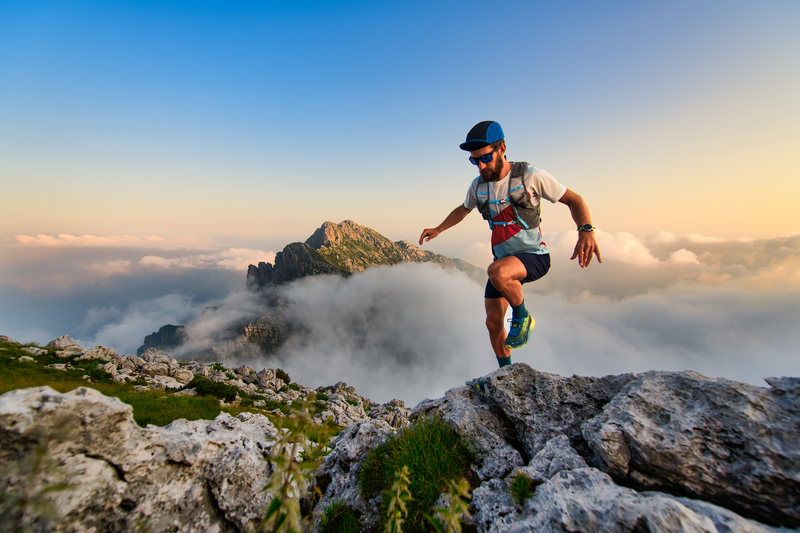
95% of researchers rate our articles as excellent or good
Learn more about the work of our research integrity team to safeguard the quality of each article we publish.
Find out more
ORIGINAL RESEARCH article
Front. Hum. Neurosci. , 07 August 2024
Sec. Speech and Language
Volume 18 - 2024 | https://doi.org/10.3389/fnhum.2024.1382102
Introduction: Functional magnetic resonance imaging (fMRI) can improve our understanding of neural processes subserving motor speech function. Yet its reproducibility remains unclear. This study aimed to evaluate the reproducibility of fMRI using a word repetition task across two time points.
Methods: Imaging data from 14 healthy controls were analysed using a multi-level general linear model.
Results: Significant activation was observed during the task in the right hemispheric cerebellar lobules IV-V, right putamen, and bilateral sensorimotor cortices. Activation between timepoints was found to be moderately reproducible across time in the cerebellum but not in other brain regions.
Discussion: Preliminary findings highlight the involvement of the cerebellum and connected cerebral regions during a motor speech task. More work is needed to determine the degree of reproducibility of speech fMRI before this could be used as a reliable marker of changes in brain activity.
Speech production includes the perceptual and motor components of language – how we produce the sounds used to share ideas and information (ASHA, 2020). It involves a complex combination of memory, perception, speech motor planning and execution. Even the final act of overt speech production is a complex, multisystem behaviour. Word selection and retrieval is primarily associated with the medial temporal and medial frontal gyri in the left cerebral hemisphere, and Crus I of the right cerebellar hemisphere (Price, 2012; Riès et al., 2017). Broca’s area plays a role in priming and preparing the motor system for articulation (Wildgruber et al., 1996; Watkins and Paus, 2004). This region of the left inferior frontal gyrus (IFG-L) coordinates information for the motor cortex prior to speech production (Flinker et al., 2015). The IFG-L is active during the word retrieval and response preparation stages of speech (Korzeniewska et al., 2011). Next, regions such as the premotor cortex, cerebellum, thalamus and putamen are involved in articulation alongside the lip, jaw and larynx regions of the primary motor and somatosensory cortices (Nota and Honda, 2004; Guenther, 2006; Price, 2012). The laryngeal musculature area of the primary motor cortex has also been linked to phonation and voluntary breath control required for speech (Correia et al., 2020). The onset of articulation occurs just prior to acoustic onset, or the vocalisation of speech (Mooshammer et al., 2012; Schaeffler et al., 2014; Jouen et al., 2021). Vocalisation similarly involves the bilateral primary motor and somatosensory cortices and inferior cerebellum, as well as the premotor cortex and supplementary motor area (Nota and Honda, 2004; Brown et al., 2021). While language is typically left lateralised, these motor components of speech production are more bilaterally represented within the cerebral cortex (Brown et al., 2009).
Studies using functional magnetic resonance imaging (fMRI) coupled with simple phrase (Nota and Honda, 2004), individual real word (Morgan et al., 2013), nonsense word (Pigdon et al., 2020) and syllable (Wildgruber et al., 2001; Riecker et al., 2005; Bohland and Guenther, 2006; Pützer et al., 2019) repetitions have assessed brain activity during speech production compared to either listening or resting baseline. Similar research investigating connectivity using fMRI and magnetoencephalography has highlighted the premotor cortex, orofacial regions of the motor cortex, medial and superior temporal cortices, cerebellum and IFG-L as key regions within the broader language production network (Kujala et al., 2006; Liljeström et al., 2015; Simonyan and Fuertinger, 2015). The orofacial motor cortex has been flagged as one of the most densely connected regions within the network, with the cerebellum also being mentioned as a highly connected area (Kujala et al., 2006; Simonyan and Fuertinger, 2015). Sensorimotor tasks activate the anterior cerebellum, while language-based tasks engage lobule VI and Crus I in the right cerebellar hemisphere (Stoodley and Schmahmann, 2009). Riecker et al. (2005) suggest the existence of two separate networks, one involved in motor preparation for speech and one for motor execution.
Speech is a known marker of neurological health, particularly in adult neurodegenerative conditions (Harris et al., 2019; Landin-Romero et al., 2021) but also increasingly seen as a promising marker of paediatric brain function (Morgan et al., 2016; Regier et al., 2016; Hiremath et al., 2021). Speech is a relatively straightforward and easy-to-collect behavioural output, making it a useful clinical marker across a range of conditions across the lifespan including autism spectrum disorders (Shriberg Lawrence et al., 2017; Talkar et al., 2020; Hiremath et al., 2021), mental health and psychotic disorders (Low et al., 2020; Koops et al., 2021). Speech has utility as a tool for differential diagnosis, and can be used to track change, as a response to treatment or disease progression (Morgan et al., 2013; Daudet et al., 2017; Chan et al., 2019; Noffs et al., 2020; Solomon Nancy et al., 2021).
