- 1Graduate Programs in Rehabilitation Sciences, University of British Columbia, Vancouver, BC, Canada
- 2Brain, Behaviour, and Development Theme, BC Children’s Hospital Research Institute, Vancouver, BC, Canada
- 3Department of Occupational Science and Occupational Therapy, University of British Columbia, Vancouver, BC, Canada
- 4Department of Pediatrics, University of British Columbia, Vancouver, BC, Canada
- 5Sunny Hill Health Centre at BC Children’s Hospital, Vancouver, BC, Canada
- 6CanChild Centre for Childhood Disability Research, Hamilton, ON, Canada
Background and Objectives: Children with developmental coordination disorder (DCD) have difficulty learning motor skills, which can affect their participation in activities of daily living and psychosocial well-being. Over 50% of children with DCD also have attention deficit hyperactivity disorder (ADHD), which further exacerbates their motor problems and impact on quality of life. A rehabilitation approach known as Cognitive Orientation to Occupational Performance uses problem-solving strategies to help children learn motor skills they wish to achieve. While this cognitive approach has been effective for children with DCD, few studies have examined the effectiveness of this approach for children with co-occurring ADHD. Further, the underlying mechanism and neural basis of this intervention are largely unknown.
Methods: In this randomized waitlist-controlled trial, we used MRI to examine white matter microstructure after intervention in 8–12-year-old children with DCD (n = 28) and with DCD and co-occurring ADHD (n = 25). Children in both groups were randomized to either a treatment group or waitlist group at their first MRI. The treatment group began the intervention after their MRI scan and returned for a post-treatment scan at 3 months, and follow-up scan at 6 months; the waitlist group waited 3 months before their second MRI, received the intervention, and then had a post-treatment scan. Each child received intervention once weekly for 10 weeks. Diffusion tensor imaging was used to acquire white matter diffusion parameters and was analyzed using tract-based spatial statistics (TBSS).
Results and Conclusion: Children with DCD showed significant improvement in white matter microstructure in the bilateral anterior thalamic radiation, bilateral sensorimotor tract, bilateral cingulum, fornix, splenium and body of corpus callosum, right inferior fronto-occipital fasciculus, and white matter pathways to bilateral inferior gyri, right middle frontal gyrus, frontal medial cortex, and left cuneus. We suggest that these rehabilitation-induced neural changes in children with DCD occurred in regions associated with attention, self-regulation, motor planning, and inter-hemispheric communication, which positively affected brain connectivity and motor function. In contrast, children with DCD and co-occurring ADHD did not show any brain changes following the intervention. Modifications to the treatment protocol might help address the attentional and self-regulatory needs of children with a dual diagnosis.
Clinical Trial Registration: ClinicalTrials.gov ID: NCT02597751.
Introduction
Up to 5 to 6% of all school-age children may be affected by developmental coordination disorder (DCD), a neurodevelopmental disorder characterized by difficulty performing and learning motor skills that significantly interferes with daily activities and academic achievement (American Psychiatric Association, 2013). These motor difficulties can lead to higher risks of anxiety, emotional and behavioral problems, low self-esteem (Hellgren et al., 1994; Green et al., 2006; Pratt and Hill, 2011; Lingam et al., 2012; Hill and Brown, 2013; Zwicker et al., 2013; Crane et al., 2017; Harrowell et al., 2017; Li et al., 2018), as well as physical consequences such as obesity and poorer physical fitness (Cairney et al., 2005, 2010; Rivilis et al., 2011; Cairney and Veldhuizen, 2013). A well-known and common co-occurrence of DCD is attention deficit hyperactivity disorder (ADHD), exacerbating motor and functional difficulties in children (Kadesjo and Gillberg, 1999; Piek et al., 1999; Dewey et al., 2000, 2002; Rasmussen and Gillberg, 2000; Pitcher et al., 2003; Martin et al., 2006; Watemberg et al., 2007; Fliers et al., 2009; Barkley, 2014; Blank et al., 2019) and increasing the risk of psychological distress (Piek et al., 2007; Missiuna et al., 2014), antisocial behavior (Rasmussen and Gillberg, 2000), and peer victimization (Dewey and Volkovinskaia, 2018). Motor problems of children with DCD with or without ADHD have been attributed to attention deficits and lack of inhibition (Kaiser et al., 2015; Fong et al., 2016; Thornton et al., 2018). An electroencephalographic (EEG) study suggests that the contribution of attention to motor performance is greater in children with co-occurring DCD and ADHD than children with DCD only (Fong et al., 2016); it, accordingly assumes that improving attention in children with DCD with or without ADHD leads to motor performance improvement (Fong et al., 2016). However, only 30% to 50% of children with DCD and ADHD show improved motor performance following attention-related medications (Bart et al., 2010; Brossard-Racine et al., 2012), and only 50% of children with ADHD receive non-pharmaceutical treatment for their motor difficulties (Fliers et al., 2010, 2011). This controversy in the literature and limited attention to motor problems add complexities to the treatment approaches for children with a dual diagnosis of DCD and ADHD.
Current neuroimaging studies reveal that DCD is associated with involvement of the cerebellum, the parietal lobe, the frontal lobe, the basal ganglia, and the limbic system (Brown-Lum and Zwicker, 2015; Biotteau et al., 2016); each of these regions play a specific role in generating internal models of motor actions (Kawato and Gomi, 1992; Blakemore et al., 2001), updating the internal model (Blakemore and Sirigu, 2003), providing optimal control during motor execution (Shadmehr and Krakauer, 2008), executing motor actions (Shadmehr and Krakauer, 2008), and managing the movement motivation (Merel et al., 2019), respectively.
However, co-occurring DCD and ADHD are associated with unique structural (Langevin et al., 2014, 2015), functional (McLeod et al., 2014, 2016; Thornton et al., 2018), and physiological (Yeh et al., 2012; Fong et al., 2016) properties of the sensorimotor and attentional networks, including the parietal and frontal lobes (Yeh et al., 2012; Langevin et al., 2014, 2015; McLeod et al., 2014, 2016; Thornton et al., 2018) as well as interhemispheric connections and asymmetry (Langevin et al., 2014, 2015). Individuals with co-occurring DCD and ADHD may use compensatory attentional control of motor coordination through increasing cerebral blood flow in the posterior cingulate cortex and the cerebellum (Yeh et al., 2012).
A treatment approach called Cognitive Orientation to daily Occupational Performance (CO-OP) is one of the recommended treatments for DCD as per international clinical guidelines (Blank et al., 2019). CO-OP is a client-centered, task-oriented approach developed for children with DCD to successfully solve motor problems (Polatajko et al., 2001). Previous studies have shown positive results in children with DCD (Ward and Rodger, 2004; Taylor et al., 2007; Zwicker et al., 2015; Capistran and Martini, 2016; Thornton et al., 2016), but given that at least 50% of children with DCD have co-occurring ADHD (Dewey et al., 2002), we wondered if this cognitive approach was effective for children with a dual diagnosis of DCD and ADHD. Results from a single case study of six children with ADHD show promise for the CO-OP approach (Gharebaghy et al., 2015), but studies with larger sample sizes and more rigorous research designs are required to determine CO-OP’s effectiveness in children with DCD, with and without ADHD. To better understand if and how CO-OP affects children with DCD with or without ADHD differently, we examined brain changes after 10 sessions of CO-OP intervention using various MRI modalities (resting state, T1-weighted images, and diffusion tensor imaging; DTI). In the current study, we focus on structural neuroplastic changes captured by DTI after CO-OP intervention in children with DCD, with and without ADHD. Understanding the neural mechanisms of CO-OP could further guide the modification and optimization of CO-OP based on specific needs of children with DCD with or without ADHD.
Diffusion MRI is a non-invasive tool measuring both structural connectivity and white matter microstructure by obtaining information about connections between brain regions and their tissue architecture (Jones et al., 2013). It measures water diffusivity in brain tissue and the amount of restriction experienced by water molecules moving in the brain. Water molecules are considerably impeded in white matter, owing to factors such as myelination, fiber diameter or density, as well as membrane permeability (Beaulieu, 2002). This impedance causes directional and anisotropic water diffusivity.
Thus far, diffusion MRI studies have used various analysis methods, including tract-based spatial statistics (TBSS; Williams et al., 2017; Brown-Lum et al., 2020), constrained spherical deconvolution (Hyde et al., 2019), tractography (Zwicker et al., 2012; Debrabant et al., 2016) and graph theory (Debrabant et al., 2016) to understand white matter microstructure in children with DCD. Results have shown that children with DCD have altered white matter microstructure in the corpus callosum (Langevin et al., 2014; Brown-Lum et al., 2020) and sensorimotor, corticospinal, cortico-cerebellar (Zwicker et al., 2012; Debrabant et al., 2016; Williams et al., 2017; Brown-Lum et al., 2020), and frontoparietal pathways (Langevin et al., 2014; Williams et al., 2017; Hyde et al., 2019; Brown-Lum et al., 2020). Structural connectivity between brain regions (e.g., cerebellar lobule VI and right superior parietal gyrus; Debrabant et al., 2016) is also implicated in children with DCD. Children with DCD and co-occurring ADHD have altered white matter in the corpus callosum (Langevin et al., 2014). However, no study investigated longitudinal changes following intervention in children with DCD. In this study, we will compare white matter microstructural properties of children with DCD, with or without ADHD, before and after CO-OP intervention.
Materials and Methods
Study Design
In this randomized waitlist-controlled trial (ClinicalTrials.gov ID: NCT02597751), we used computer-generated sequential blocks of four to six, prepared by a statistician to randomize children with DCD, with or without ADHD, into treatment and waitlist groups. To ensure a power of 90% to detect a 3% difference in axial diffusivity (AD) with a type-1 error of 0.01, we used our pilot study on DTI in this population (effect size = 1.1; Zwicker et al., 2012) and estimated a sample size of 27 per group.
Participants
From September 2014 to July 2019, children with DCD and DCD+ADHD were recruited from the Sunny Hill Health Centre for Children, BC Children’s Hospital ADHD Clinic, the Vancouver Regional Pediatric Team, and from advertisements in the community (Vancouver, BC, Canada). Children aged 8–12 years were eligible to participate in the study if they were diagnosed with DCD as per the Diagnostic and Statistical Manual–5th edition (American Psychiatric Association, 2013) and the international clinical practice recommendations (Blank et al., 2019) as follows: (1): scored ≤16th percentile on the Movement Assessment Battery for Children—2nd ed. (MABC-2; Henderson et al., 2007); (2) scored in the suspected or indicative DCD range on the DCD Questionnaire (Wilson et al., 2009); (3) parents reported motor difficulties from a young age; and (4) there was no other medical condition that could explain motor difficulties based on parent-report, clinical observations, and/or a medical examination. Children were assigned to the DCD+ADHD group if they had been diagnosed ADHD based on parent report. ADHD symptomatology was quantified for all children using the Conners ADHD Index—parent report form (Conners, 2009). Exclusion criteria included premature birth (gestational age <37 weeks), other neurodevelopmental disorders (e.g., autism spectrum disorder), claustrophobia, and MRI contraindications (e.g., metal braces). After parental consent and child assent as per ethics approval from the University of British Columbia/BC Children’s and Women’s Research Ethics Board, children were enrolled in the study.
Procedure
Participants started with MRI safety screening and an MRI simulation session. A research nurse accompanied by a graduate student scanned all the participants using MRI at baseline, after 3 months, and after 6 months. After the first MRI scan, children were randomized into treatment and waitlist groups; group assignments were concealed to the research team in an opaque, sealed envelope. Children assigned to the treatment group went through pre-intervention assessment, then received 10 sessions of CO-OP intervention (once a week), followed by post-intervention assessment, a second (post-treatment) MRI scan, and a third follow-up MRI scan 3 months later to determine if brain changes were maintained after the intervention was discontinued. In contrast, children in the waitlist group were scanned 3 months after their first MRI, received their pre-assessments and intervention, followed by a third (post-treatment) MRI. A study design schematic (Izadi-Najafabadi et al., 2020) can be found elsewhere.
