- 1Mechanical and Electrical Engineering College, Hainan University, Hainan, Haikou, China
- 2Centre for Autonomous Robotics (CENTAUR), Department of Electronic and Electrical Engineering, University of Bath, Bath, United Kingdom
- 3School of Automation Science and Engineering, South China University of Technology, Guangzhou, China
- 4Pazhou Lab, Guangzhou, China
- 5Department of Data Science and Big Data Technology, Hainan University, Haikou, China
- 6Brain-Computer Interfaces and Neural Engineering Laboratory, School of Computer Science and Electronic Engineering, University of Essex, Essex, United Kingdom
- 7MRC Brain Network Dynamics Unit, Nuffield Department of Clinical Neurosciences, University of Oxford, Oxford, United Kingdom
Editorial on the Research Topic
Explainable and advanced intelligent processing in the brain-machine interaction
Brain-machine interfaces (BMIs), also known as brain-computer interfaces (BCIs), allow their users to control external devices directly using brain signals without relying on the peripheral nervous system and muscles (Wolpaw et al., 2002). As a new bioengineering technology, BCI has a great potential in motor function enhancement to help patients disabled by diseases, such as stroke. In particular, BCI systems with interactive brain stimulation, such as deep brain stimulation (DBS), provide an active way to reveal relationships underlying the interplay between body control and brain activities, as well as to better understand pathological mechanisms underlying diseases such as Parkinson's disease (PD) and essential tremor (ET). While developing such advanced BCI systems, one of the major challenges is to apply explainable and advanced intelligent processes to decode the information embedded in brain signals such as EEG and functional Near-Infrared Spectroscopy (fNIRS) recorded non-invasively from scalp, or local field potential (LFP) recorded invasively from cortical (e.g., motor cortex) or subcortical (e.g., thalamus) brain structures (Heldman et al., 2006; Opri et al., 2020; He et al., 2021).
The goal of this Research Topic for the Brain-Computer Interface section of Frontiers in Human Neuroscience is to collect the current developments of explainable and advanced intelligent methods in BCIs based on EEG, fNIRS, or LFPs. With this aim, we collected five original research articles focusing on different aspects in developing an explainable BCI, including artifact rejection, feature extraction, classification using explainable algorithms, and hyper-parameter tuning.
Artifact removal is a common topic in the BCI community. In particular, it becomes more challenging to remove artifacts such as electrooculogram (EOG) when the number of recorded EEG signals is limited. To deal-with the over-complete issue while applying independent component analysis (ICA) to remove EOG artifacts from single-channel EEG recordings, Hu et al. proposed a method called DWT-CEEMDAN-ICA, in which a complete empirical mode decomposition method proposed by Torres et al. (2011) that can adapt to noise (CEEMDAN) is used to decompose the discrete wavelet transformation output of the raw EEG signals, ICA is then applied on the decomposed intrinsic mode functions (IMFs) to identify and remove EOG artifacts. This approach might be of interest to those dealing with EOG artifacts in EEG recordings, especially for those focusing on single-channel EEG-based BCIs, further evaluation of the effectiveness of this approach on bigger dataset is still needed though.
To improve the generalizability of motor imagery (MI)-based BCIs, Wang et al. proposed an EEG joint feature classification algorithm based on instance transfer and ensemble learning. In their method, spatial and frequency domain features are extracted through common spatial pattern (CSP, Ramoser et al., 2000) and power spectral density (PSD) analysis, then the MI classification is achieved using an ensemble learning algorithm based on kernel mean matching (KMM, Huang et al., 2007) and adaptive enhancement of transfer learning (TrAdaBoost, Dai et al., 2007). Experimental results using BCI Competition IV Dataset 2a and 2b showed that their method achieved higher decoding accuracies compared with some state-of-the-art methods, demonstrated its effectiveness. However, as the authors acknowledged, this method may not be applicable to other EEG data such as the P300 event-related potential.
