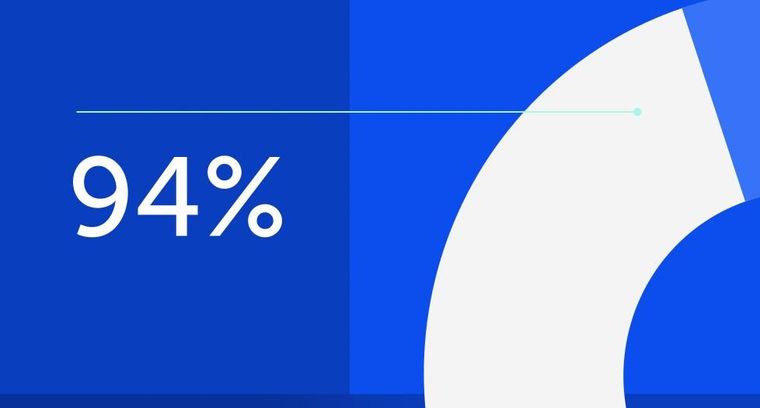
94% of researchers rate our articles as excellent or good
Learn more about the work of our research integrity team to safeguard the quality of each article we publish.
Find out more
REVIEW article
Front. Hum. Neurosci., 23 March 2023
Sec. Brain-Computer Interfaces
Volume 17 - 2023 | https://doi.org/10.3389/fnhum.2023.1085173
This article is part of the Research TopicMethods and Applications in Brain-Computer InterfacesView all 9 articles
Emerging brain technologies have significantly transformed human life in recent decades. For instance, the closed-loop brain-computer interface (BCI) is an advanced software-hardware system that interprets electrical signals from neurons, allowing communication with and control of the environment. The system then transmits these signals as controlled commands and provides feedback to the brain to execute specific tasks. This paper analyzes and presents the latest research on closed-loop BCI that utilizes electric/magnetic stimulation, optogenetic, and sonogenetic techniques. These techniques have demonstrated great potential in improving the quality of life for patients suffering from neurodegenerative or psychiatric diseases. We provide a comprehensive and systematic review of research on the modalities of closed-loop BCI in recent decades. To achieve this, the authors used a set of defined criteria to shortlist studies from well-known research databases into categories of brain stimulation techniques. These categories include deep brain stimulation, transcranial magnetic stimulation, transcranial direct-current stimulation, transcranial alternating-current stimulation, and optogenetics. These techniques have been useful in treating a wide range of disorders, such as Alzheimer's and Parkinson's disease, dementia, and depression. In total, 76 studies were shortlisted and analyzed to illustrate how closed-loop BCI can considerably improve, enhance, and restore specific brain functions. The analysis revealed that literature in the area has not adequately covered closed-loop BCI in the context of cognitive neural prosthetics and implanted neural devices. However, the authors demonstrate that the applications of closed-loop BCI are highly beneficial, and the technology is continually evolving to improve the lives of individuals with various ailments, including those with sensory-motor issues or cognitive deficiencies. By utilizing emerging techniques of stimulation, closed-loop BCI can safely improve patients' cognitive and affective skills, resulting in better healthcare outcomes.
Until recently, controlling one's environment through mental activity or by sending information to the human brain was only an artifact of science fiction. However, recent advances in brain computer interface (BCI) technology have turned this into reality. BCI allows humans to exchange information with their environment by using the electrical signals of brain activity to control any external device. It is a rapidly emerging field for developing integrated software–hardware systems that enable users to send real-time neural commands to any external device via the Artificial Intelligence-based interpretation of brain activity. The BCIs system creates an information pathway between the brain and the world by interpreting the relevant patterns of neural activity during cognitive or affective brain processes. Further, it allows for bidirectional information flow by reading and sending information to and from the brain. BCI applications aim to support, enhance, and restore human cognitive abilities, such as sensorimotor functions (Krucoff et al., 2016). In layman's terms, BCI enables people to control machines with their thoughts. It can empower people who are incapable of speaking, seeing, hearing, or moving their limbs to directly communicate with computers by essentially bypassing the normal central nervous system (CNS) pathway by using only their brain activity. Thus, closed-loop BCIs are changing the concept of restoration and rehabilitation by linking neural activity with the environment, and providing the external regulation or the self-regulation of brain functions by using many types of feedback. For example, closed-loop BCI has various applications in advanced neuroprosthetics (Pan et al., 2020) and neurofeedback training/therapy (Lotte, 2012). These closed-loop BCI systems enable reading and writing from and to the CNS, and are vital for treating neurological disorders, movement disorders, epilepsy, and memory disorders as well as for stroke rehabilitation (Lee et al., 2019). They act on central and peripheral structures, such as the cranial nerves (vagus) (Uthman et al., 1993; Ben-Menachem et al., 1994; Tatum and Helmers, 2009; Ogbonnaya et al., 2013; Johnson and Wilson, 2018); and cortical (Morrell, 2011; Heck et al., 2014; Sun and Morrell, 2014; Lee et al., 2015) and subcortical structures (Salanova et al., 2015) of the brain.
Standard BCIs can be broadly classified according to electrode placement as (1) non-invasive, (2) partially invasive, and (3) invasive. Non-invasive BCIs record signals from electrodes placed on the scalp, e.g., electroencephalography (EEG) (Wolpaw et al., 2002; Wolpaw and McFarland, 2004). Partially invasive BCIs involve electrodes planted inside the skull via craniotomy but external to the brain [e.g., intracranial EEG (iEEG); Wang et al., 2016]. Invasive BCIs use microelectrodes directly placed into the gray matter to capture the signals from neurons [e.g., electrocorticography (ECoG); Milekovic et al., 2012; Hammer et al., 2013, 2016]. By using EEG decoding, synchronous and asynchronous control and communication are established by the BCIs. These non-invasive neural systems or EEG-based BCIs are further based on two categories of brain activity: “evoked” and “spontaneous.” In “evoked” BCIs, the brain generates an immediate automated response to an external stimulus. In “spontaneous BCIs,” the EEG records brain activity associated with mental tasks performed according to the user's volition. For example, P300 and the steady-state visually evoked potential (SSVEP) are based on the “evoked” potential (Chamola et al., 2020). By contrast, motor imagery (MI) is the process by which an individual stimulates a physical reaction via mental stimulation (Pfurtscheller and Neuper, 2006). Another method of classifying BCI is based on the presence or absence of opened-loop and closed-loop feedback. Open-loop adaptive systems do not involve user feedback, and use measurements of the state of BCI users as an implicit input to execute adaptation without giving them the right to correct/adjust their actions. By contrast, closed-loop BCI is an adaptive system that uses simple or complex neurofeedback to analyze brain processes and initiate neuroplasticity, or modulate and enhance brain activity by using techniques of brain stimulation. These techniques have been used for many therapeutic applications [e.g., cranial electrotherapy stimulation (CES), deep brain stimulation (DBS), transcranial direct-current stimulation (tDCS), electroconvulsive therapy (ECT), low-field magnetic stimulation (LFMS), functional electrical stimulation (FES), magnetic seizure therapy (MST), vagus nerve stimulation (VNS), deep transcranial magnetic stimulation (Deep TMS), and responsive nerve stimulation (RNS)].
Therefore, a technology known as closed-loop brain-computer interface based brain stimulation has the potential to be utilized in a wide variety of medical contexts. It has the potential to completely change how neurologists, psychiatrists, and other medical professionals diagnose, treat, and manage neurological and mental health conditions. It is essential to do research on this technology to get an awareness of the advantages and disadvantages it may present, as well as to identify the most effective applications for it. Patients suffering from neurological and mental health conditions may be able to speak with the help of BCI-based brain stimulation, which is one of the possible benefits of this type of brain stimulation. Without having to rely on verbal communication, this might make it possible for medical professionals to identify and treat the aforementioned illnesses. Patients who have impairments may be able to regain some amount of independence as a result of this treatment option.
Hence, this systematic review aims to explore the potential benefits and publication trends with close-loop BCI-based brain stimulation in neurodegenerative and psychiatric diseases. Get a comprehensive grasp of the ways in which various forms of brain stimulation influence brain function as well as behavior.
The most advanced BCI systems use “closed-loop” strategies in which the implanted devices have in-built read–response mechanisms and embedded algorithms that automatically adjust simulation-related factors to match the patient's needs (Lee et al., 2015). These devices sense the composition of the patient and stimulate signals only when required, thereby reducing the side-effects during treatment. This can help conserve power and thus minimize battery replacement surgeries. These systems profoundly impact the clinical pathways of patients with complex nervous system diseases. This strategy has been used for mobility assistance—for example, for wheelchair control or rehabilitation purposes, such as for controlling an electrical stimulator.
A closed-loop BCI system usually involves a control paradigm, measurement, processing, prediction, and feedback from the application (Ahn et al., 2014) as shown in Figure 1. These functions help understand or modulate the user's mental condition or intention. The system uses these data to execute some predefined functions to interact with the environment by seeing, hearing, or sensing the action. An external device provides communication and neurofeedback, which enables the user to determine how well they can control the device. Closed-loop BCI can signal to the brain (e.g., through feedback via electrical/magnetic stimulation, and optogenetic and sonogenetic techniques) to correct the action or obtain additional information about it.
The control paradigm is the input provided by the BCI user to generate brain signals related to their intent. The user generates this input via mental tasks, including the kinematical/visual imagining of the physical movement of a body part, or by concentrating on a specific object to generate a P300 wave. Some BCI systems (e.g., affective BCI) do not require the users' intentions. Still, they function by identifying the emotional and mental statuses of the user, and can be active, reactive, or passive (Gürkök et al., 2012). In any case, these generated signals must be measured via invasive or noninvasive techniques. Invasive techniques, including ECoG, microelectrode arrays, and single microelectrodes, can detect signals from the brain's surface and produce high-quality signals. However, these techniques require risky implantation surgeries.
Therefore, BCI research commonly uses noninvasive methods to detect signals (e.g., magnetoencephalography and functional-magnetic resonance imaging). EEG is the most popular and preferred technique in this regard (Nicolas-Alonso and Gomez-Gil, 2012) as it is economical and portable, and can even be measured by using wireless devices. The most popular closed-loop BCI is the one based on motor imagery, where the disabled can receive a piece of additional sensory information from the BCI device. This BCI-based control system of closed-loop brain stimulation can help sense the effect of a stimulus and adjust this stimulation in response to the observed effect. The closed-loop BCI can be used to create synaptic plasticity through spike-triggered stimulation.
BCIs allow users to communicate with external or control any external devices, such as robotic arms, with their thoughts by monitoring brain activity from the motor cortex and decoding movement intentions using machine learning techniques which leads to significantly improve the quality of life of healthy and unhealthy people such as patients with spinal cord injuries or other paralysis to restore some motor functions (Gao Q. et al., 2017; Belkacem et al., 2018, 2020; Al-Nuaimi et al., 2020; Belkacem, 2020; Shao et al., 2020; Chen et al., 2021a,b; Jamil et al., 2021). Closed-loop brain stimulation can also assist motor learning and enhance the recovery of motor function in stroke patients and those with motor deficits (Xu et al., 2013).
BCIs and closed-loop brain stimulation can be used to treat a variety of neurological and psychiatric conditions, such as Parkinson's disease, epilepsy, depression, and obsessive-compulsive disorder (OCD) (Liang et al., 2010; Widge and Moritz, 2016; Arlotti et al., 2021; Sani et al., 2021). For instance, in Parkinson's disease, closed-loop brain stimulation can administer electrical stimulation to the brain in response to aberrant neural activity, alleviating symptoms such as tremors and stiffness. By detecting seizure activity and applying focused brain stimulation to avoid seizures, BCIs can also treat epilepsy.
Moreover, closed-loop BCI can improve cognitive performance in healthy persons. BCIs can increase attention and working memory, for instance, by monitoring brain activity linked with these cognitive processes and giving the user feedback. By giving tailored brain stimulation during certain cognitive activities, closed-loop brain stimulation can also be utilized to improve cognitive performance (Jamil et al., 2022).
Insomnia and sleep apnea are two examples of sleep problems that can be treated using BCIs and closed-loop brain stimulation. By administering tailored brain stimulation to encourage breathing or alertness, closed-loop brain stimulation may be used to detect and respond to sleep-related events such as apnea episodes. This type of brain stimulation can be used to detect and respond to sleep-related events. BCIs may also improve sleep quality by identifying sleep-related neural activity and giving tailored brain stimulation to enhance the sleep state. This can be accomplished by monitoring the neural activity that occurs during sleep (Choi et al., 2020).
The measured brain signals are then processed for the maximum signal-to-noise ratio. The selection is performed via certain algorithms (Bashashati et al., 2007; Roman-Gonzalez, 2012) including those for spatial and spectral filtering, to derive information from these signals, which are then used as inputs for the classification modules. The steps of prediction involve making decisions based on the user's intention or by quantifying their emotional and mental statuses. Machine learning algorithms and artificial neural networks are usually applied for prediction (Bashashati et al., 2007; Al-Ani et al., 2010). Once the user's intention has been determined, this output is used to change the environment. This change is then provided as feedback to the user. Figure 2 shows the control and communication involved in closed-loop brain feedback or stimulation: its methods, applications, and effects.
Figure 2. A brain-computer interface (BCI)-based control/communication of closed-loop brain feedback or stimulation: methods, applications, and effects.
User experience and neural feedback are very important in closed-loop BCIs as they provide information regarding the success of the effort. Furthermore, neurofeedback allows users to improve BCI control through self-regulation and learning. In neurorehabilitation, this feedback is linked to neuroplastic stimulation that can be used to modify and modulate the user's neural activity. Neurofeedback training is the conscious alteration of brain signals by the user (Boyd et al., 2017). The BCI user learns to control their brain activities based on measurements of the brain activity and feedback signals. For example, in case of diseases like stroke, the rhythm of the brain slows down and there is a substantial decrease in cortical activity, resulting in motor and cognitive impairments (Boyd et al., 2017; Kim and Winstein, 2017). Neurofeedback training aims to restore these brain signals to facilitate a more standard state, possibly leading to functional recovery (Remsik et al., 2016). Neurofeedback can be visual, auditory, and tactile. It can be used for various ailments, including depression, anxiety, stress, pain, attention deficit hyperactivity disorder, cognitive impairments, insomnia, schizophrenia, and motor recovery.
Neurofeedback, is a form of biofeedback, a noninvasive therapeutic approach that detects a patient's brain activity and delivers real-time feedback regarding how the brain works. Neurofeedback treatment is used to train patients to control their brain processes by showing them how the brain reacts to certain stimuli. It involves analyzing brain activity and offering instant feedback, frequently via visual or audio signals.
Attention deficit hyperactivity disorder (ADHD), anxiety, depression, and post-traumatic stress disorder (PTSD) have all been treated with neurofeedback, a therapy method that uses real-time brain activity displays to teach people how to regulate their brain activity. Several studies have shown that neurofeedback can assist people with these diseases manage their symptoms and improve their quality of life. For instance, a meta-analysis of trials looking at neurofeedback for ADHD discovered that it significantly reduced hyperactivity/impulsivity and improved attention, which effects persisted over time (Arns et al., 2009). It is important to note, though, that not all studies have found neurofeedback to be effective, and some have found its effects to be either temporary or restricted (Sonuga-Barke et al., 2013).
Auditory feedback (AF) is any audible output that helps the user interact better with the system. It is used during speech learning in infants. AF can be used in BCI training by manipulating the audio input to achieve the target outcome. Two commonly used manipulations are delayed auditory feedback (DAF) and altered auditory feedback (AAF). In DAF, there is no change in the auditory signal, but it is sent to the listener after a short delay. In AAF, some sections of the signal, such as its pitch or structure, are manipulated and then sent back to the listener without delay. DAF has been shown to improve fluency among people who stutter (Yates, 1963; Ryan and Van Kirk, 1974). With regard to AAF, shifts in the pitch and other parameters in the direction opposite to that of vocal compensation occur automatically, with no conscious awareness, in neurologically healthy speakers (Houde and Jordan, 1998; Liu et al., 2011).
Visual and auditory feedback has been used for many BCI modalities (P300 speller, SSVEP, and motor imagery). It can easily cause brain fatigue, dizziness, nausea, and other adverse reactions. Most visual BCIs are based on flickering stimuli, and continuous flickering can cause visual fatigue and reduce the user's comfort. Auditory BCI is not widely used due to its susceptibility to environmental interference and relatively low accuracy. In addition, it cannot protect the user's privacy from surrounding systems. Tactile feedback is an alternative with many advantages over visual/auditory feedback, such as the ability to generate ideal target signals without repeated training. For instance, BCI-based MI (MI-BCI) allows users to communicate via the imaginary movements of their extremities by using a computer.
Although MI-BCI represents a promising strategy for control, it uses visual feedback to teach the user about the system's decisions. This makes it challenging to use with visually interactive tasks. Indeed, MI-BCIs have rarely been used outside the laboratory (Jeunet et al., 2015) dowing to their flawed classification algorithms and the difficulty of sight-based learning. These mechanisms simulate the sensation of tapping as a response to touch. The response is realized via vibrations. However, device operation is not interrupted by tactile feedback (Lukoyanov et al., 2018).
Several studies have explored tactile (vibration) stimulation in MI-BCI (Cincotti et al., 2007; Leeb et al., 2013; Gwak et al., 2014). The replacement of visual feedback by vibrotactile feedback does not inhibit EEG measurements in MI-BCI (Leeb et al., 2013) and thus does not negatively affect the classification accuracy (Cincotti et al., 2007; Leeb et al., 2013). However, this replacement reduces the visuomotor load during the tracking of multiple objects (Gwak et al., 2014). Thus, MI-BCI provides tactile feedback, and pays more attention to the problem and less to the feedback (Cincotti et al., 2007), to ensure a high accuracy of classification.
In the event of an injury or a disease that compromises a particular area of the brain, neural prosthesis (neuroprosthetics) can be used to restore function in the affected area, whether it is motor, sensory, or cognitive. Swann et al. (2018) showed that fully implanted neural prostheses can be used to produce adaptive deep brain stimulation for patients of Parkinson's disease. The nanocognitive device called an “endomyccorhizae-like interface” (the future of neuroprosthetics) was created to improve features of the neural network in people with neurodegenerative diseases such as Alzheimer, Parkinson's, or dementia (Saniotis et al., 2018).
Cochlear implants are the most widely used among neuroprosthetics. Individuals with moderate-to-profound and severe sensorineural hearing loss (SNHL) benefit more from cochlear implants than hearing aids. Cochlear implants directly stimulate the auditory nerve, avoid injured cochlear hair cells, and offer salient coded information for better speech perception (Buchman et al., 2020). When the retina is damaged, it can cause visual problems and lead to total blindness in extreme situations. The retina is the part of the eye linked to the brain. It contains photoreceptors that generate electrical signals from light, which are then transmitted to the brain via the optic nerve. Many other bionic eye systems have been developed in recent years, including artificial silicon retina that uses a silicon chip containing solar-cell microphotodiodes. These photodiodes convert light energy into electrical impulses sent to the brain via the optic nerve (Buchman et al., 2020; Suresh, 2020).
Neurostimulation is the intentional modulation of the activity of the nervous system by using invasive methods, such as microelectrode implantation, or noninvasive techniques, such as transcranial magnetic stimulation (TMS). Neurons in the brain collaborate in vast networks to regulate and coordinate activities of the body, such as seeing, listening, sensing, and feeling to perform actions, and control respiration and pulse. An electrical signal is produced by the neuron whenever it is stimulated, and can be changed through TMS by applying an electromagnetic coil to the scalp. The electromagnet provides a magnetic pulse that activates nerve cells in the mood regulation- and depression-related areas of the brain in a harmless manner. It is thought to stimulate brain areas that have diminished activity in case of depression.
Deep brain stimulation (DBS) is an invasive neurostimulation technique that requires surgery to implant a neurostimulator device that sends electrical signals to specific parts of the brain responsible for body movements. Electrodes are placed on the right and left sides of the brain, and are connected via long wires. They are then are placed under the skin, traveling down the neck, and are connected to a battery-powered stimulator placed under the skin near the chest (Artusi et al., 2018). The patient can use a handheld controller to control the DBS system. The stimulation settings can be adjusted per the patient's condition. DBS can be used to treat both movement-related and psychiatric disorders. Further, it has shown therapeutic success for otherwise treatment-resistant activity-related and affective disorders, such as tremors, dystonia, Parkinson's disease, chronic pain, and psychiatric disorders (such as depression, bipolar disease, obsessive–compulsive disorder, and Tourette's syndrome; Lozano et al., 2019). DBS has recently been considered to regulate action in memory circuits, where this indicates its potential for therapeutic use to treat dementia and Alzheimer's disease (Freund et al., 2009; Kuhn et al., 2015; Mirzadeh et al., 2016). Different DBS targets have been used to treat patients with Alzheimer's disease and yielded promising outcomes, including slower cognitive decline and improved functional brain connectivity (Laxton et al., 2010; Lozano and Lipsman, 2013). As the biological history of neurodegeneration cannot be reversed in humans, DBS may serve as supplementary treatment by controlling memory circuits (Lv et al., 2018). DBS transmits electrical impulses to the area of the brain responsible for movement-related symptoms caused by Parkinson's disease. Electric impulses disrupt these symptoms, resulting in abnormal activity in the brain's circuitry. In people with Parkinson's disease, three brain regions responsible for motion control are targeted by using DBS: the subthalamic nucleus, the globus pallidus internus, and the thalamic ventral intermediate nucleus. Targeting a specific brain area in this case depends on the treatable symptoms. Transcranial direct-current stimulation (tDCS) is a non-invasive technique for brain stimulation widely used in clinical trials for neurological and psychiatric disorders. It can mitigate depression by stimulating nerves of the left dorsolateral prefrontal cortex (Shiozawa et al., 2014).
Optogenetics is a new approach that combines optics and genetics to control the activity of certain neurons. The key element of optogenetics is the use of light. Many studies have implemented optogenetics in models of diseases, such as epilepsy, Alzheimer, Parkinson's, sensory system degeneration, and depression.
Epilepsy is a prevalent neurological illness marked by seizures. Tønnesen et al. (2009) demonstrated that light-induced halorhodopsin activity can suppress epileptiform activity while hyperpolarizing the primary neurons of the hippocampus. The optogenetic activation of hippocampal neurons at 10 or 20 Hz induces seizure-like after-discharges in rats given doses of ketamine and xylazine as anesthesia (Osawa et al., 2013). Furthermore, Paz et al. (2013) made closed-loop devices that can stop seizures by stimulating the brain with light in real time.
With regard to Alzheimer's disease, Wang et al. (2019)demonstrated that optogenetics can be utilized to modulate the neuronal-glial network to improve memory in mice with Alzheimer's disease. In addition, optogenetics has been used to analyze the activation of a particular neural circuit in transgenic mice with the amyloid precursor protein (APP) to determine the causal relationship between synaptic activity and β-amyloid peptide (Aβ) disease (Yamamoto et al., 2015).
Optogenetics was used to examine the graft function and graft–host connection for Parkinson's disease (Steinbeck et al., 2015). Moreover, Magno et al. (2019) revealed dopamine-depleted male mice benefit from optogenetic stimulation of the secondary (M2) motor cortex in case of Parkinson's. Fougère et al. (2021) claimed that the optogenetic stimulation of glutamatergic neurons in the cuneiform nucleus improves locomotion, regulates speed, and elicits limb motions comparable to those seen in intact animals during spontaneous locomotion.
Optogenetics, in conjunction with behavioral paradigms, has frequently been utilized in rats to understand the significance of various types of neurons and their pathways in people with depression (Biselli et al., 2021). An immediate effect can be mediated in the model of depression by laser or LED light to activate the expressed channelrhodopsin to obtain the specific target of dopamine neurons in the ventral tegmental area (VTA) in mice by applying the optogenetic technique (Chaudhury et al., 2013; Tye et al., 2013). Therefore, optogenetics can be used to regulate neurons to cure neurodegenerative and psychiatric diseases.
Recent years have witnessed a rise in interest in targeted ultrasound techniques for neurodegenerative and psychiatric illnesses. Sonogenetics, which is noninvasive and has a high spatial resolution, involves the genetic manipulation of ultrasound-sensitive neurons and their unique responses to it through the development of mechanosensitive receptors. Fan et al. (2021) demonstrated the proof of concept for the therapeutic application of sonogenetics to slow neurodegeneration in animal models of Parkinson's disease. Leinenga and Götz (2015) claimed improvements in Alzheimer's memory tests by using repeated scanning ultrasound treatments on the mouse brain to eliminate Aβ, without requiring any extra therapeutic agent.
Sonogentics technology that combines deep penetration with a regionally focused ultrasound has evolved, with major therapeutic applications to seizure, depression, and Parkinson's disease (Leinenga et al., 2016). Duque et al. (2021) experimentally stimulated neurons in a mammalian brain by using ultrasound and observed the changes in behavior. Ultrasound is one of the effective tools in physiotherapy, surgery, chemotherapy, medication administration, and sonography (Mason, 2011). Neurostimulation improves the quality of life of patients with severe paralysis, sensory loss, and chronic pain. It plays a vital role in neuroprosthetics, such artificial organs as bionic eyes and limbs, and cochlear implants. Further, it can alter disease symptoms in cases where medications cannot be used owing to their severe side-effects. It is also an option for many movement disorders, with relatively minimal side-effects.
This review analyzes and identifies the most recent and relevant research on closed-loop BCI for neurodegenerative and psychiatric diseases based on electric/magnetic stimulations, optogenetics, and sonogenetics techniques.
The research questions (RQs) considered in this review, along with the rationales for them, are shown in Table 1.
This review adhered to the guideline for Preferred Reporting Items for Systematic Reviews and Meta-Analyses (Liberati et al., 2009). The following digital databases were searched: IEEEXplore, Scopus, and PubMed. The search began between early and mid-September 2022, and was constrained to the title of each paper, its abstract, and keywords to lower the number of results. The following was the primary search string used to find the relevant literature: (“closed loop BCI” OR “brain–computer interface” OR “brain–machine interface” OR BCI) AND (sonogenetic* OR optogenetic* OR “deep brain stimulation” OR “transcranial magnetic stimulation” OR “transcranial direct current stimulation” OR TMS OR DBS OR tDCS) AND (vision OR hearing OR motor OR sensory OR dementia OR Alzheimer OR Parkinson OR depression OR anxiety OR “psychiatric disease” OR neurodegenerat*). The search string was customized for each database. We eliminated duplicate publications after checking the titles of the papers. The titles and abstracts of all publications were evaluated to ensure their relevance. To establish the legitimacy of each article, we obtained and screened the complete text of all pertinent papers by using the criteria for inclusion. Figure 3 shows the process of retrieving articles for this review.
Figure 3. The PRISMA flowchart. EC, exclusion criteria; IC, inclusion criteria; n, number of publications.
Articles were included in this review if they met the following inclusion criteria (IC): (IC1) a primary study that used and focused on brain stimulation (TMS, DBS, tDCS, optogenetic, sonogenetic); (IC2) articles that focused on closed-loop models; (IC3) articles that concluded an improvement, enhancement, or the restoration of the brain function of the patients; and (IC4) experiments involving healthy or unhealthy participants or animals.
The exclusion criteria (EC) for this review were as follows: (EC1) articles that were non-peer-reviewed, abstracts, survey paper, and review papers; (EC2) non-English articles; (EC3) articles that focused only on EEG, MEG, ECoG, or fMRI; (EC4) articles that focused on improving or comparing machine learning or some algorithm; (EC5) articles related to ethics or organization; and (EC6) articles that could not be retrieved in full.
A full-text article was obtained for each study that satisfied the inclusion criteria with the assistance of a librarian. We retrieved the features of the articles, such as the following:
• participant, which represents the kind of participants used in the experiment;
• method of brain stimulation, which is the type of neurostimulation for the closed-loop model;
• disease, which is the kind of disease on which the researchers focused; and
• type of disease, either neurodegenerative or psychiatric disease.
This review included 76 articles out of 319 potential studies. When performing a string search in the digital database, EC1 and EC2 were automatically applied. Furthermore, several of the publication titles were manually removed during the screening phase.
The number of papers published annually in the area from 2000 to 2022 is shown in Figure 4. From 2000 to 2009 (excluding 2000 and 2007), there was no publication in the area. However, since 2010, the number of publications rose gradually until 2018. During 2019, the number of publications dramatically dropped to two, is likely multifactorial and complex possibilities such as changes in legislation or constraints on research may have contributed to a decline in 2019 publication totals. Regulatory bodies likely implemented tougher restrictions for closed loop brain stimulation research, resulting in a temporary decline in publications. Additionally, the advent of new study topics or a shift in research goals may have diverted resources away from closed loop brain stimulation research, resulting in a temporary decline in the number of publications. However, there was a substantial increase again starting in 2020, and the number of published papers in 2022 can increase further as the year has not ended. The largest number of published articles were from journals, with 11 papers in 2018. By contrast, the largest number of papers published in conference proceedings in any given year was two.
Figure 5 provides information on the ratio of published papers based on the type of brain stimulation studied. The overall trend of the data shows a steep rise in the ratio of TMS in the published papers, coupled with a decline in papers on tDCS. The most highest was that 27 journals had published these articles in TMS, which is a sharp contrast with only three papers in conferences. We found no publication on sonogenetics for closed-loop BCI.
Figure 5. Publication trend based on brain stimulation. DBS, deep brain stimulation; TMS, transcranial magnetic stimulation; tDCS, transcranial direct current stimulation; tACS, transcranial alternating current stimulation.
The numbers of published papers on different techniques of brain stimulation were different in conferences from those in journals. The largest number of conference papers were related to tDCS (five), followed by TMS (three). No conference paper related to tACS, optogenetics, and sonogenetics had been published. The most popular techniques of brain stimulation for closed loop BCI models from 2000 to 2022 were TMS, tDCS, and DBS.
Figure 6 depicts the distribution of articles with regard to the techniques of brain stimulation used and the types of diseases considered. They latter have been divided into the two clusters listed below:
• neurodegenerative diseases, which are related to conditions caused by the gradual destruction of cells and the nervous system synapses needed for movement, balance, muscle, sensibility, and cognition; and
• psychiatric diseases, which represent mental disorders determined by a mental health expert that significantly impair thoughts, emotions, or behavior. In general, many articles focused more on neurodegenerative diseases than psychiatric diseases, regardless of the method of brain stimulation used (Figure 6). Around 95% of all articles discussed neurodegenerative diseases and 5% discussed psychiatric diseases.
1. Deep brain stimulation (DBS) Among neurodegenerative diseases, Parkinson's disease was the most commonly studied by using DBS. Ten relevant articles were identified: those by Rossi et al. (2007), Little et al. (2013), Heldman et al. (2016), Swann et al. (2017), Castaño-Candamil et al. (2020), Arlotti et al. (2021), Darbin et al. (2022), Merk et al. (2022), and Neumann et al. (2021). This was followed by tremor-related disease, to which four articles were dedicated: those by Thompson et al. (2016), Herron et al. (2017), Neumann et al. (2021), and Swan et al. (2018). While Neumann et al. (2021) also discussed dystonia and tinnitus in the context of DBS. Only one article reported experiments on the motor cortex (Isaacs et al., 2000).
Among psychiatric diseases, only obsessive–compulsive disorder (OCD) was studied by using DBS (Neumann et al., 2021) to investigate the influence of the location of implants in patients on the performance of brain-sensing devices.
2. Transcranial magnetic stimulation (TMS) Problems with the motor cortex have been extensively studied by using TMS, with 18 articles dedicated to the issue: those by Ros et al. (2010), Niazi et al. (2012), Sitaram et al. (2012), Mokienko et al. (2013), Takemi et al. (2013, 2018), Hänselmann et al. (2015), Kaplan et al. (2016), Royter and Gharabaghi (2016), Schildt et al. (2016), Hasegawa et al. (2017), Mashat et al. (2017), Daly et al. (2018), Jochumsen et al. (2018), Syrov et al. (2020), Ding et al. (2021), Grigorev et al. (2021), and Mihelj et al. (2021) for neugodegenerative disease. The second most commonly studied disease by using TMS was stroke, with five articles devoted to it: those by Gharabaghi et al. (2014), Syrov et al. (2019), Cantillo-Negrete et al. (2021), Hayashi et al. (2022), and Liang et al. (2020). Four articles examine the sensorimotor cortex: those by Pichiorri et al. (2011), Niazi et al. (2014), Kraus et al. (2016), and Naros et al. (2020). Vision-related diseases were investigated by two articles: those by Losey et al. (2016) and Liburkina et al. (2018).
Meanwhile, only one article for mental imagery in the context of psychiatric disease (Vasilyev et al., 2017). It proved the correlation between psychological and neurophysiological diseases.
3. Transcranial direct current stimulation (tDCS) Only three neurodegenerative diseases were considered by using tDCS. Twelve articles considered stroke-related diseases: those by Ang et al. (2012, 2015), Kasashima-Shindo et al. (2015), Handiru et al. (2017), Hong et al. (2017), Hu et al. (2018, 2021), Rodríguez-Ugarte et al. (2018a), Mane et al. (2019), Chew et al. (2020), Quiles et al. (2020), and Bigoni et al. (2022). Ten articles used tDCS to study the motor cortex: those by Wei et al. (2013), Dutta et al. (2014), He et al. (2014), Soekadar et al. (2014, 2015), Takeuchi et al. (2015), Naros et al. (2016), Rodriguez-Ugarte et al. (2018), Rodríguez-Ugarte et al. (2018b), and Ortiz et al. (2020). Two articles used it to examine the sensorimotor cortex Baxter et al. (2016, 2017). By contrast, no article on psychiatric diseases used tDCS for close-loop BCI.
4. Transcranial alternating current stimulation (tACS) Only one article considered brain stimulation based on alternating current with a certain frequency in the context of neurodegenerative disease. It demonstrated an enhancement in self-regulation by the brain in terms of neurofeedback based on β oscillations for stroke-related disease (neurodegenerative; Naros and Gharabaghi, 2017). In the context of psychiatric disease, only one article for understanding animal behavior was used this brain stimulation (Márquez-Ruiz et al., 2016).
5. Optogenetic Five articles were classified into the neurodegenerative group. Two articles each were devoted to sensory processing disorders (Zhang et al., 2021; Sun et al., 2022) and vision related disease (Neely et al., 2018; Scheyltjens et al., 2018), respectively. One article considered the motor cortex, and the results showed that mice can identify neuronal activity caused by photostimuli (Abbasi et al., 2018). In the context of psychiatric disease, one article demonstrated the ability of animals to utilize an artificial cerebral channel in a behaviorally significant manner (Prsa et al., 2017).
Figure 6. Distribution of articles selected according to the brain stimulation and type of disease. DBS, deep brain stimulation; TMS, transcranial magnetic stimulation; tDCS, transcranial direct current stimulation; tACS, transcranial alternating current stimulation.
Overall, Figure 6 shows the potential of how the closed-loop BCI based on brain stimulation systems improves the quality of life of patients. Many researchers did experiments based on the type of disease to prove that brain stimulation is one method that can restore, replace, or repair impaired brain functioning and alleviate symptoms in individuals suffering from various neurological disorders. Results have demonstrated that closed-loop DBS is superior to open-loop DBS in symptom management and in reducing adverse effects. For instance, DBS decreased the intensity of tremors in individuals who suffered from essential tremors while simultaneously decreasing the stimulation-induced adverse effects. For example, Liang et al. (2010) demonstrated that closed-loop DBS could detect and suppress epileptic seizures in real-time. Based on Figure 6 also, the selected articles show the significant clinical experiments as the practice to demonstrate the improvement in individuals with a variety of illnesses and conditions. As research into this field develops, it is anticipated that more effective and individualized treatments will become available to patients in the hope that they will have a better quality of life.
Figure 7 was constructed using the metadata from the retrieved articles. The most frequently occurring terms among the 832 keywords are human (56 times) and humans (44 times). These words must be extensively used since they constitute the foundation of the subject (patient) for experiments. This can conclude that many of researchers aims to improve the life for human who have neurodegenerative and psychiatric diseases.
Figure 7. Bibliometric analysis of the appearance of keywords for closed-loop BCI based brain stimulation.
Table 2 presents the mapping between the technique of stimulation used and the participants in the selected studies. Only such animals as mice or rats were used in experiments on optogenetic techniques. Healthy human participants were the most commonly considered, in 41 articles, even though they were recruited in experiments for only TMS and tDCS. By contrast, non-animal was used in TMS and tDCS brain stimulation techniques. Participants with stroke or Parkinson's diseases were the most participants in the experiments for all stimulation techniques except optogenetic.
Table 2. Mapping between participants and stimulation techniques identified in the selected articles.
Table 3 shows examples of studies that focused on different techniques of brain stimulation to improve the quality of life of patients with neurodegenerative and psychiatric diseases based on close-loop BCI. We selected only one example publication for each technique of brain stimulation based on the type of publication (the top journal with the highest impact factor) and the participants involved.
The results of this review show the therapeutic potential of closed-loop BCI systems for improving the quality of life of patients with neurological disorders. During our analysis of closed-loop BCI, we identified the trend of publications on closed-loop BCI within the last decade, along with the use of brain stimulation technology to enhance and improve the life of people with neurodegenerative or psychiatric diseases. The rapid growth of academic research implies that the extent and branches of techniques of brain stimulation are expanding. Closed-loop brain stimulation for neurodegenerative and psychiatric diseases has shown excellent results in clinical tests. It has the potential to enable better management of the symptoms of patients and adverse effects while using less power than is needed for standard open-loop brain stimulation (Fleming et al., 2020). Furthermore, the number of publications as well as recently discussed topics in the context of brain stimulation and closed-loop BCI show that market acceptability and empirical work in the area are rapidly increasing. Based on these findings, we may claim that the increase in scholarly work on closed-loop BCI based on brain stimulation since 2013 reflects the need for effective and safe medical treatment that can automatically modify the settings of the stimulation based on brain activity.
The results in Figure 5 indicate that the researchers' primary objective in this context is to develop novel methodologies or expand current techniques to treat neurodegenerative and mental disorders. Each technique of brain stimulation provides a different way to stimulate nerve cells of the brain. Only DBS and TMS have been approved by the US Food and Drug Administration (FDA). Therefore, many researchers have focused on them in experiments. However, DBS is a minimally invasive surgical treatment that nonetheless entails considerable risk. The insertion of the stimulator is unlikely to cause bleeding or infection in the brain (Larson, 2014). Hence, many studies have focused on noninvasive methods of brain stimulation. Even though tDSC has not yet been approved by the FDA, it is a convenient and portable method of brain stimulation that involves applying a modest amount of electric current to the scalp. Consequently, tDSC simulations accounted for the second most commonly used technique in the articles considered.
Nevertheless, the use of optogenetics and sonogenetics remains rare in closed-loop BCI. Sonogenetic stimulation is the noninvasive manipulation of neurons and other cells carrying exogenous protein channels by using ultrasound technology. However, sonogenetic stimulation is still in doubt due this technique has proved the difficulty to target the certain cells (Sato et al., 2018). Therefore, none of the studies considered here had used it for the closed-loop BCI. Few researchers have studied the viability of an auditory BCI that uses diverse EEG input signals and auditory feedback (Sellers and Donchin, 2006; Kaongoen and Jo, 2017). Transcranial ultrasonic stimulation in humans is linked with an audio distortion that can be effectively concealed (Park et al., 2021). Future directions for optogenetic and sonogenetic approaches in humans are anticipated to entail the continued development of these techniques for safe and effective therapeutic application, as well as the expansion of the variety of illnesses that can be treated with these techniques.
Optogenetics requires additional development to enhance the transport and expression of opsins in human neurons and optimize the stimulation of light sources. In addition, new opsins with enhanced characteristics and targeting capabilities must be created to allow for more precise regulation of neuronal activity. Optogenetics might be utilized to treat a wide range of neurological and psychiatric illnesses, including Parkinson's disease, epilepsy, and depression, once these obstacles are addressed. Sonogenetics requires additional development to optimize the nanoparticles used for stimulation and modify the ultrasound delivery mechanisms to ensure safe and efficient targeting of specific brain areas. Also, additional study is required to demonstrate the long-term safety and effectiveness of sonogenetics in people. Sonogenetics might be utilized to treat illnesses such as chronic pain, epilepsy, and Parkinson's disease once these obstacles are overcome. More study is required to find the optimal therapeutic uses for optogenetics and sonogenetics. This involves researching the appropriate stimulation settings, therapy duration, and patient selection criteria for these procedures.
The frequency of papers according to types of disease is shown in Figure 6. The selected articles focused on neurodegenerative diseases. Further research is needed in this context of psychiatric disease as worldwide psychological illness had risen to 13% by 2017 according to the World Health Organization (https://www.who.int/health-topics/mental-health#tab=tab_2). The results indicate that although brain stimulation supports treatment for many diseases, the main domains of concern are the motor cortex, Parkinson's disease, and stroke. Alzheimer's disease is a potential area to further explore the use of closed-loop BCI-based brain stimulation. Around 5.8 million Americans suffered from Alzheimer's in 2020. As an alternative therapy, repetitive TMS (rMTS) without closed-loop BCI has been used to treat patients with Alzheimer's disease as well (Chou et al., 2020). The clinical impacts of rTMS have been determined by using various factors to stimulate and match different cortical areas, primarily the dorsolateral prefrontal cortex. An array of advantages in cognition have been highlighted, including with language and episodic memory, behavior, and functionality, in everyday activities for patients with Alzheimer's.
Further applications and devices can be created by using closed-loop BCI based on brain stimulation. It is an integrated software–hardware system that allows the user to control an external device by using brain signals by developing information pathways to and from the brain, and responding according to the output of a given signal or stimulation. Neuroprosthetics is a rapidly emerging field that aims to develop assistive devices to fully or partially restore lost functionality owing to neuronal damage, where these devices can be external or implanted. Implanted devices generally help restore limb movement via electrodes placed under the skin or muscles for stimulation. Visual prosthesis, popularly known as the bionic eye, is a device intended to restore functional vision in individuals who have completely or partially lost sight. Several techniques have been proposed for stimulating the retina, including electrical stimulation (Fujikado et al., 2011), neurotransmitter stimulation (Finlayson and Iezzi, 2010), ultrasound stimulation (Jiang et al., 2018), photodiode stimulation (Lowery et al., 2017), and cortical stimulation (Tochitsky et al., 2017).
Cochlear implants are small devices that electrically stimulate the cochlear nerve to enable hearing. The external part of the device is placed outside the ear, and has a microphone that detects sounds, and then processes and transfers them to the internal part of the implant. It is surgically implanted, and provides patients with moderate-to-severe sensorineural hearing loss and a modified sense of sound. The electrical signals promptly stimulate the auditory nerve. Cochlear implants are currently the world's most successful medical prostheses, with a rejection rate lower than 0.2% and a failure rate of 0.5% (Lowery et al., 2017). In addition to neurodegenerative disease, depression can be treated by supplying repetitive magnetic pulses. During an rTMS session, an electromagnetic coil is positioned along the scalp near the forehead. A magnetic pulse is then painlessly applied to stimulate the nerve cells in the brain region responsible for mood control and depression. This treatment can help psychologists regulate the patients' behavior and mood. Table 4 shows examples of studies on brain stimulation that can be extended by using closed-loop BCI.
Table 4. Selected of the potential studies and their technique for bionic eye and cochlear implants.
This paper provides an overview of the current state of closed-loop brain stimulation research and highlights its potential in the treatment of various neurological disorders such as Parkinson's disease, dementia, and depression. We also discuss the challenges of closed-loop brain stimulation, including electrode design, decoding and encoding algorithms, and the need for long-term stability and safety. Several future research directions include the development of closed-loop systems that can tailor stimulation based on multiple input signals, the use of closed-loop systems for neuromodulation of complex networks, and the integration of closed-loop systems with other systems. We also emphasize the importance of collaboration between researchers, clinicians, and patients in developing effective closed-loop brain stimulation systems that improve the quality of life of neuropathic patients.
This review has several limitations. There was heterogeneity in the comparison of closed-loop BCIs in terms of purpose, methodology, and outcome. This review mainly considered studies that involved participants in the laboratory environment, and contains scant results of work based on empirical environments because the search string did not include the words “environment,” “daily-life use,” or “company.” Additional limitations include the scope of this review. It focused more on cognitive neural BCI and less on affective BCI, particularly BCI related to emotions. Moreover, this review did not exclude animal testing or early-stage studies. Privacy of personal details concerning the users may be needed to ensure participant protection, or to comply with specified requirements for using neuroimaging in daily life. The users' subjective opinions were also not covered by this review. The articles included in this review focused on the technology used, regardless of the purpose of the research, type of tools, and the software used. Future studies should consider different types of commercial equipment to analyze differences in the impacts of each on the quality of life of the user.
This review examined research on the applications of brain stimulation-based closed-loop BCI systems. We broadly classified the types of stimulation into five categories: (i) DBS, (ii) TMS, (iii) tDCS, (iv) tACS, and (v) optogenetics. Overall, closed-loop BCI has exhibited the potential for improving the quality of life of patients by restoring, replacing, or rehabilitating their impaired functions as needed. Techniques of brain stimulation have the promising outcome of the capability of managing the symptoms of individuals with depression, dementia, Alzheimer's disease, and Parkinson's disease. DBS can treat mental problems when traditional therapies have failed, such as OCD. In addition, DBS is also reversible and customizable, allowing clinicians to maximize patient results by modifying stimulation parameters. Real-time stimulation adjustment allows for individualized and responsive therapy. Although pharmaceutical therapies may have systemic adverse effects, DBS has fewer and more localized side effects that may be treated by altering stimulation settings. The capacity of TMS and tDCS to alter the excitability of neurons in specific brain areas is one of their key advantages. TMS induces electrical currents in the brain using magnetic fields, whereas tDCS delivers a low current through electrodes implanted in the head. Depending on the stimulation parameters employed, these approaches can be used to stimulate or inhibit neuronal activity in specific brain regions. TMS has also been utilized to map the functional connectivity of the brain and enhance cognitive skills in individuals with traumatic brain injuries and other neurological illnesses.
By entraining neural oscillations, tACS may alter the activity of certain brain areas and improve cognitive abilities. tACS has also demonstrated potential in treating several neurological disorders, including schizophrenia and chronic pain. Optogenetics is a relatively recent approach involving the genetic modification of certain neurons to produce light-sensitive proteins. After the neurons have been changed, they may be manipulated using light given via implanted fiber-optic wires. Notably, optogenetics is a very recent and intrusive approach that requires genetic alteration and fiber-optic cable insertion. Before optogenetics may be utilized clinically, further study is required to establish its safety and effectiveness in people.
Such devices and applications as bionic eyes and cochlear implants can restore a significant percentage of vision and hearing. The results here show that the above areas of research have immense potential for further research. Although BCI can significantly influence the lives of people suffering from neurodegenerative and psychiatric diseases, its implementation should be preceded by a sufficiently large number of clinical trials and experiments.
This review pinpointed the different techniques of brain stimulation-based closed-loop BCI that can supplement, improve, or enhance the lives of patients. Future research in the area should seek to identify and develop more noninvasive strategies to avoid the need for surgery to reduce the risk of harm, and provide sufficient support for improving the daily lives of the affected individuals.
AB and FA conceived the topic. AB conducted the conceptual design for the review. NJ and SK conducted the literature survey. AB, NJ, and SK wrote a preliminary version of the manuscript. NJ and SK contributed to selecting the articles and analyzing the results. AB and FA have supervised the work. All authors listed have made a substantial, direct, and intellectual contribution to the work and approved it for publication.
The authors acknowledge support from the United Arab Emirates University and ASPIRE (AYIA20-002, 21T057).
The authors declare that the research was conducted in the absence of any commercial or financial relationships that could be construed as a potential conflict of interest.
All claims expressed in this article are solely those of the authors and do not necessarily represent those of their affiliated organizations, or those of the publisher, the editors and the reviewers. Any product that may be evaluated in this article, or claim that may be made by its manufacturer, is not guaranteed or endorsed by the publisher.
Abbasi, A., Goueytes, D., Shulz, D. E., Ego-Stengel, V., and Estebanez, L. (2018). A fast intracortical brain-machine interface with patterned optogenetic feedback. J. Neural Eng. 15:046011. doi: 10.1088/1741-2552/aabb80
Abbasi, J. (2020). Ultrasound brain stimulation piloted in Alzheimer study. JAMA 323:499. doi: 10.1001/jama.2020.0471
Ahn, M., Lee, M., Choi, J., and Jun, S. C. (2014). A review of brain-computer interface games and an opinion survey from researchers, developers and users. Sensors 14, 14601–14633. doi: 10.3390/s140814601
Al-Ani, T., Trad, D., and Somerset, V. S. (2010). Signal processing and classification approaches for brain-computer interface. Intell. Biosens.25–66. doi: 10.5772/7032
Al-Nuaimi, F. A., Al-Nuaimi, R. J., Al-Dhaheri, S. S., Ouhbi, S., and Belkacem, A. N. (2020). Mind drone chasing using EEG-based brain computer interface, in 2020 16th International Conference on Intelligent Environments (IE) (Madrid: IEEE), 74–79. doi: 10.1109/IE49459.2020.9154926
Ang, K. K., Guan, C., Phua, K. S., Wang, C., Teh, I., Chen, C. W., et al. (2012). Transcranial direct current stimulation and EEG-based motor imagery BCI for upper limb stroke rehabilitation. Annu Int Conf IEEE Eng Med Biol Soc. 2012, 4128–4131. doi: 10.1109/EMBC.2012.6346875
Ang, K. K., Guan, C., Phua, K. S., Wang, C., Zhao, L., Teo, W. P., et al. (2015). Facilitating effects of transcranial direct current stimulation on motor imagery brain-computer interface with robotic feedback for stroke rehabilitation. Arch. Phys. Med. Rehabil. 96, S79–S87. doi: 10.1016/j.apmr.2014.08.008
Arlotti, M., Colombo, M., Bonfanti, A., Mandat, T., Lanotte, M. M., Pirola, E., et al. (2021). A new implantable closed-loop clinical neural interface: first application in Parkinson's disease. Front. Neurosci. 15:763235. doi: 10.3389/fnins.2021.763235
Arns, M., De Ridder, S., Strehl, U., Breteler, M., and Coenen, A. (2009). Efficacy of neurofeedback treatment in ADHD: the effects on inattention, impulsivity and hyperactivity: a meta-analysis. Clin. EEG Neurosci. 40, 180–189. doi: 10.1177/155005940904000311
Artusi, C. A., Farooqi, A., Romagnolo, A., Marsili, L., Balestrino, R., Sokol, L. L., et al. (2018). Deep brain stimulation in uncommon tremor disorders: indications, targets, and programming. J. Neurol. 265, 2473–2493. doi: 10.1007/s00415-018-8823-x
Bashashati, A., Fatourechi, M., Ward, R. K., and Birch, G. E. (2007). A survey of signal processing algorithms in brain-computer interfaces based on electrical brain signals. J. Neural Eng. 4:R32. doi: 10.1088/1741-2560/4/2/R03
Baxter, B. S., Edelman, B. J., Nesbitt, N., and He, B. (2016). Sensorimotor rhythm BCI with simultaneous high definition-transcranial direct current stimulation alters task performance. Brain Stimul. 9, 834–841. doi: 10.1016/j.brs.2016.07.003
Baxter, B. S., Edelman, B. J., Sohrabpour, A., and He, B. (2017). Anodal transcranial direct current stimulation increases bilateral directed brain connectivity during motor-imagery based brain-computer interface control. Front. Neurosci. 11:691. doi: 10.3389/fnins.2017.00691
Belkacem, A. N. (2020). “Cybersecurity framework for p300-based brain computer interface,” in 2020 IEEE International Conference on Systems, Man, and Cybernetics (SMC) (Toronto, ON: IEEE), 1–6. doi: 10.1109/SMC42975.2020.9283100
Belkacem, A. N., Jamil, N., Palmer, J. A., Ouhbi, S., and Chen, C. (2020). Brain computer interfaces for improving the quality of life of older adults and elderly patients. Front. Neurosci. 14:692. doi: 10.3389/fnins.2020.00692
Belkacem, A. N., Nishio, S., Suzuki, T., Ishiguro, H., and Hirata, M. (2018). Neuromagnetic decoding of simultaneous bilateral hand movements for multidimensional brain-machine interfaces. IEEE Trans. Neural Syst. Rehabil. Eng. 26, 1301–1310. doi: 10.1109/TNSRE.2018.2837003
Ben-Menachem, E., Manon-Espaillat, R., Ristanovic, R., Wilder, B., Stefan, H., Mirza, W., et al. (1994). Vagus nerve stimulation for treatment of partial seizures: 1. a controlled study of effect on seizures. Epilepsia 35, 616–626. doi: 10.1111/j.1528-1157.1994.tb02482.x
Bigoni, C., Zandvliet, S. B., Beanato, E., Crema, A., Coscia, M., Espinosa, A., et al. (2022). A novel patient-tailored, cumulative neurotechnology-based therapy for upper-limb rehabilitation in severely impaired chronic stroke patients: the avancer study protocol. Front. Neurol. 13:919511. doi: 10.3389/fneur.2022.919511
Biselli, T., Lange, S. S., Sablottny, L., Steffen, J., and Walther, A. (2021). Optogenetic and chemogenetic insights into the neurocircuitry of depression-like behaviour: a systematic review. Eur. J. Neurosci. 53, 9–38. doi: 10.1111/ejn.14603
Boyd, L. A., Hayward, K. S., Ward, N. S., Stinear, C. M., Rosso, C., Fisher, R. J., et al. (2017). Biomarkers of stroke recovery: consensus-based core recommendations from the stroke recovery and rehabilitation roundtable. Int. J. Stroke 12, 480–493. doi: 10.1177/1747493017714176
Buchman, C. A., Gifford, R. H., Haynes, D. S., Lenarz, T., O'Donoghue, G., Adunka, O., et al. (2020). Unilateral cochlear implants for severe, profound, or moderate sloping to profound bilateral sensorineural hearing loss: a systematic review and consensus statements. JAMA Otolaryngol. Head Neck Surg. 146, 942–953. doi: 10.1001/jamaoto.2020.0998
Cantillo-Negrete, J., Carino-Escobar, R. I., Carrillo-Mora, P., Rodriguez-Barragan, M. A., Hernandez-Arenas, C., Quinzaños-Fresnedo, J., et al. (2021). Brain-computer interface coupled to a robotic hand orthosis for stroke patients' neurorehabilitation: a crossover feasibility study. Front. Hum. Neurosci. 15:656975. doi: 10.3389/fnhum.2021.656975
Castaño-Candamil, S., Piroth, T., Reinacher, P., Sajonz, B., Coenen, V. A., and Tangermann, M. (2020). Identifying controllable cortical neural markers with machine learning for adaptive deep brain stimulation in Parkinson's disease. NeuroImage 28:102376. doi: 10.1016/j.nicl.2020.102376
Chamola, V., Vineet, A., Nayyar, A., and Hossain, E. (2020). Brain-computer interface-based humanoid control: a review. Sensors 20:3620. doi: 10.3390/s20133620
Chaudhury, D., Walsh, J. J., Friedman, A. K., Juarez, B., Ku, S. M., Koo, J. W., et al. (2013). Rapid regulation of depression-related behaviours by control of midbrain dopamine neurons. Nature 493, 532–536. doi: 10.1038/nature11713
Chen, C., Chen, P., Belkacem, A. N., Lu, L., Xu, R., Tan, W., et al. (2021a). Neural activities classification of left and right finger gestures during motor execution and motor imagery. Brain-Comput. Interfaces 8, 117–127. doi: 10.1080/2326263X.2020.1782124
Chen, C., Yu, X., Belkacem, A. N., Lu, L., Li, P., Zhang, Z., et al. (2021b). EEG-based anxious states classification using affective bci-based closed neurofeedback system. J. Med. Biol. Eng. 41, 155–164. doi: 10.1007/s40846-020-00596-7
Chew, E., Teo, W.-P., Tang, N., Ang, K. K., Ng, Y. S., Zhou, J. H., et al. (2020). Using transcranial direct current stimulation to augment the effect of motor imagery-assisted brain-computer interface training in chronic stroke patients–cortical reorganization considerations. Front. Neurol. 11:948. doi: 10.3389/fneur.2020.00948
Choi, J., Kwon, M., and Jun, S. C. (2020). A systematic review of closed-loop feedback techniques in sleep studies–related issues and future directions. Sensors 20:2770. doi: 10.3390/s20102770
Chou Y.-h. That V. T. Sundman M. (2020). A systematic review and meta-analysis of RTMS effects on cognitive enhancement in mild cognitive impairment and Alzheimer's disease. Neurobiol. Aging 86, 1–10. doi: 10.1016/j.neurobiolaging.2019.08.020
Cincotti, F., Kauhanen, L., Aloise, F., Palomäki, T., Caporusso, N., Jylänki, P., et al. (2007). Vibrotactile feedback for brain-computer interface operation. Comput. Intell. Neurosci. 2007:48937. doi: 10.1155/2007/48937
Daly, I., Blanchard, C., and Holmes, N. P. (2018). Cortical excitability correlates with the event-related desynchronization during brain-computer interface control. J. Neural Eng. 15:026022. doi: 10.1088/1741-2552/aa9c8c
Darbin, O., Hatanaka, N., Takara, S., Kaneko, N., Chiken, S., Naritoku, D., et al. (2022). Subthalamic nucleus deep brain stimulation driven by primary motor cortex γ2 activity in parkinsonian monkeys. Sci. Rep. 12, 1–16. doi: 10.1038/s41598-022-10130-1
Ding, Q., Lin, T., Wu, M., Yang, W., Li, W., Jing, Y., et al. (2021). Influence of ITBS on the acute neuroplastic change after BCI training. Front. Cell. Neurosci. 15:653487. doi: 10.3389/fncel.2021.653487
Duque, M., Lee-Kubli, C. A., Tufail, Y., Magaram, U., Patel, J., Chakraborty, A., et al. (2021). Sonogenetic control of mammalian cells using exogenous transient receptor potential a1 channels. bioRxiv. doi: 10.1101/2020.10.14.338699
Dutta, A., Paulus, W., and Nitsche, M. A. (2014). Facilitating myoelectric-control with transcranial direct current stimulation: a preliminary study in healthy humans. J. Neuroeng. Rehabil. 11, 1–10. doi: 10.1186/1743-0003-11-13
Fan, C.-H., Wei, K.-C., Chiu, N.-H., Liao, E.-C., Wang, H.-C., Wu, R.-Y., et al. (2021). Sonogenetic-based neuromodulation for the amelioration of Parkinson's disease. Nano Lett. 21, 5967–5976. doi: 10.1021/acs.nanolett.1c00886
Finlayson, P. G., and Iezzi, R. (2010). Glutamate stimulation of retinal ganglion cells in normal and s334ter-4 rat retinas: a candidate for a neurotransmitter-based retinal prosthesis. Invest. Ophthal. Vis. Sci. 51, 3619–3628. doi: 10.1167/iovs.09-4877
Fischer, P., Pogosyan, A., Cheeran, B., Green, A. L., Aziz, T. Z., Hyam, J., et al. (2017). Subthalamic nucleus beta and gamma activity is modulated depending on the level of imagined grip force. Exp. Neurol. 293, 53–61. doi: 10.1016/j.expneurol.2017.03.015
Fleming, J. E., Dunn, E., and Lowery, M. M. (2020). Simulation of closed-loop deep brain stimulation control schemes for suppression of pathological beta oscillations in Parkinson's disease. Front. Neurosci. 14:166. doi: 10.3389/fnins.2020.00166
Fletcher, M. D., Cunningham, R. O., and Mills, S. R. (2020). Electro-haptic enhancement of spatial hearing in cochlear implant users. Sci. Rep. 10, 1–8. doi: 10.1038/s41598-020-58503-8
Fletcher, M. D., and Zgheib, J. (2020). Haptic sound-localisation for use in cochlear implant and hearing-aid users. Sci. Rep. 10, 1–10. doi: 10.1038/s41598-020-70379-2
Fougère, M., van der Zouwen, C. I., Boutin, J., Neszvecsko, K., Sarret, P., and Ryczko, D. (2021). Optogenetic stimulation of glutamatergic neurons in the cuneiform nucleus controls locomotion in a mouse model of Parkinson's disease. Proc. Natl. Acad. Sci. U.S.A. 118:e2110934118. doi: 10.1073/pnas.2110934118
Freund, H.-J., Kuhn, J., Lenartz, D., Mai, J. K., Schnell, T., Klosterkoetter, J., and Sturm, V. (2009). Cognitive functions in a patient with Parkinson-dementia syndrome undergoing deep brain stimulation. Arch. Neurol. 66, 781–785. doi: 10.1001/archneurol.2009.102
Fujikado, T. (2017). “Retinal prosthesis by suprachoroidal-transretinal stimulation (STS), Japanese approach,” in Artificial Vision, ed V. Gabel (Cham: Springer), 139–150. doi: 10.1007/978-3-319-41876-6_11
Fujikado, T., Kamei, M., Sakaguchi, H., Kanda, H., Endo, T., Hirota, M., et al. (2016). One-year outcome of 49-channel suprachoroidal-transretinal stimulation prosthesis in patients with advanced retinitis pigmentosa. Invest. Ophthal. Vis. Sci. 57, 6147–6157. doi: 10.1167/iovs.16-20367
Fujikado, T., Kamei, M., Sakaguchi, H., Kanda, H., Morimoto, T., Ikuno, Y., et al. (2011). Testing of semichronically implanted retinal prosthesis by suprachoroidal-transretinal stimulation in patients with retinitis pigmentosa. Invest. Ophthal. Vis. Sci. 52, 4726–4733. doi: 10.1167/iovs.10-6836
Gao, M., Yu, Y., Zhao, H., Li, G., Jiang, H., Wang, C., et al. (2017). Simulation study of an ultrasound retinal prosthesis with a novel contact-lens array for noninvasive retinal stimulation. IEEE Trans. Neural Syst. Rehabil. Eng. 25, 1605–1611. doi: 10.1109/TNSRE.2017.2682923
Gao, Q., Dou, L., Belkacem, A. N., and Chen, C. (2017). Noninvasive electroencephalogram based control of a robotic arm for writing task using hybrid BCI system. BioMed Res. Int. 2017:8316485. doi: 10.1155/2017/8316485
Gauer, J., Nagathil, A., Martin, R., Thomas, J. P., and Völter, C. (2019). Interactive evaluation of a music preprocessing scheme for cochlear implants based on spectral complexity reduction. Front. Neurosci. 13:1206. doi: 10.3389/fnins.2019.01206
Gharabaghi, A., Kraus, D., Le ao, M. T., Spüler, M., Walter, A., Bogdan, M., et al. (2014). Coupling brain-machine interfaces with cortical stimulation for brain-state dependent stimulation: enhancing motor cortex excitability for neurorehabilitation. Front. Hum. Neurosci. 8:122. doi: 10.3389/fnhum.2014.00122
Grigorev, N. A., Savosenkov, A. O., Lukoyanov, M. V., Udoratina, A., Shusharina, N. N., Kaplan, A. Y., et al. (2021). A bci-based vibrotactile neurofeedback training improves motor cortical excitability during motor imagery. IEEE Trans. Neural Syst. Rehabil. Eng. 29, 1583–1592. doi: 10.1109/TNSRE.2021.3102304
Gürkök, H., Nijholt, A., and Poel, M. (2012). “Brain-computer interface games: towards a framework,” in International Conference on Entertainment Computing (Berlin; Heidelberg: Springer), 373–380. doi: 10.1007/978-3-642-33542-6_33
Gwak, K., Leeb, R., Millán, J. d. R., and Kim, D.-S. (2014). “Quantification and reduction of visual load during bci operation,” in 2014 IEEE International Conference on Systems, Man, and Cybernetics (SMC) (San Diego, CA: IEEE), 2795–2800. doi: 10.1109/SMC.2014.6974352
Hammer, J., Fischer, J., Ruescher, J., Schulze-Bonhage, A., Aertsen, A., and Ball, T. (2013). The role of ecog magnitude and phase in decoding position, velocity, and acceleration during continuous motor behavior. Front. Neurosci. 7:200. doi: 10.3389/fnins.2013.00200
Hammer, J., Pistohl, T., Fischer, J., Kršek, P., Tomášek, M., Marusič, P., et al. (2016). Predominance of movement speed over direction in neuronal population signals of motor cortex: intracranial EEG data and a simple explanatory model. Cereb. Cortex 26, 2863–2881. doi: 10.1093/cercor/bhw033
Handiru, V. S., Vinod, A. P., Keng, A. K., Chew, E., and Guan, C. (2017). “Effects of transcranial direct current stimulation on the motor-imagery brain-computer interface for stroke recovery: an EEG source-space study,” in 2017 IEEE International Conference on Systems, Man, and Cybernetics (SMC) (Banff, AB: IEEE), 2322–2327. doi: 10.1109/SMC.2017.8122968
Hänselmann, S., Schneiders, M., Weidner, N., and Rupp, R. (2015). Transcranial magnetic stimulation for individual identification of the best electrode position for a motor imagery-based brain-computer interface. J. NeuroEng. Rehabil. 12, 1–11. doi: 10.1186/s12984-015-0063-z
Hasegawa, K., Kasuga, S., Takasaki, K., Mizuno, K., Liu, M., and Ushiba, J. (2017). Ipsilateral EEG mu rhythm reflects the excitability of uncrossed pathways projecting to shoulder muscles. J. NeuroEng. Rehabil 14, 1–11. doi: 10.1186/s12984-017-0294-2
Hayashi, M., Okuyama, K., Mizuguchi, N., Hirose, R., Okamoto, T., Kawakami, M., et al. (2022). Spatially bivariate EEG-neurofeedback can manipulate interhemispheric inhibition. Elife 11:e76411. doi: 10.7554/eLife.76411
He, W., Wei, P., Zhou, Y., and Wang, L. (2014). “Modulation effect of transcranial direct current stimulation on phase synchronization in motor imagery brain-computer interface,” in 2014 36th Annual International Conference of the IEEE Engineering in Medicine and Biology Society (Chicago, IL: IEEE), 1270–1273. doi: 10.1109/EMBC.2014.6943829
Heck, C. N., King-Stephens, D., Massey, A. D., Nair, D. R., Jobst, B. C., Barkley, G. L., et al. (2014). Two-year seizure reduction in adults with medically intractable partial onset epilepsy treated with responsive neurostimulation: final results of the RNS system pivotal trial. Epilepsia 55, 432–441. doi: 10.1111/epi.12534
Heldman, D. A., Pulliam, C. L., Mendoza, E. U., Gartner, M., Giuffrida, J. P., Montgomery, E. B. Jr, et al. (2016). Computer-guided deep brain stimulation programming for Parkinson's disease. Neuromodulation 19, 127–132. doi: 10.1111/ner.12372
Herron, J. A., Thompson, M. C., Brown, T., Chizeck, H. J., Ojemann, J. G., and Ko, A. L. (2017). Cortical brain-computer interface for closed-loop deep brain stimulation. IEEE Trans. Neural Syst. Rehabil. Eng. 25, 2180–2187. doi: 10.1109/TNSRE.2017.2705661
Hillyer, J., Elkins, E., Hazlewood, C., Watson, S. D., Arenberg, J. G., and Parbery-Clark, A. (2019). Assessing cognitive abilities in high-performing cochlear implant users. Front. Neurosci. 12:1056. doi: 10.3389/fnins.2018.01056
Hong, X., Lu, Z. K., Teh, I., Nasrallah, F. A., Teo, W. P., Ang, K. K., et al. (2017). Brain plasticity following mi-BCI training combined with TDCS in a randomized trial in chronic subcortical stroke subjects: a preliminary study. Sci. Rep. 7, 1–12. doi: 10.1038/s41598-017-08928-5
Houde, J. F., and Jordan, M. I. (1998). Sensorimotor adaptation in speech production. Science 279, 1213–1216. doi: 10.1126/science.279.5354.1213
Hu, M., Cheng, H.-J., Ji, F., Chong, J. S. X., Lu, Z., Huang, W., et al. (2021). Brain functional changes in stroke following rehabilitation using brain-computer interface-assisted motor imagery with and without tDCS: a pilot study. Front. Hum. Neurosci. 15:692304. doi: 10.3389/fnhum.2021.692304
Hu, M., Ji, F., Lu, Z., Huang, W., Khosrowabadi, R., Zhao, L., et al. (2018). Differential amplitude of low-frequency fluctuations in brain networks after BCI training with and without tDCS in stroke. Annu Int Conf IEEE Eng Med Biol Soc. 2018, 1050–1053. doi: 10.1109/EMBC.2018.8512395
Isaacs, R. E., Weber, D., and Schwartz, A. B. (2000). Work toward real-time control of a cortical neural prothesis. IEEE Trans. Rehabil. Eng. 8, 196–198. doi: 10.1109/86.847814
Jamil, N., Belkacem, A. N., and Lakas, A. (2022). On enhancing student's cognitive abilities in online learning using brain activity and eye movements. Educ. Inform. Technol. 1–35. doi: 10.1007/s10639-022-11372-2
Jamil, N., Belkacem, A. N., Ouhbi, S., and Guger, C. (2021). Cognitive and affective brain-computer interfaces for improving learning strategies and enhancing student capabilities: a systematic literature review. IEEE Access 9, 134122–134147. doi: 10.1109/ACCESS.2021.3115263
Jeunet, C., Vi, C., Spelmezan, D., N'Kaoua, B., Lotte, F., and Subramanian, S. (2015). “Continuous tactile feedback for motor-imagery based brain-computer interaction in a multitasking context,” in IFIP Conference on Human-Computer Interaction (Cham: Springer), 488–505. doi: 10.1007/978-3-319-22701-6_36
Jiang, Q., Li, G., Zhao, H., Sheng, W., Yue, L., Su, M., et al. (2018). Temporal neuromodulation of retinal ganglion cells by low-frequency focused ultrasound stimulation. IEEE Trans. Neural Syst. Rehabil. Eng. 26, 969–976. doi: 10.1109/TNSRE.2018.2821194
Jochumsen, M., Cremoux, S., Robinault, L., Lauber, J., Arceo, J. C., Navid, M. S., et al. (2018). Investigation of optimal afferent feedback modality for inducing neural plasticity with a self-paced brain-computer interface. Sensors 18:3761. doi: 10.3390/s18113761
Johnson, R. L., and Wilson, C. G. (2018). A review of vagus nerve stimulation as a therapeutic intervention. J. Inflamm. Res. 11:203. doi: 10.2147/JIR.S163248
Kaongoen, N., and Jo, S. (2017). A novel hybrid auditory bci paradigm combining ASSR and P300. J. Neurosci. Methods 279, 44–51. doi: 10.1016/j.jneumeth.2017.01.011
Kaplan, A., Vasilyev, A., Liburkina, S., and Yakovlev, L. (2016). “Poor BCI performers still could benefit from motor imagery training,” in International Conference on Augmented Cognition (Cham: Springer), 46–56. doi: 10.1007/978-3-319-39955-3_5
Kasashima-Shindo, Y., Fujiwara, T., Ushiba, J., Matsushika, Y., Kamatani, D., Oto, M., et al. (2015). Brain-computer interface training combined with transcranial direct current stimulation in patients with chronic severe hemiparesis: proof of concept study. J. Rehabil. Med. 47, 318–324. doi: 10.2340/16501977-1925
Kim, B., and Winstein, C. (2017). Can neurological biomarkers of brain impairment be used to predict poststroke motor recovery? A systematic review. Neurorehabil. Neural Repair 31, 3–24. doi: 10.1177/1545968316662708
Kraus, D., Naros, G., Bauer, R., Khademi, F., Le ao, M. T., Ziemann, U., et al. (2016). Brain state-dependent transcranial magnetic closed-loop stimulation controlled by sensorimotor desynchronization induces robust increase of corticospinal excitability. Brain Stimul. 9, 415–424. doi: 10.1016/j.brs.2016.02.007
Krucoff, M. O., Rahimpour, S., Slutzky, M. W., Edgerton, V. R., and Turner, D. A. (2016). Enhancing nervous system recovery through neurobiologics, neural interface training, and neurorehabilitation. Front. Neurosci. 10:584. doi: 10.3389/fnins.2016.00584
Kuhn, J., Hardenacke, K., Lenartz, D., Gruendler, T., Ullsperger, M., Bartsch, C., et al. (2015). Deep brain stimulation of the nucleus basalis of meynert in Alzheimer's dementia. Mol. Psychiatry 20, 353–360. doi: 10.1038/mp.2014.32
Larson, P. S. (2014). Deep brain stimulation for movement disorders. Neurotherapeutics 11, 465–474. doi: 10.1007/s13311-014-0274-1
Laxton, A. W., Tang-Wai, D. F., McAndrews, M. P., Zumsteg, D., Wennberg, R., Keren, R., et al. (2010). A phase i trial of deep brain stimulation of memory circuits in Alzheimer's disease. Ann. Neurol. 68, 521–534. doi: 10.1002/ana.22089
Lee, B., Zubair, M. N., Marquez, Y. D., Lee, D. M., Kalayjian, L. A., Heck, C. N., et al. (2015). A single-center experience with the neuropace RNS system: a review of techniques and potential problems. World Neurosurg. 84, 719–726. doi: 10.1016/j.wneu.2015.04.050
Lee, M. B., Kramer, D. R., Peng, T., Barbaro, M. F., Liu, C. Y., Kellis, S., et al. (2019). Clinical neuroprosthetics: today and tomorrow. J. Clin. Neurosci. 68, 13–19. doi: 10.1016/j.jocn.2019.07.056
Leeb, R., Gwak, K., Kim, D.-S., et al. (2013). Freeing the visual channel by exploiting vibrotactile BCI feedback. Annu Int Conf IEEE Eng Med Biol Soc. 2013, 3093–3096. doi: 10.1109/EMBC.2013.6610195
Leinenga, G., and Götz, J. (2015). Scanning ultrasound removes amyloid-β and restores memory in an Alzheimer's disease mouse model. Sci. Transl. Med. 7:278ra33. doi: 10.1126/scitranslmed.aaa2512
Leinenga, G., Langton, C., Nisbet, R., and Götz, J. (2016). Ultrasound treatment of neurological diseases–current and emerging applications. Nat. Rev. Neurol. 12, 161–174. doi: 10.1038/nrneurol.2016.13
Liang, S.-F., Shaw, F.-Z., Young, C.-P., Chang, D.-W., and Liao, Y.-C. (2010). A closed-loop brain computer interface for real-time seizure detection and control. Annu Int Conf IEEE Eng Med Biol Soc. 2010, 4950–4953. doi: 10.1109/IEMBS.2010.5627243
Liang, W., Xu, Y., Schmidt, J., Zhang, L., and Ruddy, K. (2020). Upregulating excitability of corticospinal pathways in stroke patients using TMS neurofeedback; a pilot study. NeuroImage 28:102465. doi: 10.1016/j.nicl.2020.102465
Liberati, A., Altman, D. G., Tetzlaff, J., Mulrow, C., Gòtzsche, P. C., Ioannidis, J. P., et al. (2009). The prisma statement for reporting systematic reviews and meta-analyses of studies that evaluate health care interventions: explanation and elaboration. J. Clin. Epidemiol. 62, e1–e34. doi: 10.1016/j.jclinepi.2009.06.006
Liburkina, S., Vasilyev, A., Yakovlev, L., Gordleeva, S. Y., and Kaplan, A. Y. (2018). A motor imagery-based brain-computer interface with vibrotactile stimuli. Neurosci. Behav. Physiol. 48, 1067–1077. doi: 10.1007/s11055-018-0669-2
Little, S., Pogosyan, A., Neal, S., Zavala, B., Zrinzo, L., Hariz, M., et al. (2013). Adaptive deep brain stimulation in advanced Parkinson disease. Ann. Neurol. 74, 449–457. doi: 10.1002/ana.23951
Liu, J., Qiu, S., Chen, X., Zhou, Y., Wu, J., Sun, T., et al. (2020). Focused ultrasound stimulation on human language-related acupoints modulates brain activity in cortical language processing regions. Hum. Behav. Brain 1, 22–27. doi: 10.37716/HBAB.2020010104
Liu, P., Chen, Z., Jones, J. A., Huang, D., and Liu, H. (2011). Auditory feedback control of vocal pitch during sustained vocalization: a cross-sectional study of adult aging. PLoS ONE 6:e22791. doi: 10.1371/journal.pone.0022791
Losey, D. M., Stocco, A., Abernethy, J. A., and Rao, R. P. (2016). Navigating a 2D virtual world using direct brain stimulation. Front. Robot. AI 3:72. doi: 10.3389/frobt.2016.00072
Lotte, F. (2012). On the need for alternative feedback training approaches for BCI,” in Berlin Brain-Computer Interface Workshop, Berlin.
Lowery, A. J., Rosenfeld, J. V., Rosa, M. G., Brunton, E., Rajan, R., Mann, C., et al. (2017). “Monash vision group's gennaris cortical implant for vision restoration,” in Artificial Vision, ed V. Gabel (Cham: Springer), 215–225. doi: 10.1007/978-3-319-41876-6_17
Lozano, A. M., and Lipsman, N. (2013). Probing and regulating dysfunctional circuits using deep brain stimulation. Neuron 77, 406–424. doi: 10.1016/j.neuron.2013.01.020
Lozano, A. M., Lipsman, N., Bergman, H., Brown, P., Chabardes, S., Chang, J. W., et al. (2019). Deep brain stimulation: current challenges and future directions. Nat. Rev. Neurol. 15, 148–160. doi: 10.1038/s41582-018-0128-2
Lukoyanov, M., Gordleeva, S. Y., Pimashkin, A., Grigor'ev, N., Savosenkov, A., Motailo, A., et al. (2018). The efficiency of the brain-computer interfaces based on motor imagery with tactile and visual feedback. Hum. Physiol. 44, 280–288. doi: 10.1134/S0362119718030088
Lv, Q., Du, A., Wei, W., Li, Y., Liu, G., and Wang, X. P. (2018). Deep brain stimulation: a potential treatment for dementia in Alzheimer's disease (AD) and Parkinson's disease dementia (PDD). Front. Neurosci. 12:360. doi: 10.3389/fnins.2018.00360
Magno, L. A. V., Tenza-Ferrer, H., Collodetti, M., Aguiar, M. F. G., Rodrigues, A. P. C., da Silva, R. S., et al. (2019). Optogenetic stimulation of the m2 cortex reverts motor dysfunction in a mouse model of Parkinson's disease. J. Neurosci. 39, 3234–3248. doi: 10.1523/JNEUROSCI.2277-18.2019
Mane, R., Chew, E., Phua, K. S., Ang, K. K., Robinson, N., Vinod, A., et al. (2019). Prognostic and monitory EEG-biomarkers for BCI upper-limb stroke rehabilitation. IEEE Trans. Neural Syst. Rehabil. Eng. 27, 1654–1664. doi: 10.1109/TNSRE.2019.2924742
Márquez-Ruiz, J., Ammann, C., Leal-Campanario, R., Ruffini, G., Gruart, A., and Delgado-García, J. M. (2016). Synthetic tactile perception induced by transcranial alternating-current stimulation can substitute for natural sensory stimulus in behaving rabbits. Sci. Rep. 6, 1–12. doi: 10.1038/srep19753
Mashat, M. E. M., Li, G., and Zhang, D. (2017). Human-to-human closed-loop control based on brain-to-brain interface and muscle-to-muscle interface. Sci. Rep. 7, 1–11. doi: 10.1038/s41598-017-10957-z
Mason, T. J. (2011). Therapeutic ultrasound an overview. Ultrason. Sonochem. 18, 847–852. doi: 10.1016/j.ultsonch.2011.01.004
Merk, T., Peterson, V., Lipski, W. J., Blankertz, B., Turner, R. S., Li, N., et al. (2022). Electrocorticography is superior to subthalamic local field potentials for movement decoding in Parkinson's disease. Elife 11:e75126. doi: 10.7554/eLife.75126
Mihelj, E., Bächinger, M., Kikkert, S., Ruddy, K., and Wenderoth, N. (2021). Mental individuation of imagined finger movements can be achieved using TMS-based neurofeedback. NeuroImage 242:118463. doi: 10.1016/j.neuroimage.2021.118463
Milekovic, T., Fischer, J., Pistohl, T., Ruescher, J., Schulze-Bonhage, A., Aertsen, A., et al. (2012). An online brain-machine interface using decoding of movement direction from the human electrocorticogram. J. Neural Eng. 9:046003. doi: 10.1088/1741-2560/9/4/046003
Mirzadeh, Z., Bari, A., and Lozano, A. M. (2016). The rationale for deep brain stimulation in Alzheimer's disease. J. Neural Transm. 123, 775–783. doi: 10.1007/s00702-015-1462-9
Mokienko, O. A., Chervyakov, A. V., Kulikova, S. N., Bobrov, P. D., Chernikova, L. A., Frolov, A. A., et al. (2013). Increased motor cortex excitability during motor imagery in brain-computer interface trained subjects. Front. Comput. Neurosci. 7:168. doi: 10.3389/fncom.2013.00168
Morrell, M. J. (2011). Responsive cortical stimulation for the treatment of medically intractable partial epilepsy. Neurology 77, 1295–1304. doi: 10.1212/WNL.0b013e3182302056
Naros, G., and Gharabaghi, A. (2017). Physiological and behavioral effects of β-tacs on brain self-regulation in chronic stroke. Brain Stimul. 10, 251–259. doi: 10.1016/j.brs.2016.11.003
Naros, G., Lehnertz, T., Le ao, M. T., Ziemann, U., and Gharabaghi, A. (2020). Brain state-dependent gain modulation of corticospinal output in the active motor system. Cereb. Cortex 30, 371–381. doi: 10.1093/cercor/bhz093
Naros, G., Naros, I., Grimm, F., Ziemann, U., and Gharabaghi, A. (2016). Reinforcement learning of self-regulated sensorimotor β-oscillations improves motor performance. Neuroimage 134, 142–152. doi: 10.1016/j.neuroimage.2016.03.016
Neely, R. M., Koralek, A. C., Athalye, V. R., Costa, R. M., and Carmena, J. M. (2018). Volitional modulation of primary visual cortex activity requires the basal ganglia. Neuron 97, 1356–1368. doi: 10.1016/j.neuron.2018.01.051
Neumann, W.-J., Sorkhabi, M. M., Benjaber, M., Feldmann, L. K., Saryyeva, A., Krauss, J. K., et al. (2021). The sensitivity of ECG contamination to surgical implantation site in brain computer interfaces. Brain Stimul. 14, 1301–1306. doi: 10.1016/j.brs.2021.08.016
Niazi, I. K., Jochumsen, M., Duehra, J., Kingett, M., Dremstrup, K., and Haavik, H. (2014). “Chiropractic, cortical excitability and BCI,” in Replace, Repair, Restore, Relieve-Bridging Clinical and Engineering Solutions in Neurorehabilitation, eds W. Jensen, O. Andersen, and M. Akay (Cham: Springer), 121–125. doi: 10.1007/978-3-319-08072-7_23
Niazi, I. K., Mrachacz-Kersting, N., Jiang, N., Dremstrup, K., and Farina, D. (2012). Peripheral electrical stimulation triggered by self-paced detection of motor intention enhances motor evoked potentials. IEEE Trans. Neural Syst. Rehabil. Eng. 20, 595–604. doi: 10.1109/TNSRE.2012.2194309
Nicolas-Alonso, L. F., and Gomez-Gil, J. (2012). Brain computer interfaces, a review. Sensors 12, 1211–1279. doi: 10.3390/s120201211
Ogbonnaya, S., and Kaliaperumal, C. (2013). Vagal nerve stimulator: evolving trends. J. Nat. Sci. Biol. Med. 4, 8–13. doi: 10.4103/0976-9668.107254
Ortiz, M., Iáñez, E., Gaxiola-Tirado, J. A., Gutiérrez, D., and Azorín, J. M. (2020). Study of the functional brain connectivity and lower-limb motor imagery performance after transcranial direct current stimulation. Int. J. Neural Syst. 30:2050038. doi: 10.1142/S0129065720500380
Osawa, S.-i., Iwasaki, M., Hosaka, R., Matsuzaka, Y., Tomita, H., Ishizuka, T., et al. (2013). Optogenetically induced seizure and the longitudinal hippocampal network dynamics. PLoS ONE 8:e60928. doi: 10.1371/journal.pone.0060928
Pai, A. V., Bellare, J., and Gandhi, T. K. (2016). “Chemoretina: an alternate approach to retinal prosthesis: visual stimulation strategy using chemicals,” in 2016 IEEE Annual India Conference (INDICON) (Bangalore: IEEE), 1–4. doi: 10.1109/INDICON.2016.7839036
Pan, H., Mi, W., Lei, X., and Deng, J. (2020). A closed-loop brain-machine interface framework design for motor rehabilitation. Biomed. Signal Process. Control 58:101877. doi: 10.1016/j.bspc.2020.101877
Park, C., Chen, M., and Kim, T. (2021). Implication of auditory confounding in interpreting somatosensory and motor responses in low-intensity focused transcranial ultrasound stimulation. J. Neurophysiol. 125, 2356–2360. doi: 10.1152/jn.00701.2020
Paz, J. T., Davidson, T. J., Frechette, E. S., Delord, B., Parada, I., Peng, K., et al. (2013). Closed-loop optogenetic control of thalamus as a tool for interrupting seizures after cortical injury. Nat. Neurosci. 16, 64–70. doi: 10.1038/nn.3269
Pfurtscheller, G., and Neuper, C. (2006). Future prospects of ERD/ERS in the context of brain-computer interface (BCI) developments. Prog. Brain Res. 159, 433–437. doi: 10.1016/S0079-6123(06)59028-4
Pichiorri, F., Fallani, F. D. V., Cincotti, F., Babiloni, F., Molinari, M., Kleih, S., et al. (2011). Sensorimotor rhythm-based brain-computer interface training: the impact on motor cortical responsiveness. J. Neural Eng. 8:025020. doi: 10.1088/1741-2560/8/2/025020
Prsa, M., Galiñanes, G. L., and Huber, D. (2017). Rapid integration of artificial sensory feedback during operant conditioning of motor cortex neurons. Neuron 93, 929–939. doi: 10.1016/j.neuron.2017.01.023
Quiles, V., Iáñez, E., Ortiz, M., Medina, N., Serrano, A., and Azorín, J. M. (2020). Lessons learned from clinical trials of a neurorehabilitation therapy based on tDCS, BMI, and pedaling systems. IEEE Syst. J. 15, 1873–1880. doi: 10.1109/JSYST.2020.3026242
Remsik, A., Young, B., Vermilyea, R., Kiekhoefer, L., Abrams, J., Evander Elmore, S., et al. (2016). A review of the progression and future implications of brain-computer interface therapies for restoration of distal upper extremity motor function after stroke. Expert Rev. Med. Devices 13, 445–454. doi: 10.1080/17434440.2016.1174572
Rodríguez-Ugarte, M., Iáñez, E., Ortiz, M., and Azorín, J. M. (2018a). Improving real-time lower limb motor imagery detection using tDCS and an exoskeleton. Front. Neurosci. 12:757. doi: 10.3389/fnins.2018.00757
Rodríguez-Ugarte, M., Iáñez, E., Ortíz, M., and Azorín, J. M. (2018b). “Novel tDCS montage favors lower limb motor imagery detection,” in 2018 40th Annual International Conference of the IEEE Engineering in Medicine and Biology Society (EMBC), 2170–2173. doi: 10.1109/EMBC.2018.8512656
Rodriguez-Ugarte, M. D. l. S., Iáñez, E., Ortiz-Garcia, M., and Azorín, J. M. (2018). Effects of tdcs on real-time bci detection of pedaling motor imagery. Sensors 18:1136. doi: 10.3390/s18041136
Roman-Gonzalez, A. (2012). “EEG signal processing for BCI applications,” in Human-Computer Systems Interaction: Backgrounds and Applications 2, eds Z. S. Hippe, J. L. Kulikowski, and T. Mroczek (Berlin; Heidelberg: Springer), 571–591. doi: 10.1007/978-3-642-23187-2_36
Ros, T., Munneke, M. A., Ruge, D., Gruzelier, J. H., and Rothwell, J. C. (2010). Endogenous control of waking brain rhythms induces neuroplasticity in humans. Eur. J. Neurosci. 31, 770–778. doi: 10.1111/j.1460-9568.2010.07100.x
Rossi, L., Foffani, G., Marceglia, S., Bracchi, F., Barbieri, S., and Priori, A. (2007). An electronic device for artefact suppression in human local field potential recordings during deep brain stimulation. J. Neural Eng. 4:96. doi: 10.1088/1741-2560/4/2/010
Rountree, C. M., Inayat, S., Troy, J. B., and Saggere, L. (2016). Differential stimulation of the retina with subretinally injected exogenous neurotransmitter: a biomimetic alternative to electrical stimulation. Sci. Rep. 6, 1–13. doi: 10.1038/srep38505
Royter, V., and Gharabaghi, A. (2016). Brain state-dependent closed-loop modulation of paired associative stimulation controlled by sensorimotor desynchronization. Front. Cell. Neurosci. 10:115. doi: 10.3389/fncel.2016.00115
Ryan, B. P., and Van Kirk, B. (1974). The establishment, transfer, and maintenance of fluent speech in 50 stutterers using delayed auditory feedback and operant procedures. J. Speech Hear. Disord. 39, 3–10. doi: 10.1044/jshd.3901.03
Salanova, V., Witt, T., Worth, R., Henry, T. R., Gross, R. E., Nazzaro, J. M., et al. (2015). Long-term efficacy and safety of thalamic stimulation for drug-resistant partial epilepsy. Neurology 84, 1017–1025. doi: 10.1212/WNL.0000000000001334
Sani, O. G., Yang, Y., and Shanechi, M. M. (2021). Closed-loop BCI for the treatment of neuropsychiatric disorders. Brain-Comput. Interface Res. 9, 121–125. doi: 10.1007/978-3-030-60460-8_12
Saniotis, A., Henneberg, M., and Sawalma, A.-R. (2018). Integration of nanobots into neural circuits as a future therapy for treating neurodegenerative disorders. Front. Neurosci. 12:153. doi: 10.3389/fnins.2018.00153
Sato, T., Shapiro, M. G., and Tsao, D. Y. (2018). Ultrasonic neuromodulation causes widespread cortical activation via an indirect auditory mechanism. Neuron 98, 1031–1041. doi: 10.1016/j.neuron.2018.05.009
Scheyltjens, I., Vreysen, S., Van den Haute, C., Sabanov, V., Balschun, D., Baekelandt, V., et al. (2018). Transient and localized optogenetic activation of somatostatin-interneurons in mouse visual cortex abolishes long-term cortical plasticity due to vision loss. Brain Struct. Funct. 223, 2073–2095. doi: 10.1007/s00429-018-1611-7
Schildt, C. J., Thomas, S. H., Powell, E. S., Sawaki, L., and Sunderam, S. (2016). Closed-loop afferent electrical stimulation for recovery of hand function in individuals with motor incomplete spinal injury: early clinical results. Annu Int Conf IEEE Eng Med Biol Soc. 2016, 1552–1555. doi: 10.1109/EMBC.2016.7591007
Sellers, E. W., and Donchin, E. (2006). A p300-based brain-computer interface: initial tests by ALS patients. Clin. Neurophysiol. 117, 538–548. doi: 10.1016/j.clinph.2005.06.027
Shao, L., Zhang, L., Belkacem, A. N., Zhang, Y., Chen, X., Li, J., et al. (2020). EEG-controlled wall-crawling cleaning robot using SSVEP-based brain-computer interface. J. Healthc. Eng. 2020:6968713. doi: 10.1155/2020/6968713
Shiozawa P. Fregni F. Benseñor I. M. Lotufo P. A. Berlim M. T. Daskalakis J. Z.. (2014). Transcranial direct current stimulation for major depression: an updated systematic review and meta-analysis. Int. J. Neuropsychopharmacol. 17, 1443–1452. doi: 10.1017/S1461145714000418
Sitaram, R., Veit, R., Stevens, B., Caria, A., Gerloff, C., Birbaumer, N., et al. (2012). Acquired control of ventral premotor cortex activity by feedback training: an exploratory real-time fMRI and TMS study. Neurorehabil. Neural Repair 26, 256–265. doi: 10.1177/1545968311418345
Soekadar, S. R., Witkowski, M., Birbaumer, N., and Cohen, L. G. (2015). Enhancing hebbian learning to control brain oscillatory activity. Cereb. Cortex 25, 2409–2415. doi: 10.1093/cercor/bhu043
Soekadar, S. R., Witkowski, M., Cossio, E. G., Birbaumer, N., and Cohen, L. G. (2014). Learned EEG-based brain self-regulation of motor-related oscillations during application of transcranial electric brain stimulation: feasibility and limitations. Front. Behav. Neurosci. 8:93. doi: 10.3389/fnbeh.2014.00093
Sonuga-Barke, E. J., Brandeis, D., Cortese, S., Daley, D., Ferrin, M., Holtmann, M., et al. (2013). Nonpharmacological interventions for ADHD: systematic review and meta-analyses of randomized controlled trials of dietary and psychological treatments. Am. J. Psychiatry 170, 275–289. doi: 10.1176/appi.ajp.2012.12070991
Steinbeck, J. A., Choi, S. J., Mrejeru, A., Ganat, Y., Deisseroth, K., Sulzer, D., et al. (2015). Optogenetics enables functional analysis of human embryonic stem cell-derived grafts in a Parkinson's disease model. Nat. Biotechnol. 33, 204–209. doi: 10.1038/nbt.3124
Sun, F. T., and Morrell, M. J. (2014). The RNS system: responsive cortical stimulation for the treatment of refractory partial epilepsy. Expert Rev. Med. Devices 11, 563–572. doi: 10.1586/17434440.2014.947274
Sun, G., Zeng, F., McCartin, M., Zhang, Q., Xu, H., Liu, Y., et al. (2022). Closed-loop stimulation using a multiregion brain-machine interface has analgesic effects in rodents. Sci. Transl. Med. 14:eabm5868. doi: 10.1126/scitranslmed.abm5868
Swan, B. D., Gasperson, L. B., Krucoff, M. O., Grill, W. M., and Turner, D. A. (2018). Sensory percepts induced by microwire array and dbs microstimulation in human sensory thalamus. Brain Stimul. 11, 416–422. doi: 10.1016/j.brs.2017.10.017
Swann, N. C., de Hemptinne, C., Miocinovic, S., Qasim, S., Ostrem, J. L., Galifianakis, N. B., et al. (2017). Chronic multisite brain recordings from a totally implantable bidirectional neural interface: experience in 5 patients with Parkinson's disease. J. Neurosurg. 128, 605–616. doi: 10.3171/2016.11.JNS161162
Swann, N. C., de Hemptinne, C., Thompson, M. C., Miocinovic, S., Miller, A. M., Ostrem, J. L., et al. (2018). Adaptive deep brain stimulation for Parkinson's disease using motor cortex sensing. J. Neural Eng. 15:046006. doi: 10.1088/1741-2552/aabc9b
Syrov, N., Bredichin, D., and Kaplan, A. (2020). “Processing of sensory information is affected by BCI feedback being perceived,” in International Conference on Human-Computer Interaction (Cham: Springer), 575–580. doi: 10.1007/978-3-030-50726-8_75
Syrov, N., Novichikhina, K., Kir'yanov, D., Gordleeva, S. Y., and Kaplan, A. Y. (2019). The changes of corticospinal excitability during the control of artificial hand through the brain-computer interface based on the p300 component of visual evoked potential. Human Physiol. 45, 152–157. doi: 10.1134/S0362119719020117
Takemi, M., Maeda, T., Masakado, Y., Siebner, H. R., and Ushiba, J. (2018). Muscle-selective disinhibition of corticomotor representations using a motor imagery-based brain-computer interface. Neuroimage 183, 597–605. doi: 10.1016/j.neuroimage.2018.08.070
Takemi, M., Masakado, Y., Liu, M., and Ushiba, J. (2013). Event-related desynchronization reflects downregulation of intracortical inhibition in human primary motor cortex. J. Neurophysiol. 110, 1158–1166. doi: 10.1152/jn.01092.2012
Takeuchi, N., Mori, T., Nishijima, K., Kondo, T., and Izumi, S.-I. (2015). Inhibitory transcranial direct current stimulation enhances weak beta event-related synchronization after foot motor imagery in patients with lower limb amputation. J. Clin. Neurophysiol. 32, 44–50. doi: 10.1097/WNP.0000000000000123
Tatum W. O. IV. and Helmers S. L. (2009). Vagus nerve stimulation and magnet use: optimizing benefits. Epilepsy Behav. 15, 299–302. doi: 10.1016/j.yebeh.2009.04.002
Távora-Vieira, D., Wedekind, A., Marino, R., Purdy, S. C., and Rajan, G. P. (2018). Using aided cortical assessment as an objective tool to evaluate cochlear implant fitting in users with single-sided deafness. PLoS ONE 13:e0193081. doi: 10.1371/journal.pone.0193081
Thompson, M. C., Herron, J. A., Brown, T., Ojemann, J. G., Ko, A. L., and Chizeck, H. J. (2016). “Demonstration of a stable chronic electrocorticography-based brain-computer interface using a deep brain stimulator,” in 2016 IEEE International Conference on Systems, Man, and Cybernetics (SMC) (Budapest: IEEE), 2936–2941. doi: 10.1109/SMC.2016.7844686
Tochitsky, I., Trautman, J., Gallerani, N., Malis, J. G., and Kramer, R. H. (2017). Restoring visual function to the blind retina with a potent, safe and long-lasting photoswitch. Sci. Rep. 7, 1–8. doi: 10.1038/srep45487
Tønnesen, J., Sørensen, A. T., Deisseroth, K., Lundberg, C., and Kokaia, M. (2009). Optogenetic control of epileptiform activity. Proc. Natl. Acad. Sci. U.S.A. 106, 12162–12167. doi: 10.1073/pnas.0901915106
Tye, K. M., Mirzabekov, J. J., Warden, M. R., Ferenczi, E. A., Tsai, H.-C., Finkelstein, J., et al. (2013). Dopamine neurons modulate neural encoding and expression of depression-related behaviour. Nature 493, 537–541. doi: 10.1038/nature11740
Uthman, B., Wilder, B., Penry, J., Dean, C., Ramsay, R., Reid, S., et al. (1993). Treatment of epilepsy by stimulation of the vagus nerve. Neurology 43, 1338–1338. doi: 10.1212/WNL.43.7.1338
Vasilyev, A., Liburkina, S., Yakovlev, L., Perepelkina, O., and Kaplan, A. (2017). Assessing motor imagery in brain-computer interface training: psychological and neurophysiological correlates. Neuropsychologia 97, 56–65. doi: 10.1016/j.neuropsychologia.2017.02.005
Wang, K.-W., Ye, X.-L., Huang, T., Yang, X.-F., and Zou, L.-Y. (2019). Optogenetics-induced activation of glutamate receptors improves memory function in mice with Alzheimer's disease. Neural Regener. Res. 14:2147. doi: 10.4103/1673-5374.262593
Wang, Y., Yan, J., Wen, J., Yu, T., and Li, X. (2016). An intracranial electroencephalography (IEEG) brain function mapping tool with an application to epilepsy surgery evaluation. Front. Neuroinformatics 10:15. doi: 10.3389/fninf.2016.00015
Wei, P., He, W., Zhou, Y., and Wang, L. (2013). Performance of motor imagery brain-computer interface based on anodal transcranial direct current stimulation modulation. IEEE Trans. Neural Syst. Rehabil. Eng. 21, 404–415. doi: 10.1109/TNSRE.2013.2249111
Widge, A., and Moritz, C. (2016). “Closed-loop stimulation in emotional circuits for neuro-psychiatric disorders,” in Closed Loop Neuroscience (San Diego, CA: Elsevier Inc.), 229–239. doi: 10.1016/B978-0-12-802452-2.00017-2
Wolpaw, J. R., Birbaumer, N., McFarland, D. J., Pfurtscheller, G., and Vaughan, T. M. (2002). Brain-computer interfaces for communication and control. Clin. Neurophysiol. 113, 767–791. doi: 10.1016/S1388-2457(02)00057-3
Wolpaw, J. R., and McFarland, D. J. (2004). Control of a two-dimensional movement signal by a noninvasive brain-computer interface in humans. Proc. Natl. Acad. Sci. U.S.A. 101, 17849–17854. doi: 10.1073/pnas.0403504101
Xu, R., Jiang, N., Lin, C., Mrachacz-Kersting, N., Dremstrup, K., and Farina, D. (2013). Enhanced low-latency detection of motor intention from EEG for closed-loop brain-computer interface applications. IEEE Trans. Biomed. Eng. 61, 288–296. doi: 10.1109/TBME.2013.2294203
Yamamoto, K., Tanei, Z.-I., Hashimoto, T., Wakabayashi, T., Okuno, H., Naka, Y., et al. (2015). Chronic optogenetic activation augments aβ pathology in a mouse model of Alzheimer disease. Cell Rep. 11, 859–865. doi: 10.1016/j.celrep.2015.04.017
Zhang, Q., Hu, S., Talay, R., Xiao, Z., Rosenberg, D., Liu, Y., et al. (2021). A prototype closed-loop brain-machine interface for the study and treatment of pain. Nat. Biomed. Eng. 1–13. doi: 10.1038/s41551-021-00736-7
Keywords: brain stimulation, closed-loop BCI, neurodegenerative diseases, psychiatric diseases, brain computer interface (BCI)
Citation: Belkacem AN, Jamil N, Khalid S and Alnajjar F (2023) On closed-loop brain stimulation systems for improving the quality of life of patients with neurological disorders. Front. Hum. Neurosci. 17:1085173. doi: 10.3389/fnhum.2023.1085173
Received: 31 October 2022; Accepted: 06 March 2023;
Published: 23 March 2023.
Edited by:
Bradley Jay Edelman, Max Planck Institute of Neurobiology (MPIN), GermanyReviewed by:
Yousef Salimpour, School of Medicine, Johns Hopkins University, United StatesCopyright © 2023 Belkacem, Jamil, Khalid and Alnajjar. This is an open-access article distributed under the terms of the Creative Commons Attribution License (CC BY). The use, distribution or reproduction in other forums is permitted, provided the original author(s) and the copyright owner(s) are credited and that the original publication in this journal is cited, in accordance with accepted academic practice. No use, distribution or reproduction is permitted which does not comply with these terms.
*Correspondence: Abdelkader Nasreddine Belkacem, QmVsa2FjZW1AdWFldS5hYy5hZQ==; Fady Alnajjar, ZmFkeS5hbG5hamphckB1YWV1LmFjLmFl
Disclaimer: All claims expressed in this article are solely those of the authors and do not necessarily represent those of their affiliated organizations, or those of the publisher, the editors and the reviewers. Any product that may be evaluated in this article or claim that may be made by its manufacturer is not guaranteed or endorsed by the publisher.
Research integrity at Frontiers
Learn more about the work of our research integrity team to safeguard the quality of each article we publish.