To ensure robust cross-sectional individual participant or cohort findings and to enable longitudinal monitoring, it is crucial to understand the reproducibility of speech fMRI. There is currently no single definition of reproducibility used in scientific and clinical research. For this study, we use the definition of methods reproducibility outlined by Goodman et al. (2016): “the ability to implement, as exactly as possible, the experimental and computational procedures, with the same data and tools, to obtain the same results.” Typical group-level task-based fMRI analysis is generally considered to have a low test–retest reliability (Elliott et al., 2020; Noble et al., 2021). However, other research suggests that viewing all task-based fMRI as having poor reproducibility is overly simplistic and discounts studies showing higher reproducibility (Kragel et al., 2021). fMRI reproducibility appears to be highly variable and dependent on several factors, including the task, sample size, measures used, scanner noise and subject motion (Gorgolewski et al., 2013; Kragel et al., 2021). For example, a meta-analysis of fMRI task reproducibility reported intraclass correlation coefficients (ICCs) between 0.16 and 0.88 throughout the 13 included studies (Bennett and Miller, 2010). ICC looks at differences in brain activity between subjects or timepoints, and values range from −1 to 1. Scores <0 are indicative of no agreement between activation values at each timepoint and scores close to 1 show high agreement (Caceres et al., 2009; Nettekoven et al., 2018). Notably however, none of the studies in the meta-analysis involved speech. By contrast to these findings of non-linguistic tasks, language tasks including verb generation and sentence comprehension have shown high test–retest correlations, at least when assessing the lateralisation of language in the frontal and temporoparietal regions of the cerebral cortex, suggesting a high reproducibility of findings in this regard (Harrington et al., 2006). Further, Voyvodic (2012) used a sentence completion task to assess the reproducibility of language mapping fMRI in healthy participants. Using activation mapping as a percent of local excitation, the study found significant reproducible activation in the frontal and temporal regions (Voyvodic, 2012). Laterality and spatial extent of activation were found to have 95 and 55% similarity across time, respectively, (Voyvodic, 2012). Using both ICC and the Dice coefficient, Frankford et al. (2021) found high reproducibility of speech activation maps based on overt speech tasks using pseudoword and monosyllabic word repetition. The Dice coefficient describes the overlap of activation maps and is scored between 0 (no overlap) and 1 (complete overlap; Bennett and Miller, 2010; Frankford et al., 2021). Further, Nettekoven et al. (2018) found reliable activation during speech across time using a picture-naming task, focusing on IFG-L, Wernicke’s area (part of the left STG) and the primary motor cortex (Nettekoven et al., 2018). Similarly, a sentence completion task shows moderate reproducibility of activation in inferior frontal and temporo-parietal regions of the cerebrum (Elin et al., 2022). However, the mentioned studies have a cortical focus meaning other regions of the brain involved in motor speech production, including the cerebellum, were not highlighted in the research. Gorgolewski et al. (2013) found cerebellar activation in both a lip movement motor task and a word repetition task. The reproducibility findings from this study varied: the time-series correlations were low for both tasks, but moderate-to-high within-subject Dice overlap suggests higher reproducibility.
The present study used longitudinal fMRI (two timepoints, 6 weeks apart) to examine the brain networks involved in speech preparation and articulation during a word repetition task (Morgan et al., 2013) in healthy controls. We further aimed to determine the reproducibility of the fMRI signal during this task. The speech paradigm has previously been used in cross-sectional studies examining the neural structure of dysarthria following childhood brain injury and also in an inherited speech disorder, compared to healthy controls (Morgan et al., 2013; Liégeois et al., 2019), but not in healthy adults alone. Further, while a previous study included analysis on motor speech regions of the cerebral cortex (Frankford et al., 2021), it did not include non-cortical speech regions, nor were more complex words with more than two syllables presented in the speech tasks. This study is therefore novel in including whole brain analysis of motor speech related functional activation, and in using a speech paradigm with words of varying complexity, to assess motor speech fMRI reproducibility in healthy controls. We predicted that there would be significantly increased functional activation in the sensorimotor and speech regions of the cerebrum, as well as the putamen, thalamus, and cerebellum during speech production. Further, we hypothesised that, during speech preparation, we would see significant activation of IFG-L and the premotor cortex. We expected neural activation to remain relatively stable across the two time points, showing moderate reproducibility of the speech fMRI task.
Fifteen healthy volunteers aged 18–65 (45.4 ± 15.3y) participated in the study (75% female). These participants were right-handed and spoke English as their first language. The local Human Research Ethics Committee approved the study and all participants provided voluntary written consent. One participant was excluded from analysis due to significant head motion during scanning. Therefore, the final analysis included data from the remaining 14 participants.
A longitudinal, repeated measures design with two timepoints 6 weeks apart (44 ± 5 days) was carried out with a sample of volunteers. At timepoint 1, each participant underwent several assessments, including taking of general medical history, detailed neurological assessment, and completion of the Scale of the Assessment and Rating of Ataxia to assess cerebellar function. Upper limb dystonia was also assessed using the Global Dystonia Scale. All participants scored zero on these scales. At both timepoints, participants underwent MRI scanning.
At timepoint 1, participants underwent a 3 T MRI scanning session (MAGNETOM TrioTim, Siemens, Medical Systems, Erlangen, Germany) to obtain: (a) T1-weighted volumetric sequence (TR = 11.0 ms, TE = 705.0 ms, FOV = 1536*1536 mm2, matrix = 208*256, slice thickness = 8.0 mm, flip angle = 7.0°), (b) Echo gradient echo planar imaging (EPI) fMRI (TR = 1.5 ms, TE = 33 ms, FOV = 204*204 mm, matrix = 104*104, voxel size = 2.0 × 2.0 × 2.0 mm, slice thickness = 2 mm, flip angle = 85.0°, volumes = 200, GRAPPA acceleration factor of 2, multi-band slice acceleration factor of 3). Each EPI run involved administering the audio speech task once over 6 min, providing us with two EPI runs of the speech task at timepoint 1. At timepoint 2, participants had another fMRI run with the same settings. Using an MRI-compatible microphone, audio recordings of the speech task responses were acquired for each participant. The response timing was then used to identify functional activation during only the motor speech production aspect of the task at each timepoint.
Imaging data were collected while participants completed a six-minute speech production task with an event-related design. Participants listened to an audio recording that presented 30 single words, followed by the instruction to either “listen” (“listen” condition) or “repeat” (“prepare + speech” condition) the word, at 12 s intervals (see Figure 1). The 12 s period for each stimulus was designed to include 2.5 s for stimulus presentation, 2.0 s for participant response, and 7.5 s to capture peak haemodynamic response (Morgan et al., 2013). The speech task was repeated at each timepoint, with two EPI runs conducted each time as noted above. This provided us with two 12-min sets of data, each with 60 words. At both MRI data acquisition timepoints, both word and instruction order were pseudorandomised to improve internal consistency and minimise practice effects (Morgan et al., 2013; Appendix 1).
