–322. doi: 10.1016/j.ijpsycho.2014.10.013
Kaneda, Y., Sumiyoshi, T., Keefe, R., Ishimoto, Y., Numata, S., and Ohmori, T. (2007). Brief assessment of cognition in schizophrenia: Validation of the Japanese version. Psychiatry Clin. Neurosci. 61, 602–609. doi: 10.1111/j.1440-1819.2007.01725.x
Kaneda, Y., Ueoka, Y., Sumiyoshi, T., Yasui-Furukori, N., Ito, T., Higuchi, Y., et al. (2011). [Schizophrenia cognition rating scale Japanese version (SCoRS-J) as a co-primary measure assessing cognitive function in schizophrenia]. Nihon Shinkei Seishin Yakurigaku Zasshi 31, 259–262.
Kay, S., Fiszbein, A., and Opler, L. (1987). The positive and negative syndrome scale (PANSS) for schizophrenia. Schizophr. Bull. 13, 261–276.
Keefe, R., Goldberg, T., Harvey, P., Gold, J., Poe, M., and Coughenour, L. (2004). The brief assessment of cognition in schizophrenia: Reliability, sensitivity, and comparison with a standard neurocognitive battery. Schizophr. Res. 68, 283–297. doi: 10.1016/j.schres.2003.09.011
Keefe, R., Poe, M., Walker, T., Kang, J., and Harvey, P. (2006). The schizophrenia cognition rating scale: An interview-based assessment and its relationship to cognition, real-world functioning, and functional capacity. Am. J. Psychiatry 163, 426–432. doi: 10.1176/appi.ajp.163.3.426
Klimesch, W., Doppelmayr, M., Pachinger, T., and Ripper, B. (1997). Brain oscillations and human memory: EEG correlates in the upper alpha and theta band. Neurosci. Lett. 238, 9–12. doi: 10.1016/s0304-3940(97)00771-4
Lavoie, S., Schafer, M., Whitford, T., Benninger, F., Feucht, M., Klier, C., et al. (2012). Frontal delta power associated with negative symptoms in ultra-high risk individuals who transitioned to psychosis. Schizophr. Res. 138, 206–211. doi: 10.1016/j.schres.2012.03.033
Li, G., Huang, S., Xu, W., Jiao, W., Jiang, Y., Gao, Z., et al. (2020). The impact of mental fatigue on brain activity: A comparative study both in resting state and task state using EEG. BMC Neurosci. 21:20. doi: 10.1186/s12868-020-00569-1
Matsuoka, K., Uno, M., Kasai, K., Koyama, K., and Kim, Y. (2006). Estimation of premorbid IQ in individuals with Alzheimer’s disease using Japanese ideographic script (Kanji) compound words: Japanese version of national adult reading test. Psychiatry Clin. Neurosci. 60, 332–339. doi: 10.1111/j.1440-1819.2006.01510.x
McGorry, P., Killackey, E., and Yung, A. (2008). Early intervention in psychosis: Concepts, evidence and future directions. World Psychiatry 7, 148–156. doi: 10.1002/j.2051-5545.2008.tb00182.x
Miskovic, V., Ashbaugh, A., Santesso, D., McCabe, R., Antony, M., and Schmidt, L. (2010). Frontal brain oscillations and social anxiety: A cross-frequency spectral analysis during baseline and speech anticipation. Biol. Psychol. 83, 125–132. doi: 10.1016/j.biopsycho.2009.11.010
Miyanishi, T., Sumiyoshi, T., Higuchi, Y., Seo, T., and Suzuki, M. (2013). LORETA current source density for duration mismatch negativity and neuropsychological assessment in early schizophrenia. PLoS One 8:e61152. doi: 10.1371/journal.pone.0061152
Mizuno, M., Suzuki, M., Matsumoto, K., Murakami, M., Takeshi, K., Miyakoshi, T., et al. (2009). Clinical practice and research activities for early psychiatric intervention at Japanese leading centres. Early Interv. Psychiatry 3, 5–9. doi: 10.1111/j.1751-7893.2008.00104.x
Mucci, A., Volpe, U., Merlotti, E., Bucci, P., and Galderisi, S. (2006). Pharmaco-EEG in psychiatry. Clin. EEG Neurosci. 37, 81–98. doi: 10.1177/155005940603700206
Murphy, M., and Ongur, D. (2019). Decreased peak alpha frequency and impaired visual evoked potentials in first episode psychosis. Neuroimage Clin. 22:101693. doi: 10.1016/j.nicl.2019.101693
Narayanan, B., O’Neil, K., Berwise, C., Stevens, M., Calhoun, V., Clementz, B., et al. (2014). Resting state electroencephalogram oscillatory abnormalities in schizophrenia and psychotic bipolar patients and their relatives from the bipolar and schizophrenia network on intermediate phenotypes study. Biol. Psychiatry 76, 456–465. doi: 10.1016/j.biopsych.2013.12.008
Newson, J., and Thiagarajan, T. C. (2018). EEG frequency bands in psychiatric disorders: A review of resting state studies. Front. Hum. Neurosci. 12:521. doi: 10.3389/fnhum.2018.00521
Pascual-Marqui, R., Lehmann, D., Koenig, T., Kochi, K., Merlo, M., Hell, D., et al. (1999). Low resolution brain electromagnetic tomography (LORETA) functional imaging in acute, neuroleptic-naive, first-episode, productive schizophrenia. Psychiatry Res. 90, 169–179. doi: 10.1016/s0925-4927(99)00013-x
Perrottelli, A., Giordano, G., Brando, F., Giuliani, L., and Mucci, A. (2021). EEG-based measures in at-risk mental state and early stages of schizophrenia: A systematic review. Front. Psychiatry 12:653642. doi: 10.3389/fpsyt.2021.653642
