- 1SEMA Lab, Psychology Department, Center for Consciousness Studies, University of Arizona, Tucson, AZ, United States
- 2Sanmai Technologies, PBC, Sunnyvale, CA, United States
- 3X, the Moonshot Factory, Mountain View, CA, United States
Background: Transcranial focused ultrasound (TFUS) is an emerging neuromodulation tool for temporarily altering brain activity and probing network functioning. The effects of TFUS on the default mode network (DMN) are unknown.
Objective: The study examined the effects of transcranial focused ultrasound (TFUS) on the functional connectivity of the default mode network (DMN), specifically by targeting the posterior cingulate cortex (PCC). Additionally, we investigated the subjective effects of TFUS on mood, mindfulness, and self-related processing.
Methods: The study employed a randomized, single-blind design involving 30 healthy subjects. Participants were randomly assigned to either the active TFUS group or the sham TFUS group. Resting-state functional magnetic resonance imaging (rs-fMRI) scans were conducted before and after the TFUS application. To measure subjective effects, the Toronto Mindfulness Scale, the Visual Analog Mood Scale, and the Amsterdam Resting State Questionnaire were administered at baseline and 30 min after sonication. The Self Scale and an unstructured interview were also administered 30 min after sonication.
Results: The active TFUS group exhibited significant reductions in functional connectivity along the midline of the DMN, while the sham TFUS group showed no changes. The active TFUS group demonstrated increased state mindfulness, reduced Global Vigor, and temporary alterations in the sense of ego, sense of time, and recollection of memories. The sham TFUS group showed an increase in state mindfulness, too, with no other subjective effects.
Conclusions: TFUS targeted at the PCC can alter DMN connectivity and cause changes in subjective experience. These findings support the potential of TFUS to serve both as a research tool and as a potential therapeutic intervention.
1 Introduction
The default mode network (DMN) has been the subject of extensive research since it was initially defined by Raichle et al. (2001). The DMN is anchored by two midline nodes at the medial prefrontal cortex (mPFC) and the posterior cingulate cortex (PCC) with adjacent bilateral nodes in the angular gyri and middle temporal gyri (Andrews-Hanna et al., 2010). While theoretical ideas and debate about the function of the DMN continue to evolve (Andrews-Hanna et al., 2014; Yeshurun et al., 2021), it appears to play an essential role in the inner processes of mind-wandering, planning, and self-related processing (Raichle, 2015).
The DMN has been implicated in several disorders, including depression (Scalabrini et al., 2020; Zhou et al., 2020; Ju et al., 2022), addiction (Zhang and Volkow, 2019), autism (Padmanabhan et al., 2017), ADHD (Posner et al., 2014; Harikumar et al., 2021), and schizophrenia (Hu et al., 2017). Collectively, the clinical literature suggests that a breakdown in the regulation of the DMN may be one mechanism underlying these disorders (e.g., abnormal DMN activity leading to increased rumination in depression) (Lydon-Staley et al., 2019; Zhou et al., 2023). Thus, potential treatments that normalize DMN function may be called for (Holtzheimer and Mayberg, 2011; Scalabrini et al., 2020).
Empirical research with psychedelics has implicated the DMN in constructing varying states of consciousness and representations of the self, especially the “narrative self,” in which the self is the object of thought (Nour and Carhart-Harris, 2017). The current scientific consensus establishes that disruption of resting state functional connectivity within the DMN is a central mechanism that drives their profound psychological and therapeutic effects (Carhart-Harris et al., 2012; Gattuso et al., 2022). Carhart-Harris et al. (2012) found that the degree to which psilocybin decreased cerebral blood flow and connectivity of the mPFC and PCC predicted the magnitude of the self-altering effects.
The practice of meditation can also alter resting-state brain activity, including the DMN. Brewer et al. (2013) found reduced DMN connectivity in experienced meditators during meditation in the MRI scanner. The same group demonstrated that meditators could volitionally reduce activity in their PCC using real-time fMRI neurofeedback, and that this reduction correlated with their internal meditative experience (Garrison et al., 2013).
Neurophenomenological analysis suggested that PCC deactivation was associated with an experience of “undistracted awareness” and “effortless doing” (Garrison et al., 2013). This led Brewer and colleagues (Brewer et al., 2013; Brewer and Garrison, 2014) to suggest that PCC activity plays a role in self-referential processing, particularly a tendency to “get caught up in” one’s experience. In the mindfulness literature, this quality may also be described as a lack of equanimity (Desbordes et al., 2015). Importantly, mindfulness training, as demonstrated by Korponay et al. (2019), does not simply reduce DMN activity but rather enhances one’s ability to control and inhibit it when necessary.
The extant fMRI literature in healthy individuals, clinical studies, psychedelics, and contemplative experiments all converge on the major role of the DMN in internal thought and self-related processing (Andrews-Hanna, 2012; Andrews-Hanna et al., 2014; Davey et al., 2016; Nour and Carhart-Harris, 2017; Carhart-Harris and Friston, 2019; Davey and Harrison, 2022). Non-invasive brain stimulation techniques that enable targeted manipulation of DMN regions would offer an opportunity to estimate the causal relationship between DMN activity and internal processes, paving the way to potential effective therapeutics.
A promising method for non-invasive brain modulation is transcranial focused ultrasound (TFUS). TFUS modulates brain regions with pulsed beams of focused ultrasound with millimeter precision (Blackmore et al., 2019). Unlike other non-invasive brain stimulation techniques like transcranial electrical stimulation (TES) or transcranial magnetic stimulation (TMS), TFUS can effectively reach deep subcortical regions like the thalamus (Legon et al., 2018; Kim et al., 2023) by adjusting the focal depth of the ultrasound beam. The safety profile of TFUS is favorable: the current picture is that adverse events only occur when stimulation is too long and/or intense, far in excess of FDA safety limits, causing thermal and/or biophysical damage to the targeted tissue or unintentional opening of the blood–brain barrier (Pasquinelli et al., 2019).
TFUS acts through a combination of potential mechanisms, including thermal, mechanical, and cavitation effects as a result of the acoustic energy interacting with neural tissue (Dell’Italia et al., 2022). Reviews suggest that it can be both excitatory and inhibitory in its effects (Zhang et al., 2021), but there is not always a clear differentiation between the two, as some studies show both excitatory and inhibitory effects simultaneously (Verhagen et al., 2019; Yoon et al., 2019), while others show state-dependent (Yang et al., 2021) or cell type-dependent (Wattiez et al., 2017) responses. Given that similar effects can arise from bidirectional mechanisms (e.g., inhibition of excitatory neurons or stimulation of inhibitory neurons can both produce suppression), it is more accurate to describe the neuromodulatory effects at the tissue-level. Network (Folloni et al., 2019) and distal (Cain et al., 2021) effects can also manifest, as can delayed, offline effects (Carhart-Harris et al., 2012). Sanguinetti et al. (2020) found that targeting the right prefrontal cortex induced mood enhancement and decreased functional connectivity in regions distal from the target.
Given these capabilities, TFUS is an ideal candidate for non-invasively modulating the DMN. The aim of this pilot study was to target the PCC using TFUS parameters that are expected to suppress neural firing (Dell’Italia et al., 2022), specifically by utilizing a low duty cycle value of 5.26%. The hypothesis was that this approach would enable modulation of the resting state connectivity from that node to the rest of the network. We also hypothesized that this would induce changes in phenomenology that relate to DMN activity, specifically mindfulness and self-referential processing. As a proof-of-concept, this would pave the way for TFUS to serve as a tool to probe network functioning and be used as a therapeutic intervention.
2 Materials and methods
2.1 Subjects
Thirty healthy subjects (18 female, average age 19.8 years) participated in this study. Exclusion criteria were: use of tobacco/nicotine, history of head injury, uncorrected hearing or vision impairment, history of brain or mental illness (including drug and/or alcohol dependence), use of pharmaceuticals (including psychotropic drugs), sleep disorders, drug or alcohol intoxication, history of epilepsy, history of migraines, metal implants in their head, and history of cardiac problems. Inclusion criteria were: age 18–77, normal or corrected vision, and proficient enough in English to read the consent form. Subjects received either actual or sham TFUS in a single-blind, between-subjects design.
2.2 Procedure
After screening and consent, subjects were given subjective rating scales. Subsequently, they underwent four MRI scans: T1 structural, baseline functional resting state (rs-BOLD), arterial spin labeling (rs-pcASL), and susceptibility weighted imaging (SWI). During functional scans, subjects were instructed to stare at a fixation cross and allow their thoughts to flow naturally. Subjects were removed from the scanner, and real-time neuronavigation (Visor2, ANT Neuro, Netherlands) was used to apply either active or sham TFUS to their ventral PCC based on their individual structural MRI. Sham TFUS was performed by holding an unplugged transducer against their head. Subjects then returned to the MRI scanner for further measurements. Functional MRI scans were captured in the 5 min immediately after application (t1) and at 25 min after application (t2) (see Figure 1). Post-sonication SWI and pcASL scans were also taken after t1. Upon exiting the scanner, final subjective rating scales were taken, and the subject was debriefed.
