- 1NTU Institute for Health Technologies, Interdisciplinary Graduate Programme, Nanyang Technological University, Singapore, Singapore
- 2Center for Sleep and Cognition, Center for Translational MR Research, Yong Loo Lin School of Medicine, National University of Singapore, Singapore, Singapore
- 3Department of Biomedical Engineering, Faculty of Engineering, National University of Singapore, Singapore, Singapore
- 4Institute for Infocomm Research, Agency for Science Technology and Research, Singapore, Singapore
- 5School of Computer Science and Engineering, Nanyang Technological University, Singapore, Singapore
- 6Singapore Bioimaging Consortium, Agency for Science Technology and Research, Singapore, Singapore
- 7Queensland Brain Institute and Centre for Advanced Imaging, The University of Queensland, Brisbane, QLD, Australia
- 8School of Electrical and Electronic Engineering, Nanyang Technological University, Singapore, Singapore
- 9Division of Neurology, University Medicine Cluster, National University Health System, Singapore, Singapore
- 10Department of Electrical and Computer Engineering, National University of Singapore, Singapore, Singapore
- 11Integrative Sciences and Engineering Programme (ISEP), National University of Singapore, Singapore, Singapore
Brain-computer interface-assisted motor imagery (MI-BCI) or transcranial direct current stimulation (tDCS) has been proven effective in post-stroke motor function enhancement, yet whether the combination of MI-BCI and tDCS may further benefit the rehabilitation of motor functions remains unknown. This study investigated brain functional activity and connectivity changes after a 2 week MI-BCI and tDCS combined intervention in 19 chronic subcortical stroke patients. Patients were randomized into MI-BCI with tDCS group and MI-BCI only group who underwent 10 sessions of 20 min real or sham tDCS followed by 1 h MI-BCI training with robotic feedback. We derived amplitude of low-frequency fluctuation (ALFF), regional homogeneity (ReHo), and functional connectivity (FC) from resting-state functional magnetic resonance imaging (fMRI) data pre- and post-intervention. At baseline, stroke patients had lower ALFF in the ipsilesional somatomotor network (SMN), lower ReHo in the contralesional insula, and higher ALFF/Reho in the bilateral posterior default mode network (DMN) compared to age-matched healthy controls. After the intervention, the MI-BCI only group showed increased ALFF in contralesional SMN and decreased ALFF/Reho in the posterior DMN. In contrast, no post-intervention changes were detected in the MI-BCI + tDCS group. Furthermore, higher increases in ALFF/ReHo/FC measures were related to better motor function recovery (measured by the Fugl-Meyer Assessment scores) in the MI-BCI group while the opposite association was detected in the MI-BCI + tDCS group. Taken together, our findings suggest that brain functional re-normalization and network-specific compensation were found in the MI-BCI only group but not in the MI-BCI + tDCS group although both groups gained significant motor function improvement post-intervention with no group difference. MI-BCI and tDCS may exert differential or even opposing impact on brain functional reorganization during post-stroke motor rehabilitation; therefore, the integration of the two strategies requires further refinement to improve efficacy and effectiveness.
Introduction
Robot-assisted motor imagery (MI) and brain-computer interface (BCI) system is an advanced technique for improving motor function recovery in post-stroke rehabilitation. Motor imagery allows the imagination of movements without physical execution, and electroencephalography-based brain-computer interfaces (EEG-BCIs) enables interaction with the environment using brain signals (Dodakian et al., 2014; Bockbrader et al., 2018). By combining robot-assisted MI and BCI, the brain-computer interface-assisted motor imagery (MI-BCI) system enables stroke patients to move the impaired limb driven by a robotic arm via neural signals from the motor imagery of the arm and provides multisensory feedback to patients to enhance motor learning and recovery (Ang et al., 2014, 2009; Ang and Guan, 2015; Wang H. et al., 2019; Foong et al., 2020).
Transcranial direct current stimulation (tDCS) is another promising technique for stroke rehabilitation. tDCS is a non-invasive brain stimulation technique that uses constant, low direct current delivered via electrodes (anode and cathode) on the head to provide a reversible and focal way for neuroplasticity modulation (Nitsche and Paulus, 2001, 2000). tDCS has been shown to modulate activity in both the motor and visual cortices and more recently has been shown to directly influence the excitability of the spinal cord (Zaghi et al., 2010; Butler et al., 2013; Elsner et al., 2018). Furthermore, tDCS has been reported to have a long-term effect lasting beyond the time of stimulation which might indicate the ability to improve motor function through network reorganization and modulation of brain plasticity (Reidler et al., 2011; Allman et al., 2016; Kashiwagi et al., 2018; Liu et al., 2018). Although the mechanism of tDCS has not been fully revealed yet, converging evidence demonstrated the efficacy of tDCS for motor function improvements in stroke (Allman et al., 2016; Butler et al., 2013; Elsner et al., 2018; Hordacre et al., 2018; Lindenberg et al., 2010). Given the benefits in motor function recovery from both MI-BCI and tDCS, it is unclear whether the combination of MI-BCI and tDCS would further enhance functional motor recovery in post-stroke rehabilitation. The underlying neural mechanisms of combined MI-BCI and tDCS are also largely unknown.
Resting-state functional magnetic resonance imaging (rs-fMRI) provides an indirect measure of neuronal activity and connectivity based on regional blood-oxygen-level-dependent (BOLD) signal fluctuations without any imposed task (Biswal et al., 1995). Various analytic methods have been proposed to derive complementary measures of brain functional dynamics from rs-fMRI data (Lv et al., 2018). Amplitude of Low-Frequency Fluctuation (ALFF) measures the total power of the BOLD signal within the low-frequency range between 0.01 and 0.1 Hz, which represents local brain activity (Zang et al., 2007). Regional Homogeneity (ReHo) is a voxel-based measure of the similarity between the time-series of a given voxel and its nearest neighbors, which represents local connectivity as calculated by the Kendall coefficient of concordance of the BOLD time-series (Zang et al., 2004). Seed-based functional connectivity (FC) approach measures the temporal correlations between the activity in a seed region and BOLD signals across the brain (Biswal et al., 1995). Canonical functional networks such as the default mode network (DMN) and the executive control network (ECN) have been consistently derived from FC mapping in rs-fMRI (Ng et al., 2016; Chong et al., 2019, 2017a; Cheng et al., 2021). These measurements provide important insights into the brain functional dynamics, disease mechanisms, and brain-behavior associations (Wang et al., 2016a; Chong et al., 2017b; Puig et al., 2018; Zhao et al., 2018; Chen et al., 2019; Zhang et al., 2020). The combination of different measurements incorporates both regional and global information and thus complements each other to build an efficient model for brain analysis.
Nevertheless, little work has been done to examine the brain functional changes in stroke after MI-BCI and tDCS-based intervention. Most of the previous work focused on cross-sectional brain ALFF/ReHo/FC changes in stroke patients in comparison with healthy controls. In terms of regional activity, some studies reported lower ALFF in the motor cortex and lower ALFF/ReHo in the default mode network (DMN) in stroke patients compared to healthy controls (Tsai et al., 2014; Zhu et al., 2015; La et al., 2016) while others reported no differences or contradictory results in the DMN (Liu et al., 2015). On the other hand, disrupted FC in the motor cortex and DMN were widely reported in stroke patients with motor function impairment and cognitive impairment (Park et al., 2011, 2014; Golestani et al., 2013; Tuladhar et al., 2013; Ding et al., 2014; De Bruyn et al., 2018; Puig et al., 2018; Lee et al., 2019). Among the limited work on brain functional changes post-intervention in stroke, emerging evidence demonstrated enhancement of FC in the motor and sensory-related networks after motor imagery training (Sun et al., 2013; Wang et al., 2018). To our knowledge, no study has directly compared the brain functional changes between stroke rehabilitation using MI-BCI and tDCS and how these brain measures relate to clinical outcomes such as motor recovery.
In this study, we examined the alterations in brain functional activity and connectivity in chronic subcortical stroke patients using ALFF, ReHo, and FC. The changes in the neural function of stroke patients pre- and post-intervention were investigated in the MI-BCI only group and MI-BCI combined with tDCS group separately. We hypothesized that 1) lowered ALFF/ReHo and disrupted FC in the motor cortex and DMN would be observed in stroke patients compared to healthy controls at baseline; 2) stroke patients would have higher ALFF/ReHo/FC in the motor cortex and DMN post-intervention, the degree of which would differ between the MI-BCI and the MI-BCI + tDCS groups. Lastly, we investigated whether these alterations in ALFF/ReHo/FC post-intervention would correlate with motor function recovery measured by Fugl-Meyer Assessment (FMA) scores.
Materials and Methods
Participants
Forty-two stroke patients aged from 21 to 70 years with moderate to severe motor impairments and 11 age-matched healthy controls were recruited in this study. The inclusion criteria for stroke patients were (1) first-ever subcortical stroke > 9 months before the study enrollment; and (2) unilateral moderate to severe upper extremity motor impairment measured by the upper extremity component of the FMA (score range 11–45). The exclusion criteria for stroke patients were (1) epilepsy, neglect, cognitive impairment, and other neurological or psychiatric diseases; (2) severe arm pain; (3) severe spasticity measured by the Modified Ashworth Scale in the shoulder or elbow (score > 2); (4) contraindications to tDCS; (5) weak grip strength measured by a dynamometer (weight < 10 kg); and (6) participation in other interventions or trials that target post-stroke motor recovery. Twenty-three stroke patients were excluded because they either declined to participate in the study or failed to meet the inclusion or BCI performance criteria. Detailed demographics of the remaining 19 patients and 11 healthy controls who completed the training and scans were provided in Table 1. This study was conducted in accordance with the Code of Ethics of the World Medical Association and approved by the National Healthcare Group Domain-Specific Review Board. It was also registered on ClinicalTrials.gov (Clinical Trial Registration Unique Identifier: NCT01897025, date of registration: July 8, 2013; https://clinicaltrials.gov/ct2/show/NCT01897025). Written inform consent was obtained from all participants.
