- 1Institute for Collaborative Biotechnologies, University of California, Santa Barbara, Santa Barbara, CA, United States
- 2Department of Psychological and Brain Sciences, University of California, Santa Barbara, Santa Barbara, CA, United States
- 3U. S. Army DEVCOM Soldier Center, Natick, MA, United States
- 4Center for Applied Brain and Cognitive Sciences, Tufts University, Medford, MA, United States
Introduction: Transcranial direct current stimulation (tDCS) administers low-intensity direct current electrical stimulation to brain regions via electrodes arranged on the surface of the scalp. The core promise of tDCS is its ability to modulate brain activity and affect performance on diverse cognitive functions (affording causal inferences regarding regional brain activity and behavior), but the optimal methodological parameters for maximizing behavioral effects remain to be elucidated. Here we sought to examine the effects of 10 stimulation and experimental design factors across a series of five cognitive domains: motor performance, visual search, working memory, vigilance, and response inhibition. The objective was to identify a set of optimal parameter settings that consistently and reliably maximized the behavioral effects of tDCS within each cognitive domain.
Methods: We surveyed tDCS effects on these various cognitive functions in healthy young adults, ultimately resulting in 721 effects across 106 published reports. Hierarchical Bayesian meta-regression models were fit to characterize how (and to what extent) these design parameters differentially predict the likelihood of positive/negative behavioral outcomes.
Results: Consistent with many previous meta-analyses of tDCS effects, extensive variability was observed across tasks and measured outcomes. Consequently, most design parameters did not confer consistent advantages or disadvantages to behavioral effects—a domain-general model suggested an advantage to using within-subjects designs (versus between-subjects) and the tendency for cathodal stimulation (relative to anodal stimulation) to produce reduced behavioral effects, but these associations were scarcely-evident in domain-specific models.
Discussion: These findings highlight the urgent need for tDCS studies to more systematically probe the effects of these parameters on behavior to fulfill the promise of identifying causal links between brain function and cognition.
1 Introduction
Non-invasive brain stimulation (NIBS) involves the introduction of exogenous energy (including magnetic, electrical, or ultrasonic) to cortical and subcortical brain regions to modify neuronal activity and draw causal links between regional brain function, cognition, emotion, and behavior (Dayan et al., 2013; Bestmann et al., 2015; Polania et al., 2018). Transcranial direct current stimulation (tDCS) is one of the most popular and widely-used NIBS methods, offering the ability to induce transient and subthreshold modulation of neuronal membrane potentials, with downstream consequences for the activity of neuronal populations and neural networks, neuroplasticity, and/or behavior (de Berker et al., 2013; Bestmann et al., 2015). While tDCS is a promising neuromodulatory tool, the precise mechanisms by which it exerts effects on physiology and behavior are still being discovered (Bikson et al., 2013; Dayan et al., 2013; Bestmann et al., 2015; Bikson et al., 2019). Emerging mechanistic models suggest that tDCS induces shifts of neuronal membrane potentials; that the nature of this shift (i.e., hyperpolarizing, depolarizing) is related to the orientation of neurons relative to the induced electrical field; that tDCS can induce large-scale oscillatory changes of brain activity; and that lasting after-effects of tDCS are related to changes in synaptic plasticity (Lafon et al., 2017; Bikson et al., 2019; Nitsche et al., 2019). However, much uncertainty still exists about how electrical current propagates through human tissue, which structures are effectively modulated by various electrode montages, how to effectively individualize stimulation protocols, and how various study design features might influence tDCS effects on observed outcomes (Datta et al., 2012; de Berker et al., 2013; Bestmann et al., 2015).
Ambiguity regarding tDCS mechanisms of action can make it difficult for researchers to select from myriad methodological parameters when designing experiments. An a priori review of related literature can complicate the issue due to heterogeneity of stimulation procedures and observed outcomes across studies, laboratories, and cognitive domains (Datta et al., 2012; Horvath et al., 2014; Bestmann et al., 2015). Indeed, published tDCS research varies widely in stimulation methods, study designs, and statistical methods employed; variation along each of these dimensions is likely to shape the effects of tDCS on brain physiology and behavior, and they likely interact in yet unknown ways. Herein we describe a meta-analytic, meta-regression modeling approach intended to provide an initial breadth-first understanding of relationships between methodological parameters employed in tDCS studies and behavioral outcomes across five cognitive domains, focusing only on studies examining healthy, neurotypical participants. We focused our analysis on 10 parameters of interest, including 7 stimulation-specific parameters and 3 experimental parameters (detailed in Table 1). These parameters were selected because they were relatively well represented in the literature across our outcome domains.
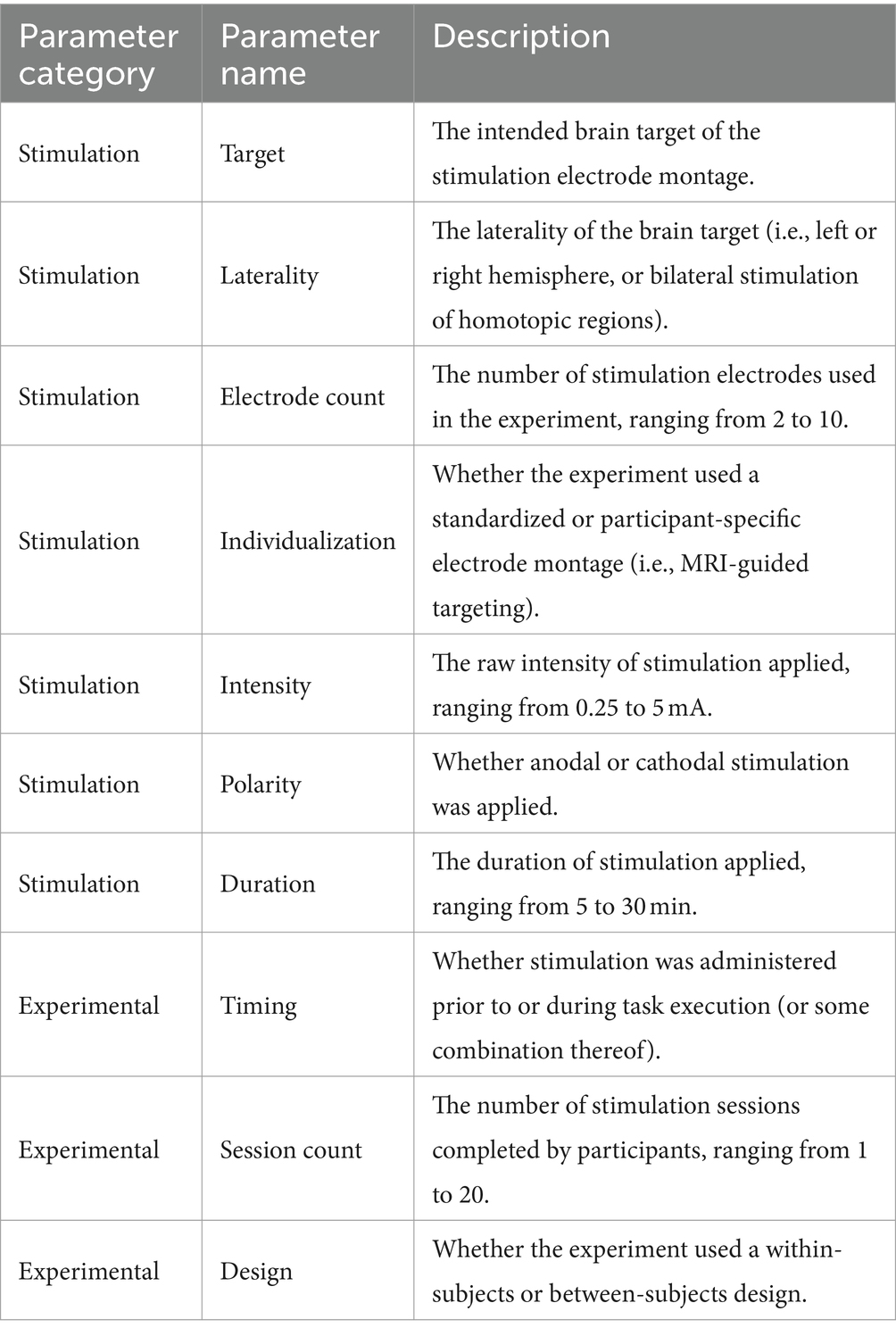
Table 1. The 10 independent variables considered under our meta-regression models, including the category, name, and a brief description of each.
The scope of our approach builds upon prior reviews and meta-analyses that are often focused on specific cognitive domains and behavioral outcomes, or alternatively, on specific neural/physiological responses to stimulation. Mixed or otherwise inconclusive patterns of results have been well-characterized across many disparate areas of neurostimulation research (Koenigs et al., 2009; Loo et al., 2010; Jacobson et al., 2012; Loo et al., 2012; Brunoni and Vanderhasselt, 2014; Lopez-Alonso et al., 2014; Wiethoff et al., 2014; Chew et al., 2015; Horvath et al., 2015a,b; Lopez-Alonso et al., 2015; Dedoncker et al., 2016; Hill et al., 2016; Horvath et al., 2016a,b; Mancuso et al., 2016; Vannorsdall et al., 2016; Heroux et al., 2017; Imburgio and Orr, 2018; Loo et al., 2018; Oldrati and Schutter, 2018; Rassovsky et al., 2018; Falcone et al., 2019; Schroeder et al., 2020; Wischnewski et al., 2021). However, the most popular approach to quantifying and describing heterogeneity is—understandably—rather piecemeal. Past studies addressing potential moderating factors (e.g., the polarity of stimulation, or the laterality of a target region) typically either parse out effects for separate analyses (e.g., looking at trends for left vs. right hemisphere stimulation independently, rather than modeling the contrast) or perform meta-regression based on small subsets of features (Jacobson et al., 2012; Brunoni and Vanderhasselt, 2014; Dedoncker et al., 2016; Hill et al., 2016; Mancuso et al., 2016; Imburgio and Orr, 2018; Oldrati and Schutter, 2018; Schroeder et al., 2020). Our goal, in contrast, is to consider a wider breadth of tasks, experiment parameters, and domains to explicitly quantify systematic differences in behavioral outcomes associated with these design factors.
1.1 Stimulation parameters
One critical consideration for experimenters is determining the arrangement of electrodes on the scalp to effectively target a brain region of interest. When doing so, experiments tend to rely upon methods demonstrated as effective in extant research, and/or on results derived from models of predicted current flow (Datta et al., 2009; Bikson et al., 2012, 2013; Ramaraju et al., 2018; Rich and Gillick, 2019). Researchers typically arrange electrodes on the scalp in reference to an anatomical coordinate system, such as the International 10–20 System (DaSilva et al., 2011), and/or based on outcomes from neuronavigation or peak activations identified in functional tests (e.g., motor-evoked potentials). In all cases, the intent is to maximize current density at a brain target believed to be functionally-relevant for a cognitive process or behavioral outcome of interest (Miranda et al., 2009; Sadleir et al., 2012; Rich et al., 2017). The target brain regions identified in our review included the dorsolateral prefrontal cortex (DLPFC), posterior parietal cortex (PPC), and primary motor cortex (M1), as well as a number of other diverse stimulation targets spanning nearly the whole brain.
The targeted brain regions were variably lateralized, with most reported effects (418 out of 721 total) localized to the left hemisphere and a minority of effects derived through bilateral stimulation of homotopic regions (59 total). When referring to laterality of stimulation, it is typically the case that the anodal electrode is placed over the targeted region (e.g., left DLPFC), and a reference cathodal electrode is placed over the contralateral hemisphere (e.g., right supraorbital area) or on an ipsilateral or contralateral extracephalic area (e.g., shoulder, bicep). While often treated as a relatively trivial decision in practice, it is important to realize that the placement of the cathode likely carries important implications for physiological and behavioral influences of tDCS (Bikson et al., 2010).
Most studies we identified used two saline-soaked sponges, one anode and one cathode, to administer tDCS; other studies used multi-electrode montages that are assumed to provide higher focality and maximize current density at both superficial and relatively medial targets (Datta et al., 2009). We also found that most studies tended to use a consistent electrode positioning across individuals, whereas a minority used individualized montages based on structural and/or functional magnetic resonance imaging (MRI) scans. Individualized montages are becoming more commonplace and are thought to compensate for varied brain morphology, functional neuroanatomy, and head sizes and shapes (Datta et al., 2012; Kim et al., 2014; Woods et al., 2016).
Stimulation procedures often involve a slow ramp-up (e.g., over a 30 s window) to a target intensity, maintaining that peak intensity level for a specific duration (e.g., 20 min), and then a slow ramp-down to terminate stimulation (Nitsche and Paulus, 2000; Gandiga et al., 2006). Peak stimulation intensity is typically between 0.5–2.0 milliamperes (mA; with the conventional limit being 2 mA) (Nitsche and Bikson, 2017), with one meta-analysis demonstrating a mean tDCS stimulation intensity of 1.5 mA when considering studies done in the cognitive domain (Jacobson et al., 2012). Modeling and experimental work suggests that stimulation intensity can have paradoxical, nonlinear effects on neuronal activity and behavioral effects of tDCS (Batsikadze et al., 2013; Bonaiuto and Bestmann, 2015; Esmaeilpour et al., 2018). For example, 1 mA stimulation intensity can produce hyperpolarization whereas 2 mA can produce depolarization of motor neurons (Batsikadze et al., 2013). One meta-analysis suggests that on the aggregate, higher intensity stimulation (operationalized as current density under the anode) is more likely to benefit task accuracy during DLPFC stimulation (Dedoncker et al., 2016). In our review, however, we focused primarily on raw stimulation intensity (as opposed to current density) and found studies administering stimulation as low as 0.25 mA intensity and as high as 5 mA.
The duration of peak stimulation intensity is typically between 5 and 30 min (as seen in our review), with one meta-analysis demonstrating an average tDCS stimulation duration of 15.2 min when considering studies performed in cognitive domains (Jacobson et al., 2012). Some of the nonlinear responses found with stimulation intensity can interact in unexpected ways with stimulation duration. For example, 1 mA of tDCS can induce depolarization during the first 13 min of stimulation, but hyperpolarization during a latter 13 min of stimulation (Monte-Silva et al., 2013).
A further critical decision point in designing a tDCS study is whether to deliver anodal or cathodal stimulation to the target site. Some mechanistic models of tDCS, namely sliding scale models, suggest that stimulation polarity influences whether tDCS can increase or decrease neural activity (Bikson et al., 2013; Bestmann et al., 2015). Specifically, neuronal populations residing in brain regions underlying the anode will experience depolarization, and populations under the cathode will experience hyperpolarization. This simplistic model has been repeatedly challenged (de Berker et al., 2013; Bestmann et al., 2015; Jackson et al., 2016; Bestmann and Walsh, 2017), but remains as a strong motivator for scientists who compare (experimentally and statistically) anodal versus cathodal stimulation over a target brain region, typically in reference to a sham condition.
Thus, stimulation parameters are variably selected and applied across studies. It is known that variation of certain parameters can elicit unexpected and nonlinear effects on brain physiology and behavior, and it is likely that many of these parameters interact in ways that have yet to be discovered. Here we focus primarily on additive, main effects due to the combinatorial explosion in model parameter space when exploring interactions between our 10 predictors of interest. However, it is still of central interest to uncover how these various factors might jointly-modulate one another in any given neurostimulation experiment.
1.2 Experimental and statistical parameters
In addition to stimulation parameters, our model also considered the influence of 3 experimental design parameters. Across studies, tDCS is variably administered either before task performance (offline), during task performance (online), or a mixture of both (offline-online) (Woods et al., 2016; Imburgio and Orr, 2018). Original research and meta-analyses suggest that offline stimulation is more likely than online stimulation to enhance performance on working memory tasks (Hill et al., 2016; Friehs and Frings, 2019; Zivanovic et al., 2021), but that the opposite might be true for skill acquisition (Martin et al., 2014) and visuospatial tasks (Oldrati et al., 2018). In our review, we indeed found that offline stimulation was employed more frequently than online stimulation (347 vs. 276 effects, respectively), with comparatively fewer studies using a mixed-timing design. Furthermore, while many studies use a single-session design involving only one (e.g., 20 min) administration of tDCS during a single visit to the laboratory, some studies involve the repeated administration of tDCS over the course of successive visits. While repeated sessions of tDCS are a common design feature in clinical research and are associated with higher treatment efficacy relative to single-session studies (Fregni et al., 2006; Boggio et al., 2007; Loo et al., 2010, 2012), they are used less frequently in studies with healthy neurotypical adults. When such a design is used, it is typically in the context of studies examining the effects of tDCS on cognitive training or skill acquisition paradigms. In general, it is thought that repeated tDCS can facilitate memory consolidation (Reis et al., 2009; Marshall et al., 2011), and meta-analyses suggest there may be insufficient evidence to evaluate any potential advantage of repeated- versus single-session tDCS on cognitive function (Horvath et al., 2015a).
In tDCS studies, within-participants designs are generally preferred to between-participants design given the inherent challenges associated with inter-participant variation in tDCS experiments, including factors such as the brain’s morphology and function, genetic attributes, consumption patterns (e.g., caffeine), menstrual cycle, aptitudes, and other trait-based variables (Lopez-Alonso et al., 2015; Vergallito et al., 2022). They are also considered the more powerful design for detecting differences among conditions because analyses partition out between-participants variance and reduce error (Kirk, 2013). That said, many studies use between-participants designs, typically for scheduling efficiency (tDCS sessions are typically separated by at least 24 h), to avoid carryover effects, or because the associated large sample size helps afford analyses of individual differences. Some studies balance power, efficiency, and scale by using mixed designs, which include one or more within- and between-participants factors. However, estimation of mixed effects, particularly in the context of the models described here (which generally focus on binary comparisons between conditions rather than factorial comparisons), is less straightforward. We therefore focus on studies using either a between-subjects or within-subjects design, which were represented fairly equivalently across the domains considered in our review.
1.3 Performance domains
The meta-analytic model was designed to understand how the 10 stimulation and experimental parameters are associated with performance outcomes across 5 cognitive domains, including vigilance, working memory, visual search, response inhibition, and motor performance. We chose these five domains because they have all received considerable attention in the scientific literature and exhibit variability in the brain regions targeted, the stimulation methods, and/or the experimental and statistical methods employed. Moreover, and perhaps most importantly, these 5 domains tend to underlie performance in many applied settings of interest including driving, aviation, and military.
Vigilance refers to a sustained focus of attention on stimulus detection tasks over lengthy periods of time (Parasuraman, 1976; Warm et al., 2008; Hancock, 2017), such as when continuously monitoring a radar screen. Maintaining vigilance is stressful and places high demands on attentional resources, resulting in vigilance decrements (i.e., reduced detection of critical stimuli) over time (Grier et al., 2003; Warm et al., 2008). Given its reliance on arousal and alertness, sustained attention, and information processing, many brain systems have been implicated in vigilance performance. These include the prefrontal cortex, reticular formation, thalamus, and basal forebrain cholinergic system (Parasuraman et al., 1998). In general, anodal tDCS targeting the DLPFC appears to reduce vigilance decrements (McIntire et al., 2014; Nelson et al., 2014), though one study showed that the same tDCS increased the rate of mind wandering during a vigilance task (Axelrod et al., 2015). From our review of the literature, we included 93 effects derived from tDCS experiments on vigilance, primarily involving DLPFC stimulation.
Working memory refers to mechanisms responsible for temporarily storing, processing, and manipulating task-relevant information in memory (Baddeley, 1992; Miyake and Shah, 1999). Working memory plays a pervasive role in daily life and is a critical process underlying performance on planning, reasoning and problem solving, and decision-making tasks (Kyllonen and Christal, 1990; Davidson and Sternberg, 2003; Hinson et al., 2003; Gilhooly, 2004). It has also been a topic of interest among cognitive neuroscientists interested in mapping working memory processes to brain regions and networks, which has found strong evidence that the lateral prefrontal cortex is involved in the temporary maintenance of task-relevant information, and that the distribution of brain activity across widespread networks is dependent on many task-related parameters such as the sensory modality being used (e.g., visual, auditory), the nature of stimuli (e.g., verbal, spatial, motor, faces) being maintained or manipulated, and whether the information is retrospective or prospective (D'Esposito, 2007). In general, the prefrontal cortex appears to be a critical node in a distributed working memory network that coordinates the involvement of other brain regions more specialized in specific functions (e.g., sensory, representational, and action-related) (Postle, 2006). The effects of tDCS on working memory performance have engendered some debate in the scientific literature, with some meta-analyses suggesting improvement of working memory (in accuracy or response times) with anodal tDCS targeting the left or right DLPFC (Brunoni and Vanderhasselt, 2014; Dedoncker et al., 2016; Hill et al., 2016; Mancuso et al., 2016), and another meta-analysis suggesting no evidence for improvement (Horvath et al., 2015a). From our review of the literature, we included 115 effects of tDCS on working memory, also primarily involving PFC stimulation.
Visual search refers to the process of finding a visual target among distractors, and is typically assumed to involve interactions between preattentive processing and focal attention (Wolfe, 2010; Eckstein, 2011; Chan and Hayward, 2013). Visual search is extremely common in applied and daily tasks, such as searching for a weapon in luggage, finding lung nodules on a radiograph, identifying suspects in a crowd, or simply finding a matching pair of socks (Eckstein, 2011). It also recruits a wide range of brain regions including ventral and dorsal regions of the prefrontal cortex (and frontal eye fields; FEF) (Anderson et al., 2007), multiple areas of the parietal cortex (Donner et al., 2000), and the occipital cortex (Nobre et al., 2003). Studies suggest that anodal and cathodal tDCS over the left FEF can improve target detection during a visual search task (Nelson et al., 2015), that cathodal stimulation of the right posterior parietal cortex (but not FEF) can reduce the benefits of practice in a visual search task (Ball et al., 2013), and that anodal stimulation of the right inferior frontal or posterior parietal cortex can enhance performance on a task involving searching for threats in complex scenes (Falcone et al., 2012; Callan et al., 2016). From our review of the literature, we included 176 tDCS effects on visual search, primarily involving PFC and parietal stimulation.
Inhibitory control refers to the ability to suppress thoughts or behaviors that are not relevant or conducive to task performance, and is considered a critical executive function that enables adaptive behavior (Chambers et al., 2009; Congdon et al., 2012). Response inhibition enables the adaptive control of cognitive processes and motor behavior, and failure of response inhibition is through to underlie both clinical and subclinical impulsivity (Horn et al., 2003). In applied and daily tasks, response inhibition enables people to inhibit prepotent behavioral responses across a range of settings—such as firing or not firing a weapon (Biggs et al., 2015), swinging or not swinging a baseball bat (Nakamoto and Mori, 2012), or not eating a second piece of cake (Appelhans, 2009). Dominant theories of inhibitory control emphasize the role of several regions of the prefrontal cortex, including the dorsolateral, inferior, and orbital frontal cortex (Duncan and Owen, 2000; Aron et al., 2004). While some debate exists regarding the modular versus distributed roles of prefrontal brain regions in inhibitory processes (Hampshire and Sharp, 2015), particular emphasis has been given to the right inferior frontal gyrus given results of functional neuroimaging, lesion, and transcranial magnetic stimulation (TMS) studies (Aron et al., 2004, 2014). With tDCS, a recent meta-analysis demonstrated generally small but significant effect of tDCS on inhibitory control outcomes (including go−/no-go task and stop-signal task), especially with right inferior frontal gyrus stimulation (Schroeder et al., 2020). From our review of the literature, we included 84 effects on inhibitory control, primarily involving PFC stimulation.
Finally, motor performance here refers to the process of acquiring new abilities to perform novel sequences of skilled behaviors to accomplish a goal, from typing on a keyboard to riding a bike. Acquiring a new skill relies upon experience-dependent neuroplasticity in the brain, often tied to practice and consolidation (Karni et al., 1998; Robertson et al., 2004), which can occur over the course of hours, days, or weeks (Korman et al., 2003). Neuroplastic changes associated with motor skill acquisition are often considered the locus of the primary motor cortex (Karni et al., 1995, 1998; Sanes and Donoghue, 2000). In addition to the motor cortex, the cerebellum has received attention due to its potential involvement in the initiation of limb movements and the improvement of motor skills (Gilbert and Thach, 1977; Houk et al., 1996; Thach, 1996; Kitazawa et al., 1998). Studies using tDCS to influence motor skill acquisition variably target the primary motor cortex and cerebellum. Reviews and meta-analyses suggest that anodal tDCS targeting the primary motor cortex can improve motor learning and motor function (Reis and Fritsch, 2011), and both anodal and cathodal tDCS targeting the cerebellum can accelerate motor learning, motor adaptation, and procedural learning (Oldrati and Schutter, 2018). This domain represented the largest in our survey of the literature, spanning 253 effects related to motor and procedural skill acquisition.
2 Methods
2.1 Study search and selection
A literature search was performed by authors S.L. and P.S. using PsycINFO, PubMed, Google Scholar, and the tDCS Database.1 The key search terms were [tDCS] or [HD-tDCS] in combination with any of the following domain-specific terms: [motor skill], [motor learning], [procedural learning], [implicit learning], [skill learning], [working memory], [vigilance], [endurance], [visual search], and [inhibition]. Unions of key terms (using the AND function) were queried using the options for All Fields or Any Field for three of the selected databases (PubMed, PsycINFO, and the tDCS Database); when searching Google Scholar, key search terms were queried in the full text, and due to the high search yield only the first 300 results were included for screening. Searches were not restricted by publication date.
Because we sought to model specific effects of experimental design parameters on behavioral performance outcomes, we applied strict inclusion criteria to narrow down relevant studies. Beyond the basic requirement of publication in a peer-reviewed scientific journal, these included: (1) no studies focused exclusively on minors, older adults, or individuals with neuropsychiatric conditions (we extracted only effects related to healthy younger adults in studies based on these comparisons); (2) application of tDCS and not tACS/tRNS (although again, in cases where these were compared, we extracted only tDCS-related effects); (3) ability to extract simple within-subject or between-subject effects; (4) binary comparisons between either anodal or cathodal stimulation vs. sham stimulation (i.e., no anodal vs. cathodal comparisons, or comparisons between active stimulation conditions across different intensities); (5) stimulation targeted over a specific brain region (e.g., focused on DLPFC alone rather than a ‘network-like’ approach to neuromodulation, exciting both frontal and parietal areas); (6) reporting of behavioral outcomes (i.e., we did not include effects on fMRI or EEG-derived brain activity); and (7) sufficiently clear reporting of all stimulation parameters/procedures. This yielded 42 studies in the motor domain (253 total effects) (Antal et al., 2004; Boggio et al., 2006; Vines et al., 2008; Tecchio et al., 2010; Leite et al., 2011; Ferrucci et al., 2013; Saucedo Marquez et al., 2013; Vollmann et al., 2013; Furuya et al., 2014; Prichard et al., 2014; Zimerman et al., 2014; Cantarero et al., 2015; Minarik et al., 2015; von Rein et al., 2015; Ambrus et al., 2016; Doppelmayr et al., 2016; Ehsani et al., 2016; Naros et al., 2016; Fan et al., 2017; Filmer et al., 2017a; Focke et al., 2017; Fujiyama et al., 2017; Hashemirad et al., 2017; Pixa et al., 2017; Rumpf et al., 2017; Samaei et al., 2017; Apsvalka et al., 2018; Dumel et al., 2018a,b; Ballard et al., 2019; Fehring et al., 2019; Jin et al., 2019; Shilo and Lavidor, 2019; Spampinato et al., 2019; Talimkhani et al., 2019; Pollok et al., 2020; Rocha et al., 2020; Ballard et al., 2021; Nguemeni et al., 2021; Parma et al., 2021; Sevilla-Sanchez et al., 2021; Toth et al., 2021), 18 visual search studies (176 effects) (Bolognini et al., 2010; Clark et al., 2012; Coffman et al., 2012; Ball et al., 2013; Ellison et al., 2014; Cosman et al., 2015; Nelson et al., 2015; Reinhart and Woodman, 2015; Callan et al., 2016; Ellison et al., 2017; Filmer et al., 2017a,b; Falcone et al., 2018; Lanina et al., 2018; Nydam et al., 2018; Sung and Gordon, 2018; Grasso et al., 2020; Wagner et al., 2020), 16 working memory studies (115 effects) (Boehringer et al., 2013; Zwissler et al., 2014; Nikolin et al., 2015; Au et al., 2016; Chrysikou et al., 2017; Naka et al., 2018; Abellaneda-Perez et al., 2019; Ankri et al., 2020; Baumert et al., 2020; Hussey et al., 2020; Murphy et al., 2020; Assecondi et al., 2021; Karthikeyan et al., 2021; Pupikova et al., 2021; Zivanovic et al., 2021; Caulfield et al., 2022), 15 vigilance studies (93 effects) (Plewnia et al., 2013; Manuel et al., 2014; McIntire et al., 2014; Nikolin et al., 2015; Hanken et al., 2016; Ironside et al., 2016; McIntire et al., 2017; Borragan et al., 2018; Brunnauer et al., 2018; Jacoby and Lavidor, 2018; Naka et al., 2018; Filmer et al., 2019; Coulborn et al., 2020; Luna et al., 2020; van Schouwenburg et al., 2021), and 15 inhibition studies (84 effects) (Hammer et al., 2011; Gladwin et al., 2012; Plewnia et al., 2013; Oldrati et al., 2016; Gomez-Ariza et al., 2017; Friedrich and Beste, 2018; Angius et al., 2019; Boudewyn et al., 2019; Denis et al., 2019; Dubreuil-Vall et al., 2019; Edgcumbe et al., 2019; Kuehne et al., 2019; Baumert et al., 2020; Hussey et al., 2020; Thomas et al., 2020). A summary flowchart of search and screening procedures is shown in Figure 1; the studies included in each domain are listed for convenience in Supplementary Table S1. We note that this represents only a subset of all published studies under each domain (some of which employed multiple tasks capturing various cognitive constructs) and is not intended to be exhaustive; however, we suggest the total number of effects considered affords a reasonable estimation of how various stimulation and study design parameters might modulate behavior.
2.2 Data extraction, effect size estimation, and parameter coding
Whenever possible, we extracted exact descriptive statistics (means and standard deviations, or standard errors) from the reported text. However, in many cases, this information was only presented graphically. We therefore used the WebPlotDigitizer2 to convert graphical displays into numerical data. Effects were excluded here if error bars were visually indistinguishable and/or if their endpoints could not be reliably identified. For each study, we attempted to extract as much behaviorally-relevant information as possible, particularly when multiple effects were reported across different experiment blocks or outcome variables (e.g., accuracy and/or reaction time), allowing us to better capture variability in the effects of tDCS on performance—which may be transient over the course of a task. Irrelevant, non-task-related measures were excluded (e.g., self-reported side effects), as were ancillary task-related measures that did not inform the cognitive domains of interest (e.g., for inhibition, we excluded reaction times to ‘congruent’ trials in Stroop tasks).
Effect sizes and their standard errors were estimated using Hedges’ g. For between-subject designs where group means, standard deviations, and sample sizes are known, this is trivial; however, for within-subject designs, the standard deviation around the mean difference depends upon the correlation between repeated measures. No study in our survey of the literature provided this information. We ultimately chose to assume a moderate correlation (r = 0.50) for all within-subject effects (equivalently, taking an average of the variances)—a common practice in power analyses when the within-subject correlation is not known a priori. Furthermore, to enable more generalized assessments of behavioral outcomes regardless of the exact task paradigm or behavioral construct being measured, each effect size was assigned a positive or negative sign depending on whether the relevant comparison indicated an improvement or detriment to behavior, respectively. For example, faster reaction times during active stimulation vs. sham were assigned a positive sign, whereas higher error rates earned a negative sign. Thus, under the model, we could assess whether certain experiment design parameters conferred positive or negative effects on average for a given domain, irrespective of specific tasks or outcomes.
Each effect was coded alongside the 10 stimulation and experiment parameters summarized in Table 1. The stimulation target parameter was given as the intended brain region targeted by the electrode montage. In cases where some brain regions were poorly-represented (i.e., were only targeted in one or two studies, or constituted an exceptionally small proportion of the total number of effects), we attempted to collapse them into an ‘Other’ category grouped with other anatomically-proximal regions. Target laterality was coded as either Left, Right, or Bilateral (in the latter case, when stimulation was applied to homotopic regions in both the left and right hemisphere). We also recorded the number of electrodes used in the montage and whether the placement of electrodes was individualized (e.g., guided by an anatomical MRI for each subject; coded as a binary Yes or No). Individualized target montages were exceedingly rare, occurring primarily in the motor performance domain. Raw stimulation intensity was recorded in milliamperes and polarity coded as either Anodal or Cathodal (always in comparison to a sham stimulation condition). The duration of stimulation was given in minutes and coded as Online (applied during task performance), Offline (prior to task performance), or Mixed (for cases when online stimulation was applied for only a portion of task performance). The total number of stimulation sessions completed by participants was also given for each effect. Finally, we recorded whether each comparison was made under a within-subjects or between-subjects framework. A summary of how these parameters were represented under each domain can be found in Supplementary Table S2.
As an additional supplementary analysis, we also attempted to extract or estimate current density over the target brain region (in mA / cm2). This was possible for all studies in included in the visual search and vigilance domains, but it resulted in some loss of data for the motor, working memory, and inhibition domains (due to, e.g., the use of customized or otherwise more complex multielectrode arrays for which it was not possible to reliably estimate current density). We found that this parameter did not inform variability in behavioral outcomes and generally exhibited much greater posterior uncertainty; thus, given our already-strict inclusion criteria, we do not use it in the models and results described below. For completeness, however, we visualize these models in Supplementary Figure S1.
2.3 Hierarchical Bayesian meta-regression: model specification and estimation
Given that nearly all studies reported multiple behavioral outcomes as a function of their tDCS manipulations, we used a hierarchical Bayesian meta-regression framework to model the average magnitude of behavioral effects associated with our design parameters of interest, accounting for both intra- and inter-study variability in the effects of neurostimulation across all reported comparisons. We elected to use a Bayesian framework over a comparable frequentist framework for several reasons. First, Bayesian methods afford probabilistic estimates in favor of hypotheses and the existence of an effect by computing full posterior distributions given the observed data, which in turn allow for more direct estimates of uncertainty surrounding an effect. Second, Bayesian methods allow us to be pragmatic in incorporating our prior beliefs (or perhaps alternatively, our lack of strong prior convictions) into a model and help us control for potential bias by retaining identical prior assumptions regardless of the data or cognitive domain. Finally, Bayesian methods allow for principled, probabilistic model comparison, useful for exploratory analyses and quantifying uncertainty in performance between models using different sets of parameters.
All models were specified and estimated using Stan3 and brms (Bürkner, 2017) in R. For consistency, and to avoid the possibility that any given model could be unduly influenced by arbitrary prior specifications, key parameters for all models were estimated under the same set of weakly-informative priors:
Note that, because we obtained standard errors around each recorded effect size, we could incorporate these values directly into the model likelihoods, precluding the need to estimate noise variance as an additional free parameter. These priors were selected based on previous recommendations for Bayesian meta-analysis (Williams et al., 2018; Reis et al., 2023) with one notable change. Typically, a traditional meta-analysis only models an intercept (capturing the average effect size pooled across studies): a Normal(0, 1) prior for this term is arguably quite sensible in these situations (this assumes with 95% probability that the ‘true’ pooled effect lies somewhere between g = [−2, 2]). However, here we are not just pooling effect sizes on average, but also determining the extent to which effect sizes are systematically moderated by differences in experiment design factors (that is, we also need to model slopes). Given that we do not necessarily have a good a priori guess as to how, for example, effect size might differ on average between Anodal and Cathodal stimulation, we use a wider, weaker prior.
In constructing the design matrix for each model, categorical regressors (e.g., stimulation polarity) were coded using sum-to-zero deviation contrasts, which enable more traditional ‘ANOVA-like’ comparisons of mean differences between a given factor level and a reference level. Continuous regressors (e.g., stimulation intensity) were centered about their respective means. We further specified a random intercept term to account for variation in reported effects across studies. Random slopes were omitted, both to minimize model complexity (and thereby ensure convergence) and because many comparisons are empirically unidentifiable within a given study (e.g., many studies reported either anodal vs. sham or cathodal vs. sham stimulation designs, so one cannot estimate differential effects between the two on an individual study level). Thus, using Wilkinson notation, an additive model containing all predictors may be written as:
Continuous predictors such as stimulation intensity imply a single slope, while categorical factors such as stimulation polarity imply k – 1 contrasts, where k is the number of factor levels (so, for example, the polarity term tests for differences associated with Anodal vs. Cathodal stimulation, whereas the stimulation timing term may imply two fit parameters: one for Offline vs. Mixed comparisons, and one for Online vs. Mixed).
Robust exploration of the posterior space for all models was performed via Hamiltonian Monte Carlo, with four independent chains of 15,000 iterations each (5,000 of which were used as warm-up samples). We ensured that all models properly converged to equilibrium for all parameters using classical benchmarks, including: the effective sample size (considering the autocorrelation between independent posterior draws); the Monte Carlo standard error (relative to the posterior SD); R-hat (the variance ratio between each chain relative to all chains); and no divergent Monte Carlo transitions after warm-up.
For statistical inference, we consider 95% credibility intervals (using the highest posterior density interval) around the posterior median parameter estimates along with the ‘probability of direction’ (pd), capturing the proportion of the posterior density above or below zero. Here, pd can range from 0.50 (indicating that the posterior is centered at zero, i.e., half its density lies above zero and half below zero) to 1 (in the most extreme case, that no proportion of the posterior density intersects zero)—thus, values of pd closer to 1 indicate more evidence for the presence of a directional/nonzero effect, and pd > 0.95 can roughly be thought of as a Bayesian analogue to the frequentist p-value, such that less than 5% of the full posterior distribution intersects zero (Makowski et al., 2019). However, an important point here is that we do not wish to frame these results in terms of ‘statistical significance’, but rather a simple consideration of evidence for nonzero differences given the posterior densities for each parameter. Bayes factors are not reported due to their sensitivity to priors and because they often (regrettably) encourage the sorts of binary thinking about hypotheses that Bayesian methods are meant to circumvent. Instead, we compute a Bayesian analogue of Cohen’s d for categorical predictors by taking the posterior draws for each regressor and dividing them by the square root of the summed residual variances and random effects variances (as per convention for hierarchical linear models) (Westfall et al., 2014), yielding a posterior distribution for each non-continuous model-level effect. Finally, fit indices were computed using the conditional R2 over the full model (taking both fixed and random effects together), and the marginal R2 capturing variance that can be attributed to the fixed effects alone (Gelman et al., 2019).
2.4 Exploratory modeling of interaction effects
The models described above focused simply on additive, main effects for each predictor of interest—we did not have any a priori hypotheses related to how these various experiment design parameters might interact to produce differential behavioral outcomes. However, it is likely that interaction effects do exist between these factors. One general challenge with modeling these potential effects in an exploratory fashion is combinatorial explosion: given the number of parameters under consideration, the possible model space is massive and may include models with many higher-order interactions that are uninterpretable.
In an effort to perform an exploratory search in a restricted, albeit principled and maximally-unbiased fashion, we focused on models containing either two-way or three-way interactions (the latter of which included all three marginal two-way interactions) constructed on a dataset comprised of all domains combined. This would allow us to identify interaction models that appear to generalize across domains and subsequently test the extent to which they inform domain-specific models. To assess the performance of candidate models against the ‘base model’ (i.e., solely including additive, main effects), we used Bayesian approximate leave-one-out cross validation with Pareto-smoothed importance sampling (PSIS) (Vehtari et al., 2017; Paananen et al., 2021; Vehtari et al., 2024). In brief, this approach takes advantage of our fully Bayesian modeling framework to circumvent typically-costly cross-validation routines requiring many model re-fits, instead using PSIS to imagine the likelihood our model would assign to each datapoint as if they were held-out, yielding a new cross-validated posterior predictive distribution over the data (a pointwise predictive density). We can then directly quantify the difference in predictive performance between candidate and base models by comparing their mean, expected log pointwise predictive densities (ELPDs), which has an associated standard error: the ratio of the ELPD differential to the standard error of the difference (akin to a Z-statistic) is a simple summary metric that can inform us whether the addition of putative interaction terms significantly improves predictive performance above the base, additive model (a fairly liberal criterion is to check whether the absolute value |ELPDDiff / SEDiff| > 2, similar to a two-tailed test at α = 0.05).
Under this framework, we first performed a comprehensive sweep over all possible inclusions of a single two-way interaction term (28 models total). None of these candidate models, however, suggested a significant improvement in predictive performance above the base model (Supplementary Table S3). We then performed a similarly exhaustive sweep over 56 candidate three-way interaction models, and here, there were three models that suggested significant improvement over the base model (Supplementary Table S4), each with the following terms: (1) Design Type (Within- vs. Between-Subjects) × Number of Electrodes in Montage × Stim Intensity (mA); (2) Design Type × Number of Electrodes × Stim Duration per Session (min); and (3) Design Type × Number of Electrodes × Stim Duration. Notably, there was considerable overlap in the relevant factors for each candidate interaction—we therefore fit a four-way interaction model that provided, at best, a marginal improvement over the base model and did not significantly outperform any of the individual three-way models (Table 2).
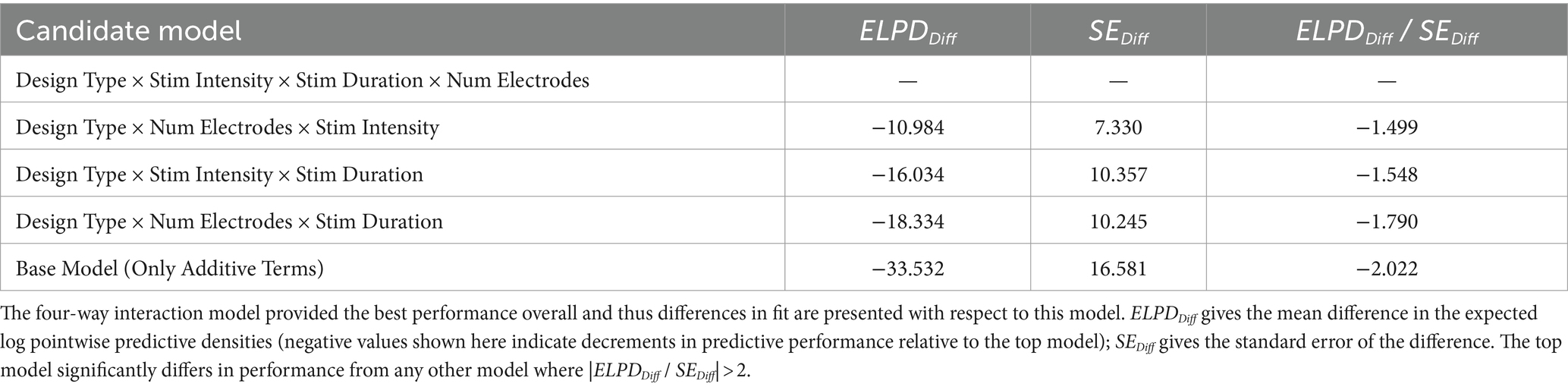
Table 2. Differences in candidate model predictive performance derived via Bayesian approximate leave-one-out cross-validation.
Because the four-way model did not confer a particular advantage over any individual three-way model, we excluded it from further testing and instead consider the various three-way models noted above to be competitive. These were subsequently applied to each individual domain to assess the extent to which they may inform domain-specific differences in behavioral effects above and beyond their respective base, additive models.
3 Results
3.1 Motor performance
Under a simple additive model, analysis of 253 effects across 42 studies in the motor domain revealed little consistency in the relationships between stimulation/experiment parameters and the likelihood of conferring positive or negative behavioral effects (Figure 2A; Table 3). However, there was a considerable advantage when using a within-subjects design vs. a between-subjects design, such that within-subjects designs were consistently associated with stronger positive effects (d = 1.35, 95CI = [0.93, 1.78]; b = 1.11 SD = 0.22, 95CI = [0.68, 1.55], pd = 1). We also observed some evidence for target-specific effects, such that stimulating DLPFC tended to yield smaller behavioral differences, relative to the more motor-relevant cerebellum (d = −0.53, 95CI = [−1.13, 0.002]; b = −0.43, SD = 0.22, 95CI = [−0.86, −0.01], pd = 0.977), although the credibility intervals for this effect lightly-intersect zero. We note that, due to restrictions on the number of possible contrasts one can uniquely-estimate for a given factor, there remain many potential comparisons that are not reflected under the model fit. However, given that this is a Bayesian framework, it is possible to ‘simulate’ new data under the generative model and derive posterior predictive distributions that describe the expected value (i.e., the likely effect size) one would observe under certain stimulation/experiment parameters. By integrating out other factors from these predictive distributions, we can approximate the average marginal effect (AME) one might expect for novel comparisons. This here reveals potential benefits to stimulating M1 vs. DLPFC (Median predictive difference = 0.35, 95CI = [0.11, 0.58]) as well as a potential detriment to stimulating online vs. offline (Median predictive difference = −0.20, 95CI = [−0.39, −0.01]).
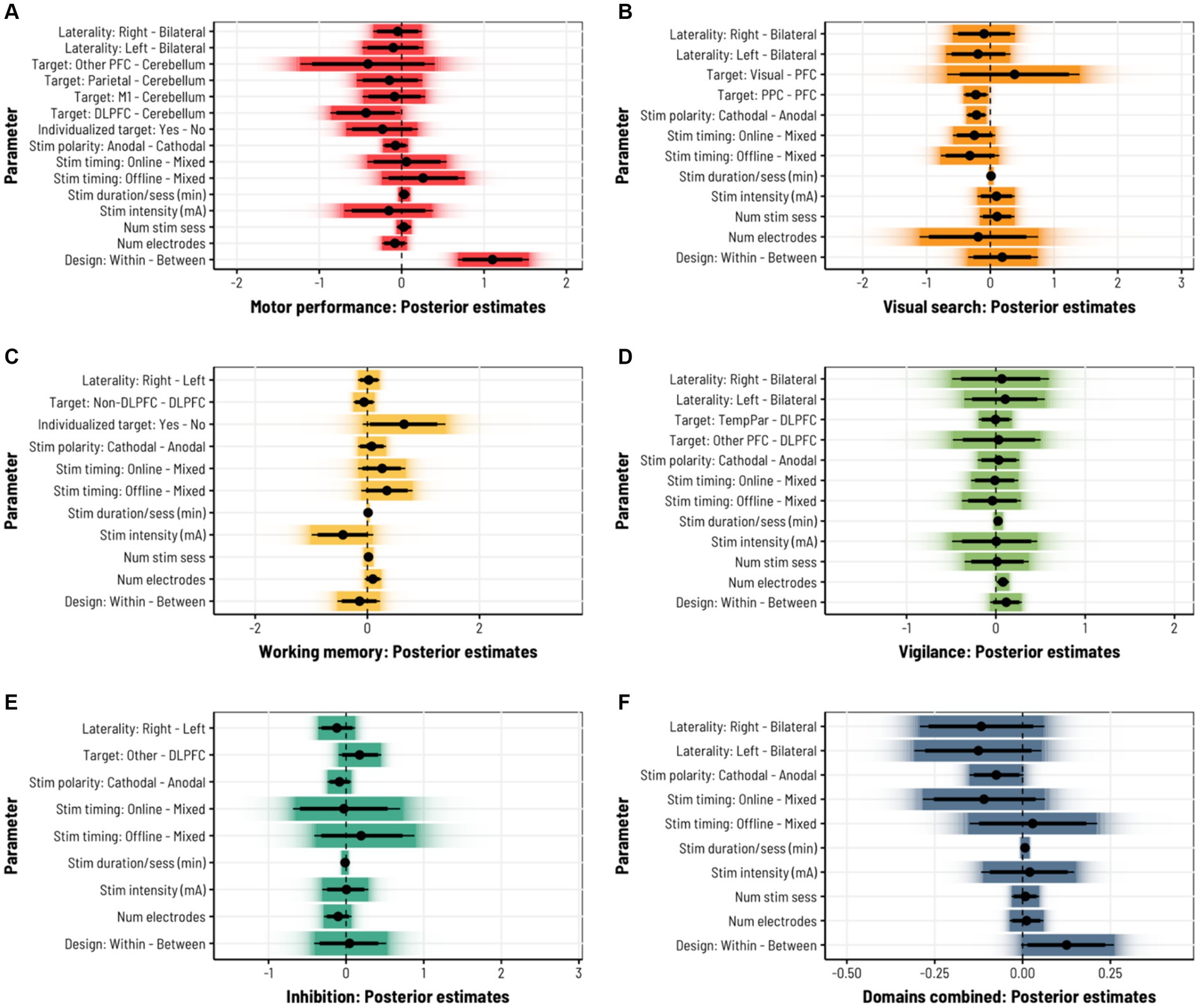
Figure 2. Contrasting estimates of multiple neurostimulation and experimental parameters on behavioral performance outcomes, both within-domain and between-domain. For categorical predictors (e.g., target laterality), contrasts were specified in an A – B fashion, such that level B served as the reference point. Thus, positive values suggest larger effect sizes (on average) for level A compared to B; negative values indicate the reverse. For continuous predictors (e.g., stimulation intensity), positive values suggest larger effect sizes with increasing values of the predictor (e.g., stronger stimulation dosages); negative values suggest the inverse association. Values closer to zero indicate weak or no association with performance outcomes, either at the level of mean differences between factor levels or a linear relationship with continuous predictors. Point intervals give 90–95% credibility intervals (i.e., highest density intervals) around the median posterior estimates (thick lines = 90% CI; thin lines = 95% CI; solid gradient areas indicate the 95% CI). (A) Motor performance, (B) Visual search, (C) Working memory, (D) Vigilance, (E) Inhibition, (F) Domain-General.
Applying the candidate interaction terms described previously to the motor domain did not reveal any significant increases in performance relative to the base model (Supplementary Table S5). The three-way interaction model including Design Type × Num Electrodes × Stim Duration provided the best overall predictive accuracy—however, this was not meaningfully different from the cross-validated performance of the base model with only additive terms (ELPDDiff = −33.53, SEDiff = 16.58, ELPDDiff / SEDiff = −1.88). That being said, while the highest-performing candidate model did not significantly outperform the base model, it nevertheless suggested the presence of potential interaction effects including (most importantly) the full three-way interaction (b = 0.76, SD = 0.28, 95CI = [0.21, 1.31], pd = 0.996). It is unclear whether the increase in model complexity is justifiable given the nonsignificant difference in predictive performance, but we report the full set of parameter estimates for this model in Supplementary Table S6.
3.2 Visual search
The visual search model spanned 176 effects across 18 studies (Figure 2B; Table 4). One challenge of this domain was the lack of variability in stimulation target sites. The posterior parietal cortex (PPC) was highly overrepresented as a stimulation target in this sample, comprising 110/176 effects; conversely, only four effects were derived from visual cortex stimulation. The remaining regions spanned various areas of the prefrontal cortex, including the FEF (14 effects) and DLPFC (16 effects). We elected to retain the visual cortex target as its own factor level (although this estimate is highly unreliable, as reflected by the wide credibility interval) and collapse all PFC regions into one group, yielding 62 total effects. Bilateral stimulation (14/176 effects) and mixed online/offline stimulation designs (15/176 effects) were also underrepresented here.
Despite the disparities across several of these parameters, we observed evidence for a moderate effect of stimulation polarity: cathodal stimulation was associated with smaller behavioral effects in these studies relative to anodal stimulation (d = −0.55, 95CI = [−1.14, −0.08]; b = −0.22, SD = 0.08, 95CI = [−0.37, −0.06], pd = 0.996). Perhaps counterintuitively, we also saw evidence for moderately weaker behavioral effects when targeting PPC compared to the combined grouping of all PFC targets (d = −0.58, 95CI = [−1.29, −0.004]; b = −0.23, SD = 0.10, 95CI = [−0.42, −0.03], pd = 0.988), although this effect is difficult to interpret given the lack of specificity in PFC regions. No additional trends were observed for other fixed effects or predictive AMEs in this domain.
With respect to potential interaction effects, here the base model provided the best predictive performance—strongly exceeding candidate interaction models with the exception of a model including a Design Type × Stim Intensity × Stim Duration interaction, with which it was tightly competitive (ELPDDiff = −0.10, SEDiff = 1.59, ELPDDiff / SEDiff = −0.06; Supplementary Table S7). Notably, however, despite the similarity in predictive performance, neither the three-way interaction term nor any of the marginal two-way interaction terms for this model provided clear evidence for nonzero effects. We observed, at best, an inconclusive trend for a two-way interaction between stimulation intensity and duration where the 95% credibility interval over the posterior included zero (b = 0.05, SD = 0.03, 95CI = [−0.003, 0.11], pd = 0.969). Beyond this, we only observed evidence for an effect of stimulation polarity in the same direction as seen in the base model (i.e., such that cathodal stimulation was associated with reduced behavioral effects on average; d = −0.95, 95CI = [−3.24, −0.02]; b = −0.23, SD = 0.08, 95CI = [−0.39, −0.07], pd = 0.998). The full summary of statistics for this competitive interaction model are compiled in Supplementary Table S8.
3.3 Working memory
We next assessed 115 effects reported in 16 working memory studies (Figure 2C; Table 5). Here we faced similar difficulties with unbalanced representation of target brain regions, with montages focused on the DLPFC in 90/115 reported effects. Other sparsely-represented regions (including the cerebellum, PPC, and temporal lobe) were therefore grouped into a ‘Non-DLPFC’ category. This domain was also the only other to employ subject-specific, individualized targeting—although this occurred only in one study with 5 reported effects. Furthermore, unlike other domains which applied anodal and cathodal stimulation fairly evenly, these studies almost exclusively applied anodal stimulation (103/115 effects). Bilateral stimulation also failed to be represented in this domain. Ultimately, none of the available contrasts suggested a reliable advantage or disadvantage to working memory performance, nor did examination of posterior predictive AMEs. Removal of highly-unbalanced factors also had no effect on the results, so we retain them here for transparency.
Turning to our model comparison set, the best performing model included a three-way interaction between Design Type × Stim Intensity × Stim Duration—but again, this failed to significantly beat the base model in terms of predictive performance (ELPDDiff = −3.10, SEDiff = 2.50, ELPDDiff / SEDiff = −1.24; Supplementary Table S9). Furthermore, this particular candidate model, while ostensibly offering the highest predictive utility, also demonstrated more degenerate behavior during sampling, consistently yielding several divergent Monte Carlo transitions regardless of numerous attempts at adjusting sampling behavior. While these make up an extremely small proportion of the total number of MCMC iterations run, it nevertheless suggests a more unwieldy posterior geometry, and this in turn can hamper the interpretability/reliability of parameter estimates. Here we see several potential effects that were not apparent in the base model, including a potential advantage for using larger electrode montages (b = 0.14, SD = 0.06, 95CI = [0.02, 0.27], pd = 0.989) and some evidence trending towards a two-way interaction between stimulation intensity and duration (b = −0.15, SD = 0.05, 95CI = [−0.25, −0.04], pd = 0.991). The full set of parameter estimates for this model can be found in Supplementary Table S10, although we urge some caution in their interpretation given the aforementioned challenges with convergence.
3.4 Vigilance
The effects of tDCS on performance in vigilance tasks were modeled over 15 studies reporting 93 effects (Figure 2D; Table 6). Similar to working memory, most studies here employed anodal stimulation designs (83 effects) focused on the DLPFC (51 effects). Additional brain regions were grouped into ‘Other PFC’ and ‘Temporal–Parietal’ categories. Most design parameters did not suggest any associations with behavioral outcomes; however, we observed a small positive relationship with the number of electrodes used in the montage (b = 0.08, SD = 0.04, 95CI = [0.01, 0.15], pd = 0.983). We note that this may have been driven by two studies using a more complex montage comprised of 10 electrodes (yielding 11 effects)—other studies employed between 2 and 5 electrodes. Thus, we caution against overinterpreting this particular effect. Further comparisons of posterior predictive densities and AMEs did not reveal additional associations beyond the estimated contrasts.
The base model further demonstrated the best performance relative to all candidate interaction models; however, it was not significantly better than any candidate model. The closest contender was again the model including a Design Type × Stim Intensity × Stim Duration interaction (ELPDDiff = −0.67, SEDiff = 1.48, ELPDDiff / SEDiff = −0.45; Supplementary Table S11)—although no individual main effects or interaction effects under this model yielded any nonzero effects (Supplementary Table S12). Thus, the inclusion of interaction terms does not inform differences under this domain any more than those scantly observed in the base model.
3.5 Inhibition
A survey of 84 effects across 15 inhibition studies did not reveal any meaningful associations between any stimulation or experiment parameters and behavior (Figure 2E; Table 7). Again, there was little variability in brain regions targeted for stimulation: 75/84 effects were localized to the DLPFC. The remaining effects spanned the inferior frontal junction and parietal cortex and were therefore collapsed into an ‘Other’ term during the original model fit. Removing this predictor from the model did not affect the results. There was also less variability in target laterality (no effects under bilateral stimulation) and the number of stimulation sessions (only one session reported per subject). Assessments of posterior predictive densities and AMEs did not reveal any other potential effects not captured in the base model. Although several parameters trended towards a nonzero effect, there is inconclusive evidence for any reliable differences in behavioral performance given variation in design parameters under this domain.
Here we again saw superior performance for the base model over candidate interaction models. This time, however, the base model consistently, significantly beat all three potential candidates—no exploratory model provided competitive predictive performance (Supplementary Table S13).
3.6 Combined model
Finally, we considered a domain-general model combining all 106 studies and 721 effects across all domains (Figure 2F; Table 8). Here we omitted the individualized target parameter (due to its scarce representation beyond motor performance tasks) as well as the regional target parameter to instead focus on broader trends related to stimulation and experiment design. Taken together, there was considerable heterogeneity across all relevant effects, with stimulation polarity and within- vs. between-subject designs providing inconclusive evidence for domain-general associations with behavior: cathodal stimulation tended to produce somewhat smaller behavioral effects on average (d = −0.19, 95CI = [−0.39, 0.02]; b = −0.07, SD = 0.04, 95CI = [−0.15, 0], pd = 0.971), while within-subjects designs produced larger effects (d = 0.32, 95CI = [0, 0.65]; b = 0.13, SD = 0.07, 95CI = [0, 0.26], pd = 0.973). Posterior predictive AMEs further suggested that online stimulation may yield smaller effects than offline stimulation (Median predictive difference = −0.14, 95CI = [−0.24, −0.04]).
As described previously, the competing set of three-way interaction models was originally defined by concatenating together the data from all domains. Thus, each candidate model significantly outperformed the base model (Supplementary Table S4) while remaining competitive with each other (Supplementary Table S14). The best-performing candidate included a strong three-way interaction between Design Type × Num Electrodes × Stim Intensity (b = −0.49, SD = 0.10, 95CI = [−0.69, −0.29], pd = 1), which suggested that the montages with more electrodes may produce larger behavioral effects as raw stimulation intensity increases under between-subjects designs—but this relationship is reversed in within-subjects designs, such that one might expect increasingly-diminishing returns with more complex montages and stronger stimulation intensities (Supplementary Figure S2; Supplementary Table S15). The second-best model included a three-way interaction for Design Type × Stim Intensity × Stim Duration (b = −0.11, SD = 0.03, 95CI = [−0.17, −0.06], pd = 1): here, we saw similar trends as in the previous model, such that longer and stronger applications of tDCS may confer an advantage in between-subjects studies, but this is again reversed for within-subjects designs (Supplementary Figure S3; Supplementary Table S16). Finally, the third candidate model provided evidence for a three-way interaction between Design Type × Num Electrodes × Stim Duration (b = 0.06, SD = 0.02, 95CI = [0.03, 0.09], pd = 1), where longer stimulation durations coupled with larger montages may provide a specific benefit to within-subject designs, whereas between-subject designs are relatively invariant to combined changes in these factors (Supplementary Figure S4; Supplementary Table S17).
4 Discussion
When designing a neurostimulation experiment, researchers are presented with a vast decision tree. For example, one must determine where in the brain to apply stimulation; to what intensity and for how long; whether to apply it online (as subjects are performing a task) or offline; and whether to apply ‘excitatory’ or ‘inhibitory’ stimulation. The net effect of neurostimulation ultimately depends on a complex constellation of these factors—and while some of these decisions have clear theoretical/empirical guides (e.g., targeting brain regions previously known to be associated with task performance), many others remain open to the subjective preferences of the researcher, and there is no clear consensus or standardization of the optimal approach. In this report, we sought to identify which of these stimulation and experimental design factors confer the strongest likelihood of behavioral effects in tDCS studies, using a hierarchical, Bayesian meta-regression approach across five cognitive domains.
We observed considerable heterogeneity in these associations, with little consensus between domains. When combining all studies and effects under a purely-additive, domain-general model, only two factors emerged with a modicum of consistency: (1) the polarity of stimulation, with cathodal vs. sham designs often producing reduced behavioral effects relative to anodal vs. sham designs (perhaps in line with the naïve notion that cathodal stimulation is primarily inhibitory), and (2) within-subject experimental designs conferring larger effects than between-subject designs (which may be unsurprising given that within-subject comparisons are generally considered to be more powerful—which is to say, for an identical assumed effect size a priori, within-subject manipulations require fewer subjects to reach the same level of power relative to between-subjects). These associations were modest at best when considering the full range of variance in reported effects; however, stronger associations with polarity and statistical design were seen across visual search and motor performance tasks, respectively.
The results of our exploratory interaction analyses are also somewhat difficult to interpret, given that no candidate model significantly generalized to individual cognitive domains—the improvements in predictive performance when including various three-way interaction terms were most appreciable when combining data across domains. However, there are several notable trends that may be worthy of consideration. For example, the use of between- vs. within-subject designs was consistently a relevant factor in each candidate model. In cases where this factor interacted with Stimulation Intensity × Num Electrodes (Supplementary Figure S2; Supplementary Table S15) and Stimulation Intensity × Stim Duration (Supplementary Figure S3; Supplementary Table S16), it appeared that the benefit of larger montages and longer stimulation durations (respectively) reversed for between- vs. within-subject designs—however, this switch primarily occurred out in the tails of the observed stimulation intensities (i.e., at least 3 mA or more). While such high intensities were seen in our review, they are not necessarily representative of most tDCS studies on the whole. At lower, more traditional stimulation intensities (e.g., ~1 mA), within-subjects designs generally still held an advantage over between-subjects. Furthermore, it is worth noting that factors such as stimulation intensity generally only informed differences in behavioral outcomes when included in an interaction—this seems reasonable given that the effectiveness of a given intensity may critically depend upon the duration of stimulation and/or the extent of an electrode montage. However, deeper interpretation of these results is made somewhat difficult given that raw intensity alone does not necessarily reflect the dosage of stimulation applied to the target (typically operationalized as current density), nor does the three-way interaction identified here. We attempted to model this factor in each domain (Supplementary Figure S1), but it was not reliably estimable for all studies (resulting in loss of data) and otherwise did not appear to be predictive of outcome variability. There are a number of potential reasons for this (e.g., individual differences in brain anatomy mediating differences in the effective current density at the target) which warrant future investigation, and it is similarly critical for other empirical work to report this information in their Methods sections to help aid in the interpretation of results. In sum, while it nevertheless remains unclear as to whether (or to what extent) specific stimulation or experimental parameters can reliably induce desired behavioral outcomes, these findings may provide a useful reference for researchers designing future behavioral studies in addition to more systematic investigations of how various tDCS parameters may interact to modulate behavior.
A growing number of published meta-analyses have attempted to quantify and characterize patterns of uncertainty and variability in neurostimulation-related effects on cognition and behavior. However, to date, many of these previous efforts have taken a more piecemeal approach, focused on specific tasks/domains, individual behavioral measures (e.g., accuracy or reaction time), or a limited range of stimulation parameters (e.g., particular target brain regions, or differentiating between anodal or cathodal stimulation alone) (Jacobson et al., 2012; Brunoni and Vanderhasselt, 2014; Dedoncker et al., 2016; Hill et al., 2016; Mancuso et al., 2016; Imburgio and Orr, 2018; Oldrati and Schutter, 2018; Schroeder et al., 2020). Our goal, by contrast, was to take a more holistic view capturing a broad range of design factors and behaviors. This presented a number of challenges, including uneven representation of some stimulation/experimental parameters across domains, making it difficult to robustly contrast certain effects. In a sense, these imbalances could be interpreted as a de facto consensus on which brain regions ‘should’ be targeted, or which type of stimulation to apply, etc., when probing behaviors under a given domain. Yet, our analyses demonstrate that there is still a profound degree of heterogeneity across these parameters, suggesting the need for deeper consideration when planning and designing tDCS studies.
4.1 Uncontrollable sources of variability
The design elements under investigation here are generally considered to be controllable sources of variability in neurostimulation studies (i.e., easily-manipulated by experimenters in a systematic fashion), but there nevertheless remain a host of uncontrollable sources of variability that may further hamper one’s ability to elicit behavioral effects and contribute to inconsistent results across studies. Critically among these are a number of neuroanatomical factors that likely influence the propagation of electrical current through the brain and the degree to which stimulation actually produces the presumed effects on neuronal activity. For example, neurons running parallel to an applied electric field generally appear to exhibit the hypothesized excitatory and inhibitory effects of anodal and cathodal stimulation (respectively), but neurons running orthogonally to the field may not (Rahman et al., 2013; Lafon et al., 2017). Inter-individual variability in the local orientation of neurons within a target brain region might therefore preclude effective neuromodulation during tDCS. Other variables such as head size, head fat content, skull thickness, and sulcal depth can also mediate the effectiveness of tDCS (Kessler et al., 2013; Truong et al., 2013; Opitz et al., 2015; Bikson et al., 2016), which together could manifest as further individual differences in behavior, washing out a mean difference between one stimulation/task condition and another.
The development of improved, neuroanatomically-informed current modeling techniques, particularly with respect to individual-specific optimization of electrode placements and stimulation dosage, may be able to mitigate some of these factors (Datta et al., 2012; Kim et al., 2014; Woods et al., 2016; Saturnino et al., 2019). Recent advances in electrical field modeling, including the Realistic Volumetric Approach to Simulate Transcranial Electrical Stimulation (ROAST) software (Huang et al., 2019), the dose-target determination index (DTDI) (Kashyap et al., 2021), and other novel approaches combining meta-analysis with simulation of electrical fields (Wischnewski et al., 2021) have highlighted that it is not sufficient to simply place an electrode montage over a given brain area and assume that it is receiving focal stimulation. The frequently-observed mixed findings across tDCS studies may in large part be due to variability and suboptimality in the actual strength/focality of electric fields over the desired target brain region (Wischnewski et al., 2021). We advocate strongly for the use of these field modeling techniques whenever possible as an additional control over stimulation targeting.
Finally, and more generally, there remain many other challenging sources of variability (e.g., baseline cognitive abilities, neurochemistry, circadian rhythms, blood pressure, genetic factors, etc.) (Li et al., 2015; Hsu et al., 2016; Rosen et al., 2016) which, while not necessarily impossible to control for, are frequently impractical or unrealistic for any given study to measure and factor into their analyses. Because these variables are often so difficult to quantify (and as such, are rarely reported), the sum total of their influence on the heterogenous nature of effects modeled here cannot be ascertained. Nevertheless, a more complete understanding of these issues is imperative as the field moves forward.
4.2 Limitations of this study
As aforementioned, we applied highly-restrictive inclusion criteria when collating data for this meta-analysis. While this was necessary to enable more straightforward tests of our key stimulation and experimental parameters, these findings may not be fully-representative of the literature on the whole (although the high degree of variability observed is in line with prior work). One critical omission of note spans studies using customized/individualized stimulation protocols beyond target placement alone: for example, paradigms which vary stimulation intensity on an individual basis to standardize the dosage (current density) applied to the target. Our models required stimulation intensity to be held constant for a given reported effect, but such individualized approaches are becoming increasingly more commonplace. Thus, while our results may reflect historical trends in stimulation outcomes (especially with respect to conventional designs), they may not adequately account for present trends using more sophisticated methods. Future meta-analytic work would benefit from more direct comparisons between conventional and contemporary approaches to stimulation designs.
As an additional limitation, we desired to identify more generalizable trends in how these design parameters relate to behavioral outcomes, both within and between domains—but collapsing across multiple task paradigms and performance metrics may actually obscure reliable effects that would otherwise be apparent in a more focal meta-analysis. Even when simplifying behavioral effects to ‘positive’ and ‘negative’ outcomes, it is unlikely that any given study tests/measures a particular psychological construct in the same way, which might amplify the inherent noise variance under the model.
Finally, while we attempted to explore potential interactions between various experiment design factors, this was still somewhat limited in scope—to limit complexity and reduce the putative model space, we considered at most the inclusion of a single three-way interaction term (along with its marginal two-way interactions) and performed this selection based on data combined across domains rather than within each domain. While on the one hand this allowed us to assess generalizability of the identified candidate models, it is also possible that each domain may produce different sets of candidate interactions. Furthermore, in order to be consistent with our base, additive models across all domains, we retained the same sets of weaky-informative priors: but in a Bayesian model comparison and variable selection scenario, sparsity-inducing priors (e.g., a regularized horseshoe) may be preferable. These are often extremely delicate in the context of hierarchical models, though, and may produce degenerate sampling behavior and difficulties with convergence. Future experimental work would greatly benefit from systematic investigations of these parameters and the potential ways in which they jointly-modulate behavioral performance outcomes, and this modeling framework could also potentially be combined with tDCS simulation methods (Wischnewski et al., 2021) to further inform experimental design principles for neurostimulation researchers.
5 Conclusion
In conclusion, our comprehensive meta-analysis across five cognitive domains reveals a complex and heterogenous landscape of associations between neurostimulation and behavioral outcomes. While some trends emerge, such as the advantage of within-subject designs and the influence of stimulation polarity, overall, there is little consensus on the optimal stimulation and experimental parameters for reliably inducing desired behavioral outcomes using tDCS. The field of neurostimulation research faces the challenge of contending with uncontrollable sources of variability, including various neuroanatomical and individual difference factors, which may further contribute to the inconsistency observed across studies. This study underscores the need for deeper consideration and standardization of experimental design in tDCS studies and highlights the importance of developing neuroanatomically-informed current modeling techniques to better understand and control for sources of variability. Future research should continue to investigate the complex interplay of these parameters and their potential interactions to advance our understanding of the effects of tDCS on cognitive and behavioral performance.
Data availability statement
The raw data supporting the conclusions of this article will be made available by the authors, without undue reservation.
Author contributions
TS: Conceptualization, Data curation, Formal analysis, Methodology, Writing – original draft, Writing – review & editing. SL: Conceptualization, Data curation, Writing – review & editing. LL: Conceptualization, Data curation, Writing – review & editing. HS: Data curation, Writing – review & editing. JS: Data curation, Writing – review & editing. PS: Data curation, Writing – review & editing. KD: Data curation, Writing – review & editing. MM: Conceptualization, Funding acquisition, Project administration, Supervision, Writing – review & editing. TB: Conceptualization, Funding acquisition, Project administration, Supervision, Writing – original draft, Writing – review & editing.
Funding
The author(s) declare financial support was received for the research, authorship, and/or publication of this article. This research was sponsored by the U.S. Army DEVCOM Soldier Center under contract #W911NF-D-19-0001 to the Institute for Collaborative Biotechnologies at the University of California, Santa Barbara. The opinions expressed herein are those of the authors and do not reflect those of the United States government. The U.S. government is authorized to reproduce and distribute reprints for Government purposes notwithstanding any copyright notation herein.
Conflict of interest
The authors declare that the research was conducted in the absence of any commercial or financial relationships that could be construed as a potential conflict of interest.
The author(s) declared that they were an editorial board member of Frontiers, at the time of submission. This had no impact on the peer review process and the final decision.
Publisher’s note
All claims expressed in this article are solely those of the authors and do not necessarily represent those of their affiliated organizations, or those of the publisher, the editors and the reviewers. Any product that may be evaluated in this article, or claim that may be made by its manufacturer, is not guaranteed or endorsed by the publisher.
Supplementary material
The Supplementary material for this article can be found online at: https://www.frontiersin.org/articles/10.3389/fnhum.2024.1305446/full#supplementary-material
Footnotes
References
Abellaneda-Perez, K., Vaque-Alcazar, L., Perellon-Alfonso, R., Bargallo, N., Kuo, M. F., Pascual-Leone, A., et al. (2019). Differential tDCS and tACS effects on working memory-related neural activity and resting-state connectivity. Front. Neurosci. 13:1440. doi: 10.3389/fnins.2019.01440
Ambrus, G. G., Chaieb, L., Stilling, R., Rothkegel, H., Antal, A., and Paulus, W. (2016). Monitoring transcranial direct current stimulation induced changes in cortical excitability during the serial reaction time task. Neurosci. Lett. 616, 98–104. doi: 10.1016/j.neulet.2016.01.039
Anderson, E. J., Mannan, S. K., Husain, M., Rees, G., Sumner, P., Mort, D. J., et al. (2007). Involvement of prefrontal cortex in visual search. Exp. Brain Res. 180, 289–302. doi: 10.1007/s00221-007-0860-0
Angius, L., Santarnecchi, E., Pascual-Leone, A., and Marcora, S. M. (2019). Transcranial direct current stimulation over the left dorsolateral prefrontal cortex improves inhibitory control and endurance performance in healthy individuals. Neuroscience 419, 34–45. doi: 10.1016/j.neuroscience.2019.08.052
Ankri, Y. L. E., Braw, Y., Luboshits, G., and Meiron, O. (2020). The effects of stress and transcranial direct current stimulation (tDCS) on working memory: a randomized controlled trial. Cogn. Affect. Behav. Neurosci. 20, 103–114. doi: 10.3758/s13415-019-00755-7
Antal, A., Nitsche, M. A., Kincses, T. Z., Kruse, W., Hoffmann, K. P., and Paulus, W. (2004). Facilitation of visuo-motor learning by transcranial direct current stimulation of the motor and extrastriate visual areas in humans. Eur. J. Neurosci. 19, 2888–2892. doi: 10.1111/j.1460-9568.2004.03367.x
Appelhans, B. M. (2009). Neurobehavioral inhibition of reward-driven feeding: implications for dieting and obesity. Obesity (Silver Spring) 17, 640–647. doi: 10.1038/oby.2008.638
Apsvalka, D., Ramsey, R., and Cross, E. S. (2018). Anodal tDCS over primary motor cortex provides no advantage to learning motor sequences via observation. Neural Plast. 2018, 1–14. doi: 10.1155/2018/1237962
Aron, A. R., Robbins, T. W., and Poldrack, R. A. (2004). Inhibition and the right inferior frontal cortex. Trends Cogn. Sci. 8, 170–177. doi: 10.1016/j.tics.2004.02.010
Aron, A. R., Robbins, T. W., and Poldrack, R. A. (2014). Inhibition and the right inferior frontal cortex: one decade on. Trends Cogn. Sci. 18, 177–185. doi: 10.1016/j.tics.2013.12.003
Assecondi, S., Hu, R., Eskes, G., Pan, X., Zhou, J., and Shapiro, K. (2021). Impact of tDCS on working memory training is enhanced by strategy instructions in individuals with low working memory capacity. Sci. Rep. 11:5531. doi: 10.1038/s41598-021-84298-3
Au, J., Katz, B., Buschkuehl, M., Bunarjo, K., Senger, T., Zabel, C., et al. (2016). Enhancing working memory training with transcranial direct current stimulation. J. Cogn. Neurosci. 28, 1419–1432. doi: 10.1162/jocn_a_00979
Axelrod, V., Rees, G., Lavidor, M., and Bar, M. (2015). Increasing propensity to mind-wander with transcranial direct current stimulation. Proc. Natl. Acad. Sci. USA 112, 3314–3319. doi: 10.1073/pnas.1421435112
Ball, K., Lane, A. R., Smith, D. T., and Ellison, A. (2013). Site-dependent effects of tDCS uncover dissociations in the communication network underlying the processing of visual search. Brain Stimul. 6, 959–965. doi: 10.1016/j.brs.2013.06.001
Ballard, H. K., Eakin, S. M., Maldonado, T., and Bernard, J. A. (2021). Using high-definition transcranial direct current stimulation to investigate the role of the dorsolateral prefrontal cortex in explicit sequence learning. PLoS One 16:e0246849. doi: 10.1371/journal.pone.0246849
Ballard, H. K., Goen, J. R. M., Maldonado, T., and Bernard, J. A. (2019). Effects of cerebellar transcranial direct current stimulation on the cognitive stage of sequence learning. J. Neurophysiol. 122, 490–499. doi: 10.1152/jn.00036.2019
Batsikadze, G., Moliadze, V., Paulus, W., Kuo, M. F., and Nitsche, M. A. (2013). Partially non-linear stimulation intensity-dependent effects of direct current stimulation on motor cortex excitability in humans. J. Physiol. 591, 1987–2000. doi: 10.1113/jphysiol.2012.249730
Baumert, A., Buchholz, N., Zinkernagel, A., Clarke, P., MacLeod, C., Osinsky, R., et al. (2020). Causal underpinnings of working memory and Stroop interference control: testing the effects of anodal and cathodal tDCS over the left DLPFC. Cogn. Affect. Behav. Neurosci. 20, 34–48. doi: 10.3758/s13415-019-00726-y
Bestmann, S., de Berker, A. O., and Bonaiuto, J. (2015). Understanding the behavioural consequences of noninvasive brain stimulation. Trends Cogn. Sci. 19, 13–20. doi: 10.1016/j.tics.2014.10.003
Bestmann, S., and Walsh, V. (2017). Transcranial electrical stimulation. Curr. Biol. 27, R1258–R1262. doi: 10.1016/j.cub.2017.11.001
Biggs, A. T., Cain, M. S., and Mitroff, S. R. (2015). Cognitive training can reduce civilian casualties in a simulated shooting environment. Psychol. Sci. 26, 1164–1176. doi: 10.1177/0956797615579274
Bikson, M., Datta, A., Rahman, A., and Scaturro, J. (2010). Electrode montages for tDCS and weak transcranial electrical stimulation: role of "return" electrode's position and size. Clin. Neurophysiol. 121, 1976–1978. doi: 10.1016/j.clinph.2010.05.020
Bikson, M., Grossman, P., Thomas, C., Zannou, A. L., Jiang, J., Adnan, T., et al. (2016). Safety of transcranial direct current stimulation: evidence based update 2016. Brain Stimul. 9, 641–661. doi: 10.1016/j.brs.2016.06.004
Bikson, M., Name, A., and Rahman, A. (2013). Origins of specificity during tDCS: anatomical, activity-selective, and input-bias mechanisms. Front. Hum. Neurosci. 7:688. doi: 10.3389/fnhum.2013.00688
Bikson, M., Paulus, W., Esmaeilpour, Z., Kronberg, G., and Nitsche, M. A. (2019). “Mechanisms of acute and after effects of transcranial direct current stimulation” in Practical guide to transcranial direct current stimulation. eds. H. Knotkova, M. Nitsche, M. Bikson, and A. Woods (Cham, Switzerland: Springer), 81–113.
Bikson, M., Rahman, A., and Datta, A. (2012). Computational models of transcranial direct current stimulation. Clin. EEG Neurosci. 43, 176–183. doi: 10.1177/1550059412445138
Boehringer, A., Macher, K., Dukart, J., Villringer, A., and Pleger, B. (2013). Cerebellar transcranial direct current stimulation modulates verbal working memory. Brain Stimul. 6, 649–653. doi: 10.1016/j.brs.2012.10.001
Boggio, P. S., Castro, L. O., Savagim, E. A., Braite, R., Cruz, V. C., Rocha, R. R., et al. (2006). Enhancement of non-dominant hand motor function by anodal transcranial direct current stimulation. Neurosci. Lett. 404, 232–236. doi: 10.1016/j.neulet.2006.05.051
Boggio, P. S., Nunes, A., Rigonatti, S. P., Nitsche, M. A., Pascual-Leone, A., and Fregni, F. (2007). Repeated sessions of noninvasive brain DC stimulation is associated with motor function improvement in stroke patients. Restor. Neurol. Neurosci. 25, 123–129.
Bolognini, N., Fregni, F., Casati, C., Olgiati, E., and Vallar, G. (2010). Brain polarization of parietal cortex augments training-induced improvement of visual exploratory and attentional skills. Brain Res. 1349, 76–89. doi: 10.1016/j.brainres.2010.06.053
Bonaiuto, J. J., and Bestmann, S. (2015). Understanding the nonlinear physiological and behavioral effects of tDCS through computational neurostimulation. Prog. Brain Res. 222, 75–103. doi: 10.1016/bs.pbr.2015.06.013
Borragan, G., Gilson, M., Guerrero-Mosquera, C., Di Ricci, E., Slama, H., and Peigneux, P. (2018). Transcranial direct current stimulation does not counteract cognitive fatigue, but induces sleepiness and an inter-hemispheric shift in brain oxygenation. Front. Psychol. 9:2351. doi: 10.3389/fpsyg.2018.02351
Boudewyn, M., Roberts, B. M., Mizrak, E., Ranganath, C., and Carter, C. S. (2019). Prefrontal transcranial direct current stimulation (tDCS) enhances behavioral and EEG markers of proactive control. Cogn. Neurosci. 10, 57–65. doi: 10.1080/17588928.2018.1551869
Brunnauer, A., Segmiller, F. M., Loschner, S., Grun, V., Padberg, F., and Palm, U. (2018). The effects of transcranial direct current stimulation (tDCS) on psychomotor and visual perception functions related to driving skills. Front. Behav. Neurosci. 12:16. doi: 10.3389/fnbeh.2018.00016
Brunoni, A. R., and Vanderhasselt, M. A. (2014). Working memory improvement with non-invasive brain stimulation of the dorsolateral prefrontal cortex: a systematic review and meta-analysis. Brain Cogn. 86, 1–9. doi: 10.1016/j.bandc.2014.01.008
Bürkner, P.-C. (2017). Brms: an R package for Bayesian multilevel models using Stan. J. Stat. Softw. 80, 1–28. doi: 10.18637/jss.v080.i01
Callan, D. E., Falcone, B., Wada, A., and Parasuraman, R. (2016). Simultaneous tDCS-fMRI identifies resting state networks correlated with visual search enhancement. Front. Hum. Neurosci. 10:72. doi: 10.3389/fnhum.2016.00072
Cantarero, G., Spampinato, D., Reis, J., Ajagbe, L., Thompson, T., Kulkarni, K., et al. (2015). Cerebellar direct current stimulation enhances on-line motor skill acquisition through an effect on accuracy. J. Neurosci. 35, 3285–3290. doi: 10.1523/JNEUROSCI.2885-14.2015
Caulfield, K. A., Indahlastari, A., Nissim, N. R., Lopez, J. W., Fleischmann, H. H., Woods, A. J., et al. (2022). Electric field strength from prefrontal transcranial direct current stimulation determines degree of working memory response: a potential application of reverse-calculation modeling? Neuromodulation 25, 578–587. doi: 10.1111/ner.13342
Chambers, C. D., Garavan, H., and Bellgrove, M. A. (2009). Insights into the neural basis of response inhibition from cognitive and clinical neuroscience. Neurosci. Biobehav. Rev. 33, 631–646. doi: 10.1016/j.neubiorev.2008.08.016
Chan, L. K. H., and Hayward, W. G. (2013). Visual search. Wiley Interdiscip. Rev. Cogn. Sci. 4, 415–429. doi: 10.1002/wcs.1235
Chew, T., Ho, K. A., and Loo, C. K. (2015). Inter- and intra-individual variability in response to transcranial direct current stimulation (tDCS) at varying current intensities. Brain Stimul. 8, 1130–1137. doi: 10.1016/j.brs.2015.07.031
Chrysikou, E. G., Gorey, C., and Aupperle, R. L. (2017). Anodal transcranial direct current stimulation over right dorsolateral prefrontal cortex alters decision making during approach-avoidance conflict. Soc. Cogn. Affect. Neurosci. 12, 468–475. doi: 10.1093/scan/nsw140
Clark, V. P., Coffman, B. A., Mayer, A. R., Weisend, M. P., Lane, T. D., Calhoun, V. D., et al. (2012). TDCS guided using fMRI significantly accelerates learning to identify concealed objects. NeuroImage 59, 117–128. doi: 10.1016/j.neuroimage.2010.11.036
Coffman, B. A., Trumbo, M. C., Flores, R. A., Garcia, C. M., van der Merwe, A. J., Wassermann, E. M., et al. (2012). Impact of tDCS on performance and learning of target detection: interaction with stimulus characteristics and experimental design. Neuropsychologia 50, 1594–1602. doi: 10.1016/j.neuropsychologia.2012.03.012
Congdon, E., Mumford, J. A., Cohen, J. R., Galvan, A., Canli, T., and Poldrack, R. A. (2012). Measurement and reliability of response inhibition. Front. Psychol. 3:37. doi: 10.3389/fpsyg.2012.00037
Cosman, J. D., Atreya, P. V., and Woodman, G. F. (2015). Transient reduction of visual distraction following electrical stimulation of the prefrontal cortex. Cognition 145, 73–76. doi: 10.1016/j.cognition.2015.08.010
Coulborn, S., Bowman, H., Miall, R. C., and Fernandez-Espejo, D. (2020). Effect of tDCS over the right inferior parietal lobule on mind-wandering propensity. Front. Hum. Neurosci. 14:230. doi: 10.3389/fnhum.2020.00230
DaSilva, A. F., Volz, M. S., Bikson, M., and Fregni, F. (2011). Electrode positioning and montage in transcranial direct current stimulation. J. Vis. Exp. 51:e2744. doi: 10.3791/2744-v
Datta, A., Bansal, V., Diaz, J., Patel, J., Reato, D., and Bikson, M. (2009). Gyri-precise head model of transcranial direct current stimulation: improved spatial focality using a ring electrode versus conventional rectangular pad. Brain Stimul. 2, 201–207, 207.e1. doi: 10.1016/j.brs.2009.03.005
Datta, A., Truong, D., Minhas, P., Parra, L. C., and Bikson, M. (2012). Inter-individual variation during transcranial direct current stimulation and normalization of dose using MRI-derived computational models. Front. Psych. 3:91. doi: 10.3389/fpsyt.2012.00091
Davidson, J. E., and Sternberg, R. J. (2003). The psychology of problem solving. New York, NY, US: Cambridge University Press.
Dayan, E., Censor, N., Buch, E. R., Sandrini, M., and Cohen, L. G. (2013). Noninvasive brain stimulation: from physiology to network dynamics and back. Nat. Neurosci. 16, 838–844. doi: 10.1038/nn.3422
de Berker, A. O., Bikson, M., and Bestmann, S. (2013). Predicting the behavioral impact of transcranial direct current stimulation: issues and limitations. Front. Hum. Neurosci. 7:613. doi: 10.3389/fnhum.2013.00613
Dedoncker, J., Brunoni, A. R., Baeken, C., and Vanderhasselt, M. A. (2016). A systematic review and Meta-analysis of the effects of transcranial direct current stimulation (tDCS) over the dorsolateral prefrontal cortex in healthy and neuropsychiatric samples: influence of stimulation parameters. Brain Stimul. 9, 501–517. doi: 10.1016/j.brs.2016.04.006
Denis, G., Zory, R., and Radel, R. (2019). Testing the role of cognitive inhibition in physical endurance using high-definition transcranial direct current stimulation over the prefrontal cortex. Hum. Mov. Sci. 67:102507. doi: 10.1016/j.humov.2019.102507
D'Esposito, M. (2007). From cognitive to neural models of working memory. Philos. Trans. R. Soc. Lond. Ser. B Biol. Sci. 362, 761–772. doi: 10.1098/rstb.2007.2086
Donner, T., Kettermann, A., Diesch, E., Ostendorf, F., Villringer, A., and Brandt, S. A. (2000). Involvement of the human frontal eye field and multiple parietal areas in covert visual selection during conjunction search. Eur. J. Neurosci. 12, 3407–3414. doi: 10.1046/j.1460-9568.2000.00223.x
Doppelmayr, M., Pixa, N. H., and Steinberg, F. (2016). Cerebellar, but not motor or parietal, high-density anodal transcranial direct current stimulation facilitates motor adaptation. J. Int. Neuropsychol. Soc. 22, 928–936. doi: 10.1017/S1355617716000345
Dubreuil-Vall, L., Chau, P., Ruffini, G., Widge, A. S., and Camprodon, J. A. (2019). tDCS to the left DLPFC modulates cognitive and physiological correlates of executive function in a state-dependent manner. Brain Stimul. 12, 1456–1463. doi: 10.1016/j.brs.2019.06.006
Dumel, G., Bourassa, M. E., Charlebois-Plante, C., Desjardins, M., Doyon, J., Saint-Amour, D., et al. (2018a). Multisession anodal transcranial direct current stimulation induces motor cortex plasticity enhancement and motor learning generalization in an aging population. Clin. Neurophysiol. 129, 494–502. doi: 10.1016/j.clinph.2017.10.041
Dumel, G., Bourassa, M. E., Charlebois-Plante, C., Desjardins, M., Doyon, J., Saint-Amour, D., et al. (2018b). Motor learning improvement remains 3 months after a multisession anodal tDCS intervention in an aging population. Front. Aging Neurosci. 10:335. doi: 10.3389/fnagi.2018.00335
Duncan, J., and Owen, A. M. (2000). Common regions of the human frontal lobe recruited by diverse cognitive demands. Trends Neurosci. 23, 475–483. doi: 10.1016/S0166-2236(00)01633-7
Edgcumbe, D. R., Thoma, V., Rivolta, D., Nitsche, M. A., and Fu, C. H. Y. (2019). Anodal transcranial direct current stimulation over the right dorsolateral prefrontal cortex enhances reflective judgment and decision-making. Brain Stimul. 12, 652–658. doi: 10.1016/j.brs.2018.12.003
Ehsani, F., Bakhtiary, A. H., Jaberzadeh, S., Talimkhani, A., and Hajihasani, A. (2016). Differential effects of primary motor cortex and cerebellar transcranial direct current stimulation on motor learning in healthy individuals: a randomized double-blind sham-controlled study. Neurosci. Res. 112, 10–19. doi: 10.1016/j.neures.2016.06.003
Ellison, A., Ball, K. L., and Lane, A. R. (2017). The behavioral effects of tDCS on visual search performance are not influenced by the location of the reference electrode. Front. Neurosci. 11:520. doi: 10.3389/fnins.2017.00520
Ellison, A., Ball, K. L., Moseley, P., Dowsett, J., Smith, D. T., Weis, S., et al. (2014). Functional interaction between right parietal and bilateral frontal cortices during visual search tasks revealed using functional magnetic imaging and transcranial direct current stimulation. PLoS One 9:e93767. doi: 10.1371/journal.pone.0093767
Esmaeilpour, Z., Marangolo, P., Hampstead, B. M., Bestmann, S., Galletta, E., Knotkova, H., et al. (2018). Incomplete evidence that increasing current intensity of tDCS boosts outcomes. Brain Stimul. 11, 310–321. doi: 10.1016/j.brs.2017.12.002
Falcone, M., Bernardo, L., Wileyto, E. P., Allenby, C., Burke, A. M., Hamilton, R., et al. (2019). Lack of effect of transcranial direct current stimulation (tDCS) on short-term smoking cessation: results of a randomized, sham-controlled clinical trial. Drug Alcohol Depend. 194, 244–251. doi: 10.1016/j.drugalcdep.2018.10.016
Falcone, B., Coffman, B. A., Clark, V. P., and Parasuraman, R. (2012). Transcranial direct current stimulation augments perceptual sensitivity and 24-hour retention in a complex threat detection task. PLoS One 7:e34993. doi: 10.1371/journal.pone.0034993
Falcone, B., Wada, A., Parasuraman, R., and Callan, D. E. (2018). Individual differences in learning correlate with modulation of brain activity induced by transcranial direct current stimulation. PLoS One 13:e0197192. doi: 10.1371/journal.pone.0197192
Fan, J., Voisin, J., Milot, M. H., Higgins, J., and Boudrias, M. H. (2017). Transcranial direct current stimulation over multiple days enhances motor performance of a grip task. Ann. Phys. Rehabil. Med. 60, 329–333. doi: 10.1016/j.rehab.2017.07.001
Fehring, D. J., Illipparampil, R., Acevedo, N., Jaberzadeh, S., Fitzgerald, P. B., and Mansouri, F. A. (2019). Interaction of task-related learning and transcranial direct current stimulation of the prefrontal cortex in modulating executive functions. Neuropsychologia 131, 148–159. doi: 10.1016/j.neuropsychologia.2019.05.011
Ferrucci, R., Brunoni, A. R., Parazzini, M., Vergari, M., Rossi, E., Fumagalli, M., et al. (2013). Modulating human procedural learning by cerebellar transcranial direct current stimulation. Cerebellum 12, 485–492. doi: 10.1007/s12311-012-0436-9
Filmer, H. L., Ehrhardt, S. E., Bollmann, S., Mattingley, J. B., and Dux, P. E. (2019). Accounting for individual differences in the response to tDCS with baseline levels of neurochemical excitability. Cortex 115, 324–334. doi: 10.1016/j.cortex.2019.02.012
Filmer, H. L., Lyons, M., Mattingley, J. B., and Dux, P. E. (2017a). Anodal tDCS applied during multitasking training leads to transferable performance gains. Sci. Rep. 7:12988. doi: 10.1038/s41598-017-13075-y
Filmer, H. L., Varghese, E., Hawkins, G. E., Mattingley, J. B., and Dux, P. E. (2017b). Improvements in attention and decision-making following combined behavioral training and brain stimulation. Cereb. Cortex 27, 3675–3682. doi: 10.1093/cercor/bhw189
Focke, J., Kemmet, S., Krause, V., Keitel, A., and Pollok, B. (2017). Cathodal transcranial direct current stimulation (tDCS) applied to the left premotor cortex (PMC) stabilizes a newly learned motor sequence. Behav. Brain Res. 316, 87–93. doi: 10.1016/j.bbr.2016.08.032
Fregni, F., Boggio, P. S., Lima, M. C., Ferreira, M. J., Wagner, T., Rigonatti, S. P., et al. (2006). A sham-controlled, phase II trial of transcranial direct current stimulation for the treatment of central pain in traumatic spinal cord injury. Pain 122, 197–209. doi: 10.1016/j.pain.2006.02.023
Friedrich, J., and Beste, C. (2018). Paradoxical, causal effects of sensory gain modulation on motor inhibitory control – a tDCS, EEG-source localization study. Sci. Rep. 8:17486. doi: 10.1038/s41598-018-35879-2
Friehs, M. A., and Frings, C. (2019). Offline beats online: transcranial direct current stimulation timing influences on working memory. Neuroreport 30, 795–799. doi: 10.1097/WNR.0000000000001272
Fujiyama, H., Hinder, M. R., Barzideh, A., Van de Vijver, C., Badache, A. C., Manrique, C. M., et al. (2017). Preconditioning tDCS facilitates subsequent tDCS effect on skill acquisition in older adults. Neurobiol. Aging 51, 31–42. doi: 10.1016/j.neurobiolaging.2016.11.012
Furuya, S., Klaus, M., Nitsche, M. A., Paulus, W., and Altenmuller, E. (2014). Ceiling effects prevent further improvement of transcranial stimulation in skilled musicians. J. Neurosci. 34, 13834–13839. doi: 10.1523/JNEUROSCI.1170-14.2014
Gandiga, P. C., Hummel, F. C., and Cohen, L. G. (2006). Transcranial DC stimulation (tDCS): a tool for double-blind sham-controlled clinical studies in brain stimulation. Clin. Neurophysiol. 117, 845–850. doi: 10.1016/j.clinph.2005.12.003
Gelman, A., Goodrich, B., Gabry, J., and Vehtari, A. (2019). R-squared for Bayesian regression models. Am. Stat. 73, 307–309. doi: 10.1080/00031305.2018.1549100
Gilbert, P. F., and Thach, W. T. (1977). Purkinje cell activity during motor learning. Brain Res. 128, 309–328. doi: 10.1016/0006-8993(77)90997-0
Gilhooly, K. J. (2004). “Working memory and planning” in The cognitive psychology of planning. eds. R. Morris and G. Ward (London: Routledge), 71–88.
Gladwin, T. E., den Uyl, T. E., Fregni, F. F., and Wiers, R. W. (2012). Enhancement of selective attention by tDCS: interaction with interference in a Sternberg task. Neurosci. Lett. 512, 33–37. doi: 10.1016/j.neulet.2012.01.056
Gomez-Ariza, C. J., Martin, M. C., and Morales, J. (2017). Tempering proactive cognitive control by transcranial direct current stimulation of the right (but not the left) lateral prefrontal cortex. Front. Neurosci. 11:282. doi: 10.3389/fnins.2017.00282
Grasso, P. A., Tonolli, E., and Miniussi, C. (2020). Effects of different transcranial direct current stimulation protocols on visuo-spatial contextual learning formation: evidence of homeostatic regulatory mechanisms. Sci. Rep. 10:4622. doi: 10.1038/s41598-020-61626-7
Grier, R. A., Warm, J. S., Dember, W. N., Matthews, G., Galinsky, T. L., and Parasuraman, R. (2003). The vigilance decrement reflects limitations in effortful attention, not mindlessness. Hum. Factors 45, 349–359. doi: 10.1518/hfes.45.3.349.27253
Hammer, A., Mohammadi, B., Schmicker, M., Saliger, S., and Munte, T. F. (2011). Errorless and errorful learning modulated by transcranial direct current stimulation. BMC Neurosci. 12:72. doi: 10.1186/1471-2202-12-72
Hampshire, A., and Sharp, D. J. (2015). Contrasting network and modular perspectives on inhibitory control. Trends Cogn. Sci. 19, 445–452. doi: 10.1016/j.tics.2015.06.006
Hancock, P. A. (2017). On the nature of vigilance. Hum. Factors 59, 35–43. doi: 10.1177/0018720816655240
Hanken, K., Bosse, M., Mohrke, K., Eling, P., Kastrup, A., Antal, A., et al. (2016). Counteracting fatigue in multiple sclerosis with right parietal anodal transcranial direct current stimulation. Front. Neurol. 7:154. doi: 10.3389/fneur.2016.00154
Hashemirad, F., Fitzgerald, P. B., Zoghi, M., and Jaberzadeh, S. (2017). Single-session anodal tDCS with small-size stimulating electrodes over Frontoparietal superficial sites does not affect motor sequence learning. Front. Hum. Neurosci. 11:153. doi: 10.3389/fnhum.2017.00153
Heroux, M. E., Loo, C. K., Taylor, J. L., and Gandevia, S. C. (2017). Questionable science and reproducibility in electrical brain stimulation research. PLoS One 12:e0175635. doi: 10.1371/journal.pone.0175635
Hill, A. T., Fitzgerald, P. B., and Hoy, K. E. (2016). Effects of anodal transcranial direct current stimulation on working memory: a systematic review and Meta-analysis of findings from healthy and neuropsychiatric populations. Brain Stimul. 9, 197–208. doi: 10.1016/j.brs.2015.10.006
Hinson, J. M., Jameson, T. L., and Whitney, P. (2003). Impulsive decision making and working memory. J. Exp. Psychol. Learn. Mem. Cogn. 29, 298–306. doi: 10.1037/0278-7393.29.2.298
Horn, N. R., Dolan, M., Elliott, R., Deakin, J. F., and Woodruff, P. W. (2003). Response inhibition and impulsivity: an fMRI study. Neuropsychologia 41, 1959–1966. doi: 10.1016/S0028-3932(03)00077-0
Horvath, J. C., Carter, O., and Forte, J. D. (2014). Transcranial direct current stimulation: five important issues we aren't discussing (but probably should be). Front. Syst. Neurosci. 8:2. doi: 10.3389/fnsys.2014.00002
Horvath, J. C., Carter, O., and Forte, J. D. (2016a). No significant effect of transcranial direct current stimulation (tDCS) found on simple motor reaction time comparing 15 different simulation protocols. Neuropsychologia 91, 544–552. doi: 10.1016/j.neuropsychologia.2016.09.017
Horvath, J. C., Forte, J. D., and Carter, O. (2015a). Quantitative review finds no evidence of cognitive effects in healthy populations from single-session transcranial direct current stimulation (tDCS). Brain Stimul. 8, 535–550. doi: 10.1016/j.brs.2015.01.400
Horvath, J. C., Forte, J. D., and Carter, O. (2015b). Evidence that transcranial direct current stimulation (tDCS) generates little-to-no reliable neurophysiologic effect beyond MEP amplitude modulation in healthy human subjects: a systematic review. Neuropsychologia 66, 213–236. doi: 10.1016/j.neuropsychologia.2014.11.021
Horvath, J. C., Vogrin, S. J., Carter, O., Cook, M. J., and Forte, J. D. (2016b). Effects of a common transcranial direct current stimulation (tDCS) protocol on motor evoked potentials found to be highly variable within individuals over 9 testing sessions. Exp. Brain Res. 234, 2629–2642. doi: 10.1007/s00221-016-4667-8
Houk, J. C., Buckingham, J. T., and Barto, A. G. (1996). Models of the cerebellum and motor learning. Behav. Brain Sci. 19, 368–383. doi: 10.1017/S0140525X00081474
Hsu, T. Y., Juan, C. H., and Tseng, P. (2016). Individual differences and state-dependent responses in transcranial direct current stimulation. Front. Hum. Neurosci. 10:643. doi: 10.3389/fnhum.2016.00643
Huang, Y., Datta, A., Bikson, M., and Parra, L. C. (2019). Realistic volumetric-approach to simulate transcranial electric stimulation-ROAST-a fully automated open-source pipeline. J. Neural Eng. 16:056006. doi: 10.1088/1741-2552/ab208d
Hussey, E. K., Fontes, E. B., Ward, N., Westfall, D. R., Kao, S. C., Kramer, A. F., et al. (2020). Combined and isolated effects of acute exercise and brain stimulation on executive function in healthy young adults. J. Clin. Med. 9:1410. doi: 10.3390/jcm9051410
Imburgio, M. J., and Orr, J. M. (2018). Effects of prefrontal tDCS on executive function: methodological considerations revealed by meta-analysis. Neuropsychologia 117, 156–166. doi: 10.1016/j.neuropsychologia.2018.04.022
Ironside, M., O'Shea, J., Cowen, P. J., and Harmer, C. J. (2016). Frontal cortex stimulation reduces vigilance to threat: implications for the treatment of depression and anxiety. Biol. Psychiatry 79, 823–830. doi: 10.1016/j.biopsych.2015.06.012
Jackson, M. P., Rahman, A., Lafon, B., Kronberg, G., Ling, D., Parra, L. C., et al. (2016). Animal models of transcranial direct current stimulation: methods and mechanisms. Clin. Neurophysiol. 127, 3425–3454. doi: 10.1016/j.clinph.2016.08.016
Jacobson, L., Koslowsky, M., and Lavidor, M. (2012). tDCS polarity effects in motor and cognitive domains: a meta-analytical review. Exp. Brain Res. 216, 1–10. doi: 10.1007/s00221-011-2891-9
Jacoby, N., and Lavidor, M. (2018). Null tDCS effects in a sustained attention task: the modulating role of learning. Front. Psychol. 9:476. doi: 10.3389/fpsyg.2018.00476
Jin, Y., Lee, J., Kim, S., and Yoon, B. (2019). Noninvasive brain stimulation over M1 and DLPFC cortex enhances the learning of bimanual isometric force control. Hum. Mov. Sci. 66, 73–83. doi: 10.1016/j.humov.2019.03.002
Karni, A., Meyer, G., Jezzard, P., Adams, M. M., Turner, R., and Ungerleider, L. G. (1995). Functional MRI evidence for adult motor cortex plasticity during motor skill learning. Nature 377, 155–158. doi: 10.1038/377155a0
Karni, A., Meyer, G., Rey-Hipolito, C., Jezzard, P., Adams, M. M., Turner, R., et al. (1998). The acquisition of skilled motor performance: fast and slow experience-driven changes in primary motor cortex. Proc. Natl. Acad. Sci. USA 95, 861–868. doi: 10.1073/pnas.95.3.861
Karthikeyan, R., Smoot, M. R., and Mehta, R. K. (2021). Anodal tDCS augments and preserves working memory beyond time-on-task deficits. Sci. Rep. 11:19134. doi: 10.1038/s41598-021-98636-y
Kashyap, R., Bhattacharjee, S., Arumugam, R., Bharath, R. D., Udupa, K., Oishi, K., et al. (2021). Focality-oriented selection of current dose for transcranial direct current stimulation. J. Pers. Med. 11:940. doi: 10.3390/jpm11090940
Kessler, S. K., Minhas, P., Woods, A. J., Rosen, A., Gorman, C., and Bikson, M. (2013). Dosage considerations for transcranial direct current stimulation in children: a computational modeling study. PLoS One 8:e76112. doi: 10.1371/journal.pone.0076112
Kim, J. H., Kim, D. W., Chang, W. H., Kim, Y. H., Kim, K., and Im, C. H. (2014). Inconsistent outcomes of transcranial direct current stimulation may originate from anatomical differences among individuals: electric field simulation using individual MRI data. Neurosci. Lett. 564, 6–10. doi: 10.1016/j.neulet.2014.01.054
Kirk, R. (2013). Experimental design: procedures for the behavioral sciences. Thousand Oaks, California Available at: https://methods.sagepub.com/book/experimental-design.
Kitazawa, S., Kimura, T., and Yin, P. B. (1998). Cerebellar complex spikes encode both destinations and errors in arm movements. Nature 392, 494–497. doi: 10.1038/33141
Koenigs, M., Ukueberuwa, D., Campion, P., Grafman, J., and Wassermann, E. (2009). Bilateral frontal transcranial direct current stimulation: failure to replicate classic findings in healthy subjects. Clin. Neurophysiol. 120, 80–84. doi: 10.1016/j.clinph.2008.10.010
Korman, M., Raz, N., Flash, T., and Karni, A. (2003). Multiple shifts in the representation of a motor sequence during the acquisition of skilled performance. Proc. Natl. Acad. Sci. USA 100, 12492–12497. doi: 10.1073/pnas.2035019100
Kuehne, M., Schmidt, K., Heinze, H. J., and Zaehle, T. (2019). Modulation of emotional conflict processing by high-definition transcranial direct current stimulation (HD-TDCS). Front. Behav. Neurosci. 13:224. doi: 10.3389/fnbeh.2019.00224
Kyllonen, P. C., and Christal, R. E. (1990). Reasoning ability is (little more than) working-memory capacity?! Intelligence 14, 389–433. doi: 10.1016/S0160-2896(05)80012-1
Lafon, B., Rahman, A., Bikson, M., and Parra, L. C. (2017). Direct current stimulation alters neuronal input/output function. Brain Stimul. 10, 36–45. doi: 10.1016/j.brs.2016.08.014
Lanina, A. A., Feurra, M., and Gorbunova, E. S. (2018). No effect of the right posterior parietal cortex tDCS in dual-target visual search. Front. Psychol. 9:2112. doi: 10.3389/fpsyg.2018.02112
Leite, J., Carvalho, S., Fregni, F., and Goncalves, O. F. (2011). Task-specific effects of tDCS-induced cortical excitability changes on cognitive and motor sequence set shifting performance. PLoS One 6:e24140. doi: 10.1371/journal.pone.0024140
Li, L. M., Uehara, K., and Hanakawa, T. (2015). The contribution of interindividual factors to variability of response in transcranial direct current stimulation studies. Front. Cell. Neurosci. 9:181. doi: 10.3389/fncel.2015.00181
Loo, C. K., Alonzo, A., Martin, D., Mitchell, P. B., Galvez, V., and Sachdev, P. (2012). Transcranial direct current stimulation for depression: 3-week, randomised, sham-controlled trial. Br. J. Psychiatry 200, 52–59. doi: 10.1192/bjp.bp.111.097634
Loo, C. K., Husain, M. M., McDonald, W. M., Aaronson, S., O'Reardon, J. P., Alonzo, A., et al. (2018). International randomized-controlled trial of transcranial direct current stimulation in depression. Brain Stimul. 11, 125–133. doi: 10.1016/j.brs.2017.10.011
Loo, C. K., Sachdev, P., Martin, D., Pigot, M., Alonzo, A., Malhi, G. S., et al. (2010). A double-blind, sham-controlled trial of transcranial direct current stimulation for the treatment of depression. Int. J. Neuropsychopharmacol. 13, 61–69. doi: 10.1017/S1461145709990411
Lopez-Alonso, V., Cheeran, B., Rio-Rodriguez, D., and Fernandez-Del-Olmo, M. (2014). Inter-individual variability in response to non-invasive brain stimulation paradigms. Brain Stimul. 7, 372–380. doi: 10.1016/j.brs.2014.02.004
Lopez-Alonso, V., Fernandez-Del-Olmo, M., Costantini, A., Gonzalez-Henriquez, J. J., and Cheeran, B. (2015). Intra-individual variability in the response to anodal transcranial direct current stimulation. Clin. Neurophysiol. 126, 2342–2347. doi: 10.1016/j.clinph.2015.03.022
Luna, F. G., Roman-Caballero, R., Barttfeld, P., Lupianez, J., and Martin-Arevalo, E. (2020). A high-definition tDCS and EEG study on attention and vigilance: brain stimulation mitigates the executive but not the arousal vigilance decrement. Neuropsychologia 142:107447. doi: 10.1016/j.neuropsychologia.2020.107447
Makowski, D., Ben-Shachar, M. S., Chen, S. H. A., and Ludecke, D. (2019). Indices of effect existence and significance in the Bayesian framework. Front. Psychol. 10:2767. doi: 10.3389/fpsyg.2019.02767
Mancuso, L. E., Ilieva, I. P., Hamilton, R. H., and Farah, M. J. (2016). Does transcranial direct current stimulation improve healthy working memory?: a Meta-analytic review. J. Cogn. Neurosci. 28, 1063–1089. doi: 10.1162/jocn_a_00956
Manuel, A. L., David, A. W., Bikson, M., and Schnider, A. (2014). Frontal tDCS modulates orbitofrontal reality filtering. Neuroscience 265, 21–27. doi: 10.1016/j.neuroscience.2014.01.052
Marshall, L., Kirov, R., Brade, J., Molle, M., and Born, J. (2011). Transcranial electrical currents to probe EEG brain rhythms and memory consolidation during sleep in humans. PLoS One 6:e16905. doi: 10.1371/journal.pone.0016905
Martin, D. M., Liu, R., Alonzo, A., Green, M., and Loo, C. K. (2014). Use of transcranial direct current stimulation (tDCS) to enhance cognitive training: effect of timing of stimulation. Exp. Brain Res. 232, 3345–3351. doi: 10.1007/s00221-014-4022-x
McIntire, L. K., McKinley, R. A., Goodyear, C., and Nelson, J. (2014). A comparison of the effects of transcranial direct current stimulation and caffeine on vigilance and cognitive performance during extended wakefulness. Brain Stimul. 7, 499–507. doi: 10.1016/j.brs.2014.04.008
McIntire, L. K., McKinley, R. A., Nelson, J. M., and Goodyear, C. (2017). Transcranial direct current stimulation versus caffeine as a fatigue countermeasure. Brain Stimul. 10, 1070–1078. doi: 10.1016/j.brs.2017.08.005
Minarik, T., Sauseng, P., Dunne, L., Berger, B., and Sterr, A. (2015). Effects of anodal transcranial direct current stimulation on visually guided learning of grip force control. Biology (Basel) 4, 173–186. doi: 10.3390/biology4010173
Miranda, P. C., Faria, P., and Hallett, M. (2009). What does the ratio of injected current to electrode area tell us about current density in the brain during tDCS? Clin. Neurophysiol. 120, 1183–1187. doi: 10.1016/j.clinph.2009.03.023
Miyake, A., and Shah, P. (1999). “Models of working memory: mechanisms of active maintenance and executive control” in Models of working memory: Mechanisms of active maintenance and executive control. eds. A. Miyake and P. Shah (New York, NY, US: Cambridge University Press), 1–27.
Monte-Silva, K., Kuo, M. F., Hessenthaler, S., Fresnoza, S., Liebetanz, D., Paulus, W., et al. (2013). Induction of late LTP-like plasticity in the human motor cortex by repeated non-invasive brain stimulation. Brain Stimul. 6, 424–432. doi: 10.1016/j.brs.2012.04.011
Murphy, O. W., Hoy, K. E., Wong, D., Bailey, N. W., Fitzgerald, P. B., and Segrave, R. A. (2020). Transcranial random noise stimulation is more effective than transcranial direct current stimulation for enhancing working memory in healthy individuals: Behavioural and electrophysiological evidence. Brain Stimul. 13, 1370–1380. doi: 10.1016/j.brs.2020.07.001
Naka, M., Matsuzawa, D., Ishii, D., Hamada, H., Uchida, T., Sugita, K., et al. (2018). Differential effects of high-definition transcranial direct current stimulation on verbal working memory performance according to sensory modality. Neurosci. Lett. 687, 131–136. doi: 10.1016/j.neulet.2018.09.047
Nakamoto, H., and Mori, S. (2012). Experts in fast-ball sports reduce anticipation timing cost by developing inhibitory control. Brain Cogn. 80, 23–32. doi: 10.1016/j.bandc.2012.04.004
Naros, G., Geyer, M., Koch, S., Mayr, L., Ellinger, T., Grimm, F., et al. (2016). Enhanced motor learning with bilateral transcranial direct current stimulation: impact of polarity or current flow direction? Clin. Neurophysiol. 127, 2119–2126. doi: 10.1016/j.clinph.2015.12.020
Nelson, J. T., McKinley, R. A., Golob, E. J., Warm, J. S., and Parasuraman, R. (2014). Enhancing vigilance in operators with prefrontal cortex transcranial direct current stimulation (tDCS). NeuroImage 85, 909–917. doi: 10.1016/j.neuroimage.2012.11.061
Nelson, J. M., McKinley, R. A., McIntire, L. K., Goodyear, C., and Walters, C. (2015). Augmenting visual search performance with transcranial direct current stimulation (tDCS). Mil. Psychol. 27, 335–347. doi: 10.1037/mil0000085
Nguemeni, C., Stiehl, A., Hiew, S., and Zeller, D. (2021). No impact of cerebellar anodal transcranial direct current stimulation at three different timings on motor learning in a sequential finger-tapping task. Front. Hum. Neurosci. 15:631517. doi: 10.3389/fnhum.2021.631517
Nikolin, S., Loo, C. K., Bai, S., Dokos, S., and Martin, D. M. (2015). Focalised stimulation using high definition transcranial direct current stimulation (HD-tDCS) to investigate declarative verbal learning and memory functioning. NeuroImage 117, 11–19. doi: 10.1016/j.neuroimage.2015.05.019
Nitsche, M. A., and Bikson, M. (2017). Extending the parameter range for tDCS: safety and tolerability of 4 mA stimulation. Brain Stimul. 10, 541–542. doi: 10.1016/j.brs.2017.03.002
Nitsche, M.A., Knotkova, H., and Woods, A.J., M. Bikson. Challenges, open questions and future direction in transcranial direct current stimulation research and applications. In: H. Knotkova, M. Nitsche, M. Bikson, and A. Woods, editors. Practical guide to transcranial direct current stimulation. Cham, Switzerland: Springer. (2019). p. 627–639.
Nitsche, M. A., and Paulus, W. (2000). Excitability changes induced in the human motor cortex by weak transcranial direct current stimulation. J. Physiol. 527, 633–639. doi: 10.1111/j.1469-7793.2000.t01-1-00633.x
Nobre, A. C., Coull, J. T., Walsh, V., and Frith, C. D. (2003). Brain activations during visual search: contributions of search efficiency versus feature binding. NeuroImage 18, 91–103. doi: 10.1006/nimg.2002.1329
Nydam, A. S., Sewell, D. K., and Dux, P. E. (2018). Cathodal electrical stimulation of frontoparietal cortex disrupts statistical learning of visual configural information. Cortex 99, 187–199. doi: 10.1016/j.cortex.2017.11.008
Oldrati, V., Colombo, B., and Antonietti, A. (2018). Combination of a short cognitive training and tDCS to enhance visuospatial skills: a comparison between online and offline neuromodulation. Brain Res. 1678, 32–39. doi: 10.1016/j.brainres.2017.10.002
Oldrati, V., Patricelli, J., Colombo, B., and Antonietti, A. (2016). The role of dorsolateral prefrontal cortex in inhibition mechanism: a study on cognitive reflection test and similar tasks through neuromodulation. Neuropsychologia 91, 499–508. doi: 10.1016/j.neuropsychologia.2016.09.010
Oldrati, V., and Schutter, D. (2018). Targeting the human cerebellum with transcranial direct current stimulation to modulate behavior: a Meta-analysis. Cerebellum 17, 228–236. doi: 10.1007/s12311-017-0877-2
Opitz, A., Paulus, W., Will, S., Antunes, A., and Thielscher, A. (2015). Determinants of the electric field during transcranial direct current stimulation. NeuroImage 109, 140–150. doi: 10.1016/j.neuroimage.2015.01.033
Paananen, T., Piironen, J., Bürkner, P. C., and Vehtari, A. (2021). Implicitly adaptive importance sampling. Stat. Comput. 31:16. doi: 10.1007/s11222-020-09982-2
Parasuraman, R. (1976). Consistency of individual differences in human vigilance performance: an abilities classification analysis. J. Appl. Psychol. 61, 486–492. doi: 10.1037/0021-9010.61.4.486
Parasuraman, R., Warm, J. S., and See, J. E. (1998). Brain systems of vigilance. The attentive brain. Cambridge, MA, US: The MIT Press, 221–256.
Parma, J. O., Profeta, V., Andrade, A. G. P., Lage, G. M., and Apolinario-Souza, T. (2021). TDCS of the primary motor cortex: learning the absolute dimension of a complex motor task. J. Mot. Behav. 53, 431–444. doi: 10.1080/00222895.2020.1792823
Pixa, N. H., Steinberg, F., and Doppelmayr, M. (2017). High-definition transcranial direct current stimulation to both primary motor cortices improves unimanual and bimanual dexterity. Neurosci. Lett. 643, 84–88. doi: 10.1016/j.neulet.2017.02.033
Plewnia, C., Zwissler, B., Langst, I., Maurer, B., Giel, K., and Kruger, R. (2013). Effects of transcranial direct current stimulation (tDCS) on executive functions: influence of COMT Val/met polymorphism. Cortex 49, 1801–1807. doi: 10.1016/j.cortex.2012.11.002
Polania, R., Nitsche, M. A., and Ruff, C. C. (2018). Studying and modifying brain function with non-invasive brain stimulation. Nat. Neurosci. 21, 174–187. doi: 10.1038/s41593-017-0054-4
Pollok, B., Keitel, A., Foerster, M., Moshiri, G., Otto, K., and Krause, V. (2020). The posterior parietal cortex mediates early offline-rather than online-motor sequence learning. Neuropsychologia 146:107555. doi: 10.1016/j.neuropsychologia.2020.107555
Postle, B. R. (2006). Working memory as an emergent property of the mind and brain. Neuroscience 139, 23–38. doi: 10.1016/j.neuroscience.2005.06.005
Prichard, G., Weiller, C., Fritsch, B., and Reis, J. (2014). Effects of different electrical brain stimulation protocols on subcomponents of motor skill learning. Brain Stimul. 7, 532–540. doi: 10.1016/j.brs.2014.04.005
Pupikova, M., Simko, P., Gajdos, M., and Rektorova, I. (2021). Modulation of working memory and resting-state fMRI by tDCS of the right Frontoparietal network. Neural Plast. 2021, 1–9. doi: 10.1155/2021/5594305
Rahman, A., Reato, D., Arlotti, M., Gasca, F., Datta, A., Parra, L. C., et al. (2013). Cellular effects of acute direct current stimulation: somatic and synaptic terminal effects. J. Physiol. 591, 2563–2578. doi: 10.1113/jphysiol.2012.247171
Ramaraju, S., Roula, M. A., and McCarthy, P. W. (2018). Modelling the effect of electrode displacement on transcranial direct current stimulation (tDCS). J. Neural Eng. 15:016019. doi: 10.1088/1741-2552/aa8d8a
Rassovsky, Y., Dunn, W., Wynn, J. K., Wu, A. D., Iacoboni, M., Hellemann, G., et al. (2018). Single transcranial direct current stimulation in schizophrenia: randomized, cross-over study of neurocognition, social cognition, ERPs, and side effects. PLoS One 13:e0197023. doi: 10.1371/journal.pone.0197023
Reinhart, R. M., and Woodman, G. F. (2015). Enhancing long-term memory with stimulation tunes visual attention in one trial. Proc. Natl. Acad. Sci. USA 112, 625–630. doi: 10.1073/pnas.1417259112
Reis, J., and Fritsch, B. (2011). Modulation of motor performance and motor learning by transcranial direct current stimulation. Curr. Opin. Neurol. 24, 590–596. doi: 10.1097/WCO.0b013e32834c3db0
Reis, D. J., Kaizer, A. M., Kinney, A. R., Bahraini, N. H., Holliday, R., Forster, J. E., et al. (2023). A practical guide to random-effects Bayesian meta-analyses with application to the psychological trauma and suicide literature. Psychol. Trauma 15, 121–130. doi: 10.1037/tra0001316
Reis, J., Schambra, H. M., Cohen, L. G., Buch, E. R., Fritsch, B., Zarahn, E., et al. (2009). Noninvasive cortical stimulation enhances motor skill acquisition over multiple days through an effect on consolidation. Proc. Natl. Acad. Sci. USA 106, 1590–1595. doi: 10.1073/pnas.0805413106
Rich, T. L., and Gillick, B. T. (2019). Electrode placement in transcranial direct current stimulation-how reliable is the determination of C3/C4? Brain Sci. 9:69. doi: 10.3390/brainsci9030069
Rich, T. L., Menk, J. S., Rudser, K. D., Chen, M., Meekins, G. D., Pena, E., et al. (2017). Determining electrode placement for transcranial direct current stimulation: a comparison of EEG- versus TMS-guided methods. Clin. EEG Neurosci. 48, 367–375. doi: 10.1177/1550059417709177
Robertson, E. M., Pascual-Leone, A., and Miall, R. C. (2004). Current concepts in procedural consolidation. Nat. Rev. Neurosci. 5, 576–582. doi: 10.1038/nrn1426
Rocha, K., Marinho, V., Magalhaes, F., Carvalho, V., Fernandes, T., Ayres, M., et al. (2020). Unskilled shooters improve both accuracy and grouping shot having as reference skilled shooters cortical area: an EEG and tDCS study. Physiol. Behav. 224:113036. doi: 10.1016/j.physbeh.2020.113036
Rosen, D. S., Erickson, B., Kim, Y. E., Mirman, D., Hamilton, R. H., and Kounios, J. (2016). Anodal tDCS to right dorsolateral prefrontal cortex facilitates performance for novice jazz improvisers but hinders experts. Front. Hum. Neurosci. 10:579. doi: 10.3389/fnhum.2016.00579
Rumpf, J. J., Wegscheider, M., Hinselmann, K., Fricke, C., King, B. R., Weise, D., et al. (2017). Enhancement of motor consolidation by post-training transcranial direct current stimulation in older people. Neurobiol. Aging 49, 1–8. doi: 10.1016/j.neurobiolaging.2016.09.003
Sadleir, R. J., Vannorsdall, T. D., Schretlen, D. J., and Gordon, B. (2012). Target optimization in transcranial direct current stimulation. Front. Psych. 3:90. doi: 10.3389/fpsyt.2012.00090
Samaei, A., Ehsani, F., Zoghi, M., Hafez Yosephi, M., and Jaberzadeh, S. (2017). Online and offline effects of cerebellar transcranial direct current stimulation on motor learning in healthy older adults: a randomized double-blind sham-controlled study. Eur. J. Neurosci. 45, 1177–1185. doi: 10.1111/ejn.13559
Sanes, J. N., and Donoghue, J. P. (2000). Plasticity and primary motor cortex. Annu. Rev. Neurosci. 23, 393–415. doi: 10.1146/annurev.neuro.23.1.393
Saturnino, G. B., Siebner, H. R., Thielscher, A., and Madsen, K. H. (2019). Accessibility of cortical regions to focal TES: dependence on spatial position, safety, and practical constraints. NeuroImage 203:116183. doi: 10.1016/j.neuroimage.2019.116183
Saucedo Marquez, C. M., Zhang, X., Swinnen, S. P., Meesen, R., and Wenderoth, N. (2013). Task-specific effect of transcranial direct current stimulation on motor learning. Front. Hum. Neurosci. 7:333. doi: 10.3389/fnhum.2013.00333
Schroeder, P. A., Schwippel, T., Wolz, I., and Svaldi, J. (2020). Meta-analysis of the effects of transcranial direct current stimulation on inhibitory control. Brain Stimul. 13, 1159–1167. doi: 10.1016/j.brs.2020.05.006
Sevilla-Sanchez, M., Hortobágyi, T., Fogelson, N., Iglesias-Soler, E., Carballeira, E., and Fernandez-del-Olmo, M. (2021). Small enhancement of bimanual typing performance after 20 sessions of tDCS in healthy young adults. Neuroscience 466, 26–35. doi: 10.1016/j.neuroscience.2021.05.001
Shilo, G., and Lavidor, M. (2019). Non-linear effects of cathodal transcranial direct current stimulation (tDCS) of the primary motor cortex on implicit motor learning. Exp. Brain Res. 237, 919–925. doi: 10.1007/s00221-019-05477-3
Spampinato, D. A., Satar, Z., and Rothwell, J. C. (2019). Combining reward and M1 transcranial direct current stimulation enhances the retention of newly learnt sensorimotor mappings. Brain Stimul. 12, 1205–1212. doi: 10.1016/j.brs.2019.05.015
Sung, K., and Gordon, B. (2018). Transcranial direct current stimulation (tDCS) facilitates overall visual search response times but does not interact with visual search task factors. PLoS One 13:e0194640. doi: 10.1371/journal.pone.0194640
Talimkhani, A., Abdollahi, I., Mohseni-Bandpei, M. A., Ehsani, F., Khalili, S., and Jaberzadeh, S. (2019). Differential effects of Unihemispheric concurrent dual-site and conventional tDCS on motor learning: a randomized, sham-controlled study. Basic Clin. Neurosci. 10, 59–72. doi: 10.32598/bcn.9.10.350
Tecchio, F., Zappasodi, F., Assenza, G., Tombini, M., Vollaro, S., Barbati, G., et al. (2010). Anodal transcranial direct current stimulation enhances procedural consolidation. J. Neurophysiol. 104, 1134–1140. doi: 10.1152/jn.00661.2009
Thach, W. T. (1996). On the specific role of the cerebellum in motor learning and cognition: clues from PET activation and lesion studies in man. Behav. Brain Sci. 19, 411–433. doi: 10.1017/S0140525X00081504
Thomas, F., Pixa, N. H., Berger, A., Cheng, M. Y., Doppelmayr, M., and Steinberg, F. (2020). Neither cathodal nor anodal transcranial direct current stimulation on the left dorsolateral prefrontal cortex alone or applied during moderate aerobic exercise modulates executive function. Neuroscience 443, 71–83. doi: 10.1016/j.neuroscience.2020.07.017
Toth, A. J., Ramsbottom, N., Constantin, C., Milliet, A., and Campbell, M. J. (2021). The effect of expertise, training and neurostimulation on sensory-motor skill in esports. Comput. Hum. Behav. 121:106782. doi: 10.1016/j.chb.2021.106782
Truong, D. Q., Magerowski, G., Blackburn, G. L., Bikson, M., and Alonso-Alonso, M. (2013). Computational modeling of transcranial direct current stimulation (tDCS) in obesity: impact of head fat and dose guidelines. Neuroimage Clin. 2, 759–766. doi: 10.1016/j.nicl.2013.05.011
van Schouwenburg, M. R., Sligte, I. G., Giffin, M. R., Günther, F., Koster, D., Spronkers, F. S., et al. (2021). Effects of Midfrontal brain stimulation on sustained attention. J. Cogn. Enhanc. 5, 62–72. doi: 10.1007/s41465-020-00179-z
Vannorsdall, T. D., van Steenburgh, J. J., Schretlen, D. J., Jayatillake, R., Skolasky, R. L., and Gordon, B. (2016). Reproducibility of tDCS results in a randomized trial: failure to replicate findings of tDCS-induced enhancement of verbal fluency. Cogn. Behav. Neurol. 29, 11–17. doi: 10.1097/WNN.0000000000000086
Vehtari, A., Gelman, A., and Gabry, J. (2017). Practical Bayesian model evaluation using leave-one-out cross-validation and WAIC. Stat. Comput. 27, 1413–1432. doi: 10.1007/s11222-016-9696-4
Vehtari, A., Simpson, D., Gelman, A., Yao, Y., and Gabry, J. (2024). Pareto smoothed importance sampling. J. Mach. Learn. Res. 25, 1–58.
Vergallito, A., Feroldi, S., Pisoni, A., and Romero Lauro, L. J. (2022). Inter-individual variability in tDCS effects: a narrative review on the contribution of stable, variable, and contextual factors. Brain Sci. 12:522. doi: 10.3390/brainsci12050522
Vines, B. W., Cerruti, C., and Schlaug, G. (2008). Dual-hemisphere tDCS facilitates greater improvements for healthy subjects' non-dominant hand compared to uni-hemisphere stimulation. BMC Neurosci. 9:103. doi: 10.1186/1471-2202-9-103
Vollmann, H., Conde, V., Sewerin, S., Taubert, M., Sehm, B., Witte, O. W., et al. (2013). Anodal transcranial direct current stimulation (tDCS) over supplementary motor area (SMA) but not pre-SMA promotes short-term visuomotor learning. Brain Stimul. 6, 101–107. doi: 10.1016/j.brs.2012.03.018
von Rein, E., Hoff, M., Kaminski, E., Sehm, B., Steele, C. J., Villringer, A., et al. (2015). Improving motor performance without training: the effect of combining mirror visual feedback with transcranial direct current stimulation. J. Neurophysiol. 113, 2383–2389. doi: 10.1152/jn.00832.2014
Wagner, J., Lo Monaco, S., Conto, F., Parrott, D., Battelli, L., and Rusconi, E. (2020). Effects of transcranial direct current stimulation over the posterior parietal cortex on novice X-ray screening performance. Cortex 132, 1–14. doi: 10.1016/j.cortex.2020.08.002
Warm, J. S., Parasuraman, R., and Matthews, G. (2008). Vigilance requires hard mental work and is stressful. Hum. Factors 50, 433–441. doi: 10.1518/001872008X312152
Westfall, J., Kenny, D. A., and Judd, C. M. (2014). Statistical power and optimal design in experiments in which samples of participants respond to samples of stimuli. J. Exp. Psychol. Gen. 143, 2020–2045. doi: 10.1037/xge0000014
Wiethoff, S., Hamada, M., and Rothwell, J. C. (2014). Variability in response to transcranial direct current stimulation of the motor cortex. Brain Stimul. 7, 468–475. doi: 10.1016/j.brs.2014.02.003
Williams, D. R., Rast, P., and Burkner, P. (2018). Bayesian meta-analysis with weakly informative prior distributions : PsyArXiv, 1–19. doi: 10.31234/osf.io/7tbrm
Wischnewski, M., Mantell, K. E., and Opitz, A. (2021). Identifying regions in prefrontal cortex related to working memory improvement: a novel meta-analytic method using electric field modeling. Neurosci. Biobehav. Rev. 130, 147–161. doi: 10.1016/j.neubiorev.2021.08.017
Woods, A. J., Antal, A., Bikson, M., Boggio, P. S., Brunoni, A. R., Celnik, P., et al. (2016). A technical guide to tDCS, and related non-invasive brain stimulation tools. Clin. Neurophysiol. 127, 1031–1048. doi: 10.1016/j.clinph.2015.11.012
Zimerman, M., Heise, K. F., Gerloff, C., Cohen, L. G., and Hummel, F. C. (2014). Disrupting the ipsilateral motor cortex interferes with training of a complex motor task in older adults. Cereb. Cortex 24, 1030–1036. doi: 10.1093/cercor/bhs385
Zivanovic, M., Paunovic, D., Konstantinovic, U., Vulic, K., Bjekic, J., and Filipovic, S. R. (2021). The effects of offline and online prefrontal vs parietal transcranial direct current stimulation (tDCS) on verbal and spatial working memory. Neurobiol. Learn. Mem. 179:107398. doi: 10.1016/j.nlm.2021.107398
Keywords: non-invasive brain stimulation, transcranial direct current stimulation, meta-analysis, meta-regression, research methods
Citation: Santander T, Leslie S, Li LJ, Skinner HE, Simonson JM, Sweeney P, Deen KP, Miller MB and Brunye TT (2024) Towards optimized methodological parameters for maximizing the behavioral effects of transcranial direct current stimulation. Front. Hum. Neurosci. 18:1305446. doi: 10.3389/fnhum.2024.1305446
Edited by:
Moussa Antoine Chalah, GHU Paris Psychiatrie et Neurosciences, FranceReviewed by:
Stefanie Verstraelen, University of Hasselt, BelgiumXuetong Zhai, University of Pittsburgh, United States
Charles Laidi, Assistance Publique Hopitaux De Paris, France
Copyright © 2024 Santander, Leslie, Li, Skinner, Simonson, Sweeney, Deen, Miller and Brunye. This is an open-access article distributed under the terms of the Creative Commons Attribution License (CC BY). The use, distribution or reproduction in other forums is permitted, provided the original author(s) and the copyright owner(s) are credited and that the original publication in this journal is cited, in accordance with accepted academic practice. No use, distribution or reproduction is permitted which does not comply with these terms.
*Correspondence: Tad T. Brunye, dGJydW55MDFAdHVmdHMuZWR1