- Department of Radiology, The Third Affiliated Hospital of Chengdu Medical College, Pidu District People's Hospital, Chengdu, China
Migraine is a chronic and idiopathic disorder leading to cognitive and affective problems. However, the neural basis of migraine without aura is still unclear. In this study, dynamic amplitude of low-frequency fluctuations (dALFF) analyses were performed in 21 patients with migraine without aura and 21 gender- and age-matched healthy controls to identify the voxel-level abnormal functional dynamics. Significantly decreased dALFF in the bilateral anterior insula, bilateral lateral orbitofrontal cortex, bilateral medial prefrontal cortex, bilateral anterior cingulate cortex, and left middle frontal cortex were found in patients with migraine without aura. The dALFF values in the anterior cingulate cortex were negatively correlated with pain intensity, i.e., visual analog scale. Finally, support vector machine was used to classify patients with migraine without aura from healthy controls and achieved an accuracy of 83.33%, sensitivity of 90.48%, and specificity of 76.19%. Our findings provide the evidence that migraine influences the brain functional activity dynamics and reveal the neural basis for migraine, which could facilitate understanding the neuropathology of migraine and future treatment.
Introduction
Migraine is an idiopathic and chronic disorder influencing the life quality of patients (Kruit et al., 2004). The frequent migraine attacks also affect patients' mental and physical health (Tietjen, 2004; Borsook et al., 2012). The previous studies have demonstrated that long-term chronic pain leads to functional damages in sensory, cognition, and affective processing (Montero-Homs, 2009; Linton, 2013; Denkinger et al., 2014). A lot of previous studies have revealed that migraine induced brain gray matter volume and white matter integrity changes using structural and diffusion magnetic resonance imaging (MRI) (DaSilva et al., 2007; Kim et al., 2008; Schmidt-Wilcke et al., 2008; Valfrè et al., 2008). With the use of resting-state functional MRI (fMRI), altered local regional homogeneity and whole-brain functional connectivity homogeneity were found in patients with migraine (Yu et al., 2012; Zhang et al., 2020). All these findings suggest that migraine could change the brain structure and functions.
The low-frequency oscillation of the brain is mainly characterized using resting-state fMRI with blood oxygen level-dependent (BOLD) signals (Biswal et al., 1995; Fox and Raichle, 2007). Resting-state fMRI has been widely applied to study the brain functional organization (Greicius et al., 2003; Fox et al., 2006; Wang et al., 2015, 2017c; Xu et al., 2019; Gao et al., 2020), to identify the functional abnormalities in patients with brain disorders (Greicius et al., 2007; Brier et al., 2012; Muller et al., 2013; Wu et al., 2016; Sun et al., 2018), and to reveal the neural basis of treatment response (Guo et al., 2012; Mulders et al., 2016; Wang et al., 2017b, 2018; Xu et al., 2020). To quantitatively measure low-frequency oscillation, the amplitude of low-frequency fluctuations (ALFF) is proposed (Zang et al., 2007). Using ALFF, Xue et al. (2013) found decreased ALFF values in the left rostral anterior cingulate cortex and bilateral prefrontal cortex as well as increased ALFF values in the right thalamus. Using ALFF to reveal the early abnormal functional activity is promising to explore early marker for migraine. Recently, using sliding-window approach, the dynamic ALFF (dALFF) method was developed by calculating the variance of ALFF over time (Fu et al., 2018). The dALFF has been applied to study the functional activity abnormalities in subjects under disease state and provides some new evidence (Fu et al., 2018; Ma et al., 2020; Pang et al., 2020). Thus, to reveal the dynamic changes of the low-frequency oscillation in migraine patients may provide supplementary information to understand its neural basis.
In this study, with 21 patients with migraine without aura and 21 gender- and age-matched healthy controls, a voxel-wise dALFF method was used to reveal the dynamic changes of low-frequency oscillation in migraine patients. Moreover, using the dALFF values in brain regions showing differences between patients and controls as features, support vector machine (SVM) was used to classify migraine patients from healthy controls to further validate the results.