Motor assessments were administered by an occupational therapist not involved in the intervention. Assessments included the following: (1) Canadian Occupational Performance Measure (COPM; Law et al., 2014), which measured the child’s perceived motor performance and satisfaction on their three chosen motor goals; (2) Performance Quality Rating Scale (PQRS; Martini et al., 2015), which objectively measured the child’s quality of motor performance on their motor goals based on a blinded occupational therapist scoring videos of the child performing their motor goals before and after the intervention; and (3) Bruininks-Oseretsky Test of Motor Proficiency-2 (BOT-2) short form (Bruininks and Bruininks, 2005), which measured general motor skills. A more detailed description of behavioral outcome measures and their results can be found elsewhere (Izadi-Najafabadi et al., under review).
Intervention
Using the Pediatric Activity Card Sort (Mandich et al., 2004), an assessment tool for developing activity profiles for children, each child with DCD (with or without ADHD) identified three functional motor goals (e.g., handwriting, playing basketball, tying shoelaces) on which to work during the CO-OP intervention. An occupational therapist administered 10 one-hour sessions of the CO-OP intervention over 10 weeks as per published protocol (Polatajko et al., 2001) at the Sunny Hill Health Centre for Children or BC Children’s Hospital. CO-OP intervention is a problem-solving rehabilitation approach that focuses on the use of cognitive strategies to solve motor problems. Children learned the global strategy of CO-OP called Goal-Plan-Do-Check in the first session and were guided to discover specific cognitive strategies (e.g., supplementing task knowledge, changing body position) to solve motor problems to achieve their chosen goals (Miller et al., 2001; Polatajko and Mandich, 2004). Parents were instructed and encouraged to use CO-OP at home and keep a record of practice time for each goal on each day, per week.
Diffusion Tensor Imaging
Acquisition
A 3-Tesla General-Electric Discovery MR750 MRI scanner with a 32-channel head coil was used to acquire DTI data. Participants were asked to lie very still during the DTI sequence while watching a movie. A minimum of two 32-direction DTI sequences were acquired so that the best sequence could be used for data analysis. DTI acquisition parameters for each DTI sequence with 32 diffusion encoded directions dispersed around a full-sphere were as follows: TR: 7,000 ms; TE: 60 ms; FOV: 220 mm; acquisition matrix: 100 × 100; slice thickness: 2.2 mm; b = 1,000. Three b0 volumes were also acquired at the beginning of each DTI sequence. A graduate student monitored movement during the scan, encouraged the child to stay still, and asked the sequence to be repeated, if needed.
Preprocessing
DTI data preprocessing and analysis were completed using FSL 6.0.1. (Smith et al., 2004). Preprocessing steps included: (1) executing eddy_cuda for distortion correction through signal loss (drop-out) detection and replacement (Andersson et al., 2016) as well as correction for susceptibility-induced distortions, eddy currents, and subject motions (within and between volumes; Andersson and Sotiropoulos, 2016; Andersson et al., 2017); (2) implementing automated quality control via QUality Assessment for DMRI (QUAD) to extract quality metrices of within-and between-volume average relative motion, absolute relative motion, signal-to-noise ratio (SNR), and contrast-to-noise ratio (CNR; Bastiani et al., 2019); for each participant, the sequence with higher CNR was carried forward for further analysis; (3) visually checking every image to identify any residual distortion and removing any motion-contaminated volumes; any image with less than 20 good quality volumes were excluded from the analysis; and (4) reconstructing diffusion tensors to estimate DTI parameters and create corresponding maps: fractional anisotropy (FA), the degree of anisotropy/directionality of water diffusion in each voxel; mean diffusivity (MD), average amount of water diffusion independent of directionality in each voxel; axial diffusivity (AD), water diffusivity along the tract; radial diffusivity (RD), water diffusivity perpendicular to the tract (Jones et al., 2013).
Statistical Analysis
We performed tract-based spatial statistics (TBSS) to analyze whole-brain white matter microstructural properties without pre-specification of tracts of interest (Smith et al., 2006). TBSS carries out a voxel-wise statistical analysis while controlling for inaccurate alignment and arbitrary smoothing in traditional voxel-based analysis (Smith et al., 2006). TBSS aligns each participant’s FA map to the FA map of the most representative participant and then to the standard template (MNI 152 1 mm). The use of the most representative participant as the first step in registration is critical to TBSS reliability (Madhyastha et al., 2014) since it increases the alignment accuracy and reduces inter-subject variability (Smith et al., 2006). A mean FA skeleton was then generated using all participants’ aligned FA maps and thresholded at 0.2 to exclude any gray matter residuals and peripheral tracts with high inter-subject variability. Each participant’s FA, MD, AD, and RD data were then projected onto the mean FA skeleton to be ready for statistical analysis (Smith et al., 2006). TBSS has shown a high test-retest reliability in longitudinal studies (Madhyastha et al., 2014).
TBSS results were fed into Permutation Analysis of Linear Models (PALM; Winkler et al., 2014) with 5,000 permutations to investigate 3-month maturation effect (scan 1 and 2 of waitlist groups), pre-post intervention effect (scan 1 and 2 of treatment group combined with scan 2 and 3 of waitlist group), and 3-month follow-up effect (scan 2 and 3 of treatment group) for children with DCD and DCD+ADHD. We used a paired t-test design controlling for ADHD-related medications. Family-wise error correction (FWE) with an alpha level of 0.05 was applied to correct for multiple testing errors. We also used PALM to run a generalized linear model and investigate the effect of motor outcomes (PQRS; Martini et al., 2015) and BOT-2 (Bruininks and Bruininks, 2005) on DTI parameters in the two groups. To report the results, we used a sensitive thresholding approach called threshold-free cluster enhancement (TFCE), in which voxel-wise values receive local spatial supports from extended areas of signal (Smith and Nichols, 2009). To label anatomic locations of white matter structures, the Johns Hopkins University ICBM-81 White-Matter Labels (Mori et al., 2005), the White Matter Tractography Atlas (Hua et al., 2008), and the Sensorimotor Tracts Atlas (Archer et al., 2018) were used.
Results
Participants
Eighty children were recruited for this study; two children with DCD + ADHD declined to participate. Of the 78 children enrolled and randomized into treatment and waitlist groups, 37 children were diagnosed with DCD [25 male, 12 female; mean (SD) age: 9.7 (1.5) years] and 41 children were diagnosed with both DCD and ADHD [38 male, 3 female; mean (SD) age: 10.2 (1.4) years]. Nine children with DCD and 16 children with DCD + ADHD were excluded from the analysis due to false inclusion (DCD: n = 3; DCD + ADHD: n = 12), low MRI quality (DCD: n = 2; DCD + ADHD: n = 1), and child disliked MRI (DCD: n = 4; DCD + ADHD: n = 3). See Figure 1 for more details.
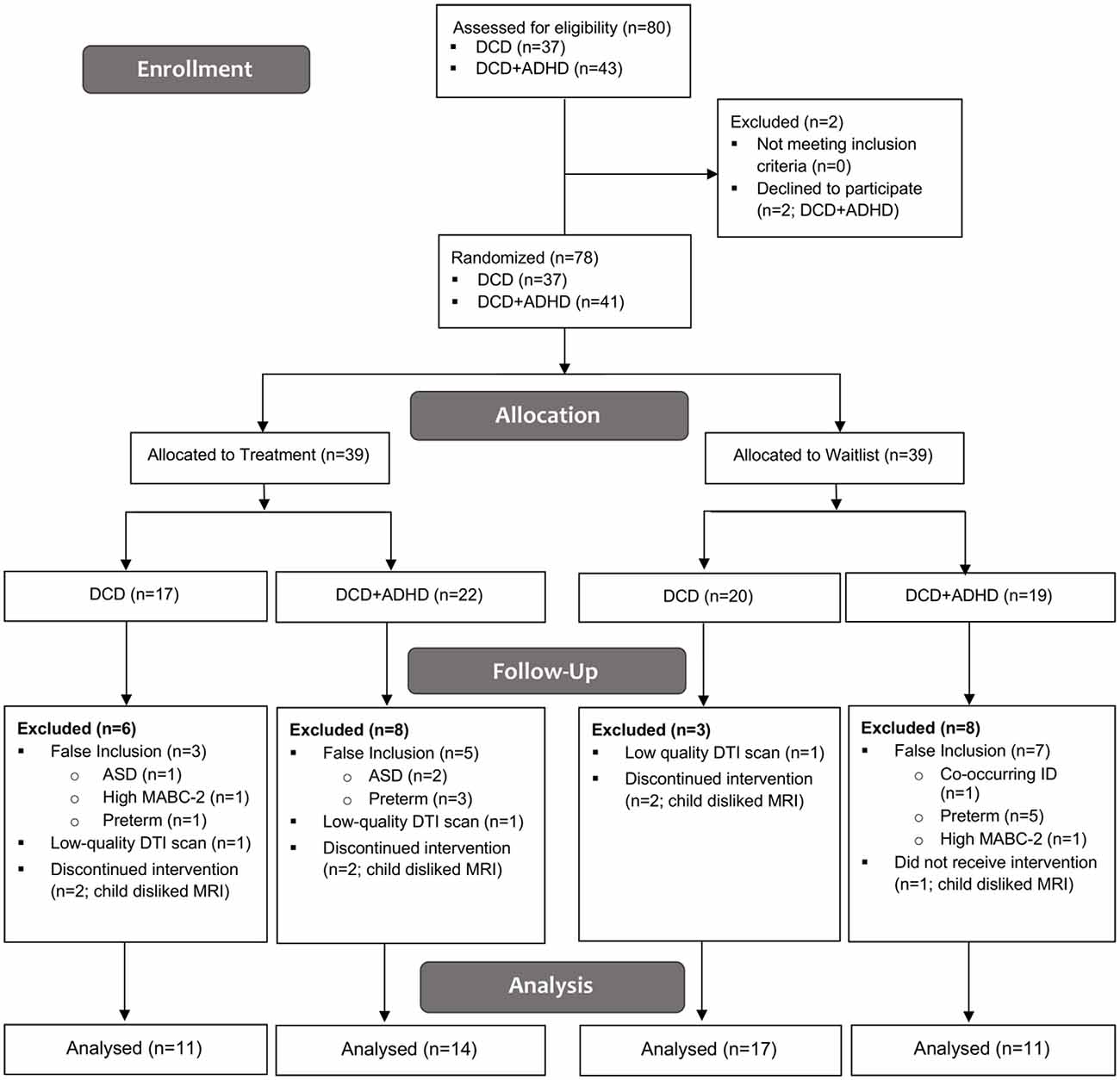
Figure 1. CONSORT Flow Diagram. ADHD, attention deficit hyperactivity disorder; ASD, autism spectrum disorder; DCD, developmental coordination disorder; DTI, diffusion tensor imaging; ID, intellectual disability; MABC-2, Movement Assessment Battery for Children—2nd ed.
Accordingly, we compared pre-and post-intervention scans from 28 children with DCD [20 male, 8 female; mean (SD) age: 9.6 (1.4) years] and 25 children with DCD + ADHD [22 male, 3 female; mean (SD) age: 9.9 (1.1) years]. Due to the quality of baseline and follow-up scans, maturation effect (DCD: n = 18; DCD + ADHD: n = 10) and follow-up analyses (DCD: n = 10; DCD + ADHD: n = 13) were performed with fewer participants. At the time of intervention, three children with DCD and 13 children with DCD + ADHD took ADHD-related medications (e.g., Adderall, Biphentin, Concerta). Table 1 summarizes participant characteristics and Table 2 highlights DTI quality and head motion parameters per group.