Recently, researchers interested in BCI studies have paid great attention to deep leaning-based approaches, in particular, convolutional neural networks (CNN)-based methods. However, there tends to be a trade-off between the accuracy and the interpretability of the trained models, and in many BCI applications, the former is more important. Focusing on this trade-off, Shibu et al. proposed an explainable artificial intelligence (xAI) system that attempts to decompose the CNN model's output onto the input variables (i.e., channels) of fNIRS signals recorded during motor execution or motor imagery. Specially, a method called DeepShap is applied to compute the shapley values, which are further used to explain the model's output (Lundberg and Lee, 2017; Alsuradi et al., 2020). In contrast, Rodriguez et al. took another path and systematically compared the accuracy of decoding movement states and the interpretability between end-to-end CNN-based methods and feature-based methods, i.e., a support vector machine (SVM), using both simulated and real LFP data recorded from ET patients. The synthetic data consisted of 7 different oscillatory patterns including power changes in the beta and gamma bands, beta waveform sharpness, non-linear phase, theta-gamma phase amplitude coupling (PAC), cross-channel phase shifts, and beta burst length. The LFP data were recorded from the bilateral ventra intermediate (VIM) nucleus of the thalamus of ET patients while they perform self-paced upper limb movement tasks (He et al., 2021). The experimental results suggested that end-to-end deep leaning-based methods can yield superior performance, especially in cases where the underlying features of interest are not well-known or not easily captured by standardized feature extraction pipelines. These studies highlighted and made important contributions on the interpretability of CNN-based methods, which may be particularly interesting to those focusing on clinical applications of BCI such as adaptive deep brain stimulation (aDBS).
The performance and interpretability of a BCI system is both somehow determined and represented by a set of hyper-parameters optimized in the trained models. In the last paper collected in this Research Topic, Martineau et al. introduced a Bayesian optimization (BO)-based pipeline for automatic hyper-parameter tuning that is applicable through the entire decoding process, including feature extraction, channel selection, classification, and state-transition stages. The authors compared the proposed method with five other real-time feature extraction methods paired with four classifiers to decode voluntary movement asynchronously based on LFPs recorded with DBS electrodes implanted in the sub-thalamic nucleus (STN) of PD patients, and demonstrated the effectiveness of the proposed pipeline. This study provides a promising solution to deal with the challenges surrounding hyper-parameter tuning while developing BCI systems.
We believe explainable and advanced intelligent processing is an important perspective while developing BCI systems, which can help to improve the performance and interpretability of the developed systems, and will further facilitate the understanding of disease mechanisms (Figure 1). In collating a few good examples of this, we hope this special topic can act as a resource for those interested in this topic, trigger further discussion, and eventually push forward development in this area.
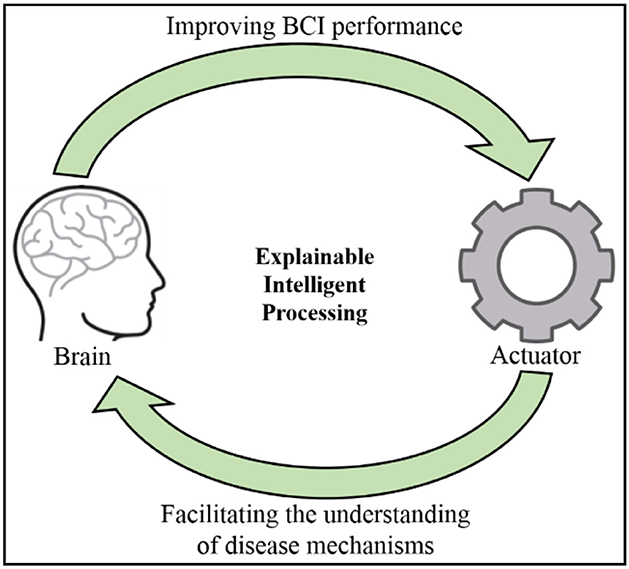
Figure 1. Improving brain-computer interface (BCI) performance and facilitating the understanding of disease mechanisms using explainable intelligent algorithms.
Author contributions
XX: Conceptualization, Project administration, Writing—review and editing. DZ: Writing—review and editing. TY: Writing—review and editing. YD: Writing—review and editing. ID: Writing—review and editing. SH: Conceptualization, Visualization, Writing—original draft, Writing—review and editing.
Funding
SH was supported by the Guarantors of Brain (HMR04170) and the Royal Society (IES\R3\213123).
Conflict of interest
The authors declare that the research was conducted in the absence of any commercial or financial relationships that could be construed as a potential conflict of interest.
