- 1Department of Psychology, University of Wisconsin–Madison, Madison, WI, United States
- 2Department of Psychological and Brain Sciences, Consortium for Interacting Minds, Dartmouth College, Hanover, NH, United States
- 3Santa Fe Institute, Santa Fe, NM, United States
The human eye is a rich source of information about where, when, and how we attend. Our gaze paths indicate where and what captures our attention, while changes in pupil size can signal surprise, revealing our expectations. Similarly, the pattern of our blinks suggests levels of alertness and when our attention shifts between external engagement and internal thought. During interactions with others, these cues reveal how we coordinate and share our mental states. To leverage these insights effectively, we need accurate, timely methods to observe these cues as they naturally unfold. Advances in eye-tracking technology now enable real-time observation of these cues, shedding light on mutual cognitive processes that foster shared understanding, collaborative thought, and social connection. This brief review highlights these advances and the new opportunities they present for future research.
1 Introduction
Social interactions form the bedrock of human experience, shaping our emotions, beliefs, and behaviors while fostering a sense of belonging and purpose (Cacioppo and Patrick, 2008). But the mechanics of this crucial behavior remain a mystery: How does the brain activity of one individual influence the brain activity of another, enabling the transfer of thoughts, feelings, and beliefs? Moreover, how do interacting minds create new ideas that cannot be traced back to either individual alone? Only recently has science begun to tackle these fundamental questions, utilizing new methods that can trace the complex dynamics of minds that adapt to each other (Risko et al., 2016). Still far from mainstream (Schilbach et al., 2013; Schilbach, 2016), the science of interacting minds is an growing field. This growth is driven by the realization that relying solely on static models and single-participant studies has constrained our understanding of the human mind (Wheatley et al., 2023).
Tackling these core questions of mind-to-mind influence presents significant challenges. The seemingly effortless and common nature of interaction masks its underlying complexity (Garrod and Pickering, 2004). Even the simplest interaction involves multiple communication channels, leading to the continuous reshaping of thought (Shockley et al., 2009). Commensurate with this complexity, many methodologies struggle to capture the ongoing, reciprocal dynamics. The restrictive environments of neuroimaging machines and concerns of motion artifact (Fargier et al., 2018) make scanning interacting minds challenging (Pinti et al., 2020) while behavioral studies can be laborious and challenging to scale. Other physiological signals, such as heart rate, offer valuable insights into the synchrony between people, a phenomenon that may be amplified by mutual attention (for example, individuals listening to the same story may experience synchronized heart rate variations (Pérez et al., 2021). Nonetheless, these indicators often fall short in providing detailed insights into how individuals coordinate their attention with one another from one moment to the next.
One technique has increasingly surmounted these challenges: eye-tracking. While not explicitly neuroscientific in traditional terms, pupil dilations under consistent lighting are tightly correlated with activity in the brain's locus coeruleus (Rajkowski, 1993; Aston-Jones et al., 1994), the neural hub integral to attention. Fluctuations in pupil size track the release of norepinephrine, providing a temporally sensitive measure of when attention is modulated (Joshi et al., 2016). Further, gaze direction and blink rate offer their own insights into what people find interesting and how they shift their attention between internal thought and the external world. This one technique thus produces multiple sources of information about the mind that are temporally sensitive and can be monitored passively without affecting the unfolding of natural responses.
Interpersonal eye-tracking—eye tracking with two or more individuals—captures the moment-by-moment attentional dynamics as people interact. This technique is already bearing fruit. For example, research has demonstrated its use in detecting the emergence of mutual understanding. When people attend in the same way, their pupil dilations synchronize providing a visual cue of minds in sync (Kang and Wheatley, 2017; Nencheva et al., 2021). Similarly, correspondence between people's gaze trajectories, blink rates and eye contact provide additional cues that reveal how minds interact (Richardson et al., 2007; Nakano, 2015; Capozzi and Ristic, 2022; Mayrand et al., 2023). With the emergence of wearable devices, such as eye-tracking glasses, we can more easily monitor these cues as they unfold naturally during social interactions in ways that are portable across diverse settings, demand minimal setup, and are scalable to larger groups. Coupled with new, innovative analytical techniques, these recent advances have made eye-tracking a portable, inexpensive, temporally precise, and efficient tool for addressing fundamental questions about the bidirectional neural influence of interaction and how these processes may differ in populations that find communication challenging (e.g., Autism Spectrum Conditions). In this mini review, we briefly describe the evolution of this technique and its promise for deepening our understanding of human sociality.
2 Major advances in eye-tracking
The first eye-trackers were designed as stationary machines, with a participant's head stabilized by a chin rest or bite-bar, restricting movement and field of view (Hartridge and Thomson, 1948; Mackworth and Mackworth, 1958; Płużyczka and Warszawski, 2018). Later, head-mounted eye-tracking cameras were developed (Mackworth and Thomas, 1962) but remained burdensome, restrictive, and required prolonged and frequent calibration, making them unsuitable for the study of social interaction (Hornof and Halverson, 2002). In recent years, eye-tracking technology has witnessed a rapid evolution. In this section, we will highlight some of these recent developments and explore how they have transformed interpersonal eye-tracking into an indispensable resource for understanding social interaction.
Recent technological progress has enabled the eye-tracking of dyads and groups without disrupting their complex exchange of communicative signals. For example, software innovations now automate calibration (e.g., Kassner et al., 2014), synchronize data from multiple devices (e.g., openSync; Razavi et al., 2022) and simplify the analysis of eye-tracking data collected in naturalistic settings (e.g., iMotions, 2022). These breakthroughs streamline device setup, eliminate the need for intrusive recalibrations, and facilitate analysis of gaze and pupil data in real-time. Packages developed within Python (e.g., PyTrack; Ghose et al., 2020), Matlab (e.g., PuPl; Kinley and Levy, 2022; e.g., CHAP; Hershman et al., 2019), and R (e.g., gazeR; Geller et al., 2020) also streamline preprocessing and analysis of eye movement and pupillometry data. Eye-tracking glasses, designed to be worn like regular glasses, afford a wider range of motion, affording natural facial expressions (Valtakari et al., 2021) and gestures, such as the frequent head nods that regulate interaction (McClave, 2000).