DCD-Only Group
Maturation
Comparison of scan 1 and scan 2 of children in the waitlist group showed a significant decrease in FA and a significant increase in MD and RD of the left anterior thalamic radiation passing through the anterior corona radiata (FA: FWE-p < 0.05; MD and RD: FWE-p < 0.01) and anterior limb of internal capsule (FA and MD: FWE-p < 0.05; RD: FWE-p < 0.01) as well as a significant increase in MD and RD (FWE-p < 0.01) of the right anterior thalamic radiation, bilateral corticospinal tract, bilateral inferior longitudinal fasciculus, bilateral superior longitudinal fasciculus, bilateral inferior fronto-occipital fasciculus, bilateral cingulum, corpus callosum, and right anterior corona radiata, posterior limb of internal capsule, and retrolenticular part of internal capsule (Table 3 and Figure 2).
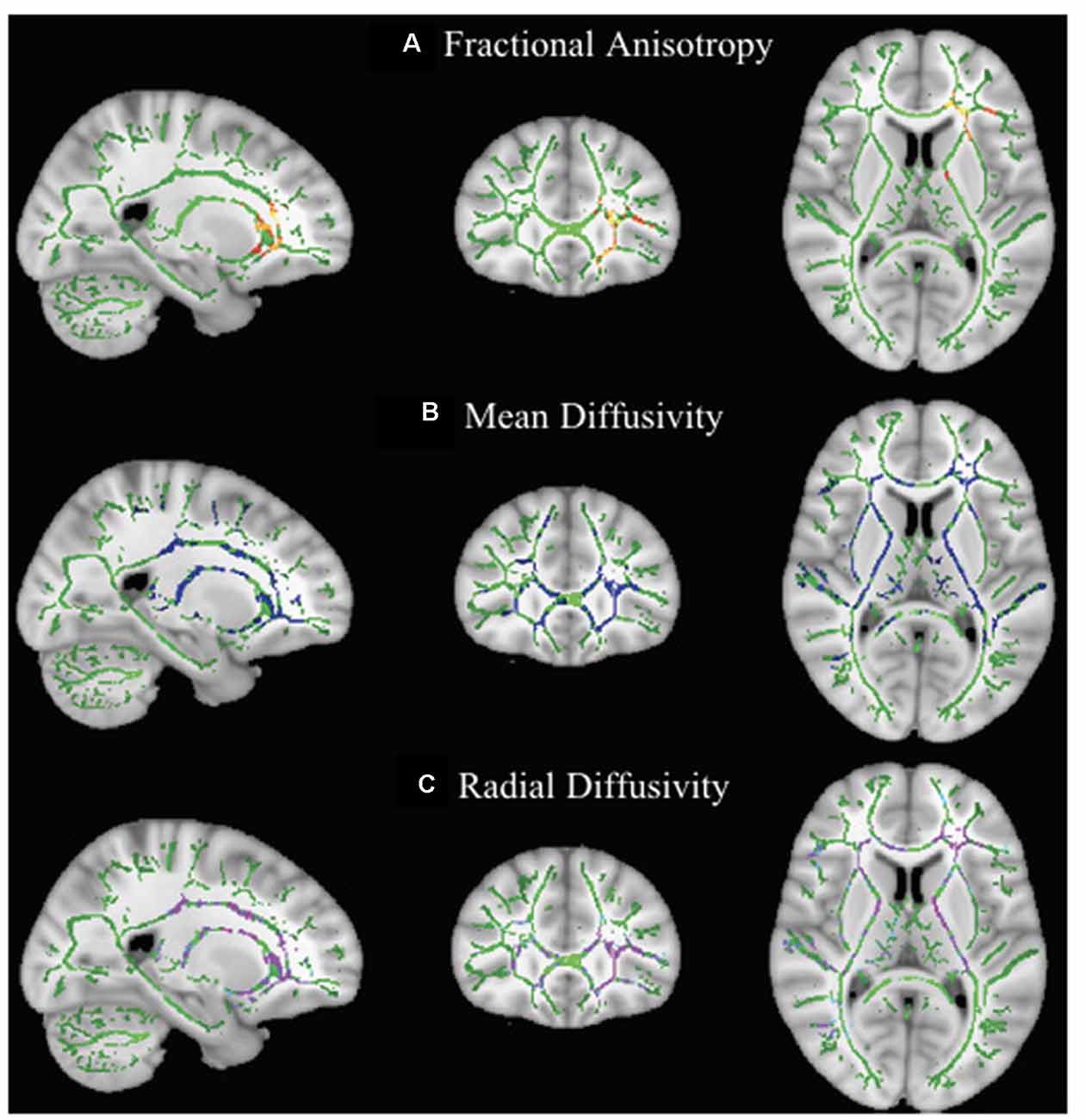
Figure 2. Effect of maturation on DTI parameters in children with DCD. In these images, the white matter skeleton is shown in green and brain structures with altered fractional anisotropy (FA; A), mean diffusivity (MD; B), and radial diffusivity (RD; C) are shown in red, blue, and purple, respectively.
Intervention Effect
Comparing pre-and post-intervention DTI parameters of 28 children with DCD showed a significant increase (FWE-p < 0.05) in FA of the bilateral anterior thalamic radiation, bilateral sensorimotor tract, bilateral cingulum, fornix, splenium and body of corpus callosum, right inferior fronto-occipital fasciculus, and white matter pathways to the bilateral inferior gyri, right middle frontal gyrus, frontal medial cortex, and left cuneus (Table 4 and Figure 3).
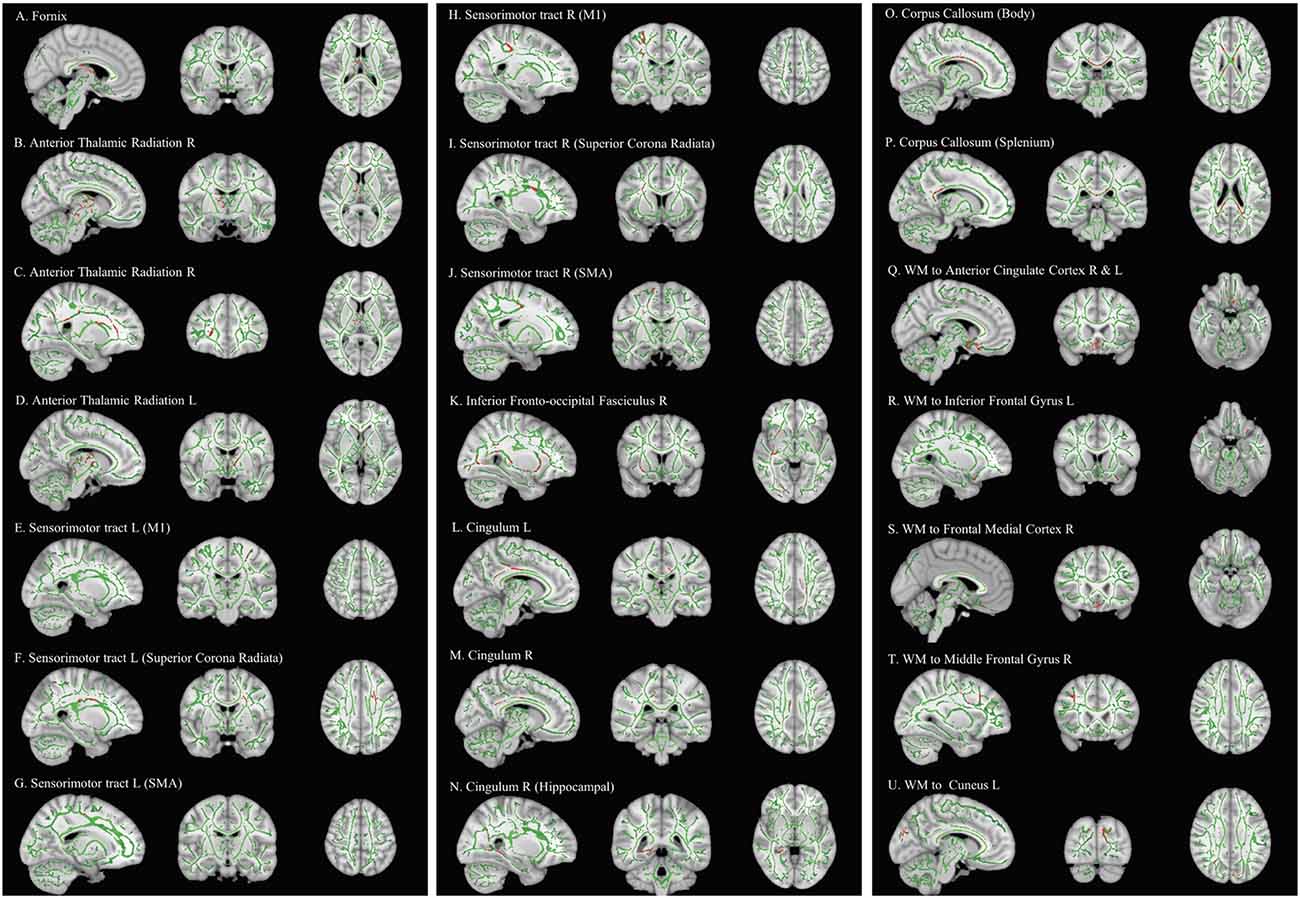
Figure 3. Effect of intervention on fractional anisotropy in children with DCD. In these images, the white matter skeleton is shown in green and brain structures with altered fractional anisotropy are shown in red. L, left; M1, primary motor cortex; R, right; SMA, supplementary motor area; WM, white matter.
Follow-up
Follow-up analysis of 10 children with DCD in the treatment group did not show any significant changes between post-intervention and follow-up MRI scans. We also did not find any significant changes from pre-intervention to follow-up scans in this small sub-sample.
Motor Outcomes
Regression analysis did not show any relationship between behavioral outcome measures (e.g., PQRS and BOT-2) and changes in white matter microstructure in children with DCD.
DCD + ADHD Group
Children with DCD + ADHD did not show any significant change (FWE-p > 0.05) in any of the DTI parameters in the 3-month period before CO-OP intervention (n = 10), immediately after the intervention (n = 25), or in the follow-up scan (n = 13). We did not find any relationship between motor performance and white matter parameters.
Discussion
Our results showed an increase in FA in white matter tracts, not associated with maturation, after CO-OP intervention in children with DCD, including the bilateral anterior thalamic radiation, bilateral sensorimotor tracts, right inferior fronto-occipital fasciculus, bilateral cingulum, fornix, and corpus callosum. During the first 3 months of the study prior to the CO-OP intervention, we observed an overall increase in RD and MD of the white matter microstructure as well as a reduction in FA of left anterior thalamic radiation in children with DCD. We showed that the CO-OP intervention reverted this pattern in children with DCD. Similar to our resting state results (Rinat et al., 2020), children with DCD + ADHD did not show any brain changes after the CO-OP intervention.
Rehabilitation-Induced Neuroplasticity in Children With DCD
Improved FA in white matter tracts is generally thought to reflect improved microstructural properties, such as axonal density and diameter, myelin integrity, or fiber coherence in these tracts (Concha, 2014); however, the specific change in microstructure cannot be identified. It is also important to note that FA values can be imprecise in regions where there are crossing fibers (O’Donnell and Westin, 2011). Notwithstanding these limitations, we showed that FA increased in the bilateral anterior thalamic radiations, bilateral sensorimotor tracts, right inferior fronto-occipital fasciculus, bilateral cingulum, fornix, corpus callosum, and white matter structures to anterior cingulate cortex and frontal lobe after CO-OP intervention in children with DCD. Our results align with preliminary results from a non-parametric combination of voxel-based morphometry and fractional anisotropy in the anterior thalamic radiation and the body and splenium of the corpus callosum after the CO-OP intervention (Izadi-Najafabadi et al., 2020). These regions play multiple roles in human behavior (e.g., emotion regulation, motor control, executive function). In what follows, we will explain how improved FA in each of these white matter tracts is related to the CO-OP intervention and its potential role in self-regulation, attention, and emotion regulation, as well as motor performance.
