- 1Phase I Clinical Trial Center, Chongqing University Cancer Hospital, Chongqing, China
- 2School of Nursing, Medical College of Soochow University, Suzhou, China
- 3Department of Radiology, The First Affiliated Hospital of Soochow University, Suzhou, China
- 4Department of Gerontology, The First Affiliated Hospital of Soochow University, Suzhou, China
- 5Department of Neurology, The First Affiliated Hospital of Soochow University, Suzhou, China
Objectives: In recent years, the desire to make a more fine-grained identification on mild cognitive impairment (MCI) has become apparent, the etiological diagnosis of MCI in particular. Nevertheless, new methods for the etiological diagnosis of MCI are currently insufficient. The objective of this study was to establish discriminative measures for amnestic mild cognitive impairment (a-MCI) and MCI caused by cerebral small vessel disease (CSVD).
Materials and methods: In total, 20 normal controls (NCs), 33 a-MCI patients, and 25 CSVD-MCI patients performed comprehensive neuropsychological assessments concerning global cognitive function and five cognitive domains as well as magnetic resonance imaging scan with diffusion tensor imaging (DTI). Diffusion parameters including fractional anisotropy and mean diffusivity of 20 major white matter metrics were obtained by ROI-based analyses. The neuropsychological tests and diffusion measurements were compared and binary logistic regression was used to identify the best differential indicator for the two MCI subgroups. The discriminating power was calculated by receiver operating characteristic analysis.
Results: Amnestic mild cognitive impairment group showed significant impairment in memory and language function, while CSVD-MCI group revealed more deficits in multi-cognitive domains of memory, language, attention and executive function than controls. Compared to the a-MCI, CSVD-MCI was significantly dysfunctional in the executive function. The CSVD-MCI group had decreased fractional anisotropy and increased mean diffusivity values throughout widespread white matter areas. CSVD-MCI presented more severe damage in the anterior thalamic radiation, forceps major, forceps minor and right inferior longitudinal fasciculus compared with a-MCI group. No significant neuropsychological tests were found in the binary logistic regression model, yet the DTI markers showed a higher discriminative power than the neuropsychological tests. The Stroop test errors had moderate potential (AUC = 0.747; sensitivity = 76.0%; specificity = 63.6%; P = 0.001; 95% CI: 0.617–0.877), and the mean diffusivity value of forceps minor demonstrated the highest predictive power to discriminate each MCI subtype (AUC = 0.815; sensitivity = 88.0%; specificity = 72.7%; P < 0.001; 95% CI: 0.698–0.932).
Conclusion: The mean diffusivity of forceps minor may serve as an optimal indicator to differentiate between a-MCI and CSVD-MCI.
Introduction
Mild cognitive impairment (MCI) is a heterogeneous cognitive impairment syndrome and a precursor of various subtypes of dementia (Anderson, 2019), such as Alzheimer’s disease (AD), vascular dementia, dementia with Lewy bodies, frontotemporal dementia, and so on. MCI patients show mild memory loss or other cognitive impairment, with basically preserved activities of daily living (Petersen and Morris, 2005). Statistics showed that 40% of MCI patients eventually develop dementia (Mitchell and Shiri-Feshki, 2009). It is particularly challenging for clinicians to identify individual MCI patients which transit from the symptomatic pre-dementia phase to dementia onset. Even more challenging is the etiological diagnosis of MCI, which is essential for the early treatment of different MCI subtypes.
We focused on two types of MCI, one is amnestic mild cognitive impairment (a-MCI), another is MCI caused by cerebral small vessel disease (CSVD-MCI). Patients with a-MCI are at higher risks of evolving toward AD. Traditionally, a-MCI is considered a typical prodromal stage of AD (Petersen, 2004; Gómez-Soria et al., 2021). With the intensive study of a-MCI, researchers have proposed the concept of the clinical subtype of a-MCI, including a-MCI-single domain (a-MCI-s) and a-MCI-multiple domain (a-MCI-m) (Petersen, 2004). Actually, identifying a-MCI-m patients by neuropsychological testing is challenging given that the deficits of multiple cognition are the defining feature of CSVD-MCI and commonly characterize a-MCI-m. There is no uniform standard for the etiological diagnosis of a-MCI and CSVD-MCI.
The cognitive profile of AD and CSVD has been extensively studied over the last decade with an emerging consensus regarding the profile which typifies two disease. Study reported that the neuropsychological profile in CSVD generally showed more deficits in memory, executive function, attention, and visual-spatial skills, whereas AD showed poor performance in memory tests (Graham et al., 2004). However, studies on the differences between the two MCI subtypes have been lacking, and previous studies have reported controversial findings on damage of cognitive domains in the two MCI patients. A study directly compared the cognition of MCI patients due to AD with that of CSVD-MCI patients discovered that the former performed relatively poorly on memory tests but performed better on executive function (Zhou and Jia, 2009). Nevertheless, some studies have failed to identify cognitive differences among patients with different MCI subtypes and the findings are heterogeneous (Meyer et al., 2002; Loewenstein et al., 2006). It was not clear whether the degree of impairment in various cognitive domains of the two MCI subtypes were different, and what role neuropsychological tests play in etiological diagnosis of MCI. We still need to further explore the differences between a-MCI and CSVD-MCI patients by means of neuropsychological tests. The diagnosis of MCI and the assessment of the degree of cognitive decline still rely mainly on cognitive testing of which the ability to diagnose the etiology of MCI is insufficient. We also need more objective tools to distinguish between the MCI subtypes.
White matter (WM) involvement in CSVD and its early stage has been demonstrated by many neuroimaging studies (Wardlaw et al., 2013; Benveniste and Nedergaard, 2022). Many studies have confirmed that white matter lesions (WMLs) can also be observed in the early stage of AD, and even WM impairment precedes gray matter changes (Li et al., 2015; Sindi et al., 2015). Changes of brain pathology in AD are usually years or even decades before clinical symptoms, and changes of WM microstructure exist before cognitive impairment (Amieva et al., 2008; Sperling et al., 2013). These neuroimaging findings provide new research insights and approaches for differentiating the two MCI subtypes. However, it is unclear whether there are different WM microstructural abnormalities between a-MCI and CSVD-MCI. It is necessary to differential diagnose a-MCI and CSVD-MCI based on objective imaging characteristics not only for the in-depth understanding of degenerative neural changes of MCI but also for the different treatment and conversion judgment for the two subtypes.
