- 1Department of Neurology, Beijing Tiantan Hospital, Capital Medical University, Beijing, China
- 2National Clinical Research Center for Neurological Diseases, Beijing, China
- 3Advanced Innovation Center for Human Brain Protection, Capital Medical University, Beijing, China
- 4Beijing Institute of Brain Disorders, Collaborative Innovation Center for Brain Disorders, Capital Medical University, Beijing, China
- 5Beijing Key Laboratory of Neuromodulation, Beijing, China
Epilepsy is caused by abnormal electrical discharges (clinically identified by electrophysiological recording) in a specific part of the brain [originating in only one part of the brain, namely, the epileptogenic zone (EZ)]. Epilepsy is now defined as an archetypical hyperexcited neural network disorder. It can be investigated through the network analysis of interictal discharges, ictal discharges, and resting-state functional connectivity. Currently, there is an increasing interest in embedding resting-state connectivity analysis into the preoperative evaluation of epilepsy. Among the various neuroimaging technologies employed to achieve brain functional networks, magnetoencephalography (MEG) with the excellent temporal resolution is an ideal tool for estimating the resting-state connectivity between brain regions, which can reveal network abnormalities in epilepsy. What value does MEG resting-state functional connectivity offer for epileptic presurgical evaluation? Regarding this topic, this paper introduced the origin of MEG and the workflow of constructing source–space functional connectivity based on MEG signals. Resting-state functional connectivity abnormalities correlate with epileptogenic networks, which are defined by the brain regions involved in the production and propagation of epileptic activities. This paper reviewed the evidence of altered epileptic connectivity based on low- or high-frequency oscillations (HFOs) and the evidence of the advantage of using simultaneous MEG and intracranial electroencephalography (iEEG) recordings. More importantly, this review highlighted that MEG-based resting-state functional connectivity has the potential to predict postsurgical outcomes. In conclusion, resting-state MEG functional connectivity has made a substantial progress toward serving as a candidate biomarker included in epileptic presurgical evaluations.
Introduction
Epilepsy is a neurological disorder that is predominantly characterized by a tendency for the recurrent and unpredictable hypersynchronous neuronal activity that interrupts the normal brain function (Fisher et al., 2005). Antiepileptic drug administration is the first and basal treatment principle, but mediation does not work well for approximately one-third of epileptic patients who require epilepsy surgery evaluation that is aimed at localizing the epileptogenic zone (EZ) for individual surgical operations (Kwan et al., 2011). In current clinical practice, ictal activities and interictal epileptiform discharges (IEDs) are widely applied to localize the EZ (Nissen et al., 2016a; Cuello-Oderiz et al., 2018); however, some routine examinations are incapable of capturing ictal activities and occasionally even fail to acquire IEDs (Feyissa et al., 2017). Moreover, epileptic patients suffer from persistent seizures after the surgical removal of the EZ, potentially suggesting that the EZ in these patients is insufficiently identified and removed (Englot et al., 2015; Jobst and Cascino, 2015). Therefore, new approaches and biomarkers that can be integrated into clinical decision-making are urgent.
Epilepsy has been treated as an archetypical neural network disorder (Kramer and Cash, 2012), with specific disruptions in networks involving one or both hemispheres; consequently, the concept of epilepsy has changed from “foci” to “network” (van Diessen et al., 2013). Network analysis has been widely utilized to gain an insight into the dynamic complexity of refractory epilepsy, which provides a framework to describe seizure progress, including preseizure, seizure initiation, spread, and termination (Kramer et al., 2008; Schindler et al., 2008), and even interictal information (Chavez et al., 2010; Horstmann et al., 2010; Liao et al., 2010; van Dellen et al., 2012b). Recent studies have reported altered networks reflecting neuropathology in epilepsy patients with focal epilepsy (Coito et al., 2016a,b; van Diessen et al., 2014; Englot et al., 2016) and generalized epilepsy (Chavez et al., 2010; Zhang et al., 2011; Elshahabi et al., 2015; Niso et al., 2015). Resting-state networks are one of the common approaches in seizure surgical clinical evaluation to uncover the intrinsic interactions between brain areas that may affect epileptic networks, whose main advantage is that they can be estimated using interictal activity without the need to wait for a seizure to occur (Hsiao et al., 2015; Krishnan et al., 2015; Li Hegner et al., 2018; Leng et al., 2020).
Resting-state functional connectivity is attracting an increasing amount of attention as a tool for the surgical evaluation of epileptic patients. Advances in neuroimaging have generated multimodal technologies to analyze the resting-state functional networks (Figure 1). Of these imaging modalities, functional magnetic resonance imaging (fMRI) has been widely employed to investigate the functional connectivity during the resting state (Biswal et al., 1995; Palacios et al., 2013; Tracy and Doucet, 2015; Chakraborty et al., 2020; Courtiol et al., 2020). However, fMRI is an indirect measure of neural activity with a delay that detects the changes in blood oxygenation using blood-oxygen-level-dependent (BOLD) contrast (Scarapicchia et al., 2017). Similar to fMRI, positron emission tomography (PET) measures the blood flow by averaging the measurements with a low temporal resolution over a few minutes. Functional near-infrared spectroscopy (fNIRS) has limited spatial and temporal resolution. Electrophysiological neuroimaging modalities include scalp electroencephalography (EEG), intracranial electroencephalography (iEEG), and magnetoencephalography (MEG). Scalp EEG measures differences in voltage; its recordings depend heavily on the selection of the reference channel, and its activities are vulnerable to skull impedance. Unlike scalp EEG, MEG performs direct measures of brain activity at a specific point, and its measures are reference-free. In terms of localization accuracy, both MEG and scalp high-density EEG, through magnetic and electric source imaging, provide a good source localization of epileptogenic foci but with different sensitivities (Carrette and Stefan, 2019, Tamilia et al., 2019), and their combination showed complementarity due to the sensitivity of EEG and MEG for radial and tangential sources in the brain (Carrette and Stefan, 2019). Different from MEG and scalp EEG, long-term iEEG monitoring, mainly including electrocorticography (ECoG) and stereotactic electroencephalography (sEEG), is not only invasive and costly but also limited to a spatial sampling of only a portion of brain areas (Hader et al., 2013). MEG is a non-invasive technique that provides direct access to the entire brain activity with submillisecond temporal resolution and millimeter spatial resolution (Baillet, 2017), which makes it an ideal tool for investigating the resting-state functional connectivity in epilepsy.
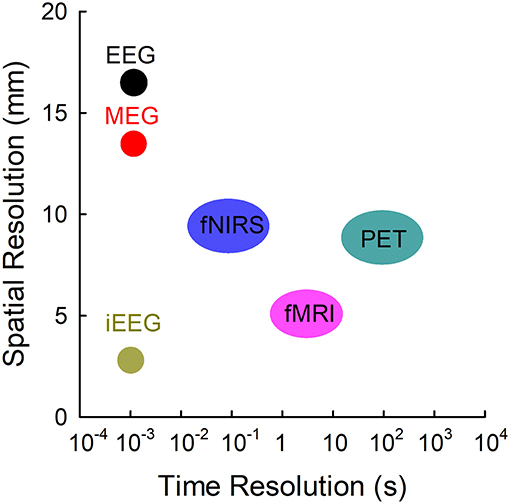
Figure 1. Temporal resolutions and spatial resolutions of different modalities commonly employed. EEG, electroencephalography; fMRI, functional MRI; fNIRS, functional near-infrared spectroscopy; iEEG, intracranial electroencephalography; MEG, magnetoencephalography; PET, positron emission tomography. Adapted from Olivi (2011).
Basic Principles of MEG Measuring Brain Activities
MEG measures the magnetic fields that are mainly generated by synchronous postsynaptic (intracellular) currents in the pyramidal neurons of the cerebral cortex (Hämäläinen et al., 1993). Some cortical pyramidal neurons are spatially aligned and perpendicular to the cortical surface, with the soma at the basal cortex and the apical dendrites at the surface of the cortex, which are the sources of magnetic fields that could be detected by MEG. When these pyramidal neurons are excited, the apical dendritic membrane becomes transiently depolarized, which consequently triggers the generation of a current that flows from the apical dendrites to the soma within the intracellular space (primary current), as well as the extracellular volume current that flows from the soma and basal dendrites to the apical dendrites. MEG signals are believed to arise from intracellular currents (Figures 2A,B).
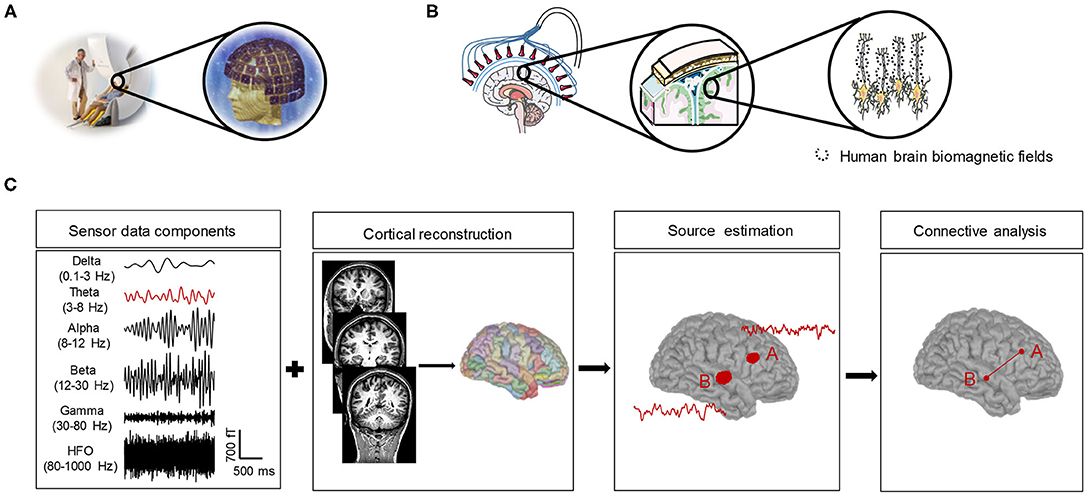
Figure 2. A simple overview of MEG and its measures and the pipeline to obtain the resting-state functional connectivity. (A) The subject sits on the MEG chair for the whole measurement process, whose head position corresponds to the sensors arranged in helmet-like arrays. (B) Some cortical pyramidal neurons are spatially aligned and perpendicular to the cortical surface. When these pyramidal neurons are excited, the apical dendritic membrane becomes transiently depolarized, which consequently triggers the generation of a current that flows from the apical dendrites to the soma within the intracellular space (primary current), and the magnetic field-generated intracellular currents are the sources of the MEG. (C) Pipeline to obtain the resting-state functional connectivity. Brain activities characterize several oscillatory bands (delta, theta, alpha, beta, gamma, and HFO), and each frequency-band signal (e.g., theta-band shown in red in the figure) of MEG sensor data is source-localized to parcellation atlases coregistered with anatomical MRI. Cortical regions with significant activity were considered ROIs (A,B). The time-series activity of the ROI can be extracted using a beamformer and fed in the connectivity analysis to obtain the functional connectivity in the source space.
Neuronal magnetic fields are considerably weaker by ~10–100 million times Earth's magnetic field (Hämäläinen et al., 1993), which highlights the need for MEG instrumentation with very sensitive sensors and the noise reduction method. In modern MEG systems, weaker fields are recorded using superconducting quantum interference devices (SQUIDs) (Kleiner et al., 2004), which are immersed in a liquid helium cooling unit set to ~-269°C that is highly sensitive to extremely subtle changes in the electromagnetic fields generated by neurons located a few centimeters from the sensors. In terms of noise reduction, in addition to the magnetically shielded room and differential (gradiometric) sensors that shield the MEG system from outside noise (Parkkonen, 2010), signal processing methods can effectively reduce noise. For example, signal space separation (SSS) decomposes multichannel MEG data based on the physical properties of magnetic fields to remove external disturbances and movement artifacts (Taulu et al., 2004). The signal space projection (SSP) uses the orthogonal projection method in the multidimensional signal space to remove the artifact associated with the spatial pattern of the magnetic field (Ramírez et al., 2011). Independent component analysis (ICA) is primarily employed to remove artifacts, such as blinking, eye muscle movement, facial muscle artifacts, and cardiac artifacts, but with poor resolution of highly correlated brain sources due to its fundamental statistical independence (Ikeda and Toyama, 2000; Iversen and Makeig, 2014).