MI-BCI and tDCS Study Design
Nineteen stroke patients were randomized into the MI-BCI + tDCS group (n = 10) and MI-BCI group (n = 9) with matching pre-training FMA scores. The MI-BCI + tDCS group received 20-min real tDCS, in which direct current was applied using a saline-soaked pair of surface sponge electrodes at an intensity of 1 mA with the anode placed over the M1 motor cortex of the ipsilesional hemisphere and the cathode placed over the contralesional M1 while the MI-BCI group received 20-min sham tDCS, in which current ramped up and down to give subjects the sensation of the stimulation. Both groups completed 10 sessions of MI-BCI training within 2 weeks. Each MI-BCI training was 40 min and consisted of 160 trials. In each trial, subjects were prepared with a visual cue and instructed to perform motor imagery by an instruction cue. The EEG segment of 0.5– 4.5 s from the instruction cue was then extracted to classify the EEG segment to perform online detection of MI or the idle condition using the Filter Bank Common Spatial Pattern (FBCSP) algorithm (Ang et al., 2012). The MIT-Manus robot provided movement feedback if the motor imagery was detected. More details of the study design can be found in Supplementary Materials and Ang and Guan (2015).
Motor function was assessed by the upper extremity component of the FMA, which is a stroke-specific, performance-based impairment index to assess motor functioning, balance, sensation and joint functioning in patients with post-stroke hemiplegia (Fugl-Meyer et al., 1975). Motor function assessments were conducted (1) 1 week before training, (2) immediately after training, and (3) 4 weeks after training.
Image Acquisition
All stroke patients completed three MRI scans: at screening, 1 week before training, and 4 weeks after training. Healthy controls underwent two MRI scans: at screening and 3 weeks after screening. The MRI scans conducted 1 week before training for patients and 3 weeks after screening for healthy controls were considered as baseline. For patients, the MRI scans conducted 1 week before training and 4 weeks after training were regarded as pre- and post-intervention respectively.
MRI data were collected using a 3T scanner (TIM Trio, Siemens, Germany) with a 32-channel head array coil. The scan protocol included a T1-weighted magnetization prepared rapid gradient-echo (MPRAGE) sequence (inversion time = 900 ms, repetition time = 1,900 ms, echo time = 2.5 ms, flip angle = 9°, field of view = 256 × 256 mm2, 176 sagittal slices, matrix size = 256 × 256, and voxel size = 1 mm3 isotropic), and a resting state T2∗-weighted echo planar imaging (repetition time = 1,750 ms, echo time = 30 ms, flip angle = 74°, field of view = 220 × 220 mm2, voxel size = 3.4 mm3 isotropic, slice thickness = 3.4 mm, 33 axial slices). During the 7-min rs-fMRI, participants were instructed to remain relaxed and awake with their eyes closed and not engage in any specific activity.
Image Analyses
fMRI Preprocessing
Preprocessing of fMRI images was performed using FMRIB software library (FSL) and analysis of functional neuroimaging (AFNI) following our standard protocol (Cox, 1996; Ng et al., 2016; Wang et al., 2016b; Chong et al., 2017a). The preprocessing steps consist of: (i) removal of first six volumes for magnetic field stabilization; (ii) slice-time correction; (iii) motion correction, (iv) despiking; (v) spatial smoothing (6-mm FWHM Gaussian kernel); (vi) grand mean scaling; (vii) band-pass temporal filtering (0.009 – 0.1 Hz); (viii) detrending (removal of first and second order); (ix) use of Boundary-Based Registration (BBR) for co-registration of T1 image (Greve and Fischl, 2009) and non-linear registration tool (FNIRT) for subsequent registration to the Montreal Neurological Institute (MNI) 152 space. Specifically, the left hemisphere was defined as the ipsilesional hemisphere and all images with lesions at the right hemisphere were flipped to investigate the effect of lesion location. Additionally, regression of nuisance signals (white matter, cerebrospinal fluid (CSF), whole-brain global signals, and six motion parameters) and motion scrubbing were performed for analysis of FC to avoid spurious connectivity (Power et al., 2012; Power et al., 2014).
Quality of co-registration and normalization were visually inspected. One post-intervention image with movement more than 4 mm was discarded. Thus after quality control, data of 19 stroke patients and 11 healthy control remained at baseline. Data of 10 subjects in the MI-BCI + tDCS group and 8 subjects in the MI-BCI group remained for pre- and post-intervention analysis.
Derivation of the Voxelwise Amplitude of Low Frequency Fluctuation Maps
The ALFF was calculated using a toolbox for Data Processing & Analysis for Brain Imaging (DPABI) (Yan et al., 2016). For each voxel, a discrete Fourier transform was performed on the resting-state time series. The ALFF was computed by measuring the average square root of the total power spectrum between 0.01 and 0.10 Hz on a voxel-by-voxel basis.
Derivation of Voxelwise Regional Homogeneity Maps
The ReHo maps were also produced using DPABI toolbox. ReHo was calculated in a voxel-wise manner using Kendall’s concordance coefficient (KCC) across the time series of a centered cubic cluster of 27 voxels. A large ReHo value for a given voxel indicates a high local synchronization of rs-fMRI signal among neighboring voxels and vice versa.
Derivation of Default Mode and Somatomotor Network Functional Connectivity
FC analysis was performed for two networks, default mode network and somatomotor network, which were previously reported to show changes in ALFF and ReHo analysis in our study. DMN and SMN were defined using Region of Interest (ROI) from a FC-based 114-node parcellation (Buckner et al., 2011). 24 ROIs were included for DMN and 10 ROIs were included for SMN (ROI names and peak coordinates in Supplementary Table 1). BOLD time series of each ROI were extracted for every subject and Pearson’s correlation between the mean time series of every pair of ROIs were computed. An FC matrix was produced for every subject by Fisher’s r-to-z transformation of correlation coefficients. FC within the DMN and SMN, as well as between the DMN and SMN were consequently derived by averaging the corresponding cells in the matrix.
Statistical Analysis
Baseline differences in ALFF, ReHo, and FC between stroke patients and healthy controls were examined using two-sample t-tests. Voxelwise analyses (i.e., ReHo and ALFF) were thresholded using a height threshold of p < 0.01 (uncorrected) and extent threshold of p < 0.05 (familywise-error (FWE) corrected).
To examine the effects of intervention as well as interaction effects between intervention and group on FMA scores, ALFF and ReHo maps, we conducted mixed-effect Analyses of Variance (ANOVA) with intervention as within-subjects factor and group as between-subject factor. Post hoc tests were subsequently performed to examine intervention effects in each group separately. Voxelwise analyses (i.e., ReHo and ALFF) were thresholded using a height threshold of p < 0.01 (uncorrected) and extent threshold of p < 0.05 (FWE corrected).
Finally, associations between functional activity/connectivity and clinical outcomes (motor function improvement measured by FMA scores) were examined using Pearson’s correlation. Eight-mm spheres created in MARSBAR (Brett et al., 2002) with peak coordinates extracted from the above interaction effect maps were selected as ROIs for ALFF and ReHo. ALFF/ReHo changes in the ROIs were correlated with changes in FMA. FC changes within DMN/SMN and between DMN and SMN were associated with changes in FMA.
Results
Improved Motor Function After Stroke Rehabilitation
There was no difference between the MI-BCI group and MI-BCI + tDCS group at baseline in terms of age, post-stroke time, or FMA scores (Table 1). A 3 (intervention: baseline, immediately after training, 4 weeks after training) × 2 (group: MI-BCI, MI-BCI + tDCS) mixed effects ANOVA performed on FMA scores indicated significant intervention effect (p = 0.002) but no interaction effect between intervention and group (p = 0.450), suggesting that both groups gained significant motor function improvement post-intervention with no group difference observed either immediately or 4 weeks after training. Post hoc tests further showed that significant FMA gains compared to baseline were only found at 4 weeks after training (MI-BCI group: 5.8 ± 6.0, p = 0.030, effect size = 0.62; MI-BCI + tDCS group: 5.0 ± 4.4, p = 0.006, effect size = 0.64) but not immediately post-training (MI-BCI group: 2.9 ± 4.2,p = 0.096, effect size = 0.32; MI-BCI + tDCS group: 0.9 ± 3.0, p = 0.362, effect size = 0.11) (Figure 1). Furthermore, the MI-BCI group showed greater motor function improvement than the MI-BCI + tDCS group at week 2, although this was not statistically significant (MI-BCI group: 2.9 ± 4.2 vs. MI-BCI + tDCS group: 0.9 ± 3.0, p = 0.130, effect size = 0.55).
