- 1Department of Magnetic Resonance Imaging, The First Affiliated Hospital of Zhengzhou University, Zhengzhou, Henan, China
- 2Key Laboratory of Magnetic Resonance and Brain Function of Henan Province, Zhengzhou, China
- 3Engineering Technology Research Center for Detection and Application of Brain Function of Henan Province, Zhengzhou, China
- 4Key Laboratory for Functional Magnetic Resonance Imaging and Molecular Imaging of Henan Province, Zhengzhou, China
- 5Engineering Research Center of Medical Imaging Intelligent Diagnosis and Treatment of Henan Province, Zhengzhou, China
- 6Zhengzhou Key Laboratory of Brain Function and Cognitive Magnetic Resonance Imaging, Zhengzhou, China
- 7Henan Key Laboratory of Imaging Intelligence Research, Zhengzhou, China
- 8Henan Engineering Research Center of Brain Function Development and Application, Zhengzhou, China
- 9Department of Radiology, The Third Affiliated Hospital of Zhengzhou University, Zhengzhou, China
Background: Chronic smokers have abnormal spontaneous regional activity and disrupted functional connectivity as revealed by previous neuroimaging studies. Combining different dimensions of resting-state functional indicators may help us learn more about the neuropathological mechanisms of smoking.
Methods: The amplitude of low frequency fluctuations (ALFF) of 86 male smokers and 56 male non-smokers were first calculated. Brain regions that displayed significant differences in ALFF between two groups were selected as seeds for further functional connectivity analysis. Besides, we examined correlations between brain areas with abnormal activity and smoking measurements.
Results: Increased ALFF in left superior frontal gyrus (SFG), left medial superior frontal gyrus (mSFG) and middle frontal gyrus (MFG) as well as decreased ALFF in right calcarine sulcus were observed in smokers compared with non-smokers. In the seed-based functional connectivity analysis, smokers showed attenuated functional connectivity with left SFG in left precuneus, left fusiform gyrus, left lingual gyrus, left cerebellum 4 5 and cerebellum 6 as well as lower functional connectivity with left mSGF in left fusiform gyrus, left lingual gyrus, left parahippocampal gyrus (PHG), left calcarine sulcus, left cerebellum 4 5, cerebellum 6 and cerebellum 8 (GRF corrected, Pvoxel < 0.005, Pcluster<0.05). Furthermore, attenuated functional connectivity with left mSGF in left lingual gyrus and PHG displayed a negative correlation with FTND scores (r = −0.308, p = 0.004; r = −0.326, p = 0.002 Bonferroni corrected).
Conclusion: Our findings of increased ALFF in SFG with reduced functional connectivity to visual attention areas and cerebellum subregions may shed new light on the pathophysiology of smoking.
1. Introduction
Cigarette smoking is among the leading causes of preventable illness and death in the earth, characterized by craving, relapsing and withdrawal symptoms (Asma et al., 2014). It is closely related to a variety of tumors, respiratory and digestive system diseases, and adversely affects human health, economics, and life (Goldfarbmuren et al., 2020; Yang et al., 2022). According to statistics, smoking contributes to roughly one in five deaths (Jamal et al., 2018). While many smokers know the adverse consequences of long-term smoking, relapse rates are higher than 85 percent among those who attempt to stop smoking by themselves (Weiland et al., 2015). Given the serious harms of smoking, understanding the neural mechanisms underlying it may contribute to guide brain-based smoking cessation treatments.
The resting state functional magnetic resonance imaging (rs-fMRI) technique, which measures brain activity under resting state using low-frequency fluctuations of the blood oxygen level dependent (BOLD) signal (Biswal et al., 1995), has been extensively employed in the study of the abnormalities of neural activity in a wide range of neuropsychiatric diseases (Zhang et al., 2019; Zhou et al., 2019; Dong et al., 2021; He et al., 2021; Han et al., 2022). Regional spontaneous neural features have shown to be significant for comprehending the neuropathologic and neurophysiological condition of disease (Raichle, 2006). Spontaneous low-frequency fluctuation of BOLD signal has been certified to be closely related to the spontaneous activity of neurons (Lu et al., 2007; Mantini et al., 2007). Amplitude of low frequency fluctuation (ALFF) served as a common approach characterizes the spontaneous regional neural activity by measuring the intensity of spontaneous fluctuation areas (Zang et al., 2007; Wang et al., 2021). Many researchers measured spontaneous brain activity in substance users by using ALFF and found that it is influenced by substance abuse (e.g., cigarettes, heroin, alcohol) (Brody, 2006; Jiang et al., 2011). Studies on humans and animals have confirmed that nicotine disturbs brain regional spontaneous activity, which is linked to the dopaminergic system and nicotinic acetylcholine receptors (Hyman et al., 2006). It has widely reported that brain activity globally reduced in acute administration of nicotine (Brody, 2006). Chronic smokers were also reported to have altered spontaneous brain activity during resting state in some studies (Yu et al., 2013; Chu et al., 2014; Wu et al., 2015; Wen et al., 2021). Wen et al. (2021) has found that smokers showed significantly increased static ALFF in the bilateral middle frontal gyrus and left inferior frontal gyrus. Increased dynamic ALFF in the left inferior frontal gyrus, left superior/medial frontal gyrus and bilateral middle frontal gyrus have also been reported in smokers than non-smokers. In addition, temporal variability of dynamic ALFF in the left superior/medial frontal gyrus and right middle frontal gyrus showed positive correlations with smoking measurements such as pack-years and Fagerström Test for Nicotine Dependence (FTND) scores. Increased spontaneous regional activity in the prefrontal cortex involved in cognitive control and reward processing were previous most commonly findings (Domino et al., 2000; Wen et al., 2021), which is an important target neural region for smoking addiction.
Amplitude of low frequency fluctuationsALFF allows us to know the amplitude of regional neuronal activity, potentially identifying abnormal local functional brain activity. In addictive diseases, more than just the spontaneous regional activity within specific brain regions drives the disease, but also coordination between them (Wang et al., 2018). Whether the functional connections between brain regions with abnormal spontaneous regional activity and other regions are altered in chronic smokers remains unexplored. Using resting-state functional connectivity (rs-FC) method, we can measure temporal correlation between BOLD signals in specific brain networks, finding the degree of interregional cooperation between various spatial regions. There was widespread FC abnormality in certain brain regions among smokers in comparison with non-smokers, according to previous studies. Wang has found decreased FC of left thalamo-precuneus in relapsing smokers (Wang et al., 2020). In smokers as opposed to non-smokers, FC has been found to be attenuated in the reward circuit (Shen et al., 2016). Furthermore, the relationship between altered FC of the brain and the severity of nicotine dependence in smokers has been investigated by many neuroimaging studies. The severity of smoking was usually measured using FTND scores (Heatherton et al., 1991). An extensive study has reported a positive correlation between FTND scores and enhanced FC in insular and visual processing cortex in smokers (Claus et al., 2013). Therefore, it is crucial to explore various aspects of resting state functioning to elucidate the neural mechanisms of smoking, since there may be differences in both local neuronal activity and connectivity patterns.
In this research, we first calculated the spontaneous regional brain activity of two groups (smokers and non-smokers) by using the ALFF. Then, brain regions that displayed significant differences in ALFF between smoking group and control group were used as seeds for further seed-based FC analysis. At last, we performed correlation analyses between brain areas with abnormal activity and smoking-related behavior. Based on previous functional research of smoking, we predicted that chronic smokers would show increased spontaneous regional activity in cognitive control network and FC analyses would reveal disrupted functional connectivity between the seeds and some regions of the brain intimately connected to their function.
2. Materials and methods
2.1. Participants
This study included 142 male participants aged 25–45 (86 smokers and 56 non-smokers) from a local hospital as well as via the Internet. Since the smoking rate is much higher among Chinese men than women, the public health burden falls mainly on this group (He et al., 2020). The current study focused on male smokers. The severity of cigarette smoking was measured by FTND scores, and pack-years was calculated as (years of smoking multiplied by the number of cigarettes smoked per day)/20 (Thomas, 2014). Smokers were included by meeting the following criteria: (1) right-handed; (2) meeting the diagnosis criteria for substance use disorder in the fifth edition of the Diagnostic and Statistical Manual for Mental Disorders (DSM-5); (3) smoking no less than 10 cigarettes per day and continue for at least 2 years (Zhang et al., 2022); (4) smokers did not attempt to stop smoking and had no abstinence history. The inclusion criteria for non-smokers were:(1) smoking less than five cigarettes in their lifetime; (2) age, gender, and education level were matched to the smokers group; (3) none of the included controls had been exposed to long-term second-hand smoke. Exclusion criteria included any physical illness including obstructive lung disease, epilepsy, brain tumor or cerebrovascular disease; addiction to other substance (except nicotine); a history of neuropsychiatric disease; contraindications to MRI. The study has been approved by the Local Medical Ethics Committee of the First Affiliated Hospital of Zhengzhou University. Informed consent was acquired from all study subjects.
2.2. MRI data acquisition
Magnetic resonance imaging data were acquired using a MAGNETOM Skyra 3T MR scanner (Siemens Healthcare, Erlangen, Germany) with 64 channel head coils. Smokers were required to smoke a cigarette 30 min before the scan to avoid nicotine withdrawal symptoms. During the scanning, each participant was asked to close their eyes, not to think of anything special, breathe quietly, and avoid falling asleep. At the end of the scan, the subjects were asked if they had fallen asleep, and those who had fallen asleep were eliminated. We used foam pads and earplugs to minimize head movement and canner noise. The following echo planar imaging parameters were used to acquire functional images: repetition time (TR)/echo time = 2,000/30 ms, matrix size = 64 × 64, flip angle = 80°, field of view = 240 × 240 mm, voxel size = 3 mm × 3 mm × 3 mm, slices = 36, slice thickness = 4 mm, and 180 volumes in total.
2.3. Data analysis
This functional imaging data set was preprocessed by using Data Processing Assistant for Resting-state fMRI Analysis Toolkit (DPARSF). Main pre-processing steps and parameters are as follows: (1) conversion of data formats; (2) first five time points were removed due to the instabilities of the initial rs-fMRI signal and patients’ inability to adapt to acquisition conditions initially; (3) slice timing; (4) realignment (excluding subjects with a maximum head motion >2.5 mm or head rotation>2.5°). No subjects were excluded from this step; (5) normalization was performed using EPI templates and resampling was done using 3 mm × 3 mm × 3 mm samples; (6) regression of 24 head motion parameters, global signal, cerebrospinal fluid signal as well as white matter signal (Friston et al., 1996); (7) Frame wise displacement (FD) was calculated for each time point (Power et al., 2012), and an average FD greater than 0.5 mm was excluded; (8) a full-width Gaussian kernel with a half-maximum of 6 mm was used for smoothing; (9) for eliminating low frequency drift and high frequency noise effects, linear trends and temporal filtering (bandpass, 0.01–0.08 Hz) are removed.
Based on Fast Fourier transform (FFT), ALFF was calculated for each voxel and its time series was converted to frequency domain without a band-pass filter. First, the square root was computed at each frequency of the power spectrum, and then the average square root of each voxel in the 0.01–0.08 Hz band was acquired. The last step was to divide the ALFF of each voxel by the global mean of ALFF values (mALFF) (Zang et al., 2007). The resulting ALFF values for each voxel were used for further analysis.
The region of interest (ROI) seed-based method was applied to assess differences in rs-FC between smokers and non-smokers. According to the results of ALFF, brain regions with significant differences in ALFF values between the smoking group and the control group were selected as ROI, which was defined as a sphere with a radius of 5 mm and centered on the peak coordinates. The Pearson correlation between the average time series of the seed region and the time series of all voxels within the whole brain was calculated to produce a rs-FC map for each subject. Correlation coefficients were transformed to z-scores using the Fisher Z-transform to fit the data to a normal distribution.
2.4. Statistical analyses
We used two-sample t-tests based on IBM SPSS Statistics software (version 26.0) to compare demographic and clinical characteristics between smokers and non-smokers. As covariates, age, years of education and mean FD were used in a two-tailed two-sample t-test to compare ALFF and ALFF-based rs-FC between two groups (Gaussian random field theory GRF corrected, Pvoxel < 0.005, Pcluster < 0.05) based on the MATLABSPM12 toolkit. In order to examine the association between brain regions with abnormal activity and clinical characteristics of smoking, we performed correlation analyses between the values of two functional indicators and smoking measurements (such as FTND scores and pack-years).
3. Results
3.1. Participant characteristics
In all, 86 male smokers and 56 male non-smokers were recruited in the current study. No significant differences were found in years of education and age between the two groups (all P-values>0.05). See Table 1 for details.
3.2. Between-group comparisons of ALFF
In comparison of the control group, smokers displayed higher ALFF in left superior frontal gyrus (SFG), left medial superior frontal gyrus (mSFG) and left middle frontal gyrus (MFG) as well as displayed lower ALFF in right calcarine sulcus (GRF corrected, Pvoxel < 0.005, Pcluster < 0.05) (Table 2 and Figure 1).
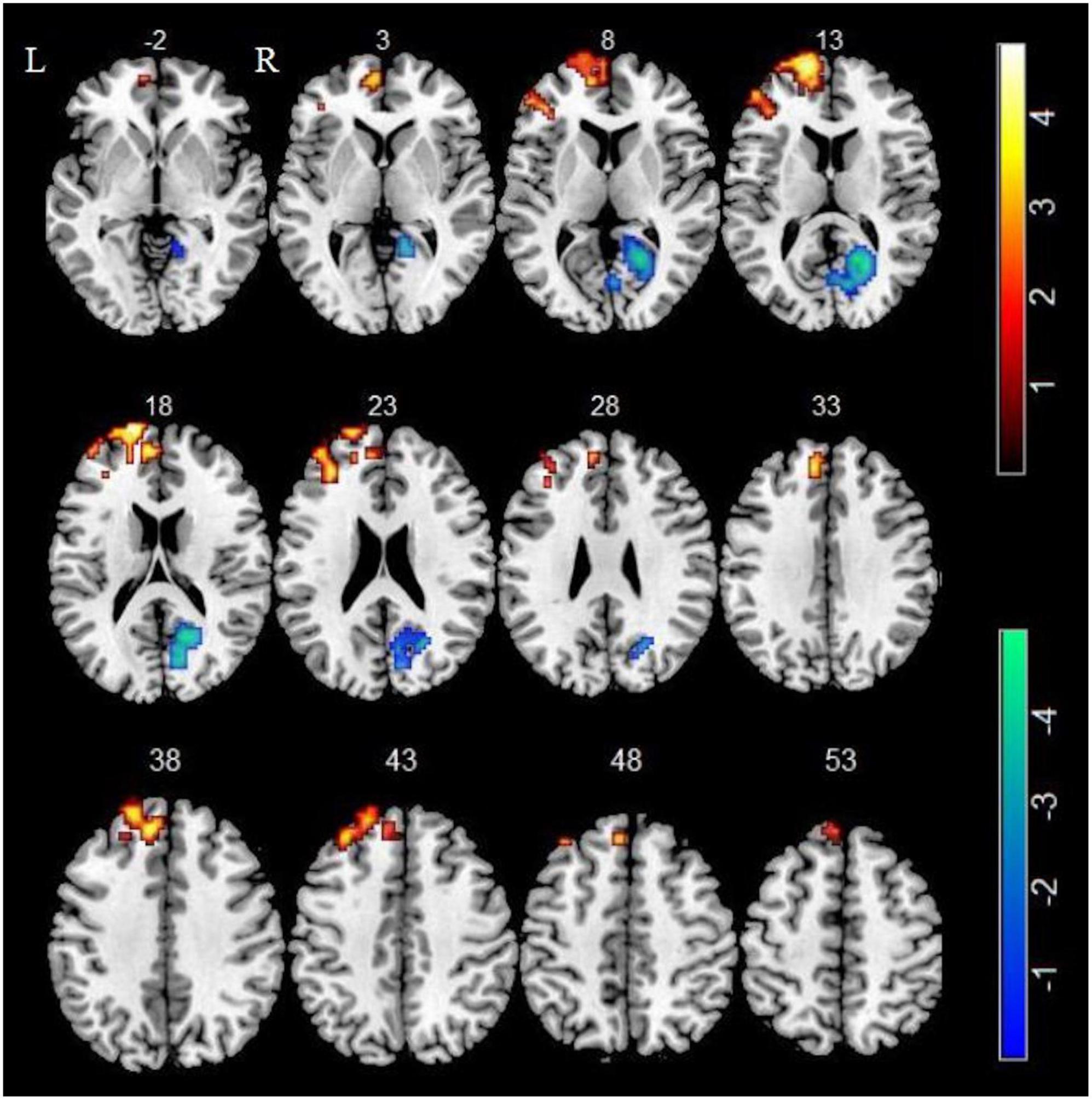
Figure 1. Compared with non-smokers, smokers showed significant differences in ALFF. Regions of increased ALFF were shown in red. Regions of decreased ALFF were shown in blue.
3.3. Between-group comparisons of ALFF-based rs-FC
In comparison of the non-smokers, smokers showed attenuated functional connectivity with left SFG in left precuneus, left fusiform, left lingual gyrus, left cerebellum 4 5 and cerebellum 6 as well as lower functional connectivity with left mSGF in left fusiform, left lingual, left parahippocampal gyrus (PHG), left calcarine sulcus, left cerebellum 4 5, left cerebellum 6 and left cerebellum 8 (GRF corrected, Pvoxel < 0.005, Pcluster < 0.05) (Table 3 and Figure 2).
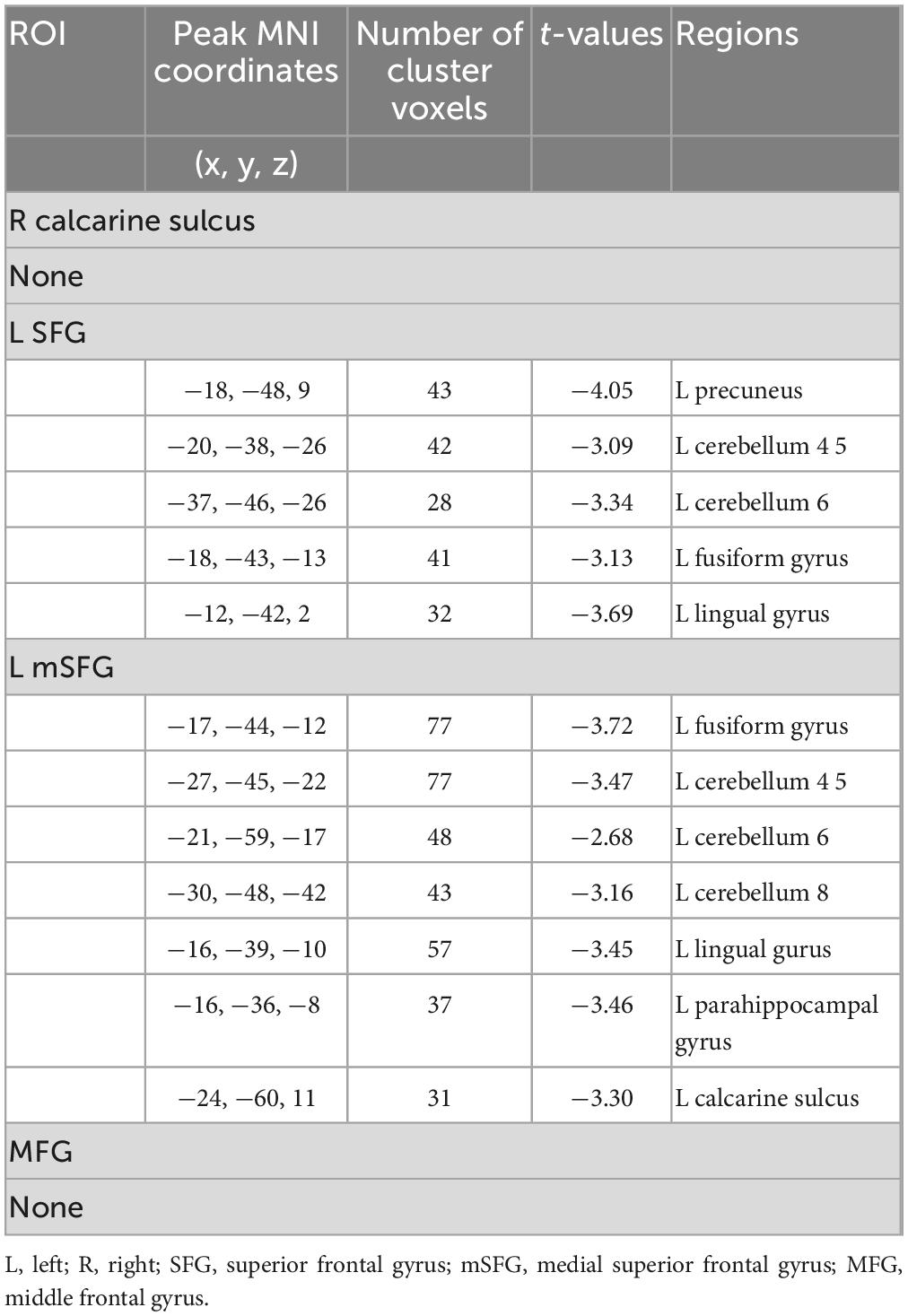
Table 3. Seed locations and regions showing significant differences in connectivity between smokers and non-smokers.
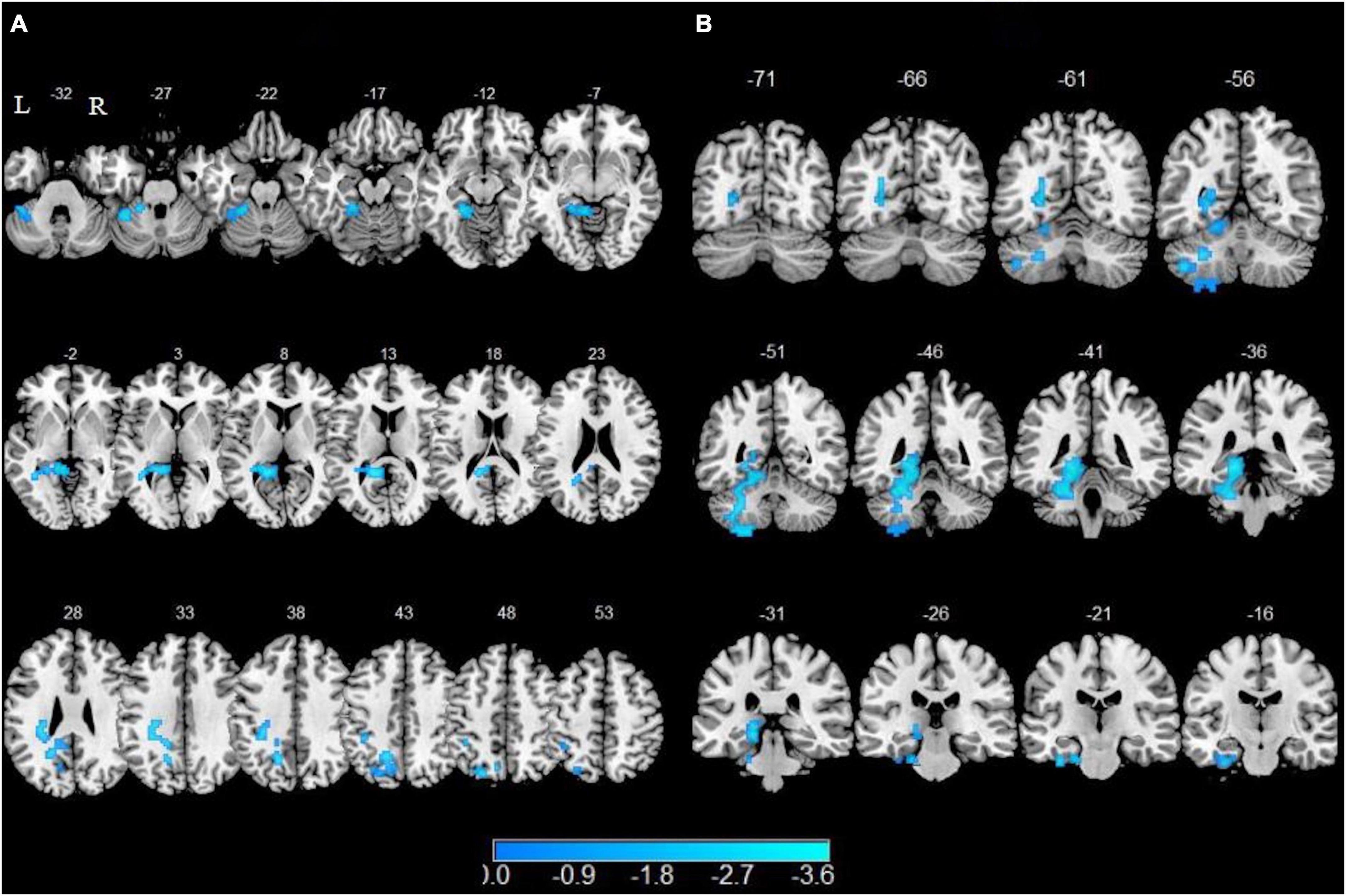
Figure 2. Altered resting-state functional connectivity with the left superior frontal gyrus (SFG) and left medial superior frontal gyrus (mSFG). Brain regions with decreased functional connectivity to the left SFG are shown in panel (A). Brain regions with decreased functional connectivity to the left mSFG are shown in panel (B).
3.4. Correlation analysis
The results demonstrated that attenuated functional connectivity with left mSGF in left lingual gyrus and left PHG displayed a negative correlation with FTND scores (r = −0.308, p = 0.004; r = −0.326, p = 0.002 Bonferroni corrected). Other brain regions were either not significantly associated with FTND scores or the results did not pass the correction (Figure 3).
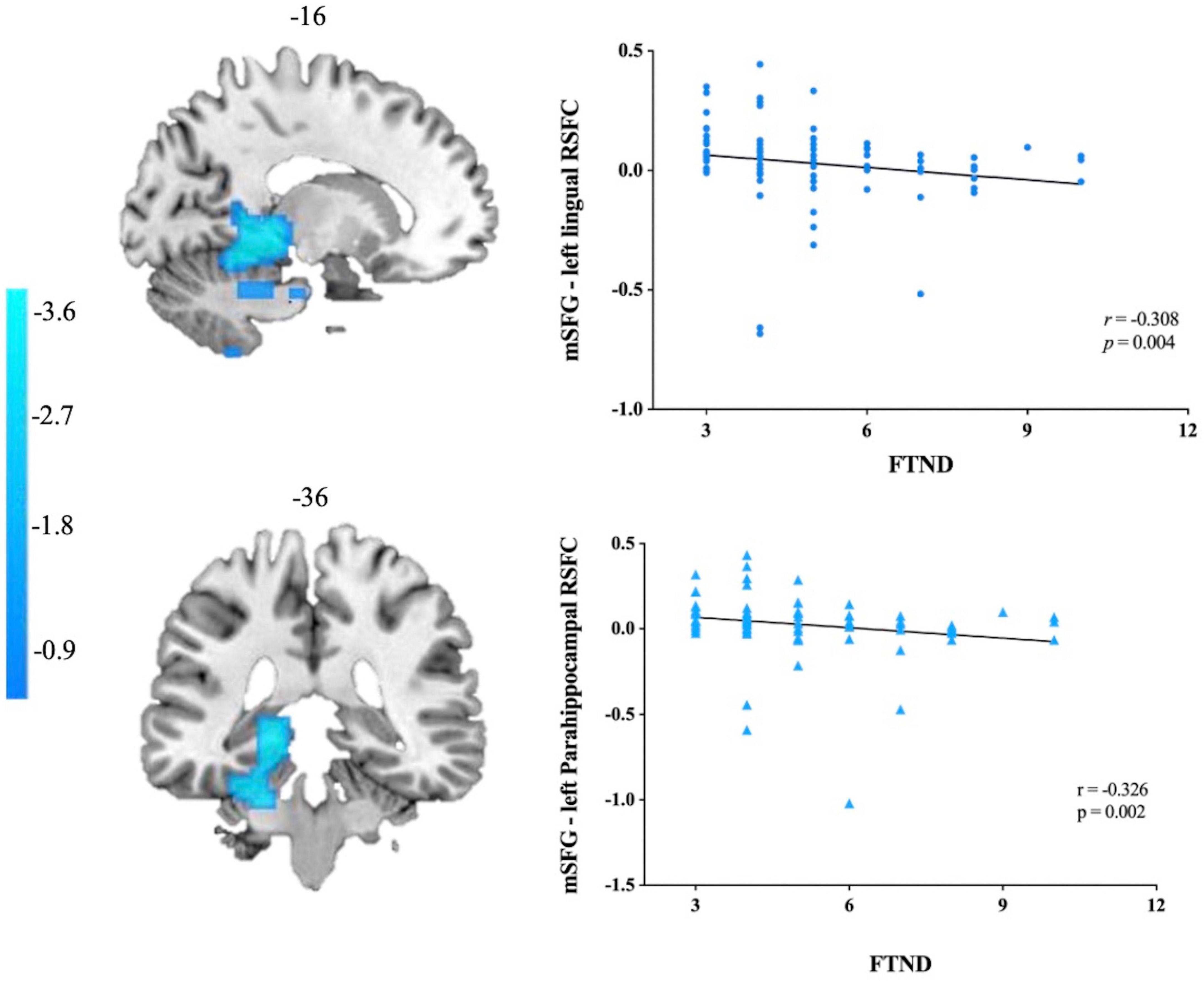
Figure 3. Correlations between mSFG–left lingual gyrus/parahippocampal gyrus rs-FC and the FTND scores in smokers. mSFG, medial superior frontal gyrus; FTND, Fagerström Test for Nicotine Dependence.
4. Discussion
Our study combined amplitude of low frequency fluctuation (ALFF) and resting-state functional connectivity (rs-FC) to identify the alterations of intrinsic brain activity and neural connectivity abnormalities in smokers. Two key findings emerged from the study. On the one hand, smokers displayed higher spontaneous regional activity in the prefrontal cortex (PFC) including left superior frontal gyrus (SFG), medial superior frontal gyrus (mSFG) and middle frontal gyrus (MFG) as well as lower spontaneous brain activity in right calcarine sulcus. On the other hand, smokers displayed lower functional connectivity between the PFC with precuneus, parahippocampal gyrus (PHG), cerebellar subregions (such as cerebellum 4 5, cerebellum 6 and cerebellum 8) as well as visual attention areas including the calcarine sulcus, lingual gyrus and fusiform gyrus. Functional connectivity of the mSFG with the PHG and lingual gyrus was negatively related to FTND scores based on correlation analyses. These findings contributed to understand the altered intrinsic brain activity and neural connectivity abnormalities in smokers and highlighted the role of visual attention areas and cerebellum in cigarette smoking.
The ALFF measures the intensity of the spontaneous fluctuation area in order to reflect the spontaneous regional activity in the resting state. It has been widely viewed as a means to reveal the intrinsic brain activity alterations in addiction. In the current study, We found increased ALFF in the left PFC of smokers in comparison of non-smokers, which was in accordance with the findings of previous fMRI studies (Wen et al., 2021). The PFC plays an important role in reward evaluation and is the basis of working memory, which stores smoking-related information and enables rapid integration and updating of other information to guide target behaviors (Hyman et al., 2006). Keeping goal representations within the PFC is vital to the cognitive control that enables goal-directed behavior to succeed (Miller, 2000; Rolls, 2004). It has been reported that the prefrontal lobe is selectively damaged by cigarette smoking (Franklin et al., 2007). The PFC not only plays a positive role in guiding organisms to achieve their goals successfully, but also plays an important role in inhibiting maladaptive responses (Hyman et al., 2006). As a result of the effects of nicotine on the PFC, smokers may have difficulty resisting the urge to smoke even though they know the dangers of smoking due to pathological valuations and a lack of top-down behavioral control.
In addition, we also noted that compared with non-smokers, smokers showed decreased spontaneous brain activity in right calcarine sulcus as well as attenuated functional connectivity between the left mSFG with visual attention areas including the left calcarine sulcus, lingual gyrus and fusiform gyrus. The mSFG is anatomically mostly associated with the anterior cingulate cortex (ACC) and the medial cingulate cortex (MCC) (Li et al., 2013) and that it is functionally related to cognitive control including conflict monitoring (Sohn et al., 2007; Ursu et al., 2009), error detection (Gehring and Fencsik, 2001; Pourtois et al., 2010), attention control (Crottaz-Herbette and Menon, 2006; Luo et al., 2007). Visual information integration and attention processing are important functions of the calcarine sulcus, which is part of the visual attention network (Bezdek et al., 2015). Yang et al. (2022) has found decreased static functional connectivity density in the calcarine sulcus in nicotine dependent individuals. Fusiform gyrus served as a vital component of the visual cortex and was involved in a range of visual cognitive functions, including face and body recognition, color information processing and emotion perception in facial stimulation (Sergent et al., 1992; Kanwisher et al., 1997; Wang et al., 2013; Weiner et al., 2014). One study found greater neural activation in fusiform gyrus in smokers after exposure to smoking-related images than neutral images (Due et al., 2002). And it has been demonstrated by a meta-analysis that subjects who are addicted to tobacco, alcohol, and cocaine have a higher gray matter volume in the fusiform gyrus (Zhang et al., 2021). The reduced functional connectivity between the mSFG and the visual attention areas in our results may suggest that neural communication between the cognitive control network and the visual attention network is impaired in chronic smokers. Top-down attentional bias mechanism in visual cortex has been widely reported (Buschschulte et al., 2014). Previous studies have found that smokers show top-down attentional bias disorder and inhibitory attentional bias disorder to cigarette cues (Marks et al., 2016; Wilcockson et al., 2021). Our results suggested that the reduced functional connectivity of these regions is connected with the attentional bias of smokers, which may make it easier for them to collect smoking-related information, thus inducing craving and even smoking behaviors.
Located between the calcarine sulcus and the fusiform gyrus, the lingual gyrus plays a crucial role in visual processing, and the collateral sulcus reaches the temporal lobe, which links the PHG (Jung et al., 2014). There have been research suggesting that the PHG plays an important role in episodic memory, associative memory, and recollection (Zola-Morgan et al., 1989; Davachi et al., 2003; Diana et al., 2010), as well as visuospatial processing (Epstein and Kanwisher, 1998; Ekstrom et al., 2003; Stevens et al., 2012). Smoking cues (such as seeing someone smoking or cigarettes related cues) can trigger recall of smoking-related memories in smokers. An fMRI smoking cue-induced task study found that activation of the PHG was inversely correlated with the severity of nicotine dependence (McClernon et al., 2008). In the current study, functional connectivity between mSFG and lingual gyrus as well as PHG was decreased, and it was negatively correlated with FTND scores. These reflected a disruption of the integrity of functional connectivity between cognitive control and visual episodic memory in smokers, and that the greater the nicotine dependence, the weaker the functional connectivity.
We also noticed that cigarette smoking had a particularly prominent effect on the functional connectivity of precuneus. The precuneus, involved in personal value attribution and emotion regulation (Zhang and Volkow, 2019), is thought to be an important component of the default mode network (DMN). Increasing evidence suggested that deregistration of DMN and interactions with other networks in substance use disorder (SUD) impairs the cognitive and affective processes that lead to craving and relapse (Li et al., 2014; Ding et al., 2015; Wang et al., 2016; Hackshaw et al., 2018). Previous studies have shown that reduced spontaneous neural activity in the precuneus is associated with long-term smoking (Wang et al., 2017). As subjects examined episodic memory, understood thoughts or behaviors of other people, appraised emotional information, and reflected on themselves, the DMN was actively engaged (Buckner et al., 2008; Spreng et al., 2009; Andrews-Hanna, 2012). The reduced functional connectivity between SFG and the precuneus in the current study may indicate that the subconscious of smokers is unable to identify the significance of external or internal factors that are influential to themselves, such as feedback on continuous smoking behavior (Konova et al., 2013). Emotional regulated cognitive control and decision-making in smokers may be affected by lower connectivity in these areas, meaning that smoking is uncontrolled despite knowing the negative consequences.
Another interesting result of this study was the attenuated functional connectivity between SFG, mSFG and cerebellar subregions including cerebellum 4 5, cerebellum 6 and cerebellum 8. The neural basis of cigarette smoking was thought to be mainly related to dopaminergic neural circuits composed of the prefrontal cortex, insula, striatum and so on (Yang et al., 2020). While the cerebellum is widely believed to be vital for coordinating movement, maintaining posture and balance, there is growing evidence that its role in emotional regulation, attentional processing and decision making cannot be ignored (Sathyanesan et al., 2019). The cerebellum has a variety of nicotinic acetylcholine receptor (nAChR) subtypes that are sensitive to nicotine sensitization (Paterson and Nordberg, 2000; Turner and Kellar, 2005). The excitotoxic effects of nicotine can cause cell loss in the cerebellum, especially Purkinje and granular neurons (Chen et al., 2003). Durazzo et al. (2004) found the effect of long-term smoking on choline concentrations in the cerebellum. The cerebellum has also been thought to be part of the addiction circuit (Miquel et al., 2016). Miquel et al found that drug-induced functional and structural alterations in the cerebellum were central to the transition from a pattern of recreational drug use to a compulsive behavioral phenotype (Miquel et al., 2009). In the cerebellum, several subregions are capable of projecting (receiving) signals to (from) the cerebral cortex (Bostan et al., 2013). According to cerebellar networks theory, interactions with the cerebral cortex may represent neural mechanism for cerebellar involvement in cue-induced craving and addiction (Bostan et al., 2013). Our results suggested that some cerebellar subregions (such as cerebellum 4 5, cerebellum 6 and cerebellum 8) are functionally connected to brain regions involved in cognitive and executive control (SFG and mSFG). As a consequence, attenuated functional connectivity between SFG, mSFG and cerebellar subregions might contribute to dysfunction associated with chronic smokers.
There are still several limitations in this study. First and foremost, the sample size of the study is relatively small, which may reduce the strength of our results. Secondly, previous studies have shown gender and racial differences in brain function in smokers (Bagga et al., 2018; McCarthy et al., 2019; Lin et al., 2021). The participants included in the current study were all Chinese males, so the results of this study may not account for all smokers. Finally, this study was cross-sectional. We will use longitudinal data to confirm and complement the brain functional alterations in smokers in the future.
5. Conclusion
In summary, our study combined amplitude of low frequency fluctuation (ALFF) and resting-state functional connectivity (rs-FC) to identify the alterations of intrinsic brain activity and neural connectivity abnormalities in smokers. The present study found increased ALFF in SFG with reduced functional connectivity to visual attention areas including the calcarine sulcus, lingual gyrus and fusiform gyrus, indicating impaired neural communication between the cognitive control function and the visual attention network, which perhaps played a significant role in the maintenance of cigarette smoking. Besides, our findings noted the role of the cerebellum in tobacco addiction extending previous findings that have primarily focused on changes in cerebrum. Overall, combing two different functional indicators to probe the alterations of neural activity in the resting state of smokers could provide a deeper insight into the neural mechanism of cigarette smoking.
Data availability statement
The datasets presented in this article are not readily available because of the privacy of all the participants. Requests to access the datasets should be directed to YZ, zzuzhangyong2013@163.com.
Ethics statement
The studies involving human participants were reviewed and approved by the Local Medical Ethics Committee of the First Affiliated Hospital of Zhengzhou University. The patients/participants provided their written informed consent to participate in this study.
Author contributions
XN, YZ, XG, MZ, and QL conceived and designed the study. XN, XG, MZ, JD, and JS collected the data. XN analyzed the data, performed the statistical study, and drafted the manuscript. YZ, JC, SH, QL, and YW revised the manuscript. All authors contributed to the article and approved the submitted version.
Funding
This study was supported by the Natural Science Foundation of China (81601467 and 81871327) and Medical Science and Technology Research Project of Henan Province (201701011).
Conflict of interest
The authors declare that the research was conducted in the absence of any commercial or financial relationships that could be construed as a potential conflict of interest.
Publisher’s note
All claims expressed in this article are solely those of the authors and do not necessarily represent those of their affiliated organizations, or those of the publisher, the editors and the reviewers. Any product that may be evaluated in this article, or claim that may be made by its manufacturer, is not guaranteed or endorsed by the publisher.
References
Andrews-Hanna, J. (2012). The brain’s default network and its adaptive role in internal mentation. Neuroscientist 18, 251–270. doi: 10.1177/1073858411403316
Asma, S., Song, Y., Cohen, J., Eriksen, M., Pechacek, T., Cohen, N., et al. (2014). CDC grand rounds: Global tobacco control. MMWR 63, 277–280.
Bagga, D., Aigner, C., Cecchetto, C., Fischmeister, F., and Schöpf, V. (2018). Investigating sex-specific characteristics of nicotine addiction using metabolic and structural magnetic resonance imaging. Eur. Addict. Res. 24, 267–277. doi: 10.1159/000494260
Bezdek, M., Gerrig, R., Wenzel, W., Shin, J., Pirog Revill, K., and Schumacher, E. (2015). Neural evidence that suspense narrows attentional focus. Neuroscience 303, 338–345. doi: 10.1016/j.neuroscience.2015.06.055
Biswal, B., Yetkin, F., Haughton, V., and Hyde, J. (1995). Functional connectivity in the motor cortex of resting human brain using echo-planar MRI. Magn. Reson. Med. 34, 537–541. doi: 10.1002/mrm.1910340409
Bostan, A., Dum, R., and Strick, P. (2013). Cerebellar networks with the cerebral cortex and basal ganglia. Trends Cogn. Sci. 17, 241–254. doi: 10.1016/j.tics.2013.03.003
Brody, A. (2006). Functional brain imaging of tobacco use and dependence. J. Psychiatr. Res. 40, 404–418. doi: 10.1016/j.jpsychires.2005.04.012
Buckner, R., Andrews-Hanna, J., and Schacter, D. (2008). The brain’s default network: Anatomy, function, and relevance to disease. Ann. N. Y. Acad. Sci. 1124, 1–38. doi: 10.1196/annals.1440.011
Buschschulte, A., Boehler, C., Strumpf, H., Stoppel, C., Heinze, H., Schoenfeld, M., et al. (2014). Reward- and attention-related biasing of sensory selection in visual cortex. J. Cogn. Neurosci. 26, 1049–1065. doi: 10.1162/jocn_a_00539
Chen, W., Edwards, R., Romero, R., Parnell, S., and Monk, R. (2003). Long-term nicotine exposure reduces Purkinje cell number in the adult rat cerebellar vermis. Neurotoxicol. Teratol. 25, 329–334. doi: 10.1016/s0892-0362(02)00350-1
Chu, S., Xiao, D., Wang, S., Peng, P., Xie, T., He, Y., et al. (2014). Spontaneous brain activity in chronic smokers revealed by fractional amplitude of low frequency fluctuation analysis: A resting state functional magnetic resonance imaging study. Chin. Med. J. 127, 1504–1509.
Claus, E. D., Blaine, S., Filbey, F., Mayer, A., and Hutchison, K. (2013). Association between nicotine dependence severity, BOLD response to smoking cues, and functional connectivity. Neuropsychopharmacology 38, 2363–2372. doi: 10.1038/npp.2013.134
Crottaz-Herbette, S., and Menon, V. (2006). Where and when the anterior cingulate cortex modulates attentional response: Combined fMRI and ERP evidence. J. Cogn. Neurosci. 18, 766–780. doi: 10.1162/jocn.2006.18.5.766
Davachi, L., Mitchell, J., and Wagner, A. (2003). Multiple routes to memory: Distinct medial temporal lobe processes build item and source memories. Proc. Natl. Acad. Sci. U. S. A. 100, 2157–2162. doi: 10.1073/pnas.0337195100
Diana, R., Yonelinas, A., and Ranganath, C. (2010). Medial temporal lobe activity during source retrieval reflects information type, not memory strength. J. Cogn. Neurosci. 22, 1808–1818. doi: 10.1162/jocn.2009.21335
Ding, X., Yang, Y., Stein, E., and Ross, T. (2015). Multivariate classification of smokers and nonsmokers using SVM-RFE on structural MRI images. Hum. Brain Mapp. 36, 4869–4879. doi: 10.1002/hbm.22956
Domino, E., Minoshima, S., Guthrie, S., Ohl, L., Ni, L., Koeppe, R., et al. (2000). Effects of nicotine on regional cerebral glucose metabolism in awake resting tobacco smokers. Neuroscience 101, 277–282. doi: 10.1016/s0306-4522(00)00357-2
Dong, H., Wang, M., Zheng, H., Zhang, J., and Dong, G. (2021). The functional connectivity between the prefrontal cortex and supplementary motor area moderates the relationship between internet gaming disorder and loneliness. Prog. Neuropsychopharmacol. Biol. Psychiatry 108:110154. doi: 10.1016/j.pnpbp.2020.110154
Due, D., Huettel, S., Hall, W., and Rubin, D. (2002). Activation in mesolimbic and visuospatial neural circuits elicited by smoking cues: Evidence from functional magnetic resonance imaging. Am. J. Psychiatry 159, 954–960. doi: 10.1176/appi.ajp.159.6.954
Durazzo, T., Gazdzinski, S., Banys, P., and Meyerhoff, D. (2004). Cigarette smoking exacerbates chronic alcohol-induced brain damage: A preliminary metabolite imaging study. Alcohol. Clin. Exp. Res. 28, 1849–1860. doi: 10.1097/01.alc.0000148112.92525.ac
Ekstrom, A., Kahana, M., Caplan, J., Fields, T., Isham, E., Newman, E., et al. (2003). Cellular networks underlying human spatial navigation. Nature 425, 184–188. doi: 10.1038/nature01964
Epstein, R., and Kanwisher, N. (1998). A cortical representation of the local visual environment. Nature 392, 598–601. doi: 10.1038/33402
Franklin, T., Wang, Z., Wang, J., Sciortino, N., Harper, D., Li, Y., et al. (2007). Limbic activation to cigarette smoking cues independent of nicotine withdrawal: A perfusion fMRI study. Neuropsychopharmacology 32, 2301–2309. doi: 10.1038/sj.npp.1301371
Friston, K., Frith, C., Fletcher, P., Liddle, P., and Frackowiak, R. (1996). Functional topography: Multidimensional scaling and functional connectivity in the brain. Cereb. Cortex 6, 156–164. doi: 10.1093/cercor/6.2.156
Gehring, W., and Fencsik, D. (2001). Functions of the medial frontal cortex in the processing of conflict and errors. J. Neurosci. 21, 9430–9437. doi: 10.1523/JNEUROSCI.21-23-09430.2001
Goldfarbmuren, K., Jackson, N., Sajuthi, S., Dyjack, N., Li, K., Rios, C., et al. (2020). Dissecting the cellular specificity of smoking effects and reconstructing lineages in the human airway epithelium. Nat. Commun. 11:2485. doi: 10.1038/s41467-020-16239-z
Hackshaw, A., Morris, J., Boniface, S., Tang, J., and Milenkoviæ, D. (2018). Low cigarette consumption and risk of coronary heart disease and stroke: Meta-analysis of 141 cohort studies in 55 study reports. BMJ 360:j5855. doi: 10.1136/bmj.j5855
Han, S., Xu, Y., Guo, H., Fang, K., Wei, Y., Liu, L., et al. (2022). Two distinct subtypes of obsessive compulsive disorder revealed by a framework integrating multimodal neuroimaging information. Hum. Brain Mapp. 43, 4254–4265. doi: 10.1002/hbm.25951
He, H., Pan, L., Cui, Z., Sun, J., Yu, C., Cao, Y., et al. (2020). Smoking prevalence, patterns, and cessation among adults in hebei province, central china: Implications from china national health survey (CNHS). Front. Public Health 8:177. doi: 10.3389/fpubh.2020.00177
He, L., Wei, D., Yang, F., Zhang, J., Cheng, W., Feng, J., et al. (2021). Functional connectome prediction of anxiety related to the COVID-19 pandemic. Am. J. Psychiatry 178, 530–540. doi: 10.1176/appi.ajp.2020.20070979
Heatherton, T., Kozlowski, L., Frecker, R., and Fagerström, K. (1991). The fagerström test for nicotine dependence: A revision of the fagerström tolerance questionnaire. Br. J. Addict. 86, 1119–1127. doi: 10.1111/j.1360-0443.1991.tb01879.x
Hyman, S., Malenka, R., and Nestler, E. (2006). Neural mechanisms of addiction: The role of reward-related learning and memory. Annu. Rev. Neurosci. 29, 565–598. doi: 10.1146/annurev.neuro.29.051605.113009
Jamal, A., Phillips, E., Gentzke, A., Homa, D., Babb, S., King, B., et al. (2018). Current cigarette smoking among adults - United States, 2016. MMWR 67, 53–59. doi: 10.15585/mmwr.mm6702a1
Jiang, G., Qiu, Y., Zhang, X., Han, L., Lv, X., Li, L., et al. (2011). Amplitude low-frequency oscillation abnormalities in the heroin users: A resting state fMRI study. Neuroimage 57, 149–154. doi: 10.1016/j.neuroimage.2011.04.004
Jung, J., Kang, J., Won, E., Nam, K., Lee, M., Tae, W., et al. (2014). Impact of lingual gyrus volume on antidepressant response and neurocognitive functions in Major Depressive Disorder: A voxel-based morphometry study. J. Affect. Disord. 169, 179–187. doi: 10.1016/j.jad.2014.08.018
Kanwisher, N., McDermott, J., and Chun, M. (1997). The fusiform face area: A module in human extrastriate cortex specialized for face perception. J. Neurosci. 17, 4302–4311. doi: 10.1523/JNEUROSCI.17-11-04302.1997
Konova, A., Moeller, S., and Goldstein, R. (2013). Common and distinct neural targets of treatment: Changing brain function in substance addiction. Neurosci. Biobehav. Rev. 37, 2806–2817. doi: 10.1016/j.neubiorev.2013.10.002
Li, M., Tian, J., Zhang, R., Qiu, Y., Wen, X., Ma, X., et al. (2014). Abnormal cortical thickness in heroin-dependent individuals. Neuroimage 88, 295–307. doi: 10.1016/j.neuroimage.2013.10.021
Li, W., Qin, W., Liu, H., Fan, L., Wang, J., Jiang, T., et al. (2013). Subregions of the human superior frontal gyrus and their connections. Neuroimage 78, 46–58. doi: 10.1016/j.neuroimage.2013.04.011
Lin, F., Han, X., Wang, Y., Ding, W., Sun, Y., Zhou, Y., et al. (2021). Sex-specific effects of cigarette smoking on caudate and amygdala volume and resting-state functional connectivity. Brain Imaging Behav. 15, 1–13. doi: 10.1007/s11682-019-00227-z
Lu, H., Zuo, Y., Gu, H., Waltz, J., Zhan, W., Scholl, C., et al. (2007). Synchronized delta oscillations correlate with the resting-state functional MRI signal. Proc. Natl. Acad. Sci. U. S. A. 104, 18265–18269. doi: 10.1073/pnas.0705791104
Luo, Q., Mitchell, D., Jones, M., Mondillo, K., Vythilingam, M., and Blair, R. (2007). Common regions of dorsal anterior cingulate and prefrontal-parietal cortices provide attentional control of distracters varying in emotionality and visibility. Neuroimage 38, 631–639. doi: 10.1016/j.neuroimage.2007.07.051
Mantini, D., Perrucci, M., Del Gratta, C., Romani, G., and Corbetta, M. (2007). Electrophysiological signatures of resting state networks in the human brain. Proc. Natl. Acad. Sci. U. S. A. 104, 13170–13175. doi: 10.1073/pnas.0700668104
Marks, K., Alcorn, J., Stoops, W., and Rush, C. (2016). Cigarette cue attentional bias in cocaine-smoking and non-cocaine-using cigarette smokers. Nicotine Tob. Res. 18, 1915–1919. doi: 10.1093/ntr/ntw026
McCarthy, J., Dumais, K., Zegel, M., Pizzagalli, D., Olson, D., Moran, L., et al. (2019). Sex differences in tobacco smokers: Executive control network and frontostriatal connectivity. Drug Alcohol Depend. 195, 59–65. doi: 10.1016/j.drugalcdep.2018.11.023
McClernon, F., Kozink, R., and Rose, J. (2008). Individual differences in nicotine dependence, withdrawal symptoms, and sex predict transient fMRI-BOLD responses to smoking cues. Neuropsychopharmacology 33, 2148–2157. doi: 10.1038/sj.npp.1301618
Miller, E. (2000). The prefrontal cortex and cognitive control. Nat. Rev. Neurosci. 1, 59–65. doi: 10.1038/35036228
Miquel, M., Toledo, R., García, L., Coria-Avila, G., and Manzo, J. (2009). Why should we keep the cerebellum in mind when thinking about addiction? Curr. Drug Abuse Rev. 2, 26–40. doi: 10.2174/1874473710902010026
Miquel, M., Vazquez-Sanroman, D., Carbo-Gas, M., Gil-Miravet, I., Sanchis-Segura, C., Carulli, D., et al. (2016). Have we been ignoring the elephant in the room? Seven arguments for considering the cerebellum as part of addiction circuitry. Neurosci. Biobehav. Rev. 60, 1–11. doi: 10.1016/j.neubiorev.2015.11.005
Paterson, D., and Nordberg, A. (2000). Neuronal nicotinic receptors in the human brain. Prog. Neurobiol. 61, 75–111. doi: 10.1016/s0301-0082(99)00045-3
Pourtois, G., Vocat, R., N’diaye, K., Spinelli, L., Seeck, M., and Vuilleumier, P. (2010). Errors recruit both cognitive and emotional monitoring systems: Simultaneous intracranial recordings in the dorsal anterior cingulate gyrus and amygdala combined with fMRI. Neuropsychologia 48, 1144–1159. doi: 10.1016/j.neuropsychologia.2009.12.020
Power, J., Barnes, K., Snyder, A., Schlaggar, B., and Petersen, S. (2012). Spurious but systematic correlations in functional connectivity MRI networks arise from subject motion. Neuroimage 59, 2142–2154. doi: 10.1016/j.neuroimage.2011.10.018
Rolls, E. (2004). The functions of the orbitofrontal cortex. Brain Cogn. 55, 11–29. doi: 10.1016/S0278-2626(03)00277-X
Sathyanesan, A., Zhou, J., Scafidi, J., Heck, D., Sillitoe, R., and Gallo, V. (2019). Emerging connections between cerebellar development, behaviour and complex brain disorders. Nat. Rev. Neurosci. 20, 298–313. doi: 10.1038/s41583-019-0152-2
Sergent, J., Ohta, S., and MacDonald, B. (1992). Functional neuroanatomy of face and object processing. A positron emission tomography study. Brain 115, 15–36. doi: 10.1093/brain/115.1.15
Shen, Z., Huang, P., Qian, W., Wang, C., Yu, H., Yang, Y., et al. (2016). Severity of dependence modulates smokers’ functional connectivity in the reward circuit: A preliminary study. Psychopharmacology 233, 2129–2137. doi: 10.1007/s00213-016-4262-5
Sohn, M., Albert, M., Jung, K., Carter, C., and Anderson, J. (2007). Anticipation of conflict monitoring in the anterior cingulate cortex and the prefrontal cortex. Proc. Natl. Acad. Sci. U. S. A. 104, 10330–10334. doi: 10.1073/pnas.0703225104
Spreng, R., Mar, R., and Kim, A. (2009). The common neural basis of autobiographical memory, prospection, navigation, theory of mind, and the default mode: A quantitative meta-analysis. J. Cogn. Neurosci. 21, 489–510. doi: 10.1162/jocn.2008.21029
Stevens, W., Kahn, I., Wig, G., and Schacter, D. (2012). Hemispheric asymmetry of visual scene processing in the human brain: Evidence from repetition priming and intrinsic activity. Cereb. Cortex 22, 1935–1949. doi: 10.1093/cercor/bhr273
Thomas, D. (2014). Invited commentary: Is it time to retire the ′pack-years″ variable? Maybe not!. Am. J. Epidemiol. 179, 299–302. doi: 10.1093/aje/kwt274
Turner, J., and Kellar, K. (2005). Nicotinic cholinergic receptors in the rat cerebellum: Multiple heteromeric subtypes. J. Neurosci. 25, 9258–9265. doi: 10.1523/JNEUROSCI.2112-05.2005
Ursu, S., Clark, K., Aizenstein, H., Stenger, V., and Carter, C. (2009). Conflict-related activity in the caudal anterior cingulate cortex in the absence of awareness. Biol. Psychol. 80, 279–286. doi: 10.1016/j.biopsycho.2008.10.008
Wang, C., Shen, Z., Huang, P., Qian, W., Zhou, C., Li, K., et al. (2020). Increased interregional functional connectivity of anterior insula is associated with improved smoking cessation outcome. Brain Imaging Behav. 14, 408–415. doi: 10.1007/s11682-019-00197-2
Wang, C., Shen, Z., Huang, P., Yu, H., Qian, W., Guan, X., et al. (2017). Altered spontaneous brain activity in chronic smokers revealed by fractional ramplitude of low-frequency fluctuation analysis: A preliminary study. Sci. Rep. 7:328. doi: 10.1038/s41598-017-00463-7
Wang, L., Zhang, Y., Lin, X., Zhou, H., Du, X., and Dong, G. (2018). Group independent component analysis reveals alternation of right executive control network in Internet gaming disorder. CNS Spectr. 23, 300–310. doi: 10.1017/S1092852917000360
Wang, L., Zou, F., Zhai, T., Lei, Y., Tan, S., Jin, X., et al. (2016). Abnormal gray matter volume and resting-state functional connectivity in former heroin-dependent individuals abstinent for multiple years. Addict. Biol. 21, 646–656. doi: 10.1111/adb.12228
Wang, S., Rao, B., Chen, L., Chen, Z., Fang, P., Miao, G., et al. (2021). Using fractional amplitude of low-frequency fluctuations and functional connectivity in patients with post-stroke cognitive impairment for a simulated stimulation program. Front. Aging Neurosci. 13:724267. doi: 10.3389/fnagi.2021.724267
Wang, X., Han, Z., He, Y., Caramazza, A., Song, L., and Bi, Y. (2013). Where color rests: Spontaneous brain activity of bilateral fusiform and lingual regions predicts object color knowledge performance. Neuroimage 76, 252–263. doi: 10.1016/j.neuroimage.2013.03.010
Weiland, B., Sabbineni, A., Calhoun, V., Welsh, R., and Hutchison, K. (2015). Reduced executive and default network functional connectivity in cigarette smokers. Hum. Brain Mapp. 36, 872–882. doi: 10.1002/hbm.22672
Weiner, K., Golarai, G., Caspers, J., Chuapoco, M., Mohlberg, H., Zilles, K., et al. (2014). The mid-fusiform sulcus: A landmark identifying both cytoarchitectonic and functional divisions of human ventral temporal cortex. Neuroimage 84, 453–465. doi: 10.1016/j.neuroimage.2013.08.068
Wen, M., Yang, Z., Wei, Y., Huang, H., Zheng, R., Wang, W., et al. (2021). More than just statics: Temporal dynamic changes of intrinsic brain activity in cigarette smoking. Addict. Biol. 26:e13050. doi: 10.1111/adb.13050
Wilcockson, T., Pothos, E., Osborne, A., and Crawford, T. (2021). Top-down and bottom-up attentional biases for smoking-related stimuli: Comparing dependent and non-dependent smokers. Addict. Behav. 118:106886. doi: 10.1016/j.addbeh.2021.106886
Wu, G., Yang, S., Zhu, L., and Lin, F. (2015). Altered spontaneous brain activity in heavy smokers revealed by regional homogeneity. Psychopharmacology 232, 2481–2489. doi: 10.1007/s00213-015-3881-6
Yang, Z., Wen, M., Wei, Y., Huang, H., Zheng, R., Wang, W., et al. (2022). Alternations in Dynamic and Static Functional Connectivity Density in Chronic Smokers. Front. Psychiatry. 13:843254. doi: 10.3389/fpsyt.2022.843254
Yang, Z., Zhang, Y., Cheng, J., and Zheng, R. (2020). Meta-analysis of brain gray matter changes in chronic smokers. Eur. J. Radiol. 132:109300. doi: 10.1016/j.ejrad.2020.109300
Yu, R., Zhao, L., Tian, J., Qin, W., Wang, W., Yuan, K., et al. (2013). Regional homogeneity changes in heavy male smokers: A resting-state functional magnetic resonance imaging study. Addict. Biol. 18, 729–731. doi: 10.1111/j.1369-1600.2011.00359.x
Zang, Y., He, Y., Zhu, C., Cao, Q., Sui, M., Liang, M., et al. (2007). Altered baseline brain activity in children with ADHD revealed by resting-state functional MRI. Brain Dev. 29, 83–91. doi: 10.1016/j.braindev.2006.07.002
Zhang, M., Gao, X., Yang, Z., Niu, X., Chen, J., Wei, Y., et al. (2022). Weight Status Modulated Brain Regional Homogeneity in Long-Term Male Smokers. Front. Psychiatry 13:857479. doi: 10.3389/fpsyt.2022.857479
Zhang, M., Gao, X., Yang, Z., Wen, M., Huang, H., Zheng, R., et al. (2021). Shared gray matter alterations in subtypes of addiction: A voxel-wise meta-analysis. Psychopharmacology 238, 2365–2379. doi: 10.1007/s00213-021-05920-w
Zhang, R., and Volkow, N. (2019). Brain default-mode network dysfunction in addiction. Neuroimage 200, 313–331. doi: 10.1016/j.neuroimage.2019.06.036
Zhang, R., Shao, R., Xu, G., Lu, W., Zheng, W., Miao, Q., et al. (2019). Aberrant brain structural-functional connectivity coupling in euthymic bipolar disorder. Hum. Brain Mapp. 40, 3452–3463. doi: 10.1002/hbm.24608
Zhou, W., Jin, Y., Meng, Q., Zhu, X., Bai, T., Tian, Y., et al. (2019). A neural circuit for comorbid depressive symptoms in chronic pain. Nat. Neurosci. 22, 1649–1658. doi: 10.1038/s41593-019-0468-2
Keywords: amplitude of low frequency fluctuation (ALFF), functional connectivity (FC), superior frontal gyrus, visual attention, cigarette smoking
Citation: Niu X, Gao X, Lv Q, Zhang M, Dang J, Sun J, Wang W, Wei Y, Cheng J, Han S and Zhang Y (2023) Increased spontaneous activity of the superior frontal gyrus with reduced functional connectivity to visual attention areas and cerebellum in male smokers. Front. Hum. Neurosci. 17:1153976. doi: 10.3389/fnhum.2023.1153976
Received: 30 January 2023; Accepted: 03 March 2023;
Published: 17 March 2023.
Edited by:
Chella Kamarajan, SUNY Downstate Health Sciences University, United StatesReviewed by:
Yan Zhou, Shanghai Jiao Tong University, ChinaFengmei Lu, Chengdu No.4 People’s Hospital, China
Copyright © 2023 Niu, Gao, Lv, Zhang, Dang, Sun, Wang, Wei, Cheng, Han and Zhang. This is an open-access article distributed under the terms of the Creative Commons Attribution License (CC BY). The use, distribution or reproduction in other forums is permitted, provided the original author(s) and the copyright owner(s) are credited and that the original publication in this journal is cited, in accordance with accepted academic practice. No use, distribution or reproduction is permitted which does not comply with these terms.
*Correspondence: Yong Zhang, zzuzhangyong2013@163.com; Shaoqiang Han, shaoqiangHan@163.com; Jingliang Cheng, cjr.chjl@vip.163.com