Pfurtscheller, G., and Lopes da Silva, F. H. (1999). Event-related EEG/MEG synchronization and desynchronization: Basic principles. Clin. Neurophysiol. 110, 1842–1857. doi: 10.1016/s1388-2457(99)00141-8
Ramyead, A., Kometer, M., Studerus, E., Koranyi, S., Ittig, S., Gschwandtner, U., et al. (2015). Aberrant current source-density and lagged phase synchronization of neural oscillations as markers for emerging psychosis. Schizophr. Bull. 41, 919–929. doi: 10.1093/schbul/sbu134
Ramyead, A., Studerus, E., Kometer, M., Uttinger, M., Gschwandtner, U., Fuhr, P., et al. (2016). Prediction of psychosis using neural oscillations and machine learning in neuroleptic-naive at-risk patients. World J. Biol. Psychiatry 17, 285–295. doi: 10.3109/15622975.2015.1083614
Ranlund, S., Nottage, J., Shaikh, M., Dutt, A., Constante, M., Walshe, M., et al. (2014). Resting EEG in psychosis and at-risk populations–a possible endophenotype? Schizophr. Res. 153, 96–102. doi: 10.1016/j.schres.2013.12.017
Ray, W., and Cole, H. W. (1985). EEG alpha activity reflects attentional demands, and beta activity reflects emotional and cognitive processes. Science 228, 750–752. doi: 10.1126/science.3992243
Reilly, T., Nottage, J., Studerus, E., Rutigliano, G., Micheli, A., Fusar-Poli, P., et al. (2018). Gamma band oscillations in the early phase of psychosis: A systematic review. Neurosci. Biobehav. Rev. 90, 381–399. doi: 10.1016/j.neubiorev.2018.04.006
Renaldi, R., Kim, M., Lee, T., Kwak, Y., Tanra, A., and Kwon, J. (2019). Predicting symptomatic and functional improvements over 1 year in patients with first-episode psychosis using resting-state electroencephalography. Psychiatry Investig. 16, 695–703. doi: 10.30773/pi.2019.06.20.1
Riecher-Rossler, A., Gschwandtner, U., Borgwardt, S., Aston, J., Pfluger, M., and Rossler, W. (2006). Early detection and treatment of schizophrenia: How early? Acta Psychiatr. Scand. Suppl. 429, 73–80. doi: 10.1111/j.1600-0447.2005.00722.x
Salazar de Pablo, G., Radua, J., Pereira, J., Bonoldi, I., Arienti, V., Besana, F., et al. (2021). Probability of transition to psychosis in individuals at clinical high risk: An updated meta-analysis. JAMA Psychiatry 78, 970–978. doi: 10.1001/jamapsychiatry.2021.0830
Schoenberg, P. (2020). Linear and nonlinear EEG-based functional networks in anxiety disorders. Adv. Exp. Med. Biol. 1191, 35–59. doi: 10.1007/978-981-32-9705-0_3
Shen, Z., Li, G., Fang, J., Zhong, H., Wang, J., Sun, Y., et al. (2022). Aberrated multidimensional EEG characteristics in patients with generalized anxiety disorder: A machine-learning based analysis framework. Sensors (Basel) 22:5420. doi: 10.3390/s22145420
Shulman, G., Astafiev, S., Franke, D., Pope, D., Snyder, A., McAvoy, M., et al. (2009). Interaction of stimulus-driven reorienting and expectation in ventral and dorsal frontoparietal and basal ganglia-cortical networks. J. Neurosci. 29, 4392–4407. doi: 10.1523/JNEUROSCI.5609-08.2009
Sikharulidze, G., van Geloven, N., Lelashvili, E., Kalandarishvili, G., Gugushvili, N., and Vermetten, E. (2017). Posttraumatic stress disorder and somatic complaints in a deployed cohort of georgian military personnel: Mediating effect of depression and anxiety. J. Trauma Stress 30, 626–634. doi: 10.1002/jts.22235
Sollychin, M., Jack, B., Polari, A., Ando, A., Amminger, G., Markulev, C., et al. (2019). Frontal slow wave resting EEG power is higher in individuals at ultra high risk for psychosis than in healthy controls but is not associated with negative symptoms or functioning. Schizophr. Res. 208, 293–299. doi: 10.1016/j.schres.2019.01.039
Spitzer, B., and Haegens, S. (2017). Beyond the status quo: A role for beta oscillations in endogenous content (Re)activation. eNeuro 4:e0170–17. doi: 10.1523/ENEURO.0170-17
Sponheim, S., Clementz, B., Iacono, W., and Beiser, M. (1994). Resting EEG in first-episode and chronic schizophrenia. Psychophysiology 31, 37–43. doi: 10.1111/j.1469-8986.1994.tb01023.x
Strik, W., Stegmayer, K., Walther, S., and Dierks, T. (2017). Systems neuroscience of psychosis: Mapping schizophrenia symptoms onto brain systems. Neuropsychobiology 75, 100–116. doi: 10.1159/000485221
Sumiyoshi, T., Higuchi, Y., Itoh, T., Matsui, M., Arai, H., Suzuki, M., et al. (2009). Effect of perospirone on P300 electrophysiological activity and social cognition in schizophrenia: A three-dimensional analysis with sloreta. Psychiatry Res. 172, 180–183. doi: 10.1016/j.pscychresns.2008.07.005
Talairach, J., and Tournoux, P. (1988). Co-planar stereotaxic atlas of the human brain thieme. New York.