Materials and Methods
Participants
In this study, we recruited 21 right-handed patients with migraine without aura (female/male = 16/5; age = 31.19 ± 6.38 years) and 21 gender- and age-matched right-handed healthy controls (female/male = 13/8; age = 30.19 ± 6.3 years) at the Third Affiliated Hospital of Chengdu Medical College, Pidu District People's Hospital. The diagnosis of migraine without aura was based on the International Headache Society criteria. The inclusion criteria for patients with migraine without aura were follows: (1) no migraine precipitated during or on the day after the scan; (2) did not suffer from a migraine attack at least 72 h before the experiment; (3) for migraine patients and healthy controls, no lifetime history of seizures, head trauma, serious medical or surgical illness, substance dependence or abuse, and contraindications for MRI. The participants were excluded if structural abnormalities were detected on MRI examination, and no subject with structural deficits was found. Written informed consent was provided and obtained from all the subjects. This study was approved by the local ethics committees of the Third Affiliated Hospital of Chengdu Medical College, Pidu District People's Hospital. The detailed information for the subjects can be found in our previous study (Zhang et al., 2020).
Resting-State Functional MRI Data Acquisition
MRI data were acquired on a 3-Tesla Siemens MRI scanner in the Department of Radiology, the Third Affiliated Hospital of Chengdu Medical College, Pidu District People's Hospital of Chengdu, China. The participants were instructed to close their eyes and not fall asleep, and earplugs and foam padding were used to reduce scanner noise and head motion. Resting-state fMRI data were acquired using a gradient-echo echo-planar imaging (GRE-EPI) sequence with the following parameters: repetition time (TR) = 2,000 ms, echo time (TE) = 30 ms, flip angle (FA) = 90°, matrix = 64 × 64, field of view (FOV) = 220 × 220 mm, slice thickness = 4 mm with inter-slice gap = 0.6 mm, 32 axial slices, and 250 time points. The information can be found in our previous study (Zhang et al., 2020).
Resting-State Functional MRI Data Preprocessing
The resting-state fMRI data were preprocessed using the toolkit of DPARSF version 2.3 (Chao-Gan and Yu-Feng, 2010) (www.restfmri.net/forum/DPARSF). To exclude unstable magnetization effect, the first 10 volumes were discarded. Then, all the remaining volumes were realigned to the first volume to correct head motion. Next, all the images were normalized to standard EPI template in Montreal Neurological Institute (MNI) space and resampled to 3-mm voxel resolution. Subsequently, 6-mm Gaussian kernel was used to smooth the fMRI data; and Friston 24-parameter of head motion, white matter, cerebrospinal fluid, and global mean signals were regressed out. Finally, the resting-state data were filtered with a frequency band of 0.01–0.08 Hz for dALFF analyses. To remove head-motion effects, the subjects were excluded if the head movement exceeds 3 mm or 3°. Additionally, “scrubbing” was used to delete the bad images before 2 time points and after 1 time point exceeding the preset criteria [frame displacement (FD) <0.5] (Power et al., 2012). We also calculated the mean FD values of healthy controls and migraine patients, and the two-sample t-test was used and did not find the significant difference (FD values of patients = 0.16 ± 0.29; FD values of controls = 0.15 ± 0.027; p = 0.49).
Dynamic Amplitude of Low-Frequency Fluctuations Calculation
The ALFF was proposed to characterize the resting-state functional activity of each voxel in brain (Zou et al., 2008). To calculate ALFF, the time series was first transformed to frequency domain, and the ALFF is computed at the power within the low-frequency range of 0.01–0.8 Hz. To calculate dALFF, a sliding window method was used. The length of sliding window is determined based on the criterion that minimum window length should be larger than 1/fmin, where fmin is the minimum frequency of time series (Leonardi and Van De Ville, 2015; Du et al., 2017; Li et al., 2019). Finally, a window length of 50 TR with step size of 5 TR was applied in this study. The ALFF map was computed in each window, and the variance of the ALFF maps across all the windows was computed to measure the dynamic. The dALFF maps were transformed to z-scores for statistical analyses. Two-sample t-test was performed to compare the dALFF maps between healthy controls and patients with migraine without aura. The significant level was set at p < 0.05 using a Gaussian random field (GRF) correction method. To further validate the results obtained with the window length of 50 TR, the window length of 30 and 70 TR were further applied.
Correlation Analyses
To explore the relationship between dALFF and clinical measures, correlation analyses were performed between the dALFF values of the areas showing differences in patients and visual analog scale (VAS), and disease duration. The significance was set at p < 0.05 with Bonferroni correction.
Support Vector Machine Classification
A linear SVM classifier was performed using LIBSVM software (Chang and Lin, 2011). For classification, the mean dALFF values of the areas showing differences between patients and controls were used as the features. To estimate the performance of our classifier, a leave-one-out cross-validation (LOOCV) test was used to assess the generalization ability because of our limited number of samples in the present study. The classification result was assessed using the classification accuracy, sensitivity, and specificity.
