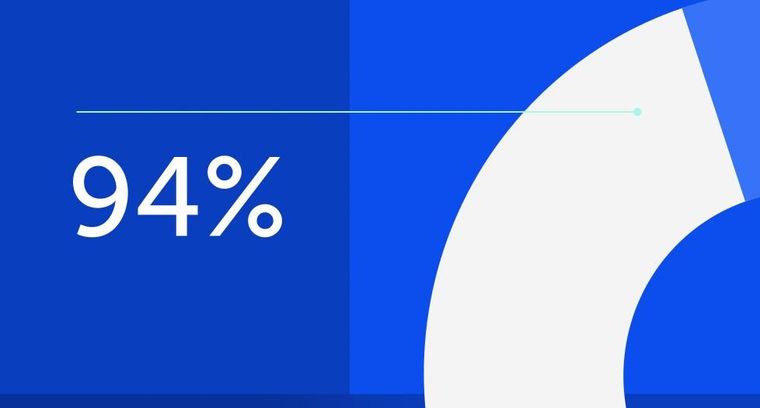
94% of researchers rate our articles as excellent or good
Learn more about the work of our research integrity team to safeguard the quality of each article we publish.
Find out more
SYSTEMATIC REVIEW article
Front. Hum. Neurosci., 04 August 2023
Sec. Brain Health and Clinical Neuroscience
Volume 17 - 2023 | https://doi.org/10.3389/fnhum.2023.1239374
This article is part of the Research TopicAdvances in Artificial Intelligence, Robotics, Augmented and Virtual Reality in NeurosurgeryView all 12 articles
Background: Autonomous navigation of catheters and guidewires in endovascular interventional surgery can decrease operation times, improve decision-making during surgery, and reduce operator radiation exposure while increasing access to treatment.
Objective: To determine from recent literature, through a systematic review, the impact, challenges, and opportunities artificial intelligence (AI) has for the autonomous navigation of catheters and guidewires for endovascular interventions.
Methods: PubMed and IEEEXplore databases were searched to identify reports of AI applied to autonomous navigation methods in endovascular interventional surgery. Eligibility criteria included studies investigating the use of AI in enabling the autonomous navigation of catheters/guidewires in endovascular interventions. Following Preferred Reporting Items for Systematic Reviews and Meta-Analysis (PRISMA), articles were assessed using Quality Assessment of Diagnostic Accuracy Studies 2 (QUADAS-2). PROSPERO: CRD42023392259.
Results: Four hundred and sixty-two studies fulfilled the search criteria, of which 14 studies were included for analysis. Reinforcement learning (RL) (9/14, 64%) and learning from expert demonstration (7/14, 50%) were used as data-driven models for autonomous navigation. These studies evaluated models on physical phantoms (10/14, 71%) and in-silico (4/14, 29%) models. Experiments within or around the blood vessels of the heart were reported by the majority of studies (10/14, 71%), while non-anatomical vessel platforms “idealized” for simple navigation were used in three studies (3/14, 21%), and the porcine liver venous system in one study. We observed that risk of bias and poor generalizability were present across studies. No procedures were performed on patients in any of the studies reviewed. Moreover, all studies were limited due to the lack of patient selection criteria, reference standards, and reproducibility, which resulted in a low level of evidence for clinical translation.
Conclusion: Despite the potential benefits of AI applied to autonomous navigation of endovascular interventions, the field is in an experimental proof-of-concept stage, with a technology readiness level of 3. We highlight that reference standards with well-identified performance metrics are crucial to allow for comparisons of data-driven algorithms proposed in the years to come.
Systematic review registration: identifier: CRD42023392259.
Cardiovascular (CV) diseases are the most common cause of death across Europe, accounting for more than four million deaths each year, with coronary heart disease (44.2%) and cerebrovascular disease (25.4%) emerging as the predominant contributors to CV-related mortality across all ages and genders (Townsend et al., 2016). Endovascular catheter-based interventions such as percutaneous coronary intervention (PCI), pulmonary vein isolation (PVI), and mechanical thrombectomy (MT) have become an established treatment for CV diseases (Thukkani and Kinlay, 2015; Goyal et al., 2016; Giacoppo et al., 2017; Lindgren et al., 2018). During such a procedure, an operator navigates a guidewire and catheter from an insertion point (typically the common femoral or radial artery) to the area of interest to perform the intervention. Intraoperative fluoroscopy is used intermittently throughout the navigation and intervention to guide the catheter and guidewire through the vasculature. Once the target site has been reached, the treatment can be performed through the catheter. This is typically thrombus removal in the case of MT, stent deployment in the case of PCI, and ablation for PVI (Brilakis, 2020).
In acute CV disease, time from symptom onset to treatment is often crucial for effective endovascular interventions. For example, the benefits of MT become non-significant after 7.3 h of stroke for non-stratified patients (Saver et al., 2016). As a result, in the UK for example, only 1.4% of stroke admissions benefit from MT despite the 10% of patients that are eligible for treatment (McMeekin et al., 2017). Other challenges for endovascular interventions relate to occasional complications including perforation, thrombosis, and dissection in the parent artery, as well as distal embolization of thrombus (Hausegger et al., 2001). Moreover, angiography requires intravascular contrast agent administration, which can occasionally lead to nephrotoxicity (Rudnick et al., 1995). For operators and their teams, the high cumulative dose of x-ray radiation from angiography is a risk factor for cancer and cataracts (Klein et al., 2009). Although exposure can be minimized with current radiation protection practice, some measures involve operators wearing heavy protective equipment which is a risk factor for orthopedic complications, and so alternative methods of exposure reduction are beneficial (Ho et al., 2007; Madder et al., 2017).
It is hoped that robotic surgical systems can either mitigate or eliminate some of the challenges currently presented by endovascular interventions. For example, robotic systems could be set up in hospitals nationwide and tele-operated remotely from a central location, increasing the speed of access to treatments such as MT beyond what is possible currently (Crinnion et al., 2022). Additionally, robotic systems might eliminate any operator physiological tremors or fatigue and allow endovascular interventions to be performed in an optimum ergonomic position while potentially increasing procedural precision (for example, procedure time), and thereby improving overall performance scores and reducing complication rates (Riga et al., 2010). Furthermore, as operators would not be required to stand next to the patient, their radiation exposure would be reduced and the need to wear heavy protective equipment would be obviated.
Commercial robotic systems are currently available to perform endovascular interventions. Hansen Medical developed the MagellanTM system (Auris Health, Redwood City, USA), the first commercially available robotic system to be used for PVI, and more recently used to successfully perform carotid artery stenting in 13 patients (Duran et al., 2014; Jones et al., 2021). This system comprises a steerable guide catheter inside a steerable sheath allowing movement in three dimensions, and a separate remote guidewire manipulator allowing linear and rotational movement. The Corpath GRX® (Corindus Vascular Robotics, USA), the next-generation system of the Corpath® 200 robot, has successfully been used for PCI and PVI. This system has performed diagnostic cerebral angiography procedures and ten carotid artery stenting procedures (Nogueira et al., 2020; Sajja et al., 2020; Weinberg et al., 2021). Furthermore, it has been recently used to perform robot-assisted, neuroendovascular interventions including aneurysm embolization and epistaxis embolization (Pereira et al., 2020; Cancelliere et al., 2022; Saber et al., 2022). These systems use a controller-operator structure, where operators remotely control and navigate a robot through a patient's vasculature to the target site. In currently available systems, the operator has complete control over the robot and makes all of the decisions.
While these robotic systems help alleviate some of the challenges of endovascular interventions, they have limitations. The controller-operator structure requires a reasonably high cognitive workload, can still result in human error and means that the procedure is limited to an individual operator's skill set (Mofatteh, 2021). These robotic systems also consist of user interfaces such as buttons and joysticks, requiring skills that are different to those used in current clinical practice. Additionally, a lack of haptic feedback from robotic systems might result in difficulties to receive tactile feedback from the catheters and guidewires as they interact with vessel walls (Crinnion et al., 2022).
One emerging method of mitigating these challenges is using artificial intelligence (AI) techniques in conjunction with robotic systems. AI, and in particular, machine learning (ML), has accelerated in recent years in its applications for data analysis and learning (Sarker, 2021), with many areas of healthcare already making use of this technology for disease prediction and diagnosis (Fatima and Pasha, 2017; Silahtaroğlu and Yılmaztürk, 2021). ML algorithms can be divided into three main groups: supervised, unsupervised, and reinforcement learning (RL). Supervised learning is the most common form of ML and involves constructing a model trained on a dataset with labels (the corresponding correct outputs). The model can then accurately predict the labels of new, unknown instances based on the patterns learned from the training data (Kotsiantis, 2007).
Unsupervised learning involves training an algorithm to represent particular input features in a way that reflects the structure of the overall collection of input patterns (Dayan, 2017). In contrast to other types of ML, the dataset is unlabeled and there are no explicit target outputs or environmental evaluations associated with each input.
RL is a form of ML, whereby an agent learns by interacting with the environment and receiving feedback in the form of rewards. The goal of RL is to maximize the cumulative reward over time by learning a policy that optimizes the agent's current state for a set of actions (Arulkumaran et al., 2017). Similar to the natural way of human learning, robotic RL automatically acquires the skills through “trials and errors” (Sutton and Barto, 2018). Applications of RL are becoming more expansive, as numerous research areas aim to use the method, for example, in precision medicine, medical imaging, and rehabilitation (Lowery and Faisal, 2013; Naros and Gharabaghi, 2015; Ghesu et al., 2018).
Learning from demonstration (LfD) is a variant of supervised learning, where input data is provided by an expert demonstrator. This can also act as a precursor for RL, whereby the agent can further improve its behavior through interaction with the environment. Table 1 describes the ML methods that are referred to later in this paper, each of which can be used to improve performance across the three types of ML described above. LfD has been separated from the other types of ML in this case, as it can be used in the context of both supervised learning and RL.
The use of these ML techniques for autonomy in medical robotics presents several challenges. To help in the consideration of regulatory, ethical, and legal barriers imposed, a six-level autonomy framework has been proposed, ranging from no autonomy at level 0, up to level 5 which involves full autonomy with no human intervention (Yang et al., 2017). This study aims to systematically review the methodology, performance and autonomy level of AI applied to the autonomous navigation of catheters and guidewires for endovascular interventions. Understanding the current developments in the field will help determine the impact, challenges, and opportunities required to direct future translational research and ultimately guide clinical practice.
This systematic review is PROSPERO (International prospective register of systematic reviews) registered (CRD42023392259). The review followed Preferred Reporting Items for Systematic Reviews and Meta-Analyses (PRISMA) guidelines (Page et al., 2021).
Included reports consisted of primary research studies, which investigated the use of AI in enabling the autonomous navigation of catheters and/or guidewires in endovascular interventions. Excluded studies did not use AI methods to achieve autonomous navigation of catheters/guidewires or looked at path planning for endovascular interventions rather than the navigation itself. Additionally, studies without an English translation were not included (Nussbaumer-Streit et al., 2020).
PubMed and IEEEXplore were used to capture original research articles, published anytime until the end of January 2023, with the following search query: “(Artificial Intelligence OR Machine Learning OR Reinforcement Learning OR Deep Learning OR Autonomous OR Learning-based) AND (Endovascular OR Vascular Intervention OR Catheter OR Guidewire) AND (Navigation OR Guidance).” Pre-prints and non-peer-reviewed articles were excluded.
A medical robotics data scientist, H.R. (3 years of research experience), searched for studies as defined in the search strategy and followed the selection process as shown in Figure 1. A medical robotics data scientist, L.K. (4 years experience in autonomous endovascular navigation using AI), independently reviewed the manuscripts against the eligibility criteria. In the case of discrepancy, consensus was reached by discussion between the two reviewers. If consensus was not reached, the multi-disciplinary authorship would make the final arbitration. The relevant data items, as defined in the following section, were extracted.
Figure 1. Preferred Reporting Items for Systematic Reviews and Meta-Analyses (PRISMA) flow diagram showing the number of articles searched and excluded at each stage of the literature search after screening titles, abstracts, and full texts.
Information extracted from each study included: the AI method used and more granular model details (where available), the current level of autonomy, the type of experiment (in vivo, in vitro, in silico), the method of tracking the catheter and/or guidewire position, the method of catheter and/or guidewire manipulation, description of the navigation path, performance measures, and key performance outcomes (where available).
The levels of autonomy followed (Yang et al., 2017). Briefly, these are level 0: no autonomy, level 1: robot assistance, level 2: task autonomy, level 3: conditional autonomy, level 4: high autonomy, and level 5: full autonomy. It should be noted that if the autonomy level was not described in the study, an appropriate level was assigned based on the content of the paper.
Where appropriate, both Quality Assessment of Diagnostic Accuracy Studies 2 (QUADAS-2) methodology alongside AI metrics from the Checklist for Artificial Intelligence in Medical Imaging (CLAIM) were used to assess the risk of bias for each study (Rutjes et al., 2011; Mongan et al., 2020).
As shown in Figure 1, 462 studies met the search criteria, and 21 full-text studies were assessed against the eligibility criteria. A total of 14 were identified for review (Rafii-Tari et al., 2013, 2014; Chi et al., 2018a,b, 2020; Behr et al., 2019; You et al., 2019; Zhao et al., 2019; Kweon et al., 2021; Meng et al., 2021, 2022; Cho et al., 2022; Karstensen et al., 2022; Wang et al., 2022). The characteristics of the fourteen studies are listed in Table 2.
Table 2. Studies resulting from our search and eligibility criteria proposing AI models for the autonomous navigation of catheters/guidewires in endovascular interventions.
According to QUADAS-2 methodology, all studies reviewed gave a high or unclear “risk of bias” and “concerns regarding applicability” in all domains. No studies performed procedures on patients and therefore had no clearly defined patient selection criteria, reference standards, or index tests. Despite the low level of evidence, there is value in discussing these individual studies as they represent the current state of the art and form a baseline for further research.
RL was used in nine studies (9/14, 64%) with algorithms including A3C, DDPG, DQN, Dueling DQN, HER, PI2, PPO, and Rainbow (Chi et al., 2018a, 2020; Behr et al., 2019; You et al., 2019; Kweon et al., 2021; Meng et al., 2021, 2022; Cho et al., 2022; Karstensen et al., 2022). Demonstrator data in some form (GAIL, Behavior Cloning, or HD) was used as a precursor in four of the studies (4/14, 29%) during training (LfD), in conjunction with other RL algorithms (Chi et al., 2018a; Behr et al., 2019; Kweon et al., 2021; Cho et al., 2022). The SOFA framework (Inria, Strasbourg, France; Faure et al., 2012) was used for training RL models in four studies (4/14, 29%; Behr et al., 2019; Cho et al., 2022; Karstensen et al., 2022; Meng et al., 2022), the Unity engine (Unity Technologies, San Francisco, USA) was used in two studies (2/14, 14%; You et al., 2019; Meng et al., 2021), while the platform used for training was not specified in three studies (3/14, 21%; Chi et al., 2018a, 2020; Kweon et al., 2021).
RL was not used in five studies (5/14, 36%) which employed LfD (not as a precursor for RL), unsupervised (GMM and HMM) and supervised (CNN and YOLO) methods alone or in combination (Rafii-Tari et al., 2013, 2014; Chi et al., 2018b; Zhao et al., 2019; Wang et al., 2022). The most common method was LfD, which was used in three studies (3/14, 21%; Rafii-Tari et al., 2013, 2014; Chi et al., 2018b). Two of these studies (2/14, 14%) used a GMM to generate the probabilistic representation of the dataset provided by a demonstrator (Rafii-Tari et al., 2013; Chi et al., 2018b), while the other study utilized HMMs to model each movement primitive (Rafii-Tari et al., 2014). The other two non-RL studies (2/14, 14%) used solely CNNs or YOLOV5s (Zhao et al., 2019; Wang et al., 2022).
Conditional autonomy (level 3) was performed in seven studies (7/14, 50%; Behr et al., 2019; You et al., 2019; Zhao et al., 2019; Kweon et al., 2021; Cho et al., 2022; Karstensen et al., 2022; Wang et al., 2022). Here, a target in the vasculature is selected by an operator and the subsequent navigation to the target of the guidewire and/or catheter takes place autonomously. Task autonomy (level 2) was performed across five studies (5/14, 36%), whereby the robotic driver automates the catheter motion and an operator manipulates the guidewire for assistance (Rafii-Tari et al., 2013, 2014; Chi et al., 2018a,b, 2020). Robot assistance (level 1) was demonstrated in two studies (2/14, 14%), where experiments were performed entirely in simulation and under continuous supervision of an operator (Meng et al., 2021, 2022).
Clinical trials were not performed in any of the studies reviewed. Physical phantoms were used in the majority of studies (11/14, 79%) reviewed (Rafii-Tari et al., 2013, 2014; Chi et al., 2018a,b, 2020; Behr et al., 2019; You et al., 2019; Zhao et al., 2019; Kweon et al., 2021; Cho et al., 2022; Wang et al., 2022). Of these studies, seven used 3D vascular phantoms (Rafii-Tari et al., 2013, 2014; Chi et al., 2018a,b, 2020; You et al., 2019; Wang et al., 2022), three used 2D phantoms (Behr et al., 2019; Zhao et al., 2019; Cho et al., 2022), and one study used both 2D and 3D phantoms (Kweon et al., 2021). Commercial phantoms were used in six studies (6/14, 43%): 3D silicone-based, transparent, anthropomorphic phantoms (Elastrat Sarl, Geneva, Switzerland) were used in 5/14 (36%) studies (Rafii-Tari et al., 2013, 2014; Chi et al., 2018a,b, 2020); and the study using both 2D and 3D phantoms used firstly, a 2D PCI trainer for beginners (Medi Alpha Co., Ltd., Tokyo, Japan) and secondly, a silicone 3D Embedded Coronary Model (Trandomed 3D Medical Technology Co., Ltd., Ningbo, China), respectively. Five studies (5/14, 36%) appeared to use in-house phantoms: one study used a silicone-based 3D printed heart model and inferior vena cava (You et al., 2019), and one used a 10 mm vessel diameter phantom made of polymethyl methacrylate (PMMA; Behr et al., 2019), while sufficient phantom detail is not provided by the other three studies (Zhao et al., 2019; Cho et al., 2022; Wang et al., 2022).
In silico methods were used in two of the studies (2/14, 14%; one used SOFA framework and one used Unity engine; Meng et al., 2021, 2022). Ex vivo experiments using porcine liver vasculature were reported by one study (Karstensen et al., 2022). Here, in silico methods were used for training models before the ex vivo experiments.
Figure 2 shows the anatomical regions where each study focuses. Experiments within or around the blood vessels of the heart were reported by the majority of studies (10/14, 71%; Rafii-Tari et al., 2013, 2014; Chi et al., 2018a,b, 2020; You et al., 2019; Kweon et al., 2021; Meng et al., 2021, 2022; Wang et al., 2022), with the study with the longest path length starting at the femoral artery and finishing at the coronary artery (Wang et al., 2022). Non-anatomical vessel platforms “idealized” for simple navigation were used in three studies (3/14, 21%; Behr et al., 2019; Zhao et al., 2019; Cho et al., 2022), and the porcine liver venous system in one study (Karstensen et al., 2022).
Figure 2. Diagram depicting the general vessels of interest for each study. *Study is in more than one area. Studies using non-anatomical platforms are also shown.
Passive tracking relies on external sensors to detect the catheter's position, active tracking involves the use of sensors located at the distal end of the catheter for real-time position tracking, and magnetic tracking utilizes external magnetic fields to guide the catheter's movement and track its position. A passive, tracking-based, method for catheter manipulation was used in eight studies (8/14, 57%; Rafii-Tari et al., 2013, 2014; Chi et al., 2018a,b, 2020; You et al., 2019; Meng et al., 2021, 2022), whereas a passive, image-based, method for catheter manipulation was used in the other six studies (6/14, 43%; Behr et al., 2019; Zhao et al., 2019; Kweon et al., 2021; Cho et al., 2022; Karstensen et al., 2022; Wang et al., 2022). None of the studies reviewed reported active or magnetic steering methods.
A top-down camera for tracking the location of the guidewire and/or catheter was implemented in five of the studies (5/14, 36%) where transparent phantoms allowed real-time video to provide software-generated tracking data (Behr et al., 2019; Zhao et al., 2019; Kweon et al., 2021; Cho et al., 2022; Wang et al., 2022). Electromagnetic (EM) position sensors were employed in six studies (6/14, 43%; Rafii-Tari et al., 2013, 2014; Chi et al., 2018a,b, 2020; You et al., 2019). An Aurora control unit and EM Generator of Aurora electromagnetic tracking system (NDI, Waterloo, Canada) were used in one of these studies (You et al., 2019), whilst custom-designed sensors (Rafii-Tari et al., 2013) were used in the other five. These five studies also employed a top-down camera simultaneously enabled through the use of transparent phantoms during data collection pre-training. One study employed continuous fluoroscopy, capturing 7.5 images per second, and used a CNN to segment the guidewire from real-time fluoroscopy images to track data that included the coordinates (Karstensen et al., 2022). Two studies (2/14, 14%) were performed entirely in silico, and hence no tracking method was required (Meng et al., 2021, 2022).
Quantitative performance measures used in the studies were heterogeneous which may reflect the low technology readiness level (TRL; Mankins, 1995) of AI applied to autonomous navigation of endovascular interventions shown by the studies in this systematic review. Common performance measures used were success rate of navigation task (7/14, 50%) and time to complete procedure (5/14, 36%). Other performance measures shared across studies were: measures of force (6/14, 43%); acceleration (4/14, 29%); various measures of speed (4/14, 29%); and path length (4/14, 29%). Half of the studies (7/14, 50%) reviewed compared manual performance against their autonomous navigation performance. The key performance outcomes of the 14 studies are listed in Table 3.
Where possible, critical outcome data for success rate, procedure time and path length were extracted from the study. Three of the 14 studies (3/14, 21%) did not measure any of these performance measures (Rafii-Tari et al., 2013; Meng et al., 2021; Wang et al., 2022). Of the seven studies (7/14, 50%) that measured success rate, the value was over 90% in four studies (4/14, 29%; Chi et al., 2018a, 2020; Zhao et al., 2019; Kweon et al., 2021).
There is no high-level evidence (Howick et al., 2011) to demonstrate that AI autonomous navigation of catheters and guidewires in endovascular intervention is non-inferior or superior to manual procedures. Currently, AI autonomous navigation of catheters and guidewires in endovascular intervention has not surpassed TRL 3. There has been no clinical validation nor has there been comprehensive laboratory validation. Over half of the studies (9/14, 64%) employed RL methodologies, particularly in recent years, where most studies used RL (8/10, 80% published beyond 2018). There are no standardized in silico, in vitro, or ex vivo experimental reference standard designs, nor are there standardized performance measures, meaning comparison of studies quantitatively is of limited value.
The primary strength of the studies reviewed came from the range of ML techniques employed. Most focused on finding a ML technique that would improve upon previous work, rather than using similar algorithms and extending the experimental environment. This is demonstrated well within the nine studies (9/14, 64%) which used RL, where a different ML-based methodology was used in every case except for two (where the simulation environment and output measurements were changed between studies). Exploring various techniques is advantageous for research, especially in the rapidly evolving field of ML, as the fast pace of development increases the likelihood that more effective algorithms are created. For example, autonomous endovascular intervention progress has been catalyzed by combining two recent approaches (LfD and RL; Chi et al., 2018a, 2020; Kweon et al., 2021; Cho et al., 2022). Here, using demonstrator data in a third of the RL studies allowed expert operator skill in complex endovascular procedures to be incorporated. This proficiency can be leveraged effectively to accelerate the RL training process. The combined approach, therefore, shortens the transition from a simulated training environment to a physical testing environment which typically presents significant challenges, as evidenced by the findings of Karstensen et al. (2022). Another benefit of accelerating the process is that in some scenarios thousands of mechanical experimental training cycles may no longer be required leading to reduced mechanical wear on the experimental equipment.
The limitations of the studies assessed encompassed three areas:
(1) Whilst it was a strength that most studies focused on finding a ML technique that would improve upon previous endovascular navigation, the lack of focus on using similar or fixed algorithms and extending the experimental environment was a limitation. The challenge of fixing many experimental variables whilst changing another, is compounded by the lack of standardized in silico, in vitro or ex vivo experimental reference standard designs for endovascular navigation, as well as a lack of standardized performance measures. As such, the ability to compare studies quantitatively was limited by confounding. For example, although some performance measures (e.g., “success rate” and “procedure time”) were common to several studies, study comparison was limited due to experimental variations between studies. Firstly, the navigation path used to test the models varied. Secondly, some studies defined “success rate” only if a task was completed within a certain time frame, whereas others had no time limit for completion. Thirdly, “procedure time” was measured using different starting points and target sites.
(2) Another limitation, also concerned with reference standards, is the importance of comparing the endovascular navigation with an autonomous system against the endovascular navigation without an autonomous system, to determine any incremental benefit through autonomy. Critically, the endovascular navigation without an autonomous system should ideally be operated by a relevant expert operating with minimal technical constraint to derive the reference standard (baseline) allowing comparison. Half the studies (7/14, 50%) reviewed did compare endovascular navigation with and without an autonomous system; however, in some cases, the operator was technically constrained by using a novel robotic system rather than using the equipment used and processes they would typically employ, during an endovascular procedure in the clinic. For instance, the reviewed robotic systems failed to replicate crucial haptic feedback experienced during manual procedures. These include viscous forces between catheters and blood, friction forces between catheters and the vessel wall, impact forces from catheter tips and guidewire, and contact with the vessel wall (Crinnion et al., 2022). Additionally, an expert is not able to use their previous experience with standard equipment and may be unfamiliar with these controls, meaning that performance at a given task will likely be affected.
(3) There were no clinical studies of autonomous endovascular navigation which is a reflection of the nascent field and current TRL of the technology. The majority of studies (11/14, 79%) were in vitro and are valuable for development and testing as they limit the number of failures during subsequent in vivo testing (Ionita et al., 2014). However, these studies did not consider whether construct, face, and context validity of endovascular navigation systems was acceptable to allow TRL progression toward the clinic. In particular, in many of the studies reviewed, there were translational concerns regarding how the guidewires and/or catheters are tracked within the vasculature, as the alternative to using fluoroscopy with standard off-the-shelf catheters and guidewires is to create entirely new tracking methods. For example, several papers (6/14, 43%) used EM-tracking to visualize the catheter in real-time, which has been shown to allow better real-time 3D orientation, facilitating navigation, reducing cannulation and total fluoroscopy times, and improving motion consistency and efficiency (Schwein et al., 2017). However, clinical translation using this method would require the introduction of new systems with specialized catheters and guidewires, resulting in additional costs and training. Furthermore, other studies (5/14, 36%) employed an experimental set-up involving a tabletop with a transparent phantom and a top-down camera. In its current state, this tracking method would not be suitable for future clinical studies, as a top-down camera would not be able to provide images of the guidewire and/or catheter through patient tissue. Nonetheless, it is noted that top-down cameras have a narrower clinical translation gap than EM-tracking, as they pose the same 2D challenges as fluoroscopy.
Using AI, it may be possible to create a robotic system capable of autonomously navigating catheters and wires through a patient's vasculature to the target site, requiring minimal assistance from an operator. If proven to be safe and effective in clinical trials, the benefits of autonomous navigation are numerous. It is plausible that in clinical specialities facing a shortage of highly-trained operators, there may be a reduced need for their expertise, potentially leading to greater accessibility of endovascular treatments globally, such as MT. For example, components of MT such as complex navigation tasks could be performed autonomously. Furthermore, autonomous systems are not limited by human factors such as fatigue or loss of focus, potentially making procedures safer and quicker (Mirnezami and Ahmed, 2018).
The concept of fully autonomous navigation in endovascular interventions is promising; however, with a TRL level of 3 (Mankins, 1995), the technology is yet to complete validation even in a laboratory environment. Due to the inadequate evidence supporting its use (the limited number of studies and its low-level; Howick et al., 2011), it is far from being used in clinical practice. It first must be demonstrated that it can reliably provide benefits over currently available treatments before it can progress toward clinical trials.
Importantly, reference standards for endovascular navigation models need to be established to allow new models to be compared. This would allow effective comparison of different AI methods to determine the most effective model for autonomous endovascular navigation. These reference standards need to be established judiciously at the in silico, in vitro, and ex vivo level with carefully-defined environments for different endovascular tasks such as PCI, PVI, and MT. It is noteworthy that at the in silico level, where there are continuous advancements in modeling research and increased computational power, other areas of clinically-orientated ML research have successfully employed reference standards to enable reproducibility of results and comparability between competing models (Russakovsky et al., 2015; Stubbs et al., 2019). This includes computer vision (ImageNet Large Scale Visual Recognition Challenge) and natural language processing (National NLP Clinical Challenges). Furthermore, a set of minimum reporting standards of performance should be defined for studies investigating the use of AI in the autonomous navigation of endovascular interventions. In combination with a reference standard, this would allow complete comparison between ML algorithms designed for this specific task.
Clear regulation is required to determine how the community designs systems for the autonomous navigation in endovascular interventions. In the seven studies (7/14, 50%) which proposed a system with “level 3” autonomy, there is an expert operator in place who can intervene in the autonomous task if needed (“human in the loop”). At higher levels of autonomy where the robot can make decisions, particularly ‘level 5' and potentially ‘level 4', it is unclear how systems will be regulated. Therefore, it may be prudent, for now, for researchers to focus on optimizing systems with ‘level 1–3' autonomy. As such future researchers may wish to optimize simple task autonomy, for example the autonomous navigation from the puncture point to the target site, in a system where an operator can stop the procedure and take over at any time. It is envisaged that as autonomous technology and regulations mature over time, systems will then be updated to carry out more difficult tasks.
Various AI methods have been used to investigate the possibility of autonomous navigation in endovascular interventions. Although it is plausible that autonomous navigation may eventually benefit patients while reducing occupational hazards for staff, there is currently no high-level evidence to support this assertion. For the technology to progress, reference standards and minimum reporting standards need to be established to allow meaningful comparisons of new system development.
The original contributions presented in the study are included in the article/supplementary material, further inquiries can be directed to the corresponding author.
HR, AG, and TB contributed to the study conception and design. HR carried out the paper identification and screening. LK reviewed the manuscripts selected by HR against the eligibility criteria. The first draft of the manuscript was written by HR and edited by AG and TB. All authors commented on versions of the manuscript, read, and approved the manuscript.
Partial financial support was received from the WELLCOME TRUST under Grant Agreement No. 203148/A/16/Z and from the Engineering and Physical Sciences Research Council Doctoral Training Partnership (EPSRC DTP) under Grant Agreement No. EP/R513064/1.
The authors acknowledge support from the Department of Health and Social Care (DHSC) through the National Institute for Health and Care Research (NIHR) MedTech Co-operative award for Cardiovascular Diseases to Guy's & St Thomas' NHS Foundation Trust in partnership with King's College London.
The authors declare that the research was conducted in the absence of any commercial or financial relationships that could be construed as a potential conflict of interest.
All claims expressed in this article are solely those of the authors and do not necessarily represent those of their affiliated organizations, or those of the publisher, the editors and the reviewers. Any product that may be evaluated in this article, or claim that may be made by its manufacturer, is not guaranteed or endorsed by the publisher.
Andrychowicz, M., Wolski, F., Ray, A., Schneider, J., Fong, R., Welinder, P., et al. (2017). “Hindsight experience replay,” in Advances in Neural Information Processing Systems, Vol. 30 (Long Beach, CA).
Arulkumaran, K., Deisenroth, M. P., Brundage, M., and Bharath, A. A. (2017). A brief survey of deep reinforcement learning. IEEE Signal Process. Mag. doi: 10.1109/MSP.2017.2743240
Behr, T., Pusch, T. P., Siegfarth, M., Hüsener, D., Mörschel, T., and Karstensen, L. (2019). Deep reinforcement learning for the navigation of neurovascular catheters. Curr. Direct. Biomed. Eng. 5, 5–8. doi: 10.1515/cdbme-2019-0002
Cancelliere, N. M., Lynch, J., Nicholson, P., Dobrocky, T., Swaminathan, S. K., Hendriks, E. J., et al. (2022). Robotic-assisted intracranial aneurysm treatment: 1 year follow-up imaging and clinical outcomes. J. Neurointervent. Surg. 14, 1229–1233. doi: 10.1136/neurintsurg-2021-017865
Chi, W., Dagnino, G., Kwok, T. M. Y., Nguyen, A., Kundrat, D., Abdelaziz, M. E. M. K., et al. (2020). “Collaborative robot-assisted endovascular catheterization with generative adversarial imitation learning,” in 2020 IEEE International Conference on Robotics and Automation (ICRA) (Paris), 2414–2420. doi: 10.1109/ICRA40945.2020.9196912
Chi, W., Liu, J., Abdelaziz, M. E. M. K., Dagnino, G., Riga, C., Bicknell, C., et al. (2018a). “Trajectory optimization of robot-assisted endovascularcatheterization with reinforcement learning,” in 2018 IEEE/RSJ International Conference on Intelligent Robots and Systems (IROS) (Madrid), 3875–3881. IEEE. doi: 10.1109/IROS.2018.8593421
Chi, W., Liu, J., Rafii-Tari, H., Riga, C., Bicknell, C., and Yang, G. Z. (2018b). Learning-based endovascular navigation through the use of non-rigid registration for collaborative robotic catheterization. Int. J. Comput. Assist. Radiol. Surg. 13, 855–864. doi: 10.1007/s11548-018-1743-5
Cho, Y., Park, J.-H., Choi, J., and Chang, D. E. (2022). “SIM-to-real transfer of image-based autonomous guidewire navigation trained by deep deterministic policy gradient with behavior cloning for fast learning,” in 2022 IEEE/RSJ International Conference on Intelligent Robots and Systems (IROS) (Kyoto), 3468–3475. doi: 10.1109/IROS47612.2022.9982168
Codevilla, F., Santana, E., López, A. M., and Gaidon, A. (2019). “Exploring the limitations of behavior cloning for autonomous driving,” in 2019 IEEE/CVF International Conference on Computer Vision (ICCV) (Seoul), 9328–9337. doi: 10.1109/ICCV.2019.00942
Crinnion, W., Jackson, B., Sood, A., Lynch, J., Bergeles, C., Liu, H., et al. (2022). Robotics in neurointerventional surgery: a systematic review of the literature. J. Neurointervent. Surg. 14, 539–545. doi: 10.1136/neurintsurg-2021-018096
Duran, C., Lumsden, A. B., and Bismuth, J. (2014). A randomized, controlled animal trial demonstrating the feasibility and safety of the Magellan endovascular robotic system. Ann. Vasc. Surg. 28, 470–478. doi: 10.1016/j.avsg.2013.07.010
Fatima, M., and Pasha, M. (2017). Survey of machine learning algorithms for disease diagnostic. J. Intell. Learn. Syst. Appl. 9, 1–16. doi: 10.4236/jilsa.2017.91001
Faure, F., Duriez, C., Delingette, H., Allard, J., Gilles, B., Marchesseau, S., et al. (2012). SOFA, a Multi-Model Framework for Interactive Physical Simulation. Berlin; Heidelberg: Springer. doi: 10.1007/8415_2012_125
Ghesu, F. C., Georgescu, B., Grbic, S., Maier, A., Hornegger, J., and Comaniciu, D. (2018). Towards intelligent robust detection of anatomical structures in incomplete volumetric data. Med. Image Anal. 48, 203–213. doi: 10.1016/j.media.2018.06.007
Giacoppo, D., Colleran, R., Cassese, S., Frangieh, A. H., Wiebe, J., Joner, M., et al. (2017). Percutaneous coronary intervention vs. coronary artery bypass grafting in patients with left main coronary artery stenosis: a systematic review and meta-analysis. JAMA Cardiol. 2, 1079–1088. doi: 10.1001/jamacardio.2017.2895
Goyal, M., Menon, B. K., Zwam, W. H. V., Dippel, D. W., Mitchell, P. J., Demchuk, A. M., et al. (2016). Endovascular thrombectomy after large-vessel ischaemic stroke: a meta-analysis of individual patient data from five randomised trials. Lancet 387, 1723–1731. doi: 10.1016/S0140-6736(16)00163-X
Hausegger, K. A., Schedlbauer, P., Deutschmann, H. A., and Tiesenhausen, K. (2001). Complications in endoluminal repair of abdominal aortic aneurysms. Eur. J. Radiol. 39, 22–33. doi: 10.1016/S0720-048X(01)00339-4
Hessel, M., Modayil, J., van Hasselt, H., Schaul, T., Ostrovski, G., Dabney, W., et al. (2017). “Rainbow: combining improvements in deep reinforcement learning,” in Proceedings of the AAAI Conference on Artificial Intelligence, Vol. 32 (San Francisco, CA). doi: 10.1609/aaai.v32i1.11796
Ho, J., and Ermon, S. (2016). “Generative adversarial imitation learning,” in Advances in Neural Information Processing Systems.
Ho, P., Cheng, S. W., Wu, P. M., Ting, A. C., Poon, J. T., Cheng, C. K., et al. (2007). Ionizing radiation absorption of vascular surgeons during endovascular procedures. J. Vasc. Surg. 46, 455–459. doi: 10.1016/j.jvs.2007.04.034
Howick, J., Chalmers, I., Lind, J., Glasziou, P., Greenhalgh, T., Heneghan, C., et al. (2011). Explanation of the 2011 Oxford Centre for Evidence-Based Medicine (OCEBM) Levels of Evidence (Background Document). Available online at: http://www.cebm.net/index.aspx?o=5653
Ionita, C. N., Mokin, M., Varble, N., Bednarek, D. R., Xiang, J., Snyder, K. V., et al. (2014). “Challenges and limitations of patient-specific vascular phantom fabrication using 3d polyjet printing,” in Medical Imaging 2014: Biomedical Applications in Molecular, Structural, and Functional Imaging, Vol. 9038 (San Francisco, CA), 90380M. SPIE. doi: 10.1117/12.2042266
Jang, B., Kim, M., Harerimana, G., and Kim, J. W. (2019). Q-learning algorithms: a comprehensive classification and applications. IEEE Access 7, 133653–133667. doi: 10.1109/ACCESS.2019.2941229
Jones, B., Riga, C., Bicknell, C., and Hamady, M. (2021). Robot-assisted carotid artery stenting: a safety and feasibility study. CardioVasc. Intervent. Radiol. 44, 795–800. doi: 10.1007/s00270-020-02759-0
Karstensen, L., Ritter, J., Hatzl, J., Pätz, T., Langejürgen, J., Uhl, C., et al. (2022). Learning-based autonomous vascular guidewire navigation without human demonstration in the venous system of a porcine liver. Int. J. Comput. Assist. Radiol. Surg. 17, 2033–2040. doi: 10.1007/s11548-022-02646-8
Klein, L. W., Miller, D. L., Balter, S., Laskey, W., Haines, D., Norbash, A., et al. (2009). Occupational health hazards in the interventional laboratory: time for a safer environment. Soc. Intervent. Radiol. 250, 538–544. doi: 10.1148/radiol.2502082558
Kotsiantis, S. B. (2007). Supervised machine learning: a review of classification techniques. Informatica 31, 249–268.
Kweon, J., Kim, K., Lee, C., Kwon, H., Park, J., Song, K., et al. (2021). Deep reinforcement learning for guidewire navigation in coronary artery phantom. IEEE Access 9, 166409–166422. doi: 10.1109/ACCESS.2021.3135277
Lillicrap, T. P., Hunt, J. J., Pritzel, A., Heess, N., Erez, T., Tassa, Y., et al. (2016). “Continuous control with deep reinforcement learning,” in 4th International Conference on Learning Representations, (ICLR) 2016, eds Y. Bengio and Y. LeCun (San Juan).
Lindgren, A., Vergouwen, M. D., van der Schaaf, I., Algra, A., Wermer, M., Clarke, M. J., et al. (2018). Endovascular coiling versus neurosurgical clipping for people with aneurysmal subarachnoid haemorrhage. Cochrane Database Syst. Rev. 15:CD003085. doi: 10.1002/14651858.CD003085.pub3
Lowery, C., and Faisal, A. A. (2013). “Towards efficient personalized anaesthesia using continuous reinforcement learning for propofol infusion control,” in 2013 6th International IEEE EMBS Conference on Neural Engineering (NER) (San Diego, CA: Institute of Electrical and Electronics Engineers IEEE Engineering in Medicine and Biology Society), 1414–1417. doi: 10.1109/NER.2013.6696208
Madder, R. D., VanOosterhout, S., Mulder, A., Elmore, M., Campbell, J., Borgman, A., et al. (2017). Impact of robotics and a suspended lead suit on physician radiation exposure during percutaneous coronary intervention. Cardiovasc. Revasc. Med. 18, 190–196. doi: 10.1016/j.carrev.2016.12.011
Mankins, J. C. (1995). Technology Readiness Level, a White Paper. NASA, Office of Space Access and Technology, Advanced Concepts Office.
McMeekin, P., White, P., James, M. A., Price, C. I., Flynn, D., and Ford, G. A. (2017). Estimating the number of UK stroke patients eligible for endovascular thrombectomy. Eur. Stroke J. 2, 319–326. doi: 10.1177/2396987317733343
Meng, F., Guo, S., Zhou, W., and Chen, Z. (2021). “Evaluation of a reinforcement learning algorithm for vascular intervention surgery,” in 2021 IEEE International Conference on Mechatronics and Automation, ICMA 2021 (Takamatsu: Institute of Electrical and Electronics Engineers Inc.), 1033–1037. doi: 10.1109/ICMA52036.2021.9512675
Meng, F., Guo, S., Zhou, W., and Chen, Z. (2022). “Evaluation of an autonomous navigation method for vascular interventional surgery in virtual environment,” in 2022 IEEE International Conference on Mechatronics and Automation, ICMA 2022 (Columbus, OH: Institute of Electrical and Electronics Engineers Inc.), 1599–1604. doi: 10.1109/ICMA54519.2022.9856107
Mirnezami, R., and Ahmed, A. (2018). Surgery 3.0, artificial intelligence and the next-generation surgeon. Br. J. Surg. 105, 463–465. doi: 10.1002/bjs.10860
Mnih, V., Badia, A. P., Mirza, M., Graves, A., Lillicrap, T. P., Harley, T., et al. (2016). “Asynchronous methods for deep reinforcement learning,” in Proceedings of the 33rd International Conference on Machine Learning - Volume 48 (New York, NY), 1928–1937.
Mnih, V., Kavukcuoglu, K., Silver, D., Graves, A., Antonoglou, I., Wierstra, D., et al. (2013). Playing atari with deep reinforcement learning. CoRR, abs/1312.5602. abs/1312.5602.
Mofatteh, M. (2021). Neurosurgery and artificial intelligence. AIMS Neurosci. 8, 477–495. doi: 10.3934/Neuroscience.2021025
Mongan, J., Moy, L., and Kahn, C. E. (2020). Checklist for artificial intelligence in medical imaging (claim): a guide for authors and reviewers. Radiology 2:e200029. doi: 10.1148/ryai.2020200029
Nair, A., McGrew, B., Andrychowicz, M., Zaremba, W., and Abbeel, P. (2017). “Overcoming exploration in reinforcement learning with demonstrations,” in 2018 IEEE International Conference on Robotics and Automation (ICRA) (Singapore), 6292–6299. doi: 10.1109/ICRA.2018.8463162
Naros, G., and Gharabaghi, A. (2015). Reinforcement learning of self-regulated beta-oscillations for motor restoration in chronic stroke. Front. Hum. Neurosci. 9:391. doi: 10.3389/fnhum.2015.00391
Nogueira, R. G., Sachdeva, R., Al-Bayati, A. R., Mohammaden, M. H., Frankel, M. R., and Haussen, D. C. (2020). Robotic assisted carotid artery stenting for the treatment of symptomatic carotid disease: technical feasibility and preliminary results. J. NeuroIntervent. Surg. 12, 341–344. doi: 10.1136/neurintsurg-2019-015754
Nussbaumer-Streit, B., Klerings, I., Dobrescu, A. I., Persad, E., Stevens, A., Garritty, C., et al. (2020). Excluding non-english publications from evidence-syntheses did not change conclusions: a meta-epidemiological study. J. Clin. Epidemiol. 118, 42–54. doi: 10.1016/j.jclinepi.2019.10.011
O'Shea, K., and Nash, R. (2015). An introduction to convolutional neural networks. ArXiv, abs/1511.08458.
Page, M. J., McKenzie, J. E., Bossuyt, P. M., Boutron, I., Hoffmann, T. C., Mulrow, C. D., et al. (2021). The PRISMA 2020 statement: an updated guideline for reporting systematic reviews. BMJ 2021:372. doi: 10.31222/osf.io/v7gm2
Pereira, M., Cancelliere, N., Nicholson, P., Radovanovic, I., Drake, K. E., Sungur, J.-M., et al. (2020). First-in-human, robotic-assisted neuroendovascular intervention. J. NeuroIntervent. Surg. 12, 338–340. doi: 10.1136/neurintsurg-2019-015671.rep
Rabiner, L. R. (1989). A tutorial on hidden markov models and selected applications in speech recognition. Proc. IEEE 77, 257–286. doi: 10.1109/5.18626
Rafii-Tari, H., Liu, J., Lee, S.-L., Bicknell, C., and Yang, G.-Z. (2013). “Learning-based modeling of endovascular navigation for collaborative robotic catheterization,” in Medical Image Computing and Computer-Assisted Intervention–MICCAI 2013 (Berlin; Heidelberg: Springer), 369–377. doi: 10.1007/978-3-642-40763-5_46
Rafii-Tari, H., Liu, J., Payne, C. J., Bicknell, C., and Yang, G.-Z. (2014). “Hierarchical hmm based learning of navigation primitives for cooperative robotic endovascular catheterization,” in Medical Image Computing and Computer-Assisted Intervention-MICCAI 2014 (Japan: Nagoya University; Springer International Publishing), 496–503. doi: 10.1007/978-3-319-10404-1_62
Redmon, J., Divvala, S. K., Girshick, R. B., and Farhadi, A. (2015). “You only look once: unified, real-time object detection,” in 2016 IEEE Conference on Computer Vision and Pattern Recognition (CVPR) (Las Vegas, NV), 779–788. doi: 10.1109/CVPR.2016.91
Reynolds, D. (2015). “Gaussian Mixture Models”, in Encyclopedia of Biometrics, eds S. Z. Li and A. K. Jain (Boston, MA: Springer). doi: 10.1007/978-1-4899-7488-4_196
Riga, C. V., Cheshire, N. J., Hamady, M. S., and Bicknell, C. D. (2010). The role of robotic endovascular catheters in fenestrated stent grafting. J. Vasc. Surg. 51, 810–820. doi: 10.1016/j.jvs.2009.08.101
Rudnick, M. R., Goldfarb, S., Wexler, L., Ludbrook, P. A., Murphy, M. J., Halpern, E. F., et al. (1995). Nephrotoxicity of ionic and nonionic contrast media in 1196 patients: a randomized trial. Kidney Int. 47, 254–261. doi: 10.1038/ki.1995.32
Russakovsky, O., Deng, J., Su, H., Krause, J., Satheesh, S., Ma, S., et al. (2015). Imagenet large scale visual recognition challenge. Int. J. Comput. Vis. 115, 211–252. doi: 10.1007/s11263-015-0816-y
Rutjes, A., Westwood, M., Deeks, J. J., Whiting, P. F., Weswood, M. E., Rutjes, A. W., et al. (2011). QUADAS-2: a revised tool for the quality assessment of diagnostic accuracy studies evaluation of quadas, a tool for the quality assessment of diagnostic accuracy studies. Ann. Intern. Med. 155, 529–536. doi: 10.7326/0003-4819-155-8-201110180-00009
Saber, H., Beaman, C., and Tateshima, S. (2022). Complete robotic intervention for acute epistaxis in a patient with COVID-19 pneumonia: technical considerations and device selection tips. J. NeuroIntervent. Surg. 14, 500–502. doi: 10.1136/neurintsurg-2021-018582
Sajja, K. C., Sweid, A., Saiegh, F. A., Chalouhi, N., Avery, M. B., Schmidt, R. F., et al. (2020). Endovascular robotic: feasibility and proof of principle for diagnostic cerebral angiography and carotid artery stenting. J. NeuroIntervent. Surg. 12, 345–349. doi: 10.1136/neurintsurg-2019-015763
Sarker, I. H. (2021). Machine learning: algorithms, real-world applications and research directions. SN Comput. Sci. 2:160. doi: 10.1007/s42979-021-00592-x
Saver, J. L., Goyal, M., Lugt, A. V. D., Menon, B. K., Majoie, C. B., Dippel, D. W., et al. (2016). Time to treatment with endovascular thrombectomy and outcomes from ischemic stroke: a meta-analysis. J. Am. Med. Assoc. 316, 1279–1288. doi: 10.1001/jama.2016.13647
Schulman, J., Wolski, F., Dhariwal, P., Radford, A., and Klimov, O. (2017). Proximal policy optimization algorithms. arXiv: abs/1707.06347.
Schwein, A., Kramer, B., Chinnadurai, P., Walker, S., O'Malley, M., Lumsden, A., and Bismuth, J. (2017). Flexible robotics with electromagnetic tracking improves safety and efficiency during in vitro endovascular navigation. J. Vasc. Surg. 65, 530–537. doi: 10.1016/j.jvs.2016.01.045
Silahtaroğlu, G., and Yılmaztürk, N. (2021). Data analysis in health and big data: a machine learning medical diagnosis model based on patients' complaints. Commun. Stat. 50, 1547–1556. doi: 10.1080/03610926.2019.1622728
Stubbs, A., Filannino, M., Soysal, E., and Henry, S. (2019). Cohort selection for clinical trials: N2c2 2018 shared task track 1. J. Am. Med. Inform. Assoc. 26, 1163–1171. doi: 10.1093/jamia/ocz163
Sutton, R. S., and Barto, A. G. (2018). Reinforcement Learning: An Introduction, 2nd Edn. Cambridge: The MIT Press.
Theodorou, E. A., Buchli, J., and Schaal, S. (2010). A generalized path integral control approach to reinforcement learning. J. Mach. Learn. Res. 11, 3137–3181. doi: 10.1109/ROBOT.2010.5509336
Thukkani, A. K., and Kinlay, S. (2015). Endovascular intervention for peripheral artery disease. Circul. Res. 116, 1599–1613. doi: 10.1161/CIRCRESAHA.116.303503
Townsend, N., Wilson, L., Bhatnagar, P., Wickramasinghe, K., Rayner, M., and Nichols, M. (2016). Cardiovascular disease in Europe: epidemiological update 2016. Eur. Heart J. 37, 3232–3245. doi: 10.1093/eurheartj/ehw334
Wang, S., Liu, Z., Shu, X., Cao, Y., Zhang, L., and Xie, L. (2022). “Study on autonomous delivery of guidewire based on improved yolov5s on vascular model platform,” in 2022 IEEE International Conference on Robotics and Biomimetics (ROBIO) (Xishuangbanna), 1–6. doi: 10.1109/ROBIO55434.2022.10011829
Wang, Z., Schaul, T., Hessel, M., van Hasselt, H., Lanctot, M., and de Freitas, N. (2016). “Dueling network architectures for deep reinforcement learning,” in Proceedings of the 33rd International Conference on Machine Learning - Volume 48 (New York, NY), 1995–2003.
Weinberg, J. H., Sweid, A., Sajja, K., Gooch, M. R., Herial, N., Tjoumakaris, S., et al. (2021). Comparison of robotic-assisted carotid stenting and manual carotid stenting through the transradial approach. J. Neurosurg. 135, 21–28. doi: 10.3171/2020.5.JNS201421
Yang, G. Z., Cambias, J., Cleary, K., Daimler, E., Drake, J., Dupont, P. E., et al. (2017). Medical robotics-regulatory, ethical, and legal considerations for increasing levels of autonomy. Sci. Robot. 2:eaam8638. doi: 10.1126/scirobotics.aam8638
You, H., Bae, E. K., Moon, Y., Kweon, J., and Choi, J. (2019). Automatic control of cardiac ablation catheter with deep reinforcement learning method. J. Mech. Sci. Technol. 33, 5415–5423. doi: 10.1007/s12206-019-1036-0
Keywords: artificial intelligence, machine learning, endovascular intervention, autonomy, navigation
Citation: Robertshaw H, Karstensen L, Jackson B, Sadati H, Rhode K, Ourselin S, Granados A and Booth TC (2023) Artificial intelligence in the autonomous navigation of endovascular interventions: a systematic review. Front. Hum. Neurosci. 17:1239374. doi: 10.3389/fnhum.2023.1239374
Received: 15 June 2023; Accepted: 20 July 2023;
Published: 04 August 2023.
Edited by:
Yu Huang, Soterix Medical Inc., United StatesReviewed by:
Mohammad Mofatteh, Queen's University Belfast, United KingdomCopyright © 2023 Robertshaw, Karstensen, Jackson, Sadati, Rhode, Ourselin, Granados and Booth. This is an open-access article distributed under the terms of the Creative Commons Attribution License (CC BY). The use, distribution or reproduction in other forums is permitted, provided the original author(s) and the copyright owner(s) are credited and that the original publication in this journal is cited, in accordance with accepted academic practice. No use, distribution or reproduction is permitted which does not comply with these terms.
*Correspondence: Thomas C. Booth, dGhvbWFzLmJvb3RoQGtjbC5hYy51aw==
Disclaimer: All claims expressed in this article are solely those of the authors and do not necessarily represent those of their affiliated organizations, or those of the publisher, the editors and the reviewers. Any product that may be evaluated in this article or claim that may be made by its manufacturer is not guaranteed or endorsed by the publisher.
Research integrity at Frontiers
Learn more about the work of our research integrity team to safeguard the quality of each article we publish.