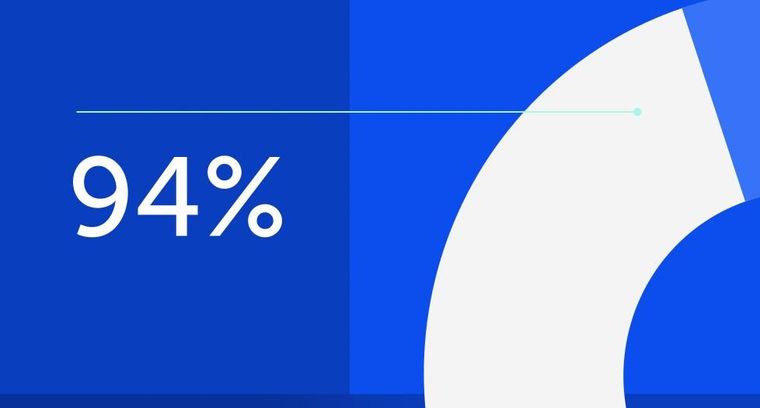
94% of researchers rate our articles as excellent or good
Learn more about the work of our research integrity team to safeguard the quality of each article we publish.
Find out more
ORIGINAL RESEARCH article
Front. Hum. Neurosci., 21 June 2024
Sec. Speech and Language
Volume 18 - 2024 | https://doi.org/10.3389/fnhum.2024.1356483
This article is part of the Research TopicNeurocomputational Models of Language ProcessingView all 8 articles
Reading is vital for acquiring knowledge and studies have demonstrated that phonology-focused interventions generally yield greater improvements than meaning-focused interventions in English among children with reading disabilities. However, the effectiveness of reading instruction can vary among individuals. Among the various factors that impact reading skills like reading exposure and oral language skills, reading instruction is critical in facilitating children’s development into skilled readers; it can significantly influence reading strategies, and contribute to individual differences in reading. To investigate this assumption, we developed a computational model of reading with an optimised MikeNet simulator. In keeping with educational practices, the model underwent training with three different instructional methods: phonology-focused training, meaning-focused training, and phonology-meaning balanced training. We used semantic reliance (SR), a measure of the relative reliance on print-to-sound and print-to-meaning mappings under the different training conditions in the model, as an indicator of individual differences in reading. The simulation results demonstrated a direct link between SR levels and the type of reading instruction. Additionally, the SR scores were able to predict model performance in reading-aloud tasks: higher SR scores were correlated with increased phonological errors and reduced phonological activation. These findings are consistent with data from both behavioral and neuroimaging studies and offer insights into the impact of instructional methods on reading behaviors, while revealing individual differences in reading and the importance of integrating OP and OS instruction approaches for beginning readers.
Reading is an acquired skill that enables people to absorb ideas through written languages. To become a proficient reader, children need to learn the mappings between orthography (O), semantics (S), and phonology (P). Past literature has shown that reading behaviors differ across readers as a function of their sensitivity to OP and OS mappings during reading (Hoffman et al., 2015; Woollams et al., 2016; Siegelman et al., 2020, 2022). Moreover, these individual differences may be critical factors that influence the effectiveness of reading instruction. For example, in designing and implementing intervention programs for children with reading disabilities (RD), Siegelman et al. (2022) demonstrated significant intervention gains through phonology-focused instruction for RD children that relied more on OP regularities than OS regularities for reading. Hence, the differences in sensitivity to OP and OS mapping in reading performance motivated the present study to examine factors influencing beginning readers’ sensitivity to different regularities.
In reading literature, several factors have been proposed to contribute to individual differences in reading, such as reading experience (Yap et al., 2012; Andrews, 2015), reading capacity (Plaut, 1997; Dilkina et al., 2008), and oral language skills (Siegelman et al., 2020; Chang, 2023). Additionally, early reading training (Taylor et al., 2017; Chang et al., 2020) could be critical concerning children’s sensitivity to OP and OS regularities. However, whether there is a direct link between them remains unclear. Consequently, in this study, we utilised a series of well-established computational models of reading trained with three different types of instructional methods including phonology-focused training, meaning-focused training, and phonology-meaning balanced training. The investigation aimed to determine the models’ sensitivity to OP and OS regularities by drawing on a measure of semantic reliance (SR hereafter) to operationalise as the utilisation of OP and OS pathways. We then compared model performance with behavioral data reported in the reading literature (Woollams et al., 2016; Siegelman et al., 2020, 2022). Here, we provided a brief overview of individual differences in reading, early reading instruction and the computational framework employed in our model development.
Studies have investigated the variability in reading behaviors among typical readers (Hoffman et al., 2015; Woollams et al., 2016; Davies et al., 2017; Siegelman et al., 2020, 2022; Chang, 2023) and dyslexic readers (Ziegler et al., 2008; Perry et al., 2019). Particularly, inter-subject variability in efficient OP and OS mapping sensitivity has been considered critical in contributing to variations in reading performance (Hoffman et al., 2015; Woollams et al., 2016; Siegelman et al., 2020, 2022). For instance, Woollams et al. (2016) examined how individual differences in the degree to which readers rely on semantic access affect reading performances in a reading-aloud task. They demonstrated that skilled readers with high SR tend to show a stronger imageability effect and produce slower responses than those with low SR particularly when reading words with inconsistent spelling-to-sound mappings (e.g., pint) in a reading-aloud task. Comparable variations in reading behaviors among individuals are also evident in research involving children (Siegelman et al., 2020, 2022). In a recent study involving a large cohort of children, it was observed that children demonstrating high sensitivity to OP regularities generally outperformed their counterparts in reading tasks, as opposed to those with lower sensitivity to OP regularities (Siegelman et al., 2020). Additionally, these children tended to show substantial gains in intervention outcomes (Siegelman et al., 2022). These findings suggest that children’s sensitivity to both OP and OS regularities may play a pivotal role in shaping their reading performance and the effectiveness of reading interventions.
As noted earlier, a number of factors may contribute to individual differences in adults and children. Critical factors, including reading experience (Yap et al., 2012; Andrews, 2015), reading capacity (Plaut, 1997; Dilkina et al., 2008), and oral language skills (Siegelman et al., 2020; Chang, 2023), have been proposed and investigated. Overall, the findings suggest that individuals with extensive reading experience, efficient processing in the reading system, and strong oral language skills typically can develop high-quality orthographic, phonological, and semantic representations, as well as efficient reading pathways (connections between these representations). Besides these factors, early reading training (Taylor et al., 2017; Chang et al., 2020) may also play a crucial role in influencing readers’ sensitivity to OP and OS regularities. However, the extent to which early reading training directly correlates with OP and OS mapping sensitivity remains uncertain.
For decades, the effectiveness of early reading instruction has been a prominent topic in English reading literature (Rayner et al., 2001; Nation, 2009; Suggate, 2016; Taylor et al., 2017; Castles et al., 2018; Torgerson et al., 2019). When it comes to learning to read in English, there are two contrasting types of instructional methods. One is phonology-focused training (e.g., Bus and van Ijzendoorn, 1999; Ehri et al., 2001), in which children are instructed to learn intensively about the relationships between print and sound. The other is meaning-focused training or a whole language approach (Levy and Lysynchuk, 1997), in which children are instructed to learn intensively about the relationships between whole words and their meanings using word cards with or without accompanying pictures.