To determine speech task timing for analysis, we separated the two conditions of the task into three aspects: (1) “listen” when the participant listens to the word without having to repeat it, (2) “prepare” when the participant listens to the word that they must subsequently repeat, and (3) “speech” when the participant says the word out loud. “Listen” and “prepare” timing was set for each run of the speech task, and “speech” timing was determined manually from audio recordings in the scanner for each run for each participant. This timing was used to establish functional activity during the 1.5 s following the onset of either the task instruction (“listen” or “prepare”) or active speech production. We used FEAT v6.00 (FSL, FMRIB, Oxford, UK) to perform fMRI analyses. Raw fMRI scans were pre-processed to correct for head motion [MCFLIRT motion correction (Jenkinson et al., 2002)], spatially smoothed (4 mm extent threshold) and registered to the main structural image using boundary-based linear registration. The scans were also registered to standard MNI space using FNIRT nonlinear registration. Performing speech tasks during image acquisition can impact the activation signals of interest (Gracco, Tremblay & Pike, 2005). Given the nature of the speech task, we then used ICA AROMA to further correct for head movement (Pruim et al., 2015). The combination of MCFLIRT and ICA AROMA has previously been used to correct for speech-related motion artifacts (Janssen and Mendieta, 2020). At this point, one participant was excluded due to significant motion remaining after both standard and additional motion correction. We used multi-level general linear model (GLM) analysis to identify regions of significant activation. We combined the two runs at each timepoint then ran analyses separately for each participant at timepoint 1 (TIME1) and timepoint 2 (TIME2). This level also calculated the main effects and calculated the level and location of functional activation at the listen, prepare and speech timestamps over both TIME1 and TIME2. Results from this level were used for single subject reproducibility analysis. Higher-level analysis was then used to determine common areas of brain activation across participants. In this level, we assessed the activation at each timepoint separately, and combined all data to find the group average activation maps for each aspect of the speech task at both timepoints. We used a z-stat threshold of >3.1 to identify regions of significant activation during “listen,” “prepare,” and “speech.” This threshold was also used to correct cluster size familywise error at p < 0.05. Given this was an exploratory study, we used the average regions of activation during “speech” as regions of interest (ROI, see Figure 2 “speech” activation map). Using the Harvard-Oxford cortical and subcortical atlases for labelling purposes, we found activation cluster 1 in the right putamen, and clusters 2, 4 and 5 in the sensorimotor areas of the pre-and post-central gyri, primarily in orofacial regions. The cerebellar atlas in MNI152 space after normalisation with FNIRT was used to identify the significant cerebellar activation during speech in the right anterior cerebellar lobules IV and V (Diedrichsen et al., 2009). We then compared the magnitude of activation within these clusters during speech production across TIME1 and TIME2.
Figure 2. Average functional activation during each aspect of the audio speech task. Image created using MRIcron. Threshold z = 3.1.
To assess reproducibility, we compared speech-related functional activation within each average ROI at TIME1 with that at TIME2 (6 weeks later). We first used paired t-tests (p < 0.05) to determine whether there were any significant differences in activation between the timepoints. Reproducibility was then quantified using ICC [based on the 95th percentile, absolute agreement, 2-way mixed-effects model (Barry et al., 2016; Koo and Li, 2016)], and the average coefficient of variation (CoV). The 95th percentile was used for these analyses due to its equivalence with a significance level of p = 0.05 and to protect against plausible but false high single-voxel correlations (Barry et al., 2016, 2018; Paul et al., 2017; Turner et al., 2018).
For single subject ICCs, we created four masks – bilateral IFG and STG, subcortical (thalamus and putamen), and cerebellum, and compared the z-stat at the 95th percentile within each mask for each participant. For the CoV, we first calculated the means and standard deviations for each ROI between the two timepoints for each participant. Dividing the standard deviations by the means gave us the CoV for each ROI in each participant.
For the group ICC, we compared the z-stat at the 95th percentile for eachROI between TIME1 and TIME2. The group-level CoVs for each ROI was the average CoV across participants. We then assessed activation location similarity within the ROIs identified with the GLM between timepoints and quantified this using a Dice coefficient.
Our GLM analysed brain activity during the listen, prepare, and speech aspects of the task. Results show widespread activation during “listen” and “prepare,” with large amounts of overlap in activated areas, including the cerebellum and sensory, motor, and auditory regions of the cerebral cortex. Additionally, there was significant overlap in activated regions between “prepare” and “speech,” largely shown in the cerebellum and bilateral sensorimotor cortices. See Figure 2 for activation patterns.
While we looked at all three aspects of the task, we focused on “speech,” given it is the aspect specifically highlighting motor speech production. No significant differences were found between TIME1 and TIME2 for any aspect of the speech task. During “speech”, we identified five average regions of significant activation (z-score > 3.1) The ROIs for this analysis identified using the GLM included bilateral sensorimotor cortices, right putamen, and right anterior cerebellum. We additionally found location overlap between TIME1 and TIME2 in the right sensorimotor cortex and right anterior cerebellar ROIs. The Dice similarity coefficients were 0.005 and 0.085, respectively. All functional activation in the right sensorimotor cortex at TIME1 and TIME2 was within the right Brodmann area 3a. In the right anterior cerebellum, 96% of activated voxels at TIME1 and 86% of activated voxels at TIME2 were within the right lobule V. See Figures 2, 3 and Tables 1, 2 for further detail.
Figure 3. Activation within ROIs at timepoint 1 (red) and timepoint 2 (green). Overlap can be seen in yellow. (A) Axial view of functional activation during speech production. (B) Activation overlap within the R sensorimotor cortex ROI. (C) Activation overlap within the R anterior cerebellar ROI. Image created using MRIcron.
Table 2. Average regions of interest during “speech” and average activation of cluster coordinates in MNI space at peak activation.
Reproducibility was determined using ICC and CoV between TIME1 and TIME2. As determined by the t-test and comparison of functional activation within the GLM, there were no significant group differences in activation magnitude found between the two timepoints (see Table 2; Figure 4). Individual ICCs varied widely (see Table 3). The cerebellar ICCs were found to be significant (p = 0.038). Cortical and subcortical ICCs were not significant. Group ICC ranged from 0.002 to 0.499. Only the cerebellar activation cluster in the right lobules IV and V had a significant moderate correlation between TIME1 and TIME2, with an ICC of 0.499 (p = 0.003) and CoV of 30.4%. ICCs for activation within the other ROIs did not approach significance.
Figure 4. Level of activation during motor speech production in each ROI at timepoint 1 and at timepoint 2.
Table 3. Individual intraclass correlations for cerebellar, cortical, and subcortical speech regions.
This study examined functional characteristics of speech during a speech fMRI task in healthy controls and determined its reproducibility. Speech-related activation was observed in the right cerebellar lobules IV and V, and connected regions of the cerebrum involved in speech, including the right putamen and bilateral sensorimotor regions. These findings support our first hypothesis and earlier work describing cerebellar contributions to speech production. The right cerebellum is thought to play a key role in motor speech production, as there is greater contralateral connectivity between the motor cortex and the cerebellum than there is bilateral connectivity (Krienen and Buckner, 2009). This implies that during speech, where the left motor cortex is active, the right cerebellum should show higher levels of activity. While there is bilateral cerebellar activation during articulation, it is higher in both magnitude and extent in the right superior cerebellum (Ackermann et al., 1998; Chen and Desmond, 2005). Crus I and cerebellar lobule VI exhibit load-dependent activation with IFG-L during articulatory rehearsal tasks, with larger areas of activation in the right cerebellar hemisphere (Chen and Desmond, 2005). This explains our findings regarding activation in the right cerebellum during “speech” and the motor cortices during both “prepare” and “speech.”