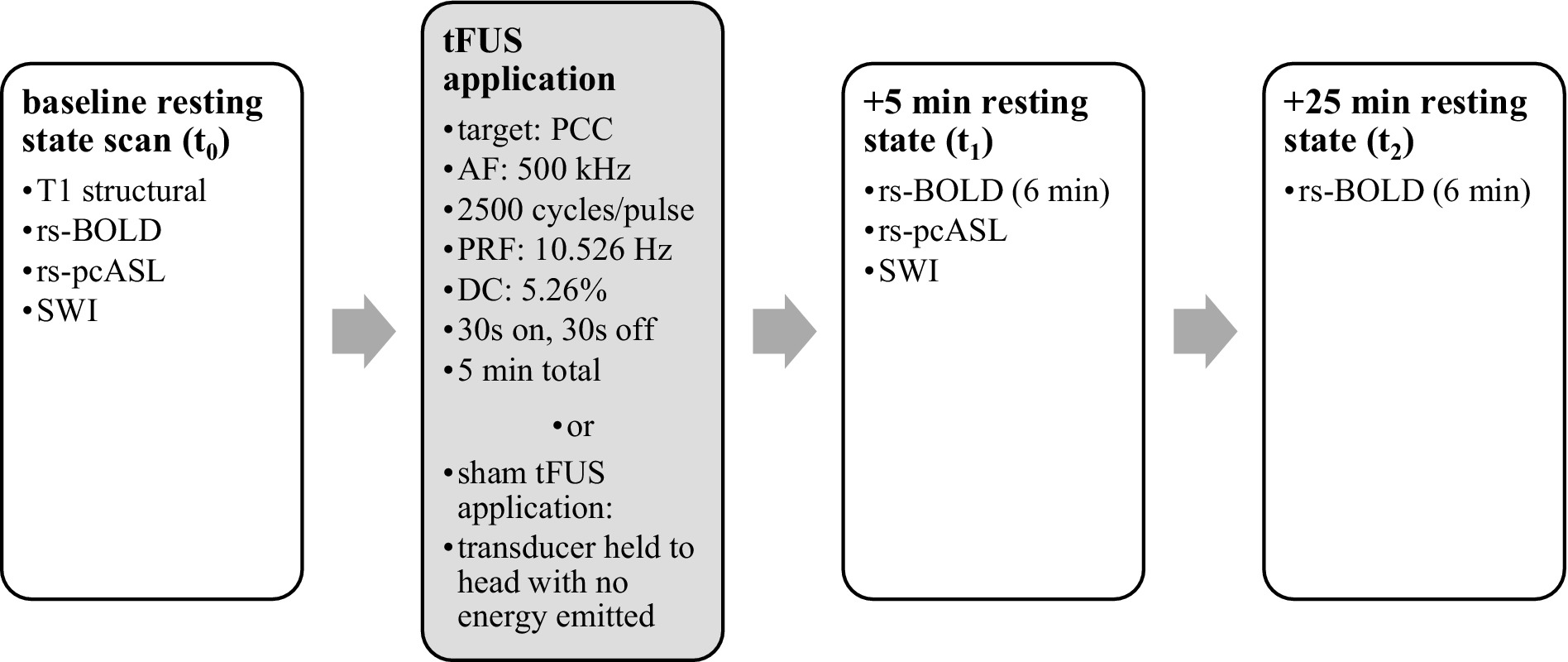
Figure 1. Timeline of MRI data acquisition and TFUS application. Data were gathered before and after TFUS application.
2.3 Subjective ratings
Before any MRI scans, subjects responded to the Visual Analog Mood Scale (VAMS) (Luria, 1975) and the Toronto Mindfulness Scale (TMS) (Lau et al., 2006). After TFUS and all subsequent MRI scans, they responded to the same scales again, along with the Self Scale (Lebedev et al., 2015) and the Amsterdam Resting-State Questionnaire (ARSQ) (Diaz et al., 2013).
2.4 Post experiment questions
Structured post-experiment questions were asked of each participant. Participants were asked to guess if they were in the “stimulation or placebo condition,” whether the ultrasound changed their “overall mental state,” if they heard anything from the transducer, and if they had changes to their “inner talk- or thinking-space.”
2.5 MRI scans
Functional BOLD images were acquired on a Siemens Skyra 3-Tesla scanner using EPI gradient echo sequence (TR = 1800 ms; TE = 25 ms; flip angle = 90; FOV = 192 mm; acquisition voxel size 3 mm × 3 mm × 3 mm). T1-weighted anatomical images were also acquired for neuronavigation and registration of the functional scans (MP-RAGE; TR = 2,500 ms; TE = 4.35 ms; TI = 900 ms; flip angle = 8; FOV = 256 mm).
2.6 Acoustic intensity measurements
Acoustic intensity was measured using a custom-built water tank setup. Data were recorded using a needle hydrophone (HNR-0500; Onda, Sunnyvale, CA, United States) with a geometric diameter of 2.5 mm. A scan volume of 12 mm (x), 12 mm (y), 68 mm (axial) was collected using 0.508 mm steps in degassed water. Pressure and intensity were calculated from the voltage recordings from the hydrophone. MI was calculated with a derated peak pressure using the attenuation coefficient of soft tissue (0.3 dB/cm) (Abbott, 1999). The measured output of this wave in degassed free water shows a peak negative pressure of 0.422 MPa. The output of the beam through a hydrated sample of cadaver parietal bone showed a peak negative pressure of 0.130 MPa (a 69.3% decrease). See Figure 2. Full properties of the wave output are presented in Table 1. The skull caused little deviation of the focus in the lateral plane, but in the axial plane focus became more shallow (axial focus water = 56.6 mm; skull = 51.7 mm; difference = −4.8 mm). See Table 2. The periodic variation in peak intensity in the axial plane (panel C of Figure 2) is thought to be due to standing waves created by the annular geometry of the transducer.
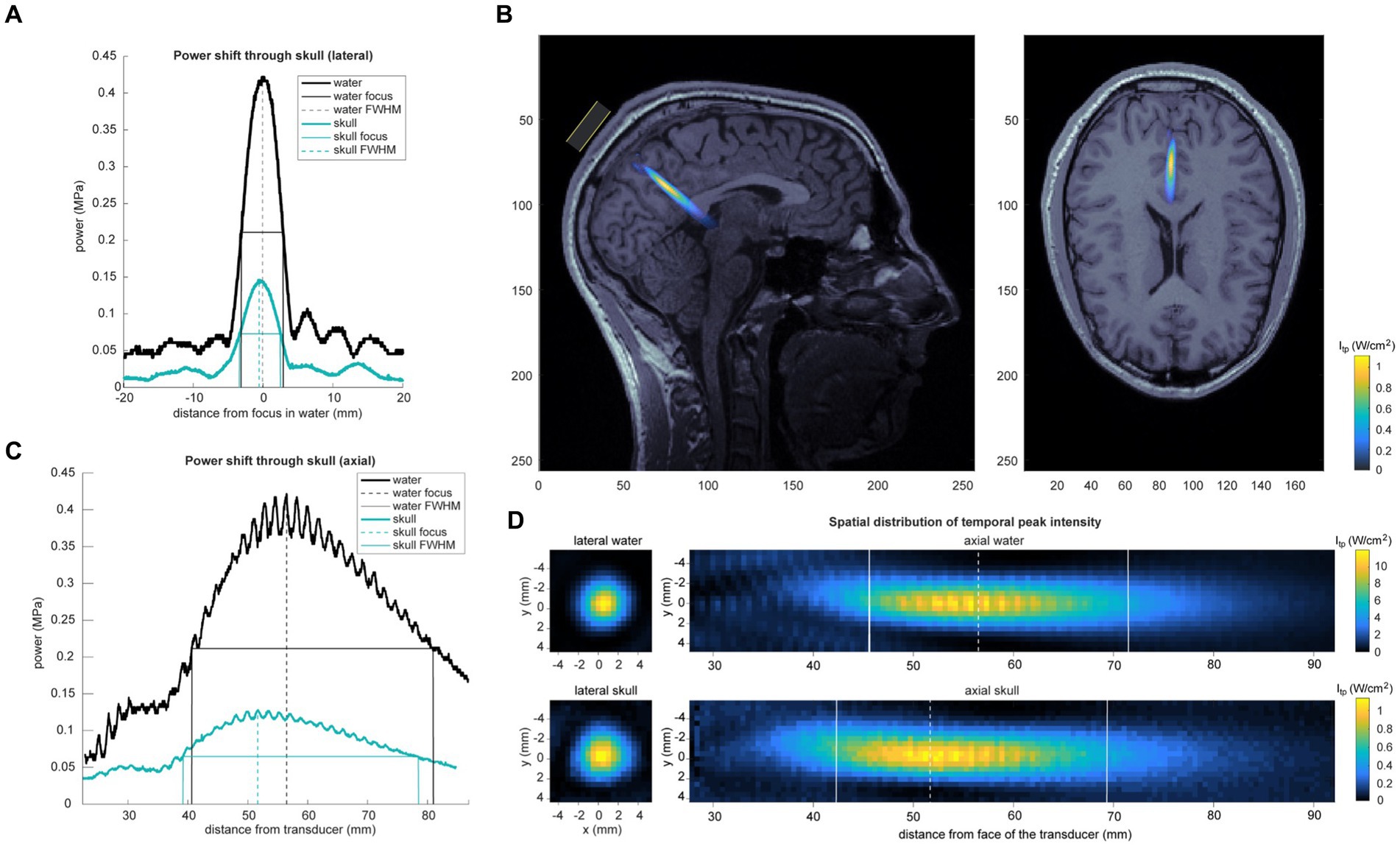
Figure 2. Acoustic intensity measurements. (A) Skull attenuation and geometric deformation of acoustic temporal peak pressure recorded in water and through a sample of cadaver parietal bone (“skull”). Center of the beam and FWHM are displayed. Lateral shift displayed in (A) and axial shift in (C). The periodic variation in peak intensity in the axial plane in panel C is thought to be due to standing waves created by the annular geometry of the transducer. (B) Skull-attenuated ultrasound intensity map is overlaid on a single subject’s MRI. Estimation of peak focus was determined using recorded neuronavigation coordinates from that subject’s TFUS session. (D) Spatial distribution of temporal peak intensity of the ultrasound beam in water (top) and through a cadaver parietal skull piece (bottom). Left panel shows lateral spatial topography of temporal peak intensity of the beam at the axial peak; right panel displays axial topography.
2.7 TFUS stimulation
Subjects were seated comfortably. An MRI-guided stereotactic system (Visor2, ANT Neuro, Netherlands) was used to guide TFUS targeting to the participant’s PCC. The focused ultrasound was delivered by a custom 4-channel ring transducer (Sonic Concepts, Bothell, WA, United States) with an outer diameter of 64 mm that uses a sealed membrane filled with degassed water for coupling, which is then housed inside a custom 3D-printed casing. The transducer was driven by an acoustic amplifier (TPO-203, Sonic Concepts, Bothell, WA, USA), with the ultrasound beam having the following parameters: acoustic frequency (AF) = 500 kHz, pulse repetition frequency (PRF) = 10.526 Hz, pulse repetition period (PRP) = 95 ms, pulse duration (PD) = 5 ms or 2,500 cycles, duty cycle = 5.26%. Badran and colleagues used similar parameters to suppress the pain pathway, except they used a 650 kHz acoustic frequency (Badran et al., 2020; Li et al., 2021).
