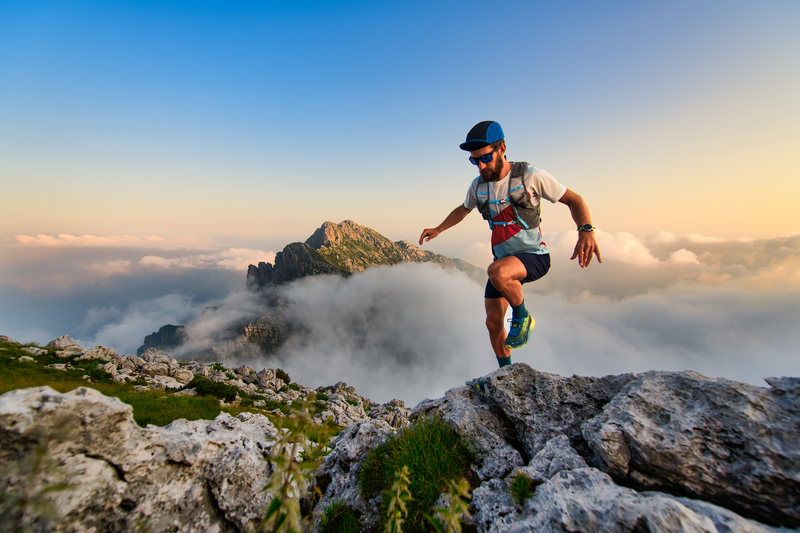
94% of researchers rate our articles as excellent or good
Learn more about the work of our research integrity team to safeguard the quality of each article we publish.
Find out more
ORIGINAL RESEARCH article
Front. Hum. Neurosci. , 11 January 2024
Sec. Brain Imaging and Stimulation
Volume 17 - 2023 | https://doi.org/10.3389/fnhum.2023.1308457
People are evolutionarily predisposed to associate threat relevant stimuli with fear or aversiveness and show an attentional bias toward threat. Attentional bias modification (ABM) has been shown to reduce threat biases, while quantitative reviews assessing the effectiveness of bias modification yielded inconsistent results. The current study examined the relationship between the training effect of attentional bias to threat and the type of threatening stimuli. Twenty-two participants performed a modified dot-probe task while undergoing functional near-infrared spectroscopy (fNIRS) imaging. Results indicated that there was a strong pattern of attentional avoidance among individuals in an animal but not human threat condition. Furthermore, findings from fNIRS confirmed that the influence from type of threatening stimulus would be modulated by cortical activation patterns, especially in the ventrolateral prefrontal cortices (vlPFC) and angular gyrus. Overall, these results suggest that stimulus-specific may play a major role in personalization of specific psychological interventions.
Being able to rapidly detect and react to threat-relevant information was of critical importance for the survival of humans and likely has a deep evolutionary origin (Mogg and Bradley, 1998; Panksepp, 1998; Montag et al., 2013; van Rooijen et al., 2017). Preparedness theory has postulated that humans are evolutionarily predisposed to associate threat-relevant stimuli with fear or aversiveness (Seligman, 1971; Ohman and Mineka, 2001). This perceptual prioritization of threat was an adaptive mechanism to prepare humans for acting upon the imminent danger to mobilize defensive resources promptly and avoid potential harm (Carlson et al., 2016). See also analysis of the fear system in different theories ranging from Gray (McNaughton and Corr, 2004; Reuter et al., 2015) to Panksepp et al. (2011), LeDoux (2014), and Montag and Davis (2018).
The claim for attentional bias for evolutionarily relevant threat has received an impressive amount of empirical support under a variety of experimental paradigms, conditions and clinical populations (Bar-Haim et al., 2007; Notebaert et al., 2011). Several studies examining visual search processes have reported that pictures of snakes and spiders elicit faster response times than other objects (e.g., flowers and mushrooms) in both humans (adults, infants and children) (Ohman et al., 2001; Lobue and DeLoache, 2008; Soares et al., 2009; LoBue, 2010; Soares and Esteves, 2013) and non-human primates (Shibasaki and Kawai, 2009). Similarly, human performance on the dot probe task has also found that other threats apart from predatory and venomous animals, including images of threatening faces and bodily harm, could also capture attention (Koster et al., 2006; Carlson et al., 2009). As can be seen, a similar pattern of attentional bias was found for both animal and human threat-relevant stimuli. These findings support the animate monitoring hypothesis, which proposed that humans are predisposed to attend preferentially to live objects in the environment (New et al., 2007). At the neural level, evidence from functional MRI studies have identified several brain regions that also respond greater to threat stimuli than for threat-irrelevant stimuli (Lacreuse et al., 2013). One of the neuroanatomical models was the stimulus-driven system associated with the amygdala, implicated in the emotional processing and directing attention to threatening information in the environment (Frewen et al., 2008; Carlson et al., 2016). Beyond this it is well known that the defensive distance between predator and prey play an important role to activate the fear system in the latter group (Mobbs et al., 2007). More importantly, attentional bias to negative-stimuli may arise from perturbation of a top-down attentional control system mediated by the ventrolateral and dorsolateral portions of the prefrontal cortices (vlPFC; dlPPFC) (Price et al., 2014; White et al., 2016; Edvinsson et al., 2017; Sylvester et al., 2017).
This selective attention to threat has been reliably observed across a range of anxious and other clinical populations, and characterizes healthy people with a vigilance particularly to highly threatening stimuli (Wilson and MacLeod, 2003; Koster et al., 2004a,b; Waters et al., 2004; Bishop, 2008; Kappenman et al., 2015; Pintzinger et al., 2016). Mounting studies suggest that the aberrant deployment of attention to negative emotional information may be a causal factor in the proximal illness process (Beck, 1976; Browning et al., 2010a; Mogg et al., 2017). Besides, these biases can be altered using cognitive training tasks (also known as attentional bias modification: ABM) (MacLeod and Mathews, 2012), confirmed in both clinical and non-clinical populations (Browning et al., 2010b). There is growing evidence that the use of ABM threat-avoidance training (e.g., the dot-probe task as the most common paradigm) in various clinical populations ameliorates symptoms [e.g., see reviews by Heeren et al. (2015), Li et al. (2016), Mogg et al. (2017), Kuckertz et al. (2019), Hang et al. (2021)], and the training effect in non-clinical participants also led to a significant decrease in attention to negative stimuli (MacLeod et al., 2002; Eldar et al., 2008; See et al., 2009; Browning et al., 2010b). Some quantitative reviews have examined the efficacy of ABM threat avoidance training on attentional bias, revealing that ABM produced a large effect in early analyses (Hakamata et al., 2010), while more recent reviews have suggested that ABM produced a small effect or was even ineffective (Cristea et al., 2015a,b; MacLeod and Clarke, 2015).