Diffusion tensor imaging (DTI) is the most sensitive magnetic resonance technique for detecting the integrity of WM tracts in the early stages of dementia by describing the diffusion motion of water molecules in three-dimensional tissues (Preti et al., 2012). The most common DTI metrics include fractional anisotropy (FA) and mean diffusivity (MD). FA reflects the directionality of molecular diffusion, and MD reveals the average displacement of water molecules (Chua et al., 2008). Decreased FA and increased MD values reflect abnormalities in the WM microstructure. By applying DTI indices, we were able to assess the integrity of WM tracts more accurately and thoroughly in this study.
In widespread WM areas, abnormal DTI indices have been found in patients with AD as well as patients with MCI (Chua et al., 2008; Nishioka et al., 2015). Furthermore, researchers found that WM abnormalities were associated with various cognitive dysfunctions (Delbeuck et al., 2007; Fellgiebel et al., 2008; Mascalchi et al., 2019). However, knowledge of the difference between WM tracts microstructural alterations and cognitive performance in patients with a-MCI and CSVD-MCI remains limited. We hope to provide new insights into the anatomical correlates of the two etiologically different MCI subtypes using neuropsychological assessments and DTI analysis with ROI-based analyses, and then provide a reliable alternative to differentiate between them.
The present study aimed to:(a) explore the cognitive profiles and WM tracts abnormalities of each MCI subtype; (b) establish discriminative measures for these two MCI patients.
Materials and methods
Participants
A total of 78 participants matched by age and education level were enrolled in this study: 33 a-MCI patients (12 males and 21 females, with average age 72.33 ± 9.400 years), 25 CSVD-MCI patients (15 males and 10 females, with average age 74.44 ± 7.995 years), and 20 normal controls (NCs) (8 males and 12 females, with average age 69.85 ± 9.326 years). This study was approved by the Institutional Review Board of the First Affiliated Hospital of Soochow University and informed consents were obtained from all participants. All participants performed a series of neuropsychological assessments and 3.0-T magnetic resonance imaging (MRI) scan. The diagnostic criteria for MCI in this study was in accordance with the criteria of Petersen (2004): (a) complaint for cognitive decline; (b) smaller than –1.5 standard deviation from mean of local population in at least one cognitive domain with the standard neuropsychological assessments; (c) preserved basic activities of daily living (BADL) with slightly impaired instrumental activities of daily living (IADL) [as measured by Activities of Daily Living Scale (ADL)]; (d) failure to meet the Diagnostic and Statistical Manual of Mental Disorders, Fourth Edition criteria for dementia (DSM-IV); (e) 18 ≤ the Montreal Cognitive Assessment (MoCA) < 26 adjusted for age and education (Nasreddine et al., 2005).
Inclusion criteria for amnestic mild cognitive impairment group
(a) MCI was diagnosed using the above criteria; (b) subjective memory complaint and an objective memory impairment for age (Petersen, 2004); (c) medial temporal lobe atrophy (MTA) visual scale (Scheltens and van de Pol, 2012) score ≥ 2 and Fazekas scale (Fazekas et al., 1987) scores ≤ 2.
Inclusion criteria for cerebral small vessel disease-mild cognitive impairment group
(a) Meeting the above diagnostic criteria of MCI; (b) MTA scale score ≤ 1 and Fazekas scale score ≥ 3.
Inclusion criteria for normal control group
(a) Without subjective cognitive decline; (b) no objective cognitive impairment determined by neuropsychological assessments, namely, the Mini-mental State Examination (MMSE) ≥ 27 and MoCA ≥ 26; (c) no abnormalities in MRI; (d) normal ADL.
Exclusion criteria
Participants in the three groups were excluded if they exhibited any of the following conditions:(a) aged under 60 years old; (b) having a history of stroke, brain tumors, epilepsy, or other neurological diseases that may lead to cognitive impairment; (c) having severe depression, schizophrenia and other mental disorders; (d) having other serious physical diseases or contraindications for MRI; (e) having visual or auditory abnormalities that make neuropsychological assessments infeasible; (f) having insufficient Mandarin language abilities to complete the assessments; (g) having signs of large vascular diseases, such as cortical, and/or cortico-subcortical, or non-lacunar infarcts and watershed infarcts or hemorrhages; (h) image artifacts caused by head movement during MRI.
Neuropsychological assessments
The cognitive function of all the subjects was evaluated by an experienced neuropsychologist. MMSE and the Beijing version of MoCA (Lu et al., 2011) were used to assess global cognitive function. To evaluate memory, language, attention, executive, and visuospatial function, we carried out a battery of neuropsychological tests including the Rey auditory verbal learning test (RAVLT); verbal fluency task (VFT); digital span test (DST); Stroop color word test (SCWT); clock drawing test (CDT; four points). The ADL scale was used to assess activities of daily living while the Hamilton Depression Rating Scale (HAMD) and Hamilton Anxiety Rating Scale (HAMA) were used for mental status examination.
Magnetic resonance imaging acquisition
All subjects underwent a cranial MRI scan by an experienced imaging physician using the same 3.0-T scanner (Signa HDxt, GE Healthcare, Milwaukee, WI, USA) with an eight-channel head coil. Single spin echo diffusion-weighted echo planar imaging (EPI) sequence was used to obtain DTI data through the following parameters: repetition time (TR) 17000 ms, echo time (TE) 85.4 ms, flip angle 90°, field of view (FOV) 256 × 256 mm, matrix size 128 × 128, voxel size 2 mm × 2 mm × 2 mm, slice thickness 2 mm, 30 different diffusion gradient directions, b = 1000 s/mm2, 5 non-diffusion weighted b = 0 s/mm2. Meanwhile, T1-weighted images were collected with scanning parameters as follows: TR 6.50 ms, TE 2.80 ms, inversion time (TI) 900 ms, flip angle 8°, FOV 256 × 256 mm, number of slices 176, and slice thickness 1 mm. Axial T2-weighted and FLAIR sequences were obtained to assess white matter hyperintensity (WMH) and hippocampal atrophy.
Diffusion tensor imaging data processing
All DTI data were processed with PANDA (Cui et al., 2013) toolbox based on FMRIB Software Library v5.0 involving several steps (brain extraction, DTI images format conversion, realignment, eddy current and motion artifact correction, FA calculation, and diffusion tensor tractography). When tracking WM fibers, a FA value threshold was set at 0.2, and a turning angle threshold of the Fiber Assignment by Continuous Tracking (FACT) algorithm was set at 45° (Basser et al., 2000). The FA and MD standard templates in the Montreal Neurological Institute (MNI) space were non-linearly registered to the FA and MD images in the native space using FSL’s FNIRT command to obtain a conversion matrix, which was used to align the 20 ROIs of the JHU WM Tractography Atlas (Hua et al., 2008) template with the individual space of each subject. Each subject produced 20 WM tracts, and then the FA and MD values of each WM tract were calculated. The main 20 WM tracts were selected as follows: anterior thalamic radiation (ATR); corticospinal tract (CST); cingulum (cingulate gyrus; CgC); cingulum (hippocampus; CgH); forceps major (Fma); forceps minor (Fmi); inferior fronto-occipital fasciculus (IFO); inferior longitudinal fasciculus (ILF); superior longitudinal fasciculus (SLF); uncinate fasciculus (UF); superior longitudinal fasciculus-temporal part (tSLF). All of the tracts were evaluated in both hemispheres, except for the Fma and Fmi.
Visual assessments of white matter hyperintensity and hippocampal atrophy
One experienced imaging physician used two visual rating scales to identify WMH and hippocampal atrophy on baseline images in each subject without knowing the clinical information of the subjects. Fazekas scale was used for quantification of WMH, MTA visual scale was used to measure hippocampal atrophy, and higher hemispheric scores were used as the MTA scores of the subject.
Statistical analysis
Statistical analyses were performed using the Statistical Package for Social Science software (SPSS, Version 20.0). The categorical demographic variables were presented in terms of frequency and percentage (%), and group comparisons were made using Pearson χ2 two-tailed test, with continuity correction for n < 5. Continuous demographic variables were presented as mean ± standard deviation, or the median and the interquartile range. One-way analyses of variance (ANOVA) and non-parametric tests were used to compare the differences across groups. The neuropsychological scores and the DTI parameters of 20 WM tracts among three groups were examined by analysis of covariance (ANCOVA) with age, gender, and education as covariates. Bonferroni correction was conducted to adjust the false-positive rate (P < 0.05/20), and significant results further underwent pairwise comparison. In addition, the neuropsychological tests and the tracts with significant between-group differences were further included as covariates in the binary logistic regression model, using the forward method, while the MCI subtype was used as dependent variable, and age, gender, and years of education as controlled variables. Finally, the discriminant validity of independent variables were explored by receiver operating characteristic (ROC) analysis. The significant threshold was set at P < 0.05.
Results
Clinical data, neuropsychological scores, and visual scores
As shown in Table 1, there were no differences across groups in age, gender, education level, and the history of diabetes mellitus, hyperlipemia, smoking, and drinking. However, there were group differences in the history of hypertension. The proportion of participants with hypertension in the CSVD-MCI group was significantly higher than that in the NC and a-MCI groups (76.0, 35.0, and 33.3%). Compared with the NC group, the a-MCI group showed significantly lower scores in MMSE, MoCA, RAVLT-IR, RAVLT-DR, and VFT, indicating that a-MCI showed significant impairment in memory and language function. As shown in Table 1, there were significant differences in MMSE, MoCA, RAVLT-IR, RAVLT-DR, VFT, DST, SCWT A performance time, SCWT B performance time, SCWT C performance time, SCWT A errors, and SCWT C errors between the NC and CSVD-MCI groups, demonstrating that CSVD-MCI had deficits in multi-cognitive domains of memory, language, attention, and executive function. A statistically significant difference between the a-MCI and CSVD-MCI subjects was only present in the scores of SCWT C performance time and errors, reflecting that the a-MCI group had better performance in executive function than the CSVD-MCI group. HAMD, HAMA, ADL scores among the three groups were not statistically different.
Fractional anisotropy values
Compared with the controls, except for CST and tSLF, the FA values of other WM tracts showed significant decrease in both MCI groups. The results of post-analysis showed that the FA of CgC, CgH, Fma, Fmi, left IFO, ILF, SLF, and UF in the a-MCI and NC groups were significantly different. CSVD-MCI group showed significantly reduced FA values widespread in most of the WM regions except for the bilateral CST, right CgC, and bilateral tSLF. Moreover, compared with the CSVD-MCI group, the a-MCI group presented significantly higher FA values in the bilateral ATR, Fmi and right ILF, as shown in Tables 2, 3 and Figure 1.
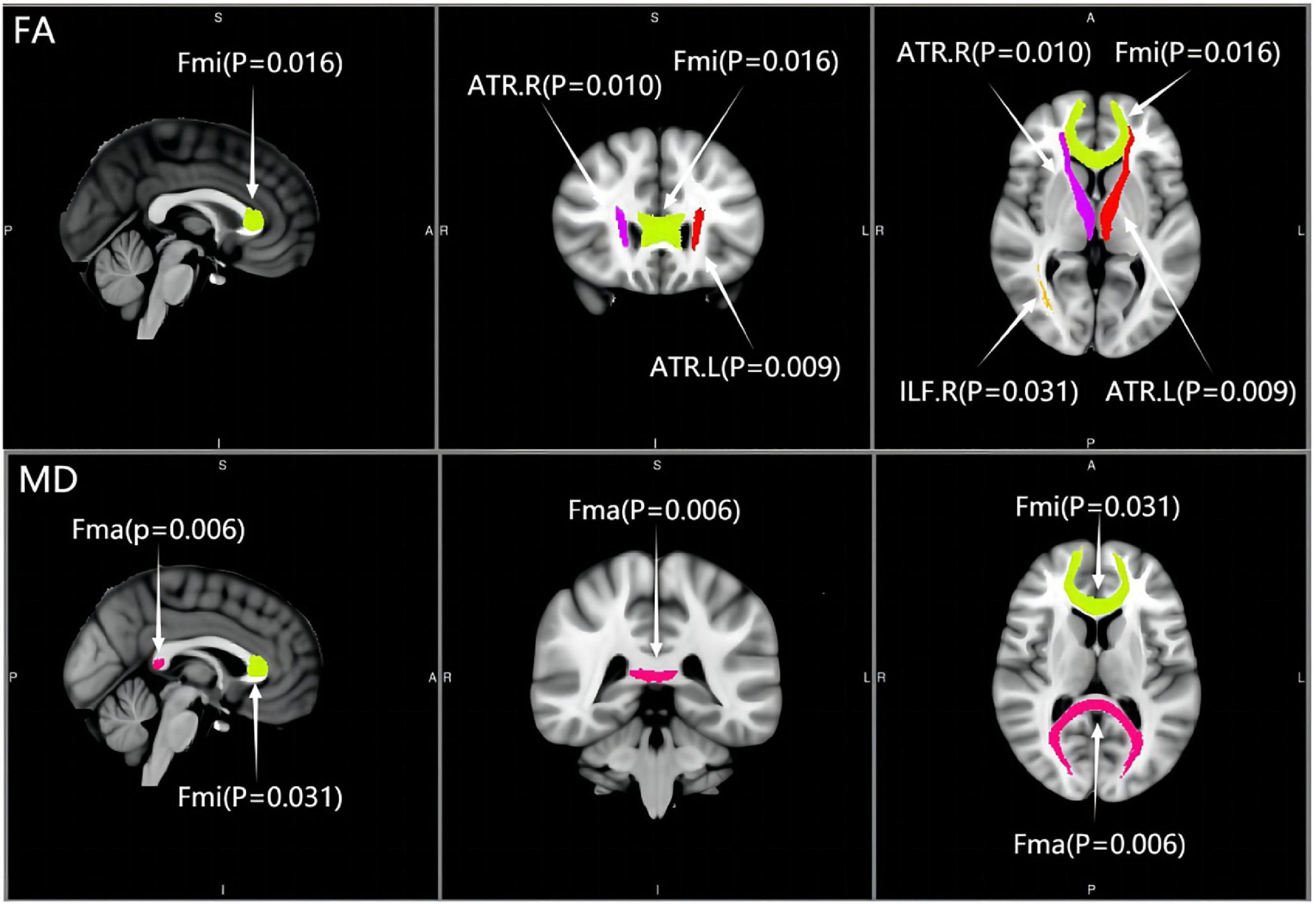
Figure 1. Distribution of significantly differential white matter tracts across the two mild cognitive impairment groups. ATR, anterior thalamic radiation; Fma, forceps major; Fmi, forceps minor; ILF, inferior longitudinal fasciculus; L, left; R, right; FA, fractional anisotropy; MD, mean diffusivity.
Mean diffusivity values
Cross-group differences of MD values are shown in Table 4. MD values were significantly different among the three groups in most of the WM tracts except for bilateral CST, IFO, and tSLF. There were significant deficits to the CgC, CgH, Fma, Fmi, left ILF, SLF, and UF in the a-MCI group compared with the NC group, while a subset of tracts including the ATR, CgC, CgH, Fma, Fmi, ILF, SLF, and UF in the CSVD-MCI group had significant deterioration from the NC group. Additionally, a statistically significant difference between the a-MCI and CSVD-MCI subjects was only present in the MD values of Fma and Fmi, as shown in Table 3 and Figure 1.
Discriminative power of major indicators between the mild cognitive impairment subgroups
In significant pairwise comparisons, only the SCWT C performance time, the SCWT C errors, the FA values of bilateral ATR, Fmi, right ILF, and the MD values of Fma and Fmi were significantly different between the two MCI groups. The above covariates and age, gender, and years of education were entered into a binary logistic regression model, using forward: LR approach. Finally only the FA value of right ILF (P < 0.05, OR = 0.737, 95% CI: 0.569–0.954) and the MD value of Fmi (P < 0.05, OR = 2.710, 95% CI: 1.210–6.071) were statistically significant (Table 5). The diagnostic accuracy of these major indices that showed significant differences between a-MCI and CSVD-MCI were explored by ROC analysis. As results shown in Table 6, the SCWT color performance time had no diagnostic significance while the SCWT color errors had moderate discriminating potential (AUC = 0.747; sensitivity = 76.0%; specificity = 63.6%; P = 0.001; 95% CI: 0.617–0.877). Six diffusion tensor parameters also had differential diagnostic significance, then the MD value of Fmi showed the best discriminating power (AUC = 0.815; sensitivity = 88.0%; specificity = 72.7%; P < 0.001; 95% CI: 0.698–0.932).
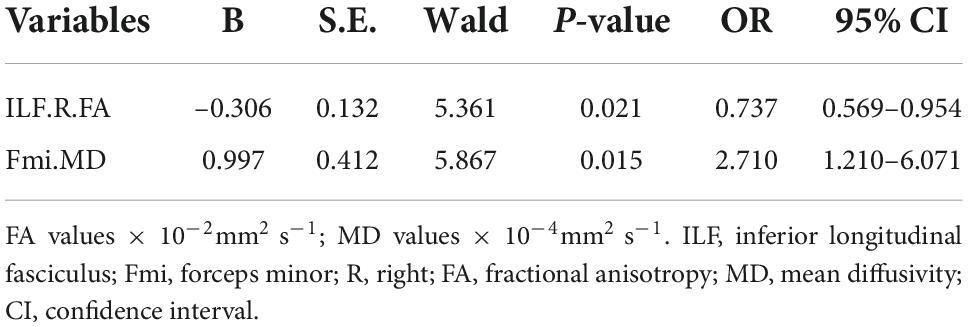
Table 5. Binary logistic regression for significant variables between the mild cognitive impairment (MCI) groups.
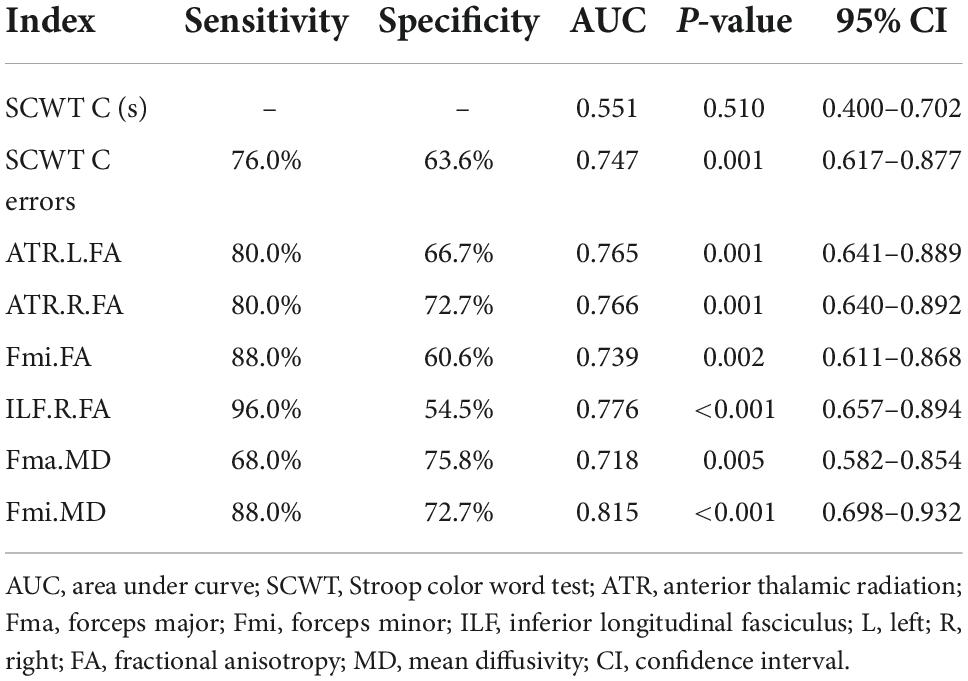
Table 6. Receiver operating characteristic (ROC) analysis of major indices between amnestic mild cognitive impairment (a-MCI) and cerebral small vessel disease-mild cognitive impairment (CSVD-MCI).
Discussion
Previous studies revealed episodic memory impairment in AD patients and impaired attention and executive function in CSVD patients (Rosas et al., 2021; Zanon Zotin et al., 2021), and suggested a non-specific neuropsychological profile for CSVD-MCI and a more specific cognitive pattern in MCI due to AD (Zhou and Jia, 2009). However, the results of the cognitive impairment profile in different etiologies of MCI patients were discordant since some authors found deficient episodic memory and speed/attention in MCI due to AD (Nordlund et al., 2008) while other study reported memory and executive function were mainly impaired in MCI patients of AD origin and multiple cognitive domains were impaired in CSVD-MCI group (Zhou and Jia, 2009). We administered comprehensive neuropsychological tests to find that compared with controls, a-MCI patients had worse performance only in the cognitive domains of memory and language functions. The current study revealed extensive cognitive deficits in four domains including memory, language, attention, and executive functions among patients with CSVD-MCI. Moreover, the two patient groups differed mainly on measures of the Stroop test, indicating that working memory and executive function were more dysfunctional in CSVD-MCI patients. Executive dysfunction is considered to be “the core characteristic of vascular cognitive impairment” (Roman et al., 2004). Studies have shown that executive function is the most sensitive cognitive domain in deterioration of the microstructural integrity of WM of vascular origin (O’Sullivan et al., 2004; Nitkunan et al., 2008).
Nordlund et al. (2011) found that the results of other neuropsychological tests in MCI resulting from AD and vascular dementia were basically consistent except for extremely poor performance of MCI patients of AD origin on memory tests. Other researchers reported differences in processing speed, memory, and visuospatial skills between the two different MCI patients (Luis et al., 2004; Zhou and Jia, 2009). Nevertheless, there was no significant difference in memory function between the two groups in the current study. These findings were inconsistent with the findings of our study and may be due to differences in subjects. Vascular MCI included non-cavitary cortical or subcortical infarction, infarction in key areas such as thalamus or basal ganglia, or large vessel hemorrhage and signs of infarction. However, our CSVD-MCI group in this study explicitly excluded patients with these conditions.
Furthermore, we found the deterioration of CgC, CgH, Fma, Fmi, left IFO, ILF, SLF, and UF in a-MCI subjects. The degeneration of WM tracts in a-MCI patients in this study were extensive and spread throughout the frontal, temporal, parietal, and other regions. Previous studies have shown that mainly damaged WM tracts including ATR, Fma, Fmi, cingulate tract, para-hippocampal cingulate tract, and IFO in early AD connected the earliest affected gray matter structures (i.e., hippocampus, cingulate gyrus, medial prefrontal cortex, and posterior cingulate cortex) in AD patients (Villain et al., 2010; Luo et al., 2019), basically consistent with the conclusion of our study.
Additionally, we observed the ATR, CgC, CgH, Fma, Fmi, IFO, ILF, SLF, and UF are impaired in patients with CSVD-MCI. The WM microstructure damage in the CSVD-MCI group was more extensive and severe than the a-MCI group, which may be related to the CSVD-MCI subjects included in this study having high visual scores of WMLs. Previous studies have found that the damaged WM in CSVD-MCI is mainly located in the prefrontal pathway of the thalamus and caudate lobe (e.g., ATR, Fmi, IFO, ILF, SLF) which are significantly associated with the executive and attention functions (Mamah et al., 2010; Biesbroek et al., 2017). As part of the anterior striatal thalamic circuit, ATR have an important effect on executive function (Mamah et al., 2010). In our study, significant impairment to ATR was not observed in the a-MCI group, meanwhile executive function was relatively preserved.
This study found that damaged tracts in a-MCI were mostly involved in memory function, while damaged tracts in CSVD-MCI were mostly associated with executive function. By comparing the two MCI groups directly, there were significant differences in the DTI parameters of bilateral ATR, Fma, Fmi, and right ILF. The degeneration of the above tracts in the CSVD-MCI group were more severe than the a-MCI group, which may be explained by more participation of these WM tracts in executive function. The Fma mainly helps the communication and connection between the hemispheres, and the integrity of WM microstructure is related to visuospatial function and working memory (Krogsrud et al., 2018). Fmi is connected to the bilateral prefrontal cortex, which is of the substantive circuits connecting the bilateral regions of the default mode network playing an important role in executive function (Fox et al., 2005; van den Heuvel et al., 2009). ILF is the main connecting fiber tract of the frontal, parietal, temporal, and occipital cortex involved in executive function and processing speed (Biesbroek et al., 2017). Binary logistic regression and ROC analysis in this study further confirmed that the altered right ILF and Fmi are important WM tracts for predicting the two MCI subtypes.
This study found significant differences in DTI parameters of the corpus callosum (including the Fma and Fmi) between each MCI subtype. ROC analyses showed that the MD value of Fmi was the best index to distinguish between the two MCI groups, showing the importance of corpus callosum. Previous researchers have suggested that corpus callosum is the most important structure to discriminate different types of dementia (Zarei et al., 2009). The WM microstructure of corpus callosum, especially the Fmi, showed significant difference in vascular dementia and AD patients (Zarei et al., 2009). Palesi et al. (2018) found that the FA value of corpus callosum decreased and all DTI parameters of corpus callosum changed in vascular dementia patients, suggesting that the damage of corpus callosum was much more severe than AD patients.
Importantly, only two neuropsychological tests and six diffusion parameters varied significantly between the two MCI groups. The Stroop test did not differ significantly in the binary logistic regression model, and based on the above results of ROC analysis, the diagnostic efficacy of the neuropsychological tests were not as high as that for the diffusion parameters, suggesting that neuroimaging markers might be more reliable than neuropsychological tests in discriminating a-MCI from CSVD-MCI. Application of neuroimaging, especially DTI, is more conducive to identifying the etiology of MCI than neuropsychological tests before more sensitive diagnostic tools are applied to the clinical and practice. Further work is needed to verify the diagnostic efficacy of DTI indices in larger samples in the future.
In conclusion, comparing cognitive deficits and the deterioration of WM tracts in the two MCI groups highlights the significance of etiological diagnosis of MCI. The MD value of Fmi could serve as an optimal indicator to differentiate between a-MCI and CSVD-MCI. Quantitative metrics of DTI should been recommended as part of the diagnostic pipeline for the etiological diagnosis of MCI.
Limitations
There are still some limitations in this study. First, the study was of a cross-sectional design without follow-up; the diagnosis of MCI lacked pathological evidences and whether the MCI patients would progress to AD or CSVD in the future was uncertain. Second, our study was a single-center study with a relatively small sample size, so there might be a certain degree of selection bias. In order to improve the reliability of the research results, a multi-center study with a larger sample size is needed in the future. Finally, due to the machine limitation, the diffusion gradient direction of MRI can only be set at 30.
Data availability statement
The original contributions presented in this study are included in the article/supplementary material, further inquiries can be directed to the corresponding authors.
Ethics statement
The studies involving human participants were reviewed and approved by the Institutional Review Board of the First Affiliated Hospital of Soochow University. The patients/participants provided their written informed consent to participate in this study.
Author contributions
YW and HD designed this study. YZ finished the statistical analysis and submitted the manuscript. YZ, MF, LD, HS, and ZZ collected the participants’ data and wrote the manuscript. YZ, LL, and YQ revised the manuscript. All authors gave final agreement to be accountable for all aspects of the work in ensuring that questions related to the accuracy or integrity of any part of the work were appropriately investigated and resolved, contributed to the article, and approved the submitted version.
Funding
This work was partially supported by the Jiangsu Province Cadre Health Project (Grant No. BJ19008), the National Natural Science Foundation of China (Grant No. 81971573), and the Humanity and Social Science Youth Foundation of Ministry of Education of China (Project No. 20YJCZH088).
Acknowledgments
We are grateful for the support to the staff of the First Affiliated Hospital of Soochow University Memory Clinic.
Conflict of interest
The authors declare that the research was conducted in the absence of any commercial or financial relationships that could be construed as a potential conflict of interest.
Publisher’s note
All claims expressed in this article are solely those of the authors and do not necessarily represent those of their affiliated organizations, or those of the publisher, the editors and the reviewers. Any product that may be evaluated in this article, or claim that may be made by its manufacturer, is not guaranteed or endorsed by the publisher.
References
Amieva, H., Le Goff, M., Millet, X., Orgogozo, J. M., Peres, K., Barberger-Gateau, P., et al. (2008). Prodromal Alzheimer’s disease: Successive emergence of the clinical symptoms. Ann. Neurol. 64, 492–498. doi: 10.1002/ana.21509
Anderson, N. D. (2019). State of the science on mild cognitive impairment (MCI). CNS Spectr. 24, 78–87. doi: 10.1017/S1092852918001347
Basser, P. J., Pajevic, S., Pierpaoli, C., Duda, J., and Aldroubi, A. (2000). In vivo fiber tractography using DT-MRI data. Magn. Reson. Med. 44, 625–632. doi: 10.1002/1522-2594(200010)44:4<625::AID-MRM17>3.0.CO;2-O
Benveniste, H., and Nedergaard, M. (2022). Cerebral small vessel disease: A glymphopathy? Curr. Opin. Neurobiol. 72, 15–21. doi: 10.1016/j.conb.2021.07.006
Biesbroek, J. M., Weaver, N. A., and Biessels, G. J. (2017). Lesion location and cognitive impact of cerebral small vessel disease. Clin. Sci. 131, 715–728. doi: 10.1042/CS20160452
Chua, T. C., Wen, W., Slavin, M. J., and Sachdev, P. S. (2008). Diffusion tensor imaging in mild cognitive impairment and Alzheimer’s disease: A review. Curr. Opin. Neurol. 21, 83–92. doi: 10.1097/WCO.0b013e3282f4594b
Cui, Z., Zhong, S., Xu, P., He, Y., and Gong, G. (2013). PANDA: A pipeline toolbox for analyzing brain diffusion images. Front. Hum. Neurosci. 7:42. doi: 10.3389/fnhum.2013.00042
Delbeuck, X., Collette, F., and Van der Linden, M. (2007). Is Alzheimer’s disease a disconnection syndrome? Evidence from a crossmodal audio-visual illusory experiment. Neuropsychologia 45, 3315–3323. doi: 10.1016/j.neuropsychologia.2007.05.001
Fazekas, F., Chawluk, J. B., Alavi, A., Hurtig, H. I., and Zimmerman, R. A. (1987). Mr signal abnormalities At 1.5-T in Alzheimer dementia and normal aging. Am. J. Roentgenol. 149, 351–356. doi: 10.2214/ajr.149.2.351
Fellgiebel, A., Schermuly, I., Gerhard, A., Keller, I., Albrecht, J., Weibrich, C., et al. (2008). Functional relevant loss of long association fibre tracts integrity in early Alzheimer’s disease. Neuropsychologia 46, 1698–1706. doi: 10.1016/j.neuropsychologia.2007.12.010
Fox, M. D., Snyder, A. Z., Vincent, J. L., Corbetta, M., Van Essen, D. C., and Raichle, M. E. (2005). The human brain is intrinsically organized into dynamic, anticorrelated functional networks. Proc. Natl. Acad. Sci. U.S.A. 102, 9673–9678.
Gómez-Soria, I., Peralta-Marrupe, P., Calatayud-Sanz, E., and Latorre, E. (2021). Efficacy of cognitive intervention programs in amnesic mild cognitive impairment: A systematic review. Arch. Gerontol. Geriatr. 94:104332. doi: 10.1016/j.archger.2020.104332
Graham, N. L., Emery, T., and Hodges, J. R. (2004). Distinctive cognitive profiles in Alzheimer’s disease and subcortical vascular dementia. J. Neurol. Neurosurg. Psychiatry 75, 61–71.
Hua, K., Zhang, J., Wakana, S., Jiang, H., Li, X., Reich, D. S., et al. (2008). Tract probability maps in stereotaxic spaces: Analyses of white matter anatomy and tract-specific quantification. Neuroimage 39, 336–347. doi: 10.1016/j.neuroimage.2007.07.053
Krogsrud, S. K., Fjell, A. M., Tamnes, C. K., Grydeland, H., Due-Tonnessen, P., Bjornerud, A., et al. (2018). Development of white matter microstructure in relation to verbal and visuospatial working memory–A longitudinal study. PLoS One 13:22. doi: 10.1371/journal.pone.0195540
Li, X., Westman, E., Stahlbom, A. K., Thordardottir, S., Almkvist, O., Blennow, K., et al. (2015). White matter changes in familial Alzheimer’s disease. J. Intern. Med. 278, 211–218.
Loewenstein, D. A., Acevedo, A., Agron, J., Issacson, R., Strauman, S., Crocco, E., et al. (2006). Cognitive profiles in Alzheimer’s disease and in mild cognitive impairment of different etiologies. Dement. Geriatr. Cogn. Dis. 21, 309–315.
Lu, J., Li, D., Li, F., Zhou, A. H., Wang, F., Zuo, X. M., et al. (2011). Montreal cognitive assessment in detecting cognitive impairment in Chinese elderly individuals: A population-based study. J. Geriatr. Psychiatry Neurol. 24, 184–190. doi: 10.1177/0891988711422528
Luis, C. A., Barker, W., Loewenstein, D. A., Crum, T. A., Rogaeva, E., Kawarai, T., et al. (2004). Conversion to dementia among two groups with cognitive impairment – A preliminary report. Dement. Geriatr. Cogn. Disord. 18, 307–313. doi: 10.1159/000080124
Luo, C., Li, M., Qin, R., Chen, H., Yang, D., Huang, L., et al. (2019). White matter microstructural damage as an early sign of subjective cognitive decline. Front. Aging Neurosci. 11:378. doi: 10.3389/fnagi.2019.00378
Mamah, D., Conturo, T. E., Harms, M. P., Akbudak, E., Wang, L., McMichael, A. R., et al. (2010). Anterior thalamic radiation integrity in schizophrenia: A diffusion-tensor imaging study. Psychiatry Res. Neuroimaging 183, 144–150.
Mascalchi, M., Salvadori, E., Toschi, N., Giannelli, M., Orsolini, S., Ciulli, S., et al. (2019). DTI-derived indexes of brain WM correlate with cognitive performance in vascular MCI and small-vessel disease. A TBSS study. Brain Imaging Behav. 13, 594–602. doi: 10.1007/s11682-018-9873-5
Meyer, J. S., Xu, G. L., Thornby, J., Chowdhury, M. H., and Quach, M. (2002). Is mild cognitive impairment prodromal for vascular dementia like Alzheimer’s disease? Stroke 33, 1981–1985.
Mitchell, A. J., and Shiri-Feshki, M. (2009). Rate of progression of mild cognitive impairment to dementia – meta-analysis of 41 robust inception cohort studies. Acta Psychiatr. Scand. 119, 252–265. doi: 10.1111/j.1600-0447.2008.01326.x
Nasreddine, Z. S., Phillips, N. A., Bedirian, V., Charbonneau, S., Whitehead, V., Collin, I., et al. (2005). The montreal cognitive assessment, MoCA: A brief screening tool for mild cognitive impairment. J. Am. Geriatr. Soc. 53, 695–699. doi: 10.1111/j.1532-5415.2005.53221.x
Nishioka, C., Poh, C., and Sun, S.-W. (2015). Alzheimer’s dis neuroimaging I. Diffusion tensor imaging reveals visual pathway damage in patients with mild cognitive impairment and Alzheimer’s disease. J. Alzheimers Dis. 45, 97–107. doi: 10.3233/JAD-141239
Nitkunan, A., Barrick, T. R., Charlton, R. A., Clark, C. A., and Markus, H. S. (2008). Multimodal MRI in cerebral small vessel disease – its relationship with cognition and sensitivity to change over time. Stroke 39, 1999–2005.
Nordlund, A., Gothlin, M., and Wallin, A. (2011). Vascular disease, Alzheimer’s disease biomarkers and cognition in mild cognitive impairment: Additive or synergetic effects? Dement. Geriatr. Cogn. Disord. 32, 250–256.
Nordlund, A., Rolstad, S., Klang, O., Lind, K., Pedersen, M., Blennow, K., et al. (2008). Episodic memory and speed/attention deficits are associated with Alzheimer-typical CSF abnormalities in MCI. J. Int. Neuropsychol. Soc. 14, 582–590. doi: 10.1017/S135561770808079X
O’Sullivan, M., Morris, R. G., Huckstep, B., Jones, D. K., Williams, S. C. R., and Markus, H. S. (2004). Diffusion tensor MRI correlates with executive dysfunction in patients with ischaemic leukoaraiosis. J. Neurol. Neurosurg. Psychiatry 75, 441–447.
Palesi, F., De Rinaldis, A., Vitali, P., Castellazzi, G., Casiraghi, L., Germani, G., et al. (2018). Specific patterns of white matter alterations help distinguishing Alzheimer’s and vascular dementia. Front. Neurosci. 12:274. doi: 10.3389/fnins.2018.00274
Petersen, R. C. (2004). Mild cognitive impairment as a diagnostic entity. J. Intern. Med. 256, 183–194.
Petersen, R. C., and Morris, J. C. (2005). Mild cognitive impairment as a clinical entity and treatment target. Arch. Neurol. 62, 1160–1163.
Preti, M. G., Baglio, F., Lagana, M. M., Griffanti, L., Nemni, R., Clerici, M., et al. (2012). Assessing corpus callosum changes in Alzheimer’s disease: Comparison between tract-based spatial statistics and atlas-based tractography. PLoS One 7:e35856. doi: 10.1371/journal.pone.0035856
Roman, G. C., Sachdev, P., Royall, D. R., Bullock, R. A., Orgogozo, J. M., Lopez-Pousa, S., et al. (2004). Vascular cognitive disorder: A new diagnostic category updating vascular cognitive impairment and vascular dementia. J. Neurol. Sci. 226, 81–87. doi: 10.1016/j.jns.2004.09.016
Rosas, A. G., Stogmann, E., and Lehrner, J. (2021). Individual cognitive changes in subjective cognitive decline, mild cognitive impairment and Alzheimer’s disease using the reliable change index methodology. Wien. Klin. Wochenschr. 133, 1064–1069.
Scheltens, P., and van de Pol, L. (2012). Atrophy of medial temporal lobes on MRI in “probable”. J. Neurol. Neurosurg. Psychiatry 83, 1038–1040.
Sindi, S., Mangialasche, F., and Kivipelto, M. (2015). Advances in the prevention of Alzheimer’s Disease. F1000Prime Rep. 7:50.
Sperling, R. A., Karlawish, J., and Johnson, K. A. (2013). Preclinical Alzheimer disease-the challenges ahead. Nat. Rev. Neurol. 9, 54–58. doi: 10.1038/nrneurol.2012.241
van den Heuvel, M. P., Mandl, R. C. W., Kahn, R. S., and Pol, H. E. H. (2009). Functionally linked resting-state networks reflect the underlying structural connectivity architecture of the human brain. Hum. Brain Mapp. 30, 3127–3141.
Villain, N., Fouquet, M., Baron, J.-C., Mezenge, F., Landeau, B., de La Sayette, V., et al. (2010). Sequential relationships between grey matter and white matter atrophy and brain metabolic abnormalities in early Alzheimer’s disease. Brain 133, 3301–3314. doi: 10.1093/brain/awq203
Wardlaw, J. M., Smith, E. E., Biessels, G. J., Cordonnier, C., Fazekas, F., Frayne, R., et al. (2013). Neuroimaging standards for research into small vessel disease and its contribution to ageing and neurodegeneration. Lancet Neurol. 12, 822–838.
Zanon Zotin, M. C., Sveikata, L., Viswanathan, A., and Yilmaz, P. (2021). Cerebral small vessel disease and vascular cognitive impairment: From diagnosis to management. Curr. Opin. Neurol. 34, 246–257.
Zarei, M., Damoiseaux, J. S., Morgese, C., Beckmann, C. F., Smith, S. M., Matthews, P. M., et al. (2009). Regional white matter integrity differentiates between vascular dementia and Alzheimer disease. Stroke 40, 773–779.
Keywords: cerebral small vessel disease, diffusion tensor imaging, neuropsychological tests, ROI-based analyses, amnestic mild cognitive impairment
Citation: Zhang Y, Lin L, Feng M, Dong L, Qin Y, Su H, Zhou Z, Dai H and Wang Y (2022) The mean diffusivity of forceps minor is useful to distinguish amnestic mild cognitive impairment from mild cognitive impairment caused by cerebral small vessel disease. Front. Hum. Neurosci. 16:1010076. doi: 10.3389/fnhum.2022.1010076
Received: 02 August 2022; Accepted: 25 October 2022;
Published: 09 November 2022.
Edited by:
Betty M. Tijms, VU Medical Center, NetherlandsReviewed by:
Lei Gao, Zhongnan Hospital of Wuhan University, ChinaGunther Helms, Lund University, Sweden
Elijah Mak, University of Cambridge, United Kingdom
Copyright © 2022 Zhang, Lin, Feng, Dong, Qin, Su, Zhou, Dai and Wang. This is an open-access article distributed under the terms of the Creative Commons Attribution License (CC BY). The use, distribution or reproduction in other forums is permitted, provided the original author(s) and the copyright owner(s) are credited and that the original publication in this journal is cited, in accordance with accepted academic practice. No use, distribution or reproduction is permitted which does not comply with these terms.
*Correspondence: Yueju Wang, d2FuZ3l1ZWp1QHN1ZGEuZWR1LmNu; Hui Dai, aHVpemkxOTgyMDhAMTI2LmNvbQ==
†These authors have contributed equally to this work and share first authorship