The beam was focused to fixed distance of 55 mm, corresponding to the average distance of the PCC from the surface of the scalp where the transducer is applied. The target was determined by inspection of each subject’s anatomical scan. The ventral PCC was chosen due to its greater association with internal directed thought rather than the cognitive control functions associated with the dorsal PCC (Leech and Sharp, 2014).
The subject’s head was registered to their structural MRI in the Visor2 neuronavigation software using three fiducials (nasion and ears) and their scalp surface. The transducer was held firmly against the subject’s head, using individual MR-guided neuronavigation, with gel applied to the scalp, to deliver 30-s stimulus intervals followed by 30-s rest periods. The pattern of [30s ON, 30s OFF] was repeated five times consecutively, totaling a 5-min duration. Participants were instructed to sit quietly with their eyes open. The researcher periodically (about every 1.5 min) asked them how they were doing, if they felt anything unusual, and if they would like to continue.
2.8 MRI analysis
Results included in this manuscript come from analyses performed using CONN (Whitfield-Gabrieli and Nieto-Castanon, 2012) (RRID:SCR_009550) release 21.a (Nieto-Castanon and Whitfield-Gabrieli, 2021) and SPM (Penny et al., 2011) (RRID:SCR_007037) release 12.7771.
2.8.1 Preprocessing
Functional and anatomical data were preprocessed using a flexible preprocessing pipeline (Nieto-Castanon, 2020a) including realignment with correction of susceptibility distortion interactions, slice timing correction, outlier detection, direct segmentation and MNI-space normalization, and smoothing. Functional data were realigned using SPM realign & unwarp procedure (Andersson et al., 2001), where all scans were coregistered to a reference image (first scan of the first session) using a least squares approach and a 6 parameter (rigid body) transformation (Friston et al., 1995), and resampled using b-spline interpolation to correct for motion and magnetic susceptibility interactions. Temporal misalignment between different slices of the functional data (acquired in interleaved Siemens order) was corrected following SPM slice-timing correction (STC) procedure (Henson et al., 1999; Sladky et al., 2011), using sinc temporal interpolation to resample each slice BOLD timeseries to a common mid-acquisition time. Potential outlier scans were identified using ART (Whitfield-Gabrieli et al., 2011) as acquisitions with framewise displacement above 0.9 mm or global BOLD signal changes above 5 standard deviations (Nieto-Castanon, 2022; Power et al., 2014), and a reference BOLD image was computed for each subject by averaging all scans excluding outliers. Functional and anatomical data were normalized into standard MNI space, segmented into grey matter, white matter, and CSF tissue classes, and resampled to 2 mm isotropic voxels following a direct normalization procedure (Calhoun et al., 2017; Nieto-Castanon, 2022) using SPM unified segmentation and normalization algorithm (Ashburner and Friston, 2005; Ashburner, 2007) with the default IXI-549 tissue probability map template. Last, functional data were smoothed using spatial convolution with a Gaussian kernel of 8 mm full width half maximum (FWHM).
2.8.2 Denoising
In addition, functional data were denoised using a standard denoising pipeline (Nieto-Castanon, 2020b) including the regression of potential confounding effects characterized by white matter timeseries (5 CompCor noise components), CSF timeseries (5 CompCor noise components), motion parameters and their first order derivatives (12 factors) (Friston et al., 1996), outlier scans (below 35 factors) (Power et al., 2014), session and task effects and their first order derivatives (6 factors), and linear trends (2 factors) within each functional run, followed by bandpass frequency filtering of the BOLD timeseries (Hallquist et al., 2013) between 0.008 Hz and 0.09 Hz. CompCor (Behzadi et al., 2007; Chai et al., 2012) noise components within white matter and CSF were estimated by computing the average BOLD signal as well as the largest principal components orthogonal to the BOLD average, motion parameters, and outlier scans within each subject’s eroded segmentation masks. From the number of noise terms included in this denoising strategy, the effective degrees of freedom of the BOLD signal after denoising were estimated to range from 130.9 to 147.6 (average 144.5) across all subjects (Nieto-Castanon, 2022).
2.8.3 First-level analysis
ROI-to-ROI connectivity (RRC) matrices were estimated characterizing the functional connectivity between each pair of regions among 100 ROIs (Schaefer et al., 2018). Functional connectivity strength was represented by Fisher-transformed bivariate correlation coefficients from a general linear model (weighted-GLM) (Nieto-Castanon, 2020c), estimated separately for each pair of ROIs, characterizing the association between their BOLD signal timeseries. Individual scans were weighted by a boxcar signal characterizing each individual task or experimental condition convolved with an SPM canonical hemodynamic response function and rectified.
2.8.4 Group-level analyses
Group-level analyses were performed using a General Linear Model (GLM) (Nieto-Castanon, 2020d). A contrast that averages both post-stimulation conditions against the baseline (t1 + t2 > baseline) was designed to estimate a general model of the effects of tFUS for each active and sham group. Time points (t1 and t2) were combined to enhance the statistical power of the model due to the small sample size. Individual time point models were also estimated for each condition (t1 > baseline and t2 > baseline) in an exploratory secondary analysis to examine the temporal nature of the effects. For each individual connection a separate GLM was estimated, with first-level connectivity measures at this connection as dependent variables (one independent sample per subject and one measurement per task or experimental condition, if applicable), and groups or other subject-level identifiers as independent variables. Connection-level hypotheses were evaluated using multivariate parametric statistics with random-effects across subjects and sample covariance estimation across multiple measurements. Inferences were performed at the level of individual clusters (groups of contiguous connections). Cluster-level inferences were based on nonparametric statistics using Threshold Free Cluster Enhancement (TFCE) (Smith and Nichols, 2009), with 1,000 residual-randomization iterations, and ROIs sorted using optimal leaf ordering based on ROI-to-ROI anatomical proximity and functional similarity metrics (Bar-Joseph et al., 2001; Nieto-Castanon, 2020e). For the primary effects model (t1 + t2 > baseline), results were conservatively thresholded using a combination of a cluster-forming p < 0.001 connection-level threshold and a familywise corrected p-FDR < 0.01 cluster-mass threshold. For the secondary exploratory analysis of individual time points, less conservative thresholds were used (connection-level p < 0.05 and cluster-level p < 0.05).
3 Results
3.1 Functional connectivity
ROI-to-ROI analysis revealed significant decreases in connectivity in the active group within 1 cluster comprising 8 ROIs and 11 connections between them, while there were no significant changes in the sham group (see Table 3). The cluster comprised a decrease in connectivity between midline nodes of the DMN. There were reductions in connectivity across hemispheres in the cingulate cortex, and the medial and dorsolateral prefrontal cortex reduced in connectivity with the posterior cingulate (see Figure 3). The same model was estimated for the sham group with no significant effects found. While a single model that contrasted active and sham groups found no significant effects, the effects found in the active model were highly significant after conservative corrections were made.
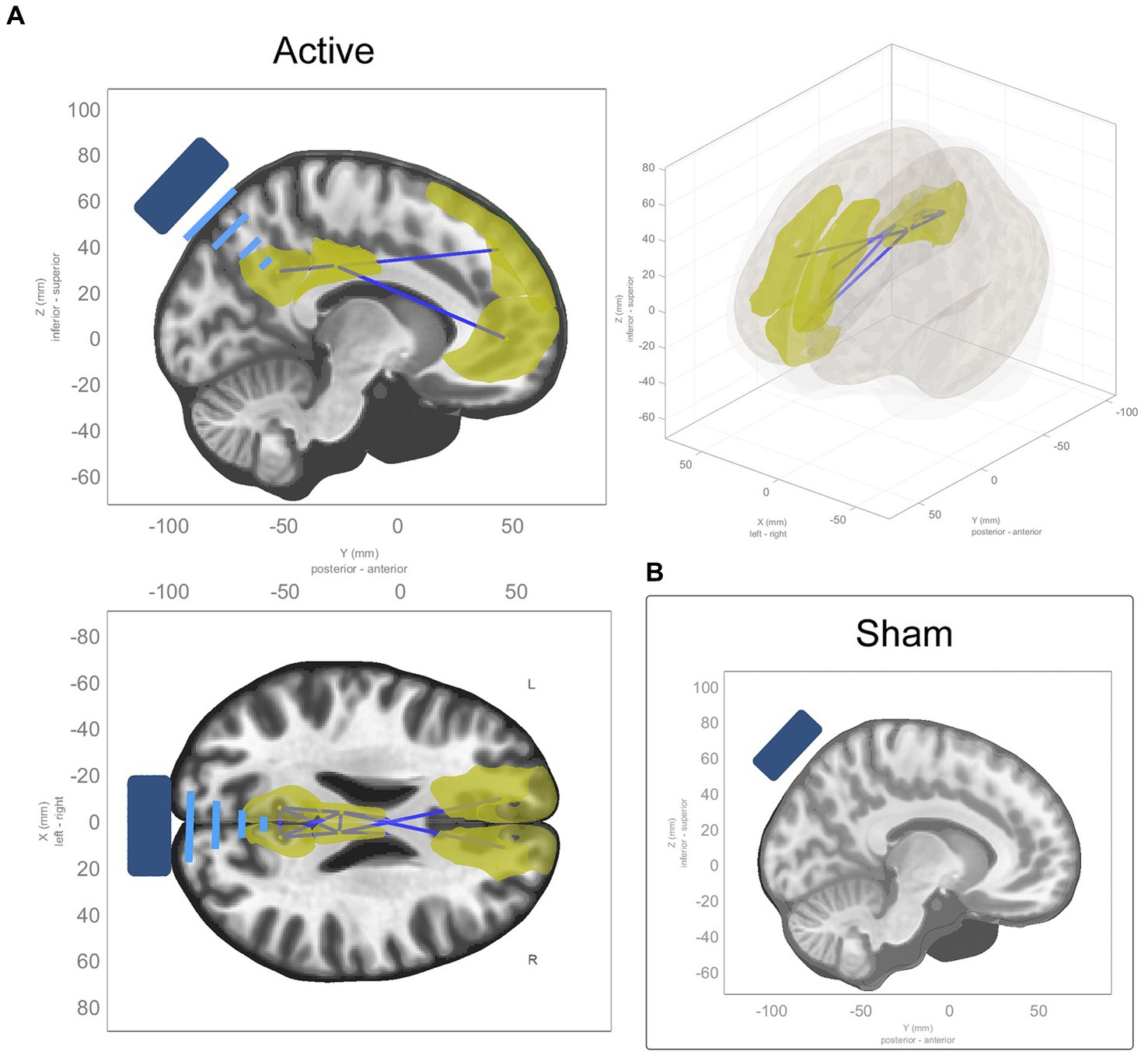
Figure 3. ROI-to-ROI functional connectivity changes from baseline to average of t1 and t2. (A) Sagittal, coronal, and axial views of significant ROI-to-ROI functional connectivity changes in active TFUS condition, all of which were decreases in functional connectivity (represented by blue connecting lines, with the affected ROIs highlighted in yellow) within and along the midline of the DMN and cingulate cortex. (B) No significant ROI-to-ROI functional connectivity changes found in the sham TFUS condition.
