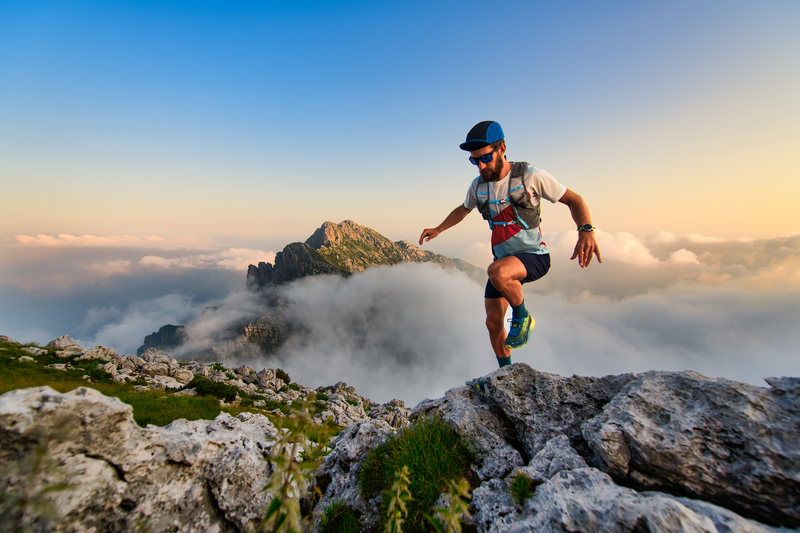
95% of researchers rate our articles as excellent or good
Learn more about the work of our research integrity team to safeguard the quality of each article we publish.
Find out more
STUDY PROTOCOL article
Front. Hum. Neurosci. , 04 April 2024
Sec. Cognitive Neuroscience
Volume 18 - 2024 | https://doi.org/10.3389/fnhum.2024.1287544
Introduction: Assistive technologies for learning are aimed at promoting academic skills, such as reading and mathematics. These technologies mainly embrace mobile and web apps addressed to children with learning difficulties. Nevertheless, most applications lack pedagogical foundation. Additionally, the task of selecting suitable technology for educational purposes becomes challenging. Hence, this protocol posits the psychophysiological assessment of an online method for learning (OML) named Smartick. This platform comprises reading and math activities for learning training. In this protocol, individual monitoring of each child is proposed to determine the progress in learning caused by Smartick.
Methods and analysis: One hundred and twelve children aged between 8 and 12 who present reading or math difficulty after a rigorous psychometric evaluation will be recruited. The study comprises four sessions. In sessions 1 and 2, collective and individual psychometric evaluations will be performed, respectively. Reading and mathematical proficiency will be assessed, as well as attentional levels and intellectual quotient. Subsequently, each child will be semi-randomly assigned to either the experimental or control groups. Afterward, a first EEG will be collected for all children in session 3. Then, experimental groups will use Smartick for 3 months, in addition to their traditional learning method. In contrast, control groups will only continue with their traditional learning method. Finally, session 4 will consist of performing a second psychometric evaluation and another EEG, so that psychophysiological parameters can be encountered that indicate learning improvements due to the OML, regardless of the traditional learning method at hand.
Discussion: Currently, few studies have validated learning improvement due to assistive technologies for learning. However, this proposal presents a psychophysiological evaluation addressed to children with reading or math difficulties who will be trained with an OML.
Elementary education allows children to develop basic cognitive skills for life, such as reading, writing, and calculation. For this reason, a wide variety of technologies have been designed to assist in the development or boosting of these abilities. Nevertheless, learning technologies often lack pedagogical foundation in their design (Ok et al., 2016; Papadakis, 2021). Unfortunately, there are no studies that validate the effects of these tools. Measuring the learning improvement is usually based on subjective parameters, such as opinion questionnaires or user experience. Conversely, there is a proliferation of digital applications on the internet (Papadakis and Kalogiannakis, 2017), and most of the download sites lack specificity at the description of these apps (Taylor et al., 2022). Therefore, the selection of an appropriate technology for learning is a challenging task for teachers, parents, or tutors. Lim and Toh (2022) proposed eight considerations to select an appropriate technology for learning:
• Determine what skills are to be developed (academic, social, or emotional)
• Learning methods, that can be oral/written, by positive feedback (giving rewards for succeeding activities), or collaborative, through co-teaching with parents or guardians
• Values encouraged by the interface
• Active interactivity with the technology for learning is highly recommended, instead of passive involvement (just watching).
• Gamification to increase motivation
• User friendly interface
• Age correspondence with the app
• Aesthetic interface design
Additionally, Noorhidawati et al. (2015) established that attention is necessary for a good performance within any app. Consequently, the learning app must fulfill three key points: (a) sensory stimulation (tactile, visual, auditory), (b) emotional expression, and (c) verbal expression. Once the user is engaged with the technology, learning comes from three directions:
• Cognitive direction, which encompasses mental processes, such as observation, recognition, comprehension and performing tasks within the app.
• Psychomotor direction, related with physical interaction with the system. Children must answer according to an incoming stimulus, either finger movement to select an appropriate option or the repetitiveness of actions that improve their performance.
• Affective direction associated with the emotional processing while using the app.
Currently, children show a growing interest in technology. By incorporating technology into the learning process, the ability to capture the child’s attention becomes faster, thereby enhancing the effectiveness of learning. In addition, there are advantages and disadvantages (Allison Academy, 1983; Brown, 2019) that must be considered about these technologies:
• Advantages
○ Attracts attention
○ Offers different learning strategies
○ Learning motivation
○ Each child learns at their own pace
○ Learning difficulties are shown easily
• Disadvantages
○ Tends to distract students
○ Social interaction is diminished
○ It can be used just for entertainment purposes
○ Excessive use of technology
○ Sustaining a learning technology can be expensive
Nowadays, some assistive technologies (AT) have been developed around the world to improve learning cognitive skills in children. These technologies embrace different mobile and web apps, augmented reality (AR) interfaces and virtual reality (VR) applications. AT for learning allows children to relieve anxiety while learning occurs in a playful way (Bryant et al., 2014). Additionally, some studies have found that using AT for learning can increase independent learning (Lindeblad et al., 2017), and enhance life functioning (Courtad and Bouck, 2013). Table 1 shows some works related to AT for learning.