Phonology-focused training can be traced back to the characteristics of the English writing system. There are more consistent mappings between spelling and sound compared to mappings between spelling and meaning, particularly for words comprising a single morpheme (i.e., monomorphemic words) (Plaut and Gonnerman, 2000). Additionally, monomorphemic words are frequently encountered in the early reading stages (Rastle, 2019). As a result, phonology-focused instruction can assist children in leveraging the systematic relationships between letters and sounds (e.g., -int in mint and hint), making it a more accessible learning approach. Moreover, in line with the self-teaching hypothesis (Share, 1995), the ability to map spelling to sound serves as a critical self-teaching mechanism for children. This skill aids in the subsequent acquisition of rapid and detailed orthographic representations of novel words and facilitates their recognition. Multiple lines of evidence from behavioral, neuroimaging and computational studies (Taylor et al., 2017; Chang et al., 2020) have demonstrated the effectiveness of phonology-focused training not only in word reading but also in tasks related to word comprehension. Taylor et al. (2017) conducted both behavioral and neuroimaging assessments, revealing that individuals undergoing phonology-focused training exhibited notable benefits in terms of accuracy and speed in a reading-aloud task compared to those who received meaning-focused training. Moreover, comparable performance between the two training methods was observed in a reading comprehension task. A subsequent modelling study by Chang et al. (2020) further highlighted that this transfer effect was largely dependent on oral language skills in addition to spelling-to-sound skills. Collectively, these findings align with the framework of the Simple View of Reading (SVR) (Gough and Tunmer, 1986), suggesting that successful reading comprehension necessitates a combination of phonological decoding and oral language skills.
Conversely, advocates of meaning-focused training put forth two arguments in support of their perspective (e.g., Davis, 2013). Firstly, they emphasise that the ultimate objective of reading is to establish a direct connection between the spelling of a word and its meaning, warranting a thorough examination of the efficacy of this direct mapping approach. Secondly, they point out the presence of morphological regularities in English, especially for words consisting of more than one morpheme, as exemplified by pairs like bake and baker. Research, as indicated by Nation and Cocksey (2009), suggests that children can access meaning from an orthography system without the need for phonological involvement. Consequently, proponents of meaning-focused training propose that it is beneficial for children to acquire OS mappings early on in reading (Levy and Lysynchuk, 1997; Nation, 2009; Taylor et al., 2015). However, as pointed out by Share (1995), meaning-focused training faces a noteworthy hurdle in providing a practical acquisition strategy for obtaining orthographic representations of unfamiliar words and, consequently, in navigating through an orthographic avalanche. This becomes particularly challenging considering that, on average, a fifth grader is exposed to approximately 10,000 new words in natural printed text (Nagy and Herman, 1987). It is important then that current educational practice integrates OP and OS strategies in early reading instruction and intervention.
The phonology-focused training and meaning-focused training are in accordance with neurocomputational models of dual language and reading pathways (Hickok and Poeppel, 2004, 2007; Rauschecker and Scott, 2009; Ueno et al., 2011; Bornkessel-Schlesewsky and Schlesewsky, 2013). For reading, a dorsal pathway generally consisting of the fusiform gyrus, inferior supramarginal, premotor cortex, and inferior frontal gyrus (pars opercularis and pars triangularis) underpins print-to-sound processes, while a ventral pathway generally consisting of the fusiform gyrus, the anterior parts of middle and superior temporal gyrus, the anterior temporal pole, and the inferior frontal gyrus (orbitalis) underpins print-to-meaning processes (Price, 2012; Carreiras et al., 2014, for a review; Taylor et al., 2013; Hoffman et al., 2015). Additionally, several studies have investigated neural activities of learning to read. It is found that modulation of dorsal pathway activity is evident when learning print-to-sound associations of new words while modulation of ventral pathway activity is elusive when learning whole object or word names (Mei et al., 2014; Quinn et al., 2017; Taylor et al., 2017). Particularly, a recent neuroimaging by Taylor et al. (2017) investigates the neural consequences of reading instruction (phonology-focused training versus meaning-focused training). The result demonstrated that meaning-focused training increased neural effort in dorsal pathway regions compared to phonology-focused training; however, there were no differences in ventral pathway activity following the two instructional approaches. Importantly, the fMRI results from Taylor et al. (2017) showed striking benefits of print-to-sound training. The authors suggest that early literacy education in alphabetic languages should focus on OP rather than OS strategies in order to enhance both reading aloud and reading comprehension.
While phonology-focused training and meaning-focused training represent distinct reading instructional approaches, they are frequently integrated to some extent in practical applications. Consequently, assessing their effectiveness and their influence on subsequent reading behaviors can be challenging.1 However, as evidence accumulates, a growing consensus in reading research suggests that phonology-focused training is crucial for acquiring the skill to read words with a semi-transparent relationship between print and sound in English, especially in the early stages of reading instruction (Castles et al., 2018, for a review; Rastle, 2019), compared to meaning-focused training. Nevertheless, research into the impact of these different types of reading instructional methods on OP and OS mapping sensitivity is still ongoing.
Progress in the computational modelling of reading has offered valuable mechanistic explanations for general processing principles within the human reading system. These models simulate human reading performance across diverse populations including children, adults and patients (Seidenberg and McClelland, 1989; Plaut et al., 1996; Harm and Seidenberg, 1999, 2004; Coltheart et al., 2001; Harm et al., 2003; Powell et al., 2006; Ziegler et al., 2008; Perry et al., 2019). Within the triangle modelling framework (Seidenberg and McClelland, 1989; Plaut et al., 1996; Harm and Seidenberg, 2004), the process of learning to read can be achieved through various pathways, depending on the division of labour along direct and indirect pathways for accessing phonology or semantics from orthography. This inherent property of the triangle model positions it as an ideal platform for investigating OP and OS mapping sensitivity in reading. Our previous modelling research, based on the triangle modelling framework, implemented different early reading instructional schemes used in the behavioral study by Taylor et al. (2017). The simulation results demonstrated the importance of oral language skills on the effectiveness of reading instruction (Chang et al., 2020). However, the simulation study did not examine how early reading instruction may contribute to individual differences in reading, particularly regarding the utilisation of OP and OS pathways in the model and its potential impact on later mature reading behaviors.
Therefore, building upon our prior modelling research (Chang et al., 2020), the current study aimed to address these challenges. Specifically, following the educational approaches reviewed in section 1.2, two contrasting early reading training schemes were implemented, each focusing on a different aspect of reading instructional methods: OP-focused and OS-focused. Based on previous behavioral and modelling studies (Taylor et al., 2017; Chang et al., 2020), the OP-focused training model underwent three times the training on OP mappings, while the OS-focused training model received three times the training on OS mappings. Considering that OP and OS training are also frequently integrated to some extent in practical applications, we implemented a mixture of OP and OS training models. As there is no specific guidance on the proportion of mixture, the model was trained with an equal amount of training on both OP and OS mappings, termed the OP-OS balanced model. Following Chang (2023), the utilisation of OP and OS pathways was characterised by SR in the model as an indicator of individual differences. Subsequently, we explored the associations between the SR in the model and various early training regimens. Additionally, we examined how SR interacted with other psycholinguistic reading effects during reading aloud.