Such inconsistency in the training effect was not an isolated finding. One possible explanation for the instability of training effects relates to the different types of stimuli used by researchers (Ghosn et al., 2019). Studies have been conducted to examine the differential effects of aversive (e.g., threatening words or images) or appetitive (e.g., alcohol, smoking, food) stimuli on ABM (Beard et al., 2012). However, to our knowledge, no direct studies have examined whether the effectiveness of ABM training on attentional biases universally to all types of threats or is differentially influenced by threats from animals (e.g., spiders and snakes) and human (e.g., mutilation and blood) in the same dot-probe paradigm. Recent research has reported that faces (or bodies, etc.) that contain social information have a greater spontaneous attentional capture effect (van Rooijen et al., 2017). In addition, some studies have indicated that the reduction of change in attentional bias achieved with ABM tasks may be a consequence of improved attention control mediated by the prefrontal cortex (Mogg et al., 2017; Mogg and Bradley, 2018), such as via increased activation of the lateral prefrontal cortex (lPFC) (Browning et al., 2010b; Clarke et al., 2014; Nelson et al., 2015), while eye gaze may induce a faster attentional orienting and an amplified performance facilitation for targets by generating an internal representation of the spatial location indicated by the cue (Bindemann et al., 2007; Zhao and Zhang, 2009; Kuhn and Tipples, 2011; Hornung et al., 2019). Accordingly, for this study, a modified version of the dot-probe task with an eye gaze cue was designed to maximize the change in attention away from threat and enhanced attentional control using the blood oxygenation level-dependent (BOLD) functional near-infrared spectroscopy (fNIRS) signal. Therefore, we predicted that individuals would show different patterns of attentional bias for both animal and human threat stimuli, and may even recruit inconsistent neural circuits during the ABM training.
A total of 24 college students participated in the study for monetary payment. All participants were right-handed and did not have brain injury or a history of mental disorder. This study was approved by the Ethics Committee of Tianjin Normal University. Each participant was informed about the general nature of tasks and stimuli prior to signing an informed consent form.
An fNIRS system (LABNIRS/16, Shimadzu Corporation, Kyoto, Japan) with a 3-wavelength (780 nm, 805 nm and 830 nm) near infrared semiconductor laser system (1M level under the IED-60825-1 standard) was used at a sampling rate of 10 Hz in this study. Based on the modified Beer-Lambert law (MBLL), changes in the concentrations of oxy-hemoglobin (HbO), deoxy-hemoglobin (HbR) and total hemoglobin (HbT) were obtained by measuring the changes in near-infrared light absorption after its transmission through the tissue. Hoshi et al. (2001) and Hoshi (2007) showed that HbO is a sensitive indicator of the change in regional cerebral blood flow during task simulation and has a higher signal-to-noise ratio than HbR and HbT. Thus, our study focused on the HbO concentration only as an analysis indicator.
Eighty pictures from the Chinese Affective Picture System (Bai et al., 2005) were selected: 48 neutral images that included scenery, 32 unpleasant images that depicted threat (animal: 16; human: 16). All pictures were cropped and resized to 433 × 325 pixels by a photo editing software (Adobe Photoshop). A total of 25 additional college students revaluated these pictures using a 9-point Likert-type scale on valence and arousal. The results showed a difference in the valence rating of neutral (M = 5.40, SD = 0.42) and threatening (M = 2.66, SD = 0.74) images. In terms of arousal, threatening scenes (M = 6.78, SD = 0.77) were rated as high-arousing images compared to neutral scenes (M = 4.24, SD = 0.38). Besides, we examined the differences in valence and arousal across types of threat stimuli. The results of paired-sample T-test revealed both threat-stimulus categories did not differ in either valence [t(15) = −0.32, p = 0.75, Cohen’s d = −0.08] and arousal [t(15) = 0.41, p = 0.68, Cohen’s d = 0.09].
We created 4 neutral faces (2 man; 2 women) as social attention (gaze cue) stimuli using FaceGen Modeller.1 Two gaze directions (maximally left and maximally right) for each face were generated in FaceGen (see Figure 1A).
Figure 1. Sample social attention stimuli and schematics of the procedure. (A) Examples of adapting stimuli (left gaze = –100%, right gaze = + 100%). (B) Example trials sequence of experimental paradigm.
This study adopted a within-subject design with two within-subject factors: probe location (congruent vs. incongruent) and stimuli type (human threats vs. animal threats). During fNIRS, prior to and after the experimental procedure, participants were, respectively, given 60 s to rest. The formal experiment (modified dot-probe task) was presented to participants using E-prime software (2.0, Psychology Software Tools, Inc., Sharpsburg, PA) and displayed on a 13-inch monitor (Resolution: 1,024 × 768; Refresh rate 60 Hz). We employed an event-related design in which the complete presentation process of one trial was shown in Figure 1B. Trials comprised a centered fixation crosshair for 500 ms, social attention cue (the gaze direction always toward the location of the neutral images) for 500 ms, immediately afterward images in pair (neutral - threatening) appeared for 500 ms, a white dot probe (0.5 × 0.5 cm) appeared either on the left or the right side of the display and remained visible until 1,100 ms had elapsed. Participants were instructed to identify the position of the dot probe by pressing the “f” or “j” keys on the keyboard quickly and accurately. Each stimulus type was presented several times leading to a total of 96 trials. A total of 48 null trials (displaying only a plus sign and requiring no response) were first included in a randomized order to establish a baseline condition. Stimuli were presented in a pseudo-randomized fashion. A trial was labeled congruent when the dot probe appeared at the location of the emotional images and was labeled incongruent when the dot probe appeared at the location of the neutral images (see Figure 1B). Variable jitter times (4,000–9,000 ms) as intertrial interval was set to reduce temporal adjustment of the subjects to the task (Emberson et al., 2015).