In terms of the reproducibility of the task, we saw no significant group differences in functional activation between timepoints. Group level right anterior cerebellar activity during speech was somewhat consistent between the two timepoints, with a moderate ICC of 0.499. Individual subject ICCs varied widely, with significant cerebellar ICCs showing low to high reproducibility depending on the participant. No other individual ICCs were significant. These findings suggest reasonable reproducibility of cerebellar activation during this specific speech fMRI task at both an individual and group level. By contrast however, no cerebral activated regions at TIME1 showed a significant voxel-wise correlation with activity in the same regions at TIME2, either in individual or group level analysis. The Dice coefficients of overlapping regions of activation within these were also very low, indicating minimal thresholded activation pattern overlap. This is a critical finding and surprising given the higher level of reproducibility of cerebral activation seen to date in this field, even when using similar tasks (Voyvodic, 2012; Nettekoven et al., 2018; Elin et al., 2022). We note here that we used the z-score at the 95th percentile for ICC, due to its equivalence to a significance level of p = 0.05 (Barry et al., 2016; Paul et al., 2017; Turner et al., 2018). We do, however, acknowledge that there are other ways to investigate this, including taking the median or maximum contrast value, the contrast at peak activation, or the ICC between measurements (Raemaekers et al., 2007; Caceres et al., 2009). This variation in voxel-wise reproducibility between ROIs may be due to individual psychophysiological factors such as fatigue and attention, variance in noise in the fMRI signal, activation outliers, thresholding sensitivity, or practice effects and changes in task strategies between timepoints (Machielsen et al., 2000; Yoo et al., 2005). We must also address the potential influence of practice effects on functional activity at TIME2. Previous research using a picture naming task found a decrease in BOLD signal at the time of the second scan compared to the first (Basso et al., 2013). Though the difference in activation between timepoints was non-significant, this is what we observed (Figure 4). As an exploratory study, the ROIs used for this analysis were also based on average level of activation during speech in this cohort, and thus were quite small. This would also impact reproducibility. While we only see minor voxel-wise overlap in activation patterns between timepoints, we do see very close activation in both the right sensorimotor and right cerebellar ROIs. All functional activation within the right sensorimotor cortex at both timepoints was confined to Brodmann area 3a, providing evidence for higher levels of reproducibility than was captured using voxel-wise overlap analyses. Given the potential influence of head motion and position in the scanner on fMRI signal (Power et al., 2014), and the exploratory nature of this study, we suggest further research be conducted using larger ROIs chosen based on past findings to improve identification of motor-speech related functional activation and increase the accuracy of reproducibility measures. It may also be beneficial to use a sparse sampling design rather than an event-related design for the speech fMRI task. In saying that, Yoo et al. (2005) found that the cerebellum had the greatest activation volume overlap between intrasession scans during a motor task when compared to activation in the motor and supplementary cortices. Further, when looking at intersession scans, activation in both the cerebellum and motor regions of the cerebrum were reproducible (Yoo et al., 2005).
As regards to the three specific “listen,” “prepare,” and “speech” components of the task, temporal and frontal regions were activated during the “listen” and “prepare” segments. Temporal and frontal regions are involved in auditory processing (Schirmer et al., 2012; Lima et al., 2016) and speech comprehension (Yue et al., 2013), and are connected to the cerebellum (Buckner et al., 2011). Results also show a significant overlap in areas of activation between “prepare” and “speech,” particularly in the cerebellum and sensorimotor cortices in the cerebrum. This observation provides possible evidence of a priming effect in these regions before speech articulation. Moreover, we see activation of IFG-L during “prepare,” but not during “speech,” which suggests a priming effect during “prepare” and explains why we do not see significant IFG-L activation during “speech.” This is consistent with previous research on the role of IFG-L in speech preparation (Wildgruber et al., 1996; Watkins and Paus, 2004; Korzeniewska et al., 2011; Flinker et al., 2015).
The study had a small sample size. Recent research demonstrates that sample size can have a considerable impact on the reproducibility of task-based fMRI (Turner et al., 2018; Bossier et al., 2020), including a high false negative rate (Lohmann et al., 2017). As such, there were few findings that were reproducible at timepoint 2. This lack of reproducibility could potentially be addressed by repeating this experiment with a larger group of participants. We acknowledge that our small sample also has a wide age range and are aware of the potential effect of age on functional activity. A recent meta-analysis indicates altered functional activation related to motor control in older adults (Zapparoli et al., 2022). Moreover, Tremblay et al. (2017) suggest that changes in motor and executive control contribute to age differences in brain function associated with speech production. This age effect additionally may have impacted reproducibility through inter-subject variability. We also did not correct for multiple comparisons. As the study called for planned comparisons, we directly compared activation within each of our ROIs between timepoints individually. We were able to control for some variables including keeping the method and environment stable, yet other aspects of fMRI that may influence reproducibility are less modifiable. Potential variables include differences in heart rate or respiration between participants affecting the BOLD signal, and physiological noise such as underlying brain activity. Further, imaging artifacts such as machine noise and head movement are more pronounced during a speech task. While the analysis included standard motion correction and the use of ICA AROMA, more substantial head movement cannot be controlled for. This led to the exclusion of one participant, further decreasing sample size. There is also the possibility of behavioural differences between sessions impacting functional activation. Due to the words presented being the same at both timepoints and the sample consisting only of healthy controls, we did not take this into account for our study. However, for further research, particularly in clinical populations, we suggest adding behavioural data as a variable. The GLM and t-tests found no significant differences in functional activation between timepoints, thus implying high reproducibility. In contrast, the ICC appears to be much more sensitive to the thresholding, picking up smaller variations in voxel-to-voxel activation than the t-tests, leading to lower correlation between timepoints and thus lower implied reproducibility of the findings. Similarly, Dice coefficients are sensitive to both thresholding level and area of calculation, where lower threshold values and whole-brain analysis increase the overlap (Frankford et al., 2021). We used standard thresholding throughout this study, but only calculated Dice coefficients within ROIs, likely decreasing the level of overlap found and lowering the calculated reproducibility. Finally, the speech task used required the articulation of single words in a list and a natural speech sample may improve ecological validity.
Our findings further confirm that a simple fMRI speech task can allow observation of brain activity associated with motor speech preparation and production. We found significant activation in the right putamen, right anterior cerebellum, and bilateral sensorimotor cortices during speech production, in line with past work. Additionally, widespread activation through bilateral cerebellar, sensorimotor, premotor, and temporal regions was observed during “prepare,” consistent with speech perception and motor speech preparation.