The author(s) declared that they were an editorial board member of Frontiers, at the time of submission. This had no impact on the peer review process and the final decision.
Publisher's note
All claims expressed in this article are solely those of the authors and do not necessarily represent those of their affiliated organizations, or those of the publisher, the editors and the reviewers. Any product that may be evaluated in this article, or claim that may be made by its manufacturer, is not guaranteed or endorsed by the publisher.
References
Alsuradi, H., Park, W., and Eid, M. (2020). “Explainable classification of EEG data for an active touch task using shapley values,” in HCI International 2020 – Late Breaking Papers: Multimodality and Intelligence 6, eds C. Stephanidis, M. Kurosu, H. Degen and L. Reinerman-Jones (Cham: Springer International Publishing) 406–416. doi: 10.1007/978-3-030-60117-1_30
Dai, W., Qiang, Y., Xue, G., and Yong, Y. (2007). “Boosting for transfer learning,” in Proceedings of the 24th International Conference on Machine Learning (Corvalis. OR) 20–24. doi: 10.1145/1273496.1273521
He, S., Baig, F., Mostofi, A., Pogosyan, A., Debarros, J., Green, A., et al. (2021). Closed-loop deep brain stimulation for essential tremor based on thalamic local field potentials. Mov. Disord. 36, 863–873. doi: 10.1002/mds.28513
Heldman, D. A., Wang, W., Chan, S. S., and Moran, D. W. (2006). Local field potential spectral tuning in motor cortex during reaching. IEEE Trans. Neur. Syst. Rehab. Eng. 14, 180–183. doi: 10.1109/TNSRE.2006.875549
Huang, J., Smola, A., Gretton, A., Borgwardt, K., and Schõlkopf, B. (2007). “Correcting sample selection bias by unlabeled data,” in Advances in Neural Information Processing Systems. doi: 10.7551/mitpress/7503.003.0080
Lundberg, S. M., and Lee, S. I. (2017). A unified approach to interpreting model predictions. Adv. Neural Inform. Process. Syst. 30, 4765–4774. doi: 10.48550/arXiv.1705.07874
Opri, E., Cernera, S., Molina, R., Eisinger, R. S., Cagle, J. N., Almeida, L., et al. (2020). Chronic embedded cortico-thalamic closed-loop deep brain stimulation for the treatment of essential tremor. Sci. Transl. Med. 12, eaay7680. doi: 10.1126/scitranslmed.aay7680
Ramoser, H., Muller-Gerking, J., and Pfurtscheller, G. (2000). Optimal spatial filtering of single trial EEG during imagined hand movement. IEEE Trans. Rehabil. Eng. 8, 441–446. doi: 10.1109/86.895946
Torres, M. E., Colominas, M. A., Schlotthauer, G., and Flandrin, P. (2011). “A complete ensemble empirical mode decomposition with adaptive noise,” in 2011 IEEE International Conference on Acoustics, Speech and Signal Processing (ICASSP) (IEEE) 4144–4147. doi: 10.1109/ICASSP.2011.5947265
Keywords: interpretability, brain machine interface, brain computer interface, machine learning, EEG, fNIRS, deep brain stimulation
Citation: Xie X, Zhang D, Yu T, Duan Y, Daly I and He S (2023) Editorial: Explainable and advanced intelligent processing in the brain-machine interaction. Front. Hum. Neurosci. 17:1280281. doi: 10.3389/fnhum.2023.1280281
Received: 19 August 2023; Accepted: 29 August 2023;
Published: 12 September 2023.
Edited and reviewed by: Gernot R. Müller-Putz, Graz University of Technology, Austria
Copyright © 2023 Xie, Zhang, Yu, Duan, Daly and He. This is an open-access article distributed under the terms of the Creative Commons Attribution License (CC BY). The use, distribution or reproduction in other forums is permitted, provided the original author(s) and the copyright owner(s) are credited and that the original publication in this journal is cited, in accordance with accepted academic practice. No use, distribution or reproduction is permitted which does not comply with these terms.
*Correspondence: Shenghong He, c2hlbmdob25nLmhlJiN4MDAwNDA7bmRjbi5veC5hYy51aw==