One “3 × 3” and one “3 × 5” multi-channel probe board was used, including 13 emitters and 11 detectors, with a 3 cm distance between probes, forming a total of 34 channels. For each participant, the Fpz and T4 set as the reference point were placed on the bottom central and the rightmost central probe, respectively, according to the international 10–20 EEG system (see Figure 2A). Furthermore, the 3D Locator (FASTRAK; Polhemus, Colchester, VT, USA) was used to obtain the 3D coordinate points and channel anatomical locations based on head landmarks (Cz, Nz, AL, and AR). The probabilistic registration method was used for registration in the NIRS-SPM system of each channel position and Montreal Neurological Institute (MNI) spatial coordinates to obtain the corresponding Brodmann areas (see Supplementary Table 1 for further details). Our choice to cover the right hemisphere was based on prior studies that there was an overall pattern with the relationship between the right hemisphere and negative expression (Maxwell et al., 2005).
Figure 2. (A) The positions of the optode probes, the red squares represent the sources, and the blue squares represent the detectors, and between a source and a detector is the channel. (B) The functional localization into 5 ROIs based on proximity of channels and anatomy. ROI1 (the purple) = Frontopolar cortex (ch 1, 2, 3, 4, 5, 6, 7), ROI2 (the dark blue) = dlPFC (ch 8, 9, 10, 11, 12, 15, 18), ROI3 (the sky blue) = vlPFC (ch 13, 14, 16, 17), ROI4 (the orange) = SupraMarginal gyrus (ch 26, 27, 28, 29, 30, 31), ROI5 (the red) = angular gyrus (ch 32, 33, 34), and the rest channels in green (ch 19–25) were not the area of interest for this study.
Our montage was designed to optimize coverage of brain structures in the frontal, temporal, and parietal lobes. Figure 2B shows the functional localization clusters. Given the current data on neural correlates of attentional bias process in both healthy and clinical populations (White et al., 2016; Sylvester et al., 2017), we focused on five ROIs, that is, the Frontopolar cortex (FPC), dlPFC (dorsolateral prefrontal cortex), vlPFC (ventrolateral prefrontal cortex), SupraMarginal gyrus (SMG), and angular gyrus.
Cerebral cortex blood oxygenation data were processed with the NIRS-Statistical Parametric Mapping (NIRS-SPM) toolbox (version; v.4.1) operated based on MATLAB (Ye et al., 2009). Noises (head movement, heart rate, etc.) and drifts of the extracted fNIRS signal were eliminated by the Hemodynamic Response Functions (HRF) and wavelet-minimum description length method (Jang et al., 2009). The degree of the reaction induced by the experimental tasks in response to the reference wave (beta value) on each channel was evaluated by the general linear model (GLM) to obtain the fitting coefficient β, and the temporal autocorrelation of this process was adjusted using the pre-coloring method (Worsley and Friston, 1995).
Two participants were excluded from the sample for the following reasons: large motion artifacts and failure to remain awake during the process of data collection, and therefore, the final analysis dataset comprised 22 participants (14 women, mean age = 21.2 ± 1.7 years). Paired-samples t-tests of the obtained beta values according to the experimental design were used to investigate if there was activation under different task conditions. The significant level was set at p < 0.05. False discovery rate (FDR) correction was used to minimize false positive results (Noble, 2009). Limited by the number of sample size, we adopted permutation testing (5,000 iterations) to verify the statistical significance (Collingridge, 2013).
Response times (RTs) of very short duration (<100 ms) or timeouts (>1,000 ms) were discarded and we analyzed only trials with correct responses (Joormann and Gotlib, 2007). In order to explore the pattern of attentional bias under the effect of eyes gaze and examine the role of stimulus type, two-factor repeated measures ANOVAs were conducted on the behavioral data. For the RT, there was a significant main effect of stimuli-type, F(1,21) = 5.131, p < 0.05, η2 = 0.20. Although the main effect of probe location on RT was not significant [F(1,21) = 1.415, p > 0.05, η2 = 0.06], there was a trend of higher mean congruent type than the incongruent type. Besides, there was a significant interaction between probe-location and stimuli-type on RT, F(1,21) = 5.798, p < 0.05, η2 = 0.22. A post hoc pairwise comparison using the Bonferroni correction showed a shorter RT of the incongruent than congruent condition under the animal stimuli [t(21) = −2.19, p < 0.05, Cohen’s d = −0.32], however, for the human stimuli participants’ RT formed no significant difference between the two locations of probe [t(21) = 1.02, p = 0.32, Cohen’s d = 0.11]. Table 1 and Figure 3 illustrates the results of RT.
Table 1. Mean (with standard deviations) of RT (ms) under different probe location and stimuli type.
Figure 3. Mean RT (bars show standard errors) under different probe and stimuli type; *indicates significant differences between.
Paired-samples t-tests between baseline and probe location for each of the stimulus types were used to investigate if there was activation during the dot-probe task, and the t value for each channel in different conditions can be found in Figure 4. The average activation of the channels representing each ROI was further analyzed. For the animal stimuli type, there was significantly higher brain activation in the congruent condition in the vlPFC, t(21) = 2.295, p = 0.032 (marginal significance after FDR corrected), the SupraMarginal gyrus (SMG), t(21) = 2.529, p = 0.019 (FDR corrected), and the angular gyrus, t(21) = 2.829, p = 0.010 (FDR corrected) compared with the baseline. No ROI areas were significantly activated when in the incongruent condition (ps > 0.05). However, for the human stimuli type, no ROI areas were significantly activated compared with baseline in any location of probe (ps > 0.05). We have also re-analyzed these data with permutation test, and found results remain the same (Congruent condition of animal stimuli type: p = 0.016 for vlPFC, p = 0.011 for SMG, p = 0.007 for angular gyrus; p > 0.05 for all other conditions).
Figure 4. Heap maps of t values comparing activations between baseline and probe location in both the animal and human stimulus types. The t values at the optode probe location are determined through nearest-neighbor interpolation of the surrounding channels, and the data for the plot is further generated by cubic interpolation. Solid circles indicate the ROIs that showed significantly increased activations.
In addition, we then compared activation differences between the congruent condition and incongruent condition using paired-samples t-tests, and the t value for each channel in both the animal and human stimulus types can be found in Figure 5. The average activation of the channels representing each ROI was further analyzed. Results showed the animal stimulus type evoked significantly higher activation in the congruent than incongruent condition in the vlPFC, t(21) = 3.608, p = 0.002 (FDR corrected), and the angular gyrus, t(21) = 2.180, p = 0.041 (marginal significance after FDR corrected). There was no significant difference found among probe location under human stimulus type (ps > 0.05). We have also re-analyzed these data with permutation test, and found results remain the same (Animal stimuli type: p = 0.001 for vlPFC, p = 0.018 for angular gyrus; p > 0.05 for all other conditions).
