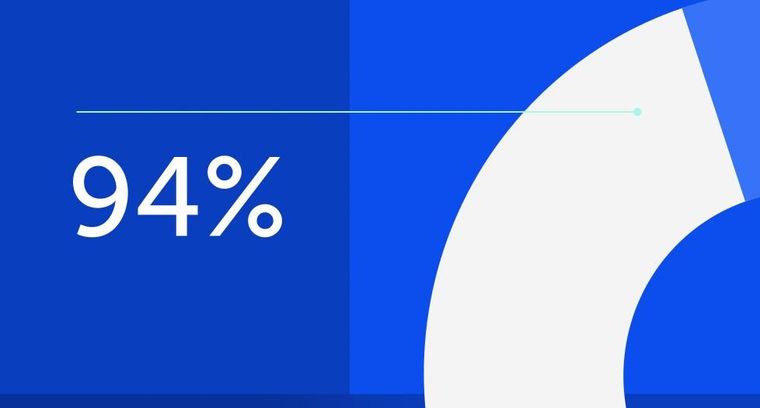
94% of researchers rate our articles as excellent or good
Learn more about the work of our research integrity team to safeguard the quality of each article we publish.
Find out more
OPINION article
Front. Hum. Neurosci., 24 October 2023
Sec. Brain-Computer Interfaces
Volume 17 - 2023 | https://doi.org/10.3389/fnhum.2023.1291983
This article is part of the Research TopicRising Stars in Brain-Computer Interfaces 2023View all 5 articles
Working memory (WM) is the cognitive system necessary for the temporary storage and manipulation of information during complex tasks like reasoning, comprehension, and learning (Baddeley, 1992, 2010). Studies have shown that the WM capacity improves in response to working memory training (WMT) through purposeful guidance and targeted cognitive training (Shipstead et al., 2012; Melby-Lervåg and Hulme, 2013; Finc et al., 2020). How to conduct effective WM training and evaluation is a crucial research topic. With the development of science and technology, the combination of brain-computer interface (BCI) and virtual reality (VR) technology, called BCI-VR, is an emerging technology with great potential for effective WMT. The BCI-VR system combines the immersive sensory feedback of VR with real-time electroencephalography (EEG) signals of brain activity, making cognitive training more engaging and efficient compared to traditional methods (Elbamby et al., 2018; Wen et al., 2021). In recent years, BCI-VR has been widely used in the field of rehabilitation medicine for a variety of diseases, including autism (Amaral et al., 2017), stroke (Lechner et al., 2014; Vourvopoulos and Bermúdez I Badia, 2016), attention deficit hyperactivity disorder (ADHD) (Rohani and Puthusserypady, 2015), Parkinson's disease (Morales-Gomez et al., 2018). However, the neuroimaging studies on BCI-VR in WM training and evaluation are still in its early stages, and more work needs to be done to ultimately effectively improve the WM capacity.
In this opinion article, we will analyze the relevant literature on current WM training and EEG signal analysis methods for WM evaluation, illustrate the value of BCI-VR and its application in WMT, and discuss the current challenges, as well as potential future directions. It is expected that these analyses will shed light on the field of WMT.
WM constitutes the core for executing various cognitive tasks, encompassing attention control, reasoning, and daily learning activities, underscoring its pivotal role in maintaining normal cognitive function. Existing research has highlighted that task-based WMT can progressively enhance WM capacity while also positively impacting broader cognitive skills and learning abilities (Vartanian et al., 2013; Gathercole et al., 2019; Sala et al., 2019). With an evolving focus on innovative WMT approaches, VR technology has been introduced into digital games. With its highly immersive environment and natural interaction, it has gradually become a research hotspot in the field of cognitive training. Notably, VR-based WMT has shown promise, with studies revealing its ability to significantly enhance sustained attention in real-life classroom environments (Coleman et al., 2019). Additionally, it has demonstrated efficacy in improving event-based prospective memory deficits in patients with major depressive disorder (MDD), suggesting its potential as a therapeutic intervention (Huang et al., 2022). The Nesplora Aquarium VR test, designed for assessing attention and WM in adults, has garnered attention for its robust psychometric properties and reliability, offering a valuable tool for evaluating attentional capacity in adults (Climent et al., 2021). Furthermore, investigations into the influence of valence and arousal on WM performance during VR gaming have yielded intriguing results, indicating that playing in VR led to improved WM performance, particularly in individuals with lower WM capacity (Gabana et al., 2017). This suggests a link between affective states and WM performance in VR gaming (Gabana et al., 2017). This convergence of research suggests the promising potential of VR-based WMT, offering an exciting avenue for advancing cognitive training paradigms beyond traditional methods.