Tolmunen, T., Lehto, S., Julkunen, J., Hintikka, J., and Kauhanen, J. (2014). Trait anxiety and somatic concerns associate with increased mortality risk: A 23-year follow-up in aging men. Ann. Epidemiol. 24, 463–468. doi: 10.1016/j.annepidem.2014.03.001
Towle, V., Bolanos, J., Suarez, D., Tan, K., Grzeszczuk, R., Levin, D., et al. (1993). The spatial location of EEG electrodes: Locating the best-fitting sphere relative to cortical anatomy. Electroencephalogr. Clin. Neurophysiol. 86, 1–6. doi: 10.1016/0013-4694(93)90061-y
Treder, M., Bahramisharif, A., Schmidt, N., van Gerven, M., and Blankertz, B. (2011). Brain-computer interfacing using modulations of alpha activity induced by covert shifts of attention. J. Neuroeng. Rehabil. 8:24. doi: 10.1186/1743-0003-8-24
Venables, N., Bernat, E., and Sponheim, S. (2009). Genetic and disorder-specific aspects of resting state EEG abnormalities in schizophrenia. Schizophr. Bull. 35, 826–839. doi: 10.1093/schbul/sbn021
Vignapiano, A., Koenig, T., Mucci, A., Giordano, G., Amodio, A., Altamura, M., et al. (2019). Disorganization and cognitive impairment in schizophrenia: New insights from electrophysiological findings. Int. J. Psychophysiol. 145, 99–108. doi: 10.1016/j.ijpsycho.2019.03.008
Yeragani, V., Cashmere, D., Miewald, J., Tancer, M., and Keshavan, M. (2006). Decreased coherence in higher frequency ranges (beta and gamma) between central and frontal EEG in patients with schizophrenia: A preliminary report. Psychiatry Res. 141, 53–60. doi: 10.1016/j.psychres.2005.07.016
Yung, A., McGorry, P., McFarlane, C., Jackson, H., Patton, G., and Rakkar, A. (1996). Monitoring and care of young people at incipient risk of psychosis. Schizophr. Bull. 22, 283–303. doi: 10.1093/schbul/22.2.283
Yung, A., Phillips, L., McGorry, P., McFarlane, C., Francey, S., Harrigan, S., et al. (1998). Prediction of psychosis. A step towards indicated prevention of schizophrenia. Br. J. Psychiatry Suppl. 172, 14–20.
Yung, A., Yuen, H., McGorry, P., Phillips, L., Kelly, D., Dell’Olio, M., et al. (2005). Mapping the onset of psychosis: The comprehensive assessment of at-risk mental states. Aust. N. Z. J. Psychiatry 39, 964–971. doi: 10.1111/j.1440-1614.2005.01714.x
Keywords: schizophrenia, at-risk mental state, clinical high risk, resting state electroencephalogram, power spectrum, biomarker, low-resolution brain electromagnetic tomography, positive and negative syndrome scale
Citation: Higuchi Y, Odagiri S, Tateno T, Suzuki M and Takahashi T (2024) Resting-state electroencephalogram in drug-free subjects with at-risk mental states who later developed psychosis: a low-resolution electromagnetic tomography analysis. Front. Hum. Neurosci. 18:1449820. doi: 10.3389/fnhum.2024.1449820
Received: 16 June 2024; Accepted: 09 August 2024;
Published: 27 August 2024.
Edited by:
Kaoru Takakusaki, Asahikawa Medical University, JapanReviewed by:
Masafumi Yoshimura, Kansai Medical University, JapanShunichiro Ikeda, Kansai Medical University, Japan
Copyright © 2024 Higuchi, Odagiri, Tateno, Suzuki and Takahashi. This is an open-access article distributed under the terms of the Creative Commons Attribution License (CC BY). The use, distribution or reproduction in other forums is permitted, provided the original author(s) and the copyright owner(s) are credited and that the original publication in this journal is cited, in accordance with accepted academic practice. No use, distribution or reproduction is permitted which does not comply with these terms.
*Correspondence: Yuko Higuchi, yhiguchi@med.u-toyama.ac.jp