Figure 5. Heap maps of t values comparing activation differences between the congruent condition and incongruent condition in both the animal and human stimulus types. The t values at the optode probe location are determined through nearest-neighbor interpolation of the surrounding channels, and the data for the plot is further generated by cubic interpolation. Solid circles indicate the ROIs that showed significantly increased activations.
We also analyzed the HbR data. The preprocess is the same way as the HbO data, and the paired-samples t-tests also showed the significant activation difference between probe location in the vlPFC and the angular gyrus (ps < 0.05, FDR corrected) under animal stimulus type. However, there was no significant difference found among baseline and probe location under animal stimulus type (ps > 0.05, FDR corrected).
To connect the significant findings in vlPFC and angular gyrus to behavior, we then tested the partial correlations between the differences of probe location [Bias Index (BI) = incongruent trials - congruent trials] (Hornung et al., 2019) of brain activation and behavioral performance after controlling for age and gender. For the vlPFC and angular gyrus the correlations were not significant. For the SupraMarginal gyrus (partial r = −0.500, p = 0.025), a negative association emerged under the human stimulus condition, but not for the animal stimulus condition. And the same trend was found in the frontopolar cortex for both animal (partial r = −0.511, p = 0.021) or human (partial r = −0.533, p = 0.016) stimuli conditions, respectively.
The current study aimed to evaluate the neural mechanism implicated in the training effect of attentional bias to threatening stimuli, and the possible modulation by stimulus type (animal threats vs. human threats) in a healthy adult sample using a modified dot-probe task with a within-subject design. The present study also provides preliminary evidence for stimulus-specific modulation that would allow the personalization of specific psychological interventions, resulting in greater effectiveness of bias modification.
Behavioral results provide evidence for cueing effects of gaze that may contribute to the modification of attentional bias toward threatening stimuli. Besides, there may be possible existence of two separate mechanisms for the modification of attentional bias across threat stimulus type. Specifically, participants responded significant slower to congruent than incongruent conditions, and this pattern of attentional avoidance only occurred within the condition of animal threats, which was not found in human threats. These findings in the human threats condition are consistent with the previous literature that humans and rhesus monkeys share mechanisms of social attention that allow the rapid detection of threatening conspecifics in the environment (Lacreuse et al., 2013). Yet, an important finding of this study is that attentional biases to conspecific threatening stimuli were stronger than non-conspecific threatening stimuli in humans. The differences can be due to multiple mechanisms. Specifically, the detection of different threats is influenced by evolutionary mechanisms (Carlson et al., 2016) and experience (LoBue, 2010). However, subjects’ greater sensitivity to human threats relative to animal threats may be due to the regulatory mechanisms of amygdala-prefrontal recruitment even under increased attentional control (Lacreuse et al., 2013; Carlson et al., 2016).
Brain imaging results further supported our hypothesis that stimulus type would modulate cortical activation patterns. When the animal threats were introduced, we found stronger involvement of the vlPFC and the angular gyrus during congruent compared to incongruent trials, while no significant neural difference was found between congruent and incongruent trials under the human threats condition. A likely explanation for this finding may be found in the theory of biased competition models of attention, which argues that captured attention may be achieved by using the lateral prefrontal cortex (LPFC) mechanisms to “prime” or strengthen the representations of stimuli that occur at a given location or other factors (Bishop et al., 2004; Bishop, 2008). Among them, the vlPFC region is crucially involved in attentional control processing (Price et al., 2014; White et al., 2016; Edvinsson et al., 2017; Sylvester et al., 2017) and thus greater vlPFC activation during animal stimulus presentation may reflect augmented top-down control to support processing of the attended stimuli influenced by the gaze-cueing effect (Bishop, 2008; Frewen et al., 2008; Browning et al., 2010b; Fani et al., 2012; Li et al., 2012). That is, improvement of attentional control can result in the avoidance of animal threats. Besides, the angular gyrus (BA 39), a subregion of the temporoparietal junction (TPJ), also played an important role in shifting of attention to allocate attentional resources to more efficiently process information from a stimulus (Friesen et al., 2004, 2005; Krumbholz et al., 2009). However, we did not find the same pattern of activation in the human stimulus condition, which may indicate consistency and combined with our behavioral results, demonstrates that there was a significant effect of stimulus-specific to attentional bias training.
In addition, negative correlations were revealed between the neural and behavioral bias scores in SupraMarginal gyrus (SMG) in the human stimulus condition and also in Frontopolar (FPC) in the arbitrary stimulus condition, suggesting that an increased behavioral bias index reflects a greater tendency of individuals’ attention to be drawn by human threats stimuli with social information (van Rooijen et al., 2017), with a concomitant reduction in the potential neural correlate (Lacreuse et al., 2013; Hornung et al., 2019). The region of SMG (BA 40) was considered to mainly constitute key nodes of the ventral attention network, which play a pivotal role in detecting unattended or unexpected stimuli and triggering shifts of attentional, leading to the allocation of more attentional resources to emotional stimuli (human threats) (Corbetta and Shulman, 2002; Hutchinson et al., 2009; Vossel et al., 2014). Previous studies showed that significantly decreased connectivity in anterior insula and SMG after ABM training (Li et al., 2016). Taking this a step further, the finding in the present study might therefore suggest that the attentional bias of human threats was also moderated by SMG. Besides, SMG has been shown to be important for empathy (Vollstädt-Klein et al., 2019). Human empathy for conspecific threatening stimuli compared to the non-conspecific may add evidence to our study of differences in response across stimulus type. The Frontopolar (BA 10), which is responsible for attentional control and was correlated in both stimulus conditions (Godier et al., 2016; Hilland et al., 2020), could be further illustrated that human threats stimuli were influenced to some extent by gaze cues, but this cueing effect was smaller than the emotional modulation of threat stimuli.