Results
Clinical Characteristics
There were no significant differences in age (p = 0.61) and sex (p = 0.51) between healthy controls and patients with migraine without aura (Table 1).
Changed Dynamic Amplitude of Low-Frequency Fluctuations
By the analysis of dALFF in window length of 50 TR, significantly decreased dALFF in the bilateral anterior insula, bilateral lateral orbitofrontal cortex, bilateral medial prefrontal cortex, bilateral anterior cingulate cortex, and left middle frontal cortex in patients with migraine without aura compared with healthy controls (Figure 1 and Table 2) was found. We also analyzed dALFF in window length of 30 and 70 TR, and similar results were found (Figure 2).
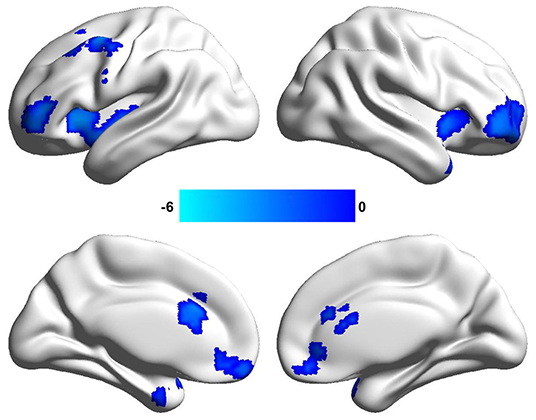
Figure 1. Changed dynamic amplitude of low-frequency fluctuations (ALFF) in window length of 50 repetition time (TR) in patients with migraine without aura. Significantly decreased dynamic ALFF in the bilateral anterior insula, bilateral anterior cingulate cortex, bilateral lateral orbitofrontal cortex, and left middle frontal cortex were found in patients with migraine without aura.
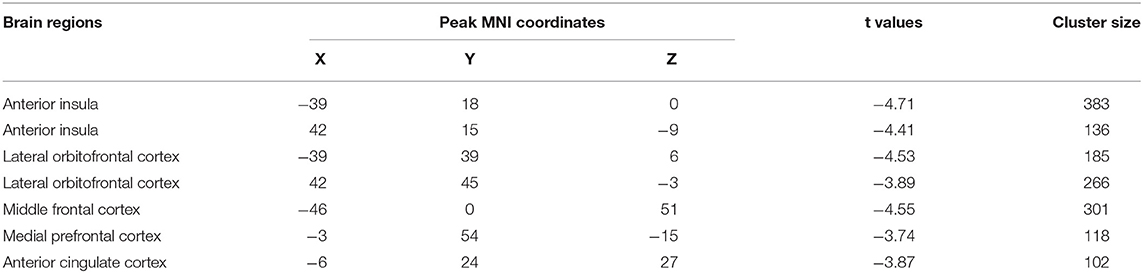
Table 2. Regions with decreased dynamical amplitude of low-frequency fluctuations in patients with migraine without aura vs. healthy controls.
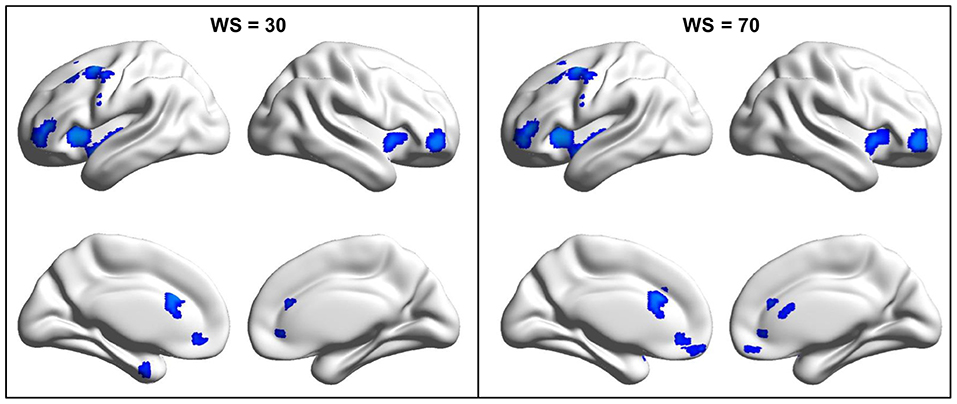
Figure 2. Changed dynamic amplitude of low-frequency fluctuations (ALFF) in migraine patients was validated in window length of 30 and 70 repetition times (TRs). To validate the findings obtained with window length of 50 TR, the same procedures were performed in window length of 30 and 70 TRs and found similar results.