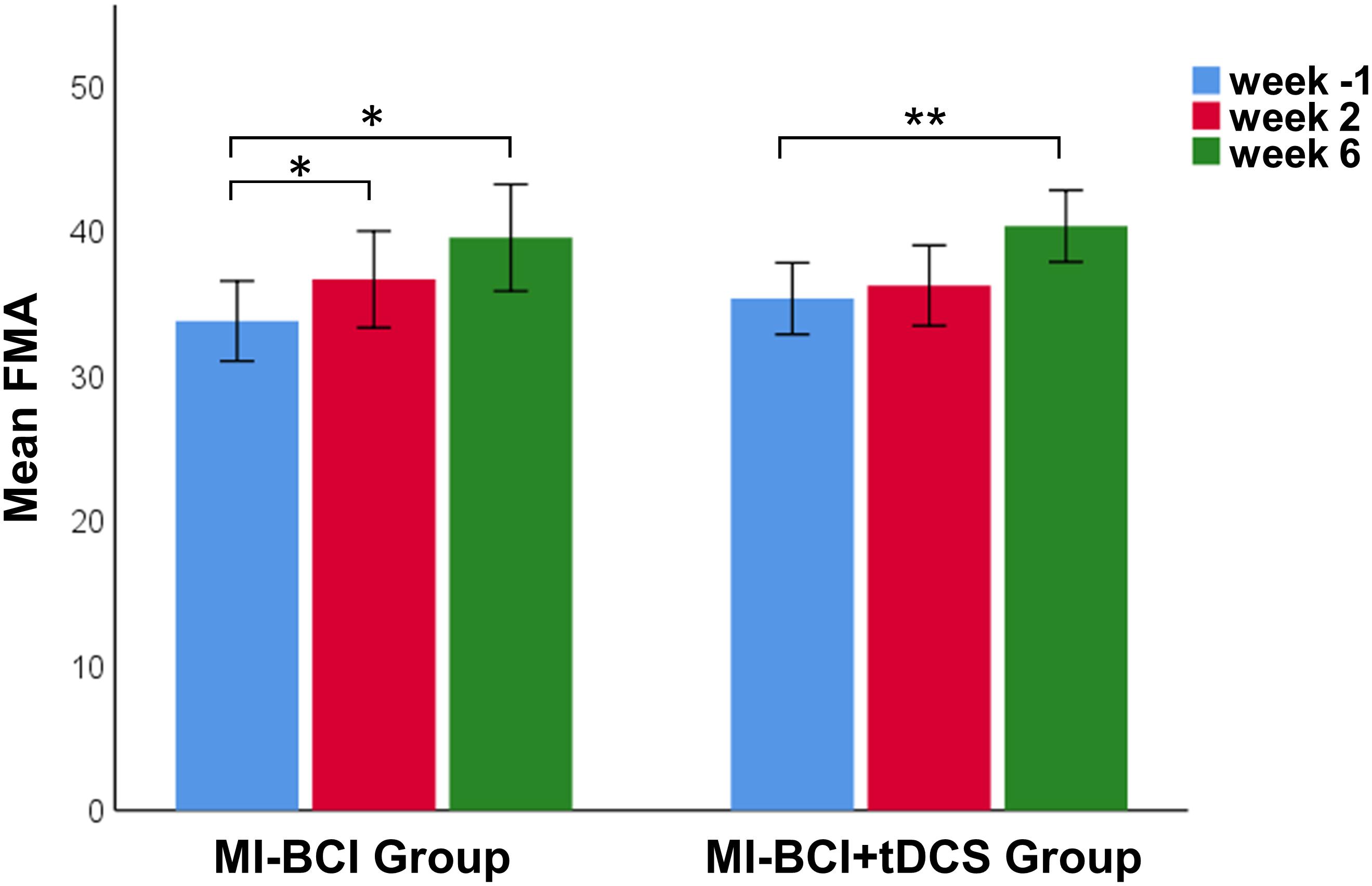
Figure 1. Motor recovery after MI-BCI intervention with and without tDCS. FMA scores at baseline and post intervention. Significant FMA gains comparing to baseline were only found at 4 weeks after training for both MI-BCI and MI-BCI + tDCS group (MI-BCI group: 5.8 ± 6.0, p = 0.030; MI-BCI + tDCS group: 5.0 ± 4.4, p = 0.006) but not immediately post training (MI-BCI group: 2.9 ± 4.2, p = 0.096; MI-BCI + tDCS group: 0.9 ± 3.0, p = 0.362). No significant intergroup differences were observed at any time point during the study. **p < 0.01; *p < 0.05. Error bars indicate ± 1SE.
Abnormal ALFF/ReHo in the SMN and DMN in Stroke Patients at Baseline
Cross-sectional comparisons conducted at baseline indicated abnormal ALFF and ReHo patterns in stroke patients compared to healthy controls. Stroke patients showed lower ALFF in the ipsilesional precentral gyrus and postcentral gyrus and higher ALFF in the precuneus, posterior cingulate gyrus, angular gyrus, middle frontal gyrus, and contralesional insula (Figure 2A). Thus, these findings suggest that stroke patients had lower ALFF in ipsilesional SMN and higher ALFF in the DMN and contralesional SAN compared to healthy controls.
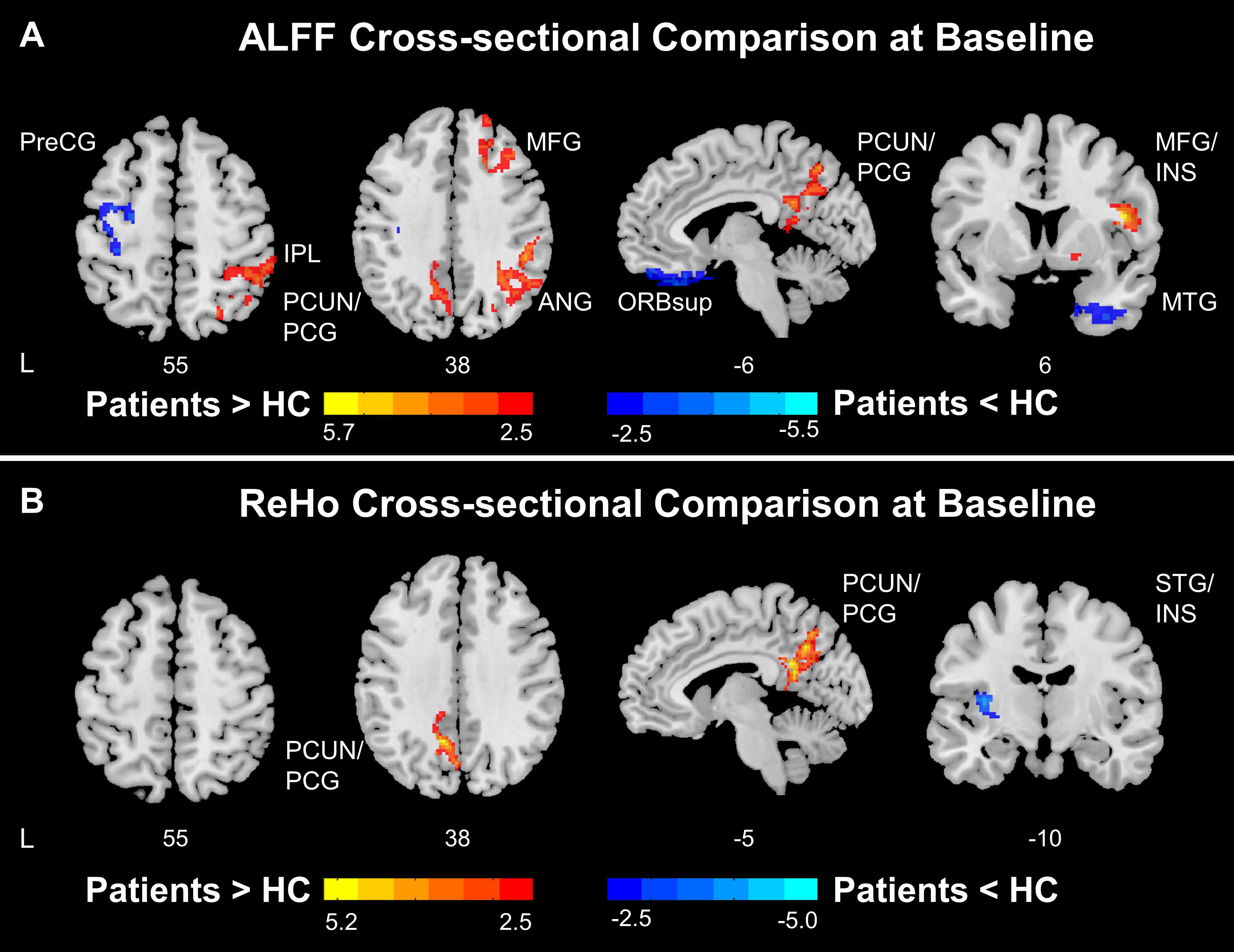
Figure 2. Functional disruptions in stroke patients. (A) ALFF comparison between HC and patients at baseline. At baseline, stroke patients showed reduced ALFF at ipsilesional SMN and increased ALFF at DMN and salience network comparing to healthy controls. (B) ReHo comparison between HC and patients at baseline. At baseline, stroke patients showed increased ReHo at DMN and decreased ReHo at insula comparing to healthy controls. Color bars indicate t-scores. L, Ipsilesional Hemisphere; DMN, Default Mode Network; SMN, Somatomotor Network; HC, Healthy Control; Precg, Precentral Gyrus; SMA, Supplementary Motor Area; IPL, Inferior Parietal; PCUN, Precuneus; PCG, Posterior Cingulate Gyrus; MFG, Middle Frontal Gyrus; ANG, Angular Gyrus; ORBsup, Superior Frontal Gyrus, Orbital Part; INS, Insula; MTG, Middle Temporal Gyrus.
Stroke patients had higher ReHo in the precuneus and posterior cingulate gyrus and lower ReHo in the ipsilesional superior temporal gyrus and insula (Figure 2B). Collectively, the results indicate that stroke patients had higher ReHo in the DMN and lower ReHo in the ipsilesional SAN compared to healthy controls.
Differential Changes in ALFF/ReHo in the MI-BCI and MI-BCI + tDCS Groups Post-intervention
Significant interaction effects between intervention (pre-training, post-training) and group (MI-BCI group, MI-BCI + tDCS group) were found in ALFF and ReHo based on mixed-effect ANOVA, suggesting differential brain functional activity and connectivity changes in the MI-BCI group and MI-BCI + tDCS group. Specifically, differential ALFF patterns post-intervention were found in the contralesional regions within the DMN and SMN, including supplementary motor area, contralesional precentral gyrus, middle frontal gyrus, precuneus, and angular gyrus (Figure 3A). On the other hand, differential ReHo patterns were found in the precuneus and posterior cingulate gyrus (Figure 4A), suggesting that the MI-BCI group and MI-BCI + tDCS group exhibited differential ReHo patterns in the DMN post-intervention.