Models were estimated for the effects at individual time points (t1 > baseline and t2 > baseline) within each condition. In t1 of the active condition, reductions in connectivity were seen along the midline of the DMN within 1 cluster comprising 7 ROIs and 11 connections between them (see Supplementary Table S1 for details). In t2, these effects are more diffuse, extending to the left and right inferior parietal lobes, the left and right medial parietal lobes, and the left temporal pole, within 1 cluster comprising 17 ROIs and 45 connections between them (see Supplementary Table S2 for details). See panels A and B in Figure 4.
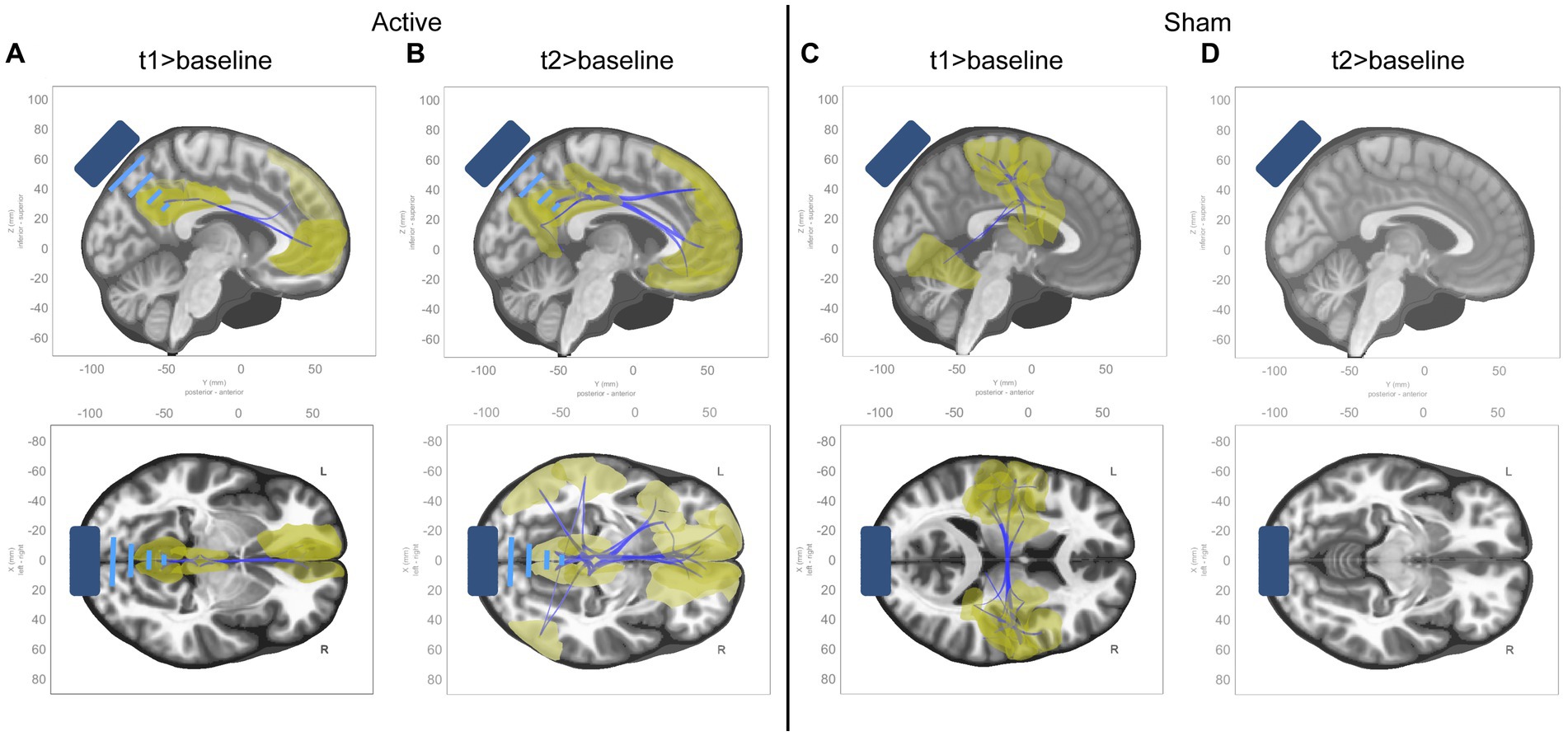
Figure 4. ROI-to-ROI functional connectivity changes from baseline to t1 and t2 in active condition. Sagittal and axial views of significant ROI-to-ROI functional connectivity changes in each timepoint compared to baseline for each condition, all of which were decreases in functional connectivity (represented by blue connecting lines, with the affected ROIs highlighted in yellow). (A) Contrast of t1 to baseline in active condition. (B) Contrast of t2 to baseline in active condition. (C) Contrast of t1 to baseline in sham condition. (D) Contrast of t2 to baseline in sham condition.
In the sham condition, connectivity reductions were seen in t1 primarily within somatomotor and dorsal attention networks, within 1 cluster comprising 26 ROIs and 69 connections between them (see Supplementary Table S3 for details). No effects were seen in t2. See panels C and D in Figure 4.
3.2 Subjective ratings
3.2.1 Psychometric scales
Due to small sample sizes (n = 15 for each condition) and skewed results in many test questions, we opted for non-parametric tests (Wilcoxon rank sum tests and linear mixed models) instead of parametric tests to analyze the psychometric scales. The Wilcoxon rank-sum test compares ranks instead of raw data, and linear mixed models are less sensitive to violations of normality. These tests are more robust, less affected by outliers or skewed data, reducing the risk of drawing incorrect conclusions.
3.2.1.1 Toronto Mindfulness Scale
We estimated a linear mixed model that included session (pre and post) and condition (active and sham) as fixed effects, their interaction, and a random intercept for subjects, for the Toronto Mindfulness Scale score. The formula for the model is Toronto Mindfulness Scale Score ~ Session * Condition + (1 | Subject) (see Table 4). There was a significant main effect of session (p = 0.0001), with the post session having higher values than the pre session. However, the main effect of condition (p = 0.552) and the interaction between session and condition (p = 0.173) were not significant.
Paired Wilcoxon rank-sum tests were performed to assess within-session differences for each condition and subscales (“curiosity” and “decentering”) of the Toronto Mindfulness Scale (see Table 5 and Figure 5). Significant differences were found within the active condition between pre and post sessions for the total mindfulness score (t(14) = −4.51, p = 0.0004), the “curiosity” subscale (t(14) = −3.98, p = 0.001), and the “decentering” subscale (t(14) = −3.24, p = 0.006). Increases in the “curiosity” subscale suggest that participants became more open to novelty and more interested in their internal experiences, with less judgment towards them. Increases in the “decentering” subscale reflect an improved ability to be detached towards one’s thoughts and feelings, avoiding identifying with them or perceiving them as accurate reflections of reality (Lau et al., 2006).
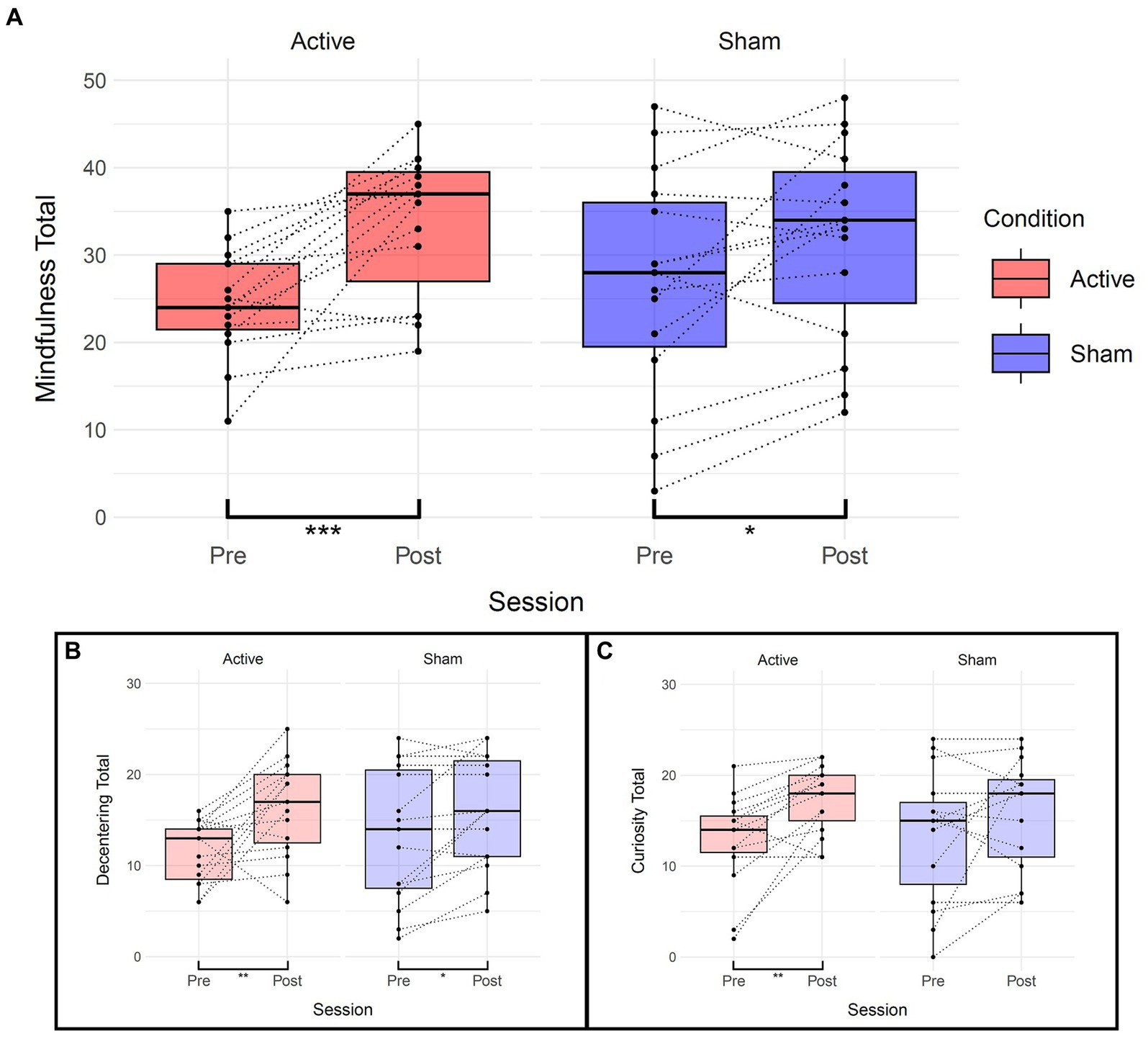
Figure 5. Effects of TFUS on Toronto Mindfulness Scale. Boxplots depicting changes measured by the Toronto Mindfulness Scale. (A) Changes in total mindfulness score between pre and post for each active and sham condition. (B) Changes in the “Decentering” subscale. (C) Changes in the “Curiosity” subscale. ***p < 0.001, **p < 0.01, *p < 0.05.