Correlation Analyses
Correlation analyses identified significantly negative correlations between dALFF values in the anterior cingulate cortex and VAS scores (r = −0.5, p = 0.022) in patients with migraine without aura after multiple comparisons correction (Figure 3).
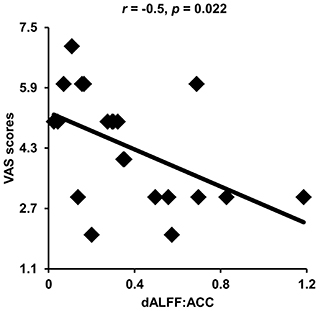
Figure 3. Correlation analyses between dynamic amplitude of low-frequency fluctuations (ALFF) and clinical measures. Significant correlation was found between the dynamic ALFF in the anterior cingulate cortex and visual analog scale (VAS) scores in migraine patients.
Classification Results
With the use of the changed dALFF values as features, experimental results showed a correct classification rate of 83.33%, sensitivity of 90.4%, and specificity of 76.19% using a leave-one-out cross-validation method (Figure 4).
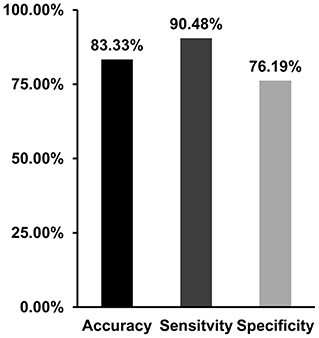
Figure 4. Support vector machine (SVM) was applied to classify migraine patients and healthy controls with the dynamic amplitude of low-frequency fluctuations (ALFF) values in brain areas showing differences between patients and controls as features. SVM achieves a classification accuracy of 83.33%, sensitivity of 90.48%, and specificity of 76.19%.
Discussion
In our study, dALFF method was applied and identified decreased dALFF in the bilateral anterior insula, bilateral orbitofrontal cortex, bilateral anterior cingulate cortex, bilateral medial prefrontal cortex, and left middle frontal cortex; and the changed dALFF in the anterior cingulate cortex was negatively correlated with VAS scores. Moreover, the changed dALFF can serve as an effective neuromarker to differentiate patients with migraine without aura from controls. Our findings revealed dynamic changes of brain functional activities in migraine patients and provide new evidence of neurophysiological abnormalities in migraine.
We found decreased dALFF in the anterior cingulate cortex and anterior insula in migraine patients without aura. The anterior insula and anterior cingulate cortex are two important nodes of salience network, which is mainly involved in coordinating the dynamic interaction between the external orient stimulus and internal self-perception (Menon and Uddin, 2010; Menon, 2011; Wang et al., 2017a, 2019b). The functional abnormality of anterior cingulate cortex and anterior insula found in our study is supported by previous functional and structural studies (Peyron et al., 2000; Kim et al., 2008; Valfrè et al., 2008). Importantly, we found that decreased dALFF in the anterior cingulate cortex is associated with clinical pain intensity. Thus, the abnormal dynamic functional activities in the anterior cingulate cortex may be a neuromarker to predict the pain intensity.
The decreased dALFF in the medial prefrontal cortex, middle frontal cortex, and lateral orbitofrontal cortex was also observed. The medial prefrontal cortex is the core brain area of the default mode network that participates in social cognition, emotional, and self-referential processing (Gusnard et al., 2001; Amodio and Frith, 2006; Etkin et al., 2011; Wang et al., 2019a,c). The abnormal functional activities in medial prefrontal cortex have been reported in previous studies by analyses of static ALFF, local regional homogeneity, and whole-brain functional connectivity homogeneity (Yu et al., 2012; Xue et al., 2013). In addition, the middle frontal cortex and lateral orbitofrontal cortex play an important role in attention and executive control of pain-related stimuli to modulate the descending pain system (Casey, 1999; Lorenz et al., 2003; Wager et al., 2004). Thus, decreased dALFF values in the medial prefrontal cortex and middle prefrontal cortex may be related to impaired cognitive and emotion processing of pain. Moreover, we found that the changed dALFF in brain areas including the bilateral anterior insula, bilateral anterior cingulate cortex, bilateral medial prefrontal cortex, and left middle frontal cortex can well distinguish the migraine patients from controls. This finding indicates that the abnormal dynamic activities in default mode network, salience network, and executive network may be the neuropathology of migraine.