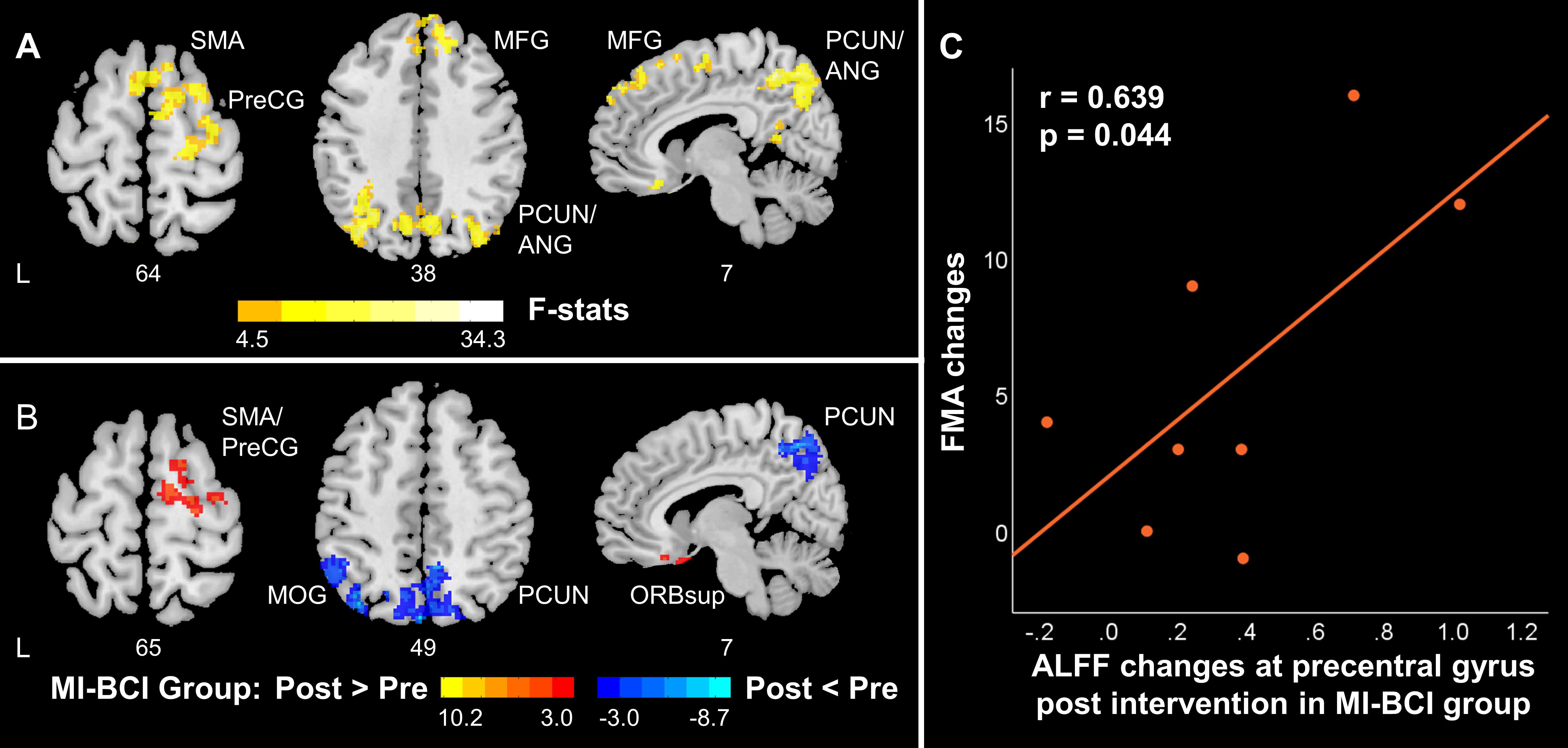
Figure 3. ALFF changes in contralesional SMN and posterior DMN in stroke patients along with motor function recovery. (A) The interaction between time and group. The MI-BCI group and MI-BCI + tDCS group showed divergent intervention effects at contralesional SMN and DMN. (B) Intervention effect in MI-BCI group. MI-BCI group showed increased ALFF at contralesional SMN and decreased ALFF at DMN. No such effect was observed in the MI-BCI + tDCS group. (C) Correlation between FMA changes and ALFF changes in the MI-BCI group. A positive correlation was observed between FMA changes and ALFF changes post intervention in the MI-BCI group at the precentral gyrus. No such correlation was observed in the MI-BCI + tDCS group. L, Ipsilesional Hemisphere; DMN, Default Mode Network; SMN, Somatomotor Network: HC, Healthy Control; Precg, Precentral Gyrus; SMA, Supplementary Motor Area; PCUN, Precuneus; PCG, Posterior Cingulate Gyrus; MFG, Middle Frontal Gyrus; ANG, Angular Gyrus; INS, Insula; MTG, Middle Temporal Gyrus.
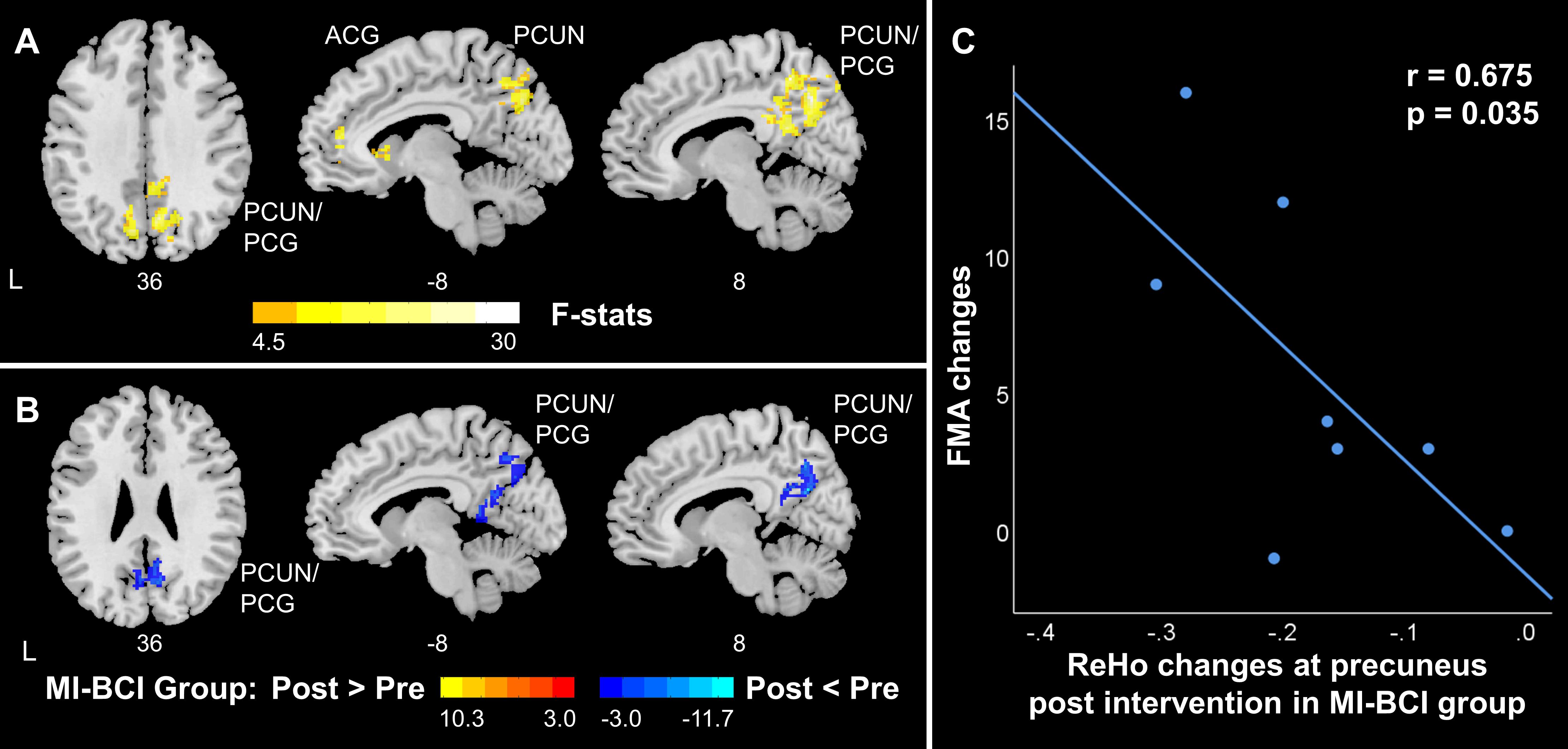
Figure 4. ReHo changes in DMN in stroke patients along with motor function recovery. (A) The interaction effect between group and time. The MI-BCI group and MI-BCI + tDCS group showed divergent intervention effects at DMN. (B) The intervention effect in the MI-BCI group. The MI-BCI group showed decreased ReHo at DMN. No such effect was observed in the MI-BCI + tDCS group. (C) Correlation between FMA changes and ReHo changes in MI-BCI group. A negative correlation was observed between FMA changes and ReHo changes post intervention in the MI-BCI group at precuneus. No such correlation was observed in the MI-BCI + tDCS group. L, Ipsilesional Hemisphere; DMN, Default Mode Network; PCUN, Precuneus; PCG, Posterior Cingulate Gyrus; ACG, Anterior cingulate and paracingulate gyri.
Post hoc analyses revealed that the MI-BCI group showed increased ALFF in the contralesional supplementary motor area and precentral gyrus post-intervention and decreased ALFF in the precuneus, inferior parietal lobule, and middle occipital gyrus (Figure 3B). The regions that showed changes post-intervention were very similar to regions that showed abnormal ALFF comparing to healthy controls at baseline (Figure 2A). Increased ALFF at baseline in the DMN was reduced post-intervention. Decreased ALFF in the ipsilesional SMN at baseline were not increased after training but increased ALFF was observed in the contralesional SMN. Similarly, decreased ReHo at bilateral precuneus and posterior cingulate gyrus was observed in the MI-BCI group post-intervention (Figure 4B). The altered regions were identical to the regions that showed abnormal ReHo changes in stroke patients at baseline comparing to healthy controls (Figure 2B). Higher ReHo in the DMN at baseline was reduced post-intervention.
In contrast, the MI-BCI with tDCS group had no significant changes in ALFF/ReHo post-intervention.
Functional Connectivity Changes Between the SMN and DMN
Given the above ALFF/ReHo patterns mainly in the SMN and DMN, we then investigated if the FC in these two networks was disrupted in stroke patients and whether there was any intervention effect. As expected, stroke patients showed lower FC within the SMN at baseline compared to healthy controls (p = 0.03, effect size = 0.93) (Figure 5A) while no differences were found for FC within the DMN and FC between the SMN and DMN. Furthermore, no intervention effect (interaction or post hoc effects) was found.