Resting-State Functional Connectivity Based on MEG Signals
Resting-state functional connectivity refers to the statistical associations or temporal correlations between two or more anatomically distinct brain regions without imposed stimuli. Although there is no established standard as to which method, modality, and analysis variant are optimal for MEG-derived, resting-state, functional connectivity, recent studies revealed methodological limitations by comparing the test–retest reliability (Colclough et al., 2016; Garcés et al., 2016), which provides a platform for inferring the connectivity of the resting state.
To calculate the resting-state functional connectivity, the activity of the cortical source responsible for MEG sensor signals should be estimated (Figure 2C). Each MEG sensor measures the signals generated by all brain sources that are active at a given time but with different weights due to the volume conduction effect (Winter et al., 2007). Given that the same connectivity information can be generated by different configurations of interacting sources, it is difficult to interpret the underlying functional connectivity based on MEG sensor signals. In addition, the head of the subject is not fixed relative to MEG sensor locations, which may cause the same MEG sensors from picking up signals from different regions of the brain for the same subject between two different runs of acquisition and different subjects with different head shapes, sizes, and positions under the helmet.
It is important to choose a suitable method for reconstructing the activity of brain regions from MEG sensor signals (Tadel et al., 2011; Xiang et al., 2014; Baillet, 2017; van Mierlo et al., 2019), which could be generally divided into two steps. In the first step, head modeling (or forward modeling) provides the mathematical relationship between the magnetic field of MEG sensors and the brain currents in a subject's head. The head model depends on the shape and conductivity of the head and can typically be constructed from structural MRI scans using methods ranging from a single sphere (Baillet et al., 2002) to overlapping spheres (Huang et al., 1999) and boundary or finite element methods (Darvas et al., 2004). Because the propagation of magnetic fields is not affected by electric conductance, the simple spherical head model often works quite well for the MEG forward model (de Munck et al., 2012; Hämäläinen et al., 2020).
In the second step, source modeling imaging depends on the head model to estimate neuronal activity from the MEG. Source model estimation of brain activity is typically obtained using two main approaches: (1) dipole modeling, where the position and amplitude of one to a few equivalent current dipoles (ECDs) are estimated over relatively short time windows (Leahy et al., 1998); and (2) distributed source modeling (DSM), which is aimed at estimating the activity in many sources in the brain by discretizing the brain (Baillet, 2017). In the ECD model, the sources of the MEG signals are assumed to consist of focal activations (Hämäläinen et al., 1993) and can be determined using non-linear optimization algorithms (Huang et al., 1998) or subspace scanning techniques (Mosher et al., 1992). ECDs are suitable and traditionally utilized in a clinic for epileptic focal localization (Bagic et al., 2011). Compared with ECDs, DSM may be more appropriate for source estimation when MEG signals are generated in a widespread manner (Hämäläinen and Ilmoniemi, 1994). The DSM approaches mainly include the weighted minimum norm estimate (MNE) (Hämäläinen and Ilmoniemi, 1994), standardized low-resolution electromagnetic tomography (sLORETA) (Pascual-Marqui, 2002), dynamic statistical parametric mapping (dSPM) (Dale and Sereno, 1993), maximum entropy on the mean (MEM) (Amblard et al., 2004), and beamforming and scanning methods (Liuzzi et al., 2017; Dimitriadis et al., 2018), each of which differs in their assumptions about cortical generators. The spatial fidelity of these approaches has been compared (Samuelsson et al., 2021). Although there is no optimal choice for the inverse solution, it is believed that the fidelity depends on the spatial and synchronization profiles of the interacting cortical sources (Hincapié et al., 2017).
Currently, although a large number of source–space connectivity estimation methods for MEG are available, there is no consensus on which functional connectivity index is most suitable for MEG resting-state studies. According to the logic of how to define the statistical dependence of the estimation source, connectivity methods can be divided into phase synchronization-based measures, coherence-based measures, generalized synchronization-based measures, and Granger causality-based measures (Niso et al., 2013). By comparing the consistency and reproducibility of some commonly employed network estimation metrics, Colclough et al. (2016) found that amplitude envelope correlation (AEC) and partial correlation are the most consistent methods, while the poorly consistent methods are phase-based or coherence-based metrics such as the phase lag index (PLI) or the imaginary part of coherency. Envelope correlation metrics for the resting-state MEG functional connectivity have also been recommended by (Garcés et al. 2016) and have been widely applied in the connectivity network analysis (Brookes et al., 2011; Hipp et al., 2012; Dimitriadis et al., 2018; Aydin et al., 2020; Routley et al., 2020). The metrics, including coherence (Coh), imaginary coherence (imCoh), pairwise phase consistency (PPC), phase-locking value (PLV), PLI, weighted phase lag index (wPLI), and weighted phase lag index debiased (wPLI2), were compared in a recent study to test the reliability of resting-state MEG functional connectivity in schizophrenia (SZ). The article indicated that the reliability of these metrics varied greatly depending on the frequency band, network, and participant group examined (Candelaria-Cook and Stephen, 2020). Although there is no uniform standard for MEG resting-state functional connectivity, some identified factors should be considered: metrics (Colclough et al., 2016), frequency band (Meng and Xiang, 2016; Marquetand et al., 2019), and measurement duration (Marquetand et al., 2019).
In practice, to facilitate the source connectivity analysis of MEG signals, several open-source applications are available to the user (Table 1), for example, Brainstorm (Tadel et al., 2011; Niso et al., 2015, 2019), eConnectome (He et al., 2011), FieldTrip (Oostenveld et al., 2011), MNE (Gramfort et al., 2014), and SPM (Litvak et al., 2011), which provide a platform for the standardization of the most common analysis processes and reduce sharing efforts across MEG communities.
Resting-State MEG Functional Connectivity Abnormalities Indicate Epileptogenic Networks
In recent years, several studies have shown that the network connectivity of the resting state in epilepsy has changed but with inconsistent results. These differences are probably attributed to the frequency band selected and the metric chosen to reconstruct the functional connectivity, as previously described, the different types of epilepsy investigated, and the different drug treatments and seizure rates in a clinic. Previous studies have reported that the resting-state functional connectivity is frequency-dependent when EEG and fMRI are applied (Samogin et al., 2020; Xia et al., 2020) and that resting-state functional connectivity derived from MEG exhibits significant changes with age from childhood to adolescence (Meng and Xiang, 2016). Moreover, the low- and high-frequency bands may represent different seizure networks (Tenney et al., 2014). Thus, we will focus on the evidence of functional connectivity in low- and high-frequency band abnormalities in epilepsy.
The activity of brain regions is characterized by several oscillatory bands with frequencies ranging from ~0.05 to 500 Hz (Buzsáki and Draguhn, 2004). As noted, MEG has shown advantages in connectivity metrics, which can exploit the rich frequency content of the MEG signal (Xiang et al., 2014; Baillet, 2017) by assessing the functional connectivity of oscillatory activities. The frequency-specific functional connectivity related to the different properties of the physical architecture of neuronal networks and to the speed of neuronal communication limited by axon conduction and synaptic delays should be considered. Based on this basic principle, the resting-state MEG functional connectivity derived from multifrequency oscillations is summarized in Table 2 and described in the next section.
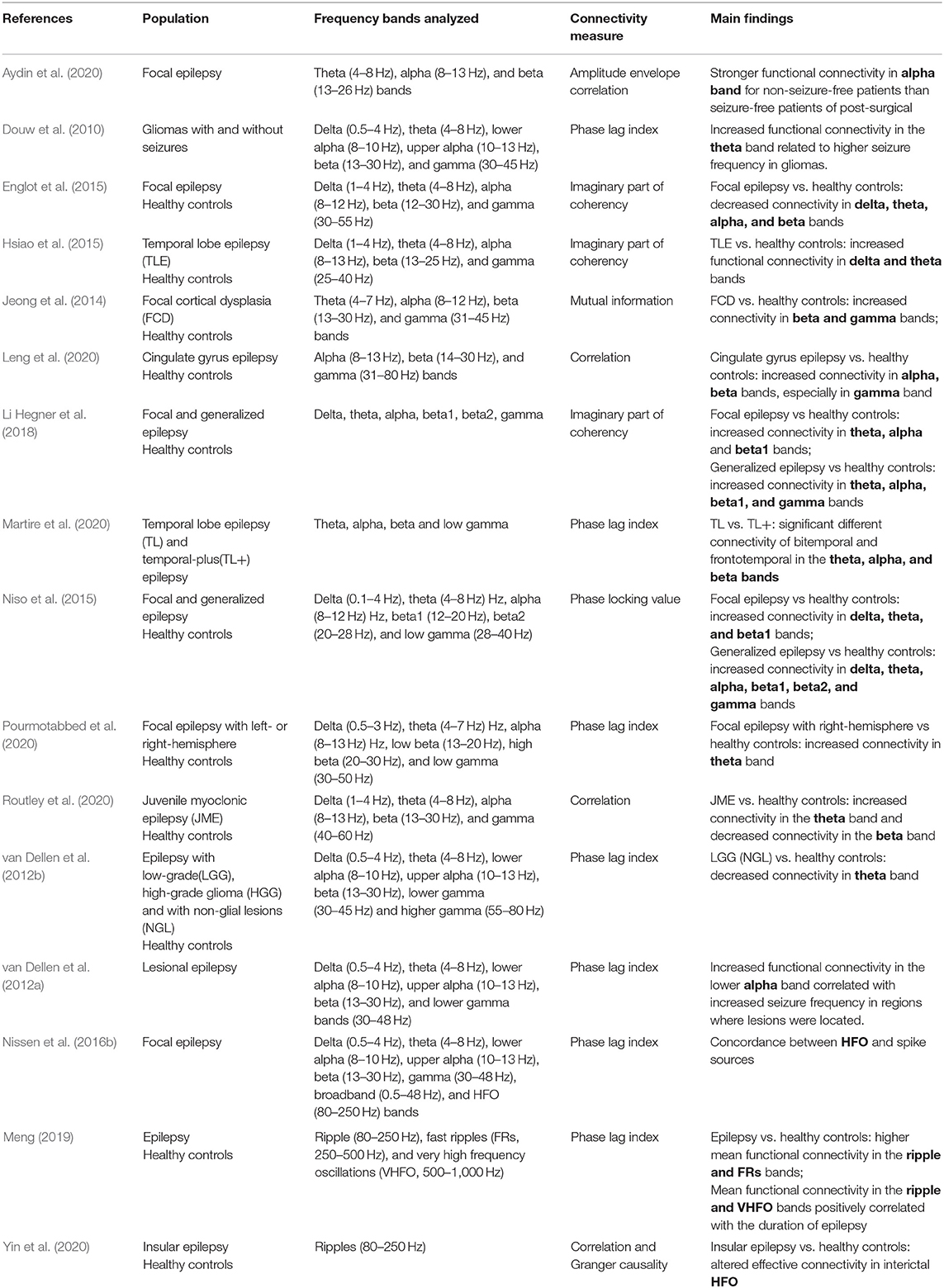
Table 2. Resting-state MEG functional connectivity based on multifrequency signals recorded from epilepsy.