BCI connects the brain to the external environment, allowing visualization of neural changes in the brain during cognitive activities through EEG signals. How to effectively conduct the EEG signal analysis to objectively describe the cognitive activities of the brain and accurately evaluate the effect of cognitive training is a crucial topic in WMT.
Studies have shown that analyzing EEG signals in specific frequency bands, can reflect changes in cognitive activities. For example, the features of EEG signals in the Alpha and Beta frequency bands, extracted through the power spectral density method, could reflect the changes in cognitive performance during noise exposure (Jafari et al., 2019). The spatial features of EEG signals in the Gamma frequency band, extracted through the co-space algorithm, could reflect the changes in different emotional performances (Li and Lu, 2009). Theta-band EEG signal fluctuations are associated with learning and memory activities (Herweg et al., 2020). As for the studies on WM, researchers investigated the neural mechanism of WM activity and examine neural changes associated with WM based on EEG signals. Herweg et al. have explored the performance of the brain when it achieves successful memory by studying the Theta-band EEG signal fluctuations, revealing that successful memory is related to both a broad-band tilt of the power spectrum and enhanced narrow-band Theta oscillations (Herweg et al., 2020). Additionally, studies have investigated the key role of “active-silent” neural states in WM using a perturbation method that can measure mnemonic hidden states in EEG, revealing that memory-item-specific information can be decoded from impulse responses, and that the accuracy of WM-guided behavior (including memory precision) could be predicted by the strength of hidden-state coding (Wolff et al., 2017).
It is also a significant scientific topic to study WM from the perspective of coupling relationships between cortical regions. Notably, it has been reported that mutual information can analyze the coupling relationship of multi-channel EEG signals. Guevara et al. (2018) reported significant differences in the mutual information of the Theta frequency band in cognitive activities. Li and Ouyang (2010) introduced a permutation conditional mutual information method to effectively estimate the directional coupling between neuronal populations that are mutually interconnected. Additionally, Buriro et al. (2018) utilized different types of inter-channel relationships in EEG as feature sets for continuous prediction of microsleep states. Toppi et al. (2018) discovered that different topological properties of EEG-derived networks, analyzed using graph-theoretic analysis, can reveal complex underlying WM phases, such as a higher small-world topology during the encoding phase of WM. More effective EEG signal analysis methods are needed for better evaluation of WMT.
Nowadays, EEG neurofeedback technology has been applied to WMT in many studies, and VR technology has also been initially introduced into WMT. However, the application of the integration of EEG and VR in WM training and evaluation remains limited and is in its early developmental stage. In addition, the methods used to evaluate the effects of WMT in current studies are still mainly limited to neuropsychological scale assessments, the accuracy of users' responses to cognitive tasks, and the subjective judgment of researchers. These methods may not be able to accurately evaluate the WM capacity, nor provide timely scientific evidence on whether the cognitive training process achieves effective enhancement in WM capacity. Hence, it is essential to integrate emerging technologies like VR with objective and quantitative evaluation methods like EEG signal analysis into WMT, to enhance the effectiveness of cognitive task training and evaluation.