Initial analyses reflected no significant differences in functional activity during speech production between our initial assessment and the second session after 6 weeks, which is suggestive of good reproducibility. Most critically, further statistical analyses showed only moderate reproducibility in cerebellar activation associated with motor speech production, and did not highlight other regions of activation as reproducible. This discrepancy is likely due to varying sensitivity to the thresholds used, but does emphasise the significant and consistent role of the cerebellum in motor speech activity. We suggest future research further investigate the potential of monitoring speech-related cerebellar activity specifically. Injury to the cerebellum can significantly impair speech, leading to a decline in quality of life (Walshe and Miller, 2011; Noffs et al., 2020). This is seen in people with neurodegenerative diseases (Piacentini et al., 2014; Lirani-Silva et al., 2015; Leite and Constantini, 2017; Noffs et al., 2018), stroke survivors (Spencer and Brown, 2018) and cases of traumatic brain injury (Gandhi et al., 2020). Further assessing this speech task’s psychometric properties could lead to its use for diagnosis of such conditions.
We can conclude that the word repetition task can assess activity in the cerebellum during motor speech preparation and production. While this is promising, further research is required to determine whether the task could be used to monitor any changes in cerebellar speech activity over time. Further, future research ought to investigate whether this task could be used as a speech marker of disease progression in diseases with significant cerebellar impact, such as multiple sclerosis or Parkinson’s disease (Noffs et al., 2018, 2020; Magee et al., 2019; Rusz et al., 2019).
The data analyzed in this study is subject to the following licenses/restrictions: data is available upon request. Requests to access these datasets should be directed to AnV, YW5uZWtlLnZhbmRlcndhbHRAbW9uYXNoLmVkdQ==.
The studies involving humans were approved by Monash University Human Research Ethics Committee. The studies were conducted in accordance with the local legislation and institutional requirements. The participants provided their written informed consent to participate in this study.
KK: Conceptualization, Formal analysis, Project administration, Validation, Visualization, Writing – original draft, Writing – review & editing. FB: Conceptualization, Data curation, Investigation, Resources, Supervision, Writing – review & editing. GN: Data curation, Formal analysis, Supervision, Writing – review & editing. AM: Methodology, Resources, Writing – review & editing. AdV: Software, Supervision, Writing – review & editing. SK: Conceptualization, Methodology, Resources, Supervision, Validation, Writing – review & editing. AnV: Conceptualization, Funding acquisition, Project administration, Resources, Supervision, Writing – review & editing.
The author(s) declare that financial support was received for the research, authorship, and/or publication of this article. Funding received from NHMRC Project Grant #1085461 AnV.
GN and AdV were employed by Redenlab Inc.
The remaining authors declare that the research was conducted in the absence of any commercial or financial relationships that could be construed as a potential conflict of interest.
The author(s) declared that they were an editorial board member of Frontiers, at the time of submission. This had no impact on the peer review process and the final decision.
All claims expressed in this article are solely those of the authors and do not necessarily represent those of their affiliated organizations, or those of the publisher, the editors and the reviewers. Any product that may be evaluated in this article, or claim that may be made by its manufacturer, is not guaranteed or endorsed by the publisher.
The Supplementary material for this article can be found online at: https://www.frontiersin.org/articles/10.3389/fnhum.2024.1382102/full#supplementary-material
Ackermann, H., Wildgruber, D., Daum, I., and Grodd, W. (1998). Does the cerebellum contribute to cognitive aspects of speech production? A functional magnetic resonance imaging (fMRI) study in humans. Neurosci. Lett. 247, 187–190. doi: 10.1016/S0304-3940(98)00328-0
ASHA (2020). What is speech? What is language? Available at: https://www.asha.org/public/speech/development/speech-and-language/ (Accessed January 11, 2024).
Barry, R. L., Conrad, B. N., Smith, S. A., and Gore, J. C. (2018). A practical protocol for measurements of spinal cord functional connectivity. Sci. Rep. 8:16512. doi: 10.1038/s41598-018-34841-6
Barry, R. L., Rogers, B. P., Conrad, B. N., Smith, S. A., and Gore, J. C. (2016). Reproducibility of resting state spinal cord networks in healthy volunteers at 7 Tesla. NeuroImage 133, 31–40. doi: 10.1016/j.neuroimage.2016.02.058
Basso, G., Magon, S., Reggiani, F., Capasso, R., Monittola, G., Yang, F.-J., et al. (2013). Distinguishable neurofunctional effects of task practice and item practice in picture naming: A BOLD fMRI study in healthy subjects. Brain Lang. 126, 302–313. doi: 10.1016/j.bandl.2013.07.002
Bennett, C. M., and Miller, M. B. (2010). How reliable are the results from functional magnetic resonance imaging? Ann. N. Y. Acad. Sci. 1191, 133–155. doi: 10.1111/j.1749-6632.2010.05446.x
Bohland, J. W., and Guenther, F. H. (2006). An fMRI investigation of syllable sequence production. NeuroImage 32, 821–841. doi: 10.1016/j.neuroimage.2006.04.173
Bossier, H., Roels, S. P., Seurinck, R., Banaschewski, T., Barker, G. J., Bokde, A. L. W., et al. (2020). The empirical replicability of task-based fMRI as a function of sample size. NeuroImage 212:116601. doi: 10.1016/j.neuroimage.2020.116601
Brown, S., Laird, A. R., Pfordresher, P. Q., Thelen, S. M., Turkeltaub, P., and Liotti, M. (2009). The somatotopy of speech: Phonation and articulation in the human motor cortex. Brain Cogn. 70, 31–41. doi: 10.1016/j.bandc.2008.12.006
Brown, S., Yuan, Y., and Belyk, M. (2021). Evolution of the speech-ready brain: The voice/jaw connection in the human motor cortex. J. Comp. Neurol. 529, 1018–1028. doi: 10.1002/cne.24997
Buckner, R. L., Krienen, F. M., Castellanos, A., Diaz, J. C., and Yeo, B. T. T. (2011). The organization of the human cerebellum estimated by intrinsic functional connectivity. J. Neurophysiol. 106, 2322–2345. doi: 10.1152/jn.00339.2011
Caceres, A., Hall, D. L., Zelaya, F. O., Williams, S. C. R., and Mehta, M. A. (2009). Measuring fMRI reliability with the intra-class correlation coefficient. NeuroImage 45, 758–768. doi: 10.1016/j.neuroimage.2008.12.035
Chan, J. C., Stout, J. C., and Vogel, A. P. (2019). Speech in prodromal and symptomatic Huntington’s disease as a model of measuring onset and progression in dominantly inherited neurodegenerative diseases. Neurosci. Biobehav. Rev. 107, 450–460. doi: 10.1016/j.neubiorev.2019.08.009