In the sham condition, significant differences were observed for the total mindfulness score (t(14) = −2.47, p = 0.027) and the “decentering” subscale (t(14) = −2.87, p = 0.012) but not the “curiosity” subscale (t(14) = −1.59, p = 0.134). The linear mixed-effects model did not show significant main effects or interactions overall, but the paired Wilcoxon rank-sum tests consistently indicated significant differences within the TFUS group and inconsistently or of smaller magnitude within the sham group.
3.2.1.2 Visual Analog Mood Scale
We estimated a linear mixed model that included session and condition as fixed effects, their interaction, and a random intercept for subjects, for each score Global Affect and Global Vigor from the VAMS. The formula for the model is [GA or GV] ~ Session * Condition + (1 | Subject) (see Table 6). There was a significant decrease in GV from the baseline to the post-session in the active condition (Estimate = −9.500, SE = 3.686, t(28) = −2.578, p = 0.016), with no significant differences in GV observed between conditions at baseline (Estimate = −3.500, SE = 5.563, t(42.591) = −0.629, p = 0.533). The interaction between session and condition was not significant (Estimate = 2.500, SE = 5.212, t(28) = 0.480, p = 0.635). The random effects structure showed a subject-specific intercept variance of 130.2 (SD = 11.41) and a residual variance of 101.9 (SD = 10.09). For GA, there were no significant differences between sessions in the active condition (Estimate = 2.500, SE = 2.589, t(28) = 0.966, p = 0.342), with no significant differences in GA observed between conditions at baseline (Estimate = −4.167, SE = 4.420, t(39.116) = −0.943, p = 0.352). The interaction between session and condition was not significant (Estimate = 3.000, SE = 3.661, t(28) = 0.819, p = 0.419). The random effects structure showed a subject-specific intercept variance of 96.28 (SD = 9.812) and a residual variance of 50.27 (SD = 7.090).
Wilcoxon rank-sum tests a significant difference in GV between sessions in the active condition (V = 75, p = 0.04238), while no significant differences were observed in GA (V = 32, p = 0.2053). In the sham condition, there were no significant differences between sessions in GV (V = 94, p = 0.05653), or GA (V = 26, p = 0.1015). See Figure 6.
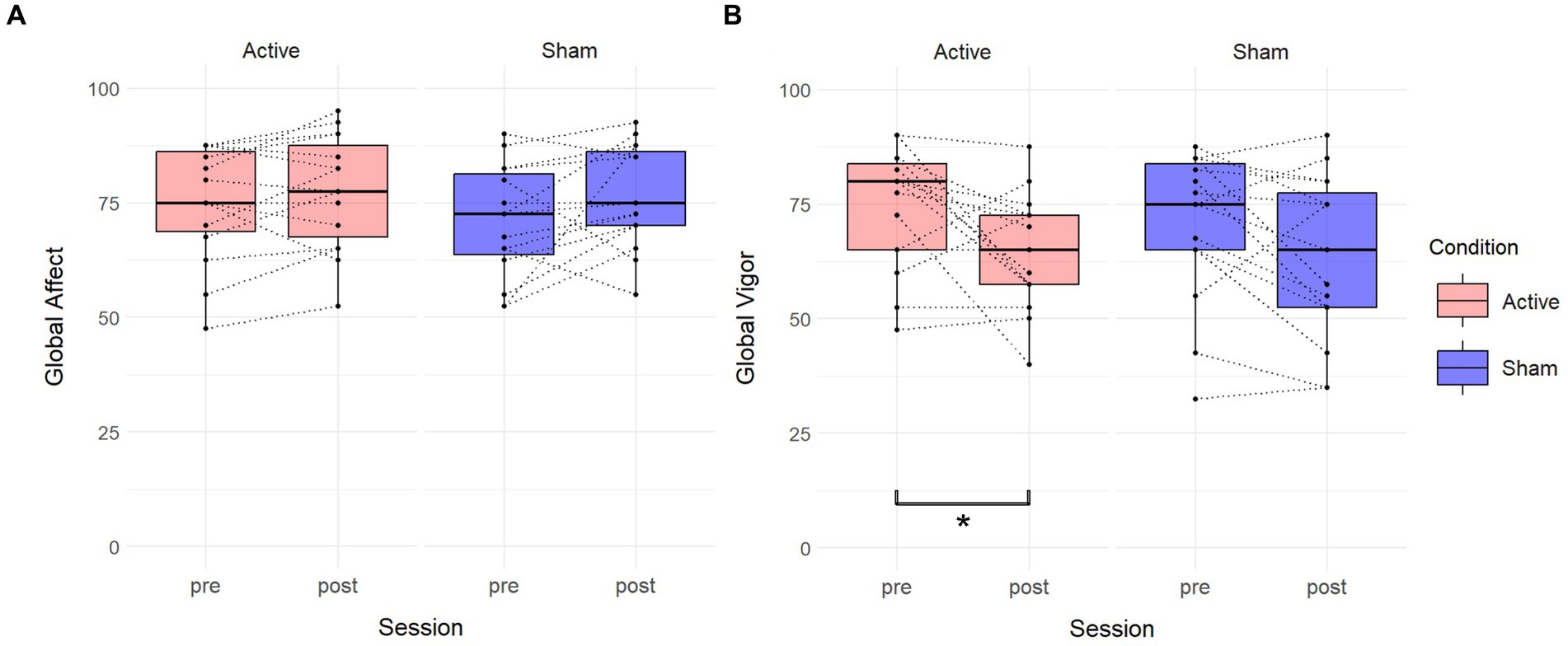
Figure 6. Effects of TFUS on Visual Analog Mood Scale. Boxplots depicting changes measured by the Visual Analog Mood Scale. (A) Changes in Global Affect score between pre and post for each active and sham condition. (B) Changes in the Global Vigor score between pre and post for each active and sham condition. A significant difference was found in the active condition. *p < 0.05.
3.2.1.3 Self Scale
Wilcoxon rank-sum tests were performed for each item to compare conditions. Three significant differences (higher scores in active) were found in the items “I saw events from my past” (W = 142, p = 0.043), “My sense of time was distorted” (W = 142, p = 0.043), and “I lost all sense of ego” (W = 148.5, p = 0.020). See Table 7 and Figure 7.
3.2.1.4 Post experiment questions
No negative experiences or adverse events were reported. When asked to guess which condition they were in, 11/15 of participants in the active condition guessed “stimulation,” and 3/15 of those in the sham condition guessed “stimulation,” Χ2 (3, N = 30) = 8.571, p = 0.0356. When asked if their mental state changed, 10/15 in the active condition said yes, and 5/15 in the sham condition said yes, Χ2 (3, N = 30) = 3.333, p = 0.3430. When asked if there were any changes in their “inner talk- or thinking-space” descriptions of felt effects included “made less thoughts,” “better,” “drifted more,” “more calm,” “mind wandering less,” “more active lighter thoughts,” “less effort to think,” “less dark thoughts,” “more calm,” “somewhat more relaxed” in the active group, and “more fluid than usual,” “I was able to organize my thoughts for the day,” “talked more,” “smoother,” “calmed down” in the sham condition. Only 3/30 of participants in either condition said they heard sounds from the transducer, and 2 of those 3 reported that sound as the hum of the amplifier. The other 1 that reported a sound described it as a “buzzing” which may have referred to the actual sound of the transducer emitting at a subaudible PRF of 10.526 Hz.
4 Discussion
This study targeted the PCC with TFUS with the aim to reduce resting state functional connectivity in the DMN, predicting that this would result in phenomenological effects on mindfulness and self-referential processing. In an ROI-to-ROI test across the whole brain, the active TFUS group showed reductions in functional connectivity along the midline of the DMN. Additionally, we found that the active TFUS group showed multiple phenomenological changes, namely, an increase in state mindfulness as measured by the Toronto Mindfulness Scale, a reduction in Global Vigor as measured by the Visual Analog Mood Scale, and changes in items related to the sense of ego, sense of time, and seeing memories from the past, as measured by the Self Scale.
We used linear mixed models to estimate a contrast between active and sham TFUS groups, and we did not find significant differences. Similarly, linear mixed models on the phenomenological measurements also did not yield significant effects. This is likely due, in part, to low statistical power for between-subject comparisons with the small sample size and to relatively low ultrasound power.