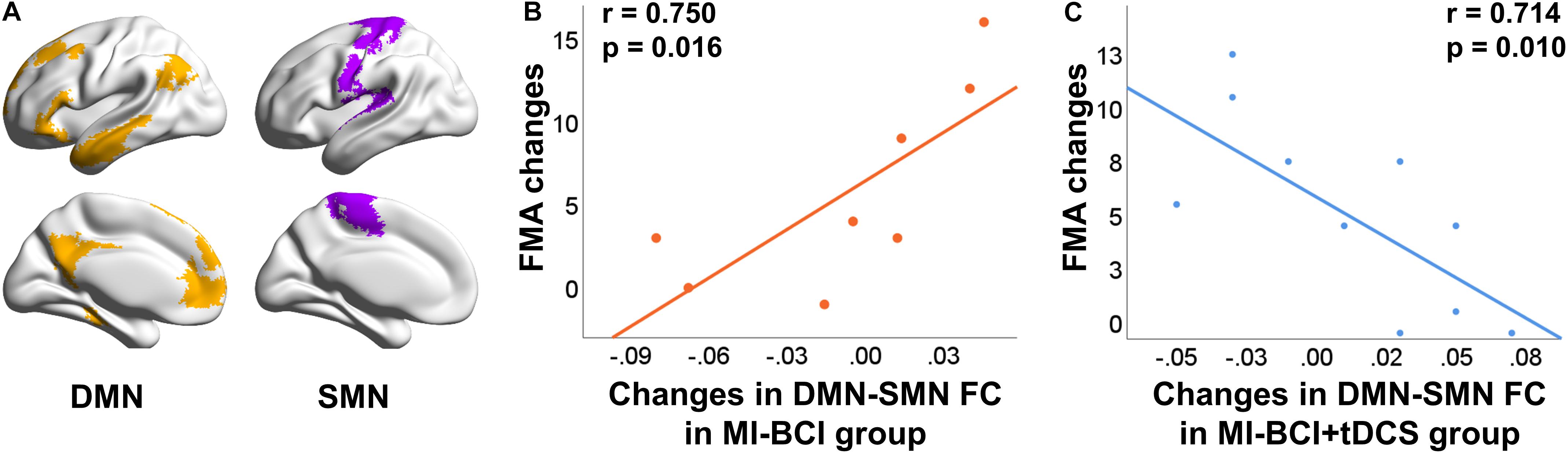
Figure 5. Differential correlation between FC changes and behavioral outcome changes using different intervention methods. (A) Stroke patients showed lower FC at SMN comparing to healthy controls at baseline (p = 0.030). (B) Correlation between FC changes and FMA changes in MI-BCI group. A positive correlation was observed between FMA changes and interconnectivity changes of DMN and SMN. (C) Correlation between FC changes and FMA changes in MI-BCI + tDCS group. A negative correlation was observed between FMA changes and interconnectivity changes of DMN and SMN.
Association Between Brain Functional Measures and Motor Function Improvement
To find the association between changes in brain functional activity/connectivity and motor function post-intervention, we investigated the correlation between the ALFF/ReHo/FC changes and FMA changes.
A positive correlation (r = 0.639, p = 0.044) between ALFF changes in the precentral gyrus and FMA changes was observed in the MI-BCI group (Figure 3C), which indicated that patients who obtained higher ALFF increase post-intervention gained greater motor function improvement.
A negative correlation (r = −0.675, p = 0.035) between ReHo changes at precuneus and FMA changes was found in the MI-BCI group (Figure 4C). The association between decreased ReHo changes and increased FMA changes indicated that patients who had larger ReHo reduction gained greater motor function improvement.
Differential associations of the FC changes between SMN and DMN and FMA changes were observed in the MI-BCI group and MI-BCI + tDCS group. We found a positive correlation (r = 0.750, p = 0.016) between inter SMN-DMN FC changes and FMA changes in the MI-BCI group (Figure 5B) while a negative correlation (r = −0.714, p = 0.010) was reported in the MI-BCI + tDCS group (Figure 5C). The contradictory association in the two groups indicated that patients who obtained higher SMN-DMN FC increase would gain greater motor function improvement in the MI-BCI group while stroke patients in the MI-BCI + tDCS group would gain lower motor function improvement.
Discussion
This study investigated the brain functional activity and connectivity changes in chronic subcortical stroke patients pre- and post-intervention with MI-BCI and tDCS. We found that stroke patients had lower ALFF in the ipsilesional SMN at baseline and had increased ALFF in the contralesional SMN in the MI-BCI group, providing new evidence for the compensation effect. Furthermore, the higher ALFF/ReHo in the DMN at baseline were decreased after MI-BCI training, illustrating the re-normalization of brain activity and connectivity post-intervention. More importantly, the divergent associations between SMN-DMN FC changes and FMA changes in the MI-BCI group and MI-BCI + tDCS group suggested differential mechanisms in the two approaches.
Compensation Effect of the Contralesional SMN After MI-BCI
The ALFF findings suggest the possible beneficial effect of brain activity in the contralesional motor cortex after MI-BCI-based intervention. Stroke patients had lower ALFF in the ipsilesional SMN at baseline; and then the MI-BCI group showed increased ALFF in the contralesional SMN post-intervention. Importantly, increased regional brain activity in the contralesional motor cortex was positively associated with motor function improvement, suggesting a compensation effect of the contralesional SMN.
A predominant hypothesis of interhemispheric interaction is that the motor cortex of one hemisphere inhibits the contralateral homolog (Ferbert et al., 1992; Gerloff et al., 1998; Di Lazzaro et al., 1999; Liepert et al., 2000; Lotze et al., 2006; Dancause et al., 2015; Lüdemann-Podubecká et al., 2016). In line with this hypothesis, studies have reported persistent contralesional sensorimotor cortex hyperexcitability in patients post-stroke (Liepert et al., 2000; Bütefisch et al., 2003). Such hyperexcitability in the contralesional hemisphere might be harmful to recovery as it inhibits the excitability of the ipsilesional hemisphere (Loubinoux et al., 2003; Manganotti et al., 2008). Repetitive transcranial magnetic stimulation (rTMS) and tDCS have also been employed to inhibit the contralesional motor cortex to improve motor function recovery (Takeuchi et al., 2005; Nowak et al., 2008; Song et al., 2014; Matsuura et al., 2015; Lüdemann-Podubecká et al., 2016).
In parallel, recent BCI-related researches have shown contradictory results against the predominant hypothesis. Patients were reported to gain significant motor function improvement by utilizing the contralesional motor cortex in BCI therapy (Song et al., 2014; Young et al., 2014; Bundy et al., 2017). Although the role of the contralesional motor cortex is still unclear, evidence showed that the contralesional motor cortex excitability is positively correlated with recovery from stroke in chronic stroke patients (Lotze et al., 2006; Schaechter and Perdue, 2008; Young et al., 2016). One hypothesis on the function of the contralesional motor cortex is that it may provide additional neural resources for compensation of ipsilesional damages (Nowak et al., 2009; Butler et al., 2013; Touvykine et al., 2016). Our findings provide new evidence supporting the compensation effect of the contralesional SMN after MI-BCI stroke rehabilitation. Our previous study reported cortical activity changes measured by transcranial magnetic stimulation in the contralesional primary motor cortex were associated with functional improvement, also suggesting a possible role for the contralesional primary motor cortex in stroke recovery (Chew et al., 2020). Nevertheless, more effort should be made to study the role of the contralesional motor cortex in stroke rehabilitation, which could possibly depend on lesion size, stroke type, stroke stage, and BCI experiment design (Buetefisch, 2015; Dodd et al., 2017).
Re-normalization of Brain Activity and Connectivity After MI-BCI
Stroke patients showed lower ALFF/FC in SMN and higher ALFF/ReHo in the DMN compared to healthy controls at baseline. Post-intervention, the MI-BCI group showed increased ALFF in the contralesional SMN and decreased ALFF/ReHo in the DMN. Our results match with previous findings on alterations of brain activity and connectivity in the DMN and the brain re-normalization hypothesis.
Alterations of brain activity and connectivity in the DMN were widely reported in stroke patients with impaired cognitive function (Tuladhar et al., 2013; Ding et al., 2014; Liu et al., 2014; Park et al., 2014; Wang J. et al., 2019). Stroke patients had significantly lower FC in the key DMN regions, namely precuneus and posterior cingulate gyrus compared to healthy controls (Tuladhar et al., 2013; Ding et al., 2014; Wang J. et al., 2019). Reduced ALFF and ReHo in the DMN were reported in stroke patients with early impairment in consciousness (Tsai et al., 2014). Our results showed higher ALFF and ReHo in the DMN in stroke patients at baseline compared to healthy controls. The difference might be due to different function impairments (cognitive impairment vs. motor function impairment) and different stroke stages (acute/subacute vs. chronic). A possible explanation for higher brain activity and connectivity in the DMN is the compensatory effect for motor function. More resources were required to achieve normal motor function due to the ipsilesional SMN decline, resulting in higher DMN activity in patients than healthy controls as compensation for declined SMN function (Pan et al., 2017).
More importantly, re-normalization of the brain activity and connectivity were observed post-intervention. The higher ALFF and ReHo in the precuneus and posterior cingulate gyrus at baseline reduced post-intervention in the MI-BCI group, which refers to re-normalization of the local activity and connectivity. Brain re-normalization in previous studies focused on alterations in FC. Increased FC after BCI rehabilitation therapy in the SMN and DMN where FC was lower at baseline was reported, which also hints at re-normalization of brain connectivity (Park et al., 2014; Tsuchimoto et al., 2019; Wang J. et al., 2019).
Differential Mechanisms of MI-BCI and MI-BCI + tDCS
The efficacy of MI-BCI on improving motor function in stroke rehabilitation has been widely reported (Ang et al., 2009; Teo and Chew, 2014; Ang and Guan, 2015; Pichiorri et al., 2015; Ramos-Murguialday et al., 2019; Foong et al., 2020). Studies on tDCS also illustrated the beneficial effects of non-invasive cortical stimulation on stroke rehabilitation (Nitsche and Paulus, 2001; Zaghi et al., 2010; Madhavan et al., 2011; Allman et al., 2016; Elsner et al., 2018; Kashiwagi et al., 2018; Liu et al., 2018). However, the combination of MI-BCI and tDCS in this study did not further improve motor function than MI-BCI therapy without tDCS. Both the MI-BCI group and MI-BCI + tDCS group gained significant motor function improvement with no group differences reported regarding FMA scores. The differential patterns of brain activity and connectivity post-intervention and the divergent associations between these brain measures and FMA in the two groups underscored differential mechanisms underlying MI-BCI and tDCS. The MI-BCI group showed increased ALFF in the contralesional SMN and reduced ALFF/ReHo in the DMN post-intervention while no significant neuroimaging findings were observed in the MI-BCI + tDCS group. Furthermore, contrasting correlations were found between FC changes and clinical outcome changes in the MI-BCI group and MI-BCI + tDCS group. Our results indicated that MI-BCI and tDCS had potentially divergent ways of modulating functional brain activity. MI-BCI system enables stroke patients to move the impaired limb driven by a robotic arm via neural signals from the motor imagery of the arm and provides multisensory feedback to patients to enhance motor learning and recovery (Ang et al., 2014, 2009). tDCS modulates activity in both the motor and visual cortices and directly influences the excitability of the spinal cord (Nitsche and Paulus, 2001; Elsner et al., 2018). Generally, anodal stimulation increases cortical excitability, while cathodal stimulation decreases it. We suspect that the inhibition effect of cathodal might prevent or reverse the brain activity/connectivity changes in the MI-BCI + tDCS group, leading to insignificant results compared to MI-BCI only group and contradictory correlation with FMA scores. Consistent with our findings, another study reported that white matter integrity in the ipsilesional corticospinal tract and bilateral corpus callosum was increased but sensorimotor cerebral blood flow (CBF) was decreased, while in the MI-BCI group contradictory results or insignificant results were reported (Hong et al., 2017). Quantitative electroencephalographic (Q-EEG) features which changed significantly during these interventions were also mutually exclusive for MI-BCI and MI-BCI + tDCS group. The relative theta power was observed to be the signature monitory biomarker for BCI intervention whereas the tDCS group was characterized by a change in brain symmetry index, indicating distinct mechanisms of neuronal repair of the two approaches (Mane et al., 2019). The detailed explanation of changes in the brain requires further understanding of both MI-BCI and tDCS mechanisms. Our results suggest that a combined rehabilitation strategy should be designed considering not only the improvement of clinical outcomes but also changes in the brain.