Finally, the Smartick1 company developed an online method for learning for the improvement of reading and mathematical skills in children. This technology is intended for both typically developed children (TDC) and those with special educational needs. Smartick has two interfaces (reading and mathematics), which are based on artificial intelligence (AI) that allows adjusting difficulty of activities according to each child capability (Arroyo and González de Vega, 2009).
Electroencephalography (EEG) has been utilized in several studies to compare brain activity in individuals with and without reading or mathematical difficulties. EEG spectral analysis is a technique that has been widely used to identify neural correlates in the processing of reading and mathematics. Pavithran et al. (2019) suggested that an increment in theta/alpha ratio in dyslexics children is related with inability to sustain oriented attention in reading exercises. Additionally, Jäncke and Alahmadi (2016) found an increment in theta/alpha ratios across central and posterior regions, along with augmented theta/beta values throughout frontal and central recording sites in learning-disabled children. Also, higher theta/alpha and theta/beta ratios were elicited from resting-state EEG in children with attention deficit hyperactivity disorder with concomitant reading disabilities (Clarke et al., 2002). In the same line, ratio of theta/alpha band power has been utilized to investigate mathematical difficulties (Nassehi et al., 2020). Similarly, it has been demonstrated that frontal alpha asymmetry and frontal theta are well-known neural markers regarding the complexity of solving mathematical operations and problem solving, respectively (Formaz, 2023).
On the other hand, different brain regions have been found to be involved in reading and math processing. Arns et al. (2011) identified increments in delta and theta bands on frontal and right temporal regions, when comparing resting state EEG in dyslexic and healthy infants. These findings agree with Nora et al. (2021), who suggested that there are right hemispheric pathways that could compensate neural impairments in the processing of reading, despite the extensive literature supporting the role of the left hemisphere in language processing. However, higher alpha and beta power have been identified in dyslexic children over centroparietal cortex, during a writing task (Che Wan Fadzal et al., 2012a,b). As noted by Soltanlou et al. (2019), an increase in theta band in frontal and parietotemporal regions are associated with arithmetic problem solving and mathematical information retrieval, respectively. Other techniques have also been applied to differentiate EEG patterns between neurotypical children from those with learning difficulties. This information is shown in Table 2.
This protocol formally posits the proposal for a comprehensive psychophysiological assessment of an online method for learning (OML) referred to as Smartick (Arroyo and González de Vega, 2009). Smartick is an AI-technology that adapts in real-time to the needs of each child, which allows learning improvements in accordance with the child’s capabilities. The assessment will comprise: (a) the application of psychometric tests that evaluate reading and mathematical abilities, as well as attentional levels and intellectual quotient (IQ), and (b) EEG signal analysis before and after the use of Smartick. The project is focused on children between third and sixth grade of elementary school with low academic performance, either in reading or mathematics.
One hundred and twelve children from third to sixth grade will be recruited, based on the following selection criteria depicted in Table 3. Sample size was estimated using G*Power2 software (v3.1.9.7) for a two-sample independent t-test (effect size d = 0.8, and confidence level 95%). The participants must show low academic performance in reading or mathematics. If both learning difficulties are exhibited, only the learning area with the greatest academic lag will be considered. These difficulties will be detected in two stages. First, three psychometric tests will be performed collectively: (a) d2-R test, to assess attention capacity (Brickenkamp et al., 2022), (b) PREDISCAL, to evaluate reading comprehension and mathematics (Pina et al., 2020), and (c) a dictation task, for orthography assessment (Zhang et al., 2020). Then, each child will be evaluated individually, according to the learning difficulty identified in the previous stage. For reading evaluation, three characteristics will be considered: (a) reading speed, (b) errors in reading, and (c) reading comprehension. The reading proficiency will be determined according to the manual of procedures for the promotion and evaluation of reading competence in the classroom (MPPERCC). This document was published in 2012 by the Secretariat of Public Education in Mexico, and it states that these three key factors summarize the reading performance in children from first grade of primary to third grade of secondary school (Secretaría de Educación Pública, 2012). On the other hand, WRAT-4 will be applied to evaluate math proficiency (Wilkinson and Robertson, 2006). For both reading and mathematical difficulties, IQ will be estimated through the WISC-IV battery (Wechsler, 2007). All psychometric evaluations are described in Table 4.
Since the sample will be composed of children, parents or legal tutors must consent to the child participation. Participation of the children will be voluntary. Both parents or tutors and children must sign an informed consent, where the objectives of the study will be explained.
EEG signals will be collected using the g.USBamp3 system (gtec, Austria) with a sample frequency of 256 Hz. A 32-channel setup will be used according to the 10/20 international system (Fp1, Fp2, F7, F3, Fz, F4, F8, FT9, FC5, FC1, FC2, FC6, FT10, T7, C3, Cz, C4, T8, CP5, CP1, CP2, CP6, TP9, P7, P3, Pz, P4, P8, TP10, O1, Oz, and O2). Left earlobe and Fpz channel will be set as reference and ground, respectively.
This study will be composed of four sessions. In the first two sessions, psychometric tests will be applied to identify low academic performance in reading or mathematics in students from third to sixth grade of elementary school. In session 1, children will be assessed collectively to estimate their reading and mathematical proficiency, as well as their attentional levels. These results will be used as a screening test to determine children capabilities in reading or mathematics. Then, in session 2, each child will be evaluated individually according to the learning difficulty identified in session 1. Subsequently, low-achieving children will be assigned to one of two categories:
• Category 1: Low academic performance in reading
• Category 2: Low academic performance in math
After that, the first EEG acquisition will be conducted in session 3. Children in category 1 will be randomly allocated in one of the following groups:
• Group 1: Children with low performance in reading who will receive reading training with Smartick (experimental group for reading)
• Group 2: Children with low performance in reading who will not receive any training with Smartick (control group for reading)
Similarly, category 2 will be randomly assigned as follows:
• Group 3: Children with low performance in math who will receive math training with Smartick (experimental group for math)
• Group 4: Children with low performance in math who will not receive any training with Smartick (control group for math)
Children in category 1 and 2 will be executing reading and mathematical activities during EEG acquisition, respectively. Moreover, if the child volunteer is not willing to collaborate in the study, he/she will not be forced to carry out any test. Afterward, both experimental groups will start using Smartick after the first EEG session. Parents will be requested to ensure that the child undertakes a minimum of five sessions per week. Conversely, control groups will not receive any training and they will continue with their traditional learning method for 3 months. Then, each child must attend session 4, which will consist of a second individual psychometric evaluation (as in session 2), and a second EEG recording (as in session 3). Finally, the psychophysiological data gathered from experimental and control groups will be contrasted. The whole experimental procedure is depicted in Figure 1, and a detailed explanation of each session is described below.