There are some limitations of our study. First, how to determine the length of sliding window is a problem for dynamic analysis. We used different window lengths to validate the findings. Second, the samples of our study are small, and the findings need to be further validated in the future studies with a larger sample.
Conclusion
This study revealed the abnormal dynamic low-frequency oscillation in default mode network, salience network, and executive control network in migraine patients. The decreased dALFF in these areas may be associated with disrupted emotion and cognitive functions. Our findings provide new evidence that migraine could influence the brain functions leading to functional impairments of emotion and cognitions in patients.
Data Availability Statement
The raw data supporting the conclusions of this article will be made available by the authors, without undue reservation.
Ethics Statement
The studies involving human participants were reviewed and approved by the Third Affiliated Hospital of Chengdu Medical College, Pidu District People's Hospital. The patients/participants provided their written informed consent to participate in this study. Written informed consent was obtained from the individual(s) for the publication of any potentially identifiable images or data included in this article.
Author Contributions
All authors have made a substantial contribution to this work.
Conflict of Interest
The authors declare that the research was conducted in the absence of any commercial or financial relationships that could be construed as a potential conflict of interest.
References
Amodio, D. M., and Frith, C. D. (2006). Meeting of minds: the medial frontal cortex and social cognition. Nat. Rev. Neurosci. 7, 268–277. doi: 10.1038/nrn1884
Biswal, B., Yetkin, F. Z., Haughton, V. M., and Hyde, J. S. (1995). Functional connectivity in the motor cortex of resting human brain using echo-planar MRI. Magnet. Resonance Med. 34, 537–541. doi: 10.1002/mrm.1910340409
Borsook, D., Maleki, N., Becerra, L., and McEwen, B. (2012). Understanding migraine through the lens of maladaptive stress responses: a model disease of allostatic load. Neuron 73, 219–234. doi: 10.1016/j.neuron.2012.01.001
Brier, M. R., Thomas, J. B., Snyder, A. Z., Benzinger, T. L., Zhang, D., Raichle, M. E., et al. (2012). Loss of intranetwork and internetwork resting state functional connections with Alzheimer's disease progression. J. Neurosci. 3, 8890–8899. doi: 10.1523/JNEUROSCI.5698-11.2012
Casey, K. L. (1999). Forebrain mechanisms of nociception and pain: analysis through imaging. Proc. Natl. Acad. Sci. U.S.A. 96, 7668–7674. doi: 10.1073/pnas.96.14.7668
Chang, C.-C., and Lin, C.-J. (2011). LIBSVM: a library for support vector machines. ACM Trans. Intell. Syst. Technol. 2, 1–27. doi: 10.1145/1961189.1961199
Chao-Gan, Y., and Yu-Feng, Z. (2010). DPARSF: a MATLAB toolbox for “Pipeline” data analysis of resting-state fMRI. Front. Systems Neurosci. 4:13. doi: 10.3389/fnsys.2010.00013
DaSilva, A. F., Granziera, C., Tuch, D. S., Snyder, J., Vincent, M., and Hadjikhani, N. (2007). Interictal alterations of the trigeminal somatosensory pathway and periaqueductal gray matter in migraine. Neuroreport 18, 301–305. doi: 10.1097/WNR.0b013e32801776bb
Denkinger, M. D., Lukas, A., Nikolaus, T., Peter, R., and Franke, S. (2014). Multisite pain, pain frequency and pain severity are associated with depression in older adults: results from the ActiFE Ulm study. Age Ageing 43, 510–514. doi: 10.1093/ageing/afu013
Du, Y., Pearlson, G. D., Lin, D., Sui, J., Chen, J., Salman, M., et al. (2017). Identifying dynamic functional connectivity biomarkers using GIG-ICA: application to schizophrenia, schizoaffective disorder, and psychotic bipolar disorder. Human Brain Mapp. 38, 2683–2708. doi: 10.1002/hbm.23553
Etkin, A., Egner, T., and Kalisch, R. (2011). Emotional processing in anterior cingulate and medial prefrontal cortex. Trends Cognitive Sci. 15, 85–93. doi: 10.1016/j.tics.2010.11.004