Several limitations of the current study need to be mentioned. First, although fNIRS is a feasible alternative to fMRI and with better spatial resolution and less susceptibility to head movement compared to EEG, it is also limited in its ability to measure hemodynamic changes in the deep brain. Further investigation of the relationship with the amygdala-PFC system may be useful for understanding the mechanism under stimulus-specific conditions during attentional bias training. Second, we used animal and human stimuli within the same task, and the two types of threat stimuli may mutually affect each other either through a priming effect or by increasing arousal (Lacreuse et al., 2013). Therefore, caution in interpretation is required when presenting both animal and human threat stimuli. Third, it has been hypothesized that over age, the pattern of attentional bias toward threats changes (van Rooijen et al., 2017). Future work with larger sample sizes across various age groups should continue to investigate possible age-related bias in reliability. Besides, Zhai et al. (2020) suggest that placement errors may be unavoidable due to the lack record of subject’s head size, and the tapered contrast vector method is better than uniform contrast vector method in ROI analysis, so further studies should take head circumferences (e.g., as covariates) and the new methodology in ROI analysis (tapered contrast vector) into account to confirm the present findings.
To conclude, the current study provides evidence that animal, but not human, threat stimuli elicit a strong pattern of attentional avoidance in healthy Chinese participants, mainly due to the cueing effect of gaze. And the neural basis further confirmed that the influence of the type of threat stimulus was modulated by cortical activation patterns, especially in the vlPFC and angular gyrus associated with the control and shift of attention. The results extend our understanding of the mechanisms of stimulus-specific conditions as a major contributor to the inconsistency of the training effect of attentional bias in individuals and may enable personalization of specific psychological interventions.
The raw data supporting the conclusions of this article will be made available by the authors, without undue reservation.
The studies involving humans were approved by the Tianjin Normal University, China. The studies were conducted in accordance with the local legislation and institutional requirements. The participants provided their written informed consent to participate in this study.
HL: Conceptualization, Data curation, Formal analysis, Methodology, Visualization, Writing—original draft. QZ: Writing—review and editing. JE: Writing—review and editing. CM: Writing—review and editing. HY: Conceptualization, Writing—review and editing.
The author(s) declare financial support was received for the research, authorship, and/or publication of this article. This study received a grant from the Humanities and Social Sciences of Tianjin Municipal Education Commission (52WR2307) and a grant from the National Natural Science Foundation of China (32271140).
The authors declare that the research was conducted in the absence of any commercial or financial relationships that could be construed as a potential conflict of interest.
All claims expressed in this article are solely those of the authors and do not necessarily represent those of their affiliated organizations, or those of the publisher, the editors and the reviewers. Any product that may be evaluated in this article, or claim that may be made by its manufacturer, is not guaranteed or endorsed by the publisher.
The Supplementary Material for this article can be found online at: https://www.frontiersin.org/articles/10.3389/fnhum.2023.1308457/full#supplementary-material
Bai, L., Ma, H., Huang, Y., and Luo, Y. (2005). The development of native Chinese affective picture system-a pretest in 46 college students. Chin. Ment. Health J. 19, 719–722.
Bar-Haim, Y., Lamy, D., Pergamin, L., Bakermans-Kranenburg, M. J., and van IJzendoorn, M. H. (2007). Threat-related attentional bias in anxious and nonanxious individuals: a meta-analytic study. Psychol. Bull. 133, 1–24. doi: 10.1037/0033-2909.133.1.1
Beard, C., Sawyer, A. T., and Hofmann, S. G. (2012). Efficacy of attention bias modification using threat and appetitive stimuli: a meta-analytic review. Behav. Ther. 43, 724–740. doi: 10.1016/j.beth.2012.01.002
Beck, A. T. (1976). Cognitive therapy and the emotional disorders. Oxford: International Universities Press.
Bindemann, M., Burton, A. M., Langton, S. R. H., Schweinberger, S. R., and Doherty, M. J. (2007). The control of attention to faces. J. Vis. 7, 15.1–8. doi: 10.1167/7.10.15
Bishop, S. J. (2008). Neural mechanisms underlying selective attention to threat. Ann. N. Y. Acad. Sci. 1129, 141–152. doi: 10.1196/annals.1417.016
Bishop, S. J., Duncan, J., Brett, M., and Lawrence, A. D. (2004). Prefrontal cortical function and anxiety: controlling attention to threat-related stimuli. Nat. Neurosci. 7, 184–188. doi: 10.1038/nn1173
Browning, M., Holmes, E. A., and Harmer, C. J. (2010a). The modification of attentional bias to emotional information: a review of the techniques, mechanisms, and relevance to emotional disorders. Cogn. Affect. Behav. Neurosci. 10, 8–20. doi: 10.3758/CABN.10.1.8
Browning, M., Holmes, E. A., Murphy, S. E., Goodwin, G. M., and Harmer, C. J. (2010b). Lateral prefrontal cortex mediates the cognitive modification of attentional bias. Biol. Psychiatry 67, 919–925. doi: 10.1016/j.biopsych.2009.10.031
Carlson, J. M., Fee, A. L., and Reinke, K. S. (2009). Backward masked snakes and guns modulate spatial attention. Evol. Psychol. 7, 534–544. doi: 10.1177/147470490900700404
Carlson, J. M., Torrence, R. D., and Vander Hyde, M. R. (2016). Beware the eyes behind the mask: the capture and hold of selective attention by backward masked fearful eyes. Motiv. Emot. 40, 498–505. doi: 10.1007/s11031-016-9542-1