Our parameters yielded an ISPTA of 293 (mW/cm2) and an ISPPA of 5.58 (W/cm2) as measured in water. Other studies have used higher intensities on human subjects: Ai et al. (2018) had an Ispta of 6.102 W/cm2 and an ISPPA of 16.95 W/cm2; Legon et al. (2018) had an ISPTA of 6.192 W/cm2 and an ISPPA of 17.2 W/cm2. Numerous animal studies have used even higher intensities to elicit their detected effects (Yoo et al., 2011; Kim et al., 2014, 2022; Yoon et al., 2019). Additionally, transmitting ultrasound through the posterior parietal portion of the skull is likely to significantly attenuate the amount of energy that gets transmitted (Mueller et al., 2017). Compared to Sanguinetti et al. (2020), who used the thin “temporal window” for transmission, we transmitted less energy to a deeper neural region. It is likely that higher intensities are needed for more consistent effects.
No significant effects were found in BOLD signal or pc-ASL analysis. This is most likely due to the low intensity of the ultrasound. It may be the case that the intensities used in this study represent the “floor” at which any effects are exhibited in brain activity. That such effects show up in BOLD functional connectivity but not simple BOLD signal suggests more of a tissue-level disruptive or suppressive effect than a straightforward inhibitory effect.
Given that we saw significant increases in mindfulness in the sham group, it is possible that the combination of the MRI scanner, the simulated brain stimulation, and the cues provided by the Toronto Mindfulness Scale and VAMS scales caused subjects to become more present-centered and mindful in their attention. Despite that, the effect sizes in the Toronto Mindfulness Scale changes for the active group were consistently larger compared to the sham group, suggesting TFUS to the DMN may enhance state mindfulness. Future studies that employ increased TFUS power, better targeting methods (such as functional-based targeting), or that combine TFUS with mindfulness training may find a clearer link between DMN changes and state mindfulness.
This study was intended to be a proof-of-concept that it is possible to modulate DMN with focused TFUS. The intention was to make the parameters suppressive by using a low duty cycle. We targeted along the midline, aiming to hit both hemispheres of the PCC. DMN functional connectivity decreased, suggesting a disruption to the stability of the network, including left and right hemisphere locations along the midline decoupling from each other. Although we targeted ventral PCC, other areas like the dorsal PCC and precuneus may have also been in the path of the beam, which may have only added to the meditation-like effects that were observed (Garrison et al., 2015). Given that our tank measurements suggest the beam’s focus was shortened by the skull (see Figure 2 and Table 2), it is very possible that the precuneus was also affected.
Exploratory analysis of each individual time point revealed that the effects appeared more focused to the midline DMN in t1 and then became more diffuse, spreading to more distal areas in t2. This could indicate the path by which the offline effects dispersed throughout functional networks. In the sham condition, broad reductions were seen in somatomotor and dorsal attention networks in t1, but these did not persist into t2, and may be the result of a disengagement of attention with external environment and a switch to a more internal mode of processing. These exploratory results should be interpreted cautiously given the relaxed statistical threshold.
It is also worth noting that these effects persisted for at least 30 min (25 min + 6 min scan) after the application of TFUS. Sanguinetti et al. (2020) found offline increases in mood for at least 30 min following TFUS to the right dlPFC, Kim et al. (2023) found offline changes in resting state functional connectivity lasting more than an hour following thalamic stimulation, and Verhagen et al. (2019) found offline effects persisted for more than an hour in macaques. These long-lasting offline effects may be due to glutamate release via the opening of TRPA1 channels in astrocytes (Oh et al., 2019), opening up the possibility of long-term plasticity effects. The full temporal extent of these offline effects should be the subject of future research. However, in the post-experiment questions, participants, even those reporting subjective effects, reported they were back to baseline by the post-questioning (~1 h post sonication).
We did not use masking or sham sound for the transducer because our PRF was in the subaudible range, and PRF is what is heard (Braun et al., 2020). Only one participant reported a “buzzing” that could conceivably be that sound. This, combined with the 11/15 of participants that correctly guessed they were in the active condition (compared to 3/15 for sham) suggests that the distinct subjective experiences played a role in their judgment.
The phenomenological effects of this treatment correspond with what one might predict would result in DMN disruption via the PCC (Brewer et al., 2013), and it demonstrates that TFUS has the potential to be used as an unprecedented neuromodulatory probe for deep brain sites. The brief subjective descriptions of “lighter” or “less” thoughts, “drifting,” and “less effort” correspond with Brewer et al.’s (2013) description of the PCC being involved in “getting caught up in” one’s thoughts. Given that we were able to reduce DMN functional connectivity in naïve undergraduate students, it would be fruitful to measure the same effects on experienced meditators. Individuals who have developed a richer interior clarity and are more capable of manipulating at will their DMN activity might find an added benefit to meditation when TFUS is applied to their PCC, such as was found in PCC reduction neurofeedback studies with experienced meditators (Garrison et al., 2013; van Lutterveld et al., 2017).
We also found reductions in the sense of ego, though to a much lesser degree than what is seen in psychedelics (Lebedev et al., 2015). Our results were also not accompanied by sensory distortions or hallucinations, likely due to the fact the effects of TFUS were isolated to the DMN. This further demonstrates how the spatial specificity of TFUS can be used by researchers to generate causal models about functional brain networks.
These effects also suggest that TFUS shows promise as a therapeutic tool for disorders associated with DMN activity, such as depression and anxiety (Scalabrini et al., 2020). It may serve in other roles, too, given that it provides superior targeting abilities compared to other non-invasive brain stimulation techniques. The offline effects would allow for the TFUS to be embedded in a larger therapeutic intervention that corrects functional imbalances in the brain’s activity.
It should finally be noted that all of this was achieved with a relatively rudimentary targeting approach. Although individual MR-guided neuronavigation was used, the transducer was held by hand, the target was selected by anatomical structure alone, and no efforts were made to minimize skull aberrations. Future research can improve on all these circumstances with robot-controlled transducers, functional-based targeting, and skull aberration modeling. That we saw such significant effects with this approach is extremely promising for the future of TFUS.
5 Conclusion
This pilot study showed that TFUS targeted at the PCC can disrupt DMN activity and cause mindfulness-increasing subjective effects. Given these effects, TFUS may serve as a therapeutic tool for treating network dysfunction. Future research should replicate these effects with a larger sample size, more precise targeting methods, and TFUS intensities matching previous human and animal studies. Future research may also investigate what ultrasound parameters, targeting, and modeling methods are optimal for neuromodulation.
Data availability statement
The raw data supporting the conclusions of this article will be made available by the authors, without undue reservation.
Ethics statement
The studies involving humans were approved by University of Arizona Human Subjects Protection Program. The studies were conducted in accordance with the local legislation and institutional requirements. The participants provided their written informed consent to participate in this study.
Author contributions
BL: Data curation, Formal analysis, Investigation, Software, Validation, Visualization, Writing – original draft, Writing – review & editing. JLS: Conceptualization, Formal analysis, Funding acquisition, Investigation, Methodology, Project administration, Software, Supervision, Writing – review & editing. LR: Investigation, Project administration, Writing – review & editing. VM: Conceptualization, Methodology, Supervision, Writing – review & editing. JS: Conceptualization, Methodology, Supervision, Writing – review & editing. SY: Conceptualization, Methodology, Supervision, Writing – review & editing. MF: Formal analysis, Investigation, Software, Writing – review & editing. JJBA: Conceptualization, Methodology, Project administration, Supervision, Writing – review & editing.
Funding
The author(s) declare financial support was received for the research, authorship, and/or publication of this article. Funding provided by: X (formerly Google X), the Moonshot Factory; SEMA Lab crowdfunding campaign through the UA Foundation. The funding source had no influence on study conduction or result evaluation.
Acknowledgments
Authors would like to thank our generous donors from the crowdfunding campaign for supporting this research. Special thanks to Desmond Chambers for his invaluable help with the tank measurements.
Conflict of interest
JLS was paid a salary and was a shareholder in Sanmai Technologies, PBC. LR was paid a salary by Sanmai Technologies, PBC. JS and VM were paid a salary by X Moonshot Factory.
The remaining authors declare that the research was conducted in the absence of any commercial or financial relationships that could be construed as a potential conflict of interest.
Publisher’s note
All claims expressed in this article are solely those of the authors and do not necessarily represent those of their affiliated organizations, or those of the publisher, the editors and the reviewers. Any product that may be evaluated in this article, or claim that may be made by its manufacturer, is not guaranteed or endorsed by the publisher.