Fox, M. D., Corbetta, M., Snyder, A. Z., Vincent, J. L., and Raichle, M. E. (2006). Spontaneous neuronal activity distinguishes human dorsal and ventral attention systems. Proc. Natl. Acad. Sci. U.S.A. 103, 10046–10051. doi: 10.1073/pnas.0604187103
Fox, M. D., and Raichle, M. E. (2007). Spontaneous fluctuations in brain activity observed with functional magnetic resonance imaging. Nat Rev. Neurosci. 8, 700–711. doi: 10.1038/nrn2201
Fu, Z., Tu, Y., Di, X., Du, Y., Pearlson, G. D., Turner, J. A., et al. (2018). Characterizing dynamic amplitude of low-frequency fluctuation and its relationship with dynamic functional connectivity: an application to schizophrenia. NeuroImage 180, 619–631. doi: 10.1016/j.neuroimage.2017.09.035
Gao, Z., Guo, X., Liu, C., Mo, Y., and Wang, J. (2020). Right inferior frontal gyrus: An integrative hub in tonal bilinguals. Human Brain Mapp. 41, 2152–2159. doi: 10.1002/hbm.24936
Greicius, M. D., Flores, B. H., Menon, V., Glover, G. H., Solvason, H. B., Kenna, H., et al. (2007). Resting-state functional connectivity in major depression: abnormally increased contributions from subgenual cingulate cortex and thalamus. Biol. Psychiatr. 62, 429–437. doi: 10.1016/j.biopsych.2006.09.020
Greicius, M. D., Krasnow, B., Reiss, A. L., and Menon, V. (2003). Functional connectivity in the resting brain: a network analysis of the default mode hypothesis. Proc. Natl. Acad. Sci. U.S.A. 100, 253–258. doi: 10.1073/pnas.0135058100
Guo, W. B., Liu, F., Chen, J. D., Gao, K., Xue, Z. M., Xu, X. J., et al. (2012). Abnormal neural activity of brain regions in treatment-resistant and treatment-sensitive major depressive disorder: a resting-state fMRI study. J. Psychiatric Res. 46, 1366–1373. doi: 10.1016/j.jpsychires.2012.07.003
Gusnard, D. A., Akbudak, E., Shulman, G. L., and Raichle, M. E. (2001). Medial prefrontal cortex and self-referential mental activity: relation to a default mode of brain function. Proc. Natl. Acad. Sci. U.S.A. 98. 4259–4264. doi: 10.1073/pnas.071043098
Kim, J. H., Suh, S. I., Seol, H. Y., Oh, K., Seo, W. K., Yu, S. W., et al. (2008). Regional grey matter changes in patients with migraine: a voxel-based morphometry study. Cephalalgia 28, 598–604. doi: 10.1111/j.1468-2982.2008.01550.x
Kruit, M. C., van Buchem, M. A., Hofman, P. A. M., Bakkers, J. T. N., Terwindt, G. M., Ferrari, M. D., et al. (2004). Migraine as a risk factor for subclinical brain lesions. JAMA 291, 427–434. doi: 10.1001/jama.291.4.427
Leonardi, N., and Van De Ville, D. (2015). On spurious and real fluctuations of dynamic functional connectivity during rest. NeuroImage 104, 430–436. doi: 10.1016/j.neuroimage.2014.09.007
Li, C., Xia, L., Ma, J., Li, S., Liang, S., Ma, X., et al. (2019). Dynamic functional abnormalities in generalized anxiety disorders and their increased network segregation of a hyperarousal brain state modulated by insomnia. J. Affect. Disord. 246, 338–345. doi: 10.1016/j.jad.2018.12.079
Linton, S. J. (2013). A transdiagnostic approach to pain and emotion. J. Appl. Biobehav. Res. 18, 82–103. doi: 10.1111/jabr.12007
Lorenz, J., Minoshima, S., and Casey, K. L. (2003). Keeping pain out of mind: the role of the dorsolateral prefrontal cortex in pain modulation. Brain 126, 1079–1091. doi: 10.1093/brain/awg102
Ma, M., Zhang, H., Liu, R., Liu, H., Yang, X., Yin, X., et al. (2020). Static and dynamic changes of amplitude of low-frequency fluctuations in cervical discogenic pain. Front. Neurosci. 14:733. doi: 10.3389/fnins.2020.00733
Menon, V. (2011). Large-scale brain networks and psychopathology: a unifying triple network model. Trends cognit. Sci. 15, 483–506. doi: 10.1016/j.tics.2011.08.003
Menon, V., and Uddin, L. Q. (2010). Saliency, switching, attention and control: a network model of insula function. Brain Struct. Funct.214, 655–667. doi: 10.1007/s00429-010-0262-0
Montero-Homs, J. (2009). Nocioceptive pain, neuropathic pain and pain memory. Neurologia 24, 419–422.