Attention and Self-regulation
Consistent with behavioral evidence of self-regulation mediating CO-OP’s effect (Jokić et al., 2013), our results suggest that CO-OP intervention might play a role in self- and attention-regulation. Most of our findings underlie the default mode network (DMN); anterior thalamic radiation, cingulum, corpus callosum, inferior fronto-occipital fasciculus, and white matter tracts to the cuneus and anterior cingulate cortex connect DMN regions in the posterior and anterior cingulate cortices, thalamus, cuneus, as well as across the two hemispheres (Teipel et al., 2010; Luo et al., 2012; Alves et al., 2019). DMN is involved in internally-directed attention, regulating attentional resources, and guiding self-regulatory processes (Bush et al., 2000; Grimm et al., 2009; Kelly et al., 2009; Wiebking et al., 2011; Dixon et al., 2014). The CO-OP intervention uses a global strategy of Goal-Plan-Do-Check where children set goals and motivate themselves, perform the task, observe their own performance, judge it to improve it, and then achieve their goal and express their self-satisfaction (Polatajko and Mandich, 2004), which is similar to the three phases of self-regulation (i.e., forethought, performance, and self-reflection) based on Triadic Model of Self-regulation (Zimmerman, 2000).
We have also shown that CO-OP intervention induces both increased FA in white matter structures underlying the DMN and increased functional connectivity between the DMN and the anterior cingulate cortex in this population (Rinat et al., 2020). High functional connectivity between regions of the DMN is related to their increased structural connectivity (van den Heuvel et al., 2008; Damoiseaux and Greicius, 2009), in particular, increased FA in the right and left cingulum (Bathelt et al., 2019).
Moreover, CO-OP intervention induces structural changes in other DMN white matter tracts, including the corona radiata (Izadi-Najafabadi et al., 2020). Based on our results from DTI analysis and other analysis (Izadi-Najafabadi et al., 2020; Rinat et al., 2020), we infer that the CO-OP intervention improves both functional and structural connectivity of the DMN, which is responsible for attention-and self-regulation (Shamloo and Helie, 2016; Reddy et al., 2018; Izadi-Najafabadi et al., 2020). We have previously suggested that these functions are implicated in children with DCD due to altered connectivity between the posterior cingulate cortex and DMN (Rinat et al., 2020).
CO-OP intervention improved white matter organization of the right inferior fronto-occipital fasciculus, right anterior thalamic radiation, and bilateral sensorimotor tracts. These regions are involved in various forms of attention-and self-regulation. The inferior fronto-occipital fasciculus guides sustained attention and other executive control functions (Leng et al., 2016). It is the only tract with a rightward asymmetry in our result, which is consistent with the right asymmetry for sustained attention (Pardo et al., 1991). Brown-Lum (2017) reported reduced FA in the right inferior fronto-occipital fasciculus in children with DCD. The white matter organization of the right inferior fronto-occipital fasciculus and the right anterior thalamic radiation are also associated with delayed discounting—the ability to tolerate delays while waiting for future rewards (Olson et al., 2009). Delayed discounting is a critical aspect of motivated behavior and self-regulatory skills, which are known to mediate the CO-OP intervention effects in children with DCD (Jokić et al., 2013).
The bilateral sensorimotor tracts immediately beneath the superior corona radiata transfer motor information from the primary motor cortex (M1) and supplementary motor area (SMA) through the corticospinal tract (Klöppel et al., 2008; Vergani et al., 2014). High FA in the superior corona radiata is also associated with improved focused and sustained attention (Stave et al., 2017) and has previously been reported in a different analysis of CO-OP-induced structural changes (Izadi-Najafabadi et al., 2020). The current analysis provides further evidence of the potential role of the CO-OP in attention regulation and motor execution.
Emotion Regulation
We found improved microstructure in regions involved in emotion regulation including the anterior thalamic radiation, SMA white matter, and inferior frontal gyrus white matter. Emotion regulation is a process of modifying emotion to increase or decrease emotional experience before, during, and after the motor performance (Beatty and Janelle, 2020). Accordingly, we suggest that the CO-OP intervention might help to regulate emotions required before, during, and after motor learning; the child-chosen nature of motor goals in CO-OP might increase emotional motivation prior to the motor performance, which is known to be the action starter (Beatty and Janelle, 2020). To achieve motor goals, CO-OP enables children to use strategies that distract them from negative emotions (e.g., frustration or embarrassment of their inability to perform a task) during motor skill acquisition. For example, when children use the domain-specific strategy of “attention to doing” during CO-OP, they focus more on their action rather than their feeling. Lastly, when children achieve their motor goals, they experience satisfaction; all these together enhance the likelihood of success in future motor performance and helps with emotion regulation (Beatty and Janelle, 2020). Moreover, similar to a key feature of CO-OP (Missiuna et al., 2001), emotions could be regulated when guided by a significant adult (e.g., therapist or parents; Williams et al., 2020).
The anterior thalamic radiation is known for its role in emotional processes and low FA in this structure is related to sadness (Coenen et al., 2012; Jia et al., 2014; Niida et al., 2018). Anterior parts of the cingulum, terminating in the frontal lobe, are also involved in attention and executive function (Takahashi et al., 2010; Chiang et al., 2016). The anterior thalamic radiation along with the cingulum are considered parts of the Papez circuit, involved in emotional processing, semantic memory, and learning (Papez, 1937; Jang and Yeo, 2013; Bubb et al., 2017; Weininger et al., 2019). Extremely high or low levels of inputs from the anterior thalamic nuclei to the anterior cingulate cortex through the anterior thalamic radiation and the cingulum could increase emotional distraction of behavior (Hartikainen et al., 2014; Sun et al., 2015) and overwhelm attentional resources required for learning (Öhman et al., 2001; Hartikainen et al., 2014). As such, we infer that CO-OP intervention might regulate emotions by allocating more attentional resources to motor performance, which is reflected in neuroplastic changes in white matter microstructure. This interpretation should be considered with caution considering that we did not assess emotion and attention regulation before and after the intervention.
FA in the white matter tract to the right inferior frontal gyrus is involved in executive functions, detection of cues (Hampshire et al., 2010), and fine movement control (Liakakis et al., 2011). The inferior frontal gyrus has structural connectivity with the SMA (Kohn et al., 2014; Vergani et al., 2014), which mediates overt and covert speech initiation (Winsler et al., 2009), as a means of emotion regulation (Beatty and Janelle, 2020). CO-OP uses self-verbalization in the form of overt and covert/inner speech production as a global strategy to solve motor problems (Missiuna et al., 2001) and children’s verbal ability predicts their motor outcomes after the CO-OP (Green et al., 2008). Self-verbalization strategies of CO-OP (e.g., global strategy of Goal-Plan-Do-Check and domain-specific strategies of verbal motor mnemonic and verbal rote script; Polatajko and Mandich, 2004) may motivate children by providing effective instructions and selectively attending to the performance-relevant information rather than negative emotions (Anderson, 1997; Beatty and Janelle, 2020). Self-verbalization helps to internalize instructions and use them to acquire the skills (Anderson, 1997). Accordingly, the observed increase in FA of white matter structures underlying the SMA and inferior frontal gyrus might be associated with CO-OP’s extensive use of self-verbalization strategy as a regulatory technique.
The SMA also plays a role in planning and executing a motor function. It has structural connectivity with the cingulate gyrus (Vergani et al., 2014), transforming emotion into motor experience in situations of reward or punishments (Northoff et al., 2000; Oliveri et al., 2003; Kohn et al., 2014). Taken together, our findings suggest that the CO-OP intervention may play a role in emotion regulation as well. More research is required to better understand CO-OP’s role in emotion regulation at behavioral and neural levels.
Motor Performance
We also found increased FA in the bilateral sensorimotor tracts immediately beneath the right and left M1, as well as left SMA and parts of the right and left superior corona radiata. Since we did not perform tractography, we are not able to distinguish between corticospinal tracts and corticobulbar tracts originating from sensorimotor cortical areas. In looking at regional microstructure, increased regional FA in the subcortical white matter areas could be explained in an intra-hemispheric or inter-hemispheric context. As for intra-hemispheric improvement, high regional FA underneath M1 might indicate high functional connectivity between M1 and secondary motor areas in each hemisphere (Klöppel et al., 2008; Vergani et al., 2014), especially in the left hemisphere lateralized for motor control (Guye et al., 2003). However, our resting state results did not show such increased functional connectivity (Izadi-Najafabadi et al., 2020). This discrepancy between our structural and functional results might have different explanations. First, it could be due to a smaller sample size in our resting state analysis or a false positive result in our DTI results. Also, considering the small effect size in the sensorimotor tracts (Cohen’s D range: 0.18–0.35), these results should be interpreted with caution. Second, it could be related to the unique role of brain function and structure in supporting a specific behavior (Zimmermann et al., 2018). Although structural and functional connectivity are equally important in understanding behavior, they capture independent and complementary features of the brain and do not necessarily show spatial overlap (Zimmermann et al., 2018). Further studies are required to better understand the relationship between brain function/ structure and motor behavior in children with DCD.
Increased FA in the bilateral sensorimotor tracts immediately beneath M1 and SMA may be indicative of improved structural connectivity between motor-related brain regions. A transcranial magnetic stimulation study showed that water diffuses from M1 to the subcortical white matter below the prefrontal areas, as well as parts of the corona radiata, internal capsule, cerebral peduncles, and corpus callosum (Klöppel et al., 2008). Also, the SMA sends fibers through the corpus callosum and through the corona radiata to join the corticospinal tract (Vergani et al., 2014). Similarly, we found increased FA in the subcortical white matter immediately underneath M1, SMA, middle and inferior frontal gyrus, frontal medial cortex, as well as parts of the superior corona radiata and corpus callosum. Therefore, we suggest that CO-OP intervention increases FA in the subcortical white matter below motor cortices, facilitates cortico-cortical connectivity between M1 and SMA and/or corticospinal connectivity; this can subsequently improve planning, initiating, and executing a motor task. This interpretation should be considered with caution and further analysis using tractography and graph theory is required to better understand the nature of increased FA in these subcortical white matter structures.
As for inter-hemispheric improvement in FA, right and left M1 and SMA are connected through transcallosal sensorimotor fibers that facilitate interhemispheric inhibition (Fling et al., 2013; Vergani et al., 2014). FA of these transcallosal sensorimotor fibers at the superior portion of the corticospinal tracts are positively correlated to motor ability of children as assessed by MABC-2 (Grohs et al., 2018). Motor learning, especially during bimanual movements, is known to enhance inter-hemispheric interactions (Takeuchi et al., 2012); in our study, all children had chosen at least one bimanual motor goal, such as tying shoelaces or cutting a fruit with a knife, which might explain the improved microstructure of transcallosal sensorimotor fibers.
We did not find any relationship between motor performance and microstructural changes while we had previously reported a relationship between improved motor performance and functional connectivity of the cerebellar lobule I-IV and the DMN related to automatization (Izadi-Najafabadi et al., 2020). Evidence suggests that the brain-behavior relationship is explained differently when considering brain function vs. brain structure. In a large study investigating the whole-brain structural connectivity-behavior relationship vs. functional connectivity-behavior, there were fewer structural connections that were linked to specific behaviors than functional connectivity. And, unlike functional connectivity, a general pattern of structural connectivity is linked to overall cognitive abilities (Zimmermann et al., 2018). These findings may explain why we found a functional connectivity-motor relationship in our results while we did not find such a specific relationship using our microstructural measures. Moreover, we suggested that CO-OP was associated with improved microstructural properties of regions involved in self-regulation, attention regulation, and emotion regulation; accordingly, we may not have found a brain-behavior relationship because we did not assess these mediatory behaviors.
Theories to Support Interpretation of Findings
Our results and interpretation of the role of self-regulation, attention, and emotion regulation on motor performance are consistent with the “sensorimotor control framework for emotion regulation” by Williams et al. (2020) and the “Optimizing Performance through Intrinsic Motivation and Attention for Learning (OPTIMAL) theory of motor learning" by Wulf and Lewthwaite (2016).
The sensorimotor control framework for emotion regulation is similar to the internal modeling hypothesis (Adams et al., 2014), with an added component of emotion. Accordingly, all actions start with a motivation or emotional value. To achieve this desired goal, children have to form internal sensorimotor models over time and to learn and plan their movements based on feedback-feedforward processes (Williams et al., 2020). Children may achieve their goal or fail, which engages their emotional response again and modulates their actions; strategies such as avoiding, selective attention, and reappraisal are used to regulate emotions until the child achieves the goal (Braunstein et al., 2017). The OPTIMAL theory of motor learning emphasizes the role of emotions and motivation in motor performance (Wulf and Lewthwaite, 2016). This theory predicts a virtuous vs. a vicious cycle in motor learning; in the virtuous cycle, motivation leads to better motor performance, a sense of autonomy, and self-efficacy, which in turn, motivates the individual to further accomplish motor goals. The vicious cycle, on the other hand, predicts that low motivation results in low motor performance and vice versa (Wulf and Lewthwaite, 2016).
Atypical Maturation in Children With DCD
The pattern of brain maturation prior to the intervention in children with DCD contradicts existing reports of longitudinal development in typically developing children and adolescents; typically, white matter microstructure develops with increased FA and decreased MD and RD (Chen et al., 2016; Krogsrud et al., 2016). The observed reduction in FA and increase in MA and RD in this study suggests an overall decline in brain microstructure in children with DCD over a 3-month period. Reduced FA in the left anterior thalamic radiation in children with DCD compared to typically developing children has previously been reported (Brown-Lum, 2017). The current study is the first to report a longitudinal decrease in FA of this white matter structure in children with DCD, suggesting atypical brain development/maturation might underlie their motor performance difficulty.
In a different analysis, we were not able to find any maturational changes in white matter FA and/or volume in a sample of 12 children with DCD +/– ADHD (Izadi-Najafabadi et al., 2020). The smaller sample size may have reduced the statistical power to detect brain changes, and the inclusion of children with DCD + ADHD could have confounded the results.
In addition, we were not able to find any maturational changes in the white matter microstructure of children with DCD + ADHD prior to the intervention, indicating that their brain development does not follow a typical trajectory. This finding should be interpreted with caution as this might be due to a smaller sample size (DCD: n = 18; DCD + ADHD: n = 10) and reduced statistical power.
No Observed Neuroplasticity in Children With DCD + ADHD
Although CO-OP improved the motor performance of children with DCD + ADHD (Izadi-Najafabadi et al., under review), they did not show any changes in white matter microstructure after the intervention. This is in line with Green et al.’s (2008) study indicating the benefits of CO-OP for children with co-occurring conditions such as ADHD while still experiencing motor problems. Our results are explained considering three evidence-informed statements: (1) the CO-OP intervention relies on attention and self-regulation to mediate its effects on motor performance (Jokić et al., 2013); (2) attention contribution to motor performance is greater in children with DCD + ADHD than children with DCD only (Fong et al., 2016); (3) children with ADHD experience greater difficulty with self-regulation (e.g., attention regulation, emotion regulation; Shiels and Hawk, 2010). In other words, the CO-OP intervention is not able to overcome the attentional and self-regulatory difficulties and its underlying neural mechanism in children with DCD + ADHD. Moreover, children with DCD + ADHD have shown unique brain structure (Langevin et al., 2014, 2015) and function (McLeod et al., 2014, 2016; Thornton et al., 2018) compared to children with DCD. Compensatory attentional control by the posterior cingulate cortex and the cerebellum has been proposed as the neural mechanism of motor coordination in children with DCD + ADHD (Yeh et al., 2012); however, our resting state (Izadi-Najafabadi et al., in press), morphometry (Izadi-Najafabadi et al., 2020), and diffusion imaging analyses did not show similar neural mechanism following the CO-OP.
Additionally, literature suggests that only hyperactivity symptoms of children with ADHD improve over time, and this improvement is highly correlated with reduced FA in the left corticospinal tract (Francx et al., 2015); it is, therefore, hypothesized that development induces a less stimulated/excited motor pathway with decreased FA, which reduces the motor hyperactivity in children with ADHD (Francx et al., 2015). On the other hand, reduced FA in white matter microstructure over time, especially in the frontal and parietal regions, could reflect poorer motor performance as seen in children with DCD (Brown-Lum et al., 2020) and DCD + ADHD (Langevin et al., 2014). Thus, it is unclear whether a reduced FA in the corticospinal tract is beneficial or detrimental in children with ADHD. This highlights how a co-occurring condition such as ADHD complicates our understanding of brain and neuroplasticity following the intervention.
Taken together, some modifications to the CO-OP protocol to better address the attentional needs of children with ADHD (Gharebaghy et al., 2015; Izadi-Najafabadi et al., 2020) or combining CO-OP intervention with medication or other self-regulatory interventions (Reid et al., 2005) might improve its effectiveness and induce permanent brain changes in children with DCD + ADHD.
Limitations
A smaller than anticipated sample size for the analysis could have biased our results. This study is the first study to use DTI to investigate training-induced brain changes in children with DCD; however, we only performed a voxel-wise analysis of the white matter microstructure, which has spatial inaccuracy (Edden and Jones, 2011). TBSS assumes an accurate registration of the data and it is nearly impossible to detect errors (Jones and Cercignani, 2010); this could add some uncertainty to the results. Further analysis using myelin water fraction analysis, tractography (probabilistic or constrained spherical deconvolution), and graph theory would help to validate our results. In addition, although we conducted the strictest available clean-up pipeline through FSL and excluded any residual motion-contaminated volumes from our data, we did not collect field map data, which would have further cleaned our data and better corrected for the susceptibility-induced distortions. Moreover, we did not find a relationship between behavioral data and white matter microstructure, meaning that behavioral interpretations should be considered with caution.
Conclusions
Our results indicate that the CO-OP intervention induces microstructural changes in white matter tracts involved in self-, attention-, and emotion-regulation and white matter structures involved in intra-and inter-hemispheric transfer of motor information. These changes were maintained 3 months after the intervention. However, children with a dual diagnosis of DCD and ADHD did not show any microstructural changes following CO-OP intervention, suggesting their different needs in motor-based interventions. These results are consistent with our functional connectivity results of improved motor performance and associated increases in functional connectivity of DMN with the pACC after CO-OP intervention in children with DCD, but not in children with a dual diagnosis of DCD and ADHD (Izadi-Najafabadi et al., 2020).
Data Availability Statement
The raw data supporting the conclusions of this article will be made available by the authors, without undue reservation.
Ethics Statement
The studies involving human participants were reviewed and approved by University of British Columbia/BC Children’s and Women’s Research Ethics Board. Written informed consent to participate in this study was provided by the participants’ parent/legal guardian. Child assent was also obtained.
Author Contributions
SI was responsible for participant screening, data collection, data processing, statistical data analysis, data interpretation, and drafting the manuscript. JZ conceived and designed the study, coordinated and supervised data collection, and critically reviewed the draft manuscript for intellectual content. All authors contributed to the article and approved the submitted version.
Funding
The research project was funded by the Canadian Institutes of Health Research (PI- JZ FDN-143258). SI was funded by the Brain Canada and NeuroDevNet Developmental Neuroscience Research Training Award and a UBC Four-Year Fellowship (4YF), University of British Columbia. JZ was funded by a Michael Smith Foundation for Health Research Scholar Award (#5762) and Canadian Child Health Clinician Scientist Program Career Development/Enhancement Award and is currently funded by the BC Children’s Hospital Research Institute, Sunny Hill Foundation, and Canadian Institutes of Health Research (CIHR 201512MSH-360883-141638).
Conflict of Interest
The authors declare that the research was conducted in the absence of any commercial or financial relationships that could be construed as a potential conflict of interest.
Acknowledgments
We thank all the families and children who participated in our study—we are truly grateful for your contribution. We would like to thank Meisan Brown-Lum, Kamaldeep Gill, Shie Rinat, Noelle Dalin, Gisela Gosse, Janet Rigney, and Alyssa Barrie for their assistance with data collection and data management, and the many occupational therapists who provided the intervention. We graciously acknowledge Dr. Deborah Giaschi aand Dr. Liisa Holsti for their feedback on previous iterations of this manuscript.
References
Adams, I. L., Lust, J. M., Wilson, P. H., and Steenbergen, B. (2014). Compromised motor control in children with DCD: a deficit in the internal model?–a systematic review. Neurosci. Biobehav. Rev. 47, 225–244. doi: 10.1016/j.neubiorev.2014.08.011
Alves, P. N., Foulon, C., Karolis, V., Bzdok, D., Margulies, D. S., Volle, E., et al. (2019). Subcortical anatomy of the default mode network: a functional and structural connectivity study. bioRxiv [Preprint]. doi: 10.1101/528679
American Psychiatric Association. (2013). Diagnostic and Statistical Manual of Mental Disorders—5th edition (DSM-5). Washington, DC: American Psychiatric Association.
Anderson, A. (1997). Learning strategies in physical education: self-talk, imagery and goal-setting. J. Phys. Educ. Recreat. Dance 68, 30–35. doi: 10.1080/07303084.1997.10604874
Andersson, J. L., Graham, M. S., Drobnjak, I., Zhang, H., Filippini, N., and Bastiani, M. (2017). Towards a comprehensive framework for movement and distortion correction of diffusion MR images: within volume movement. NeuroImage 152, 450–466. doi: 10.1016/j.neuroimage.2017.02.085
Andersson, J. L., Graham, M. S., Zsoldos, E., and Sotiropoulos, S. N. (2016). Incorporating outlier detection and replacement into a non-parametric framework for movement and distortion correction of diffusion MR images. NeuroImage 141, 556–572. doi: 10.1016/j.neuroimage.2016.06.058
Andersson, J. L., and Sotiropoulos, S. N. (2016). An integrated approach to correction for off-resonance effects and subject movement in diffusion MR imaging. NeuroImage 125, 1063–1078. doi: 10.1016/j.neuroimage.2015.10.019
Archer, D. B., Vaillancourt, D. E., and Coombes, S. A. (2018). A template and probabilistic atlas of the human sensorimotor tracts using diffusion MRI. Cereb. Cortex 28, 1685–1699. doi: 10.1093/cercor/bhx066
Barkley, R. A. (2014). Attention-Deficit Hyperactivity Disorder: A Handbook for Diagnosis and Treatment. New York, NY: Guilford Publications.
Bart, O., Podoly, T., and Bar-Haim, Y. (2010). A preliminary study on the effect of methylphenidate on motor performance in children with comorbid DCD and ADHD. Res. Dev. Disabil. 31, 1443–1447. doi: 10.1016/j.ridd.2010.06.014
Bastiani, M., Cottaar, M., Fitzgibbon, S. P., Suri, S., Alfaro-Almagro, F., Sotiropoulos, S. N., et al. (2019). Automated quality control for within and between studies diffusion MRI data using a non-parametric framework for movement and distortion correction. NeuroImage 184, 801–812. doi: 10.1016/j.neuroimage.2018.09.073
Bathelt, J., Johnson, A., Zhang, M., and Astle, D. E. (2019). The cingulum as a marker of individual differences in neurocognitive development. Sci. Rep. 9, 1–16. doi: 10.1038/s41598-019-38894-z
Beatty, G. F., and Janelle, C. M. (2020). Emotion regulation and motor performance: an integrated review and proposal of the temporal influence model of emotion regulation (TIMER). Int. Rev. Sport Exerc. Psychol. 13, 266–296. doi: 10.1080/1750984X.2019.1695140
Beaulieu, C. (2002). The basis of anisotropic water diffusion in the nervous system-a technical review. NMR Biomed. 15, 435–455. doi: 10.1002/nbm.782
Biotteau, M., Chaix, Y., Blais, M., Tallet, J., Péran, P., and Albaret, J. (2016). Neural signature of DCD: a critical review of MRI neuroimaging studies. Front. Neurol. 7:227. doi: 10.3389/fneur.2016.00227
Blakemore, S., Frith, C. D., and Wolpert, D. M. (2001). The cerebellum is involved in predicting the sensory consequences of action. Neuroreport 12, 1879–1884. doi: 10.1097/00001756-200107030-00023
Blakemore, S., and Sirigu, A. (2003). Action prediction in the cerebellum and in the parietal lobe. Exp. Brain Res. 153, 239–245. doi: 10.1007/s00221-003-1597-z
Blank, R., Barnett, A. L., Cairney, J., Green, D., Kirby, A., Polatajko, H., et al. (2019). International clinical practice recommendations on the definition, diagnosis, assessment, intervention and psychosocial aspects of developmental coordination disorder. Dev. Med. Child Neurol. 61, 242–285. doi: 10.1111/dmcn.14132
Braunstein, L. M., Gross, J. J., and Ochsner, K. N. (2017). Explicit and implicit emotion regulation: a multi-level framework. Soc. Cogn. Affect. Neurosci. 12, 1545–1557. doi: 10.1093/scan/nsx096
Brossard-Racine, M., Shevell, M., Snider, L., Bélanger, S. A., and Majnemer, A. (2012). Motor skills of children newly diagnosed with attention deficit hyperactivity disorder prior to and following treatment with stimulant medication. Res. Dev. Disabil. 33, 2080–2087. doi: 10.1016/j.ridd.2012.06.003
Brown-Lum, M., Izadi-Najafabadi, S., Oberlander, T. F., Rauscher, A., and Zwicker, J. G. (2020). Differences in white matter microstructure among children with developmental coordination disorder. JAMA Netw. Open 3:e201184. doi: 10.1001/jamanetworkopen.2020.1184
Brown-Lum, M., and Zwicker, J. G. (2015). Brain imaging increases our understanding of developmental coordination disorder: a review of literature and future directions. Curr. Dev. Disord. Rep. 2, 131–140. doi: 10.1007/s40474-015-0046-6
Brown-Lum, M. (2017). Characterizing the Neural Correlates of Children With Developmental Coordination Disorder Using Diffusion Tensor Imaging. Canada: University of British Columbia.
Bruininks, R., and Bruininks, B. (2005). Bruininks-Oseretsky Test of Motor Proficiency. Minneapolis, MN: NCS Pearson.
Bubb, E. J., Kinnavane, L., and Aggleton, J. P. (2017). Hippocampal-diencephalic-cingulate networks for memory and emotion: an anatomical guide. Brain Neurosci Adv 1:2398212817723443. doi: 10.1177/2398212817723443
Bush, G., Luu, P., and Posner, M. I. (2000). Cognitive and emotional influences in anterior cingulate cortex. Trends Cogn Sci 4, 215–222. doi: 10.1016/s1364-6613(00)01483-2
Cairney, J., Hay, J. A., Faught, B. E., Wade, T. J., Corna, L., and Flouris, A. (2005). Developmental coordination disorder, generalized self-efficacy toward physical activity and participation in organized and free play activities. J. Pediatr. 147, 515–520. doi: 10.1016/j.jpeds.2005.05.013
Cairney, J., Hay, J. A., Veldhuizen, S., Missiuna, C., and Faught, B. E. (2010). Developmental coordination disorder, sex and activity deficit over time: a longitudinal analysis of participation trajectories in children with and without coordination difficulties. Dev. Med. Child Neurol. 52, e67–e72. doi: 10.1111/j.1469-8749.2009.03520.x
Cairney, J., and Veldhuizen, S. (2013). Is developmental coordination disorder a fundamental cause of inactivity and poor health-related fitness in children? Dev. Med. Child Neurol. 55, 55–58. doi: 10.1111/dmcn.12308
Capistran, J., and Martini, R. (2016). Exploring inter-task transfer following a CO-OP approach with four children with DCD: a single subject multiple baseline design. Hum. Mov. Sci. 49, 277–290. doi: 10.1016/j.humov.2016.07.004
Chen, Z., Zhang, H., Yushkevich, P. A., Liu, M., and Beaulieu, C. (2016). Maturation along white matter tracts in human brain using a diffusion tensor surface model tract-specific analysis. Front. Neuroanat. 10:9. doi: 10.3389/fnana.2016.00009
Chiang, H., Chen, Y., Shang, C., Tseng, W. I., and Gau, S. S. (2016). Different neural substrates for executive functions in youths with ADHD: a diffusion spectrum imaging tractography study. Psychol. Med. 46, 1225–1238. doi: 10.1017/S0033291715002767
Coenen, V. A., Panksepp, J., Hurwitz, T. A., Urbach, H., and Mädler, B. (2012). Human medial forebrain bundle (MFB) and anterior thalamic radiation (ATR): imaging of two major subcortical pathways and the dynamic balance of opposite affects in understanding depression. J. Neuropsychiatry Clin. Neurosci. 24, 223–236. doi: 10.1176/appi.neuropsych.11080180
Concha, L. (2014). A macroscopic view of microstructure: using diffusion-weighted images to infer damage, repair and plasticity of white matter. Neurosci 276, 14–28. doi: 10.1016/j.neuroscience.2013.09.004
Crane, L., Sumner, E., and Hill, E. L. (2017). Emotional and behavioural problems in children with developmental coordination disorder: exploring parent and teacher reports. Res. Dev. Disabil. 70, 67–74. doi: 10.1016/j.ridd.2017.08.001
Damoiseaux, J. S., and Greicius, M. D. (2009). Greater than the sum of its parts: a review of studies combining structural connectivity and resting-state functional connectivity. Brain Struct. Funct. 213, 525–533. doi: 10.1007/s00429-009-0208-6
Debrabant, J., Vingerhoets, G., Van Waelvelde, H., Leemans, A., Taymans, T., and Caeyenberghs, K. (2016). Brain connectomics of visual-motor deficits in children with developmental coordination disorder. J. Pediatr. 169, 21–27.e2. doi: 10.1016/j.jpeds.2015.09.069
Dewey, D., Kaplan, B., Crawford, S., and Wilson, B. (2002). Developmental coordination disorder: associated problems in attention, learning and psychosocial adjustment. Hum. Mov. Sci. 21, 905–918. doi: 10.1016/s0167-9457(02)00163-x
Dewey, D., and Volkovinskaia, A. (2018). Health-related quality of life and peer relationships in adolescents with developmental coordination disorder and attention-deficit-hyperactivity disorder. Dev. Med. Child Neurol. 60, 711–717. doi: 10.1111/dmcn.13753
Dewey, D., Wilson, B., Crawford, S., and Kaplan, B. (2000). Comorbidity of developmental coordination disorder with ADHD and reading disability. J. Int. Neuropsychol. Soc. 6:152. doi: 10.1017/S1355617700233146
Dixon, M. L., Fox, K. C., and Christoff, K. (2014). A framework for understanding the relationship between externally and internally directed cognition. Neuropsychologia 62, 321–330. doi: 10.1016/j.neuropsychologia.2014.05.024
Edden, R. A., and Jones, D. K. (2011). Spatial and orientational heterogeneity in the statistical sensitivity of skeleton-based analyses of diffusion tensor MR imaging data. J. Neurosci. Methods 201, 213–219. doi: 10.1016/j.jneumeth.2011.07.025
Fliers, E., Franke, B., and Buitelaar, J. K. (2011). Motor problems in children with ADHD receive too little attention in clinical practice. Ned. Tijdschr. Geneeskd. 155:A3559.
Fliers, E., Franke, B., Lambregts-Rommelse, N. N., Altink, M. E., Buschgens, C. J., Nijhuis-van der Sanden, M. W. G., et al. (2010). Undertreatment of motor problems in children with ADHD. Child Adolesc. Ment. Health 15, 85–90. doi: 10.1111/j.1475-3588.2009.00538.x
Fliers, E., Vermeulen, S., Rijsdijk, F., Altink, M., Buschgens, C., Rommelse, N., et al. (2009). ADHD and poor motor performance from a family genetic perspective. J. Am. Acad. Child Adolesc. Psychiatry 48, 25–34. doi: 10.1097/CHI.0b013e31818b1ca2
Fling, B. W., Benson, B. L., and Seidler, R. D. (2013). Transcallosal sensorimotor fiber tract structure-function relationships. Hum. Brain Mapp. 34, 384–395. doi: 10.1002/hbm.21437
Fong, S. S., Chung, J. W., Cheng, Y. T., Yam, T. T., Chiu, H. C., Fong, D. Y., et al. (2016). Attention during functional tasks is associated with motor performance in children with developmental coordination disorder: a cross-sectional study. Medicine 95:e4935. doi: 10.1097/MD.0000000000004935
Francx, W., Zwiers, M. P., Mennes, M., Oosterlaan, J., Heslenfeld, D., Hoekstra, P. J., et al. (2015). White matter microstructure and developmental improvement of hyperactive/impulsive symptoms in attention-deficit/hyperactivity disorder. J. Child Psychol. Psychiatry 56, 1289–1297. doi: 10.1111/jcpp.12379
Gharebaghy, S., Rassafiani, M., and Cameron, D. (2015). Effect of cognitive intervention on children with ADHD. Phys. Occup. Ther. Pediatr. 35, 13–23. doi: 10.3109/01942638.2014.957428
Green, D., Baird, G., and Sugden, D. (2006). A pilot study of psychopathology in developmental coordination disorder. Child Care Health Dev. 32, 741–750. doi: 10.1111/j.1365-2214.2006.00684.x
Green, D., Chambers, M., and Sugden, D. (2008). Does subtype of developmental coordination disorder count: is there a differential effect on outcome following intervention? Hum. Mov. Sci. 27, 363–382. doi: 10.1016/j.humov.2008.02.009
Grimm, S., Boesiger, P., Beck, J., Schuepbach, D., Bermpohl, F., Walter, M., et al. (2009). Altered negative BOLD responses in the default-mode network during emotion processing in depressed subjects. Neuropsychopharmacology 34, 932–943. doi: 10.1038/npp.2008.81
Grohs, M. N., Reynolds, J. E., Dewey, D., and Lebel, C. (2018). Corpus callosum microstructure is associated with motor function in preschool children. NeuroImage 183, 828–835. doi: 10.1016/j.neuroimage.2018.09.004
Guye, M., Parker, G. J. M., Symms, M., Boulby, P., Wheeler-Kingshott, C. A. M., Salek-Haddadi, A., et al. (2003). Combined functional MRI and tractography to demonstrate the connectivity of the human primary motor cortex in vivo. NeuroImage 19, 1349–1360. doi: 10.1016/s1053-8119(03)00165-4
Hampshire, A., Chamberlain, S. R., Monti, M. M., Duncan, J., and Owen, A. M. (2010). The role of the right inferior frontal gyrus: inhibition and attentional control. NeuroImage 50, 1313–1319. doi: 10.1016/j.neuroimage.2009.12.109
Harrowell, I., Hollén, L., Lingam, R., and Emond, A. (2017). Mental health outcomes of developmental coordination disorder in late adolescence. Dev. Med. Child Neurol. 59, 973–979. doi: 10.1111/dmcn.13469
Hartikainen, K. M., Sun, L., Polvivaara, M., Brause, M., Lehtimäki, K., Haapasalo, J., et al. (2014). Immediate effects of deep brain stimulation of anterior thalamic nuclei on executive functions and emotion-attention interaction in humans. J. Clin. Exp. Neuropsychol. 36, 540–550. doi: 10.1080/13803395.2014.913554
Hellgren, L., Gillberg, C. I., Bågenholm, A., and Gillberg, C. (1994). Children with deficits in attention, motor control and perception (DAMP) almost grown up: psychiatric and personality disorders at age 16 years. J. Child Psychol. Psychiatry 35, 1255–1271. doi: 10.1111/j.1469-7610.1994.tb01233.x
Henderson, S. E., Sugden, D. A., and Barnett, A. L. (2007). Movement Assessment Battery for Children -2nd edition. London: Psychological Corporation.
Hill, E. L., and Brown, D. (2013). Mood impairments in adults previously diagnosed with developmental coordination disorder. J. Ment. Health 22, 334–340. doi: 10.3109/09638237.2012.745187
Hua, K., Zhang, J., Wakana, S., Jiang, H., Li, X., Reich, D. S., et al. (2008). Tract probability maps in stereotaxic spaces: analyses of white matter anatomy and tract-specific quantification. NeuroImage 39, 336–347. doi: 10.1016/j.neuroimage.2007.07.053
Hyde, C., Fuelscher, I., Enticott, P. G., Jones, D. K., Farquharson, S., Silk, T. J., et al. (2019). White matter organization in developmental coordination disorder: a pilot study exploring the added value of constrained spherical deconvolution. NeuroImage Clin. 21:101625. doi: 10.1016/j.nicl.2018.101625
Izadi-Najafabadi, S., Gill, K., and Zwicker, J. G. (2020). Training-induced neuroplasticity in children with developmental coordination disorder. Curr. Dev. Dis. Rep. 7, 48–58. doi: 10.1007/s40474-020-00191-0
Izadi-Najafabadi, S., Rinat, S., and Zwicker, J. G. (in press). Effect of rehabilitation intervention on brain functional connectivity in children with developmental coordination disorder: a randomized controlled trial. Pediatr. Res.
Jang, S. H., and Yeo, S. S. (2013). Thalamocortical tract between anterior thalamic nuclei and cingulate gyrus in the human brain: diffusion tensor tractography study. Brain Imaging Behav. 7, 236–241. doi: 10.1007/s11682-013-9222-7
Jia, Z., Wang, Y., Huang, X., Kuang, W., Wu, Q., Lui, S., et al. (2014). Impaired frontothalamic circuitry in suicidal patients with depression revealed by diffusion tensor imaging at 3.0 T. J. Psychiatry Neurosci. 39, 170–177. doi: 10.1503/jpn.130023
Jokić, C. S., Polatajko, H., and Whitebread, D. (2013). Self-regulation as a mediator in motor learning: the effect of the cognitive orientation to occupational performance approach on children with DCD. Adapt. Phys. Activ. Q. 30, 103–126. doi: 10.1123/apaq.30.2.103
Jones, D. K., and Cercignani, M. (2010). Twenty-five pitfalls in the analysis of diffusion MRI data. NMR Biomed. 23, 803–820. doi: 10.1002/nbm.1543
Jones, D. K., Knösche, T. R., and Turner, R. (2013). White matter integrity, fiber count and other fallacies: the do’s and don’ts of diffusion MRI. NeuroImage 73, 239–254. doi: 10.1016/j.neuroimage.2012.06.081
Kadesjo, B., and Gillberg, C. (1999). Developmental coordination disorder in Swedish 7-year-old children. J. Am. Acad. Child Adolesc. Psychiatry 38, 820–828. doi: 10.1097/00004583-199907000-00011
Kaiser, M., Schoemaker, M., Albaret, J., and Geuze, R. (2015). What is the evidence of impaired motor skills and motor control among children with attention deficit hyperactivity disorder (ADHD)? systematic review of the literature. Res. Dev. Disabil. 36, 338–357. doi: 10.1016/j.ridd.2014.09.023
Kawato, M., and Gomi, H. (1992). A computational model of four regions of the cerebellum based on feedback-error learning. Biol. Cybern. 68, 95–103. doi: 10.1007/BF00201431
Kelly, A. C., Di Martino, A., Uddin, L. Q., Shehzad, Z., Gee, D. G., Reiss, P. T., et al. (2009). Development of anterior cingulate functional connectivity from late childhood to early adulthood. Cereb. Cortex 19, 640–657. doi: 10.1093/cercor/bhn117
Klöppel, S., Bäumer, T., Kroeger, J., Koch, M. A., Büchel, C., Münchau, A., et al. (2008). The cortical motor threshold reflects microstructural properties of cerebral white matter. NeuroImage 40, 1782–1791. doi: 10.1016/j.neuroimage.2008.01.019
Kohn, N., Eickhoff, S. B., Scheller, M., Laird, A. R., Fox, P. T., and Habel, U. (2014). Neural network of cognitive emotion regulation—an ALE meta-analysis and MACM analysis. NeuroImage 87, 345–355. doi: 10.1016/j.neuroimage.2013.11.001
Krogsrud, S. K., Fjell, A. M., Tamnes, C. K., Grydeland, H., Mork, L., Due-Tønnessen, P., et al. (2016). Changes in white matter microstructure in the developing brain—a longitudinal diffusion tensor imaging study of children from 4 to 11 years of age. NeuroImage 124, 473–486. doi: 10.1016/j.neuroimage.2015.09.017
Langevin, L. M., MacMaster, F. P., Crawford, S., Lebel, C., and Dewey, D. (2014). Common white matter microstructure alterations in pediatric motor and attention disorders. J. Pediatr. 164, 1157–1164. doi: 10.1016/j.jpeds.2014.01.018
Langevin, L. M., MacMaster, F. P., and Dewey, D. (2015). Distinct patterns of cortical thinning in concurrent motor and attention disorders. Dev. Med. Child Neurol. 57, 257–264. doi: 10.1111/dmcn.12561
Law, M., Baptiste, S., Carswell, A., McColl, M., Polatajko, H., and Pollock, N. (2014). Canadian Occupational Performance Measure (COPM), 5th edition. Toronto, ON: CAOT Publications ACE.
Leng, Y., Shi, Y., Yu, Q., Van Horn, J. D., Tang, H., Li, J., et al. (2016). Phenotypic and genetic correlations between the lobar segments of the inferior fronto-occipital fasciculus and attention. Sci. Rep. 6:33015. doi: 10.1038/srep33015
Li, Y., Kwan, M. Y., Clark, H. J., Hay, J., Faught, B. E., and Cairney, J. (2018). A test of the environmental Stress hypothesis in children with and without developmental coordination disorder. Psychol. Sport Exerc. 37, 244–250. doi: 10.1016/j.psychsport.2017.11.001
Liakakis, G., Nickel, J., and Seitz, R. (2011). Diversity of the inferior frontal gyrus—a meta-analysis of neuroimaging studies. Behav. Brain Res. 225, 341–347. doi: 10.1016/j.bbr.2011.06.022
Lingam, R., Jongmans, M. J., Ellis, M., Hunt, L. P., Golding, J., and Emond, A. (2012). Mental health difficulties in children with developmental coordination disorder. Pediatrics 129, e882–e891. doi: 10.1542/peds.2011-1556
Luo, L., Xu, L., Jung, R., Pearlson, G., Adali, T., and Calhoun, V. D. (2012). Constrained source-based morphometry identifies structural networks associated with default mode network. Brain Connect. 2, 33–43. doi: 10.1089/brain.2011.0026
Madhyastha, T., Merillat, S., Hirsiger, S., Bezzola, L., Liem, F., Grabowski, T., et al. (2014). Longitudinal reliability of tract-based spatial statistics in diffusion tensor imaging. Hum. Brain Mapp. 35, 4544–4555. doi: 10.1002/hbm.22493
Mandich, A., Polatajko, H., Miller, L., and Baum, C. (2004). The Pediatric Card Sort. Ottawa, ON: CAOT Publications ACE.
Martin, N. C., Piek, J. P., and Hay, D. (2006). DCD and ADHD: a genetic study of their shared aetiology. Hum. Mov. Sci. 25, 110–124. doi: 10.1016/j.humov.2005.10.006
Martini, R., Rios, J., Polatajko, H., Wolf, T., and McEwen, S. (2015). The Performance Quality Rating Scale (PQRS): reliability, convergent validity and internal responsiveness for two scoring systems. Disabil. Rehabil. 37, 231–238. doi: 10.3109/09638288.2014.913702
McLeod, K. R., Langevin, L. M., Dewey, D., and Goodyear, B. G. (2016). Atypical within-and between-hemisphere motor network functional connections in children with developmental coordination disorder and attention-deficit/hyperactivity disorder. NeuroImage Clin. 12, 157–164. doi: 10.1016/j.nicl.2016.06.019
McLeod, K. R., Langevin, L. M., Goodyear, B. G., and Dewey, D. (2014). Functional connectivity of neural motor networks is disrupted in children with developmental coordination disorder and attention-deficit/hyperactivity disorder. NeuroImage Clin. 4, 566–575. doi: 10.1016/j.nicl.2014.03.010
Merel, J., Botvinick, M., and Wayne, G. (2019). Hierarchical motor control in mammals and machines. Nat. Commun. 10:5489. doi: 10.1038/s41467-019-13239-6
Miller, L., Polatajko, H., Missiuna, C., Mandich, A., and Macnab, J. (2001). A pilot trial of a cognitive treatment for children with developmental coordination disorder. Hum. Mov. Sci. 20, 183–210. doi: 10.1016/s0167-9457(01)00034-3
Missiuna, C., Cairney, J., Pollock, N., Campbell, W., Russell, D. J., Macdonald, K., et al. (2014). Psychological distress in children with developmental coordination disorder and attention-deficit hyperactivity disorder. Res. Dev. Disabil. 35, 1198–1207. doi: 10.1016/j.ridd.2014.01.007
Missiuna, C., Mandich, A., Polatajko, H. J., and Malloy-Miller, T. (2001). Cognitive orientation to daily occupational performance (CO-OP) part I-theoretical foundations. Phys. Occup. Ther. Pediatr. 20, 69–81. doi: 10.1080/J006v20n02_05
Mori, S., Wakana, S., Van Zijl, P. C., and Nagae-Poetscher, L. (2005). MRI Atlas of Human White Matter. Amsterdam, Netherlands: Elsevier.
Niida, R., Yamagata, B., Niida, A., Uechi, A., Matsuda, H., and Mimura, M. (2018). Aberrant anterior thalamic radiation structure in bipolar disorder: a diffusion tensor tractography study. Front. Psychiatry 9:522. doi: 10.3389/fpsyt.2018.00522
Northoff, G., Richter, A., Gessner, M., Schlagenhauf, F., Fell, J., Baumgart, F., et al. (2000). Functional dissociation between medial and lateral prefrontal cortical spatiotemporal activation in negative and positive emotions: a combined fMRI/MEG study. Cereb. Cortex 10, 93–107. doi: 10.1093/cercor/10.1.93
O’Donnell, L. J., and Westin, C.-F. (2011). An introduction to diffusion tensor image analysis. Neurosurg. Clin. N Am. 22, 185–196. doi: 10.1016/j.nec.2010.12.004
Öhman, A., Flykt, A., and Esteves, F. (2001). Emotion drives attention: detecting the snake in the grass. J. Exp. Psychol. Gen. 130, 466–478. doi: 10.1037/0096-3445.130.3.466
Oliveri, M., Babiloni, C., Filippi, M., Caltagirone, C., Babiloni, F., Cicinelli, P., et al. (2003). Influence of the supplementary motor area on primary motor cortex excitability during movements triggered by neutral or emotionally unpleasant visual cues. Exp. Brain Res. 149, 214–221. doi: 10.1007/s00221-002-1346-8
Olson, E. A., Collins, P. F., Hooper, C. J., Muetzel, R., Lim, K. O., and Luciana, M. (2009). White matter integrity predicts delay discounting behavior in 9-to 23-year-olds: a diffusion tensor imaging study. J. Cogn. Neurosci. 21, 1406–1421. doi: 10.1162/jocn.2009.21107
Papez, J. W. (1937). A proposed mechanism of emotion. Arch. Neurol. Psychiatry 38, 725–743. doi: 10.1001/archneurpsyc.1937.02260220069003
Pardo, J. V., Fox, P. T., and Raichle, M. E. (1991). Localization of a human system for sustained attention by positron emission tomography. Nature 349, 61–64. doi: 10.1038/349061a0
Piek, J. P., Pitcher, T. M., and Hay, D. A. (1999). Motor coordination and kinaesthesis in boys with attention deficit-hyperactivity disorder. Dev. Med. Child Neurol. 41, 159–165. doi: 10.1017/s0012162299000341
Piek, J. P., Rigoli, D., Pearsall-Jones, J. G., Martin, N. C., Hay, D. A., Bennett, K. S., et al. (2007). Depressive symptomatology in child and adolescent twins with attention-deficit hyperactivity disorder and/or developmental coordination disorder. Twin Res. Hum. Genet. 10, 587–596. doi: 10.3389/fpsyg.2021.665189
Pitcher, T. M., Piek, J. P., and Hay, D. A. (2003). Fine and gross motor ability in males with ADHD. Dev. Med. Child Neurol. 45, 525–535. doi: 10.1017/s0012162203000975
Polatajko, H. J., and Mandich, A. (2004). Enabling occupation in children: The Cognitive Orientation to Daily Occupational Performance (CO-OP) Approach. Toronto, ON: CAOT Publications ACE.
Polatajko, H. J., Mandich, A. D., Missiuna, C., Miller, L. T., Macnab, J. J., Malloy-Miller, T., et al. (2001). Cognitive orientation to daily occupational performance (CO-OP) part III-the protocol in brief. Phys. Occup. Ther. Pediatr. 20, 107–123. doi: 10.1080/J006v20n02_07
Pratt, M. L., and Hill, E. L. (2011). Anxiety profiles in children with and without developmental coordination disorder. Res. Dev. Disabil. 32, 1253–1259. doi: 10.1016/j.ridd.2011.02.006
Rasmussen, P., and Gillberg, C. (2000). Natural outcome of ADHD with developmental coordination disorder at age 22 years: a controlled, longitudinal, community-based study. J. Am. Acad. Child Adolesc. Psychiatry 39, 1424–1431. doi: 10.1097/00004583-200011000-00017
Reddy, P. G., Mattar, M. G., Murphy, A. C., Wymbs, N. F., Grafton, S. T., Satterthwaite, T. D., et al. (2018). Brain state flexibility accompanies motor-skill acquisition. NeuroImage 171, 135–147. doi: 10.1016/j.neuroimage.2017.12.093
Reid, R., Trout, A. L., and Schartz, M. (2005). Self-regulation interventions for children with attention deficit/hyperactivity disorder. Exceptional Child. 71:361.
Rinat, S., Izadi-Najafabadi, S., and Zwicker, J. G. (2020). Children with developmental coordination disorder show altered functional connectivity compared to peers. NeuroImage Clin. 27:102309. doi: 10.1016/j.nicl.2020.102309
Rivilis, I., Hay, J., Cairney, J., Klentrou, P., Liu, J., and Faught, B. E. (2011). Physical activity and fitness in children with developmental coordination disorder: a systematic review. Res. Dev. Disabil. 32, 894–910. doi: 10.1016/j.ridd.2011.01.017
Shadmehr, R., and Krakauer, J. W. (2008). A computational neuroanatomy for motor control. Exp. Brain Res. 185, 359–381. doi: 10.1007/s00221-008-1280-5
Shamloo, F., and Helie, S. (2016). Changes in default mode network as automaticity develops in a categorization task. Behav. Brain Res. 313, 324–333. doi: 10.1016/j.bbr.2016.07.029
Shiels, K., and Hawk, L. W. (2010). Self-regulation in ADHD: the role of error processing. Clin. Psychol. Rev. 30, 951–961. doi: 10.1016/j.cpr.2010.06.010
Smith, S. M., Jenkinson, M., Johansen-Berg, H., Rueckert, D., Nichols, T. E., Mackay, C. E., et al. (2006). Tract-based spatial statistics: voxelwise analysis of multi-subject diffusion data. NeuroImage 31, 1487–1505. doi: 10.1016/j.neuroimage.2006.02.024
Smith, S. M., Jenkinson, M., Woolrich, M. W., Beckmann, C. F., Behrens, T. E., Johansen-Berg, H., et al. (2004). Advances in functional and structural MR image analysis and implementation as FSL. NeuroImage 23, S208–S219. doi: 10.1016/j.neuroimage.2004.07.051
Smith, S. M., and Nichols, T. E. (2009). Threshold-free cluster enhancement: addressing problems of smoothing, threshold dependence and localisation in cluster inference. NeuroImage 44, 83–98. doi: 10.1016/j.neuroimage.2008.03.061
Stave, E. A., De Bellis, M. D., Hooper, S. R., Woolley, D. P., Chang, S. K., and Chen, S. D. (2017). Dimensions of attention associated with the microstructure of corona radiata white matter. J. Child. Neurol. 32, 458–466. doi: 10.1177/0883073816685652
Sun, L., Peräkylä, J., Polvivaara, M., Öhman, J., Peltola, J., Lehtimäki, K., et al. (2015). Human anterior thalamic nuclei are involved in emotion-attention interaction. Neuropsychologia 78, 88–94. doi: 10.1016/j.neuropsychologia.2015.10.001
Takahashi, M., Iwamoto, K., Fukatsu, H., Naganawa, S., Iidaka, T., and Ozaki, N. (2010). White matter microstructure of the cingulum and cerebellar peduncle is related to sustained attention and working memory: a diffusion tensor imaging study. Neurosci. Lett. 477, 72–76. doi: 10.1016/j.neulet.2010.04.031
Takeuchi, N., Oouchida, Y., and Izumi, S. (2012). Motor control and neural plasticity through interhemispheric interactions. Neural. Plast. 2012:823285. doi: 10.1155/2012/823285
Taylor, S., Fayed, N., and Mandich, A. (2007). CO-OP intervention for young children with developmental coordination disorder. OTJR 27, 124–130. doi: 10.1177/153944920702700402
Teipel, S. J., Bokde, A. L., Meindl, T., Amaro, E.Jr., Soldner, J., Reiser, M. F., et al. (2010). White matter microstructure underlying default mode network connectivity in the human brain. NeuroImage 49, 2021–2032. doi: 10.1016/j.neuroimage.2009.10.067
Thornton, A., Licari, M., Reid, S., Armstrong, J., Fallows, R., and Elliott, C. (2016). Cognitive Orientation to (daily) Occupational Performance intervention leads to improvements in impairments, activity and participation in children with developmental coordination disorder. Disabil. Rehabil. 38, 979–986. doi: 10.3109/09638288.2015.1070298
Thornton, S., Bray, S., Langevin, L. M., and Dewey, D. (2018). Functional brain correlates of motor response inhibition in children with developmental coordination disorder and attention deficit/hyperactivity disorder. Hum. Mov. Sci. 59, 134–142. doi: 10.1016/j.humov.2018.03.018
van den Heuvel, M., Mandl, R., Luigjes, J., and Hulshoff Pol, H. (2008). Microstructural organization of the cingulum tract and the level of default mode functional connectivity. J. Neurosci. 28, 10844–10851. doi: 10.1523/JNEUROSCI.2964-08.2008
Vergani, F., Lacerda, L., Martino, J., Attems, J., Morris, C., Mitchell, P., et al. (2014). White matter connections of the supplementary motor area in humans. J. Neurol. Neurosurg. Psychiatry 85, 1377–1385. doi: 10.1136/jnnp-2013-307492
Ward, A., and Rodger, S. (2004). The application of cognitive orientation to daily occupational performance (CO-OP) with children 5-7 years with developmental coordination disorder. Br. J. Occup. Ther. 67, 256–264. doi: 10.1177/030802260406700604
Watemberg, N., Waiserberg, N., Zuk, L., and Lerman-Sagie, T. (2007). Developmental coordination disorder in children with attention-deficit-hyperactivity disorder and physical therapy intervention. Dev. Med. Child Neurol. 49, 920–925. doi: 10.1111/j.1469-8749.2007.00920.x
Weininger, J. K., Roman, E., Tierney, P., Barry, D., Gallagher, H., Levins, K. J., et al. (2019). Papez’s forgotten tract: 80 years of unreconciled findings concerning the thalamocingulate tract. Front. Neuroanat. 13:14. doi: 10.3389/fnana.2019.00014
Wiebking, C., De Greck, M., Duncan, N. W., Heinzel, A., Tempelmann, C., and Northoff, G. (2011). Are emotions associated with activity during rest or interoception? An exploratory fMRI study in healthy subjects. Neurosci. Lett. 491, 87–92. doi: 10.1016/j.neulet.2011.01.012
Williams, J., Kashuk, S. R., Wilson, P. H., Thorpe, G., and Egan, G. F. (2017). White matter alterations in adults with probable developmental coordination disorder: An MRI diffusion tensor imaging study. Neuroreport 28, 87–92. doi: 10.1097/WNR.0000000000000711
Williams, J. H. G., Huggins, C. F., Zupan, B., Willis, M., Van Rheenen, T. E., Sato, W., et al. (2020). A sensorimotor control framework for understanding emotional communication and regulation. Neurosci. Biobehav. Rev. 112, 503–518. doi: 10.1016/j.neubiorev.2020.02.014
Wilson, B. N., Crawford, S. G., Green, D., Roberts, G., Aylott, A., and Kaplan, B. (2009). Psychometric properties of the revised developmental coordination disorder questionnaire. Phys. Occup. Ther. Pediatr. 29, 182–202. doi: 10.1080/01942630902784761
Winkler, A. M., Ridgway, G. R., Webster, M. A., Smith, S. M., and Nichols, T. E. (2014). Permutation inference for the general linear model. NeuroImage 92, 381–397. doi: 10.1016/j.neuroimage.2014.01.060
Winsler, A. E., Fernyhough, C. E., and Montero, I. E. (2009). Private Speech, Executive Functioning and the Development of Verbal Self-Regulation. New York, NY: Cambridge University Press.
Wulf, G., and Lewthwaite, R. (2016). Optimizing performance through intrinsic motivation and attention for learning: the OPTIMAL theory of motor learning. Psychon. Bull. Rev. 23, 1382–1414. doi: 10.3758/s13423-015-0999-9
Yeh, C., Huang, W., Lo, M., Chang, C., Ma, K., and Shyu, J. (2012). The rCBF brain mapping in adolescent ADHD comorbid developmental coordination disorder and its changes after MPH challenging. Eur. J. Paediatr. Neurol. 16, 613–618. doi: 10.1016/j.ejpn.2012.02.007
Zimmerman, B. J. (2000). “Attaining self-regulation: a social cognitive perspective,” in Handbook of Self-regulation, (Elsevier: Academic Press), 13–39.
Zimmermann, J., Griffiths, J. D., and McIntosh, A. R. (2018). Unique mapping of structural and functional connectivity on cognition. J. Neurosci. 38, 9658–9667. doi: 10.1523/JNEUROSCI.0900-18.2018
Zwicker, J. G., Harris, S., and Klassen, A. (2013). Quality of life domains affected in children with developmental coordination disorder: a systematic review. Child Care Health. Dev. 39, 562–580. doi: 10.1111/j.1365-2214.2012.01379.x
Zwicker, J. G., Missiuna, C., Harris, S. R., and Boyd, L. A. (2012). Developmental coordination disorder: a pilot diffusion tensor imaging study. Pediatr. Neurol. 46, 162–167. doi: 10.1016/j.pediatrneurol.2011.12.007
Keywords: developmental coordination disorder, motor skills disorder, rehabilitation, diffusion tensor imaging, neuroplasticity, CO-OP
Citation: Izadi-Najafabadi S and Zwicker JG (2021) White Matter Changes With Rehabilitation in Children With Developmental Coordination Disorder: A Randomized Controlled Trial. Front. Hum. Neurosci. 15:673003. doi: 10.3389/fnhum.2021.673003
Received: 26 February 2021; Accepted: 27 April 2021;
Published: 03 June 2021.
Edited by:
Catherine Purcell, Cardiff University, United KingdomReviewed by:
Christian Hyde, Deakin University, AustraliaNeeraj Upadhyay, University Hospital Bonn, Germany
Copyright © 2021 Izadi-Najafabadi and Zwicker. This is an open-access article distributed under the terms of the Creative Commons Attribution License (CC BY). The use, distribution or reproduction in other forums is permitted, provided the original author(s) and the copyright owner(s) are credited and that the original publication in this journal is cited, in accordance with accepted academic practice. No use, distribution or reproduction is permitted which does not comply with these terms.
*Correspondence: Jill G. Zwicker, jill.zwicker@ubc.ca