VR technology can simulate immersive virtual reality scenes and provide users with visual, auditory, and other sensory feedback, which makes the cognitive training more attractive and engaging. EEG- can provide the direct interaction between brain and the external devices, which can intuitively and real-time display the neural changes in subjects' brain activity during training, and can effectively evaluate subjects' WM, which is much more objective and quantitative than traditional neuropsychological scale assessment (Elbamby et al., 2018; Wen et al., 2021). Compared with other BCI technologies like functional magnetic resonance imaging (fMRI), near-infrared spectroscopy (NIRS), and magnetoencephalography (MEG), EEG provides a more cost-effective, portable, and real-time method for monitoring brain activity. Therefore, EEG-based BCI-VR might be a promising way for WM training and evaluation.
In recent years, BCI-VR has been widely used in rehabilitation medicine for various diseases. Recent studies have explored the combination of EEG and electromyography (EMG) with VR for facial palsy and consciousness disorders rehabilitation, demonstrating improved outcomes (Qidwai et al., 2019; Maggio et al., 2020). A novel P300-based BCI paradigm utilizing VR and social cues shows potential for training joint-attention skills in autism (Amaral et al., 2017). Additionally, multimodal VR simulations combined with motor priming hold promise for stroke neurorehabilitation (Vourvopoulos and Bermúdez I Badia, 2016). A portable BCI-VR tool for ADHD has shown initial promise (Rohani and Puthusserypady, 2015). Besides, VR treatment has shown effective effects on Parkinson's disease (Morales-Gomez et al., 2018).
Research on BCI-VR in WM training and evaluation is still in its early stages, and a few recent studies have preliminarily investigated its effectiveness. One study adopted a quantitative approach, utilizing EEG signals and game performance data to assess cognitive levels (Wan et al., 2021). The findings revealed that VR games outperformed 3D counterparts in enhancing WM and attention, leading to notably shorter task completion times and faster attention peaks, underscoring the significant potential of VR for advanced cognitive training (Wan et al., 2021). Another investigation examined VR's impact on visual working memory, finding minor effects on accuracy and reaction time without statistical significance, and EEG data suggested perceptual-level cognitive changes over task performance alterations (Redlinger et al., 2022). Additionally, a study explored a cognitively adaptive VR training system incorporating real-time EEG to adjust task difficulty, revealing significant brain activity changes with increasing complexity while response times remained unchanged (Dey et al., 2019). Furthermore, a study explored the integration of VR headsets with EEG in cognitive tasks, confirming that VR head-mounted displays (HMDs) could successfully elicit ERP components similar to traditional computer screens, indicating their potential utility in early ERP investigations for cognitive research (Aksoy et al., 2021). These studies collectively demonstrate the evolving landscape of WMT, underscored by the promising prospects of BCI-VR, offering more immersive and quantifiable approaches to cognitive enhancement.
For the future direction of WMT, the combination of BCI and VR technology, which can provide immersive training environments to attract the subjects to focus on training, and collect EEG signals in real-time for cognitive training evaluation, is a promising option for conducting effective WMT. In WM training, more interaction ways, such as gestures or utilizing EEG signals, can replace the manual operation in traditional digital games to control virtual characters in VR games, thus enhancing the training experience and improving the effectiveness of WMT. In addition, in WM evaluation, in terms of EEG signal analysis, multiple feature extraction methods can be used for feature coupling analysis, thus further verifying the analysis from multiple perspectives. Moreover, for WM evaluation, relying solely on EEG signals and behavioral data may not provide a comprehensive assessment. Subsequently, NIRS, fMRI, and various physiological signals such as eye movement and electrocardiogram signals, can be fused to evaluate the WMT effect from multiple perspectives. Furthermore, for the BCI-VR system, it is important to further improve its software and hardware integration to make the device more portable and low-cost, and to make WM training and evaluation more efficient and accurate.