Mulders, P. C., van Eijndhoven, P. F., Pluijmen, J., Schene, A. H., Tendolkar, I., and Beckmann, C. F. (2016). Default mode network coherence in treatment-resistant major depressive disorder during electroconvulsive therapy. J. Affect. Disord. 205, 130–137. doi: 10.1016/j.jad.2016.06.059
Muller, V. I., Cieslik, E. C., Laird, A. R., Fox, P. T., and Eickhoff, S. B. (2013). Dysregulated left inferior parietal activity in schizophrenia and depression: functional connectivity and characterization. Front. Human Neurosci. 7:268. doi: 10.3389/fnhum.2013.00268
Pang, Y., Zhang, H., Cui, Q., Yang, Q., Lu, F., Chen, H., et al. (2020). Combined static and dynamic functional connectivity signatures differentiating bipolar depression from major depressive disorder. Australian N. Zealand J. Psychiatr. 54, 832–842. doi: 10.1177/0004867420924089
Peyron, R., Laurent, B., and Garcia-Larrea, L. (2000). Functional imaging of brain responses to pain. A review and meta-analysis neurophysiologie clinique. Clin. Neurophysiol. 30, 263–288. doi: 10.1016/S0987-7053(00)00227-6
Power, J. D., Barnes, K. A., Snyder, A. Z., Schlaggar, B. L., and Petersen, S. E. (2012). Spurious but systematic correlations in functional connectivity MRI networks arise from subject motion. NeuroImage 59, 2142–2154. doi: 10.1016/j.neuroimage.2011.10.018
Schmidt-Wilcke, T., Gänssbauer, S., Neuner, T., Bogdahn, U., and May, A. (2008). Subtle grey matter changes between migraine patients and healthy controls. Cephalalgia 28, 1–4. doi: 10.1111/j.1468-2982.2007.01428.x
Sun, H., Luo, L., Yuan, X., Zhang, L., He, Y., Yao, S., et al. (2018). Regional homogeneity and functional connectivity patterns in major depressive disorder, cognitive vulnerability to depression and healthy subjects. J. Affective Disord. 235, 229–235. doi: 10.1016/j.jad.2018.04.061
Tietjen, G. E. (2004). Stroke and migraine linked by silent lesions. Lancet 3:267. doi: 10.1016/S1474-4422(04)00729-X
Valfrè, W., Rainero, I., Bergui, M., and Pinessi, L. (2008). Voxel-based morphometry reveals gray matter abnormalities in migraine. Headache 48, 109–117. doi: 10.1111/j.1526-4610.2007.00723.x
Wager, T. D., Rilling, J. K., Smith, E. E., Sokolik, A., Casey, K. L., Davidson, R. J., et al. (2004). Placebo-induced changes in FMRI in the anticipation and experience of pain. Science 303, 1162–1167. doi: 10.1126/science.1093065
Wang, C., Wu, H., Chen, F., Xu, J., Li, H., Li, H., et al. (2017a). Disrupted functional connectivity patterns of the insula subregions in drug-free major depressive disorder. J. Affect. Disord. 234, 297–304. doi: 10.1016/j.jad.2017.12.033
Wang, J., Becker, B., Wang, L., Li, H., Zhao, X., and Jiang, T. (2019a). Corresponding anatomical and coactivation architecture of the human precuneus showing similar connectivity patterns with macaques. NeuroImage 200, 562–574. doi: 10.1016/j.neuroimage.2019.07.001
Wang, J., Wei, Q., Bai, T., Zhou, X., Sun, H., Becker, B., et al. (2017b). Electroconvulsive therapy selectively enhanced feedforward connectivity from fusiform face area to amygdala in major depressive disorder. Soc. Cognit. Affective Neurosci. 12, 1983–1992. doi: 10.1093/scan/nsx100
Wang, J., Wei, Q., Wang, L., Zhang, H., Bai, T., Cheng, L., et al. (2018). Functional reorganization of intra- and internetwork connectivity in major depressive disorder after electroconvulsive therapy. Human Brain Mapp. 39, 1403–1411. doi: 10.1002/hbm.23928
Wang, J., Xie, S., Guo, X., Becker, B., Fox, P. T., Eickhoff, S. B., et al. (2017c). Correspondent functional topography of the human left inferior parietal lobule at rest and under task revealed using resting-state fmri and coactivation based parcellation. Human Brain Mapp. 38, 1659–1675. doi: 10.1002/hbm.23488