New analysis methods for continuous data are better equipped to handle non-linearity and non-stationarity, making them invaluable for quantifying the real-time interplay between the eyes of interacting dyads and groups. For example, Dynamic Time Warping (Berndt and Clifford, 1994) is a non-linear method often used in speech recognition software for aligning time-shifted signals. This method is useful for capturing alignment in social interactions in a way that accounts for noisy, high-resolution data, leader follower dynamics, or other natural features of social interactions where alignment is present but not precisely time-locked. Recent research has employed this method to measure synchrony between two continuous pupillary time series (a measure of shared attention—Kang and Wheatley, 2017; Nencheva et al., 2021; Fink et al., 2023) as people interact (see Section 3 for a detailed description of this phenomenon). Cross-recurrence quantification analysis (Zbilut et al., 1998) quantifies the shared dynamics between two systems, determining lag and identifying leaders and followers during interactions via their gaze behavior (Fusaroli et al., 2014). Advanced methods now allow scientists to analyze the interactions between multiple individuals' eye-tracking data. Multi-Level Vector Auto-Regression (Epskamp et al., 2024) estimates multiple networks of relationships between time series variables, where variables are nodes in the network and edges represent correlations between variables. This method has been used to quantify the relationships between gaze fixation duration and dispersion (Moulder et al., 2022) as well as eye contact, pupil size, and pupillary synchrony (Wohltjen and Wheatley, 2021). Other advanced methods include cross-correlation and reverse correlation (Brinkman et al., 2017), Detrended Fluctuation Analysis (Peng et al., 1994), and deconvolution (Wierda et al., 2012), with the number of analysis techniques continually increasing.
Software is continually improving and open-source, making synchronization of recordings from different eye-trackers more efficient. When analyzing the correspondence between multiple eye-tracking time series, many different methods exist that can account for the non-linearity of eye-tracking data and leverage the multiple measurements that the device captures. These advances have made it relatively simple to collect and analyze eye-tracking data from multiple interacting people across diverse settings.
3 What can we learn from interpersonal eye-tracking?
Modern eye-trackers capture several physiological correlates of social attention, including gaze trajectories, pupil dilations, and blink behavior (Figure 1). In this section, we outline how interpersonal eye-tracking leverages these signals to reveal the coordinated dynamics of interacting minds.
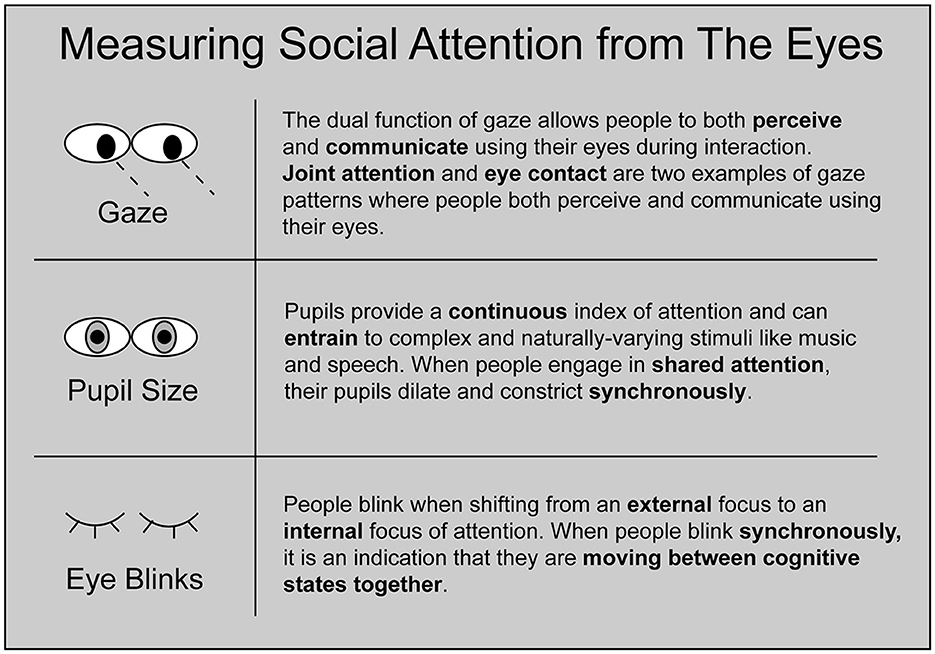
Figure 1. Schematic of three forms of social attention that can be measured using interpersonal eye-tracking. Gaze, pupil size, and eye blinks each reveal unique information about how people dynamically coordinate their attention with each other.
3.1 Interpersonal gaze: coordinating the “where” of attention
The focus of one's gaze has long been understood as a “spotlight” of attention (Posner et al., 1980), revealing what a person finds most informative about a scene. During social interaction, gaze is often concentrated on the interaction partner's eyes and mouth (Rogers et al., 2018). By virtue of this association, gaze also communicates the focus of one's attention to others (Argyle and Dean, 1965; Gobel et al., 2015).
This dual function of gaze—perception and communication—results in well-established social gaze patterns such as joint attention (Gobel et al., 2015). In joint attention, one person uses their gaze to communicate where to look, at which point their partner follows their gaze so that both people are perceiving the same thing (Scaife and Bruner, 1975). Joint attention emerges early in life and is critical for the development of neural systems that support social cognition (Mundy, 2017). Another common gaze pattern, mutual gaze (or eye contact), involves a simultaneous process of signaling and perceiving by both parties, embodying a dual role of giving and receiving within the same action (Heron, 1970). Interpersonal eye-tracking provides a unique opportunity to measure both the perceptual and communicative functions of gaze, simultaneously, as they unfold during real interactions. In this vein, a recent study demonstrated that eye contact made people more attentive to the gaze patterns of their conversation partners (Mayrand et al., 2023) and predicted greater across-brain coherence in social brain structures (Hirsch et al., 2017; Dravida et al., 2020). Coupled gaze patterns between conversation partners is associated with their amount of shared knowledge about the conversation topic at hand (Richardson et al., 2007). Thus, attention to another's gaze and the coupling of those gaze patterns appears to indicate engagement and shared understanding.
3.2 Interpersonal pupil dilations: tracking when minds are “in sync”
Under consistent lighting, pupil dilations are tightly correlated with the release of norepinephrine in the brain's locus coeruleus (Rajkowski, 1993; Aston-Jones et al., 1994; Joshi et al., 2016), the neural hub integral to attention. When presented with a periodic visual (Naber et al., 2013) or auditory (Fink et al., 2018) stimulus, the pupil dilates and constricts in tandem with the stimulus presentation, which is thought to be the product of prediction (Schwiedrzik and Sudmann, 2020). Pupillary entrainment can also occur with more complex and naturally varying stimuli such as music (Kang et al., 2014; Kang and Banaji, 2020) or speech (Kang and Wheatley, 2017).
When people attend to the same dynamic stimulus in the same way, their pupil dilations synchronize, providing a visual indicator of synchronized minds (Kang and Wheatley, 2015; Nencheva et al., 2021; Fink et al., 2023). For example, when two people listen to the same story, their pupils may constrict and dilate in synchrony indicating that they are similarly anticipating, moment by moment, what will happen next. Synchronized attention, often referred to as “shared attention,” has wide-ranging social benefits including social verification (i.e., the sense that one's subjective reality is validated by virtue of it being shared; Hardin and Higgins, 1996; Echterhoff et al., 2009), heightened perspective-taking (Smith and Mackie, 2016), better memory (Eskenazi et al., 2013; He et al., 2014), and a feeling of social connection (Cheong et al., 2023). This form of synchrony does not require interaction, it is simply a dynamic measurement of how similarly people attend to the same stimulus. It can be measured even when individuals are eye-tracked on separate occasions and are unable to see each other, ruling out pupil mimicry as an underlying cause (Prochazkova et al., 2018).
