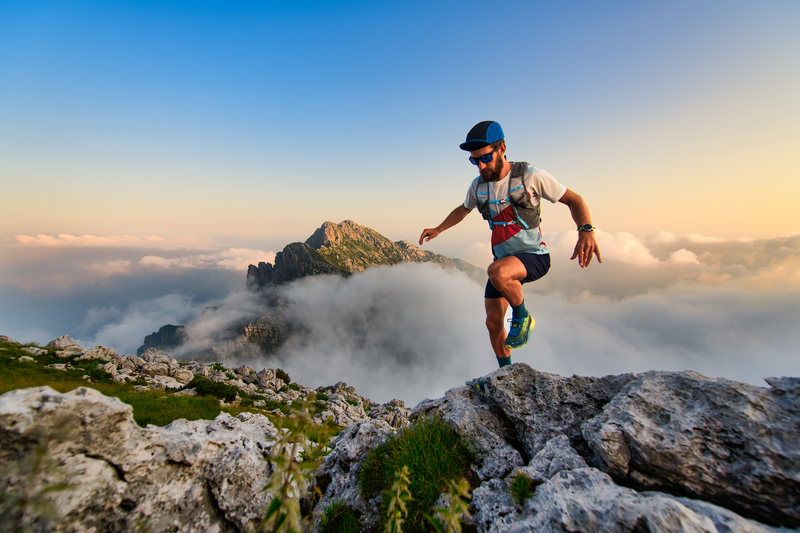
94% of researchers rate our articles as excellent or good
Learn more about the work of our research integrity team to safeguard the quality of each article we publish.
Find out more
ORIGINAL RESEARCH article
Front. Hum. Neurosci. , 30 September 2022
Sec. Brain Imaging and Stimulation
Volume 16 - 2022 | https://doi.org/10.3389/fnhum.2022.997150
This article is part of the Research Topic Advanced Neuroimaging Methods in Brain Disorders: Volume II View all 7 articles
Objectives: Autism spectrum disorder (ASD) is a juvenile onset neurodevelopmental disorder with social impairment and stereotyped behavior as the main symptoms. Unaffected relatives may also exhibit similar ASD features due to genetic factors. Although previous studies have demonstrated atypical brain morphological features as well as task-state brain function abnormalities in unaffected parents with ASD children, it remains unclear the pattern of brain function in the resting state.
Methods: A total of 42 unaffected parents of ASD children (pASD) and 39 age-, sex-, and handedness-matched controls were enrolled. Multiple resting-state fMRI (rsfMRI) analyzing methods were applied, including amplitude of low-frequency fluctuation (ALFF), regional homogeneity (ReHo), degree centrality (DC), and functional connectivity (FC), to reveal the functional abnormalities of unaffected parents in ASD-related brain regions. Spearman Rho correlation analysis between imaging metric values and the severity of ASD traits were evaluated as well.
Results: ALFF, ReHo, and DC methods all revealed abnormal brain regions in the pASD group, such as the left medial orbitofrontal cortex (mOFC) and rectal gyrus (ROI-1), bilateral supplementary motor area (ROI-2), right caudate nucleus head and right amygdala/para-hippocampal gyrus (ROI-3). FC decreasing was observed between ROI-1 and right anterior cingulate cortex (ACC), ROI-2, and bilateral precuneus. FC enhancing was observed between ROI-3 and right anterior cerebellar lobe, left medial temporal gyrus, left superior temporal gyrus, left medial frontal gyrus, left precentral gyrus, right postcentral gyrus in pASD. In addition, ALFF values in ROI-1, DC values in ROI-3 were positively correlated with AQ scores in pASD (ρ1 = 0.298, P1 = 0.007; ρ2 = 0.220, P2 = 0.040), while FC values between ROI-1 and right ACC were negatively correlated with AQ scores (ρ3 = −0.334, P3 = 0.002).
Conclusion: rsfMRI metrics could be used as biomarkers to reveal the underlying neurobiological feature of ASD for unaffected parents.
Autism spectrum disorder (ASD) is a neurodevelopmental disorder characterized by social dysfunction, stereotyped behavior, and interest inhibition (Peng et al., 2020), which is more common in preschooler and may persist throughout life (Volkmar et al., 2005; Taylor et al., 2017). The pathogenesis of ASD is complex and considered to be the interaction between genes and environmental factors (Rossignol et al., 2014). Although the etiology is still unclear, twins and family studies have shown that ASD is highly heritable, with an estimated heritability as high as 90% (Lundström et al., 2012; Tick et al., 2016; Sandin et al., 2017). Relatives of ASD children who seem to be “normal” tend to behave with mild autistic traits, such as more “subthreshold” social skills, communication characteristics, and personalities than normal people (Dell’Osso et al., 2016). These subclinical features are defined as broader autism phenotypes (BAP; Folstein and Piven, 1991; Piven et al., 1997). Early studies have confirmed that autistic traits could be observed under the threshold in family members, especially in first-degree relatives (Hughes et al., 1997; Fiorentini et al., 2012), supporting the hypothesis that ASD has genetic predisposition (Page et al., 2016).
To date, there are no clear or definitive neuropathological findings in ASD. Autopsy and structural magnetic resonance imaging studies (sMRI) suggest that there is a pathological basis in the prefrontal cortex, amygdala-hippocampal complex, cerebellar cortex, basal ganglia nucleus, and anterior and posterior cingulate gyrus in ASD individuals (Amaral et al., 2008). Functional magnetic resonance imaging (fMRI) then revealed the functional alterations in these regions. For example, task-fMRI studies have shown that in tasks related to emotion regulation or social cognition, the brain areas that make up the social networks are less activated, including amygdala, temporoparietal junction, insular, and sub-frontal cortex (Pelphrey et al., 2007; Libero et al., 2014). The resting state functional magnetic resonance imaging (rs-fMRI) studies also detected the abnormal intrinsic functional connectivity between amygdala and suboccipital cortex/sensorimotor cortex in patients with ASD (Fishman et al., 2018), as well as the vital role of the default mode network (DMN) in the dimensions of communication and social interaction (Spencer et al., 2012; Xiao et al., 2021).
For biological parents of children with ASD, the investigators hypothesize that they may have an intrinsic pattern of impaired brain function similar to that of ASD individuals based on genetic hypotheses, and it has been confirmed by previous task- fMRI studies. Baron-Cohen et al. (2006) first evaluated the visual search and advanced emotion recognition task in first-degree relatives and found that compared to healthy controls, the unaffected parents showed decreased activities not only in the visual cortex during visual tasks but also in the middle temporal gyrus during emotion recognition tasks. As language disorder is another well-known feature of ASD. Wilson et al. (2013) explored the neuronal correlation of phonological processing skills in unaffected parents and controls. It suggested the differences in activated brain areas such as bilateral supplementary motor area (SMA), posterior cingulate cortex (PCC), thalamus, cerebellum, and midbrain. However, the task design usually needs to be based on the neuroanatomical hypothesis, that is, the altered brain function observed by task-fMRI may not be comprehensive due to the unclear and inconsistent neuropathological changes in ASD till recently. In addition, successful execution of tasks highly depends on parental cooperation, which may eventually affect the activation/deactivation results of the brain area. Therefore, it is necessary to adopt a more stable method to observe the brain function in unaffected parents.
Compared with task-fMRI, rs-fMRI has more advantages in reproducibility. However, the rs-fMRI characteristics still remain elusive in unaffected parents. Only a few family studies have reported rs-fMRI abnormalities in siblings of ASD children, for example, Lin et al. (2017) tested neuroanatomical endophenotypes and associated intrinsic functional connectivity (iFC) by comparing male youth with ASD, their unaffected brothers, and typically developed males (TD), and found that unaffected brothers experienced atypical gray matter volume reduction in the middle cingulate cortex as ASD patients compared with TD men, while iFC was reduced between middle cingulate cortex and right inferior frontal gyrus. Thus, rs-fMRI methods are valuable in revealing altered brain function in family members of ASD individuals. In this present study, we applied a variety of rs-fMRI data analyses, including the amplitude of low-frequency fluctuation (ALFF), regional homogeneity (ReHo), degree centrality (DC), and functional connectivity (FC) to observe whether unaffected parents have an anomalous rs-fMRI pattern similar to that of ASD individuals. Additionally, correlations between abnormal rs-fMRI results and autism-spectrum quotient (AQ; Baron-Cohen et al., 2001) score were explored to test whether the altered brain activities were associated with the severity of autistic traits.
Forty-eight healthy parents of ASD children and 42 age-matched healthy parents of typically developed children were recruited. We used the Diagnostic and Statistical Manual of Mental Disorders (DSM-V; Battle, 2013) and Autism Behavior Checklist (ABC) to confirm the diagnosis of children with ASD. All diagnoses were made by experienced doctors in the department of child healthcare. After a detailed consultation, parents who encountered difficulties in daily life because of their intelligence level, or who were receiving treatment for any mental illness, were excluded from the study. None of the participants were clinically diagnosed as ASD. Data from nine adults were excluded due to head motion (either translation >1 mm or rotation >1° or both). Finally, we included 42 parents of children with ASD in the pASD group, and 39 parents of typically developed children as the control group (Table 1). All participants were recruited from Wuxi Children’s Hospital and Wuxi people’s Hospital. All the participants were right-handed and spoke Chinese as their first language. They filled out the AQ to measure the existence of autism traits. AQ is rated as a self-report questionnaire, with a total score ranging from 0 to 50. The higher a person’s AQ score, the closer their behavior is to an ASD individual. All participants provided a complete written informed consent to participate in the study, which was approved by the Ethics Committee of Wuxi people’s House Hospital. All methods are conducted in accordance with the guidelines and regulations of the Ethics Committee of Wuxi People’s Hospital.
MRI data were collected in a SIEMENS 3T Trio scanner using a standard head coil. The participants were instructed to rest with their eyes closed and keep still. A foam pad was used to minimize the head motion. The rs-fMRI data were acquired using gradient echo type echo planar imaging (GRE-EPI) sequence with the following parameters: TR/TE = 2,000 ms/30 ms; FA = 90°; matrix = 64 × 64; FOV = 240 × 240 mm2; slice thickness = 4 mm; and slice gap = 0.4 mm. A total of 33 axial slices were used to encompass the whole brain. Each section contained 250 volumes (500 s in total). Conventional MRI brain images such as T1WI, T2WI, DWI, and T2-FLAIR were collected to exclude anatomic abnormalities, and parameters were listed as follows, T1WI: TR/TE = 500 ms/8.5 ms; matrix = 120 × 256; FOV = 230 × 230 mm2; slice thickness = 5 mm; slice gap = 1.5 mm; slice number = 20. T2WI: TR/TE = 5,000 ms/117 ms; matrix = 248 × 320; FOV = 220 × 220 mm2; slice thickness = 5 mm; slice gap = 1 mm; slice number = 20. DWI: TR/TE = 5,100 ms/100 ms; matrix = 192 × 192; FOV = 230 × 230 mm2; slice thickness = 5 mm; slice gap = 0.4 mm; slice number = 20; b-value = (1,000). T2-FLAIR: TR/TE = 8,000 ms/94 ms; matrix = 186 × 256; FOV = 230 × 230 mm2; slice thickness = 5 mm; slice gap = 1 mm; slice number = 20.
The rs-fMRI data were preprocessed using the toolkit of Data Processing Assistant for Brain imaging (DPABI_V6.1_220101; Yan et al., 2016), a MATLAB (R2018b) Toolbox for “Pipeline” data analysis of functional images. The first 10 volumes of each participant were removed. The remaining 240 scans were slice-time corrected and then realigned to the first volume to correct the head motions. No translation or rotation parameters in any given dataset exceeded ±1 mm or ±1°. All realigned images were warped into a standard stereotaxic space at a 3 × 3 × 3 mm3 resolution, according to the Montreal Neurological Institute (MNI) echo-planar imaging template. A detrending was performed after regressing out the nuisance covariates, including white matter, cerebrospinal fluid, and global signals.
The data were spatially smoothed by convolution with a 6 mm FWHM (full width at half maximum) isotropic Gaussian kernel, to reduce the influence of deformation and noise in the standardization process, improve the signal-to-noise ratio and statistical efficiency, and enhance the image effect. Then band-pass filtering (0.01–0.08 Hz) is applied to the time series to eliminate the influence of low-frequency drift and high-frequency noise. ALFF is calculated based on the fast Fourier transform (FFT), and the time series of each voxel is transformed into the frequency domain without bandpass filtering. The square root of each frequency of the power spectrum is calculated, and then the mean square root of each voxel in the 0.01–0.08 Hz band is obtained. The last step is to divide the ALFF of each voxel by the global average of ALFF values (mALFF; Zang et al., 2007).
The ReHo brain map was constructed by calculating the Kendall coefficient of time series consistency between each voxel and its neighboring voxels. The average ReHo value of the whole brain was subtracted from that of each voxel and then divided by the standard deviation for subsequent analysis. The KCC-ReHo values of all individual voxel directions are calculated and normalized to the KCC-ReHo Z-value (Zuo et al., 2013). Finally, a 6 mm FWHM Gaussian kernel is used for smoothing to make a comparison between groups.
For each voxel, the time process of the time series is extracted, and the Pearson correlation coefficient with other voxels in the brain is calculated. The Pearson correlation coefficient matrix between any pair of voxels was obtained to construct the whole brain functional connection matrix of each participant. Finally, the binary DC was calculated and then smoothed with a 6 mm FWHM Gaussian kernel for inter-group comparison (Zuo et al., 2012).
The above three rs-fMRI metrics (ALFF, ReHo, DC) were evaluated by a two-sample t-test, and the cluster that survived was selected as seed to calculate FC based on a whole brain voxel level. Subsequently, the FC value after Z transformation was evaluated by a two-sample t-test to observe whether there is a surviving cluster. The statistical results were regressed by sex, age, and head motion (using mean FD), and corrected using GRF (voxel P < 0.005, cluster P < 0.05).
ALFF, ReHo, DC, and FC values were extracted from the corresponding survival cluster. Spearman Rho correlation analysis was performed between the above metric value and AQ score, respectively. All the results were considered significant at P < 0.05.
ALFF: Compared to the control group, ALFF increased significantly in the left medial orbitofrontal cortex (mOFC) and left rectal gyrus in pASD (Table 2). The cluster was marked as ROI-1 (Figure 1A).
Figure 1. (A) Intergroup comparison of ALFF: Compared with the control group, ALFF was significantly increased in the left mOFC and rectal gyrus (ROI-1) in pASD than that of the control group [Peak MNI coordinate = (−3, 21, −18), Peak intensity = 3.77]. (B) Intergroup comparison of FC based on ALFF seed (ROI-1): FC in right ACC was significantly attenuated in pASD compared with the controls [Peak MNI coordinate = (6, 51, 12), Peak intensity = −3.86]. The results of (A,B) were regressed for sex, age, and head motion, and were corrected using GRF (voxel P < 0.005, cluster P < 0.05). The second row of pictures in (B) presented the projection of the FC results onto the cortical surface. (C,D) Spearman Rho correlation analysis: the mALFF values of ROI-1 were positively correlated with AQ scores (ρ = 0.298, P = 0.007); zFC values between ROI-1/right ACC were negatively correlated with AQ scores (ρ = −0.334, P = 0.002). The results of (C,D) were considered statistically different at P < 0.05. Abbreviation: mOFC, medial orbitofrontal cortex; ACC, anterior cingulate cortex; mALFF, mean ALFF; zFC, z-transformed FC.
Table 2. Brain regions with significant differences of ALFF, ReHo, DC, and FC between the pASD and control group.
ReHo: The ReHo values of SMA on both sides of pASD were significantly higher than those in the control group (Table 2). The cluster was marked as ROI-2 (Figure 2A).
Figure 2. (A) Intergroup comparison of ReHo: compared with the control group, the ReHo values of bilateral supplementary motor areas (SMA) (ROI-2) of pASD were significantly higher than those of the control group [Peak MNI coordinate = (−6, 6, 60), Peak intensity = 4.27]. (B) Intergroup comparison of FC based on ReHo seed (ROI-2): compared with the controls, FC in the bilateral precuneus of pASD was significantly decreased [Peak MNI coordinate = (0, −72, 48), Peak intensity = −3.48]. The results of (A,B) were regressed for sex, age, and head motion, and were corrected using GRF (voxel P < 0.005, cluster P < 0.05). The second row of pictures in (B) presented the projection of the FC results onto the cortical surface. (C,D) Spearman Rho correlation analysis: the result between smoothed mReHo values of ROI-3 and AQ scores was ρ = 0.001, P = 0.994, and the result between zFC value of ROI-3/right ACC and AQ score was ρ = 0.130, P = 0.248. Neither result of (C) or (D) was considered statistically different at P < 0.05. Abbreviation: SMA, supplementary motor areas; mReHo, mean ReHo; zFC, z-transformed FC.
DC: The DC values of the right caudate nucleus head, right amygdale, and para-hippocampal in pASD were significantly higher than those in the control group (Table 2). The cluster was marked as ROI-3 (Figure 3A).
Figure 3. (A) Intergroup comparison of DC: DC values were significantly higher in the right caudate head, right amygdala, and para-hippocampal gyrus (ROI-3) of pASD compared with controls [Peak MNI coordinate = (12, 18, 3), Peak intensity = 4.17]. (B) Intergroup comparison of FC based on DC seed (ROI-3): compared with normal controls, pASD showed significantly enhanced FC in right anterior cerebellar lobe, left MTG, left STG, left MFG, left precentral gyrus, and right postcentral gyrus [Peak MNI coordinate = (12, −51, −15), Peak intensity = 4.78]. The results of (A,B) were regressed for sex, age, and head motion, and corrected using GRF (voxel P < 0.005, cluster P < 0.05). The second row of pictures in (B) presented the projection of the FC results onto the cortical surface. (C,D) Spearman Rho correlation analysis: the smoothed mDC values of ROI-3 were positively correlated with AQ scores (ρ = 0.220, P = 0.040). While the results between zFC values (generated from ROI-3) and AQ scores was ρ = 0.171, P = 0.127, which was not statistically correlated. The results of (C,D) were considered statistically different at P < 0.05. Abbreviation: MTG, medial temporal gyrus; STG, superior temporal gyrus; MFG, medial frontal gyrus; mDC, mean DC; zFC, z-transformed FC.
FC: For ROI-1 (left mOFC and rectal gyrus), the FC of pASD in the right anterior cingulate cortex (ACC) was significantly lower than that in the control group (Figure 1B, Table 2). For ROI-2 (bilateral SMA), the FC of pASD in the bilateral precuneus was significantly decreased compared with the control group (Figure 2B, Table 2). For ROI-3 (right caudate nucleus head, right amygdale, and para-hippocampal gyrus), the FC of pASD was significantly enhanced in the right anterior cerebellar lobe, left medial temporal gyrus (MTG), left superior temporal gyrus (STG), left medial frontal gyrus (MFG), left precentral gyrus, and right postcentral gyrus, compared with normal controls (Figure 3B, Table 2).
The mALFF value of ROI-1 (left mOFC and rectal gyrus) was positively correlated with AQ score (ρ = 0.298, P = 0.007; Figure 1C). The DC value of ROI-3 (right caudate nucleus head, right amygdala, and para-hippocampal gyrus) was positively correlated with the AQ score (ρ = 0.220, P = 0.040; Figure 3C). The FC value between ROI-1 (left mOFC and rectal gyrus) and right ACC was negatively correlated with AQ score (ρ = −0.334, P = 0.002; Figure 1D). Meanwhile, there was no correlation between ReHo value of ROI-2 (bilateral SMA) and AQ score (Figure 2C). FC results calculated from either ROI-2 or ROI-3 were not correlated with AQ scores (Figures 2D, 3D).
One of our aims was to investigate whether resting brain function differs between unaffected parents with ASD children and parents with typically developing children. By calculating rs-fMRI metrics including ALFF, ReHo, DC, we found that spontaneous activity in left mOFC and rectal gyrus, bilateral SMA, right caudate nucleus head, right amygdala, and para-hippocampal gyrus was significantly enhanced in unaffected parents compared with normal controls. Abnormalities in whole-brain voxel level were also present in FC results based on the brain regions described above. In addition, we found that brain regions with abnormally increased ALFF and DC values were positively correlated with AQ scores; while FC values based on ALFF results were negatively correlated with AQ scores. Correlation analysis results indicated that unaffected parents of children with ASD may have subclinical elevations in ASD traits.
One of the core symptoms in ASD individuals is social deficits, and the amygdala plays an important role in social and emotional interactions as a key component of the social brain (Hadjikhani et al., 2017; Lassalle et al., 2017). ASD-related abnormalities in the amygdala have already been reported in studies focusing on assessing functions such as facial and emotional expression (Baron-Cohen et al., 2000). However, other studies have also found the deactivation of the amygdala in ASD (Pierce and Redcay, 2008; Lassalle et al., 2017). Therefore, mechanistic abnormalities associated with the amygdala remain to be elucidated. A meta-analysis of ts-fMRI (Peng et al., 2020) indicated abnormal activation in social brain regions in ASD individuals compared with controls, featured by a characteristic over-activation of the right amygdala. This is consistent with the findings of the DC approach in this study. DC is a data-driven method based on Eigenvector Centrality Mapping (ECM), which can accurately and objectively detect all brain regions as communication hubs, and these hubs have stronger connectivity with other parts of the brain (Lohmann et al., 2010). We observed higher DC in the right amygdala and para-hippocampal gyrus in unaffected parents than in controls, suggesting that this node may also be an important communication hub in pASD. In addition, the global FC results based on right amygdala and para-hippocampal gyrus in this study further showed wide range of long-distance FC abnormalities by this central hub, such as enhanced FC with anterior cerebellar lobe, left MTG, left STG, left MFG, left precentral gyrus, and right postcentral gyrus. Functional connectivity abnormalities involved in these brain regions might be the basis of visuomotor deficits in ASD individuals (Guo et al., 2017). Therefore, we speculate that similar to ASD individuals, the centrality of the right amygdala and para-hippocampal complex and its FC pathways may be responsible for causes if the parents present subthreshold visual deficits related to ASD. Furthermore, as ASD individuals typically demonstrate enhanced short-distance FC and diminished long-distance FC when performing tasks (May and Kana, 2020), enhanced long-distance FC is more likely to reflect functional compensation of unaffected parents on this pathway. We also found the increased DC value in the right caudate head of pASD, indicating the importance of this hub. Anatomically, the caudate head is adjacent to the amygdala, so there might be a structural basis that partially coincides with the functional connectivity pathway in which the amygdala resides (Jung et al., 2013). Caudate head participates in the regulation of the reward circuit (Dichter et al., 2012; Kohls et al., 2013), and meta-analyses on social reward mechanisms have shown that ASD individuals experience a large number of missing clusters, as shown by hypoactivation, in social reward circuits in the bilateral caudate head during social stimulation test and are negatively correlated with the social scale rating in ASD individuals (Clements et al., 2018). Although only the abnormality of right caudate head was observed in this study, the positive correlation between DC value and AQ score suggested that dysregulation in the reward circuit of social stimuli may have emerged in pASD compared with the controls.
The medial prefrontal cortex (mPFC) is also crucial in the social brain, and mOFC is one of the most repeatedly studied mPFC structures (Mori et al., 2013; Jonker et al., 2015). mOFC plays a significant role in the process of social reinforcement and reward processes, and its impairment not only affects the completion of executive task function but also affects cognitive integrity (Pina-Camacho et al., 2012; Nestor et al., 2013). Executive disability is one of the major manifestations in ASD individuals (Happé and Frith, 2006), and meta-analysis studies have revealed that ASD individuals have significantly enhanced activation of the mOFC when performing various tasks associated with executive function, such as attention pointing and shifting, spatial attention tasks, and key press responses, compared with normal controls (Schmitz et al., 2006). In this present study, we found that ALFF was increased in the left mOFC of pASD compared with controls at rest, which implies the spontaneous activity of local neurons is significantly enhanced, suggesting that its executive function may have been affected and necessitating stronger spontaneous brain activity to cope. Further rsFC results based on left mOFC showed that pASD had significantly attenuated rsFC in right ACC, however, none of the available studies reported the mOFC/ACC pathway separately in ASD individuals. The decreased rsFC between left mOFC/right ACC found in this study reflects abnormal functional connectivity between the left and right cerebral hemispheres. Based on the cortical underconnectivity theory, aberrant patterns of rsFC in ASD can be described as local overconnectivity, whereas long-distance as underconnectivity (Courchesne and Pierce, 2005; Lau et al., 2019). As a typical long-distance connection, interhemispheric rsFC can be used as an important indicator to describe interhemispheric synchronization abnormalities in spontaneous neural activity in ASD. Abnormal rsFC across hemispheres in pASD presented in this study suggests that the medial prefrontal cortex, particularly the left mOFC, of unaffected parents, has decreased synchronization of long-distance spontaneous neuronal activity with the right ACC, which is similar to interhemispheric rsFC patterns in typical ASD individuals (Lau et al., 2019). In addition, early developmental impairment of the orbitofrontal-amygdala loop is critical to brain injury in ASD individuals, and both socio-emotional cognitive and self-regulation behavioral disorders are associated with deficits in this circuit (Watanabe et al., 2014). However, no abnormal rsFC between mOFC and amygdala was observed in pASD in this study, which suggests that the social cognition and behavior norms of unaffected parents may still be regarded as normal.
A quantitative meta-analysis of rs-fMRI in ASD individuals showed that the spontaneous activity of right SMA, middle frontal gyrus, inferior frontal gyrus, left precentral gyrus, and bilateral cerebellar hemispheric lobules (VIII/IX) in ASD patients was significantly higher than that of the controls (Wang et al., 2018). In this study, by applying the ReHo method, it was also found that the local consistency of bilateral SMA in pASD was significantly higher than that in the controls, indicating that the abnormal spontaneous activity of SMA may be a common rs-fMRI feature of ASD individuals and unaffected parents (Maximo et al., 2013; Itahashi et al., 2015; Dajani and Uddin, 2016). SMA is responsible for planning and organizing movements, especially visual movements that require selection and execution (Mosconi and Sweeney, 2015). ASD individuals experience general motor control and motor coordination dysfunction in addition to core symptoms such as social impairment (Gallese et al., 2013), therefore, functional abnormalities in SMA are likely to be associated with the aforementioned motor disorders. However, the neural mechanisms involved in motor dysfunction in ASD are unclear (Mosconi and Sweeney, 2015). A task-fMRI study based on the visual motor task paradigm (Lepping et al., 2022) showed that ASD individuals had an equal proportion of significantly increased activation in bilateral SMA, bilateral superior parietal lobule, and contralateral middle/inferior frontal gyrus with increasing visual gain, indicating that the ability to process increased visual feedback was compromised. Meanwhile, FC between parietal sensory processing and frontal cortex motor control area was weakened during behavior, suggesting that the functional integration of the parietal lobe and frontal lobe region was lower in ASD individuals. The decreased rs-FC in SMA/precuneus in pASD derived from this study has some similar characteristics to the above results, that is, the degree of functional integration in parietal and frontal lobe was reduced, and it is speculated that unaffected parents may be more likely to have intrinsic functional abnormalities in motor selection and execution. At the same time, rs-FC between SMA and precuneus belongs to long-distance connectivity, and the underconnectivity of this pathway shown by pASD has a similar impaired pattern as the long-distance under connectivity of rsFC reported for ASD individuals (Courchesne and Pierce, 2005; Lau et al., 2019).
In the present study, ALFF values in the left mOFC and rectal gyrus, DC values in the right caudate head and the right amygdala/para-hippocampal gyrus were associated with higher AQ scores in pASD, which referred to higher level of autistic traits. Both ROIs belong to prominent hubs of the social brain network (Sato and Uono, 2019), and it was speculated that pASD was likely to have subthreshold social disorders. On the other hand, there was a negative correlation between rs-FC results based on left mOFC/rectal gyrus and AQ scores, suggesting that this brain region may be more likely to reflect the underlying neurobiological features of ASD.
Limitations: In the current study, we focused on the changes in rs-fMRI patterns of unaffected parents with ASD children, and in the future, we need to investigate the potential coupling alterations in structure-function in key brain regions of unaffected parents. In addition, further studies are needed to investigate the relationship between neuroimaging and gene expression to provide more conclusive evidence for searching subthreshold ASD-related neuroimaging endophenotype.
We identified regional spontaneous brain activity abnormalities in multiple brain regions in unaffected parents with ASD children, as well as corresponding rsFC changes, by analyzing multiple rs-fMRI metrics. These abnormal brain regions all belong to the important nodes of brain damage in ASD individuals, and the abnormal patterns of rsFC analyzed by these brain regions also have similarities with the impaired patterns in ASD individuals. Particularly, spontaneous brain activity in the left mOFC and the left rectal gyrus, as well as the global FC generated from this node, were associated with higher level of autistic traits, indicating that rs-fMRI abnormalities in this area may be more reflective of a underlying neurobiological feature of ASD for unaffected parents.
The original contributions presented in the study are included in the article, further inquiries can be directed to the corresponding author/s.
The studies involving human participants were reviewed and approved by Wuxi People’s Hospital Clinical New Technology and Scientific Research Ethics Committee. The patients/participants provided their written informed consent to participate in this study.
X-WZ, L-LZ, Z-MZ, L-YW, Z-XD, and X-MF contributed to data collection, data and statistical analyses, and wrote the manuscript. All authors contributed to the article and approved the submitted version.
This work was supported by Department of Health of Zhejiang Province (grant numbers: 2021418656 and 2022519634).
The authors declare that the research was conducted in the absence of any commercial or financial relationships that could be construed as a potential conflict of interest.
All claims expressed in this article are solely those of the authors and do not necessarily represent those of their affiliated organizations, or those of the publisher, the editors and the reviewers. Any product that may be evaluated in this article, or claim that may be made by its manufacturer, is not guaranteed or endorsed by the publisher.
Amaral, D. G., Schumann, C. M., and Nordahl, C. W. (2008). Neuroanatomy of autism. Trends Neurosci. 31, 137–145. doi: 10.1016/j.tins.2007.12.005
Baron-Cohen, S., Ring, H. A., Bullmore, E. T., Wheelwright, S., Ashwin, C., and Williams, S. C. (2000). The amygdala theory of autism. Neurosci. Biobehav. Rev. 24, 355–364. doi: 10.1016/s0149-7634(00)00011-7
Baron-Cohen, S., Ring, H., Chitnis, X., Wheelwright, S., Gregory, L., Williams, S., et al. (2006). fMRI of parents of children with Asperger Syndrome: a pilot study. Brain Cogn. 61, 122–130. doi: 10.1016/j.bandc.2005.12.011
Baron-Cohen, S., Wheelwright, S., Skinner, R., Martin, J., and Clubley, E. (2001). The autism-spectrum quotient (AQ): evidence from Asperger syndrome/high-functioning autism, males and females, scientists and mathematicians. J. Autism Dev. Disord. 31, 5–17. doi: 10.1023/a:1005653411471
Battle, D. E. (2013). Diagnostic and statistical manual of mental disorders (DSM). Codas 25, 191–192. doi: 10.1023/a:1005653411471
Clements, C. C., Zoltowski, A. R., Yankowitz, L. D., Yerys, B. E., Schultz, R. T., and Herrington, J. D. (2018). Evaluation of the social motivation hypothesis of autism: a systematic review and meta-analysis. JAMA Psychiatry 75, 797–808. doi: 10.1001/jamapsychiatry.2018.1100
Courchesne, E., and Pierce, K. (2005). Why the frontal cortex in autism might be talking only to itself: local over-connectivity but long-distance disconnection. Curr. Opin. Neurobiol. 15, 225–230. doi: 10.1016/j.conb.2005.03.001
Dajani, D. R., and Uddin, L. Q. (2016). Local brain connectivity across development in autism spectrum disorder: A cross-sectional investigation. Autism Res. 9, 43–54. doi: 10.1002/aur.1494
Dell’Osso, L., Dalle Luche, R., and Maj, M. (2016). Adult autism spectrum as a transnosographic dimension. CNS Spectr. 21, 131–133. doi: 10.1017/S1092852915000450
Dichter, G. S., Richey, J. A., Rittenberg, A. M., Sabatino, A., and Bodfish, J. W. (2012). Reward circuitry function in autism during face anticipation and outcomes. J. Autism Dev. Disord. 42, 147–160. doi: 10.1007/s10803-011-1221-1
Fiorentini, C., Gray, L., Rhodes, G., Jeffery, L., and Pellicano, E. (2012). Reduced face identity aftereffects in relatives of children with autism. Neuropsychologia 50, 2926–2932. doi: 10.1016/j.neuropsychologia.2012.08.019
Fishman, I., Linke, A. C., Hau, J., Carper, R. A., and Muller, R. A. (2018). Atypical functional connectivity of amygdala related to reduced symptom severity in children with autism. J. Am. Acad. Child Adolesc. Psychiatry 57, 764–774.e3. doi: 10.1016/j.jaac.2018.06.015
Folstein, S. E., and Piven, J. (1991). Etiology of autism: genetic influences. Pediatrics 87, 767–773. doi: 10.1542/peds.87.5.767
Gallese, V., Rochat, M. J., and Berchio, C. (2013). The mirror mechanism and its potential role in autism spectrum disorder. Dev. Med. Child Neurol. 55, 15–22. doi: 10.1111/j.1469-8749.2012.04398.x
Guo, X., Chen, H., Long, Z., Duan, X., Zhang, Y., and Chen, H. (2017). Atypical developmental trajectory of local spontaneous brain activity in autism spectrum disorder. Sci. Rep. 7:39822. doi: 10.1038/srep39822
Hadjikhani, N., Åsberg Johnels, J., Zürcher, N. R., Lassalle, A., Guillon, Q., Hippolyte, L., et al. (2017). Look me in the eyes: constraining gaze in the eye-region provokes abnormally high subcortical activation in autism. Sci. Rep. 7:3163. doi: 10.1038/s41598-017-03378-5
Happé, F., and Frith, U. (2006). The weak coherence account: detail-focused cognitive style in autism spectrum disorders. J. Autism Dev. Disord. 36, 5–25. doi: 10.1007/s10803-005-0039-0
Hughes, C., Leboyer, M., and Bouvard, M. (1997). Executive function in parents of children with autism. Psychol. Med. 27, 209–220. doi: 10.1017/s0033291796004308
Itahashi, T., Yamada, T., Watanabe, H., Nakamura, M., Ohta, H., Kanai, C., et al. (2015). Alterations of local spontaneous brain activity and connectivity in adults with high-functioning autism spectrum disorder. Mol. Autism 6:30. doi: 10.1186/s13229-015-0026-z
Jonker, F. A., Jonker, C., Scheltens, P., and Scherder, E. J. (2015). The role of the orbitofrontal cortex in cognition and behavior. Rev. Neurosci. 26, 1–11. doi: 10.1515/revneuro-2014-0043
Jung, W. H., Kim, S. N., Lee, T. Y., Jang, J. H., Choi, C. H., Kang, D. H., et al. (2013). Exploring the brains of Baduk (Go) experts: gray matter morphometry, resting-state functional connectivity and graph theoretical analysis. Front. Hum. Neurosci. 7:633. doi: 10.3389/fnhum.2013.00633
Kohls, G., Schulte-Rüther, M., Nehrkorn, B., Müller, K., Fink, G. R., Kamp-Becker, I., et al. (2013). Reward system dysfunction in autism spectrum disorders. Soc. Cogn. Affect. Neurosci. 8, 565–572. doi: 10.1093/scan/nss033
Lassalle, A., Åsberg Johnels, J., Zürcher, N. R., Hippolyte, L., Billstedt, E., Ward, N., et al. (2017). Hypersensitivity to low intensity fearful faces in autism when fixation is constrained to the eyes. Hum. Brain Mapp. 38, 5943–5957. doi: 10.1002/hbm.23800
Lau, W. K. W., Leung, M.-K., and Lau, B. W. M. (2019). Resting-state abnormalities in autism spectrum disorders: a meta-analysis. Sci. Rep. 9:3892. doi: 10.1038/s41598-019-40427-7
Lepping, R. J., Mckinney, W. S., Magnon, G. C., Keedy, S. K., Wang, Z., Coombes, S. A., et al. (2022). Visuomotor brain network activation and functional connectivity among individuals with autism spectrum disorder. Hum. Brain Mapp. 43, 844–859. doi: 10.1002/hbm.25692
Libero, L. E., Maximo, J. O., Deshpande, H. D., Klinger, L. G., Klinger, M. R., and Kana, R. K. (2014). The role of mirroring and mentalizing networks in mediating action intentions in autism. Mol. Autism 5:50. doi: 10.1186/2040-2392-5-50
Lin, H. Y., Tseng, W. I., Lai, M. C., Chang, Y. T., and Gau, S. S. (2017). Shared atypical brain anatomy and intrinsic functional architecture in male youth with autism spectrum disorder and their unaffected brothers. Psychol. Med. 47, 639–654. doi: 10.1017/S0033291716002695
Lohmann, G., Margulies, D. S., Horstmann, A., Pleger, B., Lepsien, J., Goldhahn, D., et al. (2010). Eigenvector centrality mapping for analyzing connectivity patterns in fMRI data of the human brain. PLoS One 5:e10232. doi: 10.1371/journal.pone.0010232
Lundström, S., Chang, Z., Råstam, M., Gillberg, C., Larsson, H., Anckarsäter, H., et al. (2012). Autism spectrum disorders and autistic like traits: similar etiology in the extreme end and the normal variation. Arch. Gen. Psychiatry 69, 46–52. doi: 10.1001/archgenpsychiatry.2011.144
Maximo, J. O., Keown, C. L., Nair, A., and Müller, R. A. (2013). Approaches to local connectivity in autism using resting state functional connectivity MRI. Front. Hum. Neurosci. 7:605. doi: 10.3389/fnhum.2013.00605
May, K. E., and Kana, R. K. (2020). Frontoparietal network in executive functioning in autism spectrum disorder. Autism Res. 13, 1762–1777. doi: 10.1002/aur.2403
Mori, K., Toda, Y., Ito, H., Mori, T., Goji, A., Fujii, E., et al. (2013). A proton magnetic resonance spectroscopic study in autism spectrum disorders: amygdala and orbito-frontal cortex. Brain Dev. 35, 139–145. doi: 10.1016/j.braindev.2012.09.016
Mosconi, M. W., and Sweeney, J. A. (2015). Sensorimotor dysfunctions as primary features of autism spectrum disorders. Sci. China Life Sci. 58, 1016–1023. doi: 10.1007/s11427-015-4894-4
Nestor, P. G., Nakamura, M., Niznikiewicz, M., Thompson, E., Levitt, J. J., Choate, V., et al. (2013). In search of the functional neuroanatomy of sociality: MRI subdivisions of orbital frontal cortex and social cognition. Soc. Cogn. Affect. Neurosci. 8, 460–467. doi: 10.1093/scan/nss018
Page, J., Constantino, J. N., Zambrana, K., Martin, E., Tunc, I., Zhang, Y., et al. (2016). Quantitative autistic trait measurements index background genetic risk for ASD in Hispanic families. Mol. Autism 7:39. doi: 10.1186/s13229-016-0100-1
Pelphrey, K. A., Morris, J. P., Mccarthy, G., and Labar, K. S. (2007). Perception of dynamic changes in facial affect and identity in autism. Soc. Cogn. Affect. Neurosci. 2, 140–149. doi: 10.1093/scan/nsm010
Peng, Z., Chen, J., Jin, L., Han, H., Dong, C., Guo, Y., et al. (2020). Social brain dysfunctionality in individuals with autism spectrum disorder and their first-degree relatives: an activation likelihood estimation meta-analysis. Psychiatry Res. Neuroimaging 298:111063. doi: 10.1016/j.pscychresns.2020.111063
Pierce, K., and Redcay, E. (2008). Fusiform function in children with an autism spectrum disorder is a matter of “who”. Biol. Psychiatry 64, 552–560. doi: 10.1016/j.biopsych.2008.05.013
Pina-Camacho, L., Villero, S., Fraguas, D., Boada, L., Janssen, J., Navas-Sánchez, F. J., et al. (2012). Autism spectrum disorder: does neuroimaging support the DSM-5 proposal for a symptom dyad? A systematic review of functional magnetic resonance imaging and diffusion tensor imaging studies. J. Autism Dev. Disord. 42, 1326–1341. doi: 10.1007/s10803-011-1360-4
Piven, J., Palmer, P., Jacobi, D., Childress, D., and Arndt, S. (1997). Broader autism phenotype: evidence from a family history study of multiple-incidence autism families. Am. J. Psychiatry 154, 185–190. doi: 10.1176/ajp.154.2.185
Rossignol, D. A., Genuis, S. J., and Frye, R. E. (2014). Environmental toxicants and autism spectrum disorders: a systematic review. Transl. Psychiatry 4:e360. doi: 10.1038/tp.2014.4
Sandin, S., Lichtenstein, P., Kuja-Halkola, R., Hultman, C., Larsson, H., and Reichenberg, A. (2017). The heritability of autism spectrum disorder. JAMA 318, 1182–1184. doi: 10.1001/jama.2017.12141
Sato, W., and Uono, S. (2019). The atypical social brain network in autism: advances in structural and functional MRI studies. Curr. Opin. Neurol. 32, 617–621. doi: 10.1097/WCO.0000000000000713
Schmitz, N., Rubia, K., Daly, E., Smith, A., Williams, S., and Murphy, D. G. (2006). Neural correlates of executive function in autistic spectrum disorders. Biol. Psychiatry 59, 7–16. doi: 10.1016/j.biopsych.2005.06.007
Spencer, M. D., Chura, L. R., Holt, R. J., Suckling, J., Calder, A. J., Bullmore, E. T., et al. (2012). Failure to deactivate the default mode network indicates a possible endophenotype of autism. Mol. Autism 3:15. doi: 10.1186/2040-2392-3-15
Taylor, M. J., Gillberg, C., Lichtenstein, P., and Lundström, S. (2017). Etiological influences on the stability of autistic traits from childhood to early adulthood: evidence from a twin study. Mol. Autism 8:5. doi: 10.1186/s13229-017-0120-5
Tick, B., Bolton, P., Happé, F., Rutter, M., and Rijsdijk, F. (2016). Heritability of autism spectrum disorders: a meta-analysis of twin studies. J. Child Psychol. Psychiatry 57, 585–595. doi: 10.1111/jcpp.12499
Volkmar, F., Chawarska, K., and Klin, A. (2005). Autism in infancy and early childhood. Annu. Rev. Psychol. 56, 315–336. doi: 10.1146/annurev.psych.56.091103.070159
Wang, W., Liu, J., Shi, S., Liu, T., Ma, L., Ma, X., et al. (2018). Altered resting-state functional activity in patients with autism spectrum disorder: a quantitative meta-analysis. Front. Neurol. 9:556. doi: 10.3389/fneur.2018.00556
Watanabe, H., Nakamura, M., Ohno, T., Itahashi, T., Tanaka, E., Ohta, H., et al. (2014). Altered orbitofrontal sulcogyral patterns in adult males with high-functioning autism spectrum disorders. Soc. Cogn. Affect. Neurosci. 9, 520–528. doi: 10.1093/scan/nst016
Wilson, L. B., Tregellas, J. R., Slason, E., Pasko, B. E., Hepburn, S., and Rojas, D. C. (2013). Phonological processing in first-degree relatives of individuals with autism: an fMRI study. Hum. Brain Mapp. 34, 1447–1463. doi: 10.1002/hbm.22001
Xiao, J., Chen, H., Shan, X., He, C., Li, Y., Guo, X., et al. (2021). Linked social-communication dimensions and connectivity in functional brain networks in autism spectrum disorder. Cereb. Cortex 31, 3899–3910. doi: 10.1093/cercor/bhab057
Yan, C.-G., Wang, X.-D., Zuo, X.-N., and Zang, Y.-F. (2016). DPABI: data processing & analysis for (resting-state) brain imaging. Neuroinformatics 14, 339–351. doi: 10.1007/s12021-016-9299-4
Zang, Y.-F., He, Y., Zhu, C.-Z., Cao, Q.-J., Sui, M.-Q., Liang, M., et al. (2007). Altered baseline brain activity in children with ADHD revealed by resting-state functional MRI. Brain Dev. 29, 83–91. doi: 10.1016/j.braindev.2006.07.002
Zuo, X.-N., Ehmke, R., Mennes, M., Imperati, D., Castellanos, F. X., Sporns, O., et al. (2012). Network centrality in the human functional connectome. Cereb. Cortex 22, 1862–1875. doi: 10.1093/cercor/bhr269
Keywords: autism, unaffected parents, resting-state, functional magnetic resonance imaging, brain activity, functional connectivity
Citation: Zhu X-W, Zhang L-L, Zhu Z-M, Wang L-Y, Ding Z-X and Fang X-M (2022) Altered intrinsic brain activity and connectivity in unaffected parents of individuals with autism spectrum disorder: a resting-state fMRI study. Front. Hum. Neurosci. 16:997150. doi: 10.3389/fnhum.2022.997150
Received: 18 July 2022; Accepted: 16 September 2022;
Published: 30 September 2022.
Edited by:
Wei Liao, University of Electronic Science and Technology of China, ChinaReviewed by:
Yuan Wang, First Affiliated Hospital of Xi’an Jiaotong University, ChinaCopyright © 2022 Zhu, Zhang, Zhu, Wang, Ding and Fang. This is an open-access article distributed under the terms of the Creative Commons Attribution License (CC BY). The use, distribution or reproduction in other forums is permitted, provided the original author(s) and the copyright owner(s) are credited and that the original publication in this journal is cited, in accordance with accepted academic practice. No use, distribution or reproduction is permitted which does not comply with these terms.
*Correspondence: Zhong-Xiang Ding, aGFuZ3pob3Vkeng3M0AxMjYuY29t; Xiang-Ming Fang, eGlhbmdtaW5nX2ZhbmdAbmptdS5lZHUuY24=
† These authors have contributed equally to this work and share first authorship
Disclaimer: All claims expressed in this article are solely those of the authors and do not necessarily represent those of their affiliated organizations, or those of the publisher, the editors and the reviewers. Any product that may be evaluated in this article or claim that may be made by its manufacturer is not guaranteed or endorsed by the publisher.
Research integrity at Frontiers
Learn more about the work of our research integrity team to safeguard the quality of each article we publish.