Finally, while computational modelling has proven to be a valuable tool for probing the mechanisms underlying language processes, training a large-scale deep neural network model, such as the fully implemented triangle model of reading employed in this study, is often associated with high computational costs. Consequently, the time-consuming training process fundamentally limits the model’s capacity to simulate a substantial cohort of individuals. To mitigate the training burden and facilitate large-scale of computational studies, optimising and parallelising the computationally demanding algorithms is imperative by leveraging available computing hardware resources. In this study, we integrated Single Instruction Multiple Data (SIMD) and threading optimisation techniques to enhance the computational performance and efficiency. Further details about the optimisation approaches and results are reported in the Supplementary material.
Figure 1 shows the architecture of the triangle model of reading. The model was identical to the one used in previous modelling studies exploring the influence of oral language skills on variations in reading (Chang, 2023), the effectiveness of reading instruction (Chang et al., 2020), as well as investigating the impact of word sequence and language exposure on reading development (Monaghan et al., 2017; Chang and Monaghan, 2019). It had three essential processing layers including orthography (O), phonology (P), and semantics (S). There were 364 units in the orthography layer, 200 units in the phonology layer, and 2,446 units in the semantics layer. All three layers connected to each other but between every two layers was a hidden layer. The hidden layers between the phonological and semantic layers had 300 units, while those between the orthographic, phonological and semantic layers had 500 units. In phonological and semantic layers, there were attractor layers consisting of 50 units. These attractor layers helped the model develop robust and accurate phonological and semantic representations of words, wherein even partial or noisy degraded activation patterns could transition towards familiar representations (Harm and Seidenberg, 2004). Moreover, a context layer consisting of 4 units was connected to the semantic layer via a hidden layer of 10 units to handle homophones (i.e., words have the same orthographic form but multiple meanings).
Figure 1. The architecture of the triangle model of reading. Numbers in brackets indicate the number of units in that layer. S: Semantics; P: Phonology; O: Orthography. Adapted from Chang (2023).
Following previous modelling work (Chang et al., 2019; Chang and Monaghan, 2019; Chang, 2023), the model was trained with orthographic, phonological, and semantic patterns for 6,229 monosyllabic English words. This set of vocabulary encompassed most of the inflected forms found in monosyllabic English words (Harm and Seidenberg, 2004). Orthographic patterns of words were represented by 14 letter slots, each containing 26 units corresponding to the English alphabet. The alignment of words involved situating the first vowel letter on the fifth slot, and if applicable, the second vowel letter on the sixth slot. Consonant letters that preceded or followed the vowel letters were placed in slots adjacent to the two vowel letter slots. For example, yes was represented as _ _ _ y e _ s _ _ _ _ _ _ _, and great as _ _ g r e a t _ _ _ _ _ _ _.
Phonological patterns of words were represented by 8 phoneme slots, each comprising 25 units for various phonological features such as voiced, nasal, round, etc. The first three phoneme slots were designated for onset consonants, the fourth slot for the vowel, and the remaining four slots for coda consonants. For example, yes was _ _ y E s _ _ _ and great was _ g r eI t _ _ _.
Semantic representations of words were derived from Wordnet (Miller et al., 1990) and consisted of 2,446 semantic units. The representational scheme employed binary coding, where one indicated the presence of a semantic feature, and zero indicated its absence. Context representations for each meaning of the homophone family were randomly assigned by activating one of the four units at the beginning of training. None of the context units were active for words with a single meaning.
The procedure for model training was divided into two phases: the oral language training phase, which mimicked children’s learning to listen and speak, and the reading training phase, which mimicked children’s learning to read. For each training trial, the model was presented with a word randomly chosen according to its word frequency (Marcus et al., 1993).
In the oral language phase, the model was trained with four oral language tasks interleaved for two million trials. For each trial, one of the four mappings, semantics to phonology (SP), phonology to semantics (PS), phonology to phonology (PP), or semantics to semantics (SS) was selected for training. The PS and SP mappings in the model were used to simulate an oral vocabulary task and a meaning naming task in the human behavioral studies, respectively. On the other hand, the PP and SS mappings were used for the model to develop a reliable phonological attractor and a semantic attractor, respectively. The training ratio was 40% of trials for SP, 40% of trials for PS, 10% of trials for PP and 10% of trials for SS. For the SP training, the model was provided with an input to the semantic layer for eight timesteps, and the model was asked to generate the corresponding target in the phonological layer. Similarly, for the PS training, the model was provided with an input to the phonological layer for eight timesteps, and the model was asked to generate the corresponding target in the semantic layer. For the phonological attractor, the phonological representations were presented for two timesteps and the model was allowed to cycle the activation for the next six timesteps to recreate initial representations. Likewise, the semantic attractor was to map semantic to semantic representations, and the timesteps for presentation and cycling were identical to those in training the phonological attractor.
In the reading training phase, all weights between the phonological and semantic layers obtained from the oral language training phase were first loaded and frozen. The model was then trained to learn to read by learning the mappings from orthographic to phonological representations and from orthographic to semantic representations for one million trials. A word was selected and presented for twelve timesteps in each trial. Critically, three different early training schemes, OP-focused training, OS-focused training, and OP-OS balanced training were implemented by varying amounts of exposure to OP or OS mapping following previous behavioral and modelling studies (Taylor et al., 2017; Chang et al., 2020). Specifically, for OP-focused training, the probability of exposure to OP mapping was 75%, and the probability of exposure to OS mapping was 25% (i.e., OP exposure was 3x higher than OS exposure). In contrast, for OS-focused training, the probability of exposure to OP mapping was 25%, and the probability of exposure to OS mapping was 75%. For OP-OS balanced training, the probability of exposure to the OP or OS exposure was equal, both 50% (i.e., identical mapping exposure).
In both phases of model training, the same training parameters were employed. The backpropagation through time algorithm (Pearlmutter, 1989, 1995) was used to update weights by reducing the differences between target patterns and actual activations produced by the model. The learning rate was set to 0.05. To simulate variability in each reading training condition, 40 versions of the OP-focused model, the OS-focused model, and the OP-OS balanced model were trained separately with different initial weights. Thus, there were in total 120 simulations of the models.
After the end of model training, the evaluation of model performance followed the methodology established in prior simulation work (Chang and Monaghan, 2019; Chang, 2023). In assessing phonology, the error score was computed as the sum of squared error (SSE) between the model’s actual phonological pattern and its target pattern. Accuracy was determined by identifying the closest phoneme to the model’s output through Euclidean distance and verifying whether the actual and target phonemes matched across all phoneme slots. Similar approaches were used to assess semantics. The error score was calculated as the semantic SSE between the model’s actual semantic pattern and its target pattern. Accuracy, in this context, involved computing the Euclidean distance between the model’s actual semantic representation and the semantic representation of each word in the training set. The assessment then determined if the smallest distance corresponded to the target semantic representation.