In conclusion, this study analyzed the current research status of WM training and evaluation, and emphasizing challenges and future directions related to BCI-VR technology. The integration of novel VR technology into WMT, known for its immersive environment and natural interaction, has gained prominence in cognitive training research. Additionally, the real-time visualization of neural changes through EEG signals positions BCI as a valuable tool for quantitatively and objectively assessing the effects of WMT. In consequence, our opinion is that the BCI-VR technology combined with EEG signal analysis is of great potential in WM training and evaluation, and can effectively help improve individual cognitive capabilities and performance. Currently, research in BCI-VR-based WMT is mainly in the experimental phase, but with technological advancements, it will hold substantial potential to benefit diverse populations across education, healthcare, mental health, aging, and sports domains, bearing significant socioeconomic value.
DD: Funding acquisition, Writing—original draft. ZW: Writing—original draft, Investigation. YZ: Investigation, Writing—original draft. XW: Investigation, Writing—original draft. DW: Funding acquisition, Methodology, Supervision, Writing—review and editing.
The author(s) declare financial support was received for the research, authorship, and/or publication of this article. This work was supported by the National Natural Science Foundation of China (62276022 and 82001908) and the China Postdoctoral Science Foundation (2021T140065 and 2020M680419).
The authors declare that the research was conducted in the absence of any commercial or financial relationships that could be construed as a potential conflict of interest.
All claims expressed in this article are solely those of the authors and do not necessarily represent those of their affiliated organizations, or those of the publisher, the editors and the reviewers. Any product that may be evaluated in this article, or claim that may be made by its manufacturer, is not guaranteed or endorsed by the publisher.
Aksoy, M., Ufodiama, C. E., Bateson, A. D., Martin, S., and Asghar, A. U. (2021). A comparative experimental study of visual brain event-related potentials to a working memory task: virtual reality head-mounted display versus a desktop computer screen. Exp. Brain Res. 239, 3007–3022. doi: 10.1007/s00221-021-06158-w
Amaral, C. P., Simões, M. A., Mouga, S., Andrade, J., and Castelo-Branco, M. (2017). A novel brain computer interface for classification of social joint attention in autism and comparison of 3 experimental setups: a feasibility study. J. Neurosci. Methods 290, 105–115. doi: 10.1016/j.jneumeth.2017.07.029
Buriro, A. B., Shoorangiz, R., Weddell, S. J., and Jones, R. D. (2018). Predicting microsleep states using EEG inter-channel relationships. IEEE Trans. Neural Syst. Rehabil. Eng. 26, 2260–2269. doi: 10.1109/TNSRE.2018.2878587
Climent, G., Rodríguez, C., García, T., Areces, D., Mejías, M., Aierbe, A., et al. (2021). New virtual reality tool (Nesplora Aquarium) for assessing attention and working memory in adults: a normative study. Appl. Neuropsychol. Adult 28, 403–415. doi: 10.1080/23279095.2019.1646745
Coleman, B., Marion, S., Rizzo, A., Turnbull, J., and Nolty, A. (2019). Virtual reality assessment of classroom–related attention: an ecologically relevant approach to evaluating the effectiveness of working memory training. Front. Psychol. 10, 1851. doi: 10.3389/fpsyg.2019.01851
Dey, A., Chatburn, A., and Billinghurst, M. (2019). “Exploration of an EEG-based cognitively adaptive training system in virtual reality,” in 2019 IEEE Conference on Virtual Reality and 3D User Interfaces (vr) (Osaka: IEEE), 220–226. doi: 10.1109/VR.2019.8797840