Chen, S. H. A., and Desmond, J. E. (2005). Cerebrocerebellar networks during articulatory rehearsal and verbal working memory tasks. NeuroImage 24, 332–338. doi: 10.1016/j.neuroimage.2004.08.032
Correia, J. M., Caballero-Gaudes, C., Guediche, S., and Carreiras, M. (2020). Phonatory and articulatory representations of speech production in cortical and subcortical fMRI responses. Sci. Rep. 10:4529. doi: 10.1038/s41598-020-61435-y
Daudet, L., Yadav, N., Perez, M., Poellabauer, C., Schneider, S., and Huebner, A. (2017). Portable mTBI Assessment Using Temporal and Frequency Analysis of Speech. IEEE J. Biomed. Health Inform. 21, 496–506. doi: 10.1109/JBHI.2016.2633509
Diedrichsen, J., Balsters, J. H., Flavell, J., Cussans, E., and Ramnani, N. (2009). A probabilistic MR atlas of the human cerebellum. NeuroImage 46, 39–46. doi: 10.1016/j.neuroimage.2009.01.045
Elin, K., Malyutina, S., Bronov, O., Stupina, E., Marinets, A., Zhuravleva, A., et al. (2022). A new functional magnetic resonance imaging localizer for preoperative language mapping using a sentence completion task: validity, choice of baseline condition, and test-retest reliability. Front. Hum. Neurosci. 16:791577. doi: 10.3389/fnhum.2022.791577
Elliott, M. L., Knodt, A. R., Ireland, D., Morris, M. L., Poulton, R., Ramrakha, S., et al. (2020). What is the test-retest reliability of common task-functional MRI measures? new empirical evidence and a meta-analysis. Psychol. Sci. 31, 792–806. doi: 10.1177/0956797620916786
Flinker, A., Korzeniewska, A., Shestyuk, A. Y., Franaszczuk, P. J., Dronkers, N. F., Knight, R. T., et al. (2015). Redefining the role of Broca’s area in speech. Proc. Natl. Acad. Sci. 112, 2871–2875. doi: 10.1073/pnas.1414491112
Frankford, S. A., Nieto-Castañón, A., Tourville, J. A., and Guenther, F. H. (2021). Reliability of single-subject neural activation patterns in speech production tasks. Brain Lang. 212:104881. doi: 10.1016/j.bandl.2020.104881
Gandhi, P., Tobin, S., Vongphakdi, M., Copley, A., and Watter, K. (2020). A scoping review of interventions for adults with dysarthria following traumatic brain injury. Brain Inj. 34, 466–479. doi: 10.1080/02699052.2020.1725844
Goodman, S. N., Fanelli, D., and Ioannidis, J. P. A. (2016). What does research reproducibility mean? Sci. Transl. Med. 8, 341ps12–341ps12. doi: 10.1126/scitranslmed.aaf5027
Gorgolewski, K. J., Storkey, A. J., Bastin, M. E., Whittle, I., and Pernet, C. (2013). Single subject fMRI test–retest reliability metrics and confounding factors. NeuroImage 69, 231–243. doi: 10.1016/j.neuroimage.2012.10.085
Gracco, V. L., Tremblay, P., and Pike, B. (2005). Imaging speech production using fMRI. NeuroImage 26, 294–301. doi: 10.1016/j.neuroimage.2005.01.033
Guenther, F. H. (2006). Cortical interactions underlying the production of speech sounds. J. Commun. Disord. 39, 350–365. doi: 10.1016/j.jcomdis.2006.06.013
Harrington, G. S., Buonocore, M. H., and Farias, S. T. (2006). Intrasubject reproducibility of functional MR imaging activation in language tasks. AJNR Am. J. Neuroradiol. 27, 938–944.Available at: http://www.ajnr.org/content/27/4/938
Harris, J. M., Saxon, J. A., Jones, M., Snowden, J. S., and Thompson, J. C. (2019). Neuropsychological differentiation of progressive aphasic disorders. J. Neuropsychol. 13, 214–239. doi: 10.1111/jnp.12149
Hiremath, C. S., Sagar, K. J. V., Yamini, B. K., Girimaji, A. S., Kumar, R., Sravanti, S. L., et al. (2021). Emerging behavioral and neuroimaging biomarkers for early and accurate characterization of autism spectrum disorders: a systematic review. Transl. Psychiatry 11:42. doi: 10.1038/s41398-020-01178-6
Janssen, N., and Mendieta, C. C. R. (2020). The Dynamics of Speech Motor Control Revealed with Time-Resolved fMRI. Cereb. Cortex 30, 241–255. doi: 10.1093/cercor/bhz084
Jenkinson, M., Bannister, P., Brady, M., and Smith, S. (2002). Improved optimization for the robust and accurate linear registration and motion correction of brain images. NeuroImage 17, 825–841. doi: 10.1006/nimg.2002.1132
Jouen, A. L., Lancheros, M., and Laganaro, M. (2021). Microstate ERP analyses to pinpoint the articulatory onset in speech production. Brain Topogr. 34, 29–40. doi: 10.1007/s10548-020-00803-3
Koo, T. K., and Li, M. Y. (2016). A guideline of selecting and reporting intraclass correlation coefficients for reliability research. J. Chiropr. Med. 15, 155–163. doi: 10.1016/j.jcm.2016.02.012
Koops, S., Brederoo, S. G., de Boer, J. N., Nadema, F. G., Voppel, A. E., and Sommer, I. E. (2021). Speech as a biomarker for depression. CNS Neurol. Dis. Drug Targets 22, 152–160. doi: 10.2174/1871527320666211213125847
Korzeniewska, A., Franaszczuk, P. J., Crainiceanu, C. M., Kuś, R., and Crone, N. E. (2011). Dynamics of large-scale cortical interactions at high gamma frequencies during word production: Event related causality (ERC) analysis of human electrocorticography (ECoG). NeuroImage 56, 2218–2237. doi: 10.1016/j.neuroimage.2011.03.030
Kragel, P. A., Han, X., Kraynak, T. E., Gianaros, P. J., and Wager, T. D. (2021). Functional MRI can be highly reliable, but it depends on what you measure: a commentary on Elliott et al. (2020). Psychol. Sci. 32, 622–626. doi: 10.1177/0956797621989730
Krienen, F. M., and Buckner, R. L. (2009). Segregated fronto-cerebellar circuits revealed by intrinsic functional connectivity. Cereb. Cortex 19, 2485–2497. doi: 10.1093/cercor/bhp135
Kujala, J., Pammer, K., Cornelissen, P., Roebroeck, A., Formisano, E., and Salmelin, R. (2006). Phase coupling in a cerebro-cerebellar network at 8–13 Hz during reading. Cereb. Cortex 17, 1476–1485. doi: 10.1093/cercor/bhl059
Landin-Romero, R., Liang, C. T., Monroe, P. A., Higashiyama, Y., Leyton, C. E., Hodges, J. R., et al. (2021). Brain changes underlying progression of speech motor programming impairment. Brain Commun. 3:fcab 205. doi: 10.1093/braincomms/fcab205
Leite, L., and Constantini, A. C. (2017). Dysarthria and quality of life in patients with amyotrophic lateral sclerosis. Rev. CEFAC 19, 664–673. doi: 10.1590/1982-021620171954017
Liégeois, F. J., Turner, S. J., Mayes, A., Bonthrone, A. F., Boys, A., Smith, L., et al. (2019). Dorsal language stream anomalies in an inherited speech disorder. Brain 142, 966–977. doi: 10.1093/brain/awz018
Liljeström, M., Stevenson, C., Kujala, J., and Salmelin, R. (2015). Task-and stimulus-related cortical networks in language production: Exploring similarity of MEG-and fMRI-derived functional connectivity. NeuroImage 120, 75–87. doi: 10.1016/j.neuroimage.2015.07.017
Lima, C. F., Krishnan, S., and Scott, S. K. (2016). Roles of supplementary motor areas in auditory processing and auditory imagery. Trends Neurosci. 39, 527–542. doi: 10.1016/j.tins.2016.06.003
Lirani-Silva, C., Mourão, L. F., and Gobbi, L. T. B. (2015). Dysarthria and quality of life in neurologically healthy elderly and patients with Parkinson's disease. Paper presented at the CoDAS, 27, 248, 254.