A crucial aim of this study was to explore whether training focus would influence individual reading strategies in the model, in terms of the degree of utilisation of OP and OS pathways reflected by the measure of SR. As demonstrated in a recent simulation study by Chang (2023), the SR in the model could be quantified by directly measuring the use of the semantic pathway relative to the phonological pathway using a lesion technique (Welbourne et al., 2011; Chang and Lambon Ralph, 2020), or by indirectly measuring the consistency effect when reading exception words, as used in a behavioral study by Woollams et al. (2016). Critically, investigations into these two SR measures resulted in largely similar patterns regarding their predictiveness on reading performance, albeit predictability was greater for the direct approach than the indirect one.
Therefore, in the present study, the direct approach was adopted to measure SR in the model. Specifically, to quantify the use of the OS pathway in accessing semantics in the model, the OPS pathway was damaged, and semantic SSE was recorded. The reverse procedure was used to determine the use of the OPS pathway by damaging the OS pathway. The assumption is that if one pathway is damaged, and the model relies on the undamaged pathway, resulting in minimal semantic SSEs, it indicates effective functioning and high reliability of the undamaged pathway. Conversely, if reliance on the undamaged pathway leads to a substantial semantic SSE, it suggests poor functioning of the undamaged pathway. Consequently, the reciprocals of the semantic SSE obtained from the OS and OPS pathways were computed to denote the proportional contribution across the pathways. The SR was quantified by dividing the contribution of the OS pathway by the sum of the contributions from the OPS and OS pathways.
At the end of the oral language training, the model achieved 92.11% accuracy on the meaning naming task (which required SP mappings) and 91.24% on the oral vocabulary task (which required PS mappings). At the end of the reading training, the OP-focused model, the OP-OS balanced model, and the OS-focused model achieved an accuracy of 99.83, 99.96, and 99.23% in phonology, and an accuracy of 92.61, 99.43, and 98.91% in semantics, respectively.
The SR was calculated for each of the 120 models including 40 versions of the OP-focused model, 40 versions of the OP-OS balanced model, and 40 versions of the OS-focused model. Figure 2A illustrates the SR distribution, while Figure 2B presents the SR scores corresponding to each type of reading instruction. The SR ranged from 0.02 to 0.226 (M = 0.086, SD = 0.040), and the average SR was the highest for the OS-focused model (M = 0.114, SD = 0.041), followed by the OP-OS balanced model (M = 0.081, SD = 0.031), and then the OP-focused model (M = 0.062, SD = 0.028). The SR distribution demonstrated that varying training environments could lead to different degrees of SR in the model of reading.
Figure 2. (A) The distribution of the semantic reliance in the model (B) the semantic reliance generated from the models with different types of reading instructional approaches. OP: OP-focused model; OP-OS: OP-OS balanced; OS: OS-focused.
The relationship between reading instruction and SR was directly investigated using a simple regression technique with training focus, OP-focused (OP), OP-OS balanced (OP-OS), and OS-focused (OS) as the predictor and SR as the dependent variable. The regression model was significant, in which R2 value was 29.5% (Adjusted R2 = 28.3%), p < 0.001. The SR difference between the OP model and the OP-OS model was significant, β = 0.227, p = 0.013. The SR differences between the OP-OS model and the OS model, β = 0.393, p < 0.001, and between the OP model and the OS model, β = 0.62, p < 0.001, were both significant.
The regression results showed that training focus could explain a significant portion of the variance in SR, demonstrating the influence of training focus on the utilisation of OP and OS pathways in the model. To further explore the extent to which training focus might impact learning in terms of the development of precise representations in the model and its relationship with SR, we investigated the level of activations among units at the phonological layer, referred to as stress or polarity (Plaut, 1997). The concept of polarity measurement suggests that during training, units at the output layers of the model are trained to accurately represent target patterns typically consisting of binary values (i.e., 1 or 0). Consequently, higher polarity scores generated by the model for a given word indicate the development of more refined representations for that word. Following Plaut (1997), we used a formula (Equation 1) to compute the index of unit binarisation in the model, termed unit polarity:
where is the unit activation ranging from 0 to 1; is the logarithmic function with the base of 2; is the polarity measure.
We computed the average of polarities across all words in the training set at the phonological layer for each model, categorised by varying SR scores and different training focuses. The result is illustrated in Figure 3A. As can be seen, both polarity scores generated by the OP-focused models and OP-OS balanced models are higher than those generated by the OS-focused models. Critically, these scores are further modulated by SR, with an increase in SR resulting in a decrease in polarity scores especially for the OS-focused models. In Figure 3B, we also showed the corresponding phonological SSE for each model, confirming that the models that produced higher polarity scores tended to have smaller phonological SSEs, reflecting more refined representations.
Figure 3. (A) The phonological polarity (B) the phonological SSE was generated by each of 120 individual models, categorised by varying SR scores and different training focuses.
The following critical examination in this study was to explore whether the SR influenced by varying training focuses could impact reading behaviors in the model. Past behavioral investigations have shown that SR affects RTs in a reading-aloud task for both adults (Woollams et al., 2016) and children (Siegelman et al., 2022). Particularly, skilled readers with high SR tend to produce slower responses than readers with low SR; and their RTs are moderated by imageability and consistency. Therefore, to investigate whether the present model could produce similar patterns as observed in behavioral studies, we conducted a series of linear mixed-effect model (LMM) analyses on the model’s reading-aloud performance. Phonological SSE in the model was used as a proxy for human RTs in a reading-aloud task (Monaghan et al., 2017; Chang et al., 2020). LMM analyses were based on “lme4”, a package in R (version 4.1.3, 2022). For the LMM analyses, an effect was considered significant at the p < 0.05 level if its t-value was greater than 1.96 (Baayen, 2008). To examine the SR effect and its relationship with other psycholinguistic variables, we conducted two evaluation approaches, one was to replicate the findings reported in a factorial experiment reported by Woollams et al. (2016), and the other one was to examine the effect using a regression-based approach.
For the factorial test, we investigated whether the model could replicate the key behavioral patterns of the reading-aloud task observed in Woollams et al. (2016), wherein the SR effect is modulated by imageability and consistency. Four sets of stimuli, including high-imageability consistent words, low-imageability consistent words, high-imageability inconsistent words, and low-imageability inconsistent words were taken from Woollams et al. (2016), comprising 40 words for each condition. There was a total of 19,200 trials (i.e., 4*40*120). Prior to the analysis, 6.7% of the trials including words misread by the model and phonological SSEs greater than three standard deviations from the mean, were discarded as outliers. This resulted in 17,912 data points for further analysis. An LMM model was conducted with item and model version as random factors, with imageability by consistency by SR as fixed factors, and with phonological SSE as the dependent variable. All variables were scaled using z-score normalisation for evaluating interactions. The results showed that SR significantly predicted phonological SSE, β = 0.005, t = 3.72, as well as consistency, β = −0.004, t = −2.51. Imageability was not a significant predictor. The interaction between SR and consistency, β = −0.002, t = −4.13, was significant. The interaction between SR and imageability, β = −0.002, t = −2.02 was also significant. The interaction between consistency and imageability was not significant. Critically, the three-way interaction between SR, imageability, and consistency was significant, β = −0.001, t = −2.41.