Supplementary material
The Supplementary material for this article can be found online at: https://www.frontiersin.org/articles/10.3389/fnhum.2024.1392199/full#supplementary-material
References
Abbott, J. G. (1999). Rationale and derivation of MI and TI—a review. Ultrasound Med. Biol. 25, 431–441. doi: 10.1016/S0301-5629(98)00172-0
Ai, L., Bansal, P., Mueller, J. K., and Legon, W. (2018). Effects of transcranial focused ultrasound on human primary motor cortex using 7T fMRI: a pilot study. BMC Neurosci. 19, 56–10. doi: 10.1186/s12868-018-0456-6
Andersson, J. L. R., Hutton, C., Ashburner, J., Turner, R., and Friston, K. (2001). Modeling geometric deformations in EPI time series. NeuroImage 13, 903–919. doi: 10.1006/nimg.2001.0746
Andrews-Hanna, J. R. (2012). The Brain’s default network and its adaptive role in internal mentation. Neuroscientist 18, 251–270. doi: 10.1177/1073858411403316
Andrews-Hanna, J. R., Reidler, J. S., Sepulcre, J., Poulin, R., and Buckner, R. L. (2010). Functional-anatomic fractionation of the Brain’s default network. Neuron 65, 550–562. doi: 10.1016/j.neuron.2010.02.005
Andrews-Hanna, J. R., Smallwood, J., and Spreng, R. N. (2014). The default network and self-generated thought: component processes, dynamic control, and clinical relevance. Ann. N. Y. Acad. Sci. 1316, 29–52. doi: 10.1111/nyas.12360
Ashburner, J. (2007). A fast diffeomorphic image registration algorithm. NeuroImage 38, 95–113. doi: 10.1016/j.neuroimage.2007.07.007
Ashburner, J., and Friston, K. J. (2005). Unified segmentation. NeuroImage 26, 839–851. doi: 10.1016/j.neuroimage.2005.02.018
Badran, B. W., Caulfield, K. A., Stomberg-Firestein, S., Summers, P. M., Dowdle, L. T., Savoca, M., et al. (2020). Sonication of the anterior thalamus with MRI-guided transcranial focused ultrasound (tFUS) alters pain thresholds in healthy adults: a double-blind, sham-controlled study. Brain Stimulat. 13, 1805–1812. doi: 10.1016/j.brs.2020.10.007
Bar-Joseph, Z., Gifford, D. K., and Jaakkola, T. S. (2001). Fast optimal leaf ordering for hierarchical clustering. Bioinformatics 17, S22–S29. doi: 10.1093/bioinformatics/17.suppl_1.S22
Behzadi, Y., Restom, K., Liau, J., and Liu, T. T. (2007). A component based noise correction method (CompCor) for BOLD and perfusion based fMRI. NeuroImage 37, 90–101. doi: 10.1016/j.neuroimage.2007.04.042
Blackmore, J., Shrivastava, S., Sallet, J., Butler, C. R., and Cleveland, R. O. (2019). Ultrasound neuromodulation: a review of results, mechanisms and safety. Ultrasound Med. Biol. 45, 1509–1536. doi: 10.1016/j.ultrasmedbio.2018.12.015
Braun, V., Blackmore, J., Cleveland, R. O., and Butler, C. R. (2020). Transcranial ultrasound stimulation in humans is associated with an auditory confound that can be effectively masked. Brain Stimulat. 13, 1527–1534. doi: 10.1016/j.brs.2020.08.014
Brewer, J. A., and Garrison, K. A. (2014). The posterior cingulate cortex as a plausible mechanistic target of meditation: findings from neuroimaging: the PCC as a target of meditation. Ann. N. Y. Acad. Sci. 1307, 19–27. doi: 10.1111/nyas.12246
Brewer, J., Garrison, K., and Whitfield-Gabrieli, S. (2013). What about the “self” is processed in the posterior cingulate cortex? Front. Hum. Neurosci. 7:647. doi: 10.3389/fnhum.2013.00647
Cain, J. A., Visagan, S., Johnson, M. A., Crone, J., Blades, R., Spivak, N. M., et al. (2021). Real time and delayed effects of subcortical low intensity focused ultrasound. Sci. Rep. 11:6100. doi: 10.1038/s41598-021-85504-y
Calhoun, V. D., Wager, T. D., Krishnan, A., Rosch, K. S., Seymour, K. E., Nebel, M. B., et al. (2017). The impact of T1 versus EPI spatial normalization templates for fMRI data analyses. Hum. Brain Mapp. 38, 5331–5342. doi: 10.1002/hbm.23737
Carhart-Harris, R. L., Erritzoe, D., Williams, T., Stone, J. M., Reed, L. J., Colasanti, A., et al. (2012). Neural correlates of the psychedelic state as determined by fMRI studies with psilocybin. Proc. Natl. Acad. Sci. USA 109, 2138–2143. doi: 10.1073/pnas.1119598109
Carhart-Harris, R. L., and Friston, K. J. (2019). REBUS and the anarchic brain: toward a unified model of the brain action of psychedelics. Pharmacol. Rev. 71, 316–344. doi: 10.1124/pr.118.017160
Chai, X. J., Castañón, A. N., Öngür, D., and Whitfield-Gabrieli, S. (2012). Anticorrelations in resting state networks without global signal regression. NeuroImage 59, 1420–1428. doi: 10.1016/j.neuroimage.2011.08.048
Davey, C. G., and Harrison, B. J. (2022). The self on its axis: a framework for understanding depression. Transl. Psychiatry 12, 23–29. doi: 10.1038/s41398-022-01790-8
Davey, C. G., Pujol, J., and Harrison, B. J. (2016). Mapping the self in the brain’s default mode network. NeuroImage 132, 390–397. doi: 10.1016/j.neuroimage.2016.02.022
Dell’Italia, J., Sanguinetti, J. L., Monti, M. M., Bystritsky, A., and Reggente, N. (2022). Current state of potential mechanisms supporting low intensity focused ultrasound for neuromodulation. Front. Hum. Neurosci. 16:872639. doi: 10.3389/fnhum.2022.872639
Desbordes, G., Gard, T., Hoge, E. A., Hölzel, B. K., Kerr, C., Lazar, S. W., et al. (2015). Moving beyond mindfulness: defining equanimity as an outcome measure in meditation and contemplative research. Mindfulness 6, 356–372. doi: 10.1007/s12671-013-0269-8
Diaz, B. A., van der Sluis, S., Moens, S., Benjamins, J., Migliorati, F., Stoffers, D., et al. (2013). The Amsterdam resting-state questionnaire reveals multiple phenotypes of resting-state cognition. Front. Hum. Neurosci. 7:446. doi: 10.3389/fnhum.2013.00446
Folloni, D., Verhagen, L., Mars, R. B., Fouragnan, E., Constans, C., Aubry, J.-F., et al. (2019). Manipulation of subcortical and deep cortical activity in the primate brain using transcranial focused ultrasound stimulation. Neuron 101, 1109–1116.e5. doi: 10.1016/j.neuron.2019.01.019
Friston, K., Ashburner, J., Frith, C. D., Poline, J.-B., Heather, J. D., and Frackowiak, R. S. J. (1995). Spatial registration and normalization of images. Hum. Brain Mapp. 3, 165–189. doi: 10.1002/hbm.460030303
Friston, K. J., Williams, S., Howard, R., Frackowiak, R. S. J., and Turner, R. (1996). Movement-related effects in fMRI time-series. Magn. Reson. Med. 35, 346–355. doi: 10.1002/mrm.1910350312
Garrison, K., Santoyo, J., Davis, J., Thornhill, T., Kerr, C., and Brewer, J. (2013). Effortless awareness: using real time neurofeedback to investigate correlates of posterior cingulate cortex activity in meditators’ self-report. Front. Hum. Neurosci. 7:440. doi: 10.3389/fnhum.2013.00440
Garrison, K. A., Zeffiro, T. A., Scheinost, D., Constable, R. T., and Brewer, J. A. (2015). Meditation leads to reduced default mode network activity beyond an active task. Cogn. Affect. Behav. Neurosci. 15, 712–720. doi: 10.3758/s13415-015-0358-3
Gattuso, J. J., Perkins, D., Ruffell, S., Lawrence, A. J., Hoyer, D., Jacobson, L. H., et al. (2022). Default mode network modulation by psychedelics: a systematic review. Int. J. Neuropsychopharmacol. 26, 155–188. doi: 10.1093/ijnp/pyac074
Hallquist, M. N., Hwang, K., and Luna, B. (2013). The nuisance of nuisance regression: spectral misspecification in a common approach to resting-state fMRI preprocessing reintroduces noise and obscures functional connectivity. NeuroImage 82, 208–225. doi: 10.1016/j.neuroimage.2013.05.116
Harikumar, A., Evans, D. W., Dougherty, C. C., Carpenter, K. L. H., and Michael, A. M. (2021). A review of the default mode network in autism spectrum disorders and attention deficit hyperactivity disorder. Brain Connect. 11, 253–263. doi: 10.1089/brain.2020.0865
Henson, R., Büchel, C., Josephs, O., and Friston, K. (1999). The slice-timing problem in event-related fMRI. NeuroImage 9:s125
Holtzheimer, P. E., and Mayberg, H. S. (2011). Stuck in a rut: rethinking depression and its treatment. Trends Neurosci. 34, 1–9. doi: 10.1016/j.tins.2010.10.004
Hu, M.-L., Zong, X.-F., Mann, J. J., Zheng, J.-J., Liao, Y.-H., Li, Z.-C., et al. (2017). A review of the functional and anatomical default mode network in schizophrenia. Neurosci. Bull. 33, 73–84. doi: 10.1007/s12264-016-0090-1
Ju, Y., Wang, M., Liu, J., Liu, B., Yan, D., Lu, X., et al. (2022). Modulation of resting-state functional connectivity in default mode network is associated with the long-term treatment outcome in major depressive disorder. Psychol. Med. 53, 5963–5975. doi: 10.1017/S0033291722002628
Kim, H., Chiu, A., Lee, S. D., Fischer, K., and Yoo, S. S. (2014). Focused ultrasound-mediated non-invasive brain stimulation: examination of sonication parameters. Brain Stimulat. 7, 748–756. doi: 10.1016/j.brs.2014.06.011
Kim, H.-C., Lee, W., Kowsari, K., Weisholtz, D. S., and Yoo, S.-S. (2022). Effects of focused ultrasound pulse duration on stimulating cortical and subcortical motor circuits in awake sheep. PLoS One 17:e0278865. doi: 10.1371/journal.pone.0278865
Kim, H.-C., Lee, W., Weisholtz, D. S., and Yoo, S.-S. (2023). Transcranial focused ultrasound stimulation of cortical and thalamic somatosensory areas in human. PLoS One 18:e0288654. doi: 10.1371/journal.pone.0288654
Korponay, C., Dentico, D., Kral, T. R. A., Ly, M., Kruis, A., Davis, K., et al. (2019). The effect of mindfulness meditation on impulsivity and its neurobiological correlates in healthy adults. Sci. Rep. 9:11963. doi: 10.1038/s41598-019-47662-y
Lau, M. A., Bishop, S. R., Segal, Z. V., Buis, T., Anderson, N. D., Carlson, L., et al. (2006). The Toronto mindfulness scale: development and validation. J. Clin. Psychol. 62, 1445–1467. doi: 10.1002/jclp.20326
Lebedev, A. V., Lövdén, M., Rosenthal, G., Feilding, A., Nutt, D. J., and Carhart-Harris, R. L. (2015). Finding the self by losing the self: neural correlates of ego-dissolution under psilocybin. Hum. Brain Mapp. 36, 3137–3153. doi: 10.1002/hbm.22833
Leech, R., and Sharp, D. J. (2014). The role of the posterior cingulate cortex in cognition and disease. Brain 137, 12–32. doi: 10.1093/brain/awt162
Legon, W., Ai, L., Bansal, P., and Mueller, J. K. (2018). Neuromodulation with single-element transcranial focused ultrasound in human thalamus. Hum. Brain Mapp. 39, 1995–2006. doi: 10.1002/hbm.23981
Li, X., Badran, B., Dowdle, L., Caulfield, K., Summers, P., Short, B., et al. (2021). Imaged-guided transcranial focused ultrasound on the right thalamus modulates ascending pain pathway to somatosensory cortex in healthy participants. Brain Stimul. Basic Transl. Clin. Res. Neuromodulation 14:1638. doi: 10.1016/j.brs.2021.10.160
Luria, R. E. (1975). The validity and reliability of the visual analogue mood scale. J. Psychiatr. Res. 12, 51–57. doi: 10.1016/0022-3956(75)90020-5
Lydon-Staley, D. M., Kuehner, C., Zamoscik, V., Huffziger, S., Kirsch, P., and Bassett, D. S. (2019). Repetitive negative thinking in daily life and functional connectivity among default mode, fronto-parietal, and salience networks. Transl. Psychiatry 9, 234–212. doi: 10.1038/s41398-019-0560-0
Mueller, J. K., Ai, L., Bansal, P., and Legon, W. (2017). Numerical evaluation of the skull for human neuromodulation with transcranial focused ultrasound. J. Neural Eng. 14:066012. doi: 10.1088/1741-2552/aa843e
Nieto-Castanon, A. (2020a). “FMRI minimal preprocessing pipeline” in Handbook of functional connectivity magnetic resonance imaging methods in CONN (Boston, MA: Hilbert Press), 3–16.