Limitations and Future Work
This is a pilot study of stroke rehabilitation involving complex multimodal design of neuroimaging, MI-BCI and tDCS, which is limited by the small sample size. Due to the limited sample, we were not able to control for stroke severity and lesion type or location in our analysis. Also, the possible inter-variability in conventional occupational therapy was not taken into account. Future research with large sample size should incorporate these potential important factors to evaluate the long-term rehabilitation effect. Another limitation is the lack of a tDCS-only group which would help to understand the mechanism of tDCS to facilitate the development of stroke rehabilitation strategies. Although the tDCS stimulation parameters applied in this study follow the standard protocol, it would be to study how stimulation parameters such as sites and placement of electrodes could impact the efficiency. Furthermore, while the current study focused on subcortical chronic stroke patients, it is crucial to design effective rehabilitations for stroke patients in acute or subacute phase moving forward.
Conclusion
In conclusion, patients with chronic subcortical stroke showed lower ALFF/FC in the SMN and higher ALFF/ReHo in the DMN compared to controls at baseline. Such brain functional vulnerability patterns in the two networks was partially re-normalized post-intervention in the MI-BCI group while the MI-BCI + tDCS group showed no significant changes. Divergent associations between SMN-DMN FC changes and FMA changes were found in the MI-BCI group and MI-BCI + tDCS group. Our results suggested the potential differential or even opposite network-specific brain functional mechanisms underlying MI-BCI with and without tDCS in stroke recovery.
Data Availability Statement
The datasets generated during and/or analyzed during the current study are available from the corresponding author upon reasonable request.
Ethics Statement
The studies involving human participants were reviewed and approved by the National Healthcare Group Domain-Specific Review Board. The patients/participants provided their written informed consent to participate in this study.
Author Contributions
MH codesigned the study with JZ, EC, and CG performed all the data analysis, and prepared the manuscript. H-JC, FJ, and JC assisted with data preprocessing and manuscript revision. JZ, CG, and XJ supervised and revised the manuscript. EC provided the data. ZL, WH, KA, KP, and H-JC assisted in data collection and preparation. All authors reviewed the manuscript.
Funding
This research was supported by the National Medical Research Council NMRC0088/2015 and the Duke-NUS Medical School Signature Research Program funded by Ministry of Health, Singapore (JZ) and National Medical Research Council NMRC/NIG/1013/2010 (EC). The work was supported by NTU Institute for Health Technologies, Interdisciplinary Graduate Programme, Nanyang Technological University, Singapore.
Conflict of Interest
The authors declare that the research was conducted in the absence of any commercial or financial relationships that could be construed as a potential conflict of interest.
Supplementary Material
The Supplementary Material for this article can be found online at: https://www.frontiersin.org/articles/10.3389/fnhum.2021.692304/full#supplementary-material
References
Allman, C., Amadi, U., Winkler, A. M., Wilkins, L., Filippini, N., Kischka, U., et al. (2016). Ipsilesional anodal tDCS enhances the functional benefits of rehabilitation in patients after stroke. Sci. Transl. Med. 8:330re1. doi: 10.1126/scitranslmed.aad5651
Ang, K. K., Chin, Z. Y., Wang, C., Guan, C., and Zhang, H. (2012). Filter bank common spatial pattern algorithm on BCI competition IV datasets 2a and 2b. Front. Neurosci. 6:39. doi: 10.3389/fnins.2012.00039
Ang, K. K., and Guan, C. (2015). Brain-computer interface for neurorehabilitation of upper limb after stroke. Proc. IEEE 103, 944–953. doi: 10.1109/JPROC.2015.2415800
Ang, K. K., Guan, C., Chua, K. S. G., Ang, B. T., Kuah, C., Wang, C., et al. (2009). “A clinical study of motor imagery-based brain-computer interface for upper limb robotic rehabilitation,” in Proceedings of the 31st Annual International Conference of the IEEE Engineering in Medicine and Biology Society, Minneapolis, MN, 5981–5984. doi: 10.1109/IEMBS.2009.5335381
Ang, K. K., Guan, C., Phua, K. S., Wang, C., Zhou, L., Tang, K. Y., et al. (2014). Brain-computer interface-based robotic end effector system for wrist and hand rehabilitation: results of a three-armed randomized controlled trial for chronic stroke. Front. Neuroeng. 7:30. doi: 10.3389/fneng.2014.00030
Biswal, B., Zerrin Yetkin, F., Haughton, V. M., and Hyde, J. S. (1995). Functional connectivity in the motor cortex of resting human brain using echo-planar mri. Magn. Reson. Med. 34, 537–541. doi: 10.1002/mrm.1910340409
Bockbrader, M. A., Francisco, G., Lee, R., Olson, J., Solinsky, R., and Boninger, M. L. (2018). Brain computer interfaces in rehabilitation medicine. PM R 10(9) Suppl. 2 S233–S243. doi: 10.1016/j.pmrj.2018.05.028
Brett, M., Anton, J. L., Valabregue, R., and Poline, J. B. (2002). “Region of interest analysis using an SPM toolbox,” in Paper Presented at the 8th International Conferance on Functional Mapping of the Human Brain, Available on CD-ROM in NeuroImage, Sendai, 497. doi: 10.1016/S1053-8119(02)90010-8
Buckner, R. L., Krienen, F. M., Castellanos, A., Diaz, J. C., and Thomas Yeo, B. T. (2011). The organization of the human cerebellum estimated by intrinsic functional connectivity. J. Neurophysiol. 106, 2322–2345. doi: 10.1152/jn.00339.2011
Buetefisch, C. M. (2015). Role of the contralesional hemisphere in post-stroke recovery of upper extremity motor function. Front. Neurol. 6:214. doi: 10.3389/fneur.2015.00214
Bundy, D. T., Souders, L., Baranyai, K., Leonard, L., Schalk, G., Coker, R., et al. (2017). Contralesional brain-computer interface control of a powered exoskeleton for motor recovery in chronic stroke survivors. Stroke 48, 1908–1915. doi: 10.1161/STROKEAHA.116.016304
Bütefisch, C. M., Netz, J., Weßling, M., Seitz, R. J., and Hömberg, V. (2003). Remote changes in cortical excitability after stroke. Brain 126, 470–481. doi: 10.1093/brain/awg044
Butler, A. J., Shuster, M., O’Hara, E., Hurley, K., Middlebrooks, D., and Guilkey, K. (2013). A meta-analysis of the efficacy of anodal transcranial direct current stimulation for upper limb motor recovery in stroke survivors. J. Hand Ther. 26, 162–171. doi: 10.1016/j.jht.2012.07.002
Chen, J., Sun, D., Shi, Y., Jin, W., Wang, Y., Xi, Q., et al. (2019). Dynamic alterations in spontaneous neural activity in multiple brain networks in subacute stroke patients: a resting-state fMRI study. Front. Neurosci. 12:994. doi: 10.3389/fnins.2018.00994
Cheng, H.-J., Ng, K. K., Qian, X., Ji, F., Lu, Z., Teo, W. P., et al. (2021). Task-related brain functional network reconfigurations relate to motor recovery in chronic subcortical stroke. Sci. Rep. 11:8442.
Chew, E., Teo, W.-P., Tang, N., Ang, K. K., Ng, Y. S., Zhou, J. H., et al. (2020). Using transcranial direct current stimulation to augment the effect of motor imagery-assisted brain-computer interface training in chronic stroke patients—cortical reorganization considerations. Front. Neurol. 11:948. doi: 10.3389/fneur.2020.00948
Chong, J. S. X., Liu, S., Loke, Y. M., Hilal, S., Ikram, M. K., Xu, X., et al. (2017a). Influence of cerebrovascular disease on brain networks in prodromal and clinical Alzheimer’s disease. Brain 140, 3012–3022. doi: 10.1093/brain/awx224
Chong, J. S. X., Ng, G. J. P., Lee, S. C., and Zhou, J. (2017b). Salience network connectivity in the insula is associated with individual differences in interoceptive accuracy. Brain Struct. Funct. 222, 1635–1644.
Chong, J. S. X., Ng, K. K., Tandi, J., Wang, C., Poh, J.-H., Lo, J. C., et al. (2019). Longitudinal changes in the cerebral cortex functional organization of healthy elderly. J. Neurosci. 39, 5534–5550.
Cox, R. W. (1996). AFNI: software for analysis and visualization of functional magnetic resonance neuroimages. Comput. Biomed. Res. 29, 162–173. doi: 10.1006/cbmr.1996.0014
Dancause, N., Touvykine, B., and Mansoori, B. K. (2015). Inhibition of the Contralesional Hemisphere After Stroke: Reviewing a Few of the Building Blocks with a Focus on Animal Models, Progress in Brain Research, 1st Edn. Amsterdam: Elsevier B.V. doi: 10.1016/bs.pbr.2015.01.002
De Bruyn, N., Meyer, S., Kessner, S. S., Essers, B., Cheng, B., Thomalla, G., et al. (2018). Functional network connectivity is altered in patients with upper limb somatosensory impairments in the acute phase post stroke: a cross-sectional study. PLoS One 13:e0205693. doi: 10.1371/journal.pone.0205693
Di Lazzaro, V., Oliviero, A., Profice, P., Insola, A., Mazzone, P., Tonali, P., et al. (1999). Direct demonstration of interhemispheric inhibition of the human motor cortex produced by transcranial magnetic stimulation. Exp. Brain Res. 124, 520–524. doi: 10.1007/s002210050648
Ding, X., Li, C. Y., Wang, Q. S., Du, F. Z., Ke, Z. W., Peng, F., et al. (2014). Patterns in default-mode network connectivity for determining outcomes in cognitive function in acute stroke patients. Neuroscience 277, 637–646. doi: 10.1016/j.neuroscience.2014.07.060
Dodakian, L., Stewart, J. C., and Cramer, S. C. (2014). Motor imagery during movement activates the brain more than movement alone after stroke: a pilot study. J. Rehabil. Med. 46, 843–848. doi: 10.2340/16501977-1844
Dodd, K. C., Nair, V. A., and Prabhakaran, V. (2017). Role of the contralesional vs. Ipsilesional hemisphere in stroke recovery. Front. Hum. Neurosci. 11:9. doi: 10.3389/fnhum.2017.00469
Elsner, B., Kugler, J., and Mehrholz, J. (2018). Transcranial direct current stimulation (tDCS) for upper limb rehabilitation after stroke: future directions. 11 medical and health sciences 1109 neurosciences alan godfrey; rodrigo vitorio. J. Neuroeng. Rehabil. 15:106. doi: 10.1186/s12984-018-0459-7
Ferbert, A., Priori, A., Rothwell, J. C., Day, B. L., Colebatch, J. G., and Marsden, C. D. (1992). Interhemispheric inhibition of the human motor cortex. J. Physiol. 453, 525–546. doi: 10.1113/jphysiol.1992.sp019243
Foong, R., Tang, N., Chew, E., Chua, K. S. G., Ang, K. K., Quek, C., et al. (2020). Assessment of the efficacy of EEG-Based MI-BCI with visual feedback and EEG correlates of mental fatigue for upper-limb stroke rehabilitation. IEEE Trans. Biomed. Eng. 67, 786–795. doi: 10.1109/TBME.2019.2921198
Fugl-Meyer, A. R., Jääskö, L., Leyman, I., and Olsson, S. (1975). The post-stroke hemiplegic patient. 1. a method for evaluation of physical performance. Scand. J. Rehabil. Med. 7:13.
Gerloff, C., Cohen, L. G., Floeter, M. K., Chen, R., Corwell, B., and Hallett, M. (1998). Inhibitory influence of the ipsilateral motor cortex on responses to stimulation of the human cortex and pyramidal tract. J. Physiol. 510, 249–259. doi: 10.1111/j.1469-7793.1998.249bz.x
Golestani, A. M., Tymchuk, S., Demchuk, A., and Goodyear, B. G. (2013). Longitudinal evaluation of resting-state fMRI after acute stroke with hemiparesis. Neurorehabil. Neural Repair 27, 153–163. doi: 10.1177/1545968312457827
Greve, D. N., and Fischl, B. (2009). Accurate and robust brain image alignment using boundary-based registration. Neuroimage 48, 63–72. doi: 10.1016/j.neuroimage.2009.06.060
Hong, X., Lu, Z. K., Teh, I., Nasrallah, F. A., Teo, W. P., Ang, K. K., et al. (2017). Brain plasticity following MI-BCI training combined with tDCS in a randomized trial in chronic subcortical stroke subjects: a preliminary study. Sci. Rep. 7:9222. doi: 10.1038/s41598-017-08928-5
Hordacre, B., Moezzi, B., and Ridding, M. C. (2018). Neuroplasticity and network connectivity of the motor cortex following stroke: a transcranial direct current stimulation study. Hum. Brain Mapp. 39, 3326–3339. doi: 10.1002/hbm.24079
Kashiwagi, F. T., El Dib, R., Gomaa, H., Gawish, N., Suzumura, E. A., Da Silva, T. R., et al. (2018). Noninvasive brain stimulations for unilateral spatial neglect after stroke: a systematic review and meta-analysis of randomized and nonrandomized controlled trials. Neural. Plast. 2018:1638763. doi: 10.1155/2018/1638763
La, C., Nair, V. A., Mossahebi, P., Stamm, J., Birn, R., Meyerand, M. E., et al. (2016). Recovery of slow-5 oscillations in a longitudinal study of ischemic stroke patients. Neuroimage Clin. 11, 398–407. doi: 10.1016/j.nicl.2016.03.008
Lee, J. H., Kyeong, S., Kang, H., and Kim, D. H. (2019). Structural and functional connectivity correlates with motor impairment in chronic supratentorial stroke: a multimodal magnetic resonance imaging study. Neuroreport 30, 526–531. doi: 10.1097/WNR.0000000000001247
Liepert, J., Hamzei, F., and Weiller, C. (2000). Motor cortex disinhibition of the unaffected hemisphere after acute stroke. Muscle Nerve 23, 1761–1763. doi: 10.1002/1097-4598(200011)23:11<1761::AID-MUS14<3.0.CO;2-M
Lindenberg, R., Renga, V., Zhu, L. L., Nair, D., and Schlaug, G. (2010). Bihemispheric brain stimulation facilitates motor recovery in chronic stroke patients. Neurology 75, 2176–2184. doi: 10.1212/WNL.0b013e318202013a
Liu, A., Vöröslakos, M., Kronberg, G., Henin, S., Krause, M. R., Huang, Y., et al. (2018). Immediate neurophysiological effects of transcranial electrical stimulation. Nat. Commun. 9:5092. doi: 10.1038/s41467-018-07233-7
Liu, G., Dang, C., Peng, K., Xie, C., Chen, H., Xing, S., et al. (2015). Increased spontaneous neuronal activity in structurally damaged cortex is correlated with early motor recovery in patients with subcortical infarction. Eur. J. Neurol. 22, 1540–1547. doi: 10.1111/ene.12780
Liu, J., Qin, W., Wang, H., Zhang, J., Xue, R., Zhang, X., et al. (2014). Altered spontaneous activity in the default-mode network and cognitive decline in chronic subcortical stroke. J. Neurol. Sci. 347, 193–198. doi: 10.1016/j.jns.2014.08.049
Lotze, M., Markert, J., Sauseng, P., Hoppe, J., Plewnia, C., and Gerloff, C. (2006). The role of multiple contralesional motor areas for complex hand movements after internal capsular lesion. J. Neurosci. 26, 6096–6102. doi: 10.1523/JNEUROSCI.4564-05.2006
Loubinoux, I., Carel, C., Pariente, J., Dechaumont, S., Albucher, J. F., Marque, P., et al. (2003). Correlation between cerebral reorganization and motor recovery after subcortical infarcts. Neuroimage 20, 2166–2180. doi: 10.1016/j.neuroimage.2003.08.017
Lüdemann-Podubecká, J., Bösl, K., and Nowak, D. A. (2016). Inhibition of the contralesional dorsal premotor cortex improves motor function of the affected hand following stroke. Eur. J. Neurol. 23, 823–830. doi: 10.1111/ene.12949
Lv, H., Wang, Z., Tong, E., Williams, L. M., Zaharchuk, G., Zeineh, M., et al. (2018). Resting-state functional MRI: everything that nonexperts have always wanted to know. Am. J. Neuroradiol. 39, 1390–1399. doi: 10.3174/ajnr.A5527
Madhavan, S., Weber, K. A., and Stinear, J. W. (2011). Non-invasive brain stimulation enhances fine motor control of the hemiparetic ankle: implications for rehabilitation. Exp. Brain Res. 209, 9–17. doi: 10.1007/s00221-010-2511-0
Mane, R., Chew, E., Phua, K. S., Ang, K. K., Robinson, N., Vinod, A. P., et al. (2019). Prognostic and monitory EEG-biomarkers for BCI upper-limb stroke rehabilitation. IEEE Trans. Neural Syst. Rehabil. Eng. 27, 1654–1664. doi: 10.1109/TNSRE.2019.2924742
Manganotti, P., Acler, M., Zanette, G. P., Smania, N., and Fiaschi, A. (2008). Motor cortical disinhibition during early and late recovery after stroke. Neurorehabil. Neural Repair 22, 396–403. doi: 10.1177/1545968307313505
Matsuura, A., Onoda, K., Oguro, H., and Yamaguchi, S. (2015). Magnetic stimulation and movement-related cortical activity for acute stroke with hemiparesis. Eur. J. Neurol. 22, 1526–1532. doi: 10.1111/ene.12776
Ng, K. K., Lo, J. C., Lim, J. K. W., Chee, M. W. L., and Zhou, J. (2016). Reduced functional segregation between the default mode network and the executive control network in healthy older adults: a longitudinal study. Neuroimage 133, 321–330. doi: 10.1016/j.neuroimage.2016.03.029
Nitsche, M. A., and Paulus, W. (2000). Excitability changes induced in the human motor cortex by weak transcranial direct current stimulation. J. Physiol. 527, 633–639. doi: 10.1111/j.1469-7793.2000.t01-1-00633.x
Nitsche, M. A., and Paulus, W. (2001). Sustained excitability elevations induced by transcranial DC motor cortex stimulation in humans. Neurology 57, 1899–1901. doi: 10.1212/WNL.57.10.1899
Nowak, D. A., Grefkes, C., Ameli, M., and Fink, G. R. (2009). Interhemispheric competition after stroke: brain stimulation to enhance recovery of function of the affected hand. Neurorehabil. Neural Repair 23, 641–656. doi: 10.1177/1545968309336661
Nowak, D. A., Grefkes, C., Dafotakis, M., Eickhoff, S., Küst, J., Karbe, H., et al. (2008). Effects of low-frequency repetitive transcranial magnetic stimulation of the contralesional primary motor cortex on movement kinematics and neural activity in subcortical stroke. Arch. Neurol. 65, 741–747. doi: 10.1001/archneur.65.6.741
Pan, P., Zhang, Y., Liu, Y., Zhang, H., Guan, D., and Xu, Y. (2017). Abnormalities of regional brain function in Parkinson’s disease: a meta-analysis of resting state functional magnetic resonance imaging studies. Sci. Rep. 7:40469. doi: 10.1038/srep40469
Park, C. H., Chang, W. H., Ohn, S. H., Kim, S. T., Bang, O. Y., Pascual-Leone, A., et al. (2011). Longitudinal changes of resting-state functional connectivity during motor recovery after stroke. Stroke 42, 1357–1362. doi: 10.1161/STROKEAHA.110.596155
Park, J. Y., Kim, Y. H., Chang, W. H., Park, C. H., Shin, Y. I., Kim, S. T., et al. (2014). Significance of longitudinal changes in the default-mode network for cognitive recovery after stroke. Eur. J. Neurosci. 40, 2715–2722. doi: 10.1111/ejn.12640
Pichiorri, F., Morone, G., Petti, M., Toppi, J., Pisotta, I., Molinari, M., et al. (2015). Brain-computer interface boosts motor imagery practice during stroke recovery. Ann. Neurol. 77, 851–865. doi: 10.1002/ana.24390
Power, J. D., Barnes, K. A., Snyder, A. Z., Schlaggar, B. L., and Petersen, S. E. (2012). Corrigendum to “spurious but systematic correlations in functional connectivity MRI networks arise from subject motion” [NeuroImage 59 (3) (2012) 2142-2154]. Neuroimage 63:999. doi: 10.1016/j.neuroimage.2012.01.069
Power, J. D., Mitra, A., Laumann, T. O., Snyder, A. Z., Schlaggar, B. L., and Petersen, S. E. (2014). Methods to detect, characterize, and remove motion artifact in resting state fMRI. Neuroimage 84, 320–341. doi: 10.1016/j.neuroimage.2013.08.048
Puig, J., Blasco, G., Alberich-Bayarri, A., Schlaug, G., Deco, G., Biarnes, C., et al. (2018). Resting-state functional connectivity magnetic resonance imaging and outcome after acute stroke. Stroke 49, 2353–2360. doi: 10.1161/STROKEAHA.118.021319
Ramos-Murguialday, A., Curado, M. R., Broetz, D., Yilmaz, Ö, Brasil, F. L., Liberati, G., et al. (2019). Brain-machine interface in chronic stroke: randomized trial long-term follow-up. Neurorehabil. Neural Repair 33, 188–198. doi: 10.1177/1545968319827573
Reidler, J. S., Zaghi, S., and Fregni, F. (2011). Neurophysiological Effects of Transcranial Direct Current Stimulation, Neurofeedback and Neuromodulation Techniques and Applications. Amsterdam: Elsevier Inc. doi: 10.1016/B978-0-12-382235-2.00012-3
Schaechter, J. D., and Perdue, K. L. (2008). Enhanced cortical activation in the contralesional hemisphere of chronic stroke patients in response to motor skill challenge. Cereb. Cortex 18, 638–647. doi: 10.1093/cercor/bhm096
Song, J., Young, B. M., Nigogosyan, Z., Walton, L. M., Nair, V. A., Grogan, S. W., et al. (2014). Characterizing relationships of DTI, fMRI, and motor recovery in stroke rehabilitation utilizing brain-computer interface technology. Front. Neuroeng. 7:31. doi: 10.3389/fneng.2014.00031
Sun, L., Yin, D., Zhu, Y., Fan, M., Zang, L., Wu, Y., et al. (2013). Cortical reorganization after motor imagery training in chronic stroke patients with severe motor impairment: a longitudinal fMRI study. Neuroradiology 55, 913–925. doi: 10.1007/s00234-013-1188-z
Takeuchi, N., Chuma, T., Matsuo, Y., Watanabe, I., and Ikoma, K. (2005). Repetitive transcranial magnetic stimulation of contralesional primary motor cortex improves hand function after stroke. Stroke 36, 2681–2686. doi: 10.1161/01.STR.0000189658.51972.34
Teo, W. P., and Chew, E. (2014). Is motor-imagery brain-computer interface feasible in stroke rehabilitation? PM R 6, 723–728. doi: 10.1016/j.pmrj.2014.01.006
Touvykine, B., Mansoori, B. K., Jean-Charles, L., Deffeyes, J., Quessy, S., and Dancause, N. (2016). The effect of lesion size on the organization of the ipsilesional and contralesional motor cortex. Neurorehabil. Neural Repair 30, 280–292. doi: 10.1177/1545968315585356
Tsai, Y. H., Yuan, R., Huang, Y. C., Yeh, M. Y., Lin, C. P., and Biswal, B. B. (2014). Disruption of brain connectivity in acute stroke patients with early impairment in consciousness. Front. Psychol. 4:956. doi: 10.3389/fpsyg.2013.00956
Tsuchimoto, S., Shindo, K., Hotta, F., Hanakawa, T., Liu, M., and Ushiba, J. (2019). Sensorimotor connectivity after motor exercise with neurofeedback in post-stroke patients with hemiplegia. Neuroscience 416, 109–125. doi: 10.1016/j.neuroscience.2019.07.037
Tuladhar, A. M., Snaphaan, L., Shumskaya, E., Rijpkema, M., Fernandez, G., Norris, D. G., et al. (2013). Default mode network connectivity in stroke patients. PLoS One 8:e66556. doi: 10.1371/journal.pone.0066556
Wang, C., Ji, F., Hong, Z., Poh, J. S., Krishnan, R., Lee, J., et al. (2016a). Disrupted salience network functional connectivity and white-matter microstructure in persons at risk for psychosis: findings from the LYRIKS study. Psychol. Med. 46, 2771–2783. doi: 10.1017/S0033291716001410
Wang, C., Ong, J. L., Patanaik, A., Zhou, J., and Chee, M. W. L. (2016b). Spontaneous eyelid closures link vigilance fluctuation with fMRI dynamic connectivity states. Proc. Natl. Acad. Sci. U.S.A. 113, 9653–9658.
Wang, H., Sun, L., and Jia, J. (2018). The plasticity of resting-state brain networks associated with motor imagery training in chronic stroke patients. Ann. Phys. Rehabil. Med. 61:e20. doi: 10.1016/j.rehab.2018.05.043
Wang, H., Xu, G., Wang, X., Sun, C., Zhu, B., Fan, M., et al. (2019). The reorganization of resting-state brain networks associated with motor imagery training in chronic stroke patients. IEEE Trans. Neural Syst. Rehabil. Eng. 27, 2237–2245.
Wang, J., Chen, H., Liang, H., Wang, W., Liang, Y., Liang, Y., et al. (2019). Low-frequency fluctuations amplitude signals exhibit abnormalities of intrinsic brain activities and reflect cognitive impairment in leukoaraiosis patients. Med. Sci. Monit. 25, 5219–5228. doi: 10.12659/MSM.915528
Yan, C. G., Wang, X. D., Zuo, X. N., and Zang, Y. F. (2016). DPABI: data processing & analysis for (resting-state) brain imaging. Neuroinformatics 14, 339–351. doi: 10.1007/s12021-016-9299-4
Young, B. M., Nigogosyan, Z., Walton, L. M., Song, J., Nair, V. A., Grogan, S. W., et al. (2014). Changes in functional brain organization and behavioral correlations after rehabilitative therapy using a brain-computer interface. Front. Neuroeng. 7:26. doi: 10.3389/fneng.2014.00026
Young, B. M., Stamm, J. M., Song, J., Remsik, A. B., Nair, V. A., Tyler, M. E., et al. (2016). Brain-computer interface training after stroke affects patterns of brain-behavior relationships in corticospinal motor fibers. Front. Hum. Neurosci. 10:457. doi: 10.3389/fnhum.2016.00457
Zaghi, S., Acar, M., Hultgren, B., Boggio, P. S., and Fregni, F. (2010). Noninvasive brain stimulation with low-intensity electrical currents: putative mechanisms of action for direct and alternating current stimulation. Neuroscientist 16, 285–307. doi: 10.1177/1073858409336227
Zang, Y., Jiang, T., Lu, Y., He, Y., and Tian, L. (2004). Regional homogeneity approach to fMRI data analysis. Neuroimage 22, 394–400.
Zang, Y. F., Yong, H., Chao-Zhe, Z., Qing-Jiu, C., Man-Qiu, S., Meng, L., et al. (2007). Altered baseline brain activity in children with ADHD revealed by resting-state functional MRI. Brain Dev. 29, 83–91. doi: 10.1016/j.braindev.2006.07.002
Zhang, L., Zuo, X.-N., Ng, K. K., Chong, J. S. X., Shim, H. Y., Ong, M. Q. W., et al. (2020). Distinct bold variability changes in the default mode and salience networks in Alzheimer’s disease spectrum and associations with cognitive decline. Sci. Rep. 10:6457.
Zhao, Z., Tang, C., Yin, D., Wu, J., Gong, J., Sun, L., et al. (2018). Frequency-specific alterations of regional homogeneity in subcortical stroke patients with different outcomes in hand function. Hum. Brain Mapp. 39, 4373–4384. doi: 10.1002/hbm.24277
Keywords: functional magnetic resonance imaging, stroke, amplitude of low-frequency fluctuation, regional homogeneity, functional connectivity, brain-computer interface-assisted motor imagery, transcranial direct current stimulation
Citation: Hu M, Cheng H-J, Ji F, Chong JSX, Lu Z, Huang W, Ang KK, Phua KS, Chuang K-H, Jiang X, Chew E, Guan C and Zhou JH (2021) Brain Functional Changes in Stroke Following Rehabilitation Using Brain-Computer Interface-Assisted Motor Imagery With and Without tDCS: A Pilot Study. Front. Hum. Neurosci. 15:692304. doi: 10.3389/fnhum.2021.692304
Received: 08 April 2021; Accepted: 24 June 2021;
Published: 16 July 2021.
Edited by:
Selina C. Wriessnegger, Graz University of Technology, AustriaReviewed by:
Rupert Ortner, g.tec medical engineering Spain S.L., SpainCamille Jeunet, UMR 5287 Institut de Neurosciences Cognitives et Intégratives d’Aquitaine (INCIA), France
Copyright © 2021 Hu, Cheng, Ji, Chong, Lu, Huang, Ang, Phua, Chuang, Jiang, Chew, Guan and Zhou. This is an open-access article distributed under the terms of the Creative Commons Attribution License (CC BY). The use, distribution or reproduction in other forums is permitted, provided the original author(s) and the copyright owner(s) are credited and that the original publication in this journal is cited, in accordance with accepted academic practice. No use, distribution or reproduction is permitted which does not comply with these terms.
*Correspondence: Effie Chew, ZWZmaWVfY2hld0BudWhzLmVkdS5zZw==; Cuntai Guan, Y3RndWFuQG50dS5lZHUuc2c=; Juan Helen Zhou, aGVsZW4uemhvdUBudXMuZWR1LnNn
†These authors share senior authorship