For the test using the regression-based approach, in addition to SR, we included a range of psycholinguistic variables previously shown to be important for the reading-aloud task in behavioral studies: word frequency (WF), orthographic neighbourhood size (ONS) (Coltheart et al., 1977), rime consistency (RC) (Glushko, 1979), and imageability (IMG) (Cortese and Fugett, 2004). The descriptive statistics of the psycholinguistic variables and the correlations between them are reported in Table 1.
Table 1. The descriptive statistics of the psycholinguistic variables and the correlations between them.
Data cleaning procedures were similar to those in the previous section. Outliers included words that the model misread, had missing psycholinguistic measures, and phonological SSE greater than three standard deviations from the means. This removal resulted in 590,653 points. To explore whether the SR effect was moderated by imageability and consistency, an LMM analysis was conducted with all the psycholinguistic variables and the interaction between SR, imageability and consistency as independent variables, and with phonological SSE as a dependent variable. As shown in Table 2, WF, ONS, RC, and IMG all had significant effects on the model’s reading-aloud performance. Specifically, the model produced low phonological SSEs for words with higher values of WF, ONS, RC, and IMG, congruent with previous findings in behavioral studies (Balota et al., 2004; Cortese and Khanna, 2008). Additionally, SR was also a significant predictor, β = 0.006, t = 6.33, indicating the model with higher SR tends to produce more phonological SSEs.
Regarding the interaction results, there were significant two-way interactions between SR and RC, β = −0.002, t = −13.08, and between SR and IMG, β = −0.002, t = −8.44, as shown in Figure 4. The model with a larger SR generated stronger consistency and imageability effects than that with a smaller SR. On the other hand, the interaction between RC and IMG was not significant. Critically, the three-way interaction between SR, RC and IMG also reached significance, β = −0.001, t = −4.15. Overall, these results are consistent with the findings of the factorial test of the SR effect reported in the previous section and the behavioral data (Woollams et al., 2016).
Figure 4. The interaction between (A) semantic reliance (SR) and rime consistency (RC) and (B) semantic reliance (SR) and imageability (IMG).
The issue of effective early reading instruction has been a widely discussed topic in reading research (Rayner et al., 2001; Nation, 2009; Suggate, 2016; Taylor et al., 2017; Castles et al., 2018; Torgerson et al., 2019). Most studies have focused on investigating the benefits of using phonics-style or whole-word style when teaching children to read (e.g., Rayner et al., 2001; Nation, 2009; Davis, 2013; Taylor et al., 2017). However, little is known about the influence of early reading instructional methods on subsequent individual reading behaviors. Therefore, using a computational approach, we investigated whether early reading instruction could lead to variations among individuals’ sensitivity to OP and OS regularities, which in turn has an impact on reading behaviors. The simulation results demonstrated that the models receiving different training methods showed varying SR scores, displaying a spectrum of variations within each training condition (see Figure 2). On average, a model focused on print-to-meaning (the OS-focused training model) showed stronger SR for reading aloud than a model trained with a combination of OP and OS mappings (the OP-OS balanced training model), followed by a model focused on print-to-sound (the OP-focused training model).
Furthermore, by using both factorial and regression-based approaches, the LMM results indicated that the resulting SR scores were able to predict model performance on reading aloud. The models with higher SR scores tended to produce more phonological SSEs, compared to those with lower SR scores. The result was consistent with the findings of slower reading-aloud responses observed in behavioral studies (Siegelman et al., 2020, 2022) and reduced phonological activations observed in neuroimaging studies (Hoffman et al., 2015). Additionally, we also observed that the effect of SR interacted significantly with imageability and consistency as was reported in Woollams et al. (2016). Additionally, the LMM analyses revealed that several reading effects identified in behavioral studies (Balota et al., 2004; Cortese and Khanna, 2008), such as frequency, consistency, orthographic neighbourhood size, and imageability, could be accounted for by the model.
The simulation results, revealing diverse SR scores due to different reading instructional methods, align with our earlier modelling work (Chang et al., 2020), demonstrating the differential patterns of division of labour between OP and OS pathways as results of the models receiving different reading instructional methods. Our present study extends the finding by illustrating that the utilisation of the OP and OS pathways can be reframed as a measure of SR, thereby accounting for individual differences in reading.
The finding of varying degrees of utilisation of the OP and OS pathways relating to early reading instruction is potentially intriguing, particularly when considering the characteristics of English words. In early English word reading, approximately 80% of words are monomorphemic, based on token frequency (Rastle, 2019), and these words typically exhibit more systematic OP mappings compared to OS mappings (Plaut and Gonnerman, 2000). Thus, when the model learns the regularities of OP mappings of these words, the use of the direct OP pathway for a reading-aloud task should be mostly straightforward. Nevertheless, through intensive OS mapping training, there exists the potential for increased reliance on the OS pathway in the model. However, this enhancement may not be beneficial because the model may not be able to effectively exploit the systematic OP mappings, reflecting inferior performance in reading aloud for the OS-focused model compared to the OP-focused model. Considering rich evidence from behavioral and neuroimaging studies (Liberman et al., 1989; Simos et al., 2002, 2007; Castles et al., 2018; Rastle, 2019), a better practice may be to teach children OP mappings for developing phonological awareness in the early stages of reading instruction, followed by teaching OS mappings for integrating orthographic and morphological awareness.
Additionally, an important and relevant question is why the readers with high SR scores are slower in the reading-aloud task. Siegelman et al. (2022) suggest that an excessive reliance on the OS pathway might indicate an inadequate integrity of the phonological system, resulting in a suboptimal organisation of the reading system. According to contemporary neurocomputational models of language and reading processing (Hickok and Poeppel, 2004, 2007; Rauschecker and Scott, 2009; Ueno et al., 2011; Bornkessel-Schlesewsky and Schlesewsky, 2013), the dorsal and ventral pathways are involved with print-to-sound and print-to-meaning mappings, respectively. Thus, in the present context, one would anticipate observing distinctive modulations of neural activities in the dual pathways linked to the level of SR because of reading instruction. Indeed, neural activities in both pathways have been found to correlate with SR levels (Hoffman et al., 2015). Readers with higher SR scores display heightened activations in the left anterior temporal pole associated with semantic processing, and critically simultaneously exhibit reduced activations in the left precentral gyrus associated with phonological processing compared to those with lower SR scores. This neural evidence provides support for a potential explanation that high SR readers are slower in the phonologically-demanding task (Hoffman et al., 2015). This explanation aligns with Siegelman et al. (2022) account of a potential phonological deficit in the OP pathway in reading impaired children, reflected in increased SR. Our further investigations into the impact of reading instruction on learning in the model also provided computational evidence to support these accounts, demonstrating that the models with high SR scores produced lower phonological polarity scores and more phonological SSEs, especially for the OS-focused models.