Figure 1. Experimental procedure. Four sessions will be executed throughout this study. Collective and individual psychometric evaluations will be carried out in session 1 and 2. As a result, children will be randomly assigned into one of the following groups: (a) experimental group for reading, (b) control group for reading, (c) experimental group for math, and (d) control group for math. In session 3, a first EEG will be obtained. After that, experimental groups will receive the learning training with Smartick for 3 months, whereas control groups will continue with their conventional learning routine for 3 months. In session 4, an individual psychometric evaluation and a second EEG recording must be performed after the 3 months. Color code: white square, no group assignment; pink square, experimental group; blue square, control group. EEG, electroencephalography; OML, online method for learning.
It is noteworthy that providing a diagnosis of a specific learning disability is out of the scope of this work. However, psychometric results will be shared with parents or tutor, so that corrective actions can be taken as required.
The aim of this session will be to identify reading and mathematical difficulties, as well as attentional levels, in Mexican students from 3rd to 6th grade of elementary school. For this purpose, three psychometric tests will be applied collectively: (a) d2-R, for attention (Brickenkamp et al., 2022), (b) PREDISCAL, as a screening test for reading and mathematical skills (Pina et al., 2020), and (c) a word dictation for spelling proficiency (Zhang et al., 2020). At the end of this session, children will be categorized into one out of two categories: low academic performance in (a) reading or (b) mathematics. Those children with no learning difficulties will be excluded from the study. Conversely, if the child shows both learning difficulties, only the most affected area will be considered. If both learning difficulties are exhibited, only the learning area with the greatest academic lag will be considered. Prior to the execution of the tests, it is essential to create an atmosphere of immediate trust between students and the evaluator. The collective psychometric tests are described below:
The d-2 test allows evaluating selective attention and concentration (Brickenkamp, 2009). The test comprises 14 blocks with 47 characters each, where letters “d” and “p” will be randomly presented. Each character will have from one to four markers around them. The target stimuli will be letter “d” with two marks as indicated in Figure 2. The duration for each block will be 20 seconds. Once the block is completed, it will no longer be possible to revert to the previous one.
Figure 2. d2 test of attention. Yellow square, target stimulus; green square, target stimulus correctly identified; pink square, target stimulus incorrectly identified.
PREDISCAL is addressed to assess reading and arithmetical skills in children, aged between 7 and 12 years (Pina et al., 2020). PREDISCAL includes three subtests which assess: reading ability, mathematical fluency, and calculation. Regarding reading ability, decoding (grapheme-phoneme) and comprehension, syntax, basic orthography, and lexical knowledge are evaluated. On the other hand, elemental arithmetic operations (addition and subtraction) must be solved to assess mathematical fluency. Similarly, the calculation section attempts to examine mathematical dexterity by solving equivalences and equalities exercises. The PREDISCAL test is summarized in Figure 3.
Figure 3. PREDISCAL test. This assessment encompasses three subtests, which evaluate reading and mathematical ability. PREDISCAL will last 7 min (plus instructions).
This test is addressed to estimate orthographic knowledge in children. It consists in the dictation of 30 words (see Table 5), which have been found to be confusing during elementary education (Zhang et al., 2020). Each word will be dictated one by one, without repeating them. When finished, the evaluation sheets will be collected.
Those children who had low academic performance in the previous session will be individually evaluated. Additional reading and mathematical assessments will be applied to children with low academic performance in reading and mathematics, respectively. Once individual psychometric evaluations are finished, a sample of 112 children is expected to be recruited, which will be classified in four groups: (a) Group 1: 28 children with low performance in reading who will receive reading training with Smartick (experimental group for reading), (b) Group 2: 28 children with low performance in reading who will not receive any training with Smartick (control group for reading), (c) Group 3: 28 children with low performance in math who will receive math training with Smartick (experimental group for math) and (d) Group 4: 28 children with low performance in math who will not receive any training with Smartick (control group for math). Psychometric individual tests are described below:
Manual of procedures for the promotion and assessment of reading competence in the classroom (MPPARCC) is a protocol to assess reading speed, errors in reading, and reading comprehension in Mexican children. This tool is aimed at students from 1st grade of elementary school to 3rd grade of middle school (Secretaría de Educación Pública, 2012). This manual provides different texts to evaluate reading speed, where words read per minute will be considered. To implement this manual, a text will be presented to the child and then asked to read it aloud. The evaluator will measure the time it takes the child to read the whole text, and words per minute must be counted. Meanwhile, errors in reading must be identified (words modified during the reading and that were not corrected spontaneously). When the reading ends, reading comprehension will be assessed. The child will be asked three multiple-choice questions about the text. Finally, the child must tell what the story was about. The evaluation parameters for reading speed are mentioned in Table 6.
The purpose of this test is to evaluate mathematical ability so that difficulties in calculation can be identified (Wilkinson and Robertson, 2006). WRAT-4 comprises 40 exercises including counting, simple arithmetic and fraction operations, and basic algebra. WRAT-4 sheets will be distributed among children, and they will have 15 min to answer as many exercises as possible. Children with average or below-average performance will be considered for this study.
The WISC-IV evaluation (Wechsler, 2007) will be used to estimate IQ through two subtests: vocabulary (VB), and block design (BD) (Sattler, 2010). Regarding VB, the child will be asked to name four different images and to define some words, whereas in BD different geometric patterns must be reproduced, by using red and white cubes.
Participants must attend a first EEG session, where reading or mathematical tasks will be performed simultaneously with the EEG recording. Therefore, two separate EEG experimental paradigms will be designed, including reading (see Figure 4) and mathematical (see Figure 5) activities. Both paradigms will be created by using Psychopy v2022.2.4 (Peirce et al., 2019) and OpenVibe 3.3.1 (Renard et al., 2010), which are software widely used in Psychology and Neuroscience for experiment design. Reading and mathematical paradigms are explained below.
Figure 4. Experimental paradigm for reading. Reading evaluation will consist of displaying three different texts where the child must read carefully and aloud. The spacebar will have a green sticker, which must be pressed to start and end the reading. When the reading is finished, children must answer three reading comprehension questions with three possible answers each. Hence, children will choose one out of three options: (a) yellow (Key A), (b) blue (Key F) and (c) red (Key J).
Figure 5. Experimental paradigm for mathematics. Mathematical assessment involves solving 40 arithmetical operations (addition and subtraction), divided into 2 blocks. Children must press A (yellow sticker), F (blue sticker) or J (red sticker) key to answer each operation.
Electroencephalography (EEG) signals will be collected while children are reading three different texts. Before starting, a resting state EEG recording will be taken for 3 min (Jäncke and Alahmadi, 2016; Rogala et al., 2020), while the child is staring at a “X” in the screen. After that, each reading will be shown. The child will be asked to read every text aloud, according to their reading abilities. After each reading, three multiple choice comprehension questions will be displayed, where children must select 1 out of the 3 options. A brief break will be given to the participants at the end of each reading. Every EEG recording from children who do not finish the reading will be discarded.
A 3-min resting state will be recorded at the beginning of the experiment. Then, children will perform 2 blocks of 20 arithmetical operations each. EEG signals will be recorded in the process. The participant will get a short break after completing the first block. As in the reading paradigm, children must answer every mathematical operation by selecting one out of three possible answers: yellow, blue, or red stickers. EEG recordings gathered from children who solve less than 50% correct per block will be discarded.
Smartick is an OML designed in Spain in 2009 by Arroyo and González de Vega (2009). Smartick has two gamified sections: (a) reading and (b) mathematics. Both platforms are aimed at improving reading and mathematical proficiency, respectively. Each interface is AI-based where the difficulty of the activities is adjusted according to each child capability: as user demonstrates proficiency, the system elevates the challenge level, whereas, in the presence of errors, it adeptly lowers the difficulty to optimize the learning experience.
All sessions of Smartick are customized according to the needs of each child. The better performance the child has, the higher score he/she receives. This score consists in star points called ticks, which can be exchanged for many virtual prizes within Smartick. It is noteworthy that Smartick allows parents, tutors, and researchers to supervise user activity (i.e., login day and time, number of right answers and ticks earned). However, it is imperative that parents or tutor do not assist the child in solving the activities.
This research is sponsored by the Smartick company, which will grant free access to the participants involved in this study. Smartick licenses will last for 3 months, where children must interact with the app for 15-min daily. In previous studies, it has been demonstrated that this time is enough to elicit positive effects in children rehabilitation (Wuang et al., 2018; Benzing and Schmidt, 2019).
Experimental groups will be using Smartick during the 3 months after the first EEG session. For these groups, (a) average score, (b) average usage time, and (c) total days of use will be considered as behavioral data to evaluate the performance of the children. Conversely, control groups will not use Smartick, however, they will continue with their traditional learning method for 3 months. When the 3-month period is over, Smartick will be given to these children, so that learning training can be performed.
Once the use of Smartick is finished, a second EEG and individual psychometric evaluations will be conducted. The second EEG will be taken, following the experimental paradigms described in session 3 (see Figures 4, 5). Similarly, the second psychometric evaluation is referred to session 2 (see Section “2.3.2 Session 2: Individual psychometric evaluation”). Psychophysiological changes are expected to be identified between children who will use Smartick and children who will not, regardless of the learning difficulty.
Psychophysiological effects elicited in learning will be studied in children who will use and will not use an OML (independent variable). The experiment design will consider four study groups:
• Experimental groups, children with low academic performance in:
1. Reading
2. Mathematics
who will receive the learning training with Smartick.
• Control groups, children with low academic performance in:
3. Reading
4. Mathematics
who will not receive the learning training and will continue with their traditional method of learning.
Each child will be evaluated by the psychometric tests described in Table 7. These results (dependent variable 1) will determine which learning difficulty will be assisted by Smartick. From this technology, user performance (dependent variable 2) will be monitored to determine how the learning training improves the child’s proficiency. Moreover, EEG activity will be taken from both the experimental and control groups. This will allow the extraction of brain patterns (dependent variable 3) for the identification of neuroplastic changes associated with learning improvement. The study variables for each group are summarized below:
Electroencephalography (EEG) signals from all children will be analyzed by the following three steps: (a) preprocessing, and (b) processing. Both processes are depicted in Figure 6.
Figure 6. EEG signal analysis proposal. EEG data will come from children with low academic performance in reading or math. The preprocessing process (steps 1–7) will be the same for all groups. Conversely, both reading (steps 8–9) and math (steps 8–10) data will have independent signal processing.
Electroencephalography (EEG) data will be preprocessed in EEGLab toolbox (Delorme and Makeig, 2004) from MATLAB (The MathWorks Inc, 2020). An automated code will be created to clean EEG signals. First, DC offset will be removed, and the EEG data will be bandpass-filtered between 0.1 and 100 Hz. Additionally, a Notch filter for 60 Hz elimination will be implemented. After that, Artifact Subspace Reconstruction will be used to remove bad channels and exogenous artifacts. Finally, ICLabel will be used to eliminate muscular, cardiac, ocular, and line noise artifacts.
Since reading and mathematics are two independent cognitive processes, EEG data will be processed separately. For the reading paradigm, spontaneous EEG activity will be analyzed, where only pure reading activity will be recorded. Thus, a spectro-temporal analysis is proposed in terms of absolute and relative power, which can reflect a relationship to differentiate brain activity among children who will use and will not use Smartick. Regarding the mathematics paradigm, ERP will be used to determine EEG patterns related to mathematical processing. ERP will be obtained at the beginning of each arithmetical operation, just in the moment when each operation appears on the screen. Also, the response time will be calculated for each operation.
Statistical significance (p < 0.05) in brain activity before and after Smartick will be identified. EEG data will be taken with the experimental paradigms described in Sections “2.3.3.1 Experimental paradigm—Reading” and “2.3.3.2 Experimental paradigm—Mathematics.” The first EEG acquisition will be performed in session 3, where four groups will be encountered:
• Group 1: Children with low performance in reading who will receive reading training with Smartick (experimental group for reading).
• Group 2: Children with low performance in reading who will not receive any training with Smartick (control group for reading).
• Group 3: Children with low performance in math who will receive math training with Smartick (experimental group for math).
• Group 4: Children with low performance in math who will not receive any training with Smartick (control group for math).
Changes in brain activity are expected in the experimental groups, which may be significantly different than the control groups. Therefore, statistical analysis will be carried out by pairs, as stated below:
• Pair 1: Group 1 and Group 2
• Pair 2: Group 3 and Group 4
After the first EEG recording, experimental and control group of each category will be contrasted. Firstly, normality is to be determined for both pairs. If normality is satisfied, an independent t-student for two samples test will be applied. Otherwise, a Wilcoxon Sum-Rank test will be utilized. Additionally, effect size will be calculated through EEG channels to verify the magnitude of the differences. When the second EEG is taken, experimental groups will be compared before and after using Smartick to determine if improvements in reading or mathematics were encountered. The statistical analysis proposal is shown in Figure 7.
Figure 7. Statistical analysis proposal. Statistically significant differences will be determined among EEG recordings before and after Smartick.
This research aims to evaluate an OML called Smartick, which includes training programs for the improvement of reading and mathematical skills. The technology will be assessed by psychophysiological data that encompasses (a) psychometric tests to valuate reading and mathematical proficiency, attentional levels, and IQ, (b) EEG data that will be collected when reading and mathematical activities are being performed, and (c) behavioral data, through the user performance within Smartick. This methodology will be undertaken in four sessions: (a) collective psychometric evaluation, where children will go through different psychometric tests in order to determine overall performance in reading and math, (b) individual psychometric evaluation, whose assessment will be specifically for the deficient area found in previous session, (c) use and monitoring of the OML, in which a first EEG recording will be taken before the implementation of the 3-month period of Smartick, and (d) psychophysiological evaluation of the OML, where a second EEG and psychometric evaluations will be carried out, so that indicative parameters of learning enhancement due to Smartick can be detected.
As previously stated, different studies have been performed to correlate EEG data with reading and mathematical learning difficulties. Several reports have shown increasing power in delta (Arns et al., 2011), theta (Arns et al., 2011; Soltanlou et al., 2019), alpha and beta bands (Che Wan Fadzal et al., 2012a,b). Similarly, an increment in power ratios from theta/alpha (Clarke et al., 2002; Jäncke and Alahmadi, 2016; Pavithran et al., 2019; Nassehi et al., 2020), and theta/beta (Clarke et al., 2002; Jäncke and Alahmadi, 2016) was obtained in children with learning difficulties. These results suggest that children with learning difficulties manifest higher rates of theta activity, which is associated with developmental delay (Pop-Jordanova, 2012), such as inattention, and poor reaction time and calculation (Demos, 2005). In addition, various analyses have been implemented to distinguish EEG neuromarkers related to reading and math learning, such as SVM (Frid and Manevitz, 2018; Perera et al., 2018; Mahmoodin et al., 2019), approximate entropy (Andreadis et al., 2009), ERP (Frid and Manevitz, 2018; Proverbio and Carminati, 2019), wavelet (Mahmoodin et al., 2019), and coherence (González-Garrido et al., 2018).
To improve cognitive skills in children with learning difficulties, a wide variety of digital applications have been designed and applied as AT. Prior studies have developed some applications to improve learning proficiency in (a) reading skills (Dyslexia Baca, DytectiveU, EasyLexia, Namagi, Alphabetics, AlphaTots), (b) mathematics (Talasia, Calculic Kids, MathFun, Coolmath4kids), or (c) both (Cognifit, Starfall). Furthermore, alternative research has suggested additional technologies such as VR (Hwang and Hu, 2013; Maskati et al., 2021; Rodríguez-Cano et al., 2021), AR (Ho et al., 2011; Vinumol et al., 2013; Avila-Pesantez et al., 2019; Gupta et al., 2019; Tenemaza et al., 2019; Kellems et al., 2020; Miundy et al., 2020), and eye-tracking (Katona, 2023) to enhance learning abilities.
So far, there are few neuroimaging studies about learning improvement due to AT (Kucian et al., 2011; Eroglu et al., 2022). The majority of studies solely conduct subjective assessments, such as surveys or questionnaires to describe user experience. Therefore, this protocol will allow monitoring Mexican children who will use Smartick, so that a follow-up psychophysiological evaluation can be performed to show if the OML caused learning enhancement. Previous research has demonstrated that using learning technology helps to increase motivation and improves self-efficacy and self-confidence (Kovari and Katona, 2023). This work could be applied in other Spanish-speaking countries that wish to evaluate AT for learning in children. However, non-Spanish-speaking countries must implement other types of psychometric evaluations that assess reading and calculation skills according to the native language.
The Ethics Committee from the Neuroscience Institute of University of Guadalajara approved this protocol on 28 April 2023, under this number: ET122022-356.
CC-G: Conceptualization, Funding acquisition, Methodology, Project administration, Software, Writing – original draft. MR-F: Conceptualization, Methodology, Writing – review & editing. LA-V: Conceptualization, Funding acquisition, Methodology, Supervision, Writing – review & editing. DI-Z: Conceptualization, Methodology, Supervision, Writing – review & editing. VI-G: Conceptualization, Methodology, Writing – review & editing.
The authors declare that financial support was received for the research, authorship, and/or publication of this article. The acquisition of psychometric tests materials was funded by Tecnologico de Monterrey. Sistemas Virtuales de Aprendizaje (a.k.a. Smartick) was provided licenses for all children involved in this study. CC-G had a scholarship granted by the Consejo Nacional de Humanidades, Ciencias y Tecnologías (reference: 894658).
We thank Smartick for granting free licenses to those children who participated in this study.
The authors declare that the research was conducted in the absence of any commercial or financial relationships that could be construed as a potential conflict of interest.
All claims expressed in this article are solely those of the authors and do not necessarily represent those of their affiliated organizations, or those of the publisher, the editors and the reviewers. Any product that may be evaluated in this article, or claim that may be made by its manufacturer, is not guaranteed or endorsed by the publisher.
Allison Academy (1983). Advantages and disadvantages of technology in education. Miami Beach, FL: Allison Academy.
Andreadis, I., Giannakakis, G., Papageorgiou, C., and Nikita, K. (2009). “Detecting complexity abnormalities in dyslexia measuring approximate entropy of electroencephalographic signals,” in Proceedings of the 31st annual international conference of the IEEE engineering in medicine and biology society: Engineering the future of biomedicine, EMBC 2009, (Glasgow), 6292–6295.
Ariffin, M., Abd Halim, F., Arshad, N., Mehat, M., and Hashim, A. (2019). Calculic Kids© mobile app: The impact on educational effectiveness of dyscalculia children 702. International J. Innov. Technol. Explor. Eng. 8, 701–705.
Arns, M., Peters, S., Breteler, R., and Verhoeven, L. (2011). Different brain activation patterns in dyslexic children: Evidence from EEG power and coherence patterns for the double-deficit theory of dyslexia. J. Integr. Neurosci. 6, 175–190. doi: 10.1142/s0219635207001404
Arroyo, J., and González de Vega, D. (2009). Smartick. Madrid: Sistemas Virtuales de Aprendizaje S.L.
Avila-Pesantez, D., Vaca-Cardenas, L., Delgadillo Avila, R., Padilla Padilla, N., and Rivera, L. (2019). “Design of an augmented reality serious game for children with dyscalculia: A case study BT – technology trends,” in Technology trends, eds M. Botto-Tobar, G. Pizarro, M. Zúñiga-Prieto, M. D’Armas, and M. Zúñiga Sánchez (Cham: Springer International Publishing), 165–175.
Benzing, V., and Schmidt, M. (2019). The effect of exergaming on executive functions in children with ADHD: A randomized clinical trial. Scand. J. Med. Sci. Sports 29, 1243–1253.
Brickenkamp, R. (2009). “d2,” in Test de atención, 3rd Edn, ed. N. Cubero (Göttingen: Hogrefe TEA Ediciones).
Brickenkamp, R., Schmidt-Atzert, L., and Liepmann, D. (2022). “d2-R,” in Test de atención – revisado, ed. B. Ruíz-Fernández (Madrid: Hogrefe TEA Ediciones).
Brown, C. (2019). 12 pros and cons of technology in the classroom. Available online at: https://www.classcraft.com/blog/pros-and-cons-of-technology-in-the-classroom/ (accessed August 14, 2023).
Bryant, D., Bryant, B., and Ok, M. (2014). “Assistive Technologies for people with diverse abilities,” in Advances in special education, eds G. Lancioni and N. Singh (Cham: Springer), 251–276.
Che Wan Fadzal, C., Mansor, W., Lee, K., Mohamad, S., and Amirin, S. (2012a). “Frequency analysis of EEG signal generated from dyslexic children,” in Proceedings of the ISCAIE 2012 - 2012 IEEE symposium on computer applications and industrial electronics, (Coimbatore), 202–204.
Che Wan Fadzal, C., Mansor, W., Lee, Y., Mohamad, S., Mohamad, N., and Amirin, S. (2012b). “Comparison between characteristics of EEG signal generated from dyslexic and normal children,” in Proceedings of the IEEE-EMBS conference on biomedical engineering and sciences, IECBES 2012, (Penang), 843–846.
Clarke, A., Barry, R., Mccarthy, R., and Selikowitz, M. E. (2002). Analysis of children with attention-deficit/hyperactivity disorder and comorbid reading disabilities. J. Learn. Disabil. 35, 276–285.
Coolmath.com Llc (2017). Coolmath4kids. Math + Games for kids, teachers & Parents. New York, NY: Coolmath.com LLC.
Courtad, C., and Bouck, E. (2013). Assistive technology for students with learning disabilities. Adv. Spec. Educ. 25, 153–173. doi: 10.1108/S0270-401320130000025011
Daud, S., and Abas, H. (2013). “Dyslexia baca” mobile app – The learning ecosystem for dyslexic children,” in Proceedings of the 2013 international conference on advanced computer science applications and technologies, ACSAT 2013, (Washington, DC: IEEE Computer Society), 412–416.
Delorme, A., and Makeig, S. (2004). EEGLAB: An open source toolbox for analysis of single-trial EEG dynamics. J. Neurosci. Methods 13, 9–21.
Demos, J. (2005). Getting started with neurofeedback, 1st Edn. New York, NY: W. W. Norton & Company Inc, 304.
Eroglu, G., Köprülü, M., and Karabacak, B. (2022). Auto train brain mobile app for promoting dyslexia biomarker detection in children at home or at school: Feasibility, acceptability. Qeios 5, 1–17.
Medcalf, L. (2014). “Assistive technology at Easter seals crossroads.” Alphabetics: Multisensory app for those with dyslexia. Available online at: https://www.eastersealstech.com/2015/11/03/alphabetics-multisensory-app-for-those-with-dyslexia/
Formaz, C. (2023). Heart and brain: The physiological and neural signatures of learning mathematics. Maastricht: Maastricht University.
Frid, A., and Manevitz, L. (2018). Features and machine learning for correlating and classifying between brain areas and dyslexia. arXiv [Preprint]. arxiv:1812.10622.
Gómez-Velázquez, F., González-Garrido, A., Ruiz-Stovel, V., Villuendas-González, E., Martínez-Ramos, A., and Altamirano-Ríos, M. (2022). Event-related brain potentials study of arithmetic fact retrieval in children with different math achievement levels. J. Cogn. Psychol. 34, 996–1010. doi: 10.1080/20445911.2022.2090571
González-Garrido, A., Gómez-Velázquez, F., Salido-Ruiz, R., Espinoza-Valdez, A., Vélez-Pérez, H., Romo-Vazquez, R., et al. (2018). The analysis of EEG coherence reflects middle childhood differences in mathematical achievement. Brain Cogn. 124, 57–63.
Gupta, T., Sisodia, M., Fazulbhoy, S., Raju, M., and Agrawal, S. (2019). “Improving accessibility for dyslexic impairments using augmented reality,” in Proceedings of the 2019 international conference on computer communication and informatics, ICCCI 2019, (Coimbatore: IEEE), 1–4.
Ho, S., Lee, K., and Chui, J. (2011). “Enhancing learning experience of students with specific learning difficulties with augmented reality: A pilot study,” in Proceedings of the 3rd Asian conference on education, (New York, NY), 1057–1062.
Hwang, W., and Hu, S. (2013). Analysis of peer learning behaviors using multiple representations in virtual reality and their impacts on geometry problem solving. Comput. Educ. 62, 308–319. doi: 10.1016/j.compedu.2012.10.005
Jäncke, L., and Alahmadi, N. (2016). Resting state EEG in children with learning disabilities. Clin. EEG Neurosci. 47, 24–36.
Käser, T., Baschera, G., Kohn, J., Kucian, K., Richtmann, V., Grond, U., et al. (2013). Design and evaluation of the computer-based training program Calcularis for enhancing numerical cognition. Front. Psychol. 4:50596. doi: 10.3389/fpsyg.2013.00489
Katona, J. (2023). An eye movement study in unconventional usage of different software tools. Sensors 23:3823. doi: 10.3390/s23083823
Kellems, R., Eichelberger, C., Cacciatore, G., Jensen, M., Frazier, B., Simons, K., et al. (2020). Using video-based instruction via augmented reality to teach mathematics to middle school students with learning disabilities. J. Learn. Disabil. 53, 277–291.
Kovari, A., and Katona, J. (2023). Effect of software development course on programming self-efficacy. Educ. Inf. Technol. 28, 10937–10963.
Kucian, K., Grond, U., Rotzer, S., Henzi, B., Schönmann, C., Plangger, F., et al. (2011). Mental number line training in children with developmental dyscalculia. Neuroimage 57, 782–795. doi: 10.1016/j.neuroimage.2011.01.070
Lim, F., and Toh, W. (2022). Considerations on the curation of educational apps for digital play and learning. Contemp. Educ. Technol. 14:e366.
Lindeblad, E., Nilsson, S., Gustafson, S., and Svensson, I. (2017). Assistive technology as reading interventions for children with reading impairments with a one-year follow-up. Disabil. Rehabil. Assist. Technol. 12, 713–724. doi: 10.1080/17483107.2016.1253116
Little 10 Robots (2018). AlphaTots. Clever interactive games introduce letter sounds, action words. San Francisco, CA: Common sense media.
Mahmoodin, Z., Lee, K., Mansor, W., and Zainuddin, A. (2019). Support vector machine with theta-beta band power features generated from writing of dyslexic children. Int. J. Integr. Eng. 11, 42–50.
Maskati, E., Alkeraiem, F., Khalil, N., Baik, R., Aljuhani, R., and Alsobhi, A. (2021). Using virtual reality (VR) in teaching students with dyslexia. Int. J. Emerg. Technol. Learn. 16, 291–305.
Miundy, K., Manoharan, S., and Muniandy, N. (2020). PRELIMINARY IDENTIFICATION OF LEARNERS WITH DYSCALCULIA IN MALAYSIAN MAINSTREAM PRIMARY SCHOOL AS INITIAL ANALYSIS FOR EARLY INTERVENTION. Int. J. Adv. Sci. Technol. 29, 1831–1870.
Nassehi, F., Özdemir, M., and Erogul, O. (2020). “Investigating EEG based marker for diagnosis of mathematical difficulties,” in Proceedings of the 28th signal processing and communications applications conference, SIU 2020, (Gaziantep: IEEE).
Noorhidawati, A., Ghalebandi, S., and Siti Hajar, R. (2015). How do young children engage with mobile apps? Cognitive, psychomotor, and affective perspective. Comput. Educ. 87, 385–395. doi: 10.1016/j.compedu.2015.07.005
Nora, A., Renvall, H., Ronimus, M., Kere, J., Lyytinen, H., and Salmelin, R. (2021). Children at risk for dyslexia show deficient left-hemispheric memory representations for new spoken word forms. Neuroimage 229:117739. doi: 10.1016/j.neuroimage.2021.117739
Ok, M., Kim, M., Kang, E., and Bryant, B. (2016). How to find good apps: An evaluation rubric for instructional apps for teaching students with learning disabilities. Interv. Sch. Clin. 51, 244–252. doi: 10.1177/1053451215589179
Papadakis, S. (2021). Tools for evaluating educational apps for young children: A systematic review of the literature. Interact. Technol. Smart Educ. 18, 18–49.
Papadakis, S., and Kalogiannakis, M. (2017). Mobile educational applications for children: What educators and parents need to know. Int. J. Mobile Learn. Organ. 11, 256–277.
Pavithran, G., Arunkumar, K., Guhan Seshadri, N., Kumar Singh, B., Mahesh, V., and Geethanjali, B. (2019). “Index of theta/alpha ratio to quantify visual – spatial attention in dyslexics using electroencephalogram,” in Proceedings of the 5th international conference on advanced computing and communication systems, ICACCS 2019, (Coimbatore), 417–422.
Peirce, J., Gray, J., Simpson, S., MacAskill, M., Höchenberger, R., Sogo, H., et al. (2019). PsychoPy2: Experiments in behavior made easy. Behav. Res. Methods 51, 195–203. doi: 10.3758/s13428-018-01193-y
Perera, H., Shiratuddin, M., Wong, K., and Fullarton, K. E. E. G. (2018). Signal analysis of writing and typing between adults with dyslexia and normal controls. Int. J. Interact. Multimedia Artif. Intell. 5:62.
Pina, V., Hernández-Pérez, E., Antonio, J., Lorenzo, R., Javier, H., and Manual, F. (2020). Screening de dificultades lectoras y matemáticas, PREDISCAL. Madrid: Hogrefe TEA Ediciones.
Proverbio, A., and Carminati, M. (2019). Finger-counting observation interferes with number processing. Neuropsychologia 131, 275–284. doi: 10.1016/j.neuropsychologia.2019.06.001
Rello, L., Macías, A., Herrera, M., De Ros, C., Romero, E., and Bigham, J. (2017). “DytectiveU: A game to train the difficulties and the strengths of children with dyslexia,” in Proceedings of the 19th international ACM SIGACCESS conference on computers and accessibility – ASSETS 2017, (New York, NY), 319–320.
Renard, Y., Lotte, F., Gibert, G., Congedo, M., Maby, E., Delannoy, V., et al. (2010). OpenViBE: An open-source software platform to design, test and use brain-computer interfaces in real and virtual environments. Presence Teleoperat. Virt. Environ. 19, 35–53.
Rodríguez-Cano, S., Delgado-Benito, V., Ausín-Villaverde, V., and Martín, L. (2021). Design of a virtual reality software to promote the learning of students with dyslexia. Sustainability 13:8425.
Rogala, J., Kublik, E., Krauz, R., and Wróbel, A. (2020). Resting-state EEG activity predicts frontoparietal network reconfiguration and improved attentional performance. Sci. Rep. 10, 1–15. doi: 10.1038/s41598-020-61866-7
Rohizan, R., Soon, L., and Mubin, S. (2020). MathFun: Examining the effectiveness of calculic model in designing app for dyscalculia children. J. Phys. Conf. Ser. 1712:012031. doi: 10.1088/1742-6596/1712/1/012031
Sattler, J. (2010). Evaluación infantil. Fundamentos cognitivos. Guía de recursos. Mexico City: Manual Moderno.
Secretaría de Educación Pública (2012). Manual de procedimientos para el fomento y la valoración de la compentencia lectora en el aula. Mexico City: Secretaría de Educación Pública.
Skiada, R., Soroniati, E., Gardeli, A., and Zissis, D. (2014). EasyLexia 2.0: Redesigning our mobile application for children with learning difficulties. Themes Sci. Technol. Educ. 7, 119–135.
Soltanlou, M., Artemenko, C., Dresler, T., Fallgatter, A., Nuerk, H., and Ehlis, A. (2019). Oscillatory EEG changes during arithmetic learning in children. Dev. Neuropsychol. 44, 325–338. doi: 10.1080/87565641.2019.1586906
Sun, H., Shi, Q., Pazoki, S., Jia, Y., and Woltering, S. (2023). Neurophysiological correlates of reading difficulties in elementary school children. Dev. Neuropsychol. 48, 259–279. doi: 10.1080/87565641.2023.2225664
Taylor, G., Kolak, J., Bent, E., and Monaghan, P. (2022). Selecting educational apps for preschool children: How useful are website app rating systems? Br. J. Educ. Technol. 53, 1262–1282.
Tenemaza, M., Navarrete, R., Jaramillo, E., and Rodriguez, A. (2019). “Specific dyslexia exploratory test (TEDE): Two tasks using augmented reality,” in Proceedings of the AHFE 2018 international conferences on usability & user experience and human factors and assistive technology, (Orlando, FL: Springer), 925–933.
Vinumol, K., Chowdhury, A., Kambam, R., and Muralidharan, V. (2013). “Augmented reality based interactive text book: An assistive technology for students with learning disability,” in Proceedings of the 2013 15th symposium on virtual and augmented reality, SVR 2013, (Piscataway, NJ: IEEE), 232–235.
Wechsler, D. (2007). Escala wechsler de inteligencia para niños-IV. (WISC-IV). Versión estandarizada. Mexico City: Manual Moderno.
Wilkinson, G., and Robertson, G. (2006). Wide range achievement test (WRAT4), 4th Edn. Lutz, FL: Psychological Assessment Resources.
Wuang, Y., Chiu, Y., Chen, Y., Chen, C., Wang, C., Huang, C., et al. (2018). Game-based auxiliary training system for improving visual perceptual dysfunction in children with developmental disabilities: A proposed design and evaluation. Comput. Educ. 124, 27–36.
Zainuddin, A., Mansor, W., Lee, K., and Mahmoodin, Z. (2018). Performance of support vector machine in classifying EEG signal of dyslexic children using RBF Kernel. Indones. J. Electric. Eng. Comput. Sci. 9, 403–409.
Keywords: learning difficulties, EEG, assistive technology, low academic performance, psychophysiological evaluation, Smartick
Citation: Corona-González CE, Ramos-Flores M, Alonso-Valerdi LM, Ibarra-Zarate DI and Issa-Garcia V (2024) Psychophysiological evaluation of the Smartick method in children with reading and mathematical difficulties. Front. Hum. Neurosci. 18:1287544. doi: 10.3389/fnhum.2024.1287544
Received: 19 October 2023; Accepted: 11 March 2024;
Published: 04 April 2024.
Edited by:
Lutz Jäncke, University of Zurich, SwitzerlandReviewed by:
Jozsef Katona, University of Dunaújváros, HungaryCopyright © 2024 Corona-González, Ramos-Flores, Alonso-Valerdi, Ibarra-Zarate and Issa-Garcia. This is an open-access article distributed under the terms of the Creative Commons Attribution License (CC BY). The use, distribution or reproduction in other forums is permitted, provided the original author(s) and the copyright owner(s) are credited and that the original publication in this journal is cited, in accordance with accepted academic practice. No use, distribution or reproduction is permitted which does not comply with these terms.
*Correspondence: César E. Corona-González, YTAwODMzOTU5QHRlYy5teA==
Disclaimer: All claims expressed in this article are solely those of the authors and do not necessarily represent those of their affiliated organizations, or those of the publisher, the editors and the reviewers. Any product that may be evaluated in this article or claim that may be made by its manufacturer is not guaranteed or endorsed by the publisher.
Research integrity at Frontiers
Learn more about the work of our research integrity team to safeguard the quality of each article we publish.