Recent research investigating pupillary synchrony has uncovered new insights about shared attention. For example, comparing the similarity of toddlers' pupillary dilations as they listened to a story told with child-directed vs. adult-directed speech intonation, Nencheva et al. (2021) found that toddlers had more similar pupillary dilation patterns when hearing child-directed speech, suggesting that it helped entrain their attention. In a conversation study using pupillary synchrony as a metric of shared attention, researchers found that eye contact marked when shared attention rose and fell. Specifically, eye contact occurred as interpersonal pupillary synchrony peaked, at which point synchrony progressively declined until eye contact was broken (Wohltjen and Wheatley, 2021). This suggests that eye contact may communicate high shared attention but also may disrupt that shared focus, possibly to allow for the emergence of independent thinking necessary for conversation to evolve. This may help explain why conversations that are more engaging tend to have more eye contact (Argyle and Dean, 1965; Mazur et al., 1980; Jarick and Bencic, 2019; Dravida et al., 2020).
3.3 Interpersonal blink rate: marking changes in cognitive states
When measuring continuous gaze and pupil size, the signal will often be momentarily lost. These moments, caused by blinks, are commonly discarded from eye-tracking analyses yet their timing and frequency are non-random and offer their own clues about the mind (Hershman et al., 2018).
People spontaneously blink every 3 s on average, more than what is necessary for lubricating the eyes (Doane, 1980). Furthermore, the rate of spontaneous eye blinking varies with cognitive states. It changes when chunking information (Stern et al., 1984) or when attending to the rhythmic sequence of presented tones (Huber et al., 2022). Blink rate decreases as attentional demands grow and increases with boredom (Maffei and Angrilli, 2018). However, blink rate also increases with indications of engagement, such as arousal (Stern et al., 1984; Bentivoglio et al., 1997) and attentional switching (Rac-Lubashevsky et al., 2017). Although it seems paradoxical to blink more when bored and when engaged, these findings are explained by the role of blinking in the various tasks in which it has been measured. Blinks are related to increased default mode, hippocampal, and cerebellar activity and decreased dorsal and ventral attention network activity. This suggests that blinks may facilitate the transition between outward and inward states of focus (Nakano et al., 2013; Nakano, 2015). As a result, people might blink more frequently when they feel bored due to periodic disengagement, oscillating between focusing on the external environment and their internal thoughts. Additionally, increased blinking occurs in activities requiring regular alternation between external and internal attention, like when participating in conversation (Bentivoglio et al., 1997).
Scientists have discovered intriguing patterns in how people coordinate their eye blinks during interactions. Cummins (2012) observed that individuals strategically adjust their blink rates during conversations based on their partners' gaze direction, indicating shifts between internal and external attention. Moreover, researchers have found that people tend to synchronize their blinks with others during problem-solving tasks (Hoffmann et al., 2023) and conversations (Nakano and Kitazawa, 2010; Gupta et al., 2019), reflecting mutual transitions between cognitive states. Similarly, Nakano and Miyazaki (2019) noted that people who found videos engaging blinked in sync, suggesting shared processing of the content. These studies demonstrate how blinks can signify when interaction partners collectively shift between cognitive states.
Interpersonal eye-tracking records multiple dynamic features that each yield unique insights about how attention is dynamically coordinated and communicated when minds interact (Richardson et al., 2007; Nakano, 2015; Capozzi and Ristic, 2022; Mayrand et al., 2023). Blinking, eye gaze, and pupillary synchrony each reflect dissociable aspects of social attention (see Figure 1). However, these components likely complement and dynamically interact with each other to support social engagement. For example, when pupillary synchrony between conversation partners peaks, eye contact occurs. Coincident with the onset of eye contact, pupillary synchrony declines until eye contact breaks (Wohltjen and Wheatley, 2021). This precise temporal relationship between gaze and pupillary synchrony highlights the importance of combining these measures to shed light on how these components work together as an integrated system.
4 Future directions in interpersonal eye-tracking
As interpersonal eye-tracking technology continues to advance, many long-standing questions about interacting minds are newly tractable. In this section, we discuss some of these open research areas, highlighting the untapped potential of interpersonal eye-tracking for the future of social scientific research.
4.1 Testing existing theories in ecologically-valid scenarios
Social interaction is immensely complex and difficult to study in controlled laboratory conditions. The ecological validity afforded by interpersonal eye-tracking allows researchers to test how human minds naturally coordinate their attention in ways that afford the sharing and creation of knowledge (Kingstone et al., 2003; Risko et al., 2016). This additional ecological validity is instrumental in discerning the generalizability of psychological theories developed in tightly controlled conditions.
Three recent examples from eye-tracking research highlight potential discrepancies between controlled and naturalistic paradigms. First, a substantial body of research utilizing static images of faces has consistently shown that East Asian participants tend to avoid looking at the eye region more than their Western counterparts (Blais et al., 2008; Akechi et al., 2013; Senju et al., 2013). However, when examined within the context of live social interactions, a striking reversal of this pattern emerges (Haensel et al., 2022). Second, interpersonal eye-tracking has shown that people engage in significantly less mutual gaze than traditional non-naturalistic paradigms would predict. This behavior likely stems from individuals' reluctance to appear as though they are fixating on their interaction partner, a concern that is absent when looking at static images (Laidlaw et al., 2016; Macdonald and Tatler, 2018). Third, interpersonal eye-tracking has revealed how social context can change gaze behavior. For example, when pairs of participants were assigned roles in a collaborative task (e.g., “chef” and “gatherer” for baking), they looked at each more and aligned their gaze faster than pairs who were not assigned roles (Macdonald and Tatler, 2018), suggesting that social roles may help coordinate attention. In the domain of autism research, there has been ongoing debate regarding how autistic individuals use nonverbal cues, such as eye contact. Interpersonal eye-tracking has been proposed to mitigate this lack of consensus by placing experiments in more ecologically valid, interactive scenarios (Laskowitz et al., 2021). With its potential for portability and ease of use, interpersonal eye-tracking offers new opportunities to test existing psychological theories and generate new insights.
Interpersonal eye-tracking also affords direct, side-by-side comparisons of attentional dynamics between tightly controlled lab conditions and more ecologically robust (but less controlled) contexts. For example, Wohltjen et al. (2023) directly compared an individual's attention to rigidly spaced single tones with how well that individual shares attention with a storyteller. Individuals whose pupils tended to synchronize with the tones were also more likely to synchronize their pupil dilations with those of the storyteller. This suggests that the tendency to synchronize one's attention is a reliable individual difference that varies in the human population, manifests across levels of complexity (from highly structured to continuously-varying dynamics) and predicts synchrony between minds.