Lohmann, G., Stelzer, J., Müller, K., Lacosse, E., Buschmann, T., Kumar, V. J., et al. (2017). Inflated false negative rates undermine reproducibility in task-based fMRI. bioRxiv :122788. doi: 10.1101/122788
Low, D. M., Bentley, K. H., and Ghosh, S. S. (2020). Automated assessment of psychiatric disorders using speech: a systematic review. Laryngoscope Investig. Otolaryngol. 5, 96–116. doi: 10.1002/lio2.354
Machielsen, W. C., Rombouts, S. A., Barkhof, F., Scheltens, P., and Witter, M. P. (2000). FMRI of visual encoding: reproducibility of activation. Hum. Brain Mapp. 9, 156–164. doi: 10.1002/(sici)1097-0193(200003)9:3<156::aid-hbm4>3.0.co;2-q
Magee, M., Copland, D., and Vogel, A. P. (2019). Motor speech and non-motor language endophenotypes of Parkinson's disease. Expert. Rev. Neurother. 19, 1191–1200. doi: 10.1080/14737175.2019.1649142
Mooshammer, C., Goldstein, L., Nam, H., McClure, S., Saltzman, E., and Tiede, M. (2012). Bridging planning and execution: temporal planning of syllables. J. Phon. 40, 374–389. doi: 10.1016/j.wocn.2012.02.002
Morgan, A., Bonthrone, A., and Liégeois, F. J. (2016). Brain basis of childhood speech and language disorders: are we closer to clinically meaningful MRI markers? Curr. Opin. Pediatr. 28, 725–730. doi: 10.1097/mop.0000000000000420
Morgan, M., Pigdon, C., and Liégeois,. (2013). Functional magnetic resonance imaging of chronic dysarthric speech after childhood brain injury: reliance on a left-hemisphere compensatory network. Brain 136, 646–657. doi: 10.1093/brain/aws355
Nettekoven, C., Reck, N., Goldbrunner, R., Grefkes, C., and Weiß Lucas, C. (2018). Short-and long-term reliability of language fMRI. NeuroImage 176, 215–225. doi: 10.1016/j.neuroimage.2018.04.050
Noble, S., Scheinost, D., and Constable, R. T. (2021). A guide to the measurement and interpretation of fMRI test-retest reliability. Curr. Opin. Behav. Sci. 40, 27–32. doi: 10.1016/j.cobeha.2020.12.012
Noffs, G., Boonstra, F. M. C., Perera, T., Butzkueven, H., Kolbe, S. C., Maldonado, F., et al. (2020). Speech metrics, general disability, brain imaging and quality of life in MS. Eur. J. Neurol. 28, 259–268. doi: 10.1111/ene.14523
Noffs, G., Perera, T., Kolbe, S. C., Shanahan, C. J., Boonstra, F. M. C., Evans, A., et al. (2018). What speech can tell us: A systematic review of dysarthria characteristics in Multiple Sclerosis. Autoimmun. Rev. 17, 1202–1209. doi: 10.1016/j.autrev.2018.06.010
Nota, Y., and Honda, K. (2004). Brain regions involved in motor control of speech. Acoust. Sci. Technol. 25, 286–289. doi: 10.1250/ast.25.286
Paul, E. J., Turner, B., Miller, M. B., and Barbey, A. K. (2017). How sample size influences the reproducibility of task-based fMRI. bioRxiv :136259.
Piacentini, V., Mauri, I., Cattaneo, D., Gilardone, M., Montesano, A., and Schindler, A. (2014). Relationship Between Quality of Life and Dysarthria in Patients With Multiple Sclerosis. Arch. Phys. Med. Rehabil. 95, 2047–2054. doi: 10.1016/j.apmr.2014.04.023
Pigdon, L., Willmott, C., Reilly, S., Conti-Ramsden, G., Liegeois, F., Connelly, A., et al. (2020). The neural basis of nonword repetition in children with developmental speech or language disorder: An fMRI study. Neuropsychologia 138:107312. doi: 10.1016/j.neuropsychologia.2019.107312
Power, J. D., Mitra, A., Laumann, T. O., Snyder, A. Z., Schlaggar, B. L., and Petersen, S. E. (2014). Methods to detect, characterize, and remove motion artifact in resting state fMRI. NeuroImage 84, 320–341. doi: 10.1016/j.neuroimage.2013.08.048
Price, C. J. (2012). A review and synthesis of the first 20years of PET and fMRI studies of heard speech, spoken language and reading. NeuroImage 62, 816–847. doi: 10.1016/j.neuroimage.2012.04.062
Pruim, R. H. R., Mennes, M., van Rooij, D., Llera, A., Buitelaar, J. K., and Beckmann, C. F. (2015). ICA-AROMA: A robust ICA-based strategy for removing motion artifacts from fMRI data. NeuroImage 112, 267–277. doi: 10.1016/j.neuroimage.2015.02.064
Pützer, M., Moringlane, J. R., Sikos, L., Reith, W., and Krick, C. M. (2019). fMRI and acoustic analyses reveal neural correlates of gestural complexity and articulatory effort within bilateral inferior frontal gyrus during speech production. Neuropsychologia 132:107129. doi: 10.1016/j.neuropsychologia.2019.107129
Raemaekers, M., Vink, M., Zandbelt, B., van Wezel, R. J. A., Kahn, R. S., and Ramsey, N. F. (2007). Test–retest reliability of fMRI activation during prosaccades and antisaccades. NeuroImage 36, 532–542. doi: 10.1016/j.neuroimage.2007.03.061
Regier, D. S., Kwon, H. J., Johnston, J., Golas, G., Yang, S., Wiggs, E., et al. (2016). MRI/MRS as a surrogate marker for clinical progression in GM1 gangliosidosis. Am. J. Med. Genet. A 170, 634–644. doi: 10.1002/ajmg.a.37468
Riecker, A., Mathiak, K., Wildgruber, D., Erb, M., Hertrich, I., Grodd, W., et al. (2005). fMRI reveals two distinct cerebral networks subserving speech motor control. Neurology 64, 700–706. doi: 10.1212/01.Wnl.0000152156.90779.89
Riès, S. K., Dhillon, R. K., Clarke, A., King-Stephens, D., Laxer, K. D., Weber, P. B., et al. (2017). Spatiotemporal dynamics of word retrieval in speech production revealed by cortical high-frequency band activity. Proc. Natl. Acad. Sci. 114, E4530–E4538. doi: 10.1073/pnas.1620669114
Rusz, J., Tykalová, T., Salerno, G., Bancone, S., Scarpelli, J., and Pellecchia, M. T. (2019). Distinctive speech signature in cerebellar and parkinsonian subtypes of multiple system atrophy. J. Neurol. 266, 1394–1404. doi: 10.1007/s00415-019-09271-7
Schaeffler, S., Scobbie, J. M., and Schaeffler, F. (2014). Measuring reaction times: vocalisation vs. articulation.