Considering the broader implications for education, our simulation results suggest that some children entering intervention programmes already exhibit differences in their sensitivity to OP vs. OS regularities. The sensitivity differences contribute to divergent intervention outcomes, as demonstrated by Siegelman et al. (2022), wherein children relying more on OP regularities tend to show greater improvement in phonologically-weighted intervention programmes compared to those relying more on OS regularities. Interestingly, they also revealed that post-intervention, reading-impaired children who increased their reliance on OP regularities or decreased their reliance on OS regularities had better intervention gains. Their results highlight the importance of appropriate interventions to optimally shape OP and OS pathways in paving the way for reading success. Collectively, the results from computational modelling and behavioral investigations indicate that the type of training approaches used in early reading instruction and interventions can influence individuals’ reliance on the OP and OS pathways, contributing to individual differences in reading acquisition.
In this study, we have demonstrated a direct link between reading instruction and individual differences in reading by using a series of widely acknowledged triangle models of reading. Despite conducting a substantial number of simulations (i.e., 120 simulations) to capture variations in SR through an accelerated training process, this study could be enhanced by training additional simulation samples to achieve a scale similar to that of comprehensive behavioral studies (e.g., Siegelman et al., 2020, 2022) or to simulate dyslexic readers’ reading profiles (e.g., Perry et al., 2019).
Another notable aspect concerns the impact of reading instruction on language systems characterised by different forms of systematicity. The present study has focused on early English word reading, in which words generally exhibit more systematic OP mappings compared to OS mappings. Future investigations could explore language systems with a more balanced systematicity in the mappings between orthography to phonology and orthography to semantics, such as Chinese.
Lastly, the present study has focused on investigating the unique impact of early reading instruction on individual differences in reading, particularly regarding the utilisation of OP and OS pathways. However, literacy development is complex; various elements such as oral language knowledge, computational compacity, and reading experience may collectively contribute to different aspects of individual differences in reading (Plaut, 1997; Dilkina et al., 2008; Yap et al., 2012; Andrews, 2015; Siegelman et al., 2020; Chang, 2023). Therefore, in connecting to real-world applications, it is possible to extend the present simulation framework to incorporate and examine various potential factors comprehensively in future research. That could enable a better understanding of how to best tailor individual interventions and pave the way for the development of an intervention program suited to the diverse needs of beginning readers.
To conclude, this study utilised a large number of computational models of reading, developed using the optimised MikeNet simulator (see Supplementary material), to investigate the potential sources of individual differences in reading. The simulation results demonstrated a direct link between reading instruction and the variations in reliance on the OP and OS pathways within the model. This differential reliance significantly moderated model performance during reading.
The datasets presented in this study can be found in online repositories. The names of the repository/repositories and accession number(s) can be found below: https://github.com/yaningchang/Chang_et_al_RI_ID_Paper_for_FHN.git.
Y-NC: Conceptualization, Data curation, Formal analysis, Funding acquisition, Investigation, Methodology, Project administration, Resources, Software, Supervision, Validation, Visualization, Writing – original draft, Writing – review & editing. T-JC: Methodology, Software, Supervision, Writing – original draft, Writing – review & editing. W-FL: Methodology, Software, Supervision, Writing – original draft. C-EK: Methodology, Software, Writing – original draft. Y-TS: Methodology, Software, Writing – review & editing. H-WL: Methodology, Software, Writing – review & editing.
The author(s) declare financial support was received for the research, authorship, and/or publication of this article. This research was supported the National Science and Technology Council, Taiwan (MOST110-2423-H-006-001-MY3 to Y-NC) and Google Research Grant to Y-NC.
Preliminary data were presented at the 44th annual conferences of the Cognitive Science Society. The authors are very grateful to thank Chia-Fang Cheng for her comments on earlier drafts. The author also thanks the editor and reviewers for their helpful and constructive comments.
The authors declare that the research was conducted in the absence of any commercial or financial relationships that could be construed as a potential conflict of interest.
All claims expressed in this article are solely those of the authors and do not necessarily represent those of their affiliated organizations, or those of the publisher, the editors and the reviewers. Any product that may be evaluated in this article, or claim that may be made by its manufacturer, is not guaranteed or endorsed by the publisher.
The Supplementary material for this article can be found online at: https://www.frontiersin.org/articles/10.3389/fnhum.2024.1356483/full#supplementary-material
1. ^We are grateful to reviewer 3 for expanding on this perspective.
Andrews, S. (2015). “Individual differences among skilled readers: the role of lexical quality” in The Oxford handbook of reading. eds. A. Pollatsek and R. Treiman (Oxford University Press), 129–148.
Baayen, R. H. (2008). Analyzing linguistic data: A practical introduction to statistics using R. Cambridge, UK: Cambridge University Press.
Balota, D. A., Cortese, M. J., Sergent-Marshall, S. D., Spieler, D. H., and Yap, M. (2004). Visual word recognition of single-syllable words. J. Exp. Psychol: General, 133, 283–316. doi: 10.1037/0096-3445.133.2.283
Bornkessel-Schlesewsky, I., and Schlesewsky, M. (2013). Reconciling time, space and function: a new dorsal–ventral stream model of sentence comprehension. Brain Lang. 125, 60–76. doi: 10.1016/j.bandl.2013.01.010
Bus, A. G., and van Ijzendoorn, M. H. (1999). Phonological awareness and early reading: a meta-analysis of experimental training studies. J. Educ. Psychol. 91, 403–414. doi: 10.1037/0022-0663.91.3.403
Carreiras, M., Armstrong, B. C., Perea, M., and Frost, R. (2014). The what, when, where, and how of visual word recognition. Trends Cogn. Sci. 18, 90–98. doi: 10.1016/j.tics.2013.11.005
Castles, A., Rastle, K., and Nation, K. (2018). Ending the Reading wars: reading acquisition from novice to expert. Psychol. Sci. Public Interest 19, 5–51. doi: 10.1177/1529100618772271
Chang, Y.-N. (2023). The influence of oral vocabulary knowledge on individual differences in a computational model of reading. Sci. Rep. 13:1680. doi: 10.1038/s41598-023-28559-3
Chang, Y.-N., and Lambon Ralph, M. A. (2020). A unified neurocomputational bilateral model of spoken language production in healthy participants and recovery in poststroke aphasia. Proceedings of the National Academy of Sciences, 117, 32779–32790. doi: 10.1073/pnas.2010193117
Chang, Y.-N., and Monaghan, P. (2019). Quantity and diversity of Preliteracy language exposure both affect literacy development: evidence from a computational model of Reading. Sci. Stud. Read. 23, 235–253. doi: 10.1080/10888438.2018.1529177
Chang, Y.-N., Monaghan, P., and Welbourne, S. (2019). A computational model of reading across development: effects of literacy onset on language processing. J. Mem. Lang. 108:104025. doi: 10.1016/j.jml.2019.05.003
Chang, Y.-N., Taylor, J. S. H., Rastle, K., and Monaghan, P. (2020). The relationships between oral language and reading instruction: evidence from a computational model of reading. Cogn. Psychol. 123:101336. doi: 10.1016/j.cogpsych.2020.101336
Coltheart, M., Davelaar, E., Jonasson, J. T., and Besner, D. (1977). “Access to the internal lexicon” in Attention and performance VI. ed. S. Dornic (Mahwah, New Jersey, USA: Lawrence Erlbaum Associates), 535–555.