Clarke, P. J. F., Browning, M., Hammond, G., Notebaert, L., and MacLeod, C. (2014). The causal role of the dorsolateral prefrontal cortex in the modification of attentional bias: evidence from transcranial direct current stimulation. Biol. Psychiatry 76, 946–952. doi: 10.1016/j.biopsych.2014.03.003
Collingridge, D. S. (2013). A primer on quantitized data analysis and permutation testing. J. Mix. Methods Res. 7, 81–97. doi: 10.1177/1558689812454457
Corbetta, M., and Shulman, G. L. (2002). Control of goal-directed and stimulus-driven attention in the brain. Nat. Rev. Neurosci. 3, 201–215. doi: 10.1038/nrn755
Cristea, I. A., Kok, R. N., and Cuijpers, P. (2015a). Efficacy of cognitive bias modification interventions in anxiety and depression: meta-analysis. Br. J. Psychiatry J. Ment. Sci. 206, 7–16. doi: 10.1192/bjp.bp.114.146761
Cristea, I. A., Mogoaşe, C., David, D., and Cuijpers, P. (2015b). Practitioner review: cognitive bias modification for mental health problems in children and adolescents: a meta-analysis. J. Child Psychol. Psychiatry 56, 723–734. doi: 10.1111/jcpp.12383
Edvinsson, A., Skalkidou, A., Hellgren, C., Gingnell, M., Ekselius, L., Willebrand, M., et al. (2017). Different patterns of attentional bias in antenatal and postpartum depression. Brain Behav. 7:e00844. doi: 10.1002/brb3.844
Eldar, S., Ricon, T., and Bar-Haim, Y. (2008). Plasticity in attention: implications for stress response in children. Behav. Res. Ther. 46, 450–461. doi: 10.1016/j.brat.2008.01.012
Emberson, L. L., Richards, J. E., and Aslin, R. N. (2015). Top-down modulation in the infant brain: learning-induced expectations rapidly affect the sensory cortex at 6 months. Proc. Natl. Acad. Sci. U.S.A. 112, 9585–9590. doi: 10.1073/pnas.1510343112
Fani, N., Jovanovic, T., Ely, T. D., Bradley, B., Gutman, D., Tone, E. B., et al. (2012). Neural correlates of attention bias to threat in post-traumatic stress disorder. Biol. Psychol. 90, 134–142. doi: 10.1016/j.biopsycho.2012.03.001
Frewen, P. A., Dozois, D. J. A., Joanisse, M. F., and Neufeld, R. W. J. (2008). Selective attention to threat versus reward: meta-analysis and neural-network modeling of the dot-probe task. Clin. Psychol. Rev. 28, 307–337. doi: 10.1016/j.cpr.2007.05.006
Friesen, C. K., Moore, C., and Kingstone, A. (2005). Does gaze direction really trigger a reflexive shift of spatial attention? Brain Cogn. 57, 66–69. doi: 10.1016/j.bandc.2004.08.025
Friesen, C. K., Ristic, J., and Kingstone, A. (2004). Attentional effects of counterpredictive gaze and arrow cues. J. Exp. Psychol. Hum. Percept. Perform. 30, 319–329. doi: 10.1037/0096-1523.30.2.319
Ghosn, F., Perea, M., Castelló, J., Vázquez, M. Á, Yáñez, N., Marcos, I., et al. (2019). Attentional patterns to emotional faces versus scenes in children with autism spectrum disorders. J. Autism Dev. Disord. 49, 1484–1492. doi: 10.1007/s10803-018-3847-8
Godier, L. R., Scaife, J. C., Braeutigam, S., and Park, R. J. (2016). Enhanced early neuronal processing of food pictures in anorexia nervosa: a magnetoencephalography study. Psychiatry J. 2016:e1795901. doi: 10.1155/2016/1795901
Hakamata, Y., Lissek, S., Bar-Haim, Y., Britton, J. C., Fox, N. A., Leibenluft, E., et al. (2010). Attention bias modification treatment: a meta-analysis toward the establishment of novel treatment for anxiety. Biol. Psychiatry 68, 982–990. doi: 10.1016/j.biopsych.2010.07.021
Hang, Y., Xu, L., Wang, C., Zhang, G., and Zhang, N. (2021). Can attention bias modification augment the effect of CBT for anxiety disorders? A systematic review and meta-analysis. Psychiatry Res. 299:113892. doi: 10.1016/j.psychres.2021.113892
Heeren, A., Mogoase, C., Philippot, P., and McNally, R. J. (2015). Attention bias modification for social anxiety: a systematic review and meta-analysis. Clin. Psychol. Rev. 40, 76–90. doi: 10.1016/j.cpr.2015.06.001
Hilland, E., Landrø, N. I., Harmer, C. J., Browning, M., Maglanoc, L. A., and Jonassen, R. (2020). Attentional bias modification is associated with fMRI response toward negative stimuli in individuals with residual depression: a randomized controlled trial. J. Psychiatry Neurosci. 45, 23–33. doi: 10.1503/jpn.180118
Hornung, J., Noack, H., Kogler, L., and Derntl, B. (2019). Exploring the fMRI based neural correlates of the dot probe task and its modulation by sex and body odor. Psychoneuroendocrinology 99, 87–96. doi: 10.1016/j.psyneuen.2018.08.036
Hoshi, Y. (2007). Functional near-infrared spectroscopy: current status and future prospects. J. Biomed. Opt. 12:062106. doi: 10.1117/1.2804911
Hoshi, Y., Kobayashi, N., and Tamura, M. (2001). Interpretation of near-infrared spectroscopy signals: a study with a newly developed perfused rat brain model. J. Appl. Physiol. Bethesda Md 1985, 1657–1662. doi: 10.1152/jappl.2001.90.5.1657
Hutchinson, J. B., Uncapher, M. R., and Wagner, A. D. (2009). Posterior parietal cortex and episodic retrieval: convergent and divergent effects of attention and memory. Learn. Mem. 16, 343–356. doi: 10.1101/lm.919109
Jang, K. E., Tak, S., Jung, J., Jang, J., Jeong, Y., and Ye, J. C. (2009). Wavelet minimum description length detrending for near-infrared spectroscopy. J. Biomed. Opt. 14:034004. doi: 10.1117/1.3127204
Joormann, J., and Gotlib, I. H. (2007). Selective attention to emotional faces following recovery from depression. J. Abnorm. Psychol. 116, 80–85. doi: 10.1037/0021-843X.116.1.80
Kappenman, E. S., MacNamara, A., and Proudfit, G. H. (2015). Electrocortical evidence for rapid allocation of attention to threat in the dot-probe task. Soc. Cogn. Affect. Neurosci. 10, 577–583. doi: 10.1093/scan/nsu098
Koster, E. H. W., Crombez, G., Van Damme, S., Verschuere, B., and De Houwer, J. (2004a). Does imminent threat capture and hold attention? Emotion 4, 312–317. doi: 10.1037/1528-3542.4.3.312