Nieto-Castanon, A. (2020b). “FMRI denoising pipeline” in Handbook of functional connectivity magnetic resonance imaging methods in CONN (Boston, MA: Hilbert Press), 17–25.
Nieto-Castanon, A. (2020c). “Functional connectivity measures” in Handbook of functional connectivity magnetic resonance imaging methods in CONN (Boston, MA: Hilbert Press), 26–62.
Nieto-Castanon, A. (2020d). “General linear model” in Handbook of functional connectivity magnetic resonance imaging methods in CONN (Boston, MA: Hilbert Press), 63–82.
Nieto-Castanon, A. (2020e). “Cluster-level inferences” in Handbook of functional connectivity magnetic resonance imaging methods in CONN (Boston, MA: Hilbert Press), 83–104.
Nieto-Castanon, A. (2022). “Preparing fMRI data for statistical analysis” in fMRI techniques and protocols. ed. M. Filippi (Springer).
Nieto-Castanon, A., and Whitfield-Gabrieli, S. (2021). CONN functional connectivity toolbox: RRID SCR_009550, release 21. 21st Edn. Boston, MA: Hilbert Press.
Nour, M. M., and Carhart-Harris, R. L. (2017). Psychedelics and the science of self-experience. Br. J. Psychiatry 210, 177–179. doi: 10.1192/bjp.bp.116.194738
Oh, S.-J., Lee, J. M., Kim, H.-B., Lee, J., Han, S., Bae, J. Y., et al. (2019). Ultrasonic neuromodulation via astrocytic TRPA1. Curr. Biol. 29, 3386–3401.e8. doi: 10.1016/j.cub.2019.08.021
Padmanabhan, A., Lynch, C. J., Schaer, M., and Menon, V. (2017). The default mode network in autism. Biol. Psychiatry Cogn. Neurosci. Neuroimaging 2, 476–486. doi: 10.1016/j.bpsc.2017.04.004
Pasquinelli, C., Hanson, L. G., Siebner, H. R., Lee, H. J., and Thielscher, A. (2019). Safety of transcranial focused ultrasound stimulation: a systematic review of the state of knowledge from both human and animal studies. Brain Stimulat. 12, 1367–1380. doi: 10.1016/j.brs.2019.07.024
Penny, W. D., Friston, K. J., Ashburner, J. T., Kiebel, S. J., and Nichols, T. E. (2011). Statistical parametric mapping: the analysis of functional brain images. London: Elsevier.
Posner, J., Park, C., and Wang, Z. (2014). Connecting the dots: a review of resting connectivity MRI studies in attention-deficit/hyperactivity disorder. Neuropsychol. Rev. 24, 3–15. doi: 10.1007/s11065-014-9251-z
Power, J. D., Mitra, A., Laumann, T. O., Snyder, A. Z., Schlaggar, B. L., and Petersen, S. E. (2014). Methods to detect, characterize, and remove motion artifact in resting state fMRI. NeuroImage 84, 320–341. doi: 10.1016/j.neuroimage.2013.08.048
Raichle, M. E. (2015). The brain’s default mode network. Annu. Rev. Neurosci. 38, 433–447. doi: 10.1146/annurev-neuro-071013-014030
Raichle, M. E., MacLeod, A. M., Snyder, A. Z., Powers, W. J., Gusnard, D. A., and Shulman, G. L. (2001). A default mode of brain function. Proc. Natl. Acad. Sci. 98, 676–682. doi: 10.1073/pnas.98.2.676
Sanguinetti, J. L., Hameroff, S., Smith, E. E., Sato, T., Daft, C. M. W., Tyler, W. J., et al. (2020). Transcranial focused ultrasound to the right prefrontal cortex improves mood and alters functional connectivity in humans. Front. Hum. Neurosci. 14:52. doi: 10.3389/fnhum.2020.00052
Scalabrini, A., Vai, B., Poletti, S., Damiani, S., Mucci, C., Colombo, C., et al. (2020). All roads lead to the default-mode network—global source of DMN abnormalities in major depressive disorder. Neuropsychopharmacology 45, 2058–2069. doi: 10.1038/s41386-020-0785-x
Schaefer, A., Kong, R., Gordon, E. M., Laumann, T. O., Zuo, X.-N., Holmes, A. J., et al. (2018). Local-global parcellation of the human cerebral cortex from intrinsic functional connectivity MRI. Cereb. Cortex 28, 3095–3114. doi: 10.1093/cercor/bhx179
Sladky, R., Friston, K. J., Tröstl, J., Cunnington, R., Moser, E., and Windischberger, C. (2011). Slice-timing effects and their correction in functional MRI. NeuroImage 58, 588–594. doi: 10.1016/j.neuroimage.2011.06.078
Smith, S. M., and Nichols, T. E. (2009). Threshold-free cluster enhancement: addressing problems of smoothing, threshold dependence and localisation in cluster inference. NeuroImage 44, 83–98. doi: 10.1016/j.neuroimage.2008.03.061
van Lutterveld, R., Houlihan, S. D., Pal, P., Sacchet, M. D., McFarlane-Blake, C., Patel, P. R., et al. (2017). Source-space EEG neurofeedback links subjective experience with brain activity during effortless awareness meditation. NeuroImage 151, 117–127. doi: 10.1016/j.neuroimage.2016.02.047
Verhagen, L., Gallea, C., Folloni, D., Constans, C., Jensen, D. E., Ahnine, H., et al. (2019). Offline impact of transcranial focused ultrasound on cortical activation in primates. eLife 8:e40541. doi: 10.7554/eLife.40541
Wattiez, N., Constans, C., Deffieux, T., Daye, P. M., Tanter, M., Aubry, J.-F., et al. (2017). Transcranial ultrasonic stimulation modulates single-neuron discharge in macaques performing an antisaccade task. Brain Stimulat. 10, 1024–1031. doi: 10.1016/j.brs.2017.07.007
Whitfield-Gabrieli, S., and Nieto-Castanon, A. (2012). Conn: a functional connectivity toolbox for correlated and anticorrelated brain networks. Brain Connect. 2, 125–141. doi: 10.1089/brain.2012.0073
Yang, P.-F., Phipps, M. A., Jonathan, S., Newton, A. T., Byun, N., Gore, J. C., et al. (2021). Bidirectional and state-dependent modulation of brain activity by transcranial focused ultrasound in non-human primates. Brain Stimulat. 14, 261–272. doi: 10.1016/j.brs.2021.01.006
Yeshurun, Y., Nguyen, M., and Hasson, U. (2021). The default mode network: where the idiosyncratic self meets the shared social world. Nat. Rev. Neurosci. 22, 181–192. doi: 10.1038/s41583-020-00420-w
Yoo, S.-S., Bystritsky, A., Lee, J.-H., Zhang, Y., Fischer, K., Min, B.-K., et al. (2011). Focused ultrasound modulates region-specific brain activity. NeuroImage 56, 1267–1275. doi: 10.1016/j.neuroimage.2011.02.058
Yoon, K., Lee, W., Lee, J. E., Xu, L., Croce, P., Foley, L., et al. (2019). Effects of sonication parameters on transcranial focused ultrasound brain stimulation in an ovine model. PLoS One 14:e0224311. doi: 10.1371/journal.pone.0224311
Zhang, T., Pan, N., Wang, Y., Liu, C., and Hu, S. (2021). Transcranial focused ultrasound neuromodulation: a review of the excitatory and inhibitory effects on brain activity in human and animals. Front. Hum. Neurosci. 15:749162. doi: 10.3389/fnhum.2021.749162
Zhang, R., and Volkow, N. D. (2019). Brain default-mode network dysfunction in addiction. NeuroImage 200, 313–331. doi: 10.1016/j.neuroimage.2019.06.036
Zhou, H.-X., Chen, X., Shen, Y.-Q., Li, L., Chen, N.-X., Zhu, Z.-C., et al. (2020). Rumination and the default mode network: Meta-analysis of brain imaging studies and implications for depression. NeuroImage 206:116287. doi: 10.1016/j.neuroimage.2019.116287
Keywords: transcranial focused ultrasound, neuromodulation, non-invasive brain stimulation, default mode network, mindfulness, fMRI
Citation: Lord B, Sanguinetti JL, Ruiz L, Miskovic V, Segre J, Young S, Fini ME and Allen JJB (2024) Transcranial focused ultrasound to the posterior cingulate cortex modulates default mode network and subjective experience: an fMRI pilot study. Front. Hum. Neurosci. 18:1392199. doi: 10.3389/fnhum.2024.1392199
Edited by:
Vincent Clark, University of New Mexico, United StatesReviewed by:
Lei Gao, Wuhan University, ChinaSeung-Schik Yoo, Brigham and Women's Hospital and Harvard Medical School, United States
Dingjie Suo, Beijing Institute of Technology, China
Copyright © 2024 Lord, Sanguinetti, Ruiz, Miskovic, Segre, Young, Fini and Allen. This is an open-access article distributed under the terms of the Creative Commons Attribution License (CC BY). The use, distribution or reproduction in other forums is permitted, provided the original author(s) and the copyright owner(s) are credited and that the original publication in this journal is cited, in accordance with accepted academic practice. No use, distribution or reproduction is permitted which does not comply with these terms.
*Correspondence: Brian Lord, brianlord@arizona.edu