Schirmer, A., Fox, P. M., and Grandjean, D. (2012). On the spatial organization of sound processing in the human temporal lobe: a meta-analysis. NeuroImage 63, 137–147. doi: 10.1016/j.neuroimage.2012.06.025
Shriberg Lawrence, D., Strand Edythe, A., Fourakis, M., Jakielski Kathy, J., Hall Sheryl, D., Karlsson Heather, B., et al. (2017). A diagnostic marker to discriminate childhood apraxia of speech from speech delay: IV. the pause marker index. J. Speech Lang. Hear. Res. 60, S1153–S1169. doi: 10.1044/2016_JSLHR-S-16-0149
Simonyan, K., and Fuertinger, S. (2015). Speech networks at rest and in action: interactions between functional brain networks controlling speech production. J. Neurophysiol. 113, 2967–2978. doi: 10.1152/jn.00964.2014
Solomon Nancy, P., Brungart Douglas, S., Wince Jessica, R., Abramowitz Jordan, C., Eitel Megan, M., Cohen, J., et al. (2021). Syllabic Diadochokinesis in Adults With and Without Traumatic Brain Injury: Severity, Stability, and Speech Considerations. Am. J. Speech Lang. Pathol. 30, 1400–1409. doi: 10.1044/2020_AJSLP-20-00158
Spencer, K. A., and Brown, K. A. (2018). Dysarthria following stroke. Paper presented at the Seminars in speech and language.
Stoodley, C. J., and Schmahmann, J. D. (2009). Functional topography in the human cerebellum: A meta-analysis of neuroimaging studies. NeuroImage 44, 489–501. doi: 10.1016/j.neuroimage.2008.08.039
Talkar, W., Hannon, R., Yuditskaya, C., et al. (2020). Assessment of speech and fine motor coordination in children with autism spectrum disorder. IEEE Access 8, 127535–127545. doi: 10.1109/ACCESS.2020.3007348
Tremblay, P., Sato, M., and Deschamps, I. (2017). Age differences in the motor control of speech: an fMRI study of healthy aging. Hum. Brain Mapp. 38, 2751–2771. doi: 10.1002/hbm.23558
Turner, B. O., Paul, E. J., Miller, M. B., and Barbey, A. K. (2018). Small sample sizes reduce the replicability of task-based fMRI studies. Commun. Biol. 1:62. doi: 10.1038/s42003-018-0073-z
Voyvodic, J. T. (2012). Reproducibility of single-subject fMRI language mapping with AMPLE normalization. J. Mag. Resonance Imaging 36, 569–580. doi: 10.1002/jmri.23686
Walshe, M., and Miller, N. (2011). Living with acquired dysarthria: the speaker's perspective. Disabil. Rehabil. 33, 195–203. doi: 10.3109/09638288.2010.511685
Watkins, K., and Paus, T. (2004). Modulation of motor excitability during speech perception: the role of broca's area. J. Cogn. Neurosci. 16, 978–987. doi: 10.1162/0898929041502616
Wildgruber, D., Ackermann, H., and Grodd, W. (2001). Differential contributions of motor cortex, basal ganglia, and cerebellum to speech motor control: effects of syllable repetition rate evaluated by fMRI. NeuroImage 13, 101–109. doi: 10.1006/nimg.2000.0672
Wildgruber, A., Klose, K., and Grodd,. (1996). Functional lateralization of speech production at primary motor cortex: a fMRI study. Neuroreport 7, 2791–2796. doi: 10.1097/00001756-199611040-00077
Yoo, S.-S., Wei, X., Dickey, C. C., Guttmann, C. R. G., and Panych, L. P. (2005). Long-term reproducibility analysis of fmri using hand motor task. Int. J. Neurosci. 115, 55–77. doi: 10.1080/00207450490512650
Yue, Q., Zhang, L., Xu, G., Shu, H., and Li, P. (2013). Task-modulated activation and functional connectivity of the temporal and frontal areas during speech comprehension. Neuroscience 237, 87–95. doi: 10.1016/j.neuroscience.2012.12.067
Keywords: speech production, reproducibility, fMRI, cerebellum, neurolinguistics
Citation: Kenyon KH, Boonstra F, Noffs G, Morgan AT, Vogel AP, Kolbe S and Van Der Walt A (2024) The characteristics and reproducibility of motor speech functional neuroimaging in healthy controls. Front. Hum. Neurosci. 18:1382102. doi: 10.3389/fnhum.2024.1382102
Received: 05 February 2024; Accepted: 22 July 2024;
Published: 07 August 2024.
Edited by:
Peter Sörös, University of Oldenburg, GermanyReviewed by:
Carolin Weiss Lucas, University Hospital of Cologne, GermanyCopyright © 2024 Kenyon, Boonstra, Noffs, Morgan, Vogel, Kolbe and Van Der Walt. This is an open-access article distributed under the terms of the Creative Commons Attribution License (CC BY). The use, distribution or reproduction in other forums is permitted, provided the original author(s) and the copyright owner(s) are credited and that the original publication in this journal is cited, in accordance with accepted academic practice. No use, distribution or reproduction is permitted which does not comply with these terms.
*Correspondence: Katherine H. Kenyon, a2F0aGVyaW5lLmtlbnlvbkBtb25hc2guZWR1
Disclaimer: All claims expressed in this article are solely those of the authors and do not necessarily represent those of their affiliated organizations, or those of the publisher, the editors and the reviewers. Any product that may be evaluated in this article or claim that may be made by its manufacturer is not guaranteed or endorsed by the publisher.
Research integrity at Frontiers
Learn more about the work of our research integrity team to safeguard the quality of each article we publish.