Wang, J., Yang, Y., Fan, L., Xu, J., Li, C., Liu, Y., et al. (2015). Convergent functional architecture of the superior parietal lobule unraveled with multimodal neuroimaging approaches. Human Brain Mapp. 36, 238–257. doi: 10.1002/hbm.22626
Wang, L., Wei, Q., Wang, C., Xu, J., Wang, K., Tian, Y., et al. (2019b). Altered functional connectivity patterns of insular subregions in major depressive disorder after electroconvulsive therapy. Brain Imaging Behav. 14, 753–761. doi: 10.1007/s11682-018-0013-z
Wang, L., Yu, L., Wu, F., Wu, H., and Wang, J. (2019c). Altered whole brain functional connectivity pattern homogeneity in medication-free major depressive disorder. J. Affect. Disord. 253, 18–25. doi: 10.1016/j.jad.2019.04.040
Wu, Y., Zhang, Y., Liu, Y., Liu, J., Duan, Y., Wei, X., et al. (2016). Distinct changes in functional connectivity in posteromedial cortex subregions during the progress of alzheimer's disease. Front Neuroanat. 10:41. doi: 10.3389/fnana.2016.00041
Xu, J., Lyu, H., Li, T., Xu, Z., Fu, X., Jia, F., et al. (2019). Delineating functional segregations of the human middle temporal gyrus with resting-state functional connectivity and coactivation patterns. Human Brain Mapp. 40, 5159–5171. doi: 10.1002/hbm.24763
Xu, J., Wei, Q., Bai, T., Wang, L., Li, X., He, Z., et al. (2020). Electroconvulsive therapy modulates functional interactions between submodules of the emotion regulation network in major depressive disorder. Translational Psychiatr. 10:271. doi: 10.1038/s41398-020-00961-9
Xue, T., Yuan, K., Cheng, P., Zhao, L., Zhao, L., Yu, D., et al. (2013). Alterations of regional spontaneous neuronal activity and corresponding brain circuit changes during resting state in migraine without aura. NMR Biomed. 26, 1051–1058. doi: 10.1002/nbm.2917
Yu, D., Yuan, K., Zhao, L., Zhao, L., Dong, M., Liu, P., et al. (2012). Regional homogeneity abnormalities in patients with interictal migraine without aura: a resting-state study. NMR Biomed. 25, 806–812. doi: 10.1002/nbm.1796
Zang, Y. F., He, Y., Zhu, C. Z., Cao, Q. J., Sui, M. Q., Liang, M., et al. (2007). Altered baseline brain activity in children with ADHD revealed by resting-state functional MRI. Brain Dev. 29, 83–91. doi: 10.1016/j.braindev.2006.07.002
Zhang, Y., Chen, H., Zeng, M., He, J., Qi, G., Zhang, S., et al. (2020). Abnormal whole brain functional connectivity pattern homogeneity and couplings in migraine without Aura. Front. Human Neurosci. 14:619839. doi: 10.3389/fnhum.2020.619839
Keywords: migraine without aura, dynamic amplitude of low-frequency fluctuations, resting-state, functional MRI, classification 3
Citation: Chen H, Qi G, Zhang Y, Huang Y, Zhang S, Yang D, He J, Mu L, Zhou L and Zeng M (2021) Altered Dynamic Amplitude of Low-Frequency Fluctuations in Patients With Migraine Without Aura. Front. Hum. Neurosci. 15:636472. doi: 10.3389/fnhum.2021.636472
Received: 01 December 2020; Accepted: 07 January 2021;
Published: 10 February 2021.
Edited by:
Bochao Cheng, Sichuan University, ChinaReviewed by:
Lijie Wang, University of Electronic Science and Technology of China, ChinaJingjing Gao, University of Electronic Science and Technology of China, China
Copyright © 2021 Chen, Qi, Zhang, Huang, Zhang, Yang, He, Mu, Zhou and Zeng. This is an open-access article distributed under the terms of the Creative Commons Attribution License (CC BY). The use, distribution or reproduction in other forums is permitted, provided the original author(s) and the copyright owner(s) are credited and that the original publication in this journal is cited, in accordance with accepted academic practice. No use, distribution or reproduction is permitted which does not comply with these terms.
*Correspondence: Min Zeng, minzengnihao@163.com