Coltheart, M., Rastle, K., Perry, C., Langdon, R., and Ziegler, J. (2001). DRC: a dual route cascaded model of visual word recognition and reading aloud. Psychol. Rev. 108, 204–256. doi: 10.1037/0033-295X.108.1.204
Cortese, M. J., and Fugett, A. (2004). Imageability ratings for 3,000 monosyllabic words. Behav. Res. Methods Instrum. Comput. 36, 384–387. doi: 10.3758/BF03195585
Cortese, M. J., and Khanna, M. M. (2008). Age of acquisition ratings for 3,000 monosyllabic words. Behav. Res. Methods 40, 791–794. doi: 10.3758/BRM.40.3.791
Davies, R. A. I., Arnell, R., Birchenough, J. M. H., Grimmond, D., and Houlson, S. (2017). Reading through the life span: individual differences in psycholinguistic effects. J. Exp. Psychol. Learn. Mem. Cogn. 43, 1298–1338. doi: 10.1037/xlm0000366
Davis, A. (2013). To read or not to read: decoding synthetic phonics. Impact 2013, 1–38. doi: 10.1111/2048-416X.2013.12000.x
Dilkina, K., McClelland, J. L., and Plaut, D. C. (2008). A single-system account of semantic and lexical deficits in five semantic dementia patients. Cogn. Neuropsychol. 25, 136–164. doi: 10.1080/02643290701723948
Ehri, L. C., Nunes, S. R., Stahl, S. A., and Willows, D. M. (2001). Systematic phonics instruction helps students learn to read: evidence from the National Reading Panel’s Meta-analysis. Rev. Educ. Res. 71, 393–447. doi: 10.3102/00346543071003393
Glushko, R. J. (1979). Organization and activation of orthographic knowledge in reading aloud [article]. J. Exp. Psychol. Hum. Percept. Perform. 5, 674–691. doi: 10.1037/0096-1523.5.4.674
Gough, P. B., and Tunmer, W. E. (1986). Decoding, Reading, and Reading disability. Remedial Spec. Educ. 7, 6–10. doi: 10.1177/074193258600700104
Harm, M. W., McCandliss, B. D., and Seidenberg, M. S. (2003). Modeling the successes and failures of interventions for disabled readers. Sci. Stud. Read. 7, 155–182. doi: 10.1207/S1532799XSSR0702_3
Harm, M. W., and Seidenberg, M. S. (1999). Phonology, reading acquisition, and dyslexia: insights from connectionist models. Psychol. Rev. 106, 491–528. doi: 10.1037/0033-295X.106.3.491
Harm, M. W., and Seidenberg, M. S. (2004). Computing the meanings of words in reading: cooperative division of labor between visual and phonological processes [review]. Psychol. Rev. 111, 662–720. doi: 10.1037/0033-295x.111.3.662
Hickok, G., and Poeppel, D. (2004). Dorsal and ventral streams: a framework for understanding aspects of the functional anatomy of language. Cognition 92, 67–99. doi: 10.1016/j.cognition.2003.10.011
Hickok, G., and Poeppel, D. (2007). The cortical organization of speech processing. Nat. Rev. Neurosci. 8, 393–402. doi: 10.1038/nrn2113
Hoffman, P., Lambon Ralph, M. A., and Woollams, A. M. (2015). Triangulation of the neurocomputational architecture underpinning reading aloud. Proc. Natl. Acad. Sci. USA 112, E3719–E3728. doi: 10.1073/pnas.1502032112
Levy, B. A., and Lysynchuk, L. (1997). Beginning word recognition: benefits of training by segmentation and whole word methods. Sci. Stud. Read. 1, 359–387. doi: 10.1207/s1532799xssr0104_4
Liberman, I. Y., Shankweiler, D., and Liberman, A. M. (1989). “The alphabetic principle and learning to read” in Phonology and reading disability: Solving the reading puzzle (Michigan, USA: The University of Michigan Press), 1–33.
Marcus, M., Santorini, B., and Marcinkiewicz, M. A. (1993). Building a large annotated Corpus of English: the Penn treebank. Comput. Linguist. 19, 313–330.
Mei, L., Xue, G., Lu, Z. L., He, Q., Zhang, M., Wei, M., et al. (2014). Artificial language training reveals the neural substrates underlying addressed and assembled phonologies. PLoS One 9:e93548. doi: 10.1371/journal.pone.0093548
Miller, G. A., Beckwith, R., Fellbaum, C., Gross, D., and Miller, K. J. (1990). Introduction to WordNet: an on-line lexical database*. Int. J. Lexicogr. 3, 235–244. doi: 10.1093/ijl/3.4.235
Monaghan, P., Chang, Y.-N., Welbourne, S., and Brysbaert, M. (2017). Exploring the relations between word frequency, language exposure, and bilingualism in a computational model of reading. J. Mem. Lang. 93, 1–21. doi: 10.1016/j.jml.2016.08.003
Nagy, W. E., and Herman, P. A. (1987). “Breadth and depth of vocabulary knowledge: implications for acquisition and instruction” in The nature of vocabulary acquisition (Mahwah, New Jersey, USA: Lawrence Erlbaum Associates, Inc), 19–35.