Elbamby, M. S., Perfecto, C., Bennis, M., and Doppler, K. (2018). Toward low-latency and ultra-reliable virtual reality. IEEE Netw. 32, 78–84. doi: 10.1109/MNET.2018.1700268
Finc, K., Bonna, K., He, X., Lydon-Staley, D. M., Kühn, S., Duch, W., et al. (2020). Dynamic reconfiguration of functional brain networks during working memory training. Nat. Commun. 11, 2435. doi: 10.1038/s41467-020-15631-z
Gabana, D., Tokarchuk, L., Hannon, E., and Gunes, H. (2017). “Effects of valence and arousal on working memory performance in virtual reality gaming,” in 2017 Seventh International Conference on Affective Computing and Intelligent Interaction (ACII) (San Antonio, TX: IEEE), 36–41. doi: 10.1109/ACII.2017.8273576
Gathercole, S. E., Dunning, D. L., Holmes, J., and Norris, D. (2019). Working memory training involves learning new skills. J. Mem. Lang. 105, 19–42. doi: 10.1016/j.jml.2018.10.003
Guevara, M. A., Paniagua, E. I. C., González, M. H., Carrillo, I. K. S., Sepúlveda, M. L. A., Orozco, J. C. H., et al. (2018). EEG activity during the spatial span task in young men: differences between short-term and working memory. Brain Res. 1683, 86–94. doi: 10.1016/j.brainres.2018.02.004
Herweg, N. A., Solomon, E. A., and Kahana, M. J. (2020). Theta oscillations in human memory. Trends Cogn. Sci. 24, 208–227. doi: 10.1016/j.tics.2019.12.006
Huang, D., Yan, S., Shen, S., Lv, S., Lai, S., Zhong, S., et al. (2022). Effects of virtual reality working memory training on event-based prospective memory in patients with major depressive disorder. J. Psychiatr. Res. 156, 91–99. doi: 10.1016/j.jpsychires.2022.09.049
Jafari, M. J., Khosrowabadi, R., Khodakarim, S., and Mohammadian, F. (2019). The effect of noise exposure on cognitive performance and brain activity patterns. Open Access Maced. J. Med. Sci. 7, 2924. doi: 10.3889/oamjms.2019.742
Lechner, A., Ortner, R., and Guger, C. (2014). “Feedback strategies for BCI based stroke rehabilitation: evaluation of different approaches,” in Replace, Repair, Restore, Relieve–Bridging Clinical and Engineering Solutions in Neurorehabilitation: Proceedings of the 2nd International Conference on NeuroRehabilitation (ICNR2014), Aalborg, 24-26 June, 2014 (Cham: Springer), 507–512. doi: 10.1007/978-3-319-08072-7_75
Li, M., and Lu, B.-L. (2009). “Emotion classification based on gamma-band EEG,” in 2009 Annual International Conference of the IEEE Engineering in Medicine and Biology Society (Minneapolis, MN: IEEE), 1223–1226. doi: 10.1109/IEMBS.2009.5334139
Li, X., and Ouyang, G. (2010). Estimating coupling direction between neuronal populations with permutation conditional mutual information. Neuroimage 52, 497–507. doi: 10.1016/j.neuroimage.2010.05.003
Maggio, M. G., Naro, A., La Rosa, G., Cambria, A., Lauria, P., Billeri, L., et al. (2020). Virtual reality based cognitive rehabilitation in minimally conscious state: a case report with EEG findings and systematic literature review. Brain Sci. 10, 414. doi: 10.3390/brainsci10070414
Melby-Lervåg, M., and Hulme, C. (2013). Is working memory training effective? A meta-analytic review. Dev. Psychol. 49, 270. doi: 10.1037/a0028228
Morales-Gomez, S., Elizagaray-Garcia, I., Yepes-Rojas, O., De La Puente-Ranea, L., and Gil-Martinez, A. (2018). Effectiveness of virtual immersion programmes in patients with Parkinson's disease. A systematic review. Rev. Neurol. 66, 69–80. doi: 10.33588/rn.6603.2017459
Qidwai, U., Ajimsha, M., and Shakir, M. (2019). The role of EEG and EMG combined virtual reality gaming system in facial palsy rehabilitation-a case report. J. Bodyw. Mov. Ther. 23, 425–431. doi: 10.1016/j.jbmt.2019.02.012