Koster, E. H. W., Crombez, G., Verschuere, B., and De Houwer, J. (2004b). Selective attention to threat in the dot probe paradigm: differentiating vigilance and difficulty to disengage. Behav. Res. Ther. 42, 1183–1192. doi: 10.1016/j.brat.2003.08.001
Koster, E. H. W., Crombez, G., Verschuere, B., and De Houwer, J. (2006). Attention to threat in anxiety-prone individuals: mechanisms underlying attentional bias. Cogn. Ther. Res. 30, 635–643. doi: 10.1007/s10608-006-9042-9
Krumbholz, K., Nobis, E. A., Weatheritt, R. J., and Fink, G. R. (2009). Executive control of spatial attention shifts in the auditory compared to the visual modality. Hum. Brain Mapp. 30, 1457–1469. doi: 10.1002/hbm.20615
Kuckertz, J. M., Schofield, C. A., Clerkin, E. M., Primack, J., Boettcher, H., Weisberg, R. B., et al. (2019). Attentional bias modification for social anxiety disorder: what do patients think and why does it matter? Behav. Cogn. Psychother. 47, 16–38. doi: 10.1017/s1352465818000231
Kuhn, G., and Tipples, J. (2011). Increased gaze following for fearful faces. It depends on what you’re looking for! Psychon. Bull. Rev. 18, 89–95. doi: 10.3758/s13423-010-0033-1
Lacreuse, A., Schatz, K., Strazzullo, S., King, H. M., and Ready, R. (2013). Attentional biases and memory for emotional stimuli in men and male rhesus monkeys. Anim. Cogn. 16, 861–871. doi: 10.1007/s10071-013-0618-y
LeDoux, J. E. (2014). Coming to terms with fear. Proc. Natl. Acad. Sci. U.S.A. 111, 2871–2878. doi: 10.1073/pnas.1400335111
Li, C., Chen, K., Han, H., Chui, D., and Wu, J. (2012). An FMRI study of the neural systems involved in visually cued auditory top-down spatial and temporal attention. PLoS One 7:e49948. doi: 10.1371/journal.pone.0049948
Li, H., Wei, D., Browning, M., Du, X., Zhang, Q., and Qiu, J. (2016). Attentional bias modification (ABM) training induces spontaneous brain activity changes in young women with subthreshold depression: a randomized controlled trial. Psychol. Med. 46, 909–920. doi: 10.1017/s003329171500238x
LoBue, V. (2010). What’s so scary about needles and knives? Examining the role of experience in threat detection. Cogn. Emot. 24, 180–187. doi: 10.1080/02699930802542308
Lobue, V., and DeLoache, J. S. (2008). Detecting the snake in the grass: attention to fear-relevant stimuli by adults and young children. Psychol. Sci. 19, 284–289. doi: 10.1111/j.1467-9280.2008.02081.x
MacLeod, C., and Clarke, P. J. F. (2015). The attentional bias modification approach to anxiety intervention. Clin. Psychol. Sci. 3, 58–78. doi: 10.1177/2167702614560749
MacLeod, C., and Mathews, A. (2012). Cognitive bias modification approaches to anxiety. Annu. Rev. Clin. Psychol. 8, 189–217. doi: 10.1146/annurev-clinpsy-032511-143052
MacLeod, C., Rutherford, E., Campbell, L., Ebsworthy, G., and Holker, L. (2002). Selective attention and emotional vulnerability: assessing the causal basis of their association through the experimental manipulation of attentional bias. J. Abnorm. Psychol. 111, 107–123.
Maxwell, J. S., Shackman, A. J., and Davidson, R. J. (2005). Unattended facial expressions asymmetrically bias the concurrent processing of nonemotional information. J. Cogn. Neurosci. 17, 1386–1395. doi: 10.1162/0898929054985437
McNaughton, N., and Corr, P. J. (2004). A two-dimensional neuropsychology of defense: fear/anxiety and defensive distance. Neurosci. Biobehav. Rev. 28, 285–305. doi: 10.1016/j.neubiorev.2004.03.005
Mobbs, D., Petrovic, P., Marchant, J. L., Hassabis, D., Weiskopf, N., Seymour, B., et al. (2007). When fear is near: threat imminence elicits prefrontal-periaqueductal gray shifts in humans. Science 317, 1079–1083. doi: 10.1126/science.1144298
Mogg, K., and Bradley, B. P. (1998). A cognitive-motivational analysis of anxiety. Behav. Res. Ther. 36, 809–848. doi: 10.1016/s0005-7967(98)00063-1
Mogg, K., and Bradley, B. P. (2018). Anxiety and threat-related attention: cognitive-motivational framework and treatment. Trends Cogn. Sci. 22, 225–240. doi: 10.1016/j.tics.2018.01.001
Mogg, K., Waters, A. M., and Bradley, B. P. (2017). Attention bias modification (ABM): review of effects of multisession ABM training on anxiety and threat-related attention in high-anxious individuals. Clin. Psychol. Sci. J. Assoc. Psychol. Sci. 5, 698–717. doi: 10.1177/2167702617696359
Montag, C., and Davis, K. L. (2018). Affective neuroscience theory and personality: an update. Personal. Neurosci. 1:e12. doi: 10.1017/pen.2018.10
Montag, C., Reuter, M., Jurkiewicz, M., Markett, S., and Panksepp, J. (2013). Imaging the structure of the human anxious brain: a review of findings from neuroscientific personality psychology. Rev. Neurosci. 24, 167–190. doi: 10.1515/revneuro-2012-0085
Nelson, B. D., Jackson, F., Amir, N., and Hajcak, G. (2015). Single-session attention bias modification and error-related brain activity. Cogn. Affect. Behav. Neurosci. 15, 776–786. doi: 10.3758/s13415-015-0365-4
New, J., Cosmides, L., and Tooby, J. (2007). Category-specific attention for animals reflects ancestral priorities, not expertise. Proc. Natl. Acad. Sci. U.S.A. 104, 16598–16603. doi: 10.1073/pnas.0703913104
Noble, W. S. (2009). How does multiple testing correction work? Nat. Biotechnol. 27, 1135–1137. doi: 10.1038/nbt1209-1135
Notebaert, L., Crombez, G., Van Damme, S., De Houwer, J., and Theeuwes, J. (2011). Signals of threat do not capture, but prioritize, attention: a conditioning approach. Emotion 11, 81–89. doi: 10.1037/a0021286
Ohman, A., and Mineka, S. (2001). Fears, phobias, and preparedness: toward an evolved module of fear and fear learning. Psychol. Rev. 108, 483–522. doi: 10.1037/0033-295x.108.3.483
Ohman, A., Flykt, A., and Esteves, F. (2001). Emotion drives attention: detecting the snake in the grass. J. Exp. Psychol. Gen. 130, 466–478. doi: 10.1037//0096-3445.130.3.466
Panksepp, J. (1998). Affective neuroscience: the foundations of human and animal emotions. New York, NY: Oxford university press.
