- 1Department of Radiology & Biomedical Imaging, University of California, San Francisco, CA, United States
- 2Department of Psychology, University of Hagen, Hagen, Germany
Experience-dependent neural plasticity is high in the developing brain, presenting a unique window of opportunity for training. To optimize existing training programs and develop new interventions, it is important to understand what processes take place in the developing brain during training. Here, we systematically review MRI-based evidence of training-induced neural plasticity in children and adolescents. A total of 71 articles were included in the review. Significant changes in brain activation, structure, microstructure, and structural and functional connectivity were reported with different types of trainings in the majority (87%) of the studies. Significant correlation of performance improvement with neural changes was reported in 51% of the studies. Yet, only 48% of the studies had a control condition. Overall, the review supports the hypothesized neural changes with training while at the same time charting empirical and methodological desiderata for future research.
Introduction
Training one's brain to improve one's abilities and well-being is an empowering idea. Doing so at a young age when brain plasticity is high may be especially pivotal (Kleim and Jones, 2008). In order to optimize existing training programs and develop new interventions for normally developing children and children with developmental disabilities, it is important to understand what processes take place in the brain during training. Moreover, training effects need to be put into the context of maturational processes. Neuroimaging, in particular magnetic resonance imaging (MRI), has enabled us to monitor training-induced effects in the brain non-invasively (Zatorre et al., 2012). Both structural and functional MRI methods can provide valuable insights into the underlying mechanisms of experience-dependent plasticity in adults as well as children, due to their non-invasive nature and sensitivity to brain tissue contrasts.
While there exist non-systematic reviews of neural changes with training in adults (Zatorre et al., 2012) and children (Jolles and Crone, 2012), no recent systematic reviews of training-induced neural plasticity in youth are available. This is an important gap, especially given the recent publication of new research studies on this topic, such as the neuroimaging study of training-induced plasticity in children with reading disability (Romeo et al., 2018).
The overall research aim of this systematic review was to investigate the extent of changes in MRI-derived parameters in youth with training and the quality of evidence for such change.
Background and Hypotheses
The following sections will provide the theoretical and empirical background for training-related neuroplasticity, describe MRI methodology that can be used to map neural changes, and address developmental/maturational effects on training-related plasticity. In the last section, the research question and specific hypotheses will be presented.
Neuroplasticity
Neuroplasticity has been defined as experience-driven change in neural structure and function (Rapoport and Gogtay, 2008; p. 181). The most striking examples of neuroplasticity are found in cases of trauma or invasive surgery, such as hemispherectomy—where one brain hemisphere is removed, often as a measure to stop seizures (Ismail et al., 2017). The degree to which brain functions linked to the removed hemisphere recover depends on multiple factors: e.g., the disorder that led to the surgery, the patient's age, and the capacity for plasticity of the affected brain regions (Ismail et al., 2017). The recovery can sometimes be remarkable, as in the case of a child who could speak again after the removal of the left hemisphere (Fenton et al., 2009).
Such dramatic examples aside, brain plasticity underlies everyday learning (Johnston, 2009). Summarizing neuroscience-based understanding of experience-dependent neural plasticity, Kleim and Jones (2008) highlight that while lack of use of brain functions can lead to degradation, training of a specific function can lead to enhancement of the specific function. Yet, training should be adequate in intensity and level of repetition, and over the course of time can lead to different variants of plasticity. While abundant animal work has contributed to the understanding of neuroplasticity, recently, it became possible to conduct human studies of training-induced plasticity using MRI methodology described in the following section.
MRI Methodology
Several types of MRI allow for characterization of structure and function of the brain and can provide markers of neuroplasticity with training (Figure 1).
1. Structural MRI. Structural MRI (typically based on T1-weighted images) allows for delineating structures in the brain and assessing volume or thickness (Whitwell, 2009). In particular, with the voxel-based morphometry (VBM) approach one can automatically, semi-quantitatively analyze gray matter (GM) and white matter (WM) structure change over the course of training. The measures derived from the VBM analysis are often misleadingly described as GM “concentration” or “density.” However, such measures do not directly represent underlying neuronal densities. Even the GM “volume” derived using VBM depends on voxel intensities on T1-weighted images (where the boundary between WM and GM is drawn) and thus can be affected by any tissue properties that influence relaxation times (e.g., myelination).
2. Diffusion MRI. Diffusion MRI techniques have enabled assessment of WM microstructure and WM tracts (Mukherjee et al., 2008). For example, fractional anisotropy (FA) calculated based on the diffusion tensor imaging (DTI) quantifies the orientational dependence of water diffusion and reflects axonal diameter and density, myelination, etc. The principal eigenvector of the tensor in each voxel can provide information about the orientation of the WM fiber bundle passing through that voxel. By following these directional estimates, one can perform diffusion tractography and study structural connectivity in the brain. Diffusion MRI connectome analysis that utilizes graph theory has become a promising technique for investigating the human brain as a network of structural connections (Hagmann et al., 2010). Resolving crossing and kissing fibers and disentangling different contributions to unspecific metrics such as FA are the biggest challenges of diffusion MRI.
3. Functional MRI (fMRI). Task fMRI assesses task-related variations in the blood oxygen-level-dependent (BOLD) signal in order to derive the intensity and spatial distribution of brain function (Logothetis et al., 2001). Neuronal activity leads to local increases in oxygen consumption and to the associated drop in oxygenated hemoglobin and increase in deoxy-hemoglobin. These changes in hemoglobin get rapidly overcompensated by increased blood flow to the active area of the brain. Because the two types of hemoglobin have different magnetic properties, MRI can measure the occurring changes. It is important to emphasize that upregulation of BOLD signal is not the same as upregulation of neuronal (electrical) firing. Rather, it is an indirect, vascular measure. Spontaneous brain activity measured using BOLD fMRI in the absence of a specific task can also be used to map the intrinsic, resting-state functional networks of the human brain (Kelly and Castellanos, 2014). While functional connectivity (FC) can be assessed during a task, a special place in research is dedicated to resting-state FC, measured when the study participant is not asked to perform any explicit task. These networks of synchronized spontaneous fluctuations consume a lot of energy and, remarkably, appear to contain the full repertoire of functional dynamics normally captured during various types of tasks (Smith et al., 2009; Laird et al., 2011). Although there is a robust relationship between functional and structural connectivities, they are far from equivalent (Honey et al., 2009). Finally, effective connectivity analysis using dynamic causal modeling (DCM) can help investigate neuronal dynamics of the brain network in that it assesses directionality of functional connections between brain regions.
Apart from the MRI modalities outlined above, many other MRI-based methods can provide additional information on brain function and structure. For example, magnetic resonance spectroscopic imaging (MRSI) quantifies metabolite concentrations not only in a single region of interest (single voxel), but spatially mapped over larger areas (Scheau et al., 2012). Spectroscopic imaging provides indices of neuronal viability [N-acetyl-aspartate (NAA)], brain energy metabolism (creatine), cell membrane integrity (choline), and glial cell population (myo-Inositol) among others (Scheau et al., 2012).
Any imaging technique used to compare local metrics over time and/or across individuals is susceptible to errors and biases introduced by the imaged volume selection (especially in MRSI), co-registration among images, and smoothing procedures, and these need to be taken into account.
Training-Induced Plasticity Findings in Adults
The advent of advanced MRI techniques has enabled in vivo human studies of changes occurring in the brain when a person undergoes different types of training. A pioneering study showed that taxi drivers in London had larger posterior hippocampi compared with controls and that this difference was related to the length of the driving experience (Maguire et al., 2000). The most important limitation of this type of cross-sectional analysis is that one cannot differentiate the effects of training on the brain from pre-existing environmental and/or genetic differences. However, longitudinal studies with imaging being performed before and after the training intervention have also shown remarkable neuroplasticity effects in adults. In 2004, Draganski et al. (2004) used VBM to visualize brain changes in volunteers who have learned to perform a three-ball juggling task. They showed a transient increase in GM regions associated with motion processing that was linked to the juggling performance (Draganski et al., 2004). Numerous MRI studies of training-induced neuroplasticity in adult subjects have followed (including an experimental equivalent of the taxi driver study (Lövdén et al., 2012) and were reviewed extensively (Draganski and May, 2008; Zatorre et al., 2012). Different types of trainings were studied, ranging from juggling, to learning math, to meditating. Even a mere “action observation training” in healthy adults promoted competence and modified GM structure compared with controls who watched videos of landscapes (Rocca et al., 2017). In addition to structural and microstructural changes, metabolic and fMRI-derived changes were observed with training (e.g., juggling, Sampaio-Baptista et al., 2015). This in vivo evidence demonstrated functional and structural reorganization of the human brain. Yet, questions about the underlying biological mechanisms of the observed changes remained largely unanswered.
Training-Induced Biological Changes Measured With MRI
While numerous biological changes can affect MRI measures (Zatorre et al., 2012), only some of them are sufficiently backed up as candidate mechanisms by animal training studies that used “rigorous experimental design and a combination of high spatial resolution MRI and immunohistochemistry” (Thomas and Baker, 2013; p. 9). Two animal studies providing this type of evidence are summarized below, and an overview of biological changes can be found in Table 1.
In one study, Lerch et al. (2011) tested if different types of training (spatial in comparison with non-spatial) can cause structural brain changes in mice. Animals trained on the spatial version of the water maze had hippocampal volume increases, whereas those trained on a non-spatial cued version of the maze displayed striatal increases. Importantly, these MRI-derived volume changes correlated with axonal growth-associated protein-43 (GAP-43) staining and not with markers of neuron or astrocyte number or size. This indicates that the measured morphological changes are due to remodeling of neuronal processes and not due to neurogenesis.
In another study, Blumenfeld-Katzir et al. (2011) examined DTI correlates of spatial learning in a group of rats. The authors found training-related changes of DTI indices in the dentate gyrus, cingulate cortex, piriform cortex, sensory cortex, and corpus callosum. The histological analyses showed that increases in synapses and astrocytes may have contributed to the changes in the diffusion indices in the dentate gyrus. At the same time, an increase in oligodendrocytes forming the myelin sheaths was identified as the mechanism linked to the increase in FA in the corpus callosum.
In summary, the findings from these two animal studies suggest that the observed MRI changes in human training studies may be due to changes in axonal growth and myelination, as well as in change in synapses and astrocytes. Although previous publications referred to continuing neurogenesis as a candidate mechanism underlying visible MRI changes with training (Zatorre et al., 2012), this is highly unlikely, given the rapid decline in the number of new neurons emerging after birth in the human brain (Sorrells et al., 2018).
Another animal study sheds light on one more important source of training-induced changes in MRI metrics, both structural and functional. Morland et al. (2017) demonstrated an increase in density of capillaries in the sensorimotor cortex, and in the dentate gyrus of hippocampus of wild-type mice after 7 weeks of exercise, compared with sedentary controls.
All of the biological processes described above—changes in synapses, axons and dendrites, astrocytes, myelination, and density of capillaries—can affect metrics derived from different types of MRI, including fMRI (Table 1). Changes in fMRI signal with training, however, can happen faster, before any MRI-detectable structural or microstructural changes take place. An organizing framework for interpreting the plasticity of task-evoked functional activity has been proposed (Kelly and Garavan, 2005). According to this framework, the way fMRI signal will change with training will depend on a number of factors:
(1) The changes in cognitive processes involved in task performance. For example, shift in strategy used by the subject to accomplish a task can lead to a reorganization of task-evoked activity. Similarly, a redistribution of the fMRI signal is expected when practicing the task increases reliance on task-specific brain regions and decreases reliance on regions responsible for control and attentional processes (such as the prefrontal cortex and anterior cingulate cortex).
(2) Task domain. Practice has divergent effects on functional activations in different brain regions, depending on their functional domain: e.g., motor and sensory tasks vs. higher-level cognitive tasks.
(3) The timepoint at which imaging is performed. Ideally, one would image the entire trajectory of practice-related brain changes, in order to make strong conclusions with respect to the plasticity mechanisms.
Kelly and Castellanos (2014) adapted this framework to the interpretation of the effects of practice on intrinsic FC. To use training of a sensorimotor task as an example, the task would cause a redistribution of intrinsic FC. Soon after the subject starts the practice, intrinsic FC between task-domain regions and higher-level cognitive and associative cortices will increase. As the subject continues the practice and his or her performance plateaus, connectivity with the higher-level cognitive and associative cortices goes back to baseline, whereas connectivity within the network of task-domain regions increases.
As indicated in the previous paragraphs, one should keep in mind that the specific biological changes underlying the changes in MRI measures will depend on the type of training, as well as on its duration. Learning a new skill or strategy is a multistage process, with the fast learning stage and the subsequent slow learning engaging different brain regions and different cellular mechanisms (Dayan and Cohen, 2011).
Finally, training-induced physiological and behavioral changes can modulate MRI measures without directly reflecting neural neuroplasticity. For example, emotion regulation training can help subjects keep the head still during the scan and thus reduce motion artifacts. Similarly, cardiac pulsation changes after training can lead to measurable artifactual changes in fractional anisotropy in different areas of the brain (Pierpaoli et al., 2003).
Plasticity in the Developing Brain and Methodological Considerations
The young brain can display levels of neuroplasticity that significantly exceed those of the adult brain (Kleim and Jones, 2008). Here, we specifically focus on training-induced, experience-dependent neuroplasticity. This can be differentiated from developmental neuroplasticity, which reflects genetically encoded, time-dependent, sequenced maturational processes (Ismail et al., 2017). While the most drastic genetically encoded changes happen perinatally, the maturational processes continue throughout adolescence (Kadosh et al., 2013), both in gray matter (Giedd et al., 1999), and white matter (Lebel and Beaulieu, 2011). Training-induced plasticity, as one variant of experience-dependent plasticity, is especially abundant in the developing brain (Ismail et al., 2017). This adaptation to the environment is subserved by changes in neuronal processes (axons and dendrites), synapse formation and elimination, and myelin remodeling, as discussed in the previous section.
When conducting MRI studies of training-induced changes in children and adolescents, it has to be taken into account that developmental and experience-dependent neuroplasticity might occur simultaneously (Laube et al., 2020). Table 1 lists side-by-side examples of training-induced neuroplasticity and the overlapping developmental plasticity that might be considered. For example, due to maturational processes, cortical thickness exhibits a preadolescent increase followed by a thinning in adolescence and young adulthood (Giedd et al., 1999). In addition, there are regional differences in maturation with primary motor and sensory areas developing sooner than higher level association and multimodal areas (Gogtay et al., 2004). White matter volume, on the other hand, steadily increases up to adulthood (Gogtay et al., 2004). Cortical thickening in childhood may reflect changes in the level of synaptogenesis. The dissimilar pattern in gray matter and white matter in adolescence is likely to reflect pruning of redundant or unused synaptic connections (loss of gray matter) and the strengthening of relevant connections via myelination based on environmental input and experience (white matter volume increase) (Huttenlocher, 1990). Microstructural maturation of individual white matter tracts also demonstrates dependence on age and location, affecting diffusion MRI metrics (Lebel and Beaulieu, 2011). A longitudinal resting state fMRI (rs-fMRI) study demonstrated, on one hand, established primary visual and motor-sensory networks with minimal intersubject variability in neonates, and on the other hand, age- and sex-dependent maturation and more intersubject variability in higher-order association networks in the first 2 years of life (Gao et al., 2015).
The complex changes in MRI-derived metrics observed with age need to be taken into account when interpreting brain-behavior correlates. Opposite correlations with performance can be observed in children compared with adults. For example, Schnack et al. (2015) analyzed cortical thickness in relation to intelligence in a large longitudinal sample of over 1,000 MRI scans in subjects of age between 9 and 60 years. The researchers showed that at younger age, thinning of the cortex was associated with higher intelligence; however, this relationship was reversed in young adults, where higher IQ correlated positively with increase in cortical thickness (Schnack et al., 2015). Accordingly, researchers discuss how volume growth in specific areas induced by training or other environmental challenges is backed up by selection processes leading to a return to baseline in overall volume (Wenger et al., 2017).
A special concern for training studies also stems from the risk of artifactual findings due to age-related differences in parameters that do not directly reflect neural mechanisms, like head motion or physiological parameters such as neurovascular coupling (Zuo et al., 2017). Because of the ongoing maturational processes in the brain and changes in physiology and behavior during scanning with age, study design considerations are especially critical for MRI studies of training-induced changes in children and adolescents. In their review of studies investigating training-induced structural plasticity in humans, Thomas and Baker (2013) emphasized the importance of a control group.
Ideally, a randomized-controlled study design is employed, in which participants are randomized to a training or control (active or waiting) group (Table 2). With this study design, MRI measures that display a significant interaction between group and timepoint (before vs. after the training) provide evidence for training-dependent plasticity. The selection of an appropriate control group is one of the biggest challenges in training research (Green et al., 2014). Active control groups are usually considered to be the best choice, because passive control groups fail to rule out numerous possible confounds. If clinical populations are studied, the control group should have the same disorder as the training group. Sometimes it is not possible due to ethical considerations (e.g., withholding treatment from depressed adolescents). Additional inclusion of healthy age-matched controls (also randomized to training or waiting) would be ideal to see the differences between the training-induced effects in patients and healthy controls but would require considerable additional recourses.
Additional strength of evidence can be provided by correlation of observed MRI changes with behavioral changes in the training group. If detected changes in MRI measures are the true result of training, it is reasonable to expect that the change in MRI measures would correlate with changes in training-related behavioral measures. Moreover, dose-dependence of the training effects can be studied by comparing, for instance, one low-dose and one high-dose group with the same training.
Another possibility is a controlled within-subject design. In this study design, subjects are scanned three times. No training takes place between the first two scans, and this time interval serves as a control condition. The training then takes place between the second and the third scans (Table 2). This study design can be potentially more powerful than a between-subjects design, as it avoids the effect of between-subjects variance (Poldrack, 2000). One potential problem is the seasonal effects that can differently affect study participants during the no-training and training periods (e.g., school time vs. summer vacation). A counterbalanced version of this study design solves the problem of potential seasonal effects but is not always possible (e.g., when participants acquire skills during training that they are encouraged to use regularly in the everyday life after the training is completed). One can, as a compromise, shift the timelines of different participants (starting points) across different seasons.
Finally, a simple within-subject design can be employed, which would be considered to provide the weakest evidence. As mentioned above, especially in the context of the developing brain, changes detected pre-/posttraining can simply reflect maturational processes. A methodological possibility within this option is to analyze correlation of neural changes with changes in behavior or compare “responders” with “non-responders”.
Research Question and Hypotheses
Given the aforementioned availability of neuroimaging techniques suitable for studying training-induced plasticity in children and adolescents, one would expect that such studies would emerge and potentially demonstrate measurable brain changes. There are, however, no systematic reviews available for this type of research literature. In this systematic review, we aimed at closing this gap and answering the following research question: Are there measurable neural effects of training in children and adolescents that can be captured by MRI? Here, we define training as the process of improving cognitive function or behavior by means of practice. Our review is thus focused on training-induced neuroplasticity, which is a type of experience-dependent neuroplasticity that follows an intentional repeated activity (namely, training). Based on previous literature, including the reviews of relevant studies in children and adults (Jolles and Crone, 2012; Zatorre et al., 2012), the following hypotheses were formulated:
Hypothesis 1 (H1): There are differences in the MRI-derived neural metrics before and after the training in children and adolescents.
Hypothesis 2 (H2): There are differences in changes of the MRI-derived neural metrics between the training group and control group in children and adolescents.
Hypothesis 3 (H3): The improvement in performance in the training group (or among responders) correlates with the MRI-derived neural changes in children and adolescents.
More generally, by performing this systematic review, we also aimed to obtain the data about the number of published research studies on training-induced neuroplasticity in youth, as well as about the utilized MRI techniques, study designs, populations, and types of training.
Methods
Open Science Framework (OSF) preregistration of the project was conducted on 7 May 2018 (https://osf.io/ajr9k). The preanalysis document describes the hypotheses stated above and the methodology as follows.
To review the research literature on training-induced neuroplasticity in children and adolescents, electronic database search and data synthesis were conducted as described in the following sections.
Search Strategy
Electronic databases (PubMed, PsycINFO, and Google Scholar) were searched for all MRI studies of training-induced neural changes in youth published to date. The search was performed in June 2018 and updated in August 2020. To find relevant abstracts, the neuroplasticity-related search terms were neural, brain, (neuro)plasticity, change, reorganization, and reorganisation. The training-related search terms were trainingitherapy, intervention, and exercise. The imaging-related search terms were (f)MRI, magnetic resonance, and DTI. The population-related search terms were children, adolescents, and youth.
The following exclusion criteria were used:
- Studies that are not in English
- Cross-sectional studies (studies that do not include MRI-based measurements before and after the training)
- Animal studies
- Studies with participants older than 18 years
- Non-MRI studies (studies utilizing other types of neuroimaging)
- Studies involving training/therapy/intervention that is not of cognitive or behavioral nature
- Studies that are not peer reviewed.
The process of the systematic search is summarized in Figure 2. PubMed and PsychINFO search initially resulted in 232 non-overlapping records. Eligibility analysis resulted in exclusion of 189 records with the following reasons: 58 of the records were not research articles (review articles, dissertations, book chapters, etc.), 102 articles did not contain training as part of the study protocol, 17 articles did not contain pre- and posttraining MRI (e.g., prognostic pretraining MRI only), in eight of the studies the participants were adults, two of the studies did not contain brain MRI, and two articles described animal studies. Additional 18 articles meeting the eligibility criteria were identified through Google Scholar search and other sources (such as screening the references in other articles). The updated search in August 2020 resulted in 10 additional articles. The total number of articles included in qualitative synthesis was 71.
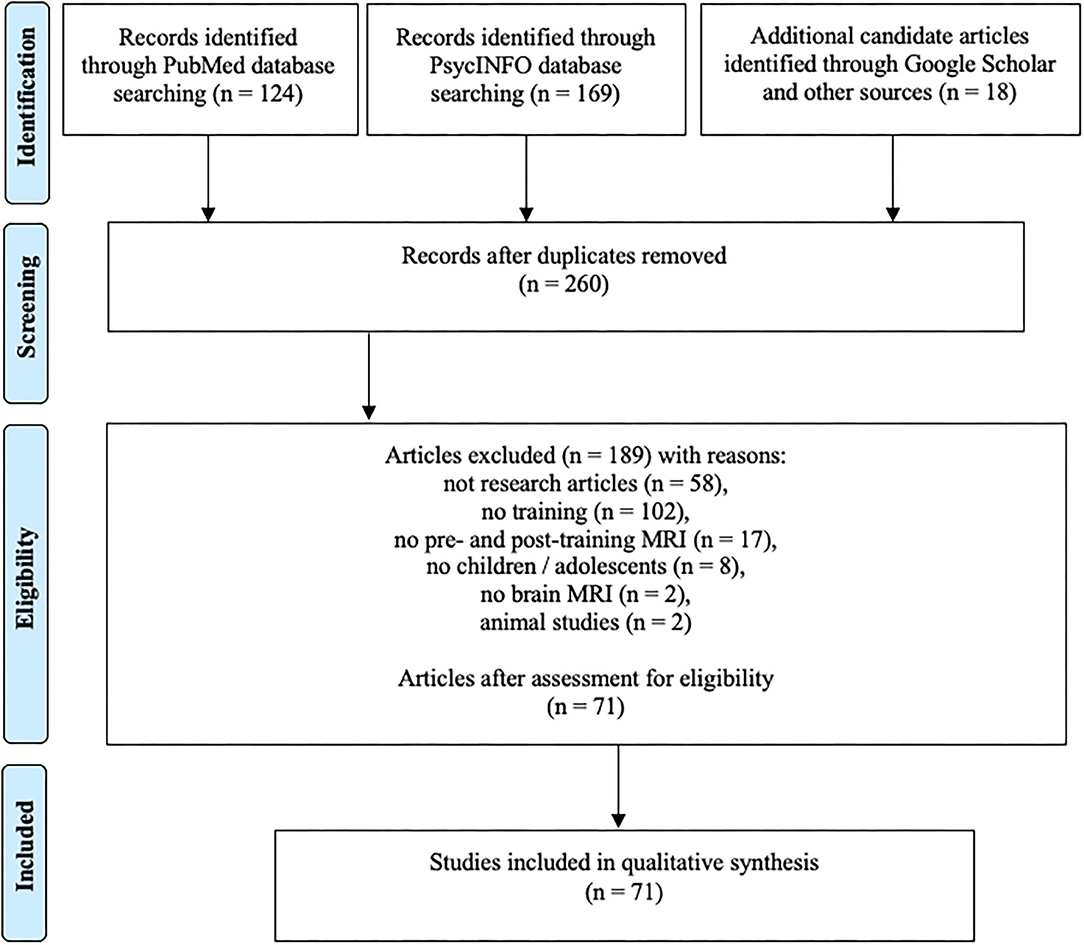
Figure 2. Literature search flow using PRISMA guidelines (Moher et al., 2009).
Data Synthesis
A high heterogeneity was expected among the studies with respect to the study population, type and duration of training, studied brain regions and, importantly, the specific MRI method. Different MRI methods capture different biological phenomena (e.g., changes in white matter myelination, cortical thickness, functional connectivity), and they most likely have different sensitivity to changes and different dynamics (e.g., fMRI changes may be noticeable with shorter trainings). Therefore, a narrative synthesis was chosen instead of a meta-analysis, as meta-analysis is not recommended for diverse study types (Centre for Reviews Dissemination, 2009). The narrative synthesis framework proposed by Popay et al. (2006) can be utilized for this type of analysis. As main elements, the framework suggests to (1) develop a theory of how the intervention works and for whom, (2) preliminarily synthesize the findings of the included studies, (3) further explore relationships in the data, and (4) assess the robustness of the synthesis (Popay et al., 2006; p. 11). These four elements were approached in an iterative manner using the tools proposed by Popay et al. (2006). For the purposes of this review article, the first element of the framework was interpreted in terms of neural changes with training (H1) or neural changes with training compared with a control condition (H2) as a measure for whether the intervention works. Our hypothesis (H3) refers to the third element of the framework (further explore relationships in the data) and states that the improvement in performance in the training group (or among responders) correlates with the MRI-derived neural changes in children and adolescents.
Results
Table 3 provides a brief summary of the study design, population, training type, MRI methodology, and results for each of the 71 included studies. Before we report on the hypotheses, we characterize the type of studies targeting training-induced neuroplasticity in children and adolescents. This is relevant for identifying for which combination of training, target group, and MRI measure we have a reasonable number of studies and which approach is less represented in the literature.
Sample Size. The number of subjects who participated in the training of interest and underwent neuroimaging was below 30 in most studies (63 out of 71 studies, 89%).
Population. We generally categorized study participants younger than 13 years old as children and those who were 13 years old or older as adolescents, since the pubertal status was not always available. The majority of the studies (N = 48, 67.6%) were on children and only two studies involved adolescents and children, limiting the basis for comparisons across age groups. Most of the studies (N = 55; 77.5%) used a special (mostly clinical) population. For instance, there were 9 studies involving ADHD, four on autism, and seven on dyslexia or dyscalculia, while 9 dealt with affective disorders and challenges.
Training Type. Training types ranged from math and piano lessons, to cognitive-behavioral therapy (CBT), to reading training, memory training, exercise, meditation, hippotherapy, and neurofeedback. Most prevalent training type was cognitive (N = 46; 64.8%), whereas 12 studies (16.9%) involved mainly motoric and 18.3% mixed training type. The type of training was distributed depending on population. Among the studies conducted by sampling from the normal population, all but one (mixed) used cognitive training, whereas there were 31 studies with cognitive training and 12 each with mixed and motoric training conducted with a special population [ = 7.81, p = 0.02]. Motoric training was only present among studies involving children (N = 12), whereas there were six mixed training studies each with children and with adolescents, as well as 30 cognitive training studies with children and 15 with adolescents [ = 7.6, p = 0.022]. Participants were younger in studies with motor interventions (M = 9.4 years, SD = 3.94 years) than in studies with mixed interventions [M = 12.5 years, SD = 3.1 years, t(23) = 2.22, p = 0.037], whereas studies with cognitive interventions were in between (M = 11.1 years, SD = 3.1) and did not differ in participant age either from the mixed intervention studies [t(57) = 1.45, p = 0.153] or from the motor studies [t(56) = 1.62, p = 0.11].
Type of MRI. In 70.4% (N = 50) of the studies, functional MRI was involved. Specifically, 58% of the studies were task-based fMRI studies utilizing a variety of tasks. Other studies employed resting-state fMRI, structural MRI, DTI, and MRSI. Studies involving functional imaging were on average conducted with older children (average lower age bound of study: M = 11.7 years, SD = 2.8 years), whereas the lower age bound of the sample was on average lower in studies only involving structural measures [M = 9.7 years, SD = 4 years, t(69) = 2.31, p = 0.024].
Hypothesis H1, stating that there are differences in the MRI-derived neural metrics before and after the training in children and adolescents, was explicitly tested in 56 (79%) of the studies. The results suggest that there are differences in the MRI-derived neural metrics before and after the training in children and adolescents. Only six studies (11%) showed no significant changes in MRI measures in youth with training (Jolles et al., 2012, 2013, 2016b; Catharine et al., 2019; Karoly et al., 2019; Vander Stappen et al., 2020), whereas the remaining 50 studies (89%) showed some changes. Additionally, four studies involving very small numbers of subjects did not report inferential statistical results (Phillips et al., 2007; Cope et al., 2010; Kwon et al., 2014; Lee et al., 2014). Thus, 46 studies out of 56 studies with statistical analysis (82%) showed statistically significant neural changes with training.
Hypothesis H2, stating that there are differences in changes of the MRI-derived neural metrics between the training group and control group in children and adolescents, was explicitly tested in 34 (48%) of the studies. The results suggest that there are differences in changes of the MRI-derived neural metrics between the training group and control group in children and adolescents. The 34 studies utilized some type of a control condition discussed in the “INTRODUCTION” (Table 2), including within-subject and non-randomized designs. One study used a different type of control condition not discussed above: They compared GM volume changes in executive functioning regions compared with control regions in the same subjects (Catharine et al., 2019). Twenty-one studies used a randomized controlled design (30% of all reviewed studies), with 10 of them using an active control group (14% of all reviewed studies). Significant differences compared with the control condition were found in 30 (88%) of the studies that tested H2. Overall, when combining the H1 and H2 results, some neural changes with training (with or without control condition) were reported in 62 (87%) of the 71 studies.
Hypothesis H3, stating that the improvement in performance in the training group (or among responders) correlates with the MRI-derived neural changes in children and adolescents, was explicitly tested in 45 (63%) of the studies. The results suggest that the improvement in performance in the training group (or among responders) correlates with the MRI-derived neural changes in children and adolescents. Specifically, 36 of the 45 studies (80% of the studies that tested H3; 51% of all studies) found significant correlations.
The directionality of the observed changes in the MRI metrics with training strongly depended on the type of MRI modality, specific brain region(s), and nature of training (Table 3, last column). The effect size was only reported in seven studies. In the first study, participants in CBT, relative to controls, showed a significant activation increase in right ventrolateral prefrontal cortex in response to angry faces, t(15) = 3.22, p < 0.01, effect size d = 1.28, cluster size = 29 voxels at p < 0.01 (Maslowsky et al., 2010). However, the authors warn that this large effect size (Cohen, 1988) should be interpreted with caution, since the use of a cluster derived from activation may inflate effect size estimate (Poldrack and Mumford, 2009). Three other fMRI activation studies reported effect sizes of Cohen's d 0.36–0.48 (Iuculano et al., 2015), 1.04 (Rosenberg-Lee et al., 2018), and 0.91–2.21 (Karoly et al., 2019). It needs to be noted that while Rosenberg-Lee et al. (2018) reported increases, Karoly et al. (2019) and Iuculano et al. (2015) reported decreases in brain activation with training, which can be explained by the different nature of training, brain regions, and fMRI tasks [e.g., arithmetic task used by Rosenberg-Lee et al. (2018) vs. visual cannabis cue-reactivity task used by Karoly et al. (2019)]. In the fifth study, Huber et al. (2018) reported effect sizes of Cohen's d = 0.75 and 0.66 for DTI changes after reading training. In the sixth study, the authors used connectomics analysis, in which only a limited number of global (summary) network metrics were derived from whole-brain diffusion MRI images (Yuan et al., 2017). Participants with traumatic brain injury underwent attention training and were compared with passive controls. The normalized clustering coefficient and small-worldness displayed significant group × timepoint interactions, both with large effect sizes: −1.1 and −1.4, respectively. Finally, in the seventh study, an effect size of Cohen's d = 0.47 was reported for gray matter volume decreases in the left posterior insula in adolescents with meditation training (Yuan et al., 2020). Interestingly, the authors report that the observed VBM changes with meditation training in the studied sample of adolescents were opposite in directionality compared with the previously published VBM literature in adults with meditation training (Yuan et al., 2020).
Discussion
This systematic review of MRI studies of training-induced neuroplasticity in children and adolescents has been conducted in order to investigate, whether there are measurable neural effects of training in children and adolescents that can be captured by MRI. We hypothesized that: (1) there would be differences in the MRI-derived neural metrics before and after the training; (2) there would be differences in changes of the MRI-derived neural metrics between the training group and control group; and (3) the improvement in performance in the training group (or among responders) would correlate with the MRI-derived neural changes.
A surprisingly large number of studies met the inclusion criteria: a total of 71 articles were included in the review. Significant changes in brain activation, structure, microstructure, and structural and functional connectivity were reported with different types of trainings in 62 studies (87% of studies), thus supporting the hypothesized neural changes with training. This is a percentage on the higher end of what is generally observed in hypothesis testing studies in the fields of psychology, clinical medicine, and neuroscience and behavior (Fanelli, 2010). Both the effect size and directionality strongly depended on the type of MRI modality, specific brain regions, and nature of training. The effect size is rarely reported in neuroimaging studies, where a large number of non-independent data points are frequently collected and then the same hypothesis test is run on tens of thousands of voxels. Among the included studies, the effect size was only reported in seven studies, ranging from 0.36 to 2.2 (medium—large effect sizes, Cohen, 1988).
The reason for the predominantly positive results can be the presence of reporting biases: publication bias (e.g., selective reporting of complete studies; Dickersin, 2005) as well as the “outcome reporting bias” (p-hacking) within individual studies (Chan et al., 2004). The latter bias is especially likely in the case of MRI-based analyses of training-induced neuroplasticity due to the multitude of brain regions, metrics, and preprocessing steps that can be potentially employed. Poldrack et al. (2017) recently discussed, for example, that in the fMRI analysis, one can apply 6,912 analysis workflows to a single data set. Although not all combinations of parameters are plausible and most studies share very similar workflow, the hidden flexibility in MRI data analysis theoretically “allows presenting anything as significant” (Simmons et al., 2011; p. 1359). Nevertheless, the authors suggest that the best solution is to allow for this flexibility but to require that researchers: (1) preregister the methods and analysis plans, for example, using the OSF and (2) clearly indicate which analyses are exploratory and ideally validate exploratory results, for example, by using a separate validation data set. Finally, a potential means to detect the outcome reporting bias among the studies is to apply p-curve analysis (Simonsohn et al., 2014), which would need to be adapted to neuroimaging, where the hypothesis is often tested for tens of thousands of non-independent voxels in the brain followed by various methods to correct for multiple comparisons.
Unfortunately, the majority of included studies were designed in such a way that only a positive outcome would be informative, thus contributing to the publication bias. That is, they were largely underpowered and therefore a null result would not give any useful information: 89% of the included studied had fewer than 30 subjects who participated in the training of interest and underwent neuroimaging. This does not mean that “null results should not be published, only that studies should be designed so that null results are worth publishing” (Green et al., 2014; p.769). It is surprising that randomized-controlled trials (RCTs) are often accompanied only by sample size calculations to detect clinical improvements, whereas the corresponding calculations for the changes in neuroimaging measures are lacking, despite the high costs of neuroimaging (Reid et al., 2017).
Another reason for the largely positive findings can be the absence of a proper control group that would allow to rule out developmental changes or regression toward the mean in clinical populations. Only 21 of the included studies used a randomized controlled design (30%), with only ten of them using an active control group. Overall, some type of control (including within-subject and non-randomized designs) was used in 34 (48%) of the studies. Thus, our Hypothesis 2, stating that there are differences in changes of MRI-derived metrics between the training group and control group, was tested by 34 studies. It needs to be noted that some of the studies had a passive control group consisting of a different population than the main studied one, for example, typically developing children instead of children with dyslexia (Huber et al., 2018). This limits our ability to make statements about differences in brain changes with vs. without training in the population of interest. We did not consider a study to have a control condition when a different population underwent the same type of training as the population of interest (e.g., Bauer et al., 2019).
Finally, the positive findings can reflect the actual impressive capacity of the developing brain to demonstrate measurable plasticity with training. Organization of human cerebral cortex is significantly less genetically heritable than that of non-human primates, which implies a higher degree of neuroplasticity and stronger environmental influence on brain development in our species (Gómez-Robles et al., 2015). This relative lack of genetic influence is most noticeable in association areas of the brain and is likely reflected in microstructural adaptations in neural circuitry. An important implication of this heightened neuroplasticity is that “the development of neural circuits that underlie behavior is shaped by the environmental, social, and cultural context more intensively in humans than in other primate species, thus providing an anatomical basis for behavioral and cognitive evolution” (Gómez-Robles et al., 2015; p. 14799).
Most studies that looked at the correlation of brain changes and behavior reported a significant correlation for at least one of the brain region's property with at least one behavioral metric (36 studies or 80% of the 45 studies that tested H3). Null results obtained in 9 studies may reflect the underpowered design or the fact that it is not always clear, which behavioral measure is supposed to correlate with the neural change. Finally, it can be a timing issue and the lack of correlation may be due to differences in temporal dynamics of neural and behavioral changes. As discussed in “Training-Induced Biological Changes Measured With MRI,” changes in performance that take place with training may have different timing compared with changes in various neural measures (Kelly and Castellanos, 2014). It may be in part due to the complex reciprocal causality between brain and behavior/performance. While the title of this paper implies that the causality arrow points from behavior (training) to neural remodeling, we also expect the reverse causality to take place: we hypothesize performance improvements caused (mediated) by this neural remodeling. One of the insights from research on Alzheimer's, Parkinson's, Huntington's, and many other brain disorders is that “behavior is the last thing to change” (Insel, 2013). It is therefore plausible to expect situations in which neural remodeling can be detected using MRI before stable performance improvement is observed. In general, it has been argued that neuromarkers often provide better predictions (neuroprognosis) of future (1) education, learning, and performance in children and adults; (2) criminality; (3) health-related behaviors; and (4) responses to pharmacological or behavioral treatments, than traditional behavioral measures (Gabrieli et al., 2015). The exact timing of neural and behavioral changes with different types of training still remains a topic for future research.
Recommendations for Future Studies
Based on the results of this systematic review, the following recommendations for future studies were derived:
- Having appropriate study design, ideally with randomization and active controls (Table 2);
- Having transparency. As discussed above, to increase transparency one can: (1) preregister the methods and analysis plans, for example, using the OSF; (2) clearly indicate which analyses are exploratory. Finally, data and code sharing can enable direct replication and thus provide maximum transparency;
- Taking into account behavioral and physiological changes (e.g., cardiac pulsation) that might affect MRI measures without reflecting underlying neuroplasticity (Table 1);
- Taking into account the complex developmental changes in MRI-derived metrics observed with age when interpreting brain-behavior correlates (Table 1). As discussed above, opposite correlations with performance can be observed in children compared with adults, e.g., in one study, thinning of the cortex was associated with higher intelligence at younger age, however, this relationship was reversed in young adults, where higher IQ correlated positively with increase in cortical thickness (Schnack et al., 2015). Similarly, one of the reviewed training studies (Yuan et al., 2020) reported that the observed VBM changes with meditation training in the studied sample of adolescents were opposite in directionality compared with the previously published VBM literature in adults with meditation training, which may be due to developmental synaptic pruning effects (Giedd et al., 1999);
- Examining long-term effects of the training by conducting additional measurements months or years after the training (e.g., as it was done in Sotnikova et al., 2012);
- Taking into account general methodological considerations that apply to all research of training, such as the lack of transfer effects known as the “curse of specificity” (Green et al., 2014). The curse of specificity refers to the situation in which individuals improve on a task with training but when a new (even very similar) task is given, no benefit of the training is observed (Green et al., 2014). This is especially the case for the laboratory based as opposed to ecological interventions (Bryck and Fisher, 2012). Another of such general methodological issues is the expectation effect (Green et al., 2014). An ethically controversial approach would be to have a control group merely for the expectation effect (i.e., telling the participants in this control group that they are in the “experimental” condition where behavioral improvements are expected). Finally, an often unresolved methodological question in training research is the number of administered tests (in case of MRI studies, both for behavioral and MRI measures). Having a large number of tests has both strong advantages (e.g., the possibility of making inferences for various measures) as well as disadvantages (e.g., cognitive fatigue or reductions in statistical power due to correction for multiple comparisons) and needs to be justified. The order of the tests should ideally vary randomly across participants to prevent one or several of the tests from carrying a disproportionate amount of order effects such as fatigue and compliance.
Future of Neuroplasticity Research
It can be expected that neuroimaging studies of neuroplasticity will continue gaining importance. In general, there are two large areas of applying the gained knowledge on neuroplasticity: clinical and educational.
- Clinical Applications. The majority of training studies in medical field have traditionally been concerned exclusively with the efficacy of the training, rather than why the training is effective (i.e., the mechanism). Recently, the National Institutes of Health (NIH) directed their focus on funding of “mechanistic” studies that would demonstrate that the hypothesized mechanism of action is indeed triggered by the intervention, before conducting a full-size RCT. Neuroimaging plays a central role in this type of studies. Future studies may also shed light on the “dark side” of brain plasticity. Neuroplasticity, the brain's response to environmental experience, resembles a double-edged sword: on one hand, it potentiates growth or recovery when an individual is exposed to normative, benign, or therapeutic environments (Sonuga-Barke, 2017). On the other hand, in an individual exposed to adversity (e.g., childhood maltreatment), the neurocognitive changes in response to the adversity can be seen as beneficial/functional and have adaptive value within that particular context but can mediate mental disorder development in the long-term (McCrory et al., 2017; Sonuga-Barke, 2017). Better understanding of this type of plasticity can have tremendous clinical significance.
- Educational Applications. Training of a specific brain function may require a certain stage of brain development. Curriculum development in the educational system will greatly benefit from the knowledge of such brain development milestones, as well as of sensitive periods (developmental periods during which experience has the most long-lasting effects). The proper foundation for brain-based learning is not yet available to educators, but research is being conducted and neuroimaging technology will keep playing an important role in advancing our knowledge of the developing brain.
In either content domain, future neuroplasticity research can benefit from measurements and models that allow to capture intervention-based change in the brain as well as other brain changes over time. For instance, Kievit et al. (2018) suggest applying latent change score models in longitudinal samples. In part, they can be of use even when there are only two measurement occasions.
Limitations of the Review
There are several limitations to this review. First, because a meta-analysis was not appropriate (see the earlier remarks in this respect) and only few of the studies reported effect size, only very general findings are reported (Table 3). Heterogeneity of MRI modalities, post-processing methods, training types, and brain regions analyzed additionally complicated comparability of results. Both increases and decreases in MRI-derived measures were observed (variable effect direction; Table 3).
A second limitation was our focus only on mechanistic, not prognostic, imaging biomarkers. Prognostic role of imaging may play a critical role in treatment planning of psychiatric and neurologic disorders in the future.
Finally, this review focused on training studies in the developing brain only and did not include comparison between pediatric and adult brain studies of neuroplasticity. To better probe developmental aspects of neuroplasticity, one interesting hypothesis for future reviews would be that the effect size in the young brain is larger than in the adult brain. In one study included in this review, similar reductions in subsequent-memory effects in task-positive brain regions were found after strategy training across age groups: in children, younger adults, and older adults (Brehmer et al., 2016). Another study included in this review directly compared effects of working memory training in children and adults, although inclusion of children was a pilot and both groups were uncontrolled (Jolles et al., 2013). The results did not show practice effects on functional connectivity in children, only in adults. This preliminary finding argues against the hypothesis of stronger neuroplasticity in a younger brain. One possible reason for the lack of the effect in children is the immature structure of their brains. As an alternative explanation, the authors suggest that children already experience working memory practice in school and therefore extra training has less impact on functional connectivity (Jolles et al., 2013). Future studies might focus on comparing children and adolescents (rather than children and adults) as the onset of puberty may affect sensitive phases for cognitive and brain development (see Laube et al., 2020, for a review). For instance, there are recent discussions on whether plasticity for higher order cognitive functioning is higher or lower during adolescence compared with childhood (e.g. Fuhrmann et al., 2015; Piekarski et al., 2017; Laube et al., 2020).
In spite of these limitations, this review provides a comprehensive summary of the published MRI-based findings in youth with various types of mental and behavioral training. On the one hand, the results suggest that there is substantial evidence for training-induced neuroplasticity with different types of training captured with different MRI measures in children and adolescents. The numerous studies contributing to this field are highly diverse with respect to training and population used as well as design and measures. At the same time, our review makes explicit that there are frequent methodological limitations (such as small sample sizes, lack of an adequate control group). The presented overview of the work on training-induced neuroplasticity in adolescents and children offers a possibility to critically evaluate the strength of the accumulated evidence and to provide recommendations for future research.
Author Contributions
OT and RG formulated the research question. OT conducted literature search and data extraction, and RG supervised this process. OT and RG wrote and revised the manuscript. All authors contributed to the article and approved the submitted version.
Funding
This work was supported by the National Center for Complementary and Integrative Health (NCCIH) R21AT009173 and R61AT009864; by the National Center for Advancing Translational Sciences (CTSI), National Institutes of Health, through UCSF-CTSI UL1TR001872; by the American Foundation for Suicide Prevention (AFSP) SRG-1-141-18; by the Eunice Kennedy Shriver National Institute of Child Health and Human Development (NICHD) R01HD072074; by UCSF Research Evaluation and Allocation Committee (REAC) and J. Jacobson Fund; and by the Fahs-Beck Fund for Research and Experimentation at The New York Community Trust. The funding sources had no involvement in study design, in the analysis and interpretation of data, in the writing of the report, and in the decision to submit the article for publication.
Conflict of Interest
The authors declare that the research was conducted in the absence of any commercial or financial relationships that could be construed as a potential conflict of interest.
References
Alves-Pinto, A., Turova, V., Blumenstein, T., Thienel, A., Wohlschläger, A., and Lampe, R. (2015). fMRI assessment of neuroplasticity in youths with neurodevelopmental-associated motor disorders after piano training. Eur. J. Paediatr. Neurol. 19, 15–28. doi: 10.1016/j.ejpn.2014.09.002
Augustijn, M. J. C. M., D'Hondt, E., Leemans, A., Van Acker, L., De Guchtenaere, A., Lenoir, M., et al. (2019). Weight loss, behavioral change, and structural neuroplasticity in children with obesity through a multidisciplinary treatment program. Human Brain Mapp. 40, 137–150. doi: 10.1002/hbm.24360
Bauer, C. C. C., Caballero, C., Scherer, E., West, M. R., Mrazek, M. D., Phillips, D. T., et al. (2019). Mindfulness training reduces stress and amygdala reactivity to fearful faces in middle-school children. Behav. Neurosci. 133, 569–585. doi: 10.1037/bne0000337
Bernardoni, F., King, J. A., Geisler, D., Stein, E., Jaite, C., Nätsch, D., et al. (2016). Weight restoration therapy rapidly reverses cortical thinning in anorexia nervosa: a longitudinal study. NeuroImage 130, 214–222. doi: 10.1016/j.neuroimage.2016.02.003
Blumenfeld-Katzir, T., Pasternak, O., Dagan, M., and Assaf, Y. (2011). Diffusion MRI of structural brain plasticity induced by a learning and memory task. PLoS ONE 6:e20678. doi: 10.1371/journal.pone.0020678
Brehmer, Y., Shing, Y. L., Heekeren, H. R., Lindenberger, U., and Bäckman, L. (2016). Training-induced changes in subsequent-memory effects: no major differences among children, younger adults, and older adults. NeuroImage 131, 214–225. doi: 10.1016/j.neuroimage.2015.11.074
Bryck, R. L., and Fisher, P. A. (2012). Training the brain: practical applications of neural plasticity from the intersection of cognitive neuroscience, developmental psychology, and prevention science. Am. Psychol. 67, 87–100. doi: 10.1037/a0024657
Catharine, V. L., Helena, V., Ellen, D., Guy, V., Karel, D., and Karen, C. (2019). Exploration of gray matter correlates of cognitive training benefit in adolescents with chronic traumatic brain injury. NeuroImage Clin. 23:101827. doi: 10.1016/j.nicl.2019.101827
Centre for Reviews and Dissemination (2009). Systematic Reviews: CRD's 16 Guidance for Undertaking Reviews in Health Care. New York, NY: University of York.
Chan, A.-W., Hróbjartsson, A., Haahr, M. T., Gøtzsche, P. C., and Altman, D. G. (2004). Empirical evidence for selective reporting of outcomes in randomized trials: comparison of protocols to published articles. JAMA 291, 2457–2465. doi: 10.1001/jama.291.20.2457
Chattopadhyay, S., Tait, R., Simas, T., van Nieuwenhuizen, A., Hagan, C. C., Holt, R. J., et al. (2017). Cognitive behavioral therapy lowers elevated functional connectivity in depressed adolescents. EBioMedicine 17, 216–222. doi: 10.1016/j.ebiom.2017.02.010
Choi, J. W., Han, D. H., Kang, K. D., Jung, H. Y., and Renshaw, P. F. (2015). Aerobic exercise and attention deficit hyperactivity disorder: brain research. Med. Sci. Sports Exercise 47, 33–39. doi: 10.1249/MSS.0000000000000373
Chuang, J.-Y., Whitaker, K. J., Murray, G. K., Elliott, R., Hagan, C. C., Graham, J. M., et al. (2016). Aberrant brain responses to emotionally valent words is normalised after cognitive behavioural therapy in female depressed adolescents. J. Affect. Disord. 189, 54–61. doi: 10.1016/j.jad.2015.09.008
Cisler, J. M., Sigel, B. A., Steele, J. S., Smitherman, S., Vanderzee, K., Pemberton, J., et al. (2016). Changes in functional connectivity of the amygdala during cognitive reappraisal predict symptom reduction during trauma-focused cognitive-behavioral therapy among adolescent girls with post-traumatic stress disorder. Psychol. Med. 46, 3013–3023. doi: 10.1017/S0033291716001847
Cohen, J. (1988). Statistical Power Analysis for the Behavioral Sciences (2nd edition). Hillsdale, NJ: Routledge.
Conklin, H. M., Ogg, R. J., Ashford, J. M., Scoggins, M. A., Zou, P., Clark, K. N., et al. (2015). Computerized cognitive training for amelioration of cognitive late effects among childhood cancer survivors: a randomized controlled trial. J. Clin. Oncol. 33, 3894–3902. doi: 10.1200/JCO.2015.61.6672
Cope, S. M., Liu, X.-C., Verber, M. D., Cayo, C., Rao, S., and Tassone, J. C. (2010). Upper limb function and brain reorganization after constraint-induced movement therapy in children with hemiplegia. Dev. Neurorehabil. 13, 19–30. doi: 10.3109/17518420903236247
Datko, M., Pineda, J. A., and Müller, R.-A. (2018). Positive effects of neurofeedback on autism symptoms correlate with brain activation during imitation and observation. Eur. J. Neurosci. 47, 579–591. doi: 10.1111/ejn.13551
Davis, C. L., Tomporowski, P. D., McDowell, J. E., Austin, B. P., Miller, P. H., Yanasak, N. E., et al. (2011). Exercise improves executive function and achievement and alters brain activation in overweight children: a randomized, controlled trial. Health Psychol. 30, 91–98. doi: 10.1037/a0021766
Dayan, E., and Cohen, L. G. (2011). Neuroplasticity subserving motor skill learning. Neuron 72, 443–454. doi: 10.1016/j.neuron.2011.10.008
de Oliveira Rosa, V., Rosa Franco, A., Abrahão Salum Júnior, G., Moreira-Maia, C. R., Wagner, F., Simioni, A., et al. (2019). Effects of computerized cognitive training as add-on treatment to stimulants in ADHD: a pilot fMRI study. Brain Imaging Behav. 14:1933–1944. doi: 10.1007/s11682-019-00137-0
Delalande, L., Moyon, M., Tissier, C., Dorriere, V., Guillois, B., Mevell, K., et al. (2020). Complex and subtle structural changes in prefrontal cortex induced by inhibitory control training from childhood to adolescence. Dev. Sci. 23:e12898. doi: 10.1111/desc.12898
Dickersin, K. (2005). “Publication bias: Recognizing the problem understanding its origins and scope, and preventing harm,” in Publication Bias in Meta-Analysis: Prevention, Assessment and Adjustments, eds H. R. Rothstein, A. J. Sutton, and M. Borenstein (Chichester: Wