- 1School of Health and Rehabilitation Sciences, The University of Queensland, St Lucia, QLD, Australia
- 2Queensland Brain Institute, The University of Queensland, St Lucia, QLD, Australia
- 3School of Human Movement and Nutrition Sciences, The University of Queensland, St Lucia, QLD, Australia
Converging evidence suggests that transcranial alternating current stimulation (tACS) may entrain endogenous neural oscillations to match the frequency and phase of the exogenously applied current and this entrainment may outlast the stimulation (although only for a few oscillatory cycles following the cessation of stimulation). However, observing entrainment in the electroencephalograph (EEG) during stimulation is extremely difficult due to the presence of complex tACS artifacts. The present study assessed entrainment to slow oscillatory (SO) tACS by measuring motor cortical excitability across different oscillatory phases during (i.e., online) and outlasting (i.e., offline) stimulation. 30 healthy participants received 60 trials of intermittent SO tACS (0.75 Hz; 16 s on/off interleaved) at an intensity of 2 mA peak-to-peak. Motor cortical excitability was assessed using transcranial magnetic stimulation (TMS) of the hand region of the primary motor cortex (M1HAND) to induce motor evoked potentials (MEPs) in the contralateral thumb. MEPs were acquired at four time-points within each trial – early online, late online, early offline, and late offline – as well as at the start and end of the overall stimulation period (to probe longer-lasting aftereffects of tACS). A significant increase in MEP amplitude was observed from pre- to post-tACS (paired-sample t-test; t29 = 2.64, P = 0.013, d = 0.48) and from the first to the last tACS block (t29 = −2.93, P = 0.02, d = 0.54). However, no phase-dependent modulation of excitability was observed. Therefore, although SO tACS had a facilitatory effect on motor cortical excitability that outlasted stimulation, there was no evidence supporting entrainment of endogenous oscillations as the underlying mechanism.
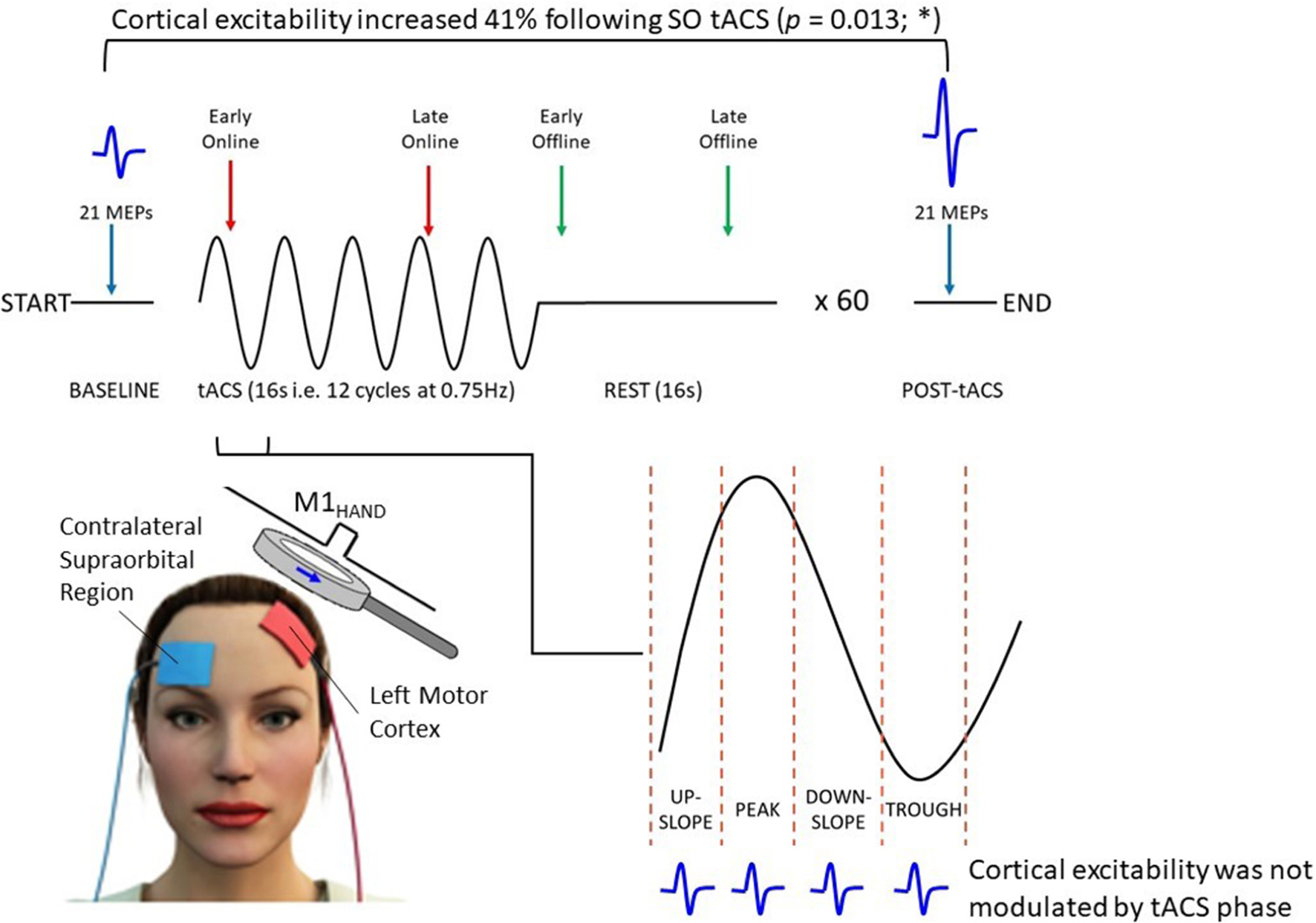
Graphical Abstract. Thirty healthy participants received 60 trials of intermittent SO (0.75 Hz) tACS (1 trial = 16 s on + 16 s off) at an intensity of 2 mA. Motor cortical excitability was assessed using TMS-induced MEPs (blue waveforms) acquired across different oscillatory phases during (i.e., online; red arrows) and outlasting (i.e., offline; green arrows) tACS, as well as at the start and end of the stimulation session (blue arrows). Mean MEP amplitude increased by ∼41% from pre- to post-tACS (P = 0.013); however, MEP amplitudes were not modulated with respect to the tACS phase.
Introduction
Neural oscillations (i.e., cyclic fluctuations in neuronal excitability) are proposed to provide phase-dependent temporal regulation of neural information processing (Buzsáki, 2006). In order to explore the functional relationships between neural oscillations and behavior in normal brain function, rhythmic subtypes of transcranial electrical stimulation (tES) have been used to attempt to modulate endogenous neural oscillatory activity experimentally. tES has already been shown to influence various aspects of behavior and cognition by modulating the power of specific neural oscillations known to be associated with such tasks (for reviews see, Antal and Paulus, 2013; Herrmann et al., 2016; Vosskuhl et al., 2018; Bland and Sale, 2019). However, despite promising findings of behavioral effects induced by transcranial alternating current stimulation (tACS), the neurophysiological mechanisms behind these effects are still not well understood.
Converging evidence from animal (e.g., Krause et al., 2019; see also review by Reato et al., 2013), computational (Reato et al., 2010; Ali et al., 2013; Huang et al., 2021), and human studies (Helfrich et al., 2014a,b; Witkowski et al., 2016) suggests that during stimulation, tES may be able to entrain (i.e., synchronize) endogenous neural oscillations with respect to the frequency and phase of the exogenously applied current. However, unlike the well-documented immediate (online) effects of tES, there is less agreement regarding the magnitude and duration of post-stimulus (offline) effects (for review see, Veniero et al., 2015). These offline effects cannot be fully explained by a direct continuation of online entrainment (referred to as entrainment “echoes”), since these “echoes” only persist for a few oscillatory cycles following cessation of stimulation (Marshall et al., 2006; Thut et al., 2011; Hanslmayr et al., 2014; van Bree et al., 2021). Therefore, longer-lasting offline effects (referred to as aftereffects) lasting up to 70 min are likely to reflect mechanisms other than entrainment per se (e.g., synaptic plasticity) (Neuling et al., 2013; Veniero et al., 2015; Vossen et al., 2015; Kasten et al., 2016).
The effects of tES on endogenous oscillatory activity have traditionally been quantified in humans using electroencephalography (EEG; Marshall et al., 2006; Kirov et al., 2009; Jones et al., 2018; Ketz et al., 2018). However, observing entrainment in the EEG concurrently with tES is extremely difficult due to the presence of complex artifacts (Noury et al., 2016; Noury and Siegel, 2017; Kasten and Herrmann, 2019) and we have therefore used an alternative method to assess entrainment of endogenous neural oscillations by tES. Single-pulse transcranial magnetic stimulation (TMS) is a form of non-invasive brain stimulation that can be used to indirectly probe the excitability of neocortical networks with high spatiotemporal precision of the order of millimeters and milliseconds (Hallett, 2007). When applied to the hand area of the primary motor cortex (M1HAND), each TMS pulse induces a motor evoked potential (MEP) in the contralateral target muscle, the amplitude of which can then be measured using electromyography (EMG; Barker et al., 1985). These MEP amplitudes provide an indirect measure of motor cortical excitability with good topographical specificity (Di Lazzaro et al., 2004; Hallett, 2007; Ilmoniemi and Kicić, 2010; Bergmann et al., 2012). By applying TMS pulses within a particular oscillatory phase of tES (referred to as phase-dependent stimulation), TMS can be used to assess entrainment of endogenous neural oscillations by tES (i.e., whether motor cortical excitability is modulated with respect to the phase of tES; Raco et al., 2016; Zrenner et al., 2018; Schaworonkow et al., 2019). Importantly, the artifact issues of EEG are not present with TMS–EMG measures, thus allowing for an unambiguous investigation of the phasic effects of tES on motor cortical excitability.
Because we wanted to probe the phase-specific effects of tES, we chose to apply tES at a low frequency to allow MEP sampling across the different phases of stimulation. In this manner, the phase-cycle of low-frequency tACS could be conceptualized as representing alternating periods of classic “anodal” and “cathodal” transcranial direct current stimulation (tDCS), on which much earlier work has focused (Antal and Paulus, 2013; Reato et al., 2013; Liu et al., 2018; Bland and Sale, 2019). Therefore, in the present investigation, we chose to examine the online and offline effects of slow oscillatory (SO; 0.75 Hz) tACS on motor cortical excitability using TMS.
Slow oscillations are typically prevalent during slow-wave sleep and play an important role in sleep-dependent consolidation of motor learning (Marshall and Binder, 2013). Despite the lack of endogenous SO activity during wakefulness, anodal SO tDCS during wakefulness has been shown to increase endogenous SO EEG power with relatively short-lasting offline effects (<1 min) (Kirov et al., 2009), though the exact duration of these offline effects was not thoroughly assessed. However, it is important to note that these increases in SO power were more restricted topographically to the prefrontal cortex (the predominant source of endogenous slow oscillations during sleep) and were less pronounced than those observed following SO tDCS applied during sleep (Marshall et al., 2006). Furthermore, due to the previously mentioned complexity of tES artifacts in the EEG, the authors could not determine whether these localized increases in EEG SO power were in fact due to the entrainment of slow oscillations by SO tDCS. Therefore, it remains unclear whether slow oscillations can be reliably entrained in the awake brain at intensities typical of tES (i.e., 1–2 mA).
Anodal SO tDCS during wakefulness has also been shown to induce lasting increases in motor cortical excitability that persist beyond stimulation (Bergmann et al., 2009; Groppa et al., 2010). However, due to the anodal component (i.e., positive current offset) of this stimulation—which in itself can cause an increase in cortical excitability (Nitsche and Paulus, 2000; Nitsche et al., 2007; Bergmann et al., 2009)—it cannot be concluded that these effects are a direct result of the influence of the applied slow oscillations. tACS has a significant technical advantage over tDCS in this regard, since it has no DC offset (i.e., an average current of 0 mA). Despite this, the effects of SO tACS on motor cortical excitability have not been thoroughly examined in previous literature. Antal et al. (2008) found no significant changes in motor cortical excitability following SO (1 Hz) tACS; however, their stimulation protocol was suboptimal for inducing changes in endogenous oscillatory activity due to the low stimulation intensity (0.4 mA; Reato et al., 2010; Huang et al., 2017) and constant rather than intermittent application of tACS (Jones et al., 2018; Ketz et al., 2018).
The aims of this study were: (1) to investigate the online effects of SO tACS applied intermittently at high intensity on motor cortical excitability; (2) to determine if tACS-induced changes in motor cortical excitability persist beyond each trial of stimulation (i.e., entrainment echoes) as well as beyond the total stimulation period (i.e., offline aftereffects).
It was hypothesized that SO tACS will induce SO-like sinusoidal changes in motor cortical excitability that correspond with the tACS phase, with high MEP amplitudes at oscillatory peaks and low amplitudes at oscillatory troughs, supporting online entrainment. Secondly, that sinusoidal changes in motor cortical excitability will persist for a few oscillatory cycles immediately following each trial of stimulation, thus demonstrating entrainment echoes. Thirdly, that motor cortical excitability will increase over the total duration of stimulation (although this relationship may not necessarily be linear), and this increase will be sustained beyond the total stimulation period (i.e., offline aftereffects).
Materials and Methods
Subjects
Forty-one neurologically healthy, right-handed participants (17 male, aged 24 ± 4 years) were recruited by advertisement, although 11 participants were excluded from the final analysis (see “MEP screening” below) leaving a sample size of 30 participants. All participants completed a safety screening questionnaire (Keel et al., 2001) and provided a written statement of informed consent prior to commencing the experiment. The exclusion criteria for participants included: personal or family history of epilepsy/seizures, medication that could affect seizure threshold, history of brain injury/condition (e.g., stroke, concussion, etc.), implanted devices or metal in the head, frequent or severe headaches, or current pregnancy. Approval was granted by The University of Queensland Human Research Ethics Committee.
Quantification of Motor Cortical Excitability Using TMS
Motor cortical excitability was assessed by measuring TMS-induced MEP amplitudes that were recorded from the target muscle using surface EMG. The target site for the TMS was the left M1HAND region, specifically the region associated with the abductor pollicis brevis (APB), a large thumb muscle.
Experimental Setup
EMG
Participants were seated comfortably in a chair and their right forearm placed on a foam mat with their forearm supinated. EMG activity of the APB muscle was recorded using disposable surface electrodes (H124SG 30 mm × 24 mm). The active electrode was placed over the APB muscle belly, the reference electrode was placed over the first metacarpophalangeal joint, and the ground electrode was placed on the anterior surface of the wrist.
TMS
Transcranial magnetic stimulation pulses were applied to the left M1HAND region using a Magstim Double 70 mm Remote Control Coil charged by a Magstim 2002 stimulator (Magstim, United Kingdom). The individual location of the left M1HAND region as well as the TMS intensity were determined for each participant using manual TMS “hot-spotting” (Rossini et al., 1994). This involves systematically adjusting the position of the TMS coil on the participant’s head whilst also adjusting the stimulation intensity until MEPs are consistently induced (i.e., in at least five out of ten successive trials) with amplitudes within a desired range (in our case, 0.5–1.5 mV; see Cuypers et al., 2014; Thies et al., 2018; Ogata et al., 2019). The location of the left M1HAND region was then marked on the participant’s scalp using an erasable marker.
tACS
Transcranial alternating current stimulation was applied using a NeuroConn DC Stimulator Plus. The 42 × 45 mm tACS pad electrodes were applied to the scalp using a classical M1-contralateral supraorbital region electrode montage (see Heise et al., 2016), with the target electrode placed over the left M1 (which roughly corresponds with the EEG coordinate C3) and the return electrode placed over the contralateral supraorbital region. However, the electrode targeting the left M1 was not placed directly over the TMS hotspot itself, but rather ∼2 cm posterolateral to the hotspot (which roughly corresponds with Cp3). This slight increase in inter-electrode distance is thought to reduce current shunting through the scalp and cerebrospinal fluid, thus, maximizing current density at the target site and increasing the effectiveness of the tACS (Faria et al., 2011). Before attaching the electrodes, the scalp was rubbed with ethanol (70%) and Ten20 conductive paste was applied to the electrodes to minimize resistance between the electrodes and scalp.
Recording tACS Output
The tACS output was recorded using disposable surface electrodes (H124SG 30 mm × 24 mm). These electrodes were placed over the tACS pad targeting the supraorbital region and on the left side of the forehead, and referenced to the nose tip. The tACS artifact was used to synchronize stimulation with the computer used for MEP acquisition (i.e., such that each probe by TMS was timed with respect to the phase of tACS).
Data Collection
All surface electrode measurements (i.e., APB EMG and tACS output electrodes) were acquired (1 KHz sampling rate; 20–1000 Hz band pass filtering) via an electrode adaptor (Model CED1902-1 1/2), before being amplified by a CED1902, and finally recorded by a CED1401 MICRO3 (Cambridge Electronic Designs, Cambridge, United Kingdom). TMS triggers were directly recorded by the CED1401 MICRO3. All data were then transferred from the CED1401 MICRO3 to a PC and saved via Signal (Ver. 6.04) software (Cambridge Electronic Designs, Cambridge, United Kingdom), before being exported to MATLAB (Ver. R2019a) and subsequently JASP (Ver. 0.14.1.0) for analysis.
Experimental Procedure
tACS Paradigm
Participants received 60 trials of tACS, with each trial consisting of 16 s (12 cycles at 0.75 Hz) of tACS at an intensity of 2 mA (“Online”), followed by 16 s of no tACS (“Offline”), for a total of 16 min of tACS and 16 min of no tACS (Figure 1A). The entire stimulation period was divided into 3 blocks (∼10 min comprising 20 trials each), with 5-min rest periods (no tACS or TMS delivered) between blocks.
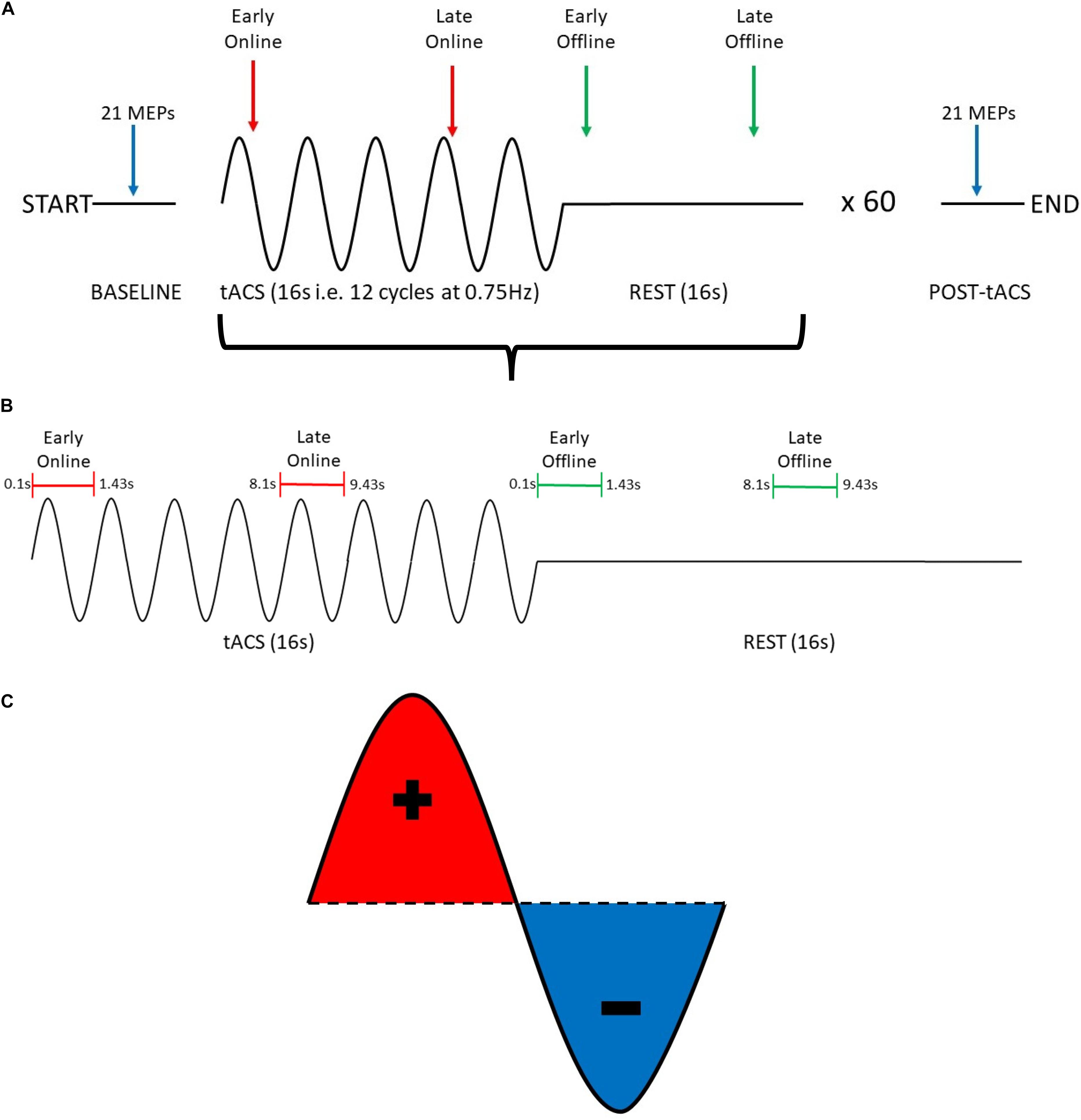
Figure 1. Summary of experimental procedure for probing changes in motor cortical excitability induced by SO tACS. (A) The experimental session consisted of a 16-s tACS period (represented as sine waves) followed by a 16-s rest period (represented as flat lines), repeated 60 times for a total of ∼32 min. To probe how tACS-induced changes in motor cortical excitability evolve over time, 21 TMS-induced MEPs were acquired at the start and the end of the experimental session (blue arrows), 2 MEPs were acquired at each tACS period (red arrows), and 2 MEPs were acquired at each rest period (green arrows). (B) Within each tACS trial (i.e., tACS + rest period), MEPs were acquired at 4 distinct time-points (1 MEP per time-point): early online, late online, early offline, and late offline. (C) tACS alternates polarity between the anode and cathode to produce a sinusoidal current with both positive (red) and negative (blue) peaks.
TMS Paradigm
To examine the online and offline effects of SO tACS on motor cortical excitability, MEPs were acquired at 4 time-points within each trial (1 MEP per time-point): early online (0.1–1.43 s after tACS starts), late online (8.1–9.43 s after tACS starts), early offline (0.1–1.43 s after tACS ends), and late offline (8.1–9.43 s after tACS ends) (Figure 1B). Therefore, 60 MEPs were acquired for each time-point (i.e., once each per trial).
To examine if the effects of SO tACS on motor cortical excitability are specific to the tACS phase (both online and offline), sufficient MEPs need to be acquired across the different phases of the tACS (Zoefel et al., 2019). This was achieved by implementing a “jitter” (i.e., a randomized time delay that covers the length of a single tACS cycle) to the delivery of TMS so that the delivery was not locked to a specific phase of the tACS (i.e., the timing of TMS delivery was random across the tACS cycle), and thus, TMS pulses were approximately uniformly delivered across the different phases across the entire stimulation block (Figure 1C).
To examine the cumulative effects of the entire tACS paradigm on motor cortical excitability, 21 TMS-induced MEPs were acquired both at baseline and at the end of the entire period of tACS delivery (Figure 1A). TMS was delivered at ∼0.2 Hz.
Statistical Analysis
Data Transformation
The first MEP for each data set (as well as the first MEP after each of the rest periods) was always excluded, since initial MEP amplitudes may be larger (Brasil-Neto et al., 1994) and more variable (Schmidt et al., 2009) than subsequent MEPs, which can impact the reliability of TMS measures of cortical excitability. Further, individual MEPs were excluded if voluntary pre-MEP EMG activity was detected in the 500 ms prior to TMS delivery (2.72% of MEPs excluded). Finally, participants with mean pre-tACS amplitudes less than 0.5 or greater than 1.5 mV were excluded from the final analysis (11 participants excluded). This is because excessively small or large pre-tACS MEPs may have introduced floor and ceiling effects, respectively (Cuypers et al., 2014).
The TMS triggers were then automatically categorized into their respective time-points (i.e., early online, late online, early offline, and late offline–see Figure 1B) and the “late online” triggers were used to calculate the tACS phase, since these triggers are the only ones where tACS was present both before and after TMS was applied, thus, providing the most reliable estimate of tACS phase. If tACS-induced phase entrainment persists beyond stimulation, we would expect the tACS phase to continue into the offline period. To assess this, the computed phase for the late online triggers was extrapolated (both forward and backward) and its values computed at each of the other time-points (Figure 2).
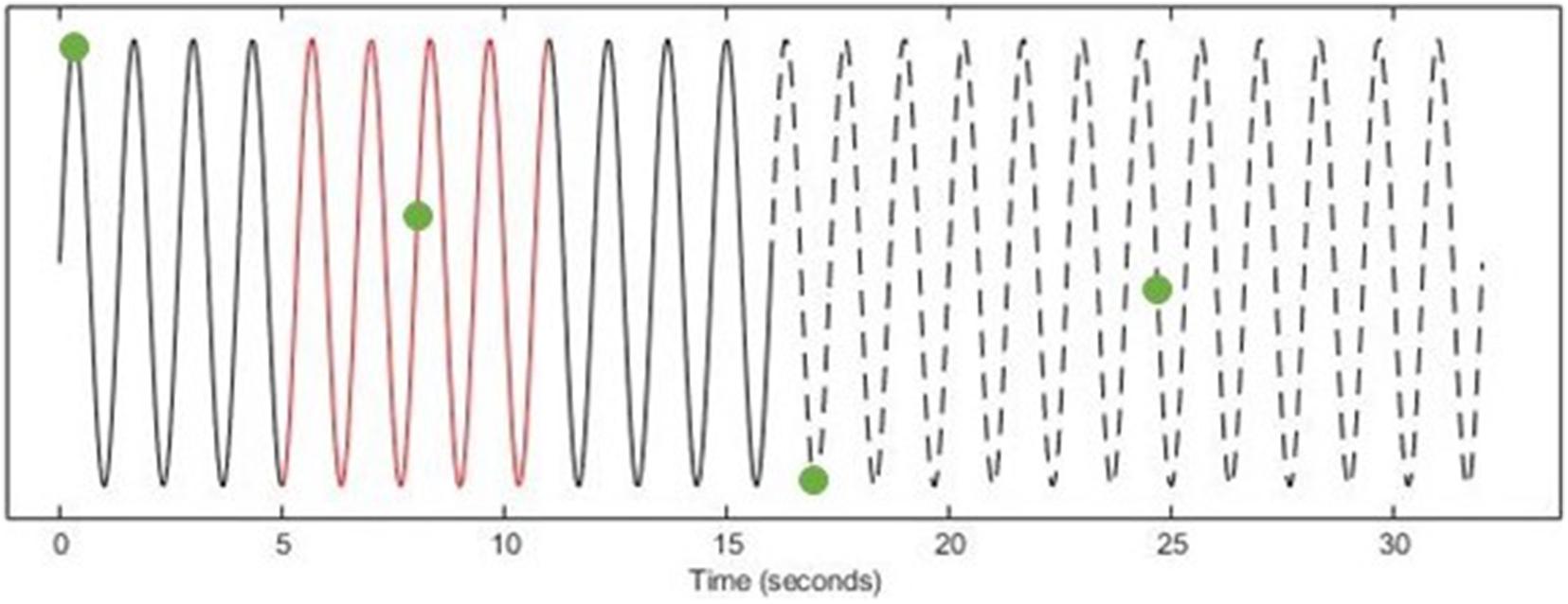
Figure 2. Determining tACS phase at TMS triggers (EXAMPLE ONLY). Using the tACS-output recording (Solid Line), a 6-s window (Red) of the instantaneous phase (centered on each late online trigger) was computed. The computed phase was then extrapolated both forward into the offline period (Dashed Line) and backward to the early online triggers (Solid Line) and the phase was computed at each of the other time-points (Green Dots).
Data Analysis
To determine if the effects of SO tACS on motor cortical excitability are specific to the tACS phase a permutation analysis was performed (Zoefel et al., 2019). This analysis was performed separately for each of the four time-points (∼60 MEPs per time-point per participant) as well as for all online and offline MEPs (∼120 MEPs online/offline per participant).
For the permutation analysis, an ideal (i.e., best-fitting, 0.75 Hz) sinusoidal model was fitted to each participant’s observed MEP amplitudes based on their phase for each of the four time-points (Bland and Sale, 2019), and the amplitudes of these models were summed. Because these models were fitted with bias (baseline), amplitude, and phase all free to vary across participants, there was no need for alignment of individual “preferred” phase. The MEP amplitudes were then shuffled with respect to their phases to form a surrogate distribution of expected amplitudes under the null hypothesis (which assumes that the MEP amplitudes are not modulated with respect to phase). Next, ideal sinusoidal models were fitted to the shuffled data, and the amplitudes of these shuffled models were summed. This process was repeated for a total of 1000 permutations per participant. The true and shuffled summed amplitudes were then compared. In this analysis, the P-value is the proportion of shuffled summed amplitudes exceeding the true sum of amplitudes, remembering that under the null hypothesis the amplitude of these sinusoidal models should be small (i.e., closer to zero). Because the permutation procedure disrupts any phasic effects that may be present, the shuffled MEPs act as a negative control for the true MEPs, and thus, the permutation analysis does not require a sham stimulation condition as a negative control.
To determine if there was a significant difference in mean MEP amplitudes between the pre- and post-tACS measurements, a paired sample t-test was performed. To determine if there was a significant difference in mean MEP amplitudes between the online and offline measurements or between the three tACS blocks, a two-way repeated measures ANOVA was performed with stimulation (online, offline) and block (1, 2, 3) as the two repeated measures factors. Post hoc t-tests (corrected for multiple comparisons using Holm’s method) were then performed to compare the individual groups against each other. To examine how offline changes in MEP amplitudes evolve throughout the tACS period, mean MEP amplitudes for the pre- and post-tACS MEPs and the offline MEPs of each tACS block were compared using a one-way repeated measures ANOVA with time as the repeated measures factor (5 levels). Again, post hoc t-tests were then performed to compare the individual groups against each other. For the repeated measures ANOVAs, standardized effect sizes for any significant differences were calculated as η2 values. For the post hoc t-tests, standardized effect sizes for any significant differences were calculated as Cohen’s d values.
Results
Phase-Specificity of tACS Effects
The phase-specificity of acute changes in motor cortical excitability induced by SO tACS was assessed by a permutation analysis. Ideal sinusoidal models were fitted to each participant’s observed MEP amplitudes based on their phase for each TMS time-point (∼60 MEPs per time-point per participant, see Supplementary Figure S1) and the amplitudes of these models (see Supplementary Figure S2) were summed. An example of one of these fitted sinusoidal models is shown in Figure 3. The true sum of amplitudes was then compared against the summed amplitudes of 1000 permutations of the MEP amplitudes, with P-values representing the proportion of shuffled summed amplitudes exceeding the true sum of amplitudes. The permutation analysis did not reveal significant phase-specific modulation of motor cortical excitability at any of the four TMS time-points (P = 0.86, 0.81, 0.21, and 0.70 for early online, late online, early offline, and late offline, respectively). Combining the early and late online/offline MEPs (∼120 MEPs online/offline per participant) also failed to reveal any significant phase-specific modulation of motor cortical excitability online or offline to tACS (P = 0.89 and 0.90, respectively).
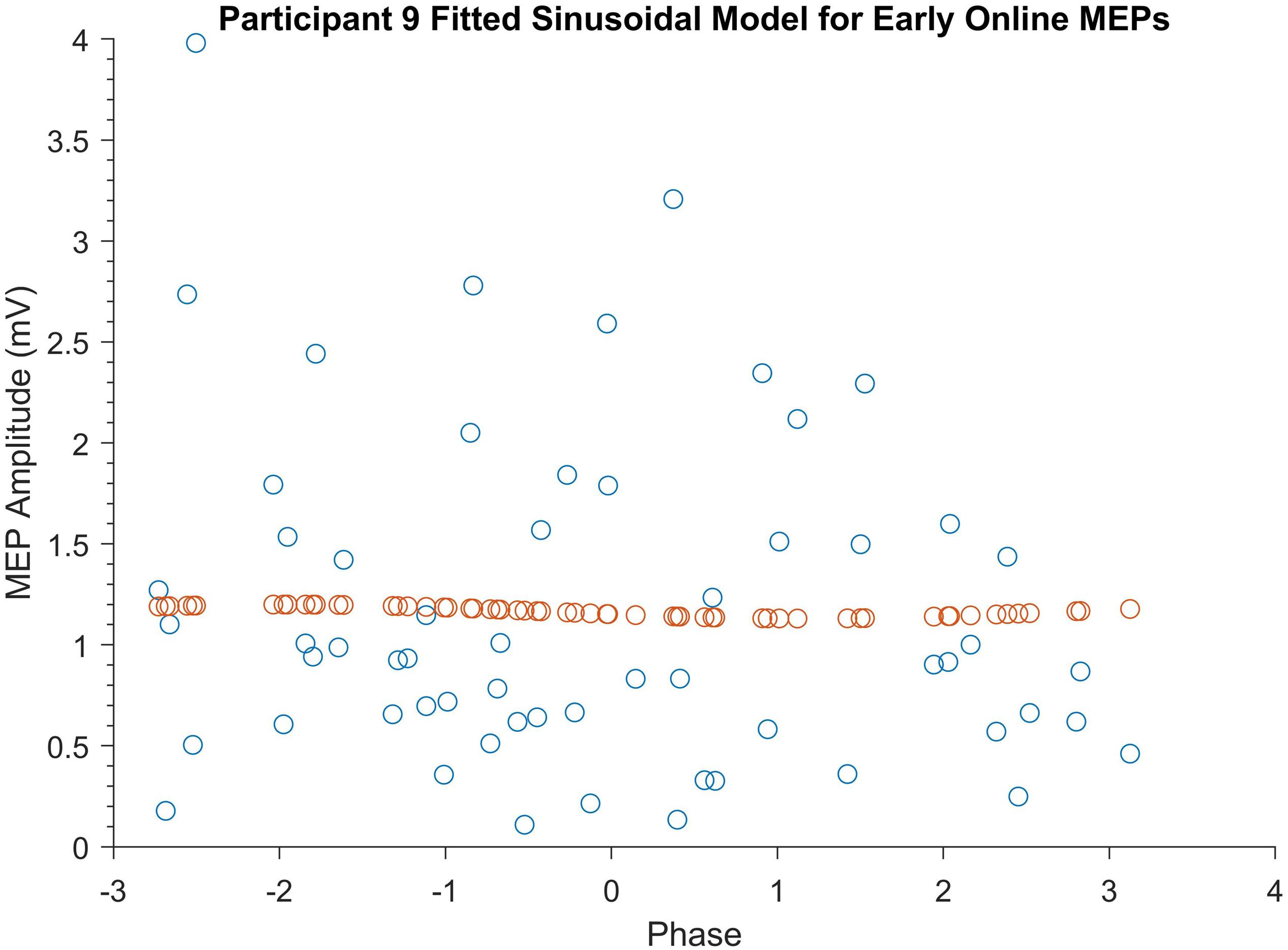
Figure 3. Example of a participant’s fitted sinusoidal model for early online MEPs. Blue dots represent Participant 9’s early online MEPs sorted according to tACS phase. Orange dots represent the fitted sinusoidal model for these MEPs.
Cumulative Effects of SO tACS on Motor Cortical Excitability
The cumulative effects of the tACS paradigm on motor cortical excitability was assessed by comparing mean MEP amplitudes pre- and post-tACS. As shown in Figure 4, MEP amplitudes were found to be significantly greater post-tACS (mean = 1.19 mV ± 0.84) compared to pre-tACS (mean = 0.82 mV ± 0.26) (t29 = 2.64, P = 0.013, d = 0.48).
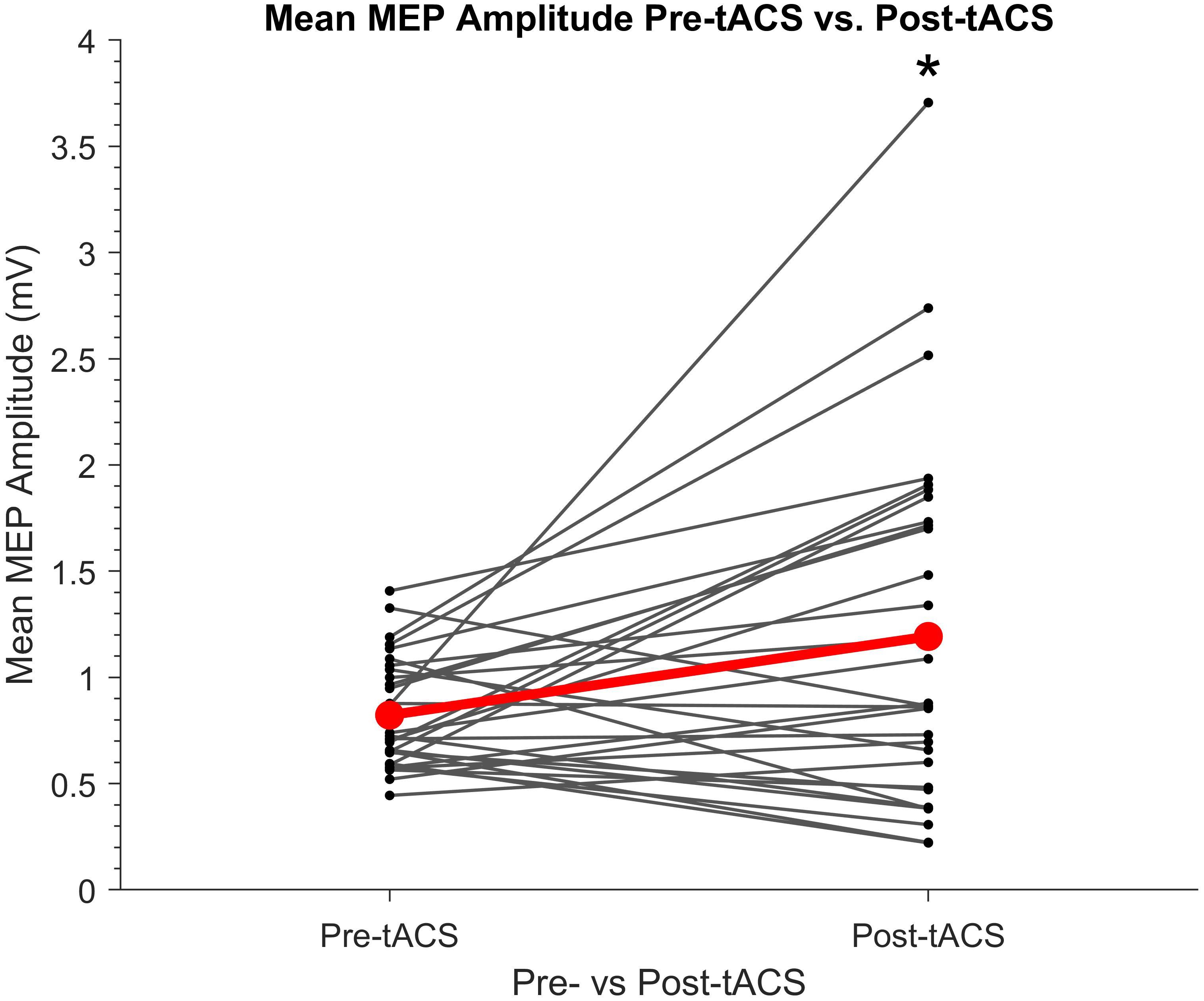
Figure 4. Individual mean amplitudes of TMS-induced MEPs before and after SO tACS. Points represent each participant’s mean MEP amplitude from 20 TMS-induced MEPs (per participant) acquired before (pre-tACS) and after (post-tACS) receiving 60 “trials” (∼32 min) of SO tACS, with each trial consisting of 16 s of tACS (12 cycles at 0.75 Hz) followed by 16 s of rest. Individual differences in mean MEP amplitude are represented by the solid lines. The global mean MEP amplitude for each group is represented by a red point, with the global mean difference from pre- to post-tACS represented by a red line connecting the two red points. A significant increase in MEP amplitude from pre-tACS to post-tACS was reported (paired sample t-test t29 = 2.64, P = 0.013, d = 0.48; indicated by *); n = 30.
Because there was a significant increase in mean MEP amplitude from pre- to post-tACS, the question arose of whether this overall change in MEP amplitude occurred gradually over time within the stimulation period. We therefore compared online and offline mean MEP amplitudes across each of the three tACS blocks. A two-way repeated measures ANOVA revealed a significant main effect of block (F2,58 = 3.77, P = 0.03, η2 = 0.1) but no main effect of stimulation (F1,29 = 0.65, P = 0.43) and no significant block × stimulation interaction (F2,58 = 1.29, P = 0.28). As shown in Figure 5, subsequent post hoc t-tests confirmed a significant increase in MEP amplitudes between the 1st (mean = 1.19 mV ± 0.72) and 3rd (mean = 1.46 mV ± 0.94) blocks (t29 = −2.93, P = 0.02, d = 0.54), whereas there were no significant differences between the 1st and 2nd (mean = 1.33 mV ± 0.79) blocks or between the 2nd and 3rd blocks (t29 = −1.6 and −1.04, respectively, P = 0.24 and 0.31, respectively). This suggests a gradual build-up of cortical excitability from tACS.
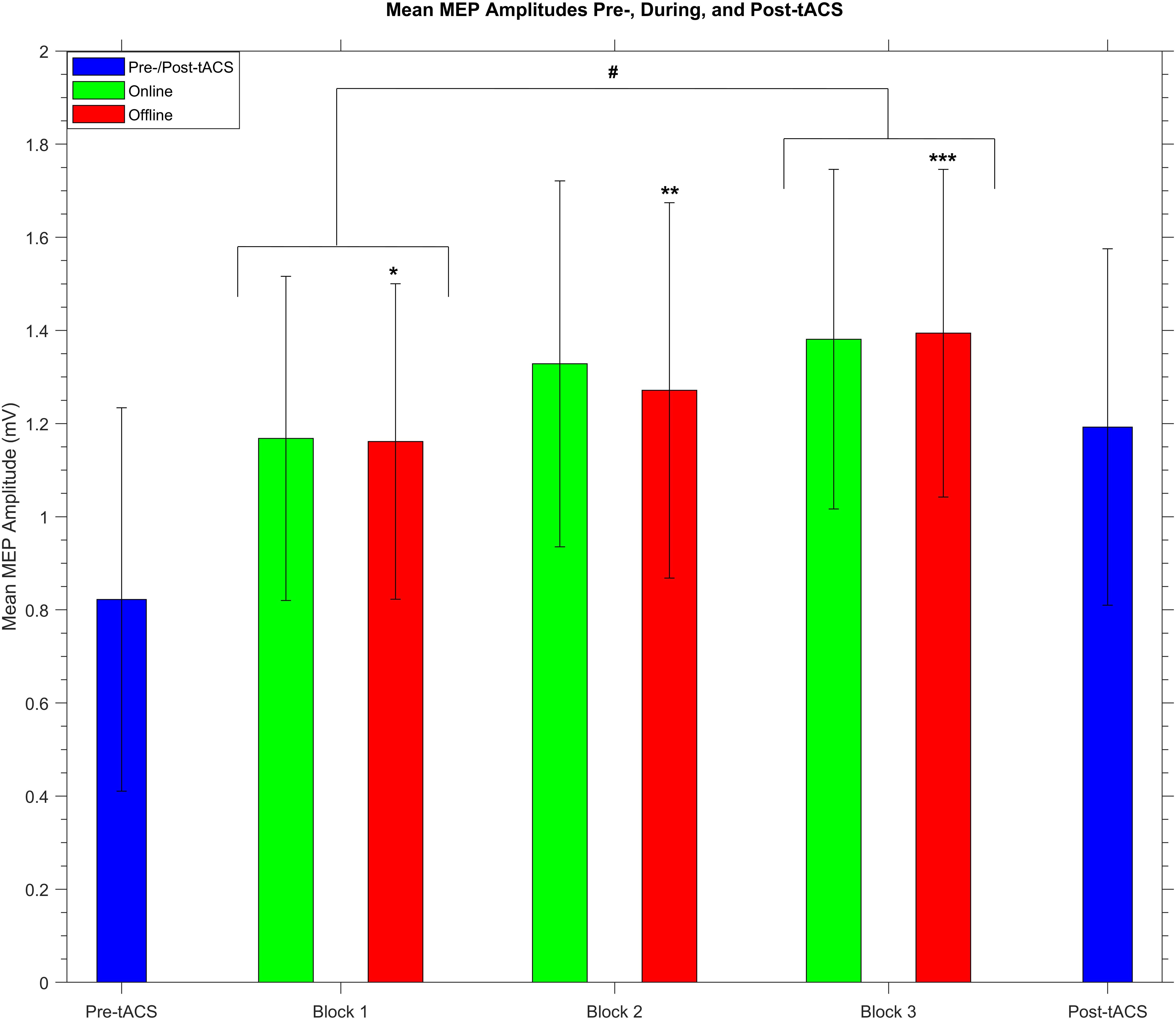
Figure 5. Group mean MEP amplitudes before, during, and after receiving SO tACS. Blue bars represent mean MEP amplitudes (with within-subjects error bars) from 20 TMS-induced MEPs (per participant) acquired before (pre-tACS) and after (post-tACS) receiving 60 “trials” (∼32 min, split into 3 20-trial “blocks”) of SO tACS, with each trial consisting of 16 s of tACS (online, 12 cycles at 0.75 Hz) followed by 16 s of rest (offline). Mean MEP amplitudes from 40 MEPs acquired during the online and offline periods of each block are represented by green and red bars, respectively. There was a significant increase in mean amplitude from the 1st to the 3rd block (t29 = −2.93, P = 0.02, d = 0.54; indicated by #). Furthermore, offline mean amplitudes were significantly greater than pre-tACS mean amplitudes for all three blocks (t29 = −3.24, −4.02, and −4.56; P = 0.024, 0.003, and <0.001; d = 0.59, 0.73, and 0.83 for blocks 1, 2, and 3, respectively; indicated by *, **, and ***, respectively); n = 30.
We also wished to compare mean MEP amplitudes from the offline periods of each block against each other as well as against the pre- and post-tACS mean amplitudes. A one-way repeated measures ANOVA revealed a significant main effect of time (F4,116 = 7.84, P < 0.001, η2 = 0.21). As shown in Figure 5, subsequent post hoc t-tests revealed that offline mean MEP amplitudes for all 3 blocks were significantly greater than pre-tACS mean amplitudes (t29 = −3.24, −4.02, and −4.56; P = 0.024, 0.003, and <0.001; d = 0.59, 0.73, and 0.83 for blocks 1, 2, and 3, respectively). However, no other significant differences in offline mean amplitude were reported when comparing the blocks against each other or against the post-tACS amplitudes, although the difference between the 1st and 3rd blocks was only marginally insignificant (t29 = −2.81, P = 0.06).
It is worth mentioning that widening the exclusion criteria for participants based on their pre-tACS mean MEP amplitudes (0.5–1.5 mV to 0.4–2 mV) did not affect the significance of the cumulative or phase-specific effects of SO tACS in the present study, despite increasing the sample size from 30 to 36 participants.
Discussion
Although there has been a plethora of studies in the last decade reporting behavioral, perceptual, and electrophysiological effects induced by tACS (for reviews see, Antal and Paulus, 2013; Herrmann et al., 2016; Vosskuhl et al., 2018; Bland and Sale, 2019), the mechanisms underlying these effects remain only rudimentarily understood. In the present study, we aimed to probe SO tACS-induced entrainment of endogenous slow oscillations both online and offline by assessing motor cortical excitability across different oscillatory phases using TMS-induced MEP amplitudes. We also assessed the cumulative effects of SO tACS on motor cortical excitability by comparing mean excitability pre- and post-stimulation as well as comparing mean excitability across stimulation blocks.
Regarding the cumulative effects of SO tACS, we present the first evidence of enhanced motor cortical excitability induced by SO tACS in the awake brain, with a significant increase in TMS-induced MEP amplitudes from pre- to post-tACS as well as from the 1st to the 3rd tACS block. Excitability increases induced by anodal SO tDCS have been reported previously (Bergmann et al., 2009; Groppa et al., 2010). However, compared to the SO tDCS study by Bergmann et al. (2009), the present study using SO tACS demonstrated a greater MEP amplitude increase (45.05% vs. 22%) despite shorter stimulation periods (16 s vs. 30 s), shorter total duration of stimulation (16 min vs. 17.5 min), longer rest periods between trials (16 s vs. 5 s) and two additional 5-min rest periods. Critically, due to the lack of an anodal component for tACS, this excitatory effect cannot be attributed to a general depolarization of cortical motor neurons and is thus driven by some other factor.
It is theoretically possible that the cumulative increase in motor cortical excitability was associated with an entrainment of endogenous slow oscillations (Marshall et al., 2006; Kirov et al., 2009; Jones et al., 2018; Ketz et al., 2018). However, the acute effects of stimulation did not appear to be dependent on the tACS phase, with the permutation analysis providing no evidence for phase-specific modulation of motor cortical excitability at any of the four TMS time-points (i.e., early/late online/offline) or for the combined online/offline MEPs.
The most likely explanation for the lack of an entrainment effect in the present results is that endogenous slow oscillations are not prevalent enough in the wake brain to be effectively entrained by SO tACS. This conclusion is in line with previous SO tDCS/tACS studies (Marshall et al., 2006; Bergmann et al., 2009; Kirov et al., 2009; Groppa et al., 2010; Jones et al., 2018; Ketz et al., 2018) as well as tACS studies using different stimulation frequencies (Antal et al., 2008; Kanai et al., 2008; Ali et al., 2013) that found stimulation to be most effective when the frequency of the exogenously applied oscillations closely matches the frequency of the predominant endogenous oscillations. These findings suggest that network resonance is a key underlying mechanism by which tACS modulates large-scale cortical network activity. These resonance dynamics are characterized by a phenomenon called an “Arnold Tongue,” where the current intensity required to induce a particular oscillation increases the more the frequency of that oscillation deviates from the resonant (eigen) frequency of the network (Ali et al., 2013; Thut et al., 2017; Liu et al., 2018).
If slow oscillations were in fact entrained by SO tACS, these entrained slow oscillations would likely be of a smaller magnitude than those that naturally occur during sleep (Marshall et al., 2006; Kirov et al., 2009; Jones et al., 2018; Ketz et al., 2018). Therefore, it is possible that the sensitivity of the current permutation analysis was insufficient to detect such a subtle entrainment effect. The sensitivity of our permutation analysis may have been impacted by the relatively low number of MEPs (60 MEPs per time-point per participant), which we know from simulation studies impacts detectability (Zoefel et al., 2019). However, it is important to note that the number of MEPs we could acquire was limited by a number of practical considerations, including coil recharge time, coil heating, and session length, whereas simulation MEP numbers are unencumbered by practical limitations. Because the number of MEPs we can acquire in a single stimulation session is limited by these practical considerations, an alternative option to increase the number of MEPs would be to increase the number of sessions per participant and then pool the MEPs across sessions.
In future experiments, tACS will instead be applied at a frequency that is naturally present in the motor cortex during wakefulness, such as the sensorimotor mu (μ) rhythm (8–13 Hz; Antal et al., 2008; Wach et al., 2013; Gundlach et al., 2017; Thies et al., 2018; Feurra et al., 2019; Madsen et al., 2019). This will also allow us to determine if the excitatory effect observed in the present experiment is specific to SO tACS or if similar effects are observed for other stimulation frequencies.
Alternatively, because the present experiment did not include a negative control stimulation condition for SO tACS (e.g., sham stimulation), it is theoretically possible that the observed increase in motor cortical excitability is simply a time-dependent effect and not mediated by tACS and this is a limitation of the experiment. However, this seems highly unlikely given that a recent meta-analysis by Dissanayaka et al. (2018) reported no significant effects of sham tES on cortical excitability compared to baseline, even up to 90 min following sham tES (Moliadze et al., 2010, 2012; Chaieb et al., 2011). Although only some of the assessed tES studies specifically investigated tACS, all of the studies used a comparable fade-in, short stimulation, fade-out (FISSFO) sham condition, and thus, they can all be used to make inferences about the likely tACS-free changes in MEP amplitude (i.e., solely due to time). This provides a compelling null comparator for the significant increase in MEP amplitude by SO tACS.
Although the underlying cause of the observed increase in MEP amplitude cannot be concluded from the present results, the lack of phase-specific entrainment suggests that this excitatory effect may instead be driven by plasticity-related mechanisms, such as spike-timing dependent plasticity (STDP; Veniero et al., 2015; Vossen et al., 2015). In the STDP model, even a slight mismatch between the stimulation frequency and an individual’s spontaneous peak frequency could influence the direction of any induced changes, which may explain the heterogeneity of tACS aftereffects across studies (Veniero et al., 2015). Tests of this model should therefore tailor stimulation frequency to each participant’s individual peak frequency rather than use a standard frequency such as was used in the present study.
It is important to note that entrainment and plastic-like effects induced by tACS are not mutually exclusive (Vosskuhl et al., 2018). In fact, Helfrich et al. (2014a, b) found the magnitude of induced aftereffects to be positively correlated with the magnitude of online entrainment and also demonstrated that online effects occurred within a narrow frequency range whilst offline effects occurred across a broader band around the frequency of tACS. This suggests that whilst online effects may be explained by entrainment, sustained aftereffects may be better explained by entrainment-mediated changes to network strength, which then oscillates close (but not necessarily equal) to the frequency of stimulation.
Elucidation of the mechanisms underlying the online and offline effects of tACS will better its therapeutic applications. For example, the ability to induce lasting plastic changes in the motor cortex using tACS may improve the effectiveness of existing rehabilitation for neurological conditions where motor function is compromised.
Conclusion
In summary, the significant increase in TMS-induced MEP amplitudes from pre- to post-SO tACS as well as from the 1st to the 3rd SO tACS block suggests that, similar to previously reported excitability increases induced by anodal SO tDCS, SO tACS had a facilitatory effect on motor cortical excitability that outlasted the stimulation period. Importantly, the present findings suggest that these motor cortical excitability increases are not simply due to anodal stimulation. However, given the acute effects of SO tACS were independent of phase, this study does not support entrainment of endogenous slow oscillations as an underlying mechanism for this excitatory effect.
Data Availability Statement
The datasets presented in this study can be found in online repositories. The names of the repository/repositories and accession number(s) can be found below: https://doi.org/10.17605/OSF.IO/DAV4T, Open Science Framework, Reference “SO tACS 2019.”
Ethics Statement
The studies involving human participants were reviewed and approved by The University of Queensland Human Research Ethics Committee. The patients/participants provided their written informed consent to participate in this study.
Author Contributions
AG: conceptualization, methodology, software, formal analysis, investigation, data curation, writing–original draft, and visualization. NB: conceptualization, methodology, software, data curation, writing–review and editing, visualization, and supervision. MS: conceptualization, methodology, validation, resources, writing–review and editing, project administration, funding acquisition, and supervision. All authors contributed to the article and approved the submitted version.
Funding
This work was supported by the U.S. Office of Naval Research Global (grant number N62909-17-1-2139) awarded to MS. The funding body had no involvement in the study design; the collection, analysis, and interpretation of data; the writing of the report; or the decision to submit the article for publication.
Conflict of Interest
The authors declare that the research was conducted in the absence of any commercial or financial relationships that could be construed as a potential conflict of interest.
Publisher’s Note
All claims expressed in this article are solely those of the authors and do not necessarily represent those of their affiliated organizations, or those of the publisher, the editors and the reviewers. Any product that may be evaluated in this article, or claim that may be made by its manufacturer, is not guaranteed or endorsed by the publisher.
Acknowledgments
We would like to thank Kylie Tucker for her role as co-supervisor during AG’s honors research program. We would also like to thank Laurie and Gina Geffen for their help in proofreading the manuscript.
Supplementary Material
The Supplementary Material for this article can be found online at: https://www.frontiersin.org/articles/10.3389/fnhum.2021.726604/full#supplementary-material
Supplementary Figure S1 | Fitted sinusoidal models for each participant’s late online MEPs. Each scatter plot represents one participant (n = 30), with blue dots (n = 60) representing individual MEP amplitudes (y-axis) sorted according to tACS phase (x-axis). Orange dots represent the sinusoidal model fitted to each scatter plot. A permutation analysis across all participants revealed no significant modulation of late online MEP amplitudes with respect to tACS phase (p = 0.81). Note there was a “gap” in phase values for the first 3 participants due to an error in the MATLAB script that determined the timing of TMS delivery, but this error was addressed after the 3rd participant and the phase gap is not present in any of the other participants.
Supplementary Figure S2 | Histograms of fitted sinusoidal model amplitudes for each position. Bars represent the number of participants with fitted sinusoidal model amplitudes within the range specified on the x-axis for (A) early online, (B) late online, (C) early offline, and (D) late offline MEPs.
Abbreviations
tES, transcranial electrical stimulation; tACS, transcranial alternating current stimulation; tDCS, transcranial direct current stimulation; SO, slow oscillatory; TMS, transcranial magnetic stimulation; EEG, electroencephalography; EMG, electromyography; MEP, motor evoked potential; M1HAND, hand area of the primary motor cortex; APB, abductor pollicis brevis; FISSFO, fade-in, short stimulation, fade-out; STDP, spike-timing dependent plasticity.
References
Ali, M. M., Sellers, K. K., and Frohlich, F. (2013). Transcranial alternating current stimulation modulates large-scale cortical network activity by network resonance. J. Neurosci. 33, 11262–11275. doi: 10.1523/jneurosci.5867-12.2013
Antal, A., Boros, K., Poreisz, C., Chaieb, L., Terney, D., and Paulus, W. (2008). Comparatively weak after-effects of transcranial alternating current stimulation (tACS) on cortical excitability in humans. Brain Stimul. 1, 97–105. doi: 10.1016/j.brs.2007.10.001
Antal, A., and Paulus, W. (2013). Transcranial alternating current stimulation (tACS). Front. Hum. Neurosci. 7:317. doi: 10.3389/fnhum.2013.00317
Barker, A. T., Jalinous, R., and Freeston, I. L. (1985). Non-invasive magnetic stimulation of human motor cortex. Lancet 325, 1106–1107. doi: 10.1016/S0140-6736(85)92413-4
Bergmann, T. O., Groppa, S., Seeger, M., Mölle, M., Marshall, L., and Siebner, H. R. (2009). Acute changes in motor cortical excitability during slow oscillatory and constant anodal transcranial direct current stimulation. J. Neurophysiol. 102, 2303–2311. doi: 10.1152/jn.00437.2009
Bergmann, T. O., Mölle, M., Schmidt, M. A., Lindner, C., Marshall, L., Born, J., et al. (2012). EEG-guided transcranial magnetic stimulation reveals rapid shifts in motor cortical excitability during the human sleep slow oscillation. J. Neurosci. 32, 243–253. doi: 10.1523/JNEUROSCI.4792-11.2012
Bland, N. S., and Sale, M. V. (2019). Current challenges: the ups and downs of tACS. Exp. Brain Res. 237, 3071–3088. doi: 10.1007/s00221-019-05666-0
Brasil−Neto, J. P., Cohen, L. G., and Hallett, M. (1994). Central fatigue as revealed by postexercise decrement of motor evoked potentials. Muscle Nerve 17, 713–719. doi: 10.1002/mus.880170702
Chaieb, L., Antal, A., and Paulus, W. (2011). Transcranial alternating current stimulation in the low kHz range increases motor cortex excitability. Restorat. Neurol. Neurosci. 29, 167–175. doi: 10.3233/RNN-2011-0589
Cuypers, K., Thijs, H., and Meesen, R. L. J. (2014). Optimization of the transcranial magnetic stimulation protocol by defining a reliable estimate for corticospinal excitability. PLoS One 9:e86380. doi: 10.1371/journal.pone.0086380
Di Lazzaro, V., Oliviero, A., Pilato, F., Saturno, E., Dileone, M., Mazzone, P., et al. (2004). The physiological basis of transcranial motor cortex stimulation in conscious humans. Clin. Neurophysiol. 115, 255–266. doi: 10.1016/j.clinph.2003.10.009
Dissanayaka, T., Zoghi, M., Farrell, M., Egan, G. F., and Jaberzadeh, S. (2018). Sham transcranial electrical stimulation and its effects on corticospinal excitability: a systematic review and meta-analysis. Rev. Neurosci. 29, 223–232. doi: 10.1515/revneuro-2017-0026
Faria, P., Hallett, M., and Miranda, P. C. (2011). A finite element analysis of the effect of electrode area and inter-electrode distance on the spatial distribution of the current density in tDCS. J. Neural Eng. 8:066017. doi: 10.1088/1741-2560/8/6/066017
Feurra, M., Blagovechtchenski, E., Nikulin, V. V., Nazarova, M., Lebedeva, A., Pozdeeva, D., et al. (2019). State-dependent effects of transcranial oscillatory currents on the motor system during action observation. Sci. Rep. 9:12858. doi: 10.1038/s41598-019-49166-1
Groppa, S., Bergmann, T. O., Siems, C., Mölle, M., Marshall, L., and Siebner, H. R. (2010). Slow-oscillatory transcranial direct current stimulation can induce bidirectional shifts in motor cortical excitability in awake humans. Neuroscience 166, 1219–1225. doi: 10.1016/j.neuroscience.2010.01.019
Gundlach, C., Müller, M. M., Nierhaus, T., Villringer, A., and Sehm, B. (2017). Modulation of somatosensory alpha rhythm by transcranial alternating current stimulation at mu-frequency. Front. Hum. Neurosci. 11:432. doi: 10.3389/fnhum.2017.00432
Hallett, M. (2007). Transcranial magnetic stimulation: a primer. Neuron 55, 187–199. doi: 10.1016/j.neuron.2007.06.026
Hanslmayr, S., Matuschek, J., and Fellner, M.-C. (2014). Entrainment of prefrontal beta oscillations induces an endogenous echo and impairs memory formation. Curr. Biol. 24, 904–909. doi: 10.1016/j.cub.2014.03.007
Heise, K.-F., Kortzorg, N., Saturnino, G. B., Fujiyama, H., Cuypers, K., Thielscher, A., et al. (2016). Evaluation of a modified high-definition electrode montage for transcranial alternating current stimulation (tACS) of pre-central areas. Brain Stimul. 9, 700–704. doi: 10.1016/j.brs.2016.04.009
Helfrich, R. F., Knepper, H., Nolte, G., Strüber, D., Rach, S., Herrmann, C. S., et al. (2014a). Selective modulation of interhemispheric functional connectivity by HD-tACS shapes perception. PLoS Biol. 12:e1002031. doi: 10.1371/journal.pbio.1002031
Helfrich, R. F., Schneider, T. R., Rach, S., Trautmann-Lengsfeld, S. A., Engel, A. K., and Herrmann, C. S. (2014b). Entrainment of brain oscillations by transcranial alternating current stimulation. Curr. Biol. 24, 333–339. doi: 10.1016/j.cub.2013.12.041
Herrmann, C. S., Strüber, D., Helfrich, R. F., and Engel, A. K. (2016). EEG oscillations: from correlation to causality. Int. J. Psychophysiol. 103, 12–21. doi: 10.1016/j.ijpsycho.2015.02.003
Huang, W. A., Stitt, I. M., Negahbani, E., Passey, D. J., Ahn, S., Davey, M., et al. (2021). Transcranial alternating current stimulation entrains alpha oscillations by preferential phase synchronization of fast-spiking cortical neurons to stimulation waveform. Nat. Commun. 12:3151. doi: 10.1038/s41467-021-23021-2
Huang, Y., Liu, A. A., Lafon, B., Friedman, D., Dayan, M., Wang, X., et al. (2017). Measurements and models of electric fields in the in vivo human brain during transcranial electric stimulation. eLife 6:e18834. doi: 10.7554/eLife.18834
Ilmoniemi, R. J., and Kicić, D. (2010). Methodology for combined TMS and EEG. Brain Topog. 22, 233–248. doi: 10.1007/s10548-009-0123-4
Jones, A. P., Choe, J., Bryant, N. B., Robinson, C. S. H., Ketz, N. A., Skorheim, S. W., et al. (2018). Dose-dependent effects of closed-loop tACS delivered during slow-wave oscillations on memory consolidation. Front. Neurosci. 12:867. doi: 10.3389/fnins.2018.00867
Kanai, R., Chaieb, L., Antal, A., Walsh, V., and Paulus, W. (2008). Frequency-dependent electrical stimulation of the visual cortex. Curr. Biol. 18, 1839–1843. doi: 10.1016/j.cub.2008.10.027
Kasten, F. H., Dowsett, J., and Herrmann, C. S. (2016). Sustained aftereffect of α-tACS lasts up to 70 min after stimulation. Front. Hum. Neurosci. 10:245. doi: 10.3389/fnhum.2016.00245
Kasten, F. H., and Herrmann, C. S. (2019). Recovering brain dynamics during concurrent tACS-M/EEG: an overview of analysis approaches and their methodological and interpretational pitfalls. Brain Topography 32, 1013–1019. doi: 10.1007/s10548-019-00727-7
Keel, J. C., Smith, M. J., and Wassermann, E. M. (2001). A safety screening questionnaire for transcranial magnetic stimulation. Clin. Neurophysiol. 112:720. doi: 10.1016/s1388-2457(00)00518-6
Ketz, N. A., Jones, A. P., Bryant, N. B., Clark, V. P., and Pilly, P. K. (2018). Closed-loop slow-wave tACS improves sleep-dependent long-term memory generalization by modulating endogenous oscillations. J. Neurosci. 38, 7314–7326. doi: 10.1523/jneurosci.0273-18.2018
Kirov, R., Weiss, C., Siebner, H. R., Born, J., and Marshall, L. (2009). Slow oscillation electrical brain stimulation during waking promotes EEG theta activity and memory encoding. PNAS 106, 15460–15465. doi: 10.1073/pnas.0904438106
Krause, M. R., Vieira, P. G., Csorba, B. A., Pilly, P. K., and Pack, C. C. (2019). Transcranial alternating current stimulation entrains single-neuron activity in the primate brain. PNAS 116, 5747–5755. doi: 10.1073/pnas.1815958116
Liu, A. A., Vöröslakos, M., Kronberg, G., Henin, S., Krause, M. R., Huang, Y., et al. (2018). Immediate neurophysiological effects of transcranial electrical stimulation. Nat. Commun. 9, 5092–5092. doi: 10.1038/s41467-018-07233-7
Madsen, M., Takemi, M., Kesselheim, J., Tashiro, S., and Siebner, H. R. (2019). Focal TACS of the primary motor hand area at individual mu and beta rhythm–effects on cortical excitability. Brain Stimul. 12:572. doi: 10.1016/j.brs.2018.12.896
Marshall, L., and Binder, S. (2013). Contribution of transcranial oscillatory stimulation to research on neural networks: an emphasis on hippocampo-neocortical rhythms. Front. Hum. Neurosci. 7:614. doi: 10.3389/fnhum.2013.00614
Marshall, L., Helgadóttir, H., Mölle, M., and Born, J. (2006). Boosting slow oscillations during sleep potentiates memory. Nature 444:610. doi: 10.1038/nature05278
Moliadze, V., Antal, A., and Paulus, W. (2010). Boosting brain excitability by transcranial high frequency stimulation in the ripple range. J. Physiol. 588, 4891–4904. doi: 10.1113/jphysiol.2010.196998
Moliadze, V., Atalay, D., Antal, A., and Paulus, W. (2012). Close to threshold transcranial electrical stimulation preferentially activates inhibitory networks before switching to excitation with higher intensities. Brain Stimul. 5, 505–511. doi: 10.1016/j.brs.2011.11.004
Neuling, T., Rach, S., and Herrmann, C. S. (2013). Orchestrating neuronal networks: sustained after-effects of transcranial alternating current stimulation depend upon brain states. Front. Hum. Neurosci. 7:161. doi: 10.3389/fnhum.2013.00161
Nitsche, M. A., Doemkes, S., Karaköse, T., Antal, A., Liebetanz, D., Lang, N., et al. (2007). Shaping the effects of transcranial direct current stimulation of the human motor cortex. J. Neurophysiol. 97, 3109–3117. doi: 10.1152/jn.01312.2006
Nitsche, M. A., and Paulus, W. (2000). Excitability changes induced in the human motor cortex by weak transcranial direct current stimulation. J. Physiol. 527, 633–639. doi: 10.1111/j.1469-7793.2000.t01-1-00633.x
Noury, N., Hipp, J. F., and Siegel, M. (2016). Physiological processes non-linearly affect electrophysiological recordings during transcranial electric stimulation. NeuroImage 140, 99–109. doi: 10.1016/j.neuroimage.2016.03.065
Noury, N., and Siegel, M. (2017). Phase properties of transcranial electrical stimulation artifacts in electrophysiological recordings. NeuroImage 158, 406–416. doi: 10.1016/j.neuroimage.2017.07.010
Ogata, K., Nakazono, H., Uehara, T., and Tobimatsu, S. (2019). Prestimulus cortical EEG oscillations can predict the excitability of the primary motor cortex. Brain Stimul. 12, 1508–1516. doi: 10.1016/j.brs.2019.06.013
Raco, V., Bauer, R., Tharsan, S., and Gharabaghi, A. (2016). Combining TMS and tACS for closed-loop phase-dependent modulation of corticospinal excitability: a feasibility study. Front. Cell. Neurosci. 10:143. doi: 10.3389/fncel.2016.00143
Reato, D., Rahman, A., Bikson, M., and Parra, L. C. (2010). Low-intensity electrical stimulation affects network dynamics by modulating population rate and spike timing. J. Neurosci. 30, 15067–15079. doi: 10.1523/JNEUROSCI.2059-10.2010
Reato, D., Rahman, A., Bikson, M., and Parra, L. C. (2013). Effects of weak transcranial alternating current stimulation on brain activity—a review of known mechanisms from animal studies. Front. Hum. Neurosci. 7:687. doi: 10.3389/fnhum.2013.00687
Rossini, P. M., Barker, A. T., Berardelli, A., Caramia, M. D., Caruso, G., Cracco, R. Q., et al. (1994). Non-invasive electrical and magnetic stimulation of the brain, spinal cord and roots: basic principles and procedures for routine clinical application. Report of an IFCN committee. Electroencephalogr. Clin. Neurophysiol. 91, 79–92. doi: 10.1016/0013-4694(94)90029-9
Schaworonkow, N., Triesch, J., Ziemann, U., and Zrenner, C. (2019). EEG-triggered TMS reveals stronger brain state-dependent modulation of motor evoked potentials at weaker stimulation intensities. Brain Stimul. 12, 110–118. doi: 10.1016/j.brs.2018.09.009
Schmidt, S., Cichy, R. M., Kraft, A., Brocke, J., Irlbacher, K., and Brandt, S. A. (2009). An initial transient-state and reliable measures of corticospinal excitability in TMS studies. Clin. Neurophysiol. 120, 987–993. doi: 10.1016/j.clinph.2009.02.164
Thies, M., Zrenner, C., Ziemann, U., and Bergmann, T. O. (2018). Sensorimotor mu-alpha power is positively related to corticospinal excitability. Brain Stimul. 11, 1119–1122. doi: 10.1016/j.brs.2018.06.006
Thut, G., Bergmann, T. O., Fröhlich, F., Soekadar, S. R., Brittain, J.-S., Valero- Cabré, A., et al. (2017). Guiding transcranial brain stimulation by EEG/MEG to interact with ongoing brain activity and associated functions: a position paper. Clin. Neurophysiol. 128, 843–857. doi: 10.1016/j.clinph.2017.01.003
Thut, G., Veniero, D., Romei, V., Miniussi, C., Schyns, P., and Gross, J. (2011). Rhythmic TMS causes local entrainment of natural oscillatory signatures. Curr. Biol. 21, 1176–1185. doi: 10.1016/j.cub.2011.05.049
van Bree, S., Sohoglu, E., Davis, M. H., and Zoefel, B. (2021). Sustained neural rhythms reveal endogenous oscillations supporting speech perception. PLoS Biol. 19:e3001142. doi: 10.1371/journal.pbio.3001142
Veniero, D., Vossen, A., Gross, J., and Thut, G. (2015). Lasting EEG/MEG aftereffects of rhythmic transcranial brain stimulation: level of control over oscillatory network activity. Front. Cell. Neurosci. 9:477. doi: 10.3389/fncel.2015.00477
Vossen, A., Gross, J., and Thut, G. (2015). Alpha power increase after transcranial alternating current stimulation at alpha frequency (α-tACS) reflects plastic changes rather than entrainment. Brain Stimul. 8, 499–508. doi: 10.1016/j.brs.2014.12.004
Vosskuhl, J., Strüber, D., and Herrmann, C. S. (2018). Non-invasive brain stimulation: a paradigm shift in understanding brain oscillations. Front. Hum. Neurosci. 12:211. doi: 10.3389/fnhum.2018.00211
Wach, C., Krause, V., Moliadze, V., Paulus, W., Schnitzler, A., and Pollok, B. (2013). Effects of 10Hz and 20Hz transcranial alternating current stimulation (tACS) on motor functions and motor cortical excitability. Behav. Brain Res. 241, 1–6. doi: 10.1016/j.bbr.2012.11.038
Witkowski, M., Garcia-Cossio, E., Chander, B. S., Braun, C., Birbaumer, N., Robinson, S. E., et al. (2016). Mapping entrained brain oscillations during transcranial alternating current stimulation (tACS). NeuroImage 140, 89–98. doi: 10.1016/j.neuroimage.2015.10.024
Zoefel, B., Davis, M. H., Valente, G., and Riecke, L. (2019). How to test for phasic modulation of neural and behavioural responses. NeuroImage 202:116175. doi: 10.1101/517243
Keywords: transcranial alternating current stimulation, transcranial magnetic stimulation, entrainment, plasticity, neural oscillations
Citation: Geffen A, Bland N and Sale MV (2021) Effects of Slow Oscillatory Transcranial Alternating Current Stimulation on Motor Cortical Excitability Assessed by Transcranial Magnetic Stimulation. Front. Hum. Neurosci. 15:726604. doi: 10.3389/fnhum.2021.726604
Received: 17 June 2021; Accepted: 24 August 2021;
Published: 13 September 2021.
Edited by:
Masaki Sekino, The University of Tokyo, JapanReviewed by:
Joaquim Pereira Brasil-Neto, Unieuro, BrazilTill R. Schneider, University of Hamburg, Germany
Copyright © 2021 Geffen, Bland and Sale. This is an open-access article distributed under the terms of the Creative Commons Attribution License (CC BY). The use, distribution or reproduction in other forums is permitted, provided the original author(s) and the copyright owner(s) are credited and that the original publication in this journal is cited, in accordance with accepted academic practice. No use, distribution or reproduction is permitted which does not comply with these terms.
*Correspondence: Asher Geffen, a.geffen@uq.net.au
†ORCID: Asher Geffen, orcid.org/0000-0002-9982-9534; Nicholas Bland, orcid.org/0000-0001-9610-9972; Martin V. Sale, orcid.org/0000-0002-2913-9212