Panksepp, J., Fuchs, T., and Iacobucci, P. (2011). The basic neuroscience of emotional experiences in mammals: the case of subcortical FEAR circuitry and implications for clinical anxiety. Appl. Anim. Behav. Sci. 129, 1–17. doi: 10.1016/j.applanim.2010.09.014
Pintzinger, N. M., Pfabigan, D. M., Tran, U. S., Kryspin-Exner, I., and Lamm, C. (2016). Attentional biases in healthy adults: exploring the impact of temperament and gender. J. Behav. Ther. Exp. Psychiatry 52, 29–37. doi: 10.1016/j.jbtep.2016.02.003
Price, R. B., Siegle, G. J., Silk, J. S., Ladouceur, C. D., McFarland, A., Dahl, R. E., et al. (2014). Looking under the hood of the dot-probe task: an fMRI study in anxious youth. Depress. Anxiety 31, 178–187. doi: 10.1002/da.22255
Reuter, M., Cooper, A. J., Smillie, L. D., Markett, S., and Montag, C. (2015). A new measure for the revised reinforcement sensitivity theory: psychometric criteria and genetic validation. Front. Syst. Neurosci. 9:38. doi: 10.3389/fnsys.2015.00038
See, J., MacLeod, C., and Bridle, R. (2009). The reduction of anxiety vulnerability through the modification of attentional bias: a real-world study using a home-based cognitive bias modification procedure. J. Abnorm. Psychol. 118, 65–75. doi: 10.1037/a0014377
Seligman, M. E. P. (1971). Phobias and preparedness. Behav. Ther. 2, 307–320. doi: 10.1016/S0005-7894(71)80064-3
Shibasaki, M., and Kawai, N. (2009). Rapid detection of snakes by Japanese monkeys (Macaca fuscata): an evolutionarily predisposed visual system. J. Comp. Psychol. 123, 131–135. doi: 10.1037/a0015095
Soares, S. C., and Esteves, F. (2013). A glimpse of fear: fast detection of threatening targets in visual search with brief stimulus durations. Psych J. 2, 11–16. doi: 10.1002/pchj.18
Soares, S. C., Esteves, F., Lundqvist, D., and Öhman, A. (2009). Some animal specific fears are more specific than others: evidence from attention and emotion measures. Behav. Res. Ther. 47, 1032–1042. doi: 10.1016/j.brat.2009.07.022
Sylvester, C. M., Petersen, S. E., Luby, J. L., and Barch, D. M. (2017). Face processing in adolescents with positive and negative threat bias. Psychol. Med. 47, 800–809. doi: 10.1017/s003329171600310x
van Rooijen, R., Ploeger, A., and Kret, M. E. (2017). The dot-probe task to measure emotional attention: a suitable measure in comparative studies? Psychon. Bull. Rev. 24, 1686–1717. doi: 10.3758/s13423-016-1224-1
Vollstädt-Klein, S., Bumb, J. M., Otto, A., Dinter, C., Karl, D., Koopmann, A., et al. (2019). The effects of nalmefene on emotion processing in alcohol use disorder - A randomized, controlled fMRI study. Eur. Neuropsychopharmacol. J. Eur. Coll. Neuropsychopharmacol. 29, 1442–1452. doi: 10.1016/j.euroneuro.2019.10.014
Vossel, S., Geng, J. J., and Fink, G. R. (2014). Dorsal and ventral attention systems: distinct neural circuits but collaborative roles. Neurosci. Rev. J. Bringing Neurobiol. Neurol. Psychiatry 20, 150–159. doi: 10.1177/1073858413494269
Waters, A. M., Lipp, O. V., and Spence, S. H. (2004). Attentional bias toward fear-related stimuli: an investigation with nonselected children and adults and children with anxiety disorders. J. Exp. Child Psychol. 89, 320–337. doi: 10.1016/j.jecp.2004.06.003
White, L. K., Britton, J. C., Sequeira, S., Ronkin, E. G., Chen, G., Bar-Haim, Y., et al. (2016). Behavioral and neural stability of attention bias to threat in healthy adolescents. Neuroimage 136, 84–93. doi: 10.1016/j.neuroimage.2016.04.058
Wilson, E., and MacLeod, C. (2003). Contrasting two accounts of anxiety-linked attentional bias: selective attention to varying levels of stimulus threat intensity. J. Abnorm. Psychol. 112, 212–218. doi: 10.1037/0021-843x.112.2.212
Worsley, K., and Friston, K. J. (1995). Analysis of fMRI time-series revisited—again. Neuroimage 2, 173–181. doi: 10.1006/nimg.1995.1023
Ye, J. C., Tak, S., Jang, K. E., Jung, J., and Jang, J. (2009). NIRS-SPM: statistical parametric mapping for near-infrared spectroscopy. Neuroimage 44, 428–447. doi: 10.1016/j.neuroimage.2008.08.036
Zhai, X., Santosa, H., and Huppert, T. J. (2020). Using anatomically defined regions-of-interest to adjust for head-size and probe alignment in functional near-infrared spectroscopy. Neurophotonics 7:035008. doi: 10.1117/1.NPh.7.3.035008
Keywords: attentional bias, eye gaze, functional near-infrared spectroscopy (fNIRS), threat type, attentional bias modification (ABM)
Citation: Liu H, Zhang Q, Elhai JD, Montag C and Yang H (2024) Attentional bias to threat is modulated by stimulus content: an fNIRS study. Front. Hum. Neurosci. 17:1308457. doi: 10.3389/fnhum.2023.1308457
Received: 06 October 2023; Accepted: 26 December 2023;
Published: 11 January 2024.
Edited by:
Tetsuo Kida, Institute for Developmental Research, JapanReviewed by:
Xuetong Zhai, University of Pittsburgh, United StatesCopyright © 2024 Liu, Zhang, Elhai, Montag and Yang. This is an open-access article distributed under the terms of the Creative Commons Attribution License (CC BY). The use, distribution or reproduction in other forums is permitted, provided the original author(s) and the copyright owner(s) are credited and that the original publication in this journal is cited, in accordance with accepted academic practice. No use, distribution or reproduction is permitted which does not comply with these terms.
*Correspondence: Haibo Yang, eWFuZ2hhaWJvQHRqbnUuZWR1LmNu
Disclaimer: All claims expressed in this article are solely those of the authors and do not necessarily represent those of their affiliated organizations, or those of the publisher, the editors and the reviewers. Any product that may be evaluated in this article or claim that may be made by its manufacturer is not guaranteed or endorsed by the publisher.
Research integrity at Frontiers
Learn more about the work of our research integrity team to safeguard the quality of each article we publish.