Nation, K. (2009). Form-meaning links in the development of visual word recognition. Philos. Trans. R. Soc. Lond. Ser. B Biol. Sci. 364, 3665–3674. doi: 10.1098/rstb.2009.0119
Nation, K., and Cocksey, J. (2009). Beginning readers activate semantics from sub-word orthography. Cognition 110, 273–278. doi: 10.1016/j.cognition.2008.11.004
Pearlmutter, B. (1989). Learning state space trajectories in recurrent neural networks. Neural Comput. 1, 263–269. doi: 10.1162/neco.1989.1.2.263
Pearlmutter, B. (1995). Gradient calculations for dynamic recurrent neural networks: a survey. IEEE Trans. Neural Netw. 6, 1212–1228. doi: 10.1109/72.410363
Perry, C., Zorzi, M., and Ziegler, J. C. (2019). Understanding dyslexia through personalized large-scale computational models. Psychol. Sci. 30, 386–395. doi: 10.1177/0956797618823540
Plaut, D. C. (1997). Structure and function in the lexical system: insights from distributed models of word Reading and lexical decision. Lang. Cognit. Proc. 12, 765–806. doi: 10.1080/016909697386682
Plaut, D. C., and Gonnerman, L. M. (2000). Are non-semantic morphological effects incompatible with a distributed connectionist approach to lexical processing? Lang. Cognit. Proc. 15, 445–485. doi: 10.1080/01690960050119661
Plaut, D. C., McClelland, J. L., Seidenberg, M. S., and Patterson, K. (1996). Understanding normal and impaired word reading: computational principles in quasi-regular domains. Psychol. Rev. 103, 56–115. doi: 10.1037/0033-295X.103.1.56
Powell, D., Plaut, D., and Funnell, E. (2006). Does the PMSP connectionist model of single word reading learn to read in the same way as a child? J. Res. Read. 29, 229–250. doi: 10.1111/j.1467-9817.2006.00300.x
Price, C. J. (2012). A review and synthesis of the first 20 years of PET and fMRI studies of heard speech, spoken language and reading. NeuroImage 62, 816–847. doi: 10.1016/j.neuroimage.2012.04.062
Quinn, C., Taylor, J. S. H., and Davis, M. H. (2017). Learning and retrieving holistic and componential visual-verbal associations in reading and object naming. Neuropsychologia 98, 68–84. doi: 10.1016/j.neuropsychologia.2016.09.025
Rastle, K. (2019). The place of morphology in learning to read in English. Cortex 116, 45–54. doi: 10.1016/j.cortex.2018.02.008
Rauschecker, J. P., and Scott, S. K. (2009). Maps and streams in the auditory cortex: nonhuman primates illuminate human speech processing. Nat. Neurosci. 12, 718–724. doi: 10.1038/nn.2331
Rayner, K., Foorman, B. R., Perfetti, C. A., Pesetsky, D., and Seidenberg, M. S. (2001). How psychological science informs the teaching of Reading. Psychol. Sci. Public Interest 2, 31–74. doi: 10.1111/1529-1006.00004
Seidenberg, M. S., and McClelland, J. L. (1989). A distributed, developmental model of word recognition and naming [review]. Psychol. Rev. 96, 523–568. doi: 10.1037/0033-295X.96.4.523
Share, D. L. (1995). Phonological recoding and self-teaching: sine qua non of reading acquisition. Cognition 55, 151–218. doi: 10.1016/0010-0277(94)00645-2
Siegelman, N., Rueckl, J. G., Steacy, L. M., Frost, S. J., van den Bunt, M., Zevin, J. D., et al. (2020). Individual differences in learning the regularities between orthography, phonology and semantics predict early reading skills. J. Mem. Lang. 114:104145. doi: 10.1016/j.jml.2020.104145
Siegelman, N., Rueckl, J. G., van den Bunt, M., Frijters, J. C., Zevin, J. D., Lovett, M. W., et al. (2022). How you read affects what you gain: individual differences in the functional organization of the reading system predict intervention gains in children with reading disabilities. J. Educ. Psychol. 114, 855–869. doi: 10.1037/edu0000672
Simos, P. G., Fletcher, J. M., Bergman, E., Breier, J. I., Foorman, B. R., Castillo, E. M., et al. (2002). Dyslexia-specific brain activation profile becomes normal following successful remedial training. Neurology 58, 1203–1213. doi: 10.1212/WNL.58.8.1203
Simos, P. G., Fletcher, J. M., Sarkari, S., Billingsley-Marshall, R., Denton, C. A., and Papanicolaou, A. C. (2007). Intensive instruction affects brain magnetic activity associated with oral word reading in children with persistent reading disabilities. J. Learn. Disabil. 40, 37–48. doi: 10.1177/00222194070400010301
Suggate, S. P. (2016). A meta-analysis of the long-term effects of phonemic awareness, phonics, fluency, and reading comprehension interventions. J. Learn. Disabil. 49, 77–96. doi: 10.1177/0022219414528540
Taylor, J. S. H., Davis, M. H., and Rastle, K. (2017). Comparing and validating methods of reading instruction using behavioural and neural findings in an artificial orthography. J. Exp. Psychol. Gen. 146, 826–858. doi: 10.1037/xge0000301
Taylor, J. S. H., Duff, F. J., Woollams, A. M., Monaghan, P., and Ricketts, J. (2015). How word meaning influences word Reading. Curr. Dir. Psychol. Sci. 24, 322–328. doi: 10.1177/0963721415574980
Taylor, J. S. H., Rastle, K., and Davis, M. H. (2013). Can cognitive models explain brain activation during word and pseudoword reading? A meta-analysis of 36 neuroimaging studies. Psychol. Bull. 139, 766–791. doi: 10.1037/a0030266
Torgerson, C., Brooks, G., Gascoine, L., and Higgins, S. (2019). Phonics: reading policy and the evidence of effectiveness from a systematic ‘tertiary’review. Res. Pap. Educ. 34, 208–238. doi: 10.1080/02671522.2017.1420816
Ueno, T., Saito, S., Rogers, T. T., Ralph, L., and Matthew, A. (2011). Lichtheim 2: synthesizing aphasia and the neural basis of language in a Neurocomputational model of the dual dorsal-ventral language pathways. Neuron 72, 385–396. doi: 10.1016/j.neuron.2011.09.013
Welbourne, S. R., Woollams, A. M., Crisp, J., and Ralph, M. A. L. (2011). The role of plasticity-related functional reorganization in the explanation of central dyslexias. Cogn. Neuropsychol. 28, 65–108. doi: 10.1080/02643294.2011.621937
Woollams, A. M., Lambon Ralph, M. A., Madrid, G., and Patterson, K. E. (2016). Do you read how I read? Systematic individual differences in semantic reliance amongst Normal readers [original research]. Front. Psychol. 7:1757. doi: 10.3389/fpsyg.2016.01757
Yap, M. J., Balota, D. A., Sibley, D. E., and Ratcliff, R. (2012). Individual differences in visual word recognition: insights from the English lexicon project. J. Exp. Psychol. Hum. Percept. Perform. 38, 53–79. doi: 10.1037/a0024177
Keywords: individual differences, word reading, computational modelling, reading instruction, semantic reliance
Citation: Chang Y-N, Chang T-J, Lin W-F, Kuo C-E, Shi Y-T and Lee H-W (2024) Modelling individual differences in reading using an optimised MikeNet simulator: the impact of reading instruction. Front. Hum. Neurosci. 18:1356483. doi: 10.3389/fnhum.2024.1356483
Received: 15 December 2023; Accepted: 03 June 2024;
Published: 21 June 2024.
Edited by:
Maria Mody, Massachusetts General Hospital and Harvard Medical School, United StatesReviewed by:
Paz Suárez-Coalla, University of Oviedo, SpainCopyright © 2024 Chang, Chang, Lin, Kuo, Shi and Lee. This is an open-access article distributed under the terms of the Creative Commons Attribution License (CC BY). The use, distribution or reproduction in other forums is permitted, provided the original author(s) and the copyright owner(s) are credited and that the original publication in this journal is cited, in accordance with accepted academic practice. No use, distribution or reproduction is permitted which does not comply with these terms.
*Correspondence: Ya-Ning Chang, eWFuaW5nY2hhbmdAZ21haWwuY29t
Disclaimer: All claims expressed in this article are solely those of the authors and do not necessarily represent those of their affiliated organizations, or those of the publisher, the editors and the reviewers. Any product that may be evaluated in this article or claim that may be made by its manufacturer is not guaranteed or endorsed by the publisher.
Research integrity at Frontiers
Learn more about the work of our research integrity team to safeguard the quality of each article we publish.