Redlinger, E., Glas, B., and Rong, Y. (2022). Impact of visual game-like features on cognitive performance in a virtual reality working memory task: within-subjects experiment. JMIR Serious Games 10, e35295. doi: 10.2196/35295
Rohani, D. A., and Puthusserypady, S. (2015). BCI inside a virtual reality classroom: a potential training tool for attention. EPJ Nonlinear Biomed. Phys. 3, 1–14. doi: 10.1140/epjnbp/s40366-015-0027-z
Sala, G., Aksayli, N. D., Tatlidil, K. S., Gondo, Y., and Gobet, F. (2019). Working memory training does not enhance older adults' cognitive skills: a comprehensive meta-analysis. Intelligence 77, 101386. doi: 10.1016/j.intell.2019.101386
Shipstead, Z., Redick, T. S., and Engle, R. W. (2012). Is working memory training effective? Psychol. Bull. 138, 628. doi: 10.1037/a0027473
Toppi, J., Astolfi, L., Risetti, M., Anzolin, A., Kober, S. E., Wood, G., et al. (2018). Different topological properties of EEG-derived networks describe working memory phases as revealed by graph theoretical analysis. Front. Hum. Neurosci. 11, 637. doi: 10.3389/fnhum.2017.00637
Vartanian, O., Jobidon, M.-E., Bouak, F., Nakashima, A., Smith, I., Lam, Q., et al. (2013). Working memory training is associated with lower prefrontal cortex activation in a divergent thinking task. Neuroscience 236, 186–194. doi: 10.1016/j.neuroscience.2012.12.060
Vourvopoulos, A., and Bermúdez I Badia, S. (2016). Motor priming in virtual reality can augment motor-imagery training efficacy in restorative brain-computer interaction: a within-subject analysis. J. Neuroeng. Rehabil. 13, 1–14. doi: 10.1186/s12984-016-0173-2
Wan, B., Wang, Q., Su, K., Dong, C., Song, W., Pang, M., et al. (2021). Measuring the impacts of virtual reality games on cognitive ability using EEG signals and game performance data. IEEE Access 9, 18326–18344. doi: 10.1109/ACCESS.2021.3053621
Wen, D., Fan, Y., Hsu, S.-H., Xu, J., Zhou, Y., Tao, J., et al. (2021). Combining brain–computer interface and virtual reality for rehabilitation in neurological diseases: a narrative review. Ann. Phys. Rehabil. Med. 64, 101404. doi: 10.1016/j.rehab.2020.03.015
Keywords: working memory evaluation, working memory training, brain-computer interface, virtual reality, electroencephalography
Citation: Duan D, Wu Z, Zhou Y, Wan X and Wen D (2023) Working memory training and evaluation based on brain-computer interface and virtual reality: our opinion. Front. Hum. Neurosci. 17:1291983. doi: 10.3389/fnhum.2023.1291983
Received: 10 September 2023; Accepted: 09 October 2023;
Published: 24 October 2023.
Edited by:
Mengfan Li, Hebei University of Technology, ChinaReviewed by:
Xulong Liu, Northeastern University, ChinaCopyright © 2023 Duan, Wu, Zhou, Wan and Wen. This is an open-access article distributed under the terms of the Creative Commons Attribution License (CC BY). The use, distribution or reproduction in other forums is permitted, provided the original author(s) and the copyright owner(s) are credited and that the original publication in this journal is cited, in accordance with accepted academic practice. No use, distribution or reproduction is permitted which does not comply with these terms.
*Correspondence: Dong Wen, YWl3ZDE2OEB1c3RiLmVkdS5jbg==
Disclaimer: All claims expressed in this article are solely those of the authors and do not necessarily represent those of their affiliated organizations, or those of the publisher, the editors and the reviewers. Any product that may be evaluated in this article or claim that may be made by its manufacturer is not guaranteed or endorsed by the publisher.
Research integrity at Frontiers
Learn more about the work of our research integrity team to safeguard the quality of each article we publish.