4.2 Tracking moment-to-moment fluctuations in coupled attention
In social interactions, behaviors are dynamic, constantly adjusting to the evolving needs of one's partner and the surrounding context. Techniques that sample a behavior sparsely in time, aggregate over time, or record from a single individual in a noninteractive setting, miss important information relevant to interaction. An illustration of this issue can be found in the synchrony literature, which has long emphasized the benefits of synchrony for successful communication, shared understanding, and many other positive outcomes (Wheatley et al., 2012; Hasson and Frith, 2016; Launay et al., 2016; Mogan et al., 2017). Recent research using interpersonal eye-tracking suggests that synchrony is not always beneficial. Rather, intermittently breaking synchrony appears to be equally important (Dahan et al., 2016; Wohltjen and Wheatley, 2021; Ravreby et al., 2022). Mayo and Gordon (2020) suggest that the tendencies to synchronize with one another as well as act independently both exist during social interaction, and that flexibly moving between these two states is the hallmark of a truly adaptive social system. It is possible that several conversational mechanisms prompt this mental state-switching (e.g., topic changes; Egbert, 1997), turn taking (David Mortensen, 2011), and segments of conversation that communicate complete thoughts or Turn Construction Units (Sacks et al., 1978; Clayman, 2012). Future work should investigate how fluctuations of pupillary synchrony, eye blinks and other nonverbal cues help coordinate the coupling-decoupling dynamics between minds that optimize the goals of social interaction.
4.3 Tracking the coordinated dynamics of groups
Interpersonal eye-tracking research has traditionally concentrated on dyadic interactions, but the introduction of cost-effective wearable eye-tracking devices has ushered in new possibilities for exploring the intricacies of social interactions within both small and larger groups (Pfeiffer et al., 2013; Cañigueral and Hamilton, 2019; Mayrand et al., 2023). For example, when studying group dynamics, wearable devices allow for the spontaneous head and body movements that naturally occur when interacting with multiple people, such as turning one's head to orient to the current speaker. Recent studies employing wearable or mobile eye-tracking technology in group settings have demonstrated a nuanced interplay of gaze direction, shared attention, and the exchange of nonverbal communication cues (Capozzi et al., 2019; Maran et al., 2021; Capozzi and Ristic, 2022). These studies suggest that interactions are not only about where individuals look but also about the timing and duration of their gaze shifts. Moreover, these studies have highlighted the profound role of gaze as a potent social tool that contributes to the establishment of rapport (Mayrand et al., 2023), the facilitation of group cohesion (Capozzi and Ristic, 2022), and the negotiation of social hierarchies (Capozzi et al., 2019). By increasingly extending the scope of interpersonal eye-tracking research beyond dyads, we stand to gain a more comprehensive understanding of the full spectrum of human social dynamics.
5 Discussion
Social interaction is a remarkably intricate process that involves the integration of numerous continuous streams of information. Our comprehension of this crucial behavior has been limited by historical and methodological constraints that have made it challenging to study more than one individual at a time. However, recent advances in eye-tracking technology have revolutionized our ability to measure interactions with high temporal precision, in natural social settings, and in ways that are scalable from dyads to larger groups. The term “eye tracking” belies the wealth of data these devices capture. Parameters such as gaze direction, pupillary dynamics, and blinks each offer unique insights into the human mind. In combination, these metrics shed light on how minds work together to facilitate the sharing and co-creation of thought.
Interpersonal eye-tracking provides exciting opportunities for clinical and educational applications. For example, the relatively low-cost, ease of setup and ability to capture attentional dynamics unobtrusively during interaction make interpersonal eye tracking a promising clinical tool for studying communication difficulties in neurodiverse populations, such as people with Autism Spectrum Conditions (Laskowitz et al., 2021). A recent meta-analysis found that pupil responses in ASC have longer latencies (de Vries et al., 2021), with implications for coordination dynamics in turn-taking. Further, gaze patterns are also diagnostic of ASC from infancy (Zwaigenbaum et al., 2005; Chawarska et al., 2012), with implications for how gaze regulates social interaction (Cañigueral and Hamilton, 2019). Eye-tracking research has also shown that people with aphasia have language comprehension deficits that are partially explained by difficulties in dynamically allocating attention (Heuer and Hallowell, 2015). By using eye-tracking to pinpoint the moments in natural social interaction that increase attention demands, we can learn how social interaction may be adjusted to aid people with attentional difficulties. Interpersonal eye-tracking also has clear implications for understanding how teacher-student and peer-to-peer interactions scaffold learning (e.g., Dikker et al., 2017). We are excited by the accelerating pace of eye-tracking research in naturalistic social interactions that promise to extend our understanding of these and other important domains.
It is important to note that all methods have limitations and eye-tracking is no exception. For instance, changes in pupil size can signal activation in the locus coeruleus, associated with increased attention (Aston-Jones and Cohen, 2005). Yet, this measurement does not clarify which cognitive function benefits from this attentional “gain” (Aston-Jones and Cohen, 2005). Pinpointing a particular mental process or semantic representation, would require incorporating other behavioral assessments, establish comparison conditions, or techniques with higher spatial resolution, such as fMRI. Another challenge arises with the use of wearable eye-tracking technology. While these devices mitigate the issue of signal loss caused by natural head movements, they cannot eliminate it entirely. The freedom of head movement that wearable devices allow can also complicate the interpretation of gaze patterns (such as fixations, quick eye movements, or smooth following movements; Hessels et al., 2020) because gaze is tracked in relation to the head's position.
Despite these challenges, both wearable and stationary eye-tracking technologies offer valuable insights on how people coordinate their attention in real time. The continuous recording of pupil dilations, gaze direction, and blink rate sheds light on the ways that minds mutually adapt, facilitating the exchange of knowledge, shared understanding, and social bonding. By capturing the attentional dynamics of interacting minds, interpersonal eye-tracking offers a unique window into the mechanisms that scaffold social interaction.
Author contributions
SW: Conceptualization, Writing – original draft, Writing – review & editing. TW: Conceptualization, Funding acquisition, Resources, Writing – original draft, Writing – review & editing.
Funding
The author(s) declare that no financial support was received for the research, authorship, and/or publication of this article.
Conflict of interest
The authors declare that the research was conducted in the absence of any commercial or financial relationships that could be construed as a potential conflict of interest.
Publisher's note
All claims expressed in this article are solely those of the authors and do not necessarily represent those of their affiliated organizations, or those of the publisher, the editors and the reviewers. Any product that may be evaluated in this article, or claim that may be made by its manufacturer, is not guaranteed or endorsed by the publisher.
References
Akechi, H., Senju, A., Uibo, H., Kikuchi, Y., Hasegawa, T., Hietanen, J. K., et al. (2013). Attention to eye contact in the West and East: autonomic responses and evaluative ratings. PLoS ONE 8:e59312. doi: 10.1371/journal.pone.0059312
Argyle, M., and Dean, J. (1965). Eye-contact, distance and affiliation. Sociometry 28, 289–304. doi: 10.2307/2786027
Aston-Jones, G., and Cohen, J. D. (2005). An integrative theory of locus coeruleus-norepinephrine function: adaptive gain and optimal performance. Annu. Rev. Neurosci. 28, 403–450. doi: 10.1146/annurev.neuro.28.061604.135709
Aston-Jones, G., Rajkowski, J., Kubiak, P., and Alexinsky, T. (1994). Locus coeruleus neurons in monkey are selectively activated by attended cues in a vigilance task. J. Neurosci. 14, 4467–4480. doi: 10.1523/JNEUROSCI.14-07-04467.1994
Bentivoglio, A. R., Bressman, S. B., Cassetta, E., Carretta, D., Tonali, P., Albanese, A., et al. (1997). Analysis of blink rate patterns in normal subjects. Mov. Disord. 12, 1028–1034. doi: 10.1002/mds.870120629
Berndt, D. J., and Clifford, J. (1994). Using dynamic time warping to find patterns in time series. KDD Workshop 10, 359–370.
Blais, C., Jack, R. E., Scheepers, C., Fiset, D., and Caldara, R. (2008). Culture shapes how we look at faces. PLoS ONE 3:e3022. doi: 10.1371/journal.pone.0003022
Brinkman, L., Todorov, A., and Dotsch, R. (2017). Visualising mental representations: a primer on noise-based reverse correlation in social psychology. Eur. Rev. Soc. Psychol. 28, 333–361. doi: 10.1080/10463283.2017.1381469
Cacioppo, J. T., and Patrick, W. (2008). Loneliness: Human Nature and the Need for Social Connection. New York, NY: W. W. Norton and Company.
Cañigueral, R., and Hamilton, A. F. C. (2019). The role of eye gaze during natural social interactions in typical and autistic people. Front. Psychol. 10:560. doi: 10.3389/fpsyg.2019.00560
Capozzi, F., Beyan, C., Pierro, A., Koul, A., Murino, V., Livi, S., et al. (2019). Tracking the leader: gaze behavior in group interactions. iScience 16, 242–249. doi: 10.1016/j.isci.2019.05.035
Capozzi, F., and Ristic, J. (2022). Attentional gaze dynamics in group interactions. Vis. Cogn. 30, 135–150. doi: 10.1080/13506285.2021.1925799
Chawarska, K., Macari, S., and Shic, F. (2012). Context modulates attention to social scenes in toddlers with autism. J. Child Psychol. Psychiatry 53, 903–913. doi: 10.1111/j.1469-7610.2012.02538.x
Cheong, J. H., Molani, Z., Sadhukha, S., and Chang, L. (2023). Synchronized affect in shared experiences strengthens social connection. Commun. Biol. 6:1099. doi: 10.1038/s42003-023-05461-2
Clayman, S. E. (2012). “Turn-constructional units and the transition-relevance place,” in The Handbook of Conversation Analysis, eds J. Sidnell, and? T. Stivers (Hoboken, NJ: John Wiley and Sons, Ltd), 151–166. doi: 10.1002/9781118325001.ch8
Cummins, F. (2012). Gaze and blinking in dyadic conversation: a study in coordinated behaviour among individuals. Lang. Cogn. Processes 27, 1525–1549. doi: 10.1080/01690965.2011.615220
Dahan, A., Noy, L., Hart, Y., Mayo, A., and Alon, U. (2016). Exit from synchrony in joint improvised motion. PLoS ONE 11:e0160747. doi: 10.1371/journal.pone.0160747
de Vries, L., Fouquaet, I., Boets, B., Naulaers, G., and Steyaert, J. (2021). Autism spectrum disorder and pupillometry: a systematic review and meta-analysis. Neurosci. Biobehav. Rev. 120, 479–508. doi: 10.1016/j.neubiorev.2020.09.032
Dikker, S., Wan, L., Davidesco, I., Kaggen, L., Oostrik, M., McClintock, J., et al. (2017). Brain-to-brain synchrony tracks real-world dynamic group interactions in the classroom. Curr. Biol. 27, 1375–1380. doi: 10.1016/j.cub.2017.04.002
Doane, M. G. (1980). Interactions of eyelids and tears in corneal wetting and the dynamics of the normal human eyeblink. Am. J. Ophthalmol. 89, 507–516. doi: 10.1016/0002-9394(80)90058-6
Dravida, S., Noah, J. A., Zhang, X., and Hirsch, J. (2020). Joint attention during live person-to-person contact activates rTPJ, including a sub-component associated with spontaneous eye-to-eye contact. Front. Hum. Neurosci. 14:201. doi: 10.3389/fnhum.2020.00201
Echterhoff, G., Higgins, E. T., and Levine, J. M. (2009). Shared reality: experiencing commonality with others' inner states about the world. Perspect. Psychol. Sci. 4, 496–521. doi: 10.1111/j.1745-6924.2009.01161.x
Egbert, M. M. (1997). Schisming: the collaborative transformation from a single conversation to multiple conversations. Res. Lang. Soc. Interact. 30, 1–51. doi: 10.1207/s15327973rlsi3001_1
Epskamp, S., Deserno, M. K., and Bringmann, L. F. (2024). mlVAR: Multi-level Vector Autoregression. R Package Version 0.5.2. Available online at: https://CRAN.R-project.org/package=mlVAR (accessed February 27, 2024).
Eskenazi, T., Doerrfeld, A., Logan, G. D., Knoblich, G., and Sebanz, N. (2013). Your words are my words: effects of acting together on encoding. Q. J. Exp. Physiol. 66, 1026–1034. doi: 10.1080/17470218.2012.725058
Fargier, R., Bürki, A., Pinet, S., Alario, F.-X., and Laganaro, M. (2018). Word onset phonetic properties and motor artifacts in speech production EEG recordings. Psychophysiology 55, 1–10. doi: 10.1111/psyp.12982
Fink, L., Simola, J., Tavano, A., Lange, E., Wallot, S., Laeng, B., et al. (2023). From pre-processing to advanced dynamic modeling of pupil data. Behav Res Methods. doi: 10.3758/s13428-023-02098-1
Fink, L. K., Hurley, B. K., Geng, J. J., and Janata, P. (2018). A linear oscillator model predicts dynamic temporal attention and pupillary entrainment to rhythmic patterns. J. Eye Mov. Res. 11, 1–24. doi: 10.16910/jemr.11.2.12
Fusaroli, R., Konvalinka, I., and Wallot, S. (2014). Analyzing social interactions: the promises and challenges of using cross recurrence quantification analysis. Transl. Recurrences 137−155. doi: 10.1007/978-3-319-09531-8_9
Garrod, S., and Pickering, M. J. (2004). Why is conversation so easy? Trends Cogn. Sci. 8, 8–11. doi: 10.1016/j.tics.2003.10.016
Geller, J., Winn, M. B., Mahr, T., and Mirman, D. (2020). GazeR: a package for processing gaze position and pupil size data. Behav. Res. Methods 52, 2232–2255. doi: 10.3758/s13428-020-01374-8
Ghose, U., Srinivasan, A. A., Boyce, W. P., Xu, H., and Chng, E. S. (2020). PyTrack: an end-to-end analysis toolkit for eye tracking. Behav. Res. Methods 52, 2588–2603. doi: 10.3758/s13428-020-01392-6
Gobel, M. S., Kim, H. S., and Richardson, D. C. (2015). The dual function of social gaze. Cognition 136, 359–364. doi: 10.1016/j.cognition.2014.11.040
Gupta, A., Strivens, F. L., Tag, B., Kunze, K., and Ward, J. A. (2019). “Blink as you sync: uncovering eye and nod synchrony in conversation using wearable sensing,” in Proceedings of the 2019 ACM International Symposium on Wearable Computers (New York, NY: ACM), 66–71. doi: 10.1145/3341163.3347736
Haensel, J. X., Smith, T. J., and Senju, A. (2022). Cultural differences in mutual gaze during face-to-face interactions: a dual head-mounted eye-tracking study. Vis. Cogn. 30, 100–115. doi: 10.1080/13506285.2021.1928354
Hardin, C. D., and Higgins, E. T. (1996). “Shared reality: how social verification makes the subjective objective,” in Handbook of Motivation and Cognition, Vol. 3, ed. R. M. Sorrentino (New York, NY: The Guilford Press), 28–84, xxvi.
Hartridge, H., and Thomson, L. C. (1948). Methods of investigating eye movements. Br. J. Ophthalmol. 32, 581–591. doi: 10.1136/bjo.32.9.581
Hasson, U., and Frith, C. D. (2016). Mirroring and beyond: coupled dynamics as a generalized framework for modelling social interactions. Philos. Trans. R. Soc. Lond. B: Biol. Sci. 371:20150366. doi: 10.1098/rstb.2015.0366
He, X., Sebanz, N., Sui, J., and Humphreys, G. W. (2014). Individualism-collectivism and interpersonal memory guidance of attention. J. Exp. Soc. Psychol. 54, 102–114. doi: 10.1016/j.jesp.2014.04.010
Heron, J. (1970). The phenomenology of social encounter: the gaze. Philos. Phenomenol. Res. 31, 243–264. doi: 10.2307/2105742
Hershman, R., Henik, A., and Cohen, N. (2018). A novel blink detection method based on pupillometry noise. Behav. Res. Methods 50, 107–114. doi: 10.3758/s13428-017-1008-1
Hershman, R., Henik, A., and Cohen, N. (2019). CHAP: open-source software for processing and analyzing pupillometry data. Behav. Res. Methods 51, 1059–1074. doi: 10.3758/s13428-018-01190-1
Hessels, R. S., Niehorster, D. C., Holleman, G. A., Benjamins, J. S., and Hooge, I. T. C. (2020). Wearable technology for “real-world research”: realistic or not? Perception 49, 611–615. doi: 10.1177/0301006620928324
Heuer, S., and Hallowell, B. (2015). A novel eye-tracking method to assess attention allocation in individuals with and without aphasia using a dual-task paradigm. J. Commun. Disord. 55, 15–30. doi: 10.1016/j.jcomdis.2015.01.005
Hirsch, J., Zhang, X., Noah, J. A., and Ono, Y. (2017). Frontal temporal and parietal systems synchronize within and across brains during live eye-to-eye contact. Neuroimage 157, 314–330. doi: 10.1016/j.neuroimage.2017.06.018
Hoffmann, A., Schellhorn, A.-M., Ritter, M., Sachse, P., and Maran, T. (2023). Blink synchronization increases over time and predicts problem-solving performance in virtual teams. Small Group Res. 1–23. doi: 10.1177/10464964231195618
Hornof, A. J., and Halverson, T. (2002). Cleaning up systematic error in eye-tracking data by using required fixation locations. Beh. Res. Methods Instrum. Comput. 34, 592–604. doi: 10.3758/BF03195487
Huber, S. E., Martini, M., and Sachse, P. (2022). Patterns of eye blinks are modulated by auditory input in humans. Cognition 221:104982. doi: 10.1016/j.cognition.2021.104982
Jarick, M., and Bencic, R. (2019). Eye contact is a two-way street: arousal is elicited by the sending and receiving of eye gaze information. Front. Psychol. 10:1262. doi: 10.3389/fpsyg.2019.01262
Joshi, S., Li, Y., Kalwani, R. M., and Gold, J. I. (2016). Relationships between pupil diameter and neuronal activity in the locus coeruleus, colliculi, and cingulate cortex. Neuron 89, 221–234. doi: 10.1016/j.neuron.2015.11.028
Kang, O., and Banaji, M. R. (2020). Pupillometric decoding of high-level musical imagery. Conscious. Cogn. 77:102862. doi: 10.1016/j.concog.2019.102862
Kang, O., and Wheatley, T. (2015). Pupil dilation patterns reflect the contents of consciousness. Conscious. Cogn. 35, 128–135. doi: 10.1016/j.concog.2015.05.001
Kang, O., and Wheatley, T. (2017). Pupil dilation patterns spontaneously synchronize across individuals during shared attention. J. Exp. Psychol. Gen. 146, 569–576. doi: 10.1037/xge0000271
Kang, O. E., Huffer, K. E., and Wheatley, T. P. (2014). Pupil dilation dynamics track attention to high-level information. PLoS ONE 9:e102463. doi: 10.1371/journal.pone.0102463
Kassner, M., Patera, W., and Bulling, A. (2014). “Pupil: an open source platform for pervasive eye tracking and mobile gaze-based interaction,” in Proceedings of the 2014 ACM International Joint Conference on Pervasive and Ubiquitous Computing: Adjunct Publication (New York, NY: ACM), 1151–1160. doi: 10.1145/2638728.2641695
Kingstone, A., Smilek, D., Ristic, J., Kelland Friesen, C., and Eastwood, J. D. (2003). Attention, researchers! It is time to take a look at the real world. Curr. Dir. Psychol. Sci. 12, 176–180. doi: 10.1111/1467-8721.01255
Kinley, I., and Levy, Y. (2022). PuPl: an open-source tool for processing pupillometry data. Behav. Res. Methods 54, 2046–2069. doi: 10.3758/s13428-021-01717-z
Laidlaw, K. E. W., Risko, E. F., and Kingstone, A. (2016). “Levels of complexity and the duality of gaze: how social attention changes from lab to life,” in Shared Representations: Sensorimotor Foundations of Social Life, eds S. S. Obhi, and E. S. Cross (Cambridge: Cambridge University Press), 195–215. doi: 10.1017/CBO9781107279353.011
Laskowitz, S., Griffin, J. W., Geier, C. F., and Scherf, K. S. (2021). Cracking the code of live human social interactions in autism: a review of the eye-tracking literature. Proc. Mach. Learn. Res. 173, 242–264.
Launay, J., Tarr, B., and Dunbar, R. I. M. (2016). Synchrony as an adaptive mechanism for large-scale human social bonding. Ethology 122, 779–789. doi: 10.1111/eth.12528
Macdonald, R. G., and Tatler, B. W. (2018). Gaze in a real-world social interaction: a dual eye-tracking study. Q. J. Exp. Physiol. 71, 2162–2173. doi: 10.1177/1747021817739221
Mackworth, J. F., and Mackworth, N. H. (1958). Eye fixations recorded on changing visual scenes by the television eye-marker. J. Opt. Soc. Am. 48, 439–445. doi: 10.1364/JOSA.48.000439
Mackworth, N. H., and Thomas, E. L. (1962). Head-mounted eye-marker camera. J. Opt. Soc. Am. 52, 713–716. doi: 10.1364/JOSA.52.000713
Maffei, A., and Angrilli, A. (2018). Spontaneous eye blink rate: an index of dopaminergic component of sustained attention and fatigue. Int. J. Psychophysiol. 123, 58–63. doi: 10.1016/j.ijpsycho.2017.11.009
Maran, T., Furtner, M., Liegl, S., Ravet-Brown, T., Haraped, L., Sachse, P., et al. (2021). Visual attention in real-world conversation: gaze patterns are modulated by communication and group size. Appl. Psychol. 70, 1602–1627. doi: 10.1111/apps.12291
Mayo, O., and Gordon, I. (2020). In and out of synchrony-Behavioral and physiological dynamics of dyadic interpersonal coordination. Psychophysiology 57:e13574. doi: 10.1111/psyp.13574
Mayrand, F., Capozzi, F., and Ristic, J. (2023). A dual mobile eye tracking study on natural eye contact during live interactions. Sci. Rep. 13:11385. doi: 10.1038/s41598-023-38346-9
Mazur, A., Rosa, E., Faupel, M., Heller, J., Leen, R., Thurman, B., et al. (1980). Physiological aspects of communication via mutual gaze. Am. J. Sociol. 86, 50–74. doi: 10.1086/227202
McClave, E. Z. (2000). Linguistic functions of head movements in the context of speech. J. Pragmat. 32, 855–878. doi: 10.1016/S0378-2166(99)00079-X
Mogan, R., Fischer, R., and Bulbulia, J. A. (2017). To be in synchrony or not? A meta-analysis of synchrony's effects on behavior, perception, cognition and affect. J. Exp. Soc. Psychol. 72, 13–20. doi: 10.1016/j.jesp.2017.03.009
Moulder, R. G., Duran, N. D., and D'Mello, S. K. (2022). “Assessing multimodal dynamics in multi-party collaborative interactions with multi-level vector autoregression,” in Proceedings of the 2022 International Conference on Multimodal Interaction (New York, NY: ACM), 615–625. doi: 10.1145/3536221.3556595
Mundy, P. (2017). A review of joint attention and social-cognitive brain systems in typical development and autism spectrum disorder. Eur. J. Neurosci. 47, 497–514. doi: 10.1111/ejn.13720
Naber, M., Alvarez, G. A., and Nakayama, K. (2013). Tracking the allocation of attention using human pupillary oscillations. Front. Psychol. 4:919. doi: 10.3389/fpsyg.2013.00919
Nakano, T. (2015). Blink-related dynamic switching between internal and external orienting networks while viewing videos. Neurosci. Res. 96, 54–58. doi: 10.1016/j.neures.2015.02.010
Nakano, T., Kato, M., Morito, Y., Itoi, S., and Kitazawa, S. (2013). Blink-related momentary activation of the default mode network while viewing videos. Proc. Nat. Acad. Sci. 110, 702–706. doi: 10.1073/pnas.1214804110
Nakano, T., and Kitazawa, S. (2010). Eyeblink entrainment at breakpoints of speech. Exp. Brain Res. 205, 577–581. doi: 10.1007/s00221-010-2387-z
Nakano, T., and Miyazaki, Y. (2019). Blink synchronization is an indicator of interest while viewing videos. Int. J. Psychophysiol. 135, 1–11. doi: 10.1016/j.ijpsycho.2018.10.012
Nencheva, M. L., Piazza, E. A., and Lew-Williams, C. (2021). The moment-to-moment pitch dynamics of child-directed speech shape toddlers' attention and learning. Dev. Sci. 24:e12997. doi: 10.1111/desc.12997
Peng, C. K., Buldyrev, S. V., Havlin, S., Simons, M., Stanley, H. E., Goldberger, A. L., et al. (1994). Mosaic organization of DNA nucleotides. Phys. Rev. E Stat. Phys. Plasmas Fluids Relat. Interdiscip. Topics 49, 1685–1689. doi: 10.1103/PhysRevE.49.1685
Pérez, P., Madsen, J., Banellis, L., Türker, B., Raimondo, F., Perlbarg, V., et al. (2021). Conscious processing of narrative stimuli synchronizes heart rate between individuals. Cell Rep. 36:109692. doi: 10.1016/j.celrep.2021.109692
Pfeiffer, U. J., Vogeley, K., and Schilbach, L. (2013). From gaze cueing to dual eye-tracking: novel approaches to investigate the neural correlates of gaze in social interaction. Neurosci. Biobehav. Rev. 37, 2516–2528. doi: 10.1016/j.neubiorev.2013.07.017
Pinti, P., Tachtsidis, I., Hamilton, A., Hirsch, J., Aichelburg, C., Gilbert, S., et al. (2020). The present and future use of functional near-infrared spectroscopy (fNIRS) for cognitive neuroscience. Ann. N. Y. Acad. Sci. 1464, 5–29. doi: 10.1111/nyas.13948
Płużyczka, M., and Warszawski, U. (2018). The first hundred years: a history of eye tracking as a research method. Appl. Linguist. Pap. 4, 101–116. doi: 10.32612/uw.25449354.2018.4.pp.101-116
Posner, M. I., Snyder, C. R., and Davidson, B. J. (1980). Attention and the detection of signals. J. Exp. Psychol. 109, 160–174. doi: 10.1037/0096-3445.109.2.160
Prochazkova, E., Prochazkova, L., Giffin, M. R., Scholte, H. S., De Dreu, C. K. W., and Kret, M. E. (2018). Pupil mimicry promotes trust through the theory-of-mind network. Proc. Natl. Acad. Sci. USA. 115, E7265–E7274. doi: 10.1073/pnas.1803916115
Rac-Lubashevsky, R., Slagter, H. A., and Kessler, Y. (2017). Tracking real-time changes in working memory updating and gating with the event-based eye-blink rate. Sci. Rep. 7:2547. doi: 10.1038/s41598-017-02942-3
Rajkowski, J. (1993). Correlations between locus coeruleus (LC) neural activity, pupil diameter and behavior in monkey support a role of LC in attention. Soc. Neurosc. Abstract, Washington, DC. Available online at: https://ci.nii.ac.jp/naid/10021384962/ (accessed February 27, 2024).
Ravreby, I., Shilat, Y., and Yeshurun, Y. (2022). Liking as a balance between synchronization, complexity, and novelty. Nat. Sci. Rep. 12, 1–12. doi: 10.1038/s41598-022-06610-z
Razavi, M., Janfaza, V., Yamauchi, T., Leontyev, A., Longmire-Monford, S., Orr, J., et al. (2022). OpenSync: an open-source platform for synchronizing multiple measures in neuroscience experiments. J. Neurosci. Methods 369:109458. doi: 10.1016/j.jneumeth.2021.109458
Richardson, D. C., Dale, R., and Kirkham, N. Z. (2007). The Art of Conversation Is Coordination. Psychol. Sci. 18, 407–413. doi: 10.1111/j.1467-9280.2007.01914.x
Risko, E. F., Richardson, D. C., and Kingstone, A. (2016). Breaking the fourth wall of cognitive science: real-world social attention and the dual function of gaze. Curr. Dir. Psychol. Sci. 25, 70–74. doi: 10.1177/0963721415617806
Rogers, S. L., Speelman, C. P., Guidetti, O., and Longmuir, M. (2018). Using dual eye tracking to uncover personal gaze patterns during social interaction. Sci. Rep. 8:4271. doi: 10.1038/s41598-018-22726-7
Sacks, H., Schegloff, E. A., and Jefferson, G. (1978). A simplest systematics for the organization of turn taking for conversation. In the Organization of Conversational. Available oline at: https://www.sciencedirect.com/science/article/pii/B9780126235500500082 (accessed February 27, 2024).
Scaife, M., and Bruner, J. S. (1975). The capacity for joint visual attention in the infant. Nature 253, 265–266. doi: 10.1038/253265a0
Schilbach, L. (2016). Towards a second-person neuropsychiatry. Philos. Trans. R. Soc. Lond. B: Biol. Sci. 371, 20150081. doi: 10.1098/rstb.2015.0081
Schilbach, L., Timmermans, B., Reddy, V., Costall, A., Bente, G., Schlicht, T., et al. (2013). Toward a second-person neuroscience. Behav. Brain Sci. 36, 393–414. doi: 10.1017/S0140525X12000660
Schwiedrzik, C. M., and Sudmann, S. S. (2020). Pupil diameter tracks statistical structure in the environment to increase visual sensitivity. J. Neurosci. 40, 4565–4575. doi: 10.1523/JNEUROSCI.0216-20.2020
Senju, A., Vernetti, A., Kikuchi, Y., Akechi, H., Hasegawa, T., Johnson, M. H., et al. (2013). Cultural background modulates how we look at other persons' gaze. Int. J. Behav. Dev. 37, 131–136. doi: 10.1177/0165025412465360
Shockley, K., Richardson, D. C., and Dale, R. (2009). Conversation and coordinative structures. Top. Cogn. Sci. 1, 305–319. doi: 10.1111/j.1756-8765.2009.01021.x
Smith, E. R., and Mackie, D. M. (2016). Representation and incorporation of close others' responses: the RICOR model of social influence. Pers. Soc. Psychol. Rev. 20, 311–331. doi: 10.1177/1088868315598256
Stern, J. A., Walrath, L. C., and Goldstein, R. (1984). The endogenous eyeblink. Psychophysiology 21, 22–33. doi: 10.1111/j.1469-8986.1984.tb02312.x
Valtakari, N. V., Hooge, I. T. C., Viktorsson, C., Nyström, P., Falck-Ytter, T., Hessels, R. S., et al. (2021). Eye tracking in human interaction: possibilities and limitations. Behav. Res. Methods 53, 1592–1608. doi: 10.3758/s13428-020-01517-x
Wheatley, T., Kang, O., Parkinson, C., and Looser, C. E. (2012). From mind perception to mental connection: Synchrony as a mechanism for social understanding. Soc. Personal. Psychol. Compass 6, 589–606. doi: 10.1111/j.1751-9004.2012.00450.x
Wheatley, T., Thornton, M., Stolk, A., and Chang, L. J. (2023). The emerging science of interacting minds. Perspect. Psychol. Sci. 1–19. doi: 10.1177/17456916231200177
Wierda, S. M., van Rijn, H., Taatgen, N. A., and Martens, S. (2012). Pupil dilation deconvolution reveals the dynamics of attention at high temporal resolution. Proc. Natl. Acad. Sci. USA. 109, 8456–8460. doi: 10.1073/pnas.1201858109
Wohltjen, S., Toth, B., Boncz, A., and Wheatley, T. (2023). Synchrony to a beat predicts synchrony with other minds. Sci. Rep. 13:3591. doi: 10.1038/s41598-023-29776-6
Wohltjen, S., and Wheatley, T. (2021). Eye contact marks the rise and fall of shared attention in conversation. Proc. Natl. Acad. Sci. USA. 118:e2106645118. doi: 10.1073/pnas.2106645118
Zbilut, J. P., Giuliani, A., and Webber, C. L. (1998). Detecting deterministic signals in exceptionally noisy environments using cross-recurrence quantification. Phys. Lett. A 246, 122–128. doi: 10.1016/S0375-9601(98)00457-5
Keywords: eye-tracking, social attention, gaze, blinking, pupillometry, social interaction, naturalistic experimental design
Citation: Wohltjen S and Wheatley T (2024) Interpersonal eye-tracking reveals the dynamics of interacting minds. Front. Hum. Neurosci. 18:1356680. doi: 10.3389/fnhum.2024.1356680
Received: 16 December 2023; Accepted: 20 February 2024;
Published: 12 March 2024.
Edited by:
Ilanit Gordon, Bar-Ilan University, IsraelReviewed by:
Kuan-Hua Chen, University of Nebraska Medical Center, United StatesTakahiko Koike, RIKEN Center for Brain Science (CBS), Japan
Copyright © 2024 Wohltjen and Wheatley. This is an open-access article distributed under the terms of the Creative Commons Attribution License (CC BY). The use, distribution or reproduction in other forums is permitted, provided the original author(s) and the copyright owner(s) are credited and that the original publication in this journal is cited, in accordance with accepted academic practice. No use, distribution or reproduction is permitted which does not comply with these terms.
*Correspondence: Sophie Wohltjen, d29obHRqZW5Ad2lzYy5lZHU=