High-Frequency Oscillations (HFOs)
Brain activities consisting of at least four clearly continuous oscillations with frequencies >80 Hz, compared with the background, are referred to as high-frequency oscillations (HFOs) (Jacobs et al., 2012; von Ellenrieder et al., 2016; Tamilia et al., 2017). The detection of HFOs is conventionally performed in an invasive intracranial EEG from patients with drug-resistant epilepsy (Akiyama et al., 2005; Jirsch et al., 2006; Urrestarazu et al., 2006; Fuertinger et al., 2016; von Ellenrieder et al., 2016; Qi et al., 2020; Ren et al., 2020; Zhao et al., 2020), whereas recent advances in MEG have shown promising results for non-invasive detection of HFOs in epilepsy patients (Miao et al., 2014; Nissen et al., 2016b; van Klink et al., 2016; von Ellenrieder et al., 2016; Meng, 2019; Yin et al., 2019). According to previous studies (Bragin et al., 1999; Usui et al., 2010, 2015; Brázdil et al., 2017), HFOs are further subclassified into three groups: ripples (80–250 Hz), fast ripples (250–500 Hz), and a very-high-frequency oscillation (VHFO) band (500–1,000 Hz) (Meng, 2019).
Proof of the validity of higher HFO rates within the seizure-onset zone (SOZ) has proposed HFOs as a promising biomarker of localization of the EZ (Thomschewski et al., 2019), which is attracting an increasing number of researchers to investigate HFOs in epilepsy with the goal of providing helpful information for a comprehensive preoperative evaluation. In general, HFOs are considered to be more focal and specific for identifying the SOZ than classical epileptic spikes (Jacobs et al., 2008, 2012; Andrade-Valença et al., 2012). Moreover, in regard to the identification of the SOZ, ripples have higher sensitivity but lower specificity than FR (Andrade-Valença et al., 2012). It seems that ripples and FR are generated by different pathophysiological mechanisms: Ripples are likely generated by synchronous firing coordinated by inhibitory currents (Schönberger et al., 2014), whereas FR may reflect the in-phase and out-of-phase firing of different pyramidal cell clusters (Demont-Guignard et al., 2012). Better results regarding the outcome prediction were reported for VHFO than for ripples and FR (Brázdil et al., 2017). However, there are still challenges regarding HFOs that need to be carefully addressed. One such issue is the detection of HFOs that are characterized by varied morphometry (Zijlmans et al., 2017), which should be addressed by exploiting an appropriate method to precisely detect HFOs (Ren et al., 2018; Zhao et al., 2020), considering the time-consuming and non-objective visual marking of HFOs. Another issue involves distinguishing pathologic HFOs from physiologic HFOs, representing a fundamental challenge for evaluating the effectiveness of HFOs as an epileptic biomarker (Thomschewski et al., 2019). Physiologic HFOs in the hippocampus and the occipital lobe of humans are a common phenomenon (Melani et al., 2013). Various analytical methods based on amplitude, frequency, and duration have been developed to separate HFOs (Cimbalnik et al., 2018; Thomschewski et al., 2019). Recently, Liu et al. (2018) identified a waveform morphological difference between pathologic HFOs and physiologic HFOs. Specifically, pathologic HFO waveforms are highly similar to those localized within the SOZs, whereas physiologic HFOs in random waveforms belong to the functional regions. An oddball cognitive task can be employed to further facilitate the discrimination of HFOs generated by epileptic and non-epileptic hippocampi (Pail et al., 2020).
A large number of studies have focused on the biomarker value of HFOs in the localization of the EZ (Zijlmans et al., 2012; Tamilia et al., 2017, 2020; Thomschewski et al., 2019; Velmurugan et al., 2019), whereas only a few studies have investigated the functional connectivity in HFOs using the resting-state MEG signals. Nissen et al. (2016b) recorded the resting-state MEG signals from 12 patients with refractory epilepsy and reported the enhanced functional connectivity in HFOs in the affected hemisphere compared to the non-affected hemisphere. However, Nissen et al. (2016b) analyzed HFOs in virtual MEG electrodes belonging to the sensor lever, which may include background noise. Using the accumulated source imaging method, Meng (2019) sourced HFO activities, reconstructed the brain network modulated by HFOs, and revealed that the brain networks of epileptic patients displayed altered patterns compared to those of healthy controls, especially within the ripple and FR bands (Figure 3). A recent study by Yin et al. (2020) indicated that patients with insular epilepsy showed an altered effective connectivity network in HFOs recorded from the resting-state MEG in contrast to healthy subjects.
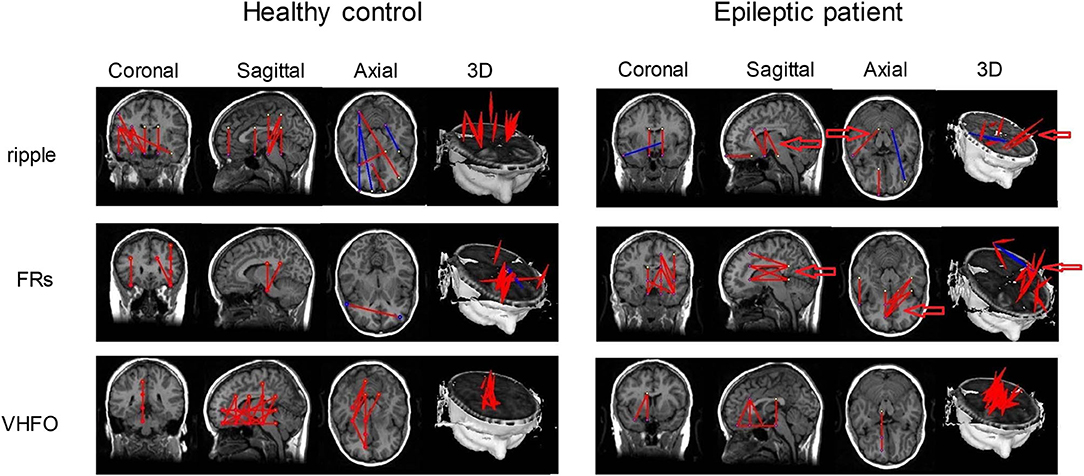
Figure 3. Functional connectivity networks based on HFOs from a healthy control and an epileptic patient. Altered connectivity patterns in the ripple and FR bands were found in epileptic patients. Arrows point to the significantly altered regions between epileptic patients and healthy controls. Inhibitory connections and excitatory connections are shown as blue lines and red lines, respectively. Adapted from Meng (2019).
Low-Frequency Signals
Brain rhythms (<80 Hz) can be divided into five types: delta band (0.1–4 Hz), theta band (4–8 Hz), alpha band (8–12 Hz), beta band (12–30 Hz), and gamma band (30–80 Hz), which are referred to as “low-frequency signals” relative to HFOs (Xiang et al., 2014; Meng, 2019). Each type represents a different meaning in the neural system; for example, the delta band predominates during slow, deep, dreamless sleep, or pathological states during wakefulness. The theta and alpha bands are functions of arousal, attention, working memory, and long-range interactions (Palva and Palva, 2011). During the local stimulus or saliency process, focal network activity will involve more beta and gamma bands (von Stein and Sarnthein, 2000). Connectivity analysis based on MEG signals at different frequency bands could reveal different resting-state patterns (Brookes et al., 2011; Hillebrand et al., 2012), which has the potential to provide additional information for the preoperative evaluation of epilepsy and link specific patterns to specific forms of epilepsy. For example, Hillebrand et al. (2012) performed source-based analysis from MEG data for 13 healthy participants using a PLI estimator. They revealed for the first time the frequency-dependent functional connectivity within the resting-state networks across the cortex: Alpha-band connectivity and beta band connectivity were confined to posterior areas and sensorimotor areas, respectively, while gamma band connectivity was in a more dispersed pattern.
Altered functional connectivity derived from the resting-state MEG was observed in both focal epilepsy patients and generalized epilepsy patients. Some studies have reported that increased resting-state connectivity correlated with epilepsy. Resting-state functional connectivity based on beta and gamma frequency bands in focal cortical dysplasia (FCD) patients is stronger than that of healthy controls (Jeong et al., 2014). One study by Hsiao et al. (2015) indicated that the resting-state functional connectivity within the default mode network at the delta and theta bands was reinforced in temporal lobe epilepsy. Li Hegner et al. (2018) compared MEG-based resting-state functional connectivity among focal epilepsy, generalized epilepsy, and healthy controls. Compared with healthy subjects, they found that focal epilepsy showed an increased connectivity within the theta, alpha, and beta1 bands over bilateral temporal, parietal, insula, and frontal regions and that generalized epilepsy showed an increased connectivity in the theta, alpha, beta1, and gamma bands over widespread bilateral temporal, parietal, insula, and medio-frontal regions. Moreover, beta2- and gamma-band functional MEG connectivity in bilateral mesio-frontal and motor regions was increased for generalized epilepsy patients compared with focal epilepsy patients (Li Hegner et al., 2018). In addition, an increased functional connectivity in the alpha band correlated with an increased seizure frequency in regions where lesions existed. In a recent study by Aydin et al. (2020), the functional connectivity in the alpha band was stronger for non-seizure-free patients than for seizure-free patients after surgery.
However, some studies have reported the decreased resting-state connectivity in epilepsy patients. Using the metric of the imaginary part of coherency to calculate the functional connectivity, Englot et al. (2015) found that focal epilepsy showed a decreased connectivity in delta, theta, alpha, and beta bands compared to the healthy controls. The increased alpha-band functional connectivity in the MEG resting-state network for low-grade glioma patients after resective surgery was suggested to be related to improved cognitive performance (van Dellen et al., 2012a). A recent study by Leng et al. (2020) investigated the functional connectivity of the default mode network in cingulate gyrus epilepsy using the resting-state MEG signals, analyzed multifrequency signals, and revealed that cingulate gyrus epilepsy has enhanced functional connectivity in alpha, beta, and gamma bands between the angular gyrus and the posterior cingulate cortex (PCC) of the left hemisphere compared to healthy controls. Moreover, the dominant functional connectivity in the gamma band over that in the alpha and beta bands indicates the significant contribution of the gamma band to the default mode network in cingulate gyrus epilepsy. Since the PCC is considered to be the only node in the default mode network that directly interacts with almost all other nodes (Fransson and Marrelec, 2008), the prominent functional connectivity in the gamma band between the PCC and left angular gyrus may be a potential biomarker for cingulate gyrus epilepsy.
In addition, complex patterns of increased and decreased connectivity were also reported in epilepsy. Juvenile myoclonic epilepsy (JME) is one of the most common epilepsy syndromes and is considered a brain network disorder with predominantly frontal (Chowdhury et al., 2014) but also parieto-occipital and subcortical involvement (Gotman et al., 2005). Routley et al. (2020) investigated the differences in the resting-state MEG functional connectivity using the AEC metric between patients with JME and healthy controls and found an increased connectivity in posterior theta and alpha bands and a decreased beta-band connectivity in sensorimotor brain regions in JME patients (Figure 4). The alterations in connectivity were similar to previous reports from EEG recordings in JME patients with an increased alpha-band connectivity and a decreased beta-band connectivity (Clemens et al., 2011). Theta and alpha bands have the function of attention and working memory, while patients with JME show cognitive dysfunction in attention and working memory (Pascalicchio et al., 2007). In addition, beta-band oscillation is considered to be involved in sensorimotor regulation (Engel and Fries, 2010). Routley et al. (2020) further suggested that the altered resting-state MEG connectivity may be the resting neurophysiological hallmark of JME.
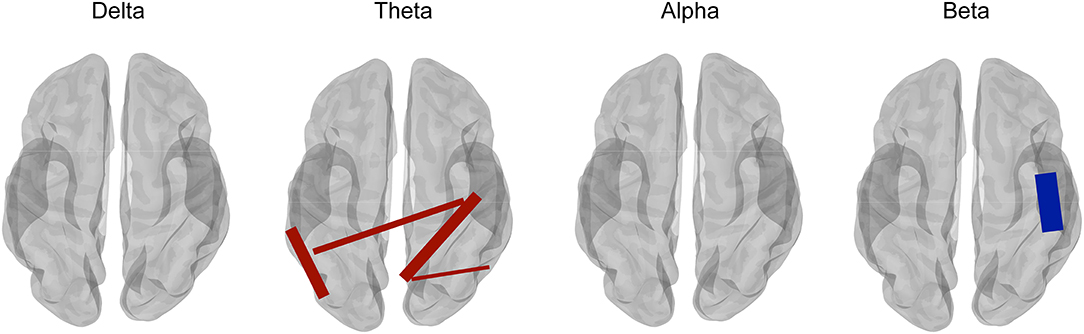
Figure 4. Comparison between juvenile myoclonic epilepsy (JME) patients and healthy controls for multifrequency bands with corrected t-tests. Compared with healthy controls, JME showed an increased connectivity in the theta band (4–8 Hz) (shown in red lines) and a decreased connectivity in the beta band (13–30 Hz) (shown in blue lines). However, connectivity in the frequency bands of delta (1–4 Hz) and alpha (8–13 Hz) did not show a significant difference with corrected t-test between JME and controls. Adapted from Routley et al. (2020).
In summary, the different changes reported here may be attributed to clinical differences, types of epilepsy, and methodology. Although the aim of linking specific-frequency patterns to specific types of epilepsy based on the MEG resting-state functional connectivity is a major challenge, it represents a promising method in the future and provides additional information. The metric and procedure used to calculate the functional connectivity need a unified standard, and more added information should be obtained by combining multimodal approaches.
Multimodal Integration of MEG and iEEG
As previously described, the resting-state functional connectivity in the multifrequency band can be investigated by multimodal neuroimaging approaches. Integration of different technologies may provide complementary information to more precisely characterize epileptogenic networks. As a “gold standard,” iEEG, including ECoG and sEEG, provides the opportunity to directly detect activities of the deep regions of the brain but is limited to only a portion of brain areas. Accumulating studies have demonstrated that MEG provides a broad whole-head view of brain activities that further guide implantation sites for intracranial recordings (Knowlton et al., 2006; Grova et al., 2016). Therefore, the integration of MEG and iEEG approaches with the advantage of obtaining local and global activities of the brain should be considered to study the resting-state functional connectivity that supports clinical decision-making.
The correlation between MEG and iEEG performed on separate recordings in presurgical evaluation has been reported in previous studies (Oishi et al., 2002, 2006; Knowlton et al., 2009; Bouet et al., 2012; Almubarak et al., 2014; Grova et al., 2016; Murakami et al., 2016), and concordant evaluation based on MEG and iEEG is associated with more favorable surgical outcomes than inconsistent evaluation. A comparison of networks based on oscillatory activities and spikes in MEG and iEEG was made, indicating the presence of overlapping but different networks for oscillations and spikes, which provide complementary information for presurgical assessment (Jmail et al., 2016).
In addition to considering the correlation between MEG and iEEG data, another crucial method is to verify the technical and clinical aspects applied to simultaneous MEG and iEEG recordings (Santiuste et al., 2008; Dubarry et al., 2014; Gavaret et al., 2016; Badier et al., 2017). Moreover, simultaneous MEG and iEEG recordings increase the sensitivity for localizing the epileptogenic region (Kakisaka et al., 2012; Vivekananda et al., 2020). This approach offers unique opportunities for cognitive neurophysiology research. For instance, Crespo-García et al. (2016) demonstrated a negative correlation between slow-theta activities in the hippocampus and spatial memory accuracy using simultaneous MEG and iEEG methods. Therefore, it is expected that the resting-state connectivity analysis will benefit from multimodal simultaneous recordings of MEG and iEEG signals at multiple spatial scales in the future.
MEG Resting-State Connectivity: Potential Value as a Predictor of Surgical Outcome
Seizure-free status is the evaluation criterion for the quality of life of patients with epilepsy. Although advancements in localizing the SOZ assist in clinical decision-making, approximately one-third of patients continue to experience postoperative seizures (Malmgren and Edelvik, 2017). Although the challenge is mainly attributed to heterogeneous patients and the complexity of brain network interactions, a correlation between the surgical outcome and the resting-state functional connectivity has been reported, as suggested mostly using fMRI (Tracy and Doucet, 2015; Englot et al., 2016; He et al., 2017; Morgan et al., 2019; Pressl et al., 2019). As a neuroimaging technology with the advantage of high spatial and temporal resolution, will MEG resting-state connectivity be a candidate predictor of surgical outcome in epilepsy?
The MEG resting-state connectivity reconstructed from the low-frequency oscillations has been assumed to be associated with surgical outcome. Using the metric of the imaginary part of the coherence on the resting-state MEG signals, Englot et al. (2015) demonstrated that patients with increased connectivity in the alpha band within the resected region were more likely to have favorable outcomes. However, inconsistent results have reported a connectivity in the alpha band between the brain regions where IEDs are generated and that the remainder of the cortex was weaker for seizure-free patients but stronger for non-seizure-free patients compared with the connectivity in the alpha band between the corresponding contralateral homologous region and the remainder of the cortex (Aydin et al., 2020). One possible reason for this finding involves the different metrics selected, i.e., imaginary part of the coherence and AECs, to calculate the resting-state functional connectivity. Several MEG studies have investigated the resting-state connectivity based on HFO signals (Nissen et al., 2016b; Meng, 2019; Yin et al., 2020) without investigating the correlation between HFO connectivity and postoperative outcomes. However, an increased directed connectivity in the ripple band was found in the resected area of patients with good postoperative outcomes using ECoG (Zweiphenning et al., 2019).
MEG network hubs, namely, regions with high network connectivity, are associated with the surgical outcome. Nissen et al. (2017) investigated whether MEG network hubs overlapped more with the resection area in seizure-free patients and found that hubs were localized within the area later resected in nine of 14 seizure-free patients and in none of eight patients who were not seizure-free. They subsequently investigated whether this overlap is distinct between seizure-free patients and non-seizure-free patients after surgery but found no significant difference in the overlap between the two surgery outcomes (Nissen et al., 2018). Moreover, a recent study investigated MEG network hubs and their removal in a cohort of 31 patients with refractory focal epilepsy and reported that seizure-free patients had more network hubs surgically removed than non-seizure-free patients (Ramaraju et al., 2020), which suggests that removing MEG network hubs may be a method for improving surgical outcomes. As a result, MEG resting-state connectivity should be considered a potential predictor of surgical outcome, which would be added to the presurgical evaluation of epilepsy.
MEG Resting-State Connectivity in Eyes-Closed and Eyes-Open States
Considering that the subjects requested to keep their eyes closed (EC) or eyes open (EO) during the resting-state MEG scan, it is important to know whether the choice of keeping EC or EO affects the resting-state functional connectivity. Previous studies have demonstrated significant differences in the resting-state connectivity patterns between EC conditions and EO conditions using fMRI (Yan et al., 2009; van Dijk et al., 2010; Liu et al., 2013; Patriat et al., 2013; Wei et al., 2018; Agcaoglu et al., 2019, 2020; Weng et al., 2020). However, few studies have investigated the potential difference in the resting-state functional connectivity in the EC and EO states using MEG in the same manner as fMRI. Horstmann et al. (2010) analyzed the resting-state functional brain networks of epileptic patients and healthy controls from EEG and MEG data recorded in EC and EO conditions and indicated that functional networks in both groups are more regular during the EC state compared with the EO state, which is derived from the EEG recordings but hardly derived from the MEG recordings. Liu et al. (2010) reported the distinct alpha-band activities of MEG signals under EC and EO conditions. Jin et al. (2014) further investigated the brain functional network in the EC and EO conditions of the MEG resting state from 39 healthy subjects and found an enhanced functional connectivity in the theta and alpha bands during the EO state relative to the EC state. In a recent study, functional connectivity in the theta band was significantly different between healthy subjects in the EO state and focal epileptic patients in the EC state (Pourmotabbed et al., 2020); however, the role of eye behavior states was uncertain.
These significant differences in the resting-state functional connectivity between the EO state and the EC state may support the hypothesis of Marx et al. (2003) on mental states, including the “interoceptive” network, which was characterized by imagination and multisensory activity during EC, and the “exteroceptive” network, which can be characterized by the attention and ocular motor activity during the EO state. Both of these states constitute intrinsic brain activity. The alpha activity was assumed to be associated with eye states, and EEG and MEG were simultaneously employed to analyze the “alpha” functional network of the specific regions of the brain insusceptible to the different eye conditions. The functional connectivity of the distinct brain regions decreased over time (Anwar et al., 2014). Considering that the resting state with EO or EC might influence functional connectivity, performing an analysis using data from both states would add further information to this area of uncertainty.
Conclusion
Epilepsy has been widely considered an archetypical brain network disorder that is commonly investigated using the resting-state functional connectivity. With its advantages of high temporal resolution and spatial resolution, MEG has been increasingly used to investigate the resting-state functional connectivity in epileptic presurgical evaluation. In this paper, the different metrics of abnormal functional connectivity are reviewed based on low-frequency signals and high-frequency oscillations. The application of MEG combined with iEEG approaches enhances the characterization of functional connectivity in the resting-state network, which provides complementary information for improving epilepsy surgery. More importantly, the resting-state functional connectivity based on MEG signals represents a potential predictor of postsurgical seizure outcome. Considering the resting-state connectivity differences between eyes-open conditions and eyes-closed conditions, an approach performing analysis using data from both states would be added to obtain more information about this area of uncertainty. The findings reviewed here provide grounds that the resting-state functional connectivity derived from MEG signals provides a strong contribution to a presurgical evaluation in epilepsy.
Author Contributions
NX, WS, JQ, JW, and QW all contributed to the writing and revision of this manuscript. All authors contributed to the article and approved the submitted version.
Funding
The study was financially supported by the National Key R&D Program of China grant (2017YFC1307500), the Capital Health Research and Development of Special grants (2016-1-2011 and 2020-1-2013), the Beijing-Tianjin-Hebei Cooperative Basic Research Program (H2018206435), and the Beijing Natural Science Foundation (Z200024).
Conflict of Interest
The authors declare that the research was conducted in the absence of any commercial or financial relationships that could be construed as a potential conflict of interest.
References
Agcaoglu, O., Wilson, T. W., Wang, Y. P., Stephen, J., and Calhoun, V. D. (2019). Resting state connectivity differences in eyes open versus eyes closed conditions. Hum. Brain Mapp. 40, 2488–2498. doi: 10.1002/hbm.24539
Agcaoglu, O., Wilson, T. W., Wang, Y. P., Stephen, J. M., and Calhoun, V. D. (2020). Dynamic resting-state connectivity differences in eyes open versus eyes closed conditions. Brain Connect. 10, 504–519. doi: 10.1089/brain.2020.0768
Akiyama, T., Otsubo, H., Ochi, A., Ishiguro, T., Kadokura, G., Ramachandrannair, R., et al. (2005). Focal cortical high-frequency oscillations trigger epileptic spasms: confirmation by digital video subdural EEG. Clin. Neurophysiol. 116, 2819–2825. doi: 10.1016/j.clinph.2005.08.029
Almubarak, S., Alexopoulos, A., Von-Podewils, F., Wang, Z. I., Kakisaka, Y., Mosher, J. C., et al. (2014). The correlation of magnetoencephalography to intracranial EEG in localizing the epileptogenic zone: a study of the surgical resection outcome. Epilepsy Res. 108, 1581–1590. doi: 10.1016/j.eplepsyres.2014.08.016
Amblard, C., Lapalme, E., and Lina, J. M. (2004). Biomagnetic source detection by maximum entropy and graphical models. IEEE Trans. Biomed. Eng. 51, 427–442. doi: 10.1109/tbme.2003.820999
Andrade-Valença, L., Mari, F., Jacobs, J., Zijlmans, M., Olivier, A., Gotman, J., et al. (2012). Interictal high frequency oscillations (HFOs) in patients with focal epilepsy and normal MRI. Clin. Neurophysiol. 123, 100–105. doi: 10.1016/j.clinph.2011.06.004
Anwar, A. R., Mideska, K. G., Hellriegel, H., Hoogenboom, N., Krause, H., Schnitzler, A., et al. (2014). Multi-modal causality analysis of eyes-open and eyes-closed data from simultaneously recorded EEG and MEG. Annu. Int. Conf. IEEE Eng. Med. Biol. Soc. 2014, 2825–2828. doi: 10.1109/EMBC.2014.6944211
Aydin, Ü., Pellegrino, G., Ali, O. B. K., Abdallah, C., Dubeau, F., Lina, J. M., et al. (2020). Magnetoencephalography resting state connectivity patterns as indicatives of surgical outcome in epilepsy patients. J. Neural Eng. 17:035007. doi: 10.1088/1741-2552/ab8113
Badier, J. M., Dubarry, A. S., Gavaret, M., Chen, S., Trébuchon, A. S., Marquis, P., et al. (2017). Technical solutions for simultaneous MEG and SEEG recordings: towards routine clinical use. Physiol. Meas. 38, N118–N127. doi: 10.1088/1361-6579/aa7655
Bagic, A. I., Knowlton, R. C., Rose, D. F., Ebersole, J. S., and ACMEGS Clinical Practice Guideline (CPG) Committee. (2011). American clinical magnetoencephalography society clinical practice guideline 1: recording and analysis of spontaneous cerebral activity. J. Clin. Neurophysiol. 28, 348–354. doi: 10.1097/WNP.0b013e3182272fed
Baillet, S. (2017). Magnetoencephalography for brain electrophysiology and imaging. Nat. Neurosci. 20, 327–339. doi: 10.1038/nn.4504
Baillet, S., Mosher, J. C., and Leahy, R. M. (2002). Electromagnetic brain mapping. IEEE Signal Proc. Mag. 18, 14–30. doi: 10.1109/79.962275
Biswal, B., Yetkin, F. Z., Haughton, V. M., and Hyde, J. S. (1995). Functional connectivity in the motor cortex of resting human brain using echo-planar MRI. Magn. Reson. Med. 34, 537–541. doi: 10.1002/mrm.1910340409
Bouet, R., Jung, J., Delpuech, C., Ryvlin, P., Isnard, J., Guenot, M., et al. (2012). Towards source volume estimation of interictal spikes in focal epilepsy using magnetoencephalography. Neuroimage 59, 3955–3966. doi: 10.1016/j.neuroimage.2011.10.052
Bragin, A., Engel, J., Wilson, C. L., Fried, I., and Buzsáki, G. J. H. (1999). High-frequency oscillations in human brain. Hippocampus 9, 137–142. doi: 10.1002/(sici)1098-1063(1999)9:2<137::aid-hipo5>3.0.co;2-0
Brázdil, M., Pail, M., Halámek, J., Plešinger, F., Cimbálník, J., Roman, R., et al. (2017). Very high-frequency oscillations: novel biomarkers of the epileptogenic zone. Ann. Neurol. 82, 299–310. doi: 10.1002/ana.25006
Brookes, M. J., Woolrich, M., Luckhoo, H., Price, D., Hale, J. R., Stephenson, M. C., et al. (2011). Investigating the electrophysiological basis of resting state networks using magnetoencephalography. Proc. Natl. Acad. Sci. U.S.A. 108, 16783–16788. doi: 10.1073/pnas.1112685108
Buzsáki, G., and Draguhn, A. (2004). Neuronal oscillations in cortical networks. Science 304, 1926–1929. doi: 10.1126/science.1099745
Candelaria-Cook, F. T., and Stephen, J. M. (2020). Test-retest reliability of magnetoencephalography resting-state functional connectivity in schizophrenia. Front. Psychiatry 11:551952. doi: 10.3389/fpsyt.2020.551952
Carrette, E., and Stefan, H. (2019). Evidence for the role of magnetic source imaging in the presurgical evaluation of refractory epilepsy patients. Front. Neurol. 10:933. doi: 10.3389/fneur.2019.00933
Chakraborty, A. R., Almeida, N. C., Prather, K. Y., O'Neal, C. M., Wells, A. A., Chen, S., et al. (2020). Resting-state functional magnetic resonance imaging with independent component analysis for presurgical seizure onset zone localization: a systematic review and meta-analysis. Epilepsia 61, 1958–1968. doi: 10.1111/epi.16637
Chavez, M., Valencia, M., Navarro, V., Latora, V., and Martinerie, J. (2010). Functional modularity of background activities in normal and epileptic brain networks. Phys. Rev. Lett. 104:118701. doi: 10.1103/PhysRevLett.104.118701
Chowdhury, F. A., Woldman, W., FitzGerald, T. H., Elwes, R. D., Nashef, L., Terry, J. R., et al. (2014). Revealing a brain network endophenotype in families with idiopathic generalised epilepsy. PLoS ONE 9:e110136. doi: 10.1371/journal.pone.0110136
Cimbalnik, J., Brinkmann, B., Kremen, V., Jurak, P., Berry, B., Gompel, J. V., et al. (2018). Physiological and pathological high frequency oscillations in focal epilepsy. Ann. Clin. Transl. Neurol. 5, 1062–1076. doi: 10.1002/acn3.618
Clemens, B., Puskas, S., Bessenyei, M., Emri, M., Spisak, T., Koselak, M., et al. (2011). EEG functional connectivity of the intrahemispheric cortico-cortical network of idiopathic generalized epilepsy. Epilepsy Res. 96, 11–23. doi: 10.1016/j.eplepsyres.2011.04.011
Coito, A., Genetti, M., Pittau, F., Iannotti, G. R., Thomschewski, A., Höller, Y., et al. (2016b). Altered directed functional connectivity in temporal lobe epilepsy in the absence of interictal spikes: a high density EEG study. Epilepsia 57, 402–411. doi: 10.1111/epi.13308
Coito, A., Michel, C. M., van Mierlo, P., Vulliemoz, S., and Plomp, G. (2016a). Directed functional brain connectivity based on EEG source imaging: methodology and application to temporal lobe epilepsy. IEEE Trans. Biomed. Eng. 63, 2619–2628. doi: 10.1109/tbme.2016.2619665
Colclough, G. L., Woolrich, M. W., Tewarie, P. K., Brookes, M. J., Quinn, A. J., and Smith, S. M. (2016). How reliable are MEG resting-state connectivity metrics? Neuroimage 138, 284–293. doi: 10.1016/j.neuroimage.2016.05.070
Courtiol, J., Guye, M., Bartolomei, F., Petkoski, S., and Jirsa, V. K. (2020). Dynamical mechanisms of interictal resting-state functional connectivity in epilepsy. J. Neurosci. 40, 5572–5588. doi: 10.1523/jneurosci.0905-19.2020
Crespo-García, M., Zeiller, M., Leupold, C., Kreiselmeyer, G., Rampp, S., Hamer, H. M., et al. (2016). Slow-theta power decreases during item-place encoding predict spatial accuracy of subsequent context recall. Neuroimage 142, 533–543. doi: 10.1016/j.neuroimage.2016.08.021
Cuello-Oderiz, C., von Ellenrieder, N., Sankhe, R., Olivier, A., Hall, J., Dubeau, F., et al. (2018). Value of ictal and interictal epileptiform discharges and high frequency oscillations for delineating the epileptogenic zone in patients with focal cortical dysplasia. Clin. Neurophysiol. 129, 1311–1319. doi: 10.1016/j.clinph.2018.02.003
Dale, A. M., and Sereno, M. I. (1993). Improved localizadon of cortical activity by combining EEG and MEG with MRI cortical surface reconstruction: a linear approach. J. Cogn. Neurosci. 5, 162–176. doi: 10.1162/jocn.1993.5.2.162
Darvas, F., Pantazis, D., Kucukaltun-Yildirim, E., and Leahy, R. M. (2004). Mapping human brain function with MEG and EEG: methods and validation. Neuroimage 23 (Suppl. 1), S289–299. doi: 10.1016/j.neuroimage.2004.07.014
de Munck, J. C., Wolters, C. H., and Clerc, M. (2012). “EEG and MEG-forward modelling,” in Handbook of Neural Activity Measurement, eds R. Brette and A. Destexhe (Cambridge: Cambridge University Press), 192–256.
Demont-Guignard, S., Benquet, P., Gerber, U., Biraben, A., Martin, B., and Wendling, F. (2012). Distinct hyperexcitability mechanisms underlie fast ripples and epileptic spikes. Ann. Neurol. 71, 342–352. doi: 10.1002/ana.22610
Dimitriadis, S. I., Routley, B., Linden, D. E., and Singh, K. D. (2018). Reliability of static and dynamic network metrics in the resting-state: a MEG-beamformed connectivity analysis. Front. Neurosci. 12:506. doi: 10.3389/fnins.2018.00506
Douw, L., van Dellen, E., de Groot, M., Heimans, J. J., Klein, M., Stam, C. J., et al. (2010). Epilepsy is related to theta band brain connectivity and network topology in brain tumor patients. BMC Neurosci. 11:103. doi: 10.1186/1471-2202-11-103
Dubarry, A. S., Badier, J. M., Trébuchon-Da Fonseca, A., Gavaret, M., Carron, R., Bartolomei, F., et al. (2014). Simultaneous recording of MEG, EEG and intracerebral EEG during visual stimulation: from feasibility to single-trial analysis. Neuroimage 99, 548–558. doi: 10.1016/j.neuroimage.2014.05.055
Elshahabi, A., Klamer, S., Sahib, A. K., Lerche, H., Braun, C., and Focke, N. K. (2015). Magnetoencephalography reveals a widespread increase in network connectivity in Idiopathic/Genetic generalized epilepsy. PLoS ONE 10:e0138119. doi: 10.1371/journal.pone.0138119
Engel, A. K., and Fries, P. (2010). Beta-band oscillations-signalling the status quo? Curr. Opin. Neurobiol. 20, 156–165. doi: 10.1016/j.conb.2010.02.015
Englot, D. J., Hinkley, L. B., Kort, N. S., Imber, B. S., Mizuiri, D., Honma, S. M., et al. (2015). Global and regional functional connectivity maps of neural oscillations in focal epilepsy. Brain 138, 2249–2262. doi: 10.1093/brain/awv130
Englot, D. J., Konrad, P. E., and Morgan, V. L. (2016). Regional and global connectivity disturbances in focal epilepsy, related neurocognitive sequelae, and potential mechanistic underpinnings. Epilepsia 57, 1546–1557. doi: 10.1111/epi.13510
Feyissa, A. M., Britton, J. W., Van Gompel, J., Lagerlund, T. L., So, E., Wong-Kisiel, L. C., et al. (2017). High density scalp EEG in frontal lobe epilepsy. Epilepsy Res. 129, 157–161. doi: 10.1016/j.eplepsyres.2016.12.016
Fisher, R. S., Van Emde Boas, W., Blume, W., Elger, C., Genton, P., Lee, P., et al. (2005). Response: definitions proposed by the international league against epilepsy (ILAE) and the international bureau for epilepsy (IBE). Epilepsia 46, 1701–1702. doi: 10.1111/j.1528-1167.2005.00273_4.x
Fransson, P., and Marrelec, G. (2008). The precuneus/posterior cingulate cortex plays a pivotal role in the default mode network: evidence from a partial correlation network analysis. Neuroimage 42, 1178–1184. doi: 10.1016/j.neuroimage.2008.05.059
Fuertinger, S., Simonyan, K., Sperling, M. R., Sharan, A. D., and Hamzei-Sichani, F. (2016). High-frequency brain networks undergo modular breakdown during epileptic seizures. Epilepsia 57, 1097–1108. doi: 10.1111/epi.13413
Garcés, P., Martín-Buro, M. C., and Maestú, F. (2016). Quantifying the test-retest reliability of magnetoencephalography resting-state functional connectivity. Brain Connect. 6, 448–460. doi: 10.1089/brain.2015.0416
Gavaret, M., Dubarry, A. S., Carron, R., Bartolomei, F., Trébuchon, A., and Bénar, C. G. (2016). Simultaneous SEEG-MEG-EEG recordings overcome the SEEG limited spatial sampling. Epilepsy Res. 128, 68–72. doi: 10.1016/j.eplepsyres.2016.10.013
Gotman, J., Grova, C., Bagshaw, A., Kobayashi, E., Aghakhani, Y., and Dubeau, F. (2005). Generalized epileptic discharges show thalamocortical activation and suspension of the default state of the brain. Proc. Natl. Acad. Sci. U.S.A 102, 15236–15240. doi: 10.1073/pnas.0504935102
Gramfort, A., Luessi, M., Larson, E., Engemann, D. A., Strohmeier, D., Brodbeck, C., et al. (2014). MNE software for processing MEG and EEG data. Neuroimage 86, 446–460. doi: 10.1016/j.neuroimage.2013.10.027
Grova, C., Aiguabella, M., Zelmann, R., Lina, J. M., Hall, J. A., and Kobayashi, E. (2016). Intracranial EEG potentials estimated from MEG sources: a new approach to correlate MEG and iEEG data in epilepsy. Hum. Brain Mapp. 37, 1661–1683. doi: 10.1002/hbm.23127
Hader, W. J., Tellez-Zenteno, J., Metcalfe, A., Hernandez-Ronquillo, L., Wiebe, S., Kwon, C. S., et al. (2013). Complications of epilepsy surgery: a systematic review of focal surgical resections and invasive EEG monitoring. Epilepsia 54, 840–847. doi: 10.1111/epi.12161
Hämäläinen, M., Hari, R., Ilmoniemi, R., Knuutila, J., and Lounasmaa, O. (1993). Magnetoencephalography-theory, instrumentation, and applications to noninvasive studies of the working human brain. Rev. Mod. Phys. 65:413. doi: 10.1103/RevModPhys.65.413
Hämäläinen, M., Huang, M. X., and Bowyer, S. M. (2020). Magnetoencephalography signal processing, forward modeling, magnetoencephalography inverse source imaging, and coherence analysis. Neuroimaging Clin. N. Am. 30, 125–143. doi: 10.1016/j.nic.2020.02.001
Hämäläinen, M. S., and Ilmoniemi, R. J. (1994). Interpreting magnetic fields of the brain: minimum norm estimates. Med. Biol. Eng. Comput. 32, 35–42. doi: 10.1007/bf02512476
He, B., Dai, Y., Astolfi, L., Babiloni, F., Yuan, H., and Yang, L. (2011). eConnectome: a MATLAB toolbox for mapping and imaging of brain functional connectivity. J. Neurosci. Methods 195, 261–269. doi: 10.1016/j.jneumeth.2010.11.015
He, X., Doucet, G. E., Pustina, D., Sperling, M. R., Sharan, A. D., and Tracy, J. I. (2017). Presurgical thalamic “hubness” predicts surgical outcome in temporal lobe epilepsy. Neurology 88, 2285–2293. doi: 10.1212/wnl.0000000000004035
Hillebrand, A., Barnes, G. R., Bosboom, J. L., Berendse, H. W., and Stam, C. J. (2012). Frequency-dependent functional connectivity within resting-state networks: an atlas-based MEG beamformer solution. Neuroimage 59, 3909–3921. doi: 10.1016/j.neuroimage.2011.11.005
Hincapié, A. S., Kujala, J., Mattout, J., Pascarella, A., Daligault, S., Delpuech, C., et al. (2017). The impact of MEG source reconstruction method on source-space connectivity estimation: A comparison between minimum-norm solution and beamforming. Neuroimage 156, 29–42. doi: 10.1016/j.neuroimage.2017.04.038
Hipp, J. F., Hawellek, D. J., Corbetta, M., Siegel, M., and Engel, A. K. (2012). Large-scale cortical correlation structure of spontaneous oscillatory activity. Nat. Neurosci. 15, 884–890. doi: 10.1038/nn.3101
Horstmann, M. T., Bialonski, S., Noennig, N., Mai, H., Prusseit, J., Wellmer, J., et al. (2010). State dependent properties of epileptic brain networks: comparative graph-theoretical analyses of simultaneously recorded EEG and MEG. Clin. Neurophysiol. 121, 172–185. doi: 10.1016/j.clinph.2009.10.013
Hsiao, F. J., Yu, H. Y., Chen, W. T., Kwan, S. Y., Chen, C., Yen, D. J., et al. (2015). Increased intrinsic connectivity of the default mode network in temporal lobe epilepsy: evidence from resting-state MEG recordings. PLoS ONE 10:e0128787. doi: 10.1371/journal.pone.0128787
Huang, M., Aine, C. J., Supek, S., Best, E., Ranken, D., and Flynn, E. R. (1998). Multi-start downhill simplex method for spatio-temporal source localization in magnetoencephalography. Electroencephalogr. Clin. Neurophysiol. 108, 32–44. doi: 10.1016/s0168-5597(97)00091-9
Huang, M. X., Mosher, J. C., and Leahy, R. M. (1999). A sensor-weighted overlapping-sphere head model and exhaustive head model comparison for MEG. Phys. Med. Biol. 44, 423–440. doi: 10.1088/0031-9155/44/2/010
Ikeda, S., and Toyama, K. (2000). Independent component analysis for noisy data–MEG data analysis. Neural Netw. 13, 1063–1074. doi: 10.1016/s0893-6080(00)00071-x
Iversen, J. R., and Makeig, S. (2014). “MEG/EEG data analysis using EEGLAB,” in Magnetoencephalography. From Signals to Dynamic Cortical Networks, eds S. Supek and C. J. Aine (Berlin, Germany: Springer), 199–212.
Jacobs, J., LeVan, P., Chander, R., Hall, J., Dubeau, F., and Gotman, J. (2008). Interictal high-frequency oscillations (80-500 Hz) are an indicator of seizure onset areas independent of spikes in the human epileptic brain. Epilepsia 49, 1893–1907. doi: 10.1111/j.1528-1167.2008.01656.x
Jacobs, J., Staba, R., Asano, E., Otsubo, H., Wu, J. Y., Zijlmans, M., et al. (2012). High-frequency oscillations (HFOs) in clinical epilepsy. Prog. Neurobiol. 98, 302–315. doi: 10.1016/j.pneurobio.2012.03.001
Jeong, W., Jin, S. H., Kim, M., Kim, J. S., and Chung, C. K. (2014). Abnormal functional brain network in epilepsy patients with focal cortical dysplasia. Epilepsy Res. 108, 1618–1626. doi: 10.1016/j.eplepsyres.2014.09.006
Jin, S. H., Jeong, W., Lee, D. S., Jeon, B. S., and Chung, C. K. (2014). Preserved high-centrality hubs but efficient network reorganization during eyes-open state compared with eyes-closed resting state: an MEG study. J. Neurophysiol. 111, 1455–1465. doi: 10.1152/jn.00585.2013
Jirsch, J. D., Urrestarazu, E., LeVan, P., Olivier, A., Dubeau, F., and Gotman, J. (2006). High-frequency oscillations during human focal seizures. Brain 129, 1593–1608. doi: 10.1093/brain/awl085
Jmail, N., Gavaret, M., Bartolomei, F., Chauvel, P., Badier, J. M., and Bénar, C. G. (2016). Comparison of brain networks during interictal oscillations and spikes on magnetoencephalography and intracerebral EEG. Brain Topogr. 29, 752–765. doi: 10.1007/s10548-016-0501-7
Jobst, B. C., and Cascino, G. D. (2015). Resective epilepsy surgery for drug-resistant focal epilepsy: a review. JAMA 313, 285–293. doi: 10.1001/jama.2014.17426
Kakisaka, Y., Kubota, Y., Wang, Z. I., Piao, Z., Mosher, J. C., Gonzalez-Martinez, J., et al. (2012). Use of simultaneous depth and MEG recording may provide complementary information regarding the epileptogenic region. Epileptic Disord. 14, 298–303. doi: 10.1684/epd.2012.0517
Kleiner, R., Koelle, D., Ludwig, F., and Clarke, J. J. (2004). Superconducting quantum interference devices: state of the art and applications. Proc. IEEE 92, 1534–1548. doi: 10.1109/JPROC.2004.833655
Knowlton, R. C., Elgavish, R., Howell, J., Blount, J., Burneo, J. G., Faught, E., et al. (2006). Magnetic source imaging versus intracranial electroencephalogram in epilepsy surgery: a prospective study. Ann. Neurol. 59, 835–842. doi: 10.1002/ana.20857
Knowlton, R. C., Razdan, S. N., Limdi, N., Elgavish, R. A., Killen, J., Blount, J., et al. (2009). Effect of epilepsy magnetic source imaging on intracranial electrode placement. Ann. Neurol. 65, 716–723. doi: 10.1002/ana.21660
Kramer, M. A., and Cash, S. S. (2012). Epilepsy as a disorder of cortical network organization. Neuroscientist 18, 360–372. doi: 10.1177/1073858411422754
Kramer, M. A., Kolaczyk, E. D., and Kirsch, H. E. (2008). Emergent network topology at seizure onset in humans. Epilepsy Res. 79, 173–186. doi: 10.1016/j.eplepsyres.2008.02.002
Krishnan, B., Vlachos, I., Wang, Z. I., Mosher, J., Najm, I., Burgess, R., et al. (2015). Epileptic focus localization based on resting state interictal MEG recordings is feasible irrespective of the presence or absence of spikes. Clin. Neurophysiol. 126, 667–674. doi: 10.1016/j.clinph.2014.07.014
Kwan, P., Schachter, S. C., and Brodie, M. J. (2011). Drug-resistant epilepsy. New Engl. J. Med. 365, 919–926. doi: 10.1056/NEJMra1004418
Leahy, R. M., Mosher, J. C., Spencer, M. E., Huang, M. X., and Lewine, J. D. (1998). A study of dipole localization accuracy for MEG and EEG using a human skull phantom. Electroencephalogr. Clin. Neurophysiol. 107, 159–173. doi: 10.1016/s0013-4694(98)00057-1
Leng, X., Xiang, J., Yang, Y., Yu, T., Qi, X., Zhang, X., et al. (2020). Frequency-specific changes in the default mode network in patients with cingulate gyrus epilepsy. Hum. Brain Mapp. 41, 2447–2459. doi: 10.1002/hbm.24956
Li Hegner, Y., Marquetand, J., Elshahabi, A., Klamer, S., Lerche, H., Braun, C., et al. (2018). Increased functional MEG connectivity as a hallmark of MRI-negative focal and generalized epilepsy. Brain Topogr. 31, 863–874. doi: 10.1007/s10548-018-0649-4
Liao, W., Zhang, Z., Pan, Z., Mantini, D., Ding, J., Duan, X., et al. (2010). Altered functional connectivity and small-world in mesial temporal lobe epilepsy. PLoS ONE 5:e8525. doi: 10.1371/journal.pone.0008525
Litvak, V., Mattout, J., Kiebel, S., Phillips, C., Henson, R., Kilner, J., et al. (2011). EEG and MEG data analysis in SPM8. Comput. Intell. Neurosci. 2011, 852961. doi: 10.1155/2011/852961
Liu, D., Dong, Z., Zuo, X., Wang, J., and Zang, Y. (2013). Eyes-open/eyes-closed dataset sharing for reproducibility evaluation of resting state fMRI data analysis methods. Neuroinformatics 11, 469–476. doi: 10.1007/s12021-013-9187-0
Liu, S., Gurses, C., Sha, Z., Quach, M. M., Sencer, A., Bebek, N., et al. (2018). Stereotyped high-frequency oscillations discriminate seizure onset zones and critical functional cortex in focal epilepsy. Brain 141, 713–730. doi: 10.1093/brain/awx374
Liu, Z., Fukunaga, M., de Zwart, J. A., and Duyn, J. H. (2010). Large-scale spontaneous fluctuations and correlations in brain electrical activity observed with magnetoencephalography. Neuroimage 51, 102–111. doi: 10.1016/j.neuroimage.2010.01.092
Liuzzi, L., Gascoyne, L. E., Tewarie, P. K., Barratt, E. L., Boto, E., and Brookes, M. J. (2017). Optimising experimental design for MEG resting state functional connectivity measurement. Neuroimage 155, 565–576. doi: 10.1016/j.neuroimage.2016.11.064
Malmgren, K., and Edelvik, A. (2017). Long-term outcomes of surgical treatment for epilepsy in adults with regard to seizures, antiepileptic drug treatment and employment. Seizure 44, 217–224. doi: 10.1016/j.seizure.2016. 10.015
Marquetand, J., Vannoni, S., Carboni, M., Li Hegner, Y., Stier, C., Braun, C., et al. (2019). Reliability of magnetoencephalography and high-density electroencephalography resting-state functional connectivity metrics. Brain Connect. 9, 539–553. doi: 10.1089/brain.2019.0662
Martire, D. J., Wong, S., Workewych, A., Pang, E., Boutros, S., Smith, M. L., et al. (2020). Temporal-plus epilepsy in children: a connectomic analysis in magnetoencephalography. Epilepsia 61, 1691–1700. doi: 10.1111/epi.16591
Marx, E., Stephan, T., Nolte, A., Deutschländer, A., Seelos, K. C., Dieterich, M., et al. (2003). Eye closure in darkness animates sensory systems. Neuroimage 19, 924–934. doi: 10.1016/s1053-8119(03)00150-2
Melani, F., Zelmann, R., Mari, F., and Gotman, J. (2013). Continuous high frequency activity: a peculiar SEEG pattern related to specific brain regions. Clin. Neurophysiol. 124, 1507–1516. doi: 10.1016/j.clinph.2012.11.016
Meng, L. (2019). A Magnetoencephalography study of pediatric interictal neuromagnetic activity changes and brain network alterations caused by epilepsy in the high frequency (80-1000 Hz). IEEE Trans. Neural Syst. Rehabil. Eng. 27, 389–399. doi: 10.1109/TNSRE.2019.2898683
Meng, L., and Xiang, J. (2016). Frequency specific patterns of resting-state networks development from childhood to adolescence: a magnetoencephalography study. Brain Dev. 38, 893–902. doi: 10.1016/j.braindev.2016.05.004
Miao, A., Xiang, J., Tang, L., Ge, H., Liu, H., Wu, T., et al. (2014). Using ictal high-frequency oscillations (80-500Hz) to localize seizure onset zones in childhood absence epilepsy: a MEG study. Neurosci. Lett. 566, 21–26. doi: 10.1016/j.neulet.2014.02.038
Morgan, V. L., Rogers, B. P., González, H. F. J., Goodale, S. E., and Englot, D. J. (2019). Characterization of postsurgical functional connectivity changes in temporal lobe epilepsy. J. Neurosurg. 14, 1–11. doi: 10.3171/2019.3.Jns19350
Mosher, J. C., Lewis, P. S., and Leahy, R. M. (1992). Multiple dipole modeling and localization from spatio-temporal MEG data. IEEE Trans. Biomed. Eng. 39, 541–557. doi: 10.1109/10.141192
Murakami, H., Wang, Z. I., Marashly, A., Krishnan, B., Prayson, R. A., Kakisaka, Y., et al. (2016). Correlating magnetoencephalography to stereo-electroencephalography in patients undergoing epilepsy surgery. Brain 139, 2935–2947. doi: 10.1093/brain/aww215
Niso, G., Bruña, R., Pereda, E., Gutiérrez, R., Bajo, R., Maest,ú, F., et al. (2013). HERMES: towards an integrated toolbox to characterize functional and effective brain connectivity. Neuroinformatics 11, 405–434. doi: 10.1007/s12021-013-9186-1
Niso, G., Carrasco, S., Gudín, M., Maestú, F., Del-Pozo, F., and Pereda, E. (2015). What graph theory actually tells us about resting state interictal MEG epileptic activity. Neuroimage Clin. 8, 503–515. doi: 10.1016/j.nicl.2015.05.008
Niso, G., Tadel, F., Bock, E., Cousineau, M., Santos, A., and Baillet, S. (2019). Brainstorm pipeline analysis of resting-state data from the open MEG archive. Front. Neurosci. 13:284. doi: 10.3389/fnins.2019.00284
Nissen, I. A., Stam, C. J., Citroen, J., Reijneveld, J. C., and Hillebrand, A. (2016a). Preoperative evaluation using magnetoencephalography: experience in 382 epilepsy patients. Epilepsy Res. 124, 23–33. doi: 10.1016/j.eplepsyres.2016.05.002
Nissen, I. A., Stam, C. J., Reijneveld, J. C., van Straaten, I. E., Hendriks, E. J., Baayen, J. C., et al. (2017). Identifying the epileptogenic zone in interictal resting-state MEG source-space networks. Epilepsia 58, 137–148. doi: 10.1111/epi.13622
Nissen, I. A., Stam, C. J., van Straaten, E. C. W., Wottschel, V., Reijneveld, J. C., Baayen, J. C., et al. (2018). Localization of the epileptogenic zone using interictal MEG and machine learning in a large cohort of drug-resistant epilepsy patients. Front. Neurol. 9:647. doi: 10.3389/fneur.2018.00647
Nissen, I. A., van Klink, N. E., Zijlmans, M., Stam, C. J., and Hillebrand, A. (2016b). Brain areas with epileptic high frequency oscillations are functionally isolated in MEG virtual electrode networks. Clin. Neurophysiol. 127, 2581–2591. doi: 10.1016/j.clinph.2016.04.013
Oishi, M., Kameyama, S., Masuda, H., Masuda, H., Tohyama, J., Kanazawa, O., et al. (2006). Single and multiple clusters of magnetoencephalographic dipoles in neocortical epilepsy: significance in characterizing the epileptogenic zone. Epilepsia 47, 355–364. doi: 10.1111/j.1528-1167.2006.00428.x
Oishi, M., Otsubo, H., Kameyama, S., Morota, N., Masuda, H., Kitayama, M., et al. (2002). Epileptic spikes: magnetoencephalography versus simultaneous electrocorticography. Epilepsia 43, 1390–1395. doi: 10.1046/j.1528-1157.2002.10702.x
Olivi, E. (2011). Coupling of Numerical Methods for the Forward Problem in Magneto- and Electro-Encephalography. Numerical Analysis [cs.NA]. Nice: Université Nice Sophia Antipolis. English.
Oostenveld, R., Fries, P., Maris, E., and Schoffelen, J. M. (2011). FieldTrip: open source software for advanced analysis of MEG, EEG, and invasive electrophysiological data. Comput. Intell. Neurosci. 2011:156869. doi: 10.1155/2011/156869
Pail, M., Cimbálník, J., Roman, R., Daniel, P., Shaw, D. J., Chrastina, J., et al. (2020). High frequency oscillations in epileptic and non-epileptic human hippocampus during a cognitive task. Sci. Rep. 10:18147. doi: 10.1038/s41598-020-74306-3
Palacios, E. M., Sala-Llonch, R., Junque, C., Roig, T., Tormos, J. M., Bargallo, N., et al. (2013). Resting-state functional magnetic resonance imaging activity and connectivity and cognitive outcome in traumatic brain injury. JAMA Neurol. 70, 845–851. doi: 10.1001/jamaneurol.2013.38
Palva, S., and Palva, J. M. (2011). Functional roles of alpha-band phase synchronization in local and large-scale cortical networks. Front. Psychol. 2:204. doi: 10.3389/fpsyg.2011.00204
Parkkonen, L. (2010). “Instrumentation and data preprocessing,” in MEG: An Introduction to Methods, eds P. Hansen, M. Kringelbach, and R. Salmelin (New York, NY: Oxford University Press), 24–64.
Pascalicchio, T. F., de Araujo Filho, G. M., da Silva Noffs, M. H., Lin, K., Caboclo, L. O., Vidal-Dourado, M., et al. (2007). Neuropsychological profile of patients with juvenile myoclonic epilepsy: a controlled study of 50 patients. Epilepsy Behav. 10, 263–267. doi: 10.1016/j.yebeh.2006.11.012
Pascual-Marqui, R. D. (2002). Standardized low-resolution brain electromagnetic tomography (sLORETA): technical details. Methods Find. Exp. Clin. Pharmacol. 24 (Suppl. D), 5–12. doi: 10.1002/med.10000
Patriat, R., Molloy, E. K., Meier, T. B., Kirk, G. R., Nair, V. A., Meyerand, M. E., et al. (2013). The effect of resting condition on resting-state fMRI reliability and consistency: a comparison between resting with eyes open, closed, and fixated. Neuroimage 78, 463–473. doi: 10.1016/j.neuroimage.2013.04.013
Pourmotabbed, H., Wheless, J. W., and Babajani-Feremi, A. (2020). Lateralization of epilepsy using intra-hemispheric brain networks based on resting-state MEG data. Hum. Brain Mapp. 41, 2964–2979. doi: 10.1002/hbm.24990
Pressl, C., Brandner, P., Schaffelhofer, S., Blackmon, K., Dugan, P., Holmes, M., et al. (2019). Resting state functional connectivity patterns associated with pharmacological treatment resistance in temporal lobe epilepsy. Epilepsy Res. 149, 37–43. doi: 10.1016/j.eplepsyres.2018.11.002
Qi, L., Fan, X., Tao, X., Chai, Q., Zhang, K., Meng, F., et al. (2020). Identifying the epileptogenic zone with the relative strength of high-frequency oscillation: a stereoelectroencephalography study. Front. Hum. Neurosci. 14:186. doi: 10.3389/fnhum.2020.00186
Ramaraju, S., Wang, Y., Sinha, N., McEvoy, A. W., Miserocchi, A., de Tisi, J., et al. (2020). Removal of interictal MEG-derived network hubs is associated with postoperative seizure freedom. Front. Neurol. 11:563847. doi: 10.3389/fneur.2020.563847
Ramírez, R. R., Kopell, B. H., Butson, C. R., Hiner, B. C., and Baillet, S. (2011). Spectral signal space projection algorithm for frequency domain MEG and EEG denoising, whitening, and source imaging. Neuroimage 56, 78–92. doi: 10.1016/j.neuroimage.2011.02.002
Ren, G., Yan, J., Sun, Y., Ren, J., Dai, J., Mei, S., et al. (2020). Association between interictal high-frequency oscillations and slow wave in refractory focal epilepsy with good surgical outcome. Front. Hum. Neurosci. 14, 335. doi: 10.3389/fnhum.2020.00335
Ren, G. P., Yan, J. Q., Yu, Z. X., Wang, D., Li, X. N., Mei, S. S., et al. (2018). Automated detector of high frequency oscillations in epilepsy based on maximum distributed peak points. Int. J. Neural. Syst. 28:1750029. doi: 10.1142/S0129065717500290
Routley, B., Shaw, A., Muthukumaraswamy, S. D., Singh, K. D., and Hamandi, K. (2020). Juvenile myoclonic epilepsy shows increased posterior theta, and reduced sensorimotor beta resting connectivity. Epilepsy Res. 163:106324. doi: 10.1016/j.eplepsyres.2020.106324
Samogin, J., Marino, M., Porcaro, C., Wenderoth, N., Dupont, P., Swinnen, S. P., et al. (2020). Frequency-dependent functional connectivity in resting state networks. Hum. Brain Mapp. 41, 5187–5198. doi: 10.1002/hbm.25184
Samuelsson, J. G., Peled, N., Mamashli, F., Ahveninen, J., and Hämäläinen, M. S. (2021). Spatial fidelity of MEG/EEG source estimates: a general evaluation approach. Neuroimage 224:117430. doi: 10.1016/j.neuroimage.2020.117430
Santiuste, M., Nowak, R., Russi, A., Tarancon, T., Oliver, B., Ayats, E., et al. (2008). Simultaneous magnetoencephalography and intracranial EEG registration: technical and clinical aspects. J. Clin. Neurophysio. 25, 331–339. doi: 10.1097/WNP.0b013e31818e7913
Scarapicchia, V., Brown, C., Mayo, C., and Gawryluk, J. R. (2017). Functional magnetic resonance imaging and functional Near-Infrared Spectroscopy: insights from combined recording studies. Front. Hum. Neurosci. 11:419. doi: 10.3389/fnhum.2017.00419
Schindler, K. A., Bialonski, S., Horstmann, M. T., Elger, C. E., and Lehnertz, K. (2008). Evolving functional network properties and synchronizability during human epileptic seizures. Chaos 18:033119. doi: 10.1063/1.2966112
Schönberger, J., Draguhn, A., and Both, M. (2014). Lamina-specific contribution of glutamatergic and GABAergic potentials to hippocampal sharp wave-ripple complexes. Front. Neural Circuits 8:103. doi: 10.3389/fncir.2014.00103
Tadel, F., Baillet, S., Mosher, J. C., Pantazis, D., and Leahy, R. M. (2011). Brainstorm: a user-friendly application for MEG/EEG analysis. Comput. Intell. Neurosci. 2011:879716. doi: 10.1155/2011/879716
Tamilia, E., AlHilani, M., Tanaka, N., Tsuboyama, M., Peters, J. M., Grant, P. E., et al. (2019). Assessing the localization accuracy and clinical utility of electric and magnetic source imaging in children with epilepsy. Clin. Neurophysiol. 130, 491–504. doi: 10.1016/j.clinph.2019.01.009
Tamilia, E., Dirodi, M., Alhilani, M., Grant, P. E., Madsen, J. R., Stufflebeam, S. M., et al. (2020). Scalp ripples as prognostic biomarkers of epileptogenicity in pediatric surgery. Ann. Clin. Transl. Neurol. 7, 329–342. doi: 10.1002/acn3.50994
Tamilia, E., Madsen, J. R., Grant, P. E., Pearl, P. L., and Papadelis, C. (2017). Current and emerging potential of magnetoencephalography in the detection and localization of high-frequency oscillations in epilepsy. Front. Neurol. 8:14. doi: 10.3389/fneur.2017.00014
Taulu, S., Kajola, M., and Simola, J. (2004). Suppression of interference and artifacts by the signal space separation method. Brain Topogr. 16, 269–275. doi: 10.1023/b:brat.0000032864.93890.f9
Tenney, J. R., Fujiwara, H., Horn, P. S., Vannest, J., Xiang, J., Glauser, T. A., et al. (2014). Low- and high-frequency oscillations reveal distinct absence seizure networks. Ann. Neurol. 76, 558–567. doi: 10.1002/ana.24231
Thomschewski, A., Hincapie, A. S., and Frauscher, B. (2019). Localization of the epileptogenic zone using high frequency oscillations. Front. Neurol. 10:94. doi: 10.3389/fneur.2019.00094
Tracy, J. I., and Doucet, G. E. (2015). Resting-state functional connectivity in epilepsy: growing relevance for clinical decision making. Curr. Opin. Neurol. 28, 158–165. doi: 10.1097/wco.0000000000000178
Urrestarazu, E., Jirsch, J. D., LeVan, P., Hall, J., Avoli, M., Dubeau, F., et al. (2006). High-frequency intracerebral EEG activity (100–500 Hz) following interictal spikes. Epilepsia 47, 1465–1476. doi: 10.1111/j.1528-1167.2006.00618.x
Usui, N., Terada, K., Baba, K., Matsuda, K., Nakamura, F., Usui, K., et al. (2010). Very high frequency oscillations (over 1000 Hz) in human epilepsy. Clin. Neurophysiol. 121, 1825–1831. doi: 10.1016/j.clinph.2010.04.018
Usui, N., Terada, K., Baba, K., Matsuda, K., Usui, K., Tottori, T., et al. (2015). Significance of very-high-frequency oscillations (over 1,000 Hz) in epilepsy. Ann. Neurol. 78, 295–302. doi: 10.1002/ana.24440
van Dellen, E., de Witt Hamer, P. C., Douw, L., Klein, M., Heimans, J. J., Stam, C. J., et al. (2012a). Connectivity in MEG resting-state networks increases after resective surgery for low-grade glioma and correlates with improved cognitive performance. Neuroimage Clin. 2, 1–7. doi: 10.1016/j.nicl.2012.10.007
van Dellen, E., Douw, L., Hillebrand, A., Ris-Hilgersom, I. H., Schoonheim, M. M., Baayen, J. C., et al. (2012b). MEG network differences between low- and high-grade glioma related to epilepsy and cognition. PLoS ONE 7:e50122. doi: 10.1371/journal.pone.0050122
van Diessen, E., Diederen, S. J., Braun, K. P., Jansen, F. E., and Stam, C. J. (2013). Functional and structural brain networks in epilepsy: what have we learned? Epilepsia 54, 1855–1865. doi: 10.1111/epi.12350
van Diessen, E., Zweiphenning, W. J., Jansen, F. E., Stam, C. J., Braun, K. P., and Otte, W. M. (2014). Brain network organization in focal epilepsy: a systematic review and meta-analysis. PLoS ONE 9:e114606. doi: 10.1371/journal.pone.0114606
van Dijk, K. R., Hedden, T., Venkataraman, A., Evans, K. C., Lazar, S. W., and Buckner, R. L. (2010). Intrinsic functional connectivity as a tool for human connectomics: theory, properties, and optimization. J. Neurophysiol. 103, 297–321. doi: 10.1152/jn.00783.2009
van Klink, N., Hillebrand, A., and Zijlmans, M. (2016). Identification of epileptic high frequency oscillations in the time domain by using MEG beamformer-based virtual sensors. Clin. Neurophysiol. 127, 197–208. doi: 10.1016/j.clinph.2015.06.008
van Mierlo, P., Holler, Y., Focke, N. K., and Vulliemoz, S. (2019). Network perspectives on epilepsy using EEG/MEG source connectivity. Front. Neurol. 10:721. doi: 10.3389/fneur.2019.00721
Velmurugan, J., Nagarajan, S. S., Mariyappa, N., Mundlamuri, R. C., Raghavendra, K., Bharath, R. D., et al. (2019). Magnetoencephalography imaging of high frequency oscillations strengthens presurgical localization and outcome prediction. Brain 142, 3514–3529. doi: 10.1093/brain/awz284
Vivekananda, U., Cao, C., Liu, W., Zhang, J., Rugg-Gunn, F., Walker, M., et al. (2020). The use of simultaneous SEEG and MEG in localising seizure onset. medRxiv preprint. doi: 10.1101/2020.01.31.20019505
von Ellenrieder, N., Pellegrino, G., Hedrich, T., Gotman, J., Lina, J. M., Grova, C., et al. (2016). Detection and magnetic source imaging of fast oscillations (40–160 Hz) recorded with magnetoencephalography in focal epilepsy patients. Brain Topogr. 29, 218–231. doi: 10.1007/s10548-016-0471-9
von Stein, A., and Sarnthein, J. (2000). Different frequencies for different scales of cortical integration: from local gamma to long range alpha/theta synchronization. Int. J. Psychophysiol. 38, 301–313. doi: 10.1016/s0167-8760(00)00172-0
Wei, J., Chen, T., Li, C., Liu, G., Qiu, J., and Wei, D. (2018). Eyes-open and eyes-closed resting states with opposite brain activity in sensorimotor and occipital regions: multidimensional evidences from machine learning perspective. Front. Hum. Neurosci. 12:422. doi: 10.3389/fnhum.2018.00422
Weng, Y., Liu, X., Hu, H., Huang, H., Zheng, S., Chen, Q., et al. (2020). Open eyes and closed eyes elicit different temporal properties of brain functional networks. Neuroimage 222:117230. doi: 10.1016/j.neuroimage.2020.117230
Winter, W. R., Nunez, P. L., Ding, J., and Srinivasan, R. (2007). Comparison of the effect of volume conduction on EEG coherence with the effect of field spread on MEG coherence. Stat. Med. 26, 3946–3957. doi: 10.1002/sim.2978
Xia, G., Hu, Z., Zhou, F., Duan, W., Wang, M., Gong, H., et al. (2020). Functional connectivity density with frequency-dependent changes in patients with diffuse axonal injury: a resting-state functional magnetic resonance imaging study. Neuropsych. Dis. Treat. 16, 2733–2742. doi: 10.2147/ndt.S267023
Xiang, J., Luo, Q., Kotecha, R., Korman, A., Zhang, F., Luo, H., et al. (2014). Accumulated source imaging of brain activity with both low and high-frequency neuromagnetic signals. Front. Neuroinform. 8:57. doi: 10.3389/fninf.2014.00057
Yan, C., Liu, D., He, Y., Zou, Q., Zhu, C., Zuo, X., et al. (2009). Spontaneous brain activity in the default mode network is sensitive to different resting-state conditions with limited cognitive load. PLoS ONE 4:e5743. doi: 10.1371/journal.pone.0005743
Yin, C., Zhang, X., Chen, Z., Li, X., Wu, S., Lv, P., et al. (2019). Detection and localization of interictal ripples with magnetoencephalography in the presurgical evaluation of drug-resistant insular epilepsy. Brain Res. 1706, 147–156. doi: 10.1016/j.brainres.2018.11.006
Yin, C., Zhang, X., Xiang, J., Chen, Z., Li, X., Wu, S., et al. (2020). Altered effective connectivity network in patients with insular epilepsy: a high-frequency oscillations magnetoencephalography study. Clin. Neurophysiol. 131, 377–384. doi: 10.1016/j.clinph.2019.11.021
Zhang, Z., Liao, W., Chen, H., Mantini, D., Ding, J. R., Xu, Q., et al. (2011). Altered functional-structural coupling of large-scale brain networks in idiopathic generalized epilepsy. Brain 134, 2912–2928. doi: 10.1093/brain/awr223
Zhao, B., Hu, W., Zhang, C., Wang, X., Wang, Y., Liu, C., et al. (2020). Integrated automatic detection, classification and imaging of high frequency oscillations with stereoelectroencephalography. Front. Neurosci. 14:546. doi: 10.3389/fnins.2020.00546
Zijlmans, M., Jiruska, P., Zelmann, R., Leijten, F. S., Jefferys, J. G., and Gotman, J. (2012). High-frequency oscillations as a new biomarker in epilepsy. Ann. Neurol. 71, 169–178. doi: 10.1002/ana.22548
Zijlmans, M., Worrell, G. A., Dümpelmann, M., Stieglitz, T., Barborica, A., Heers, M., et al. (2017). How to record high-frequency oscillations in epilepsy: a practical guideline. Epilepsia 58, 1305–1315. doi: 10.1111/epi.13814
Keywords: magnetoencephalography, intracranial electroencephalogram, epilepsy, resting-state functional connectivity, surgical outcome
Citation: Xu N, Shan W, Qi J, Wu J and Wang Q (2021) Presurgical Evaluation of Epilepsy Using Resting-State MEG Functional Connectivity. Front. Hum. Neurosci. 15:649074. doi: 10.3389/fnhum.2021.649074
Received: 03 January 2021; Accepted: 07 June 2021;
Published: 02 July 2021.
Edited by:
Zhong Irene Wang, Cleveland Clinic, United StatesReviewed by:
Christos Papadelis, Cook Children's Medical Center, United StatesBalu Krishnan, Cleveland Clinic, United States
Copyright © 2021 Xu, Shan, Qi, Wu and Wang. This is an open-access article distributed under the terms of the Creative Commons Attribution License (CC BY). The use, distribution or reproduction in other forums is permitted, provided the original author(s) and the copyright owner(s) are credited and that the original publication in this journal is cited, in accordance with accepted academic practice. No use, distribution or reproduction is permitted which does not comply with these terms.
*Correspondence: Qun Wang, d2FuZ3EmI3gwMDA0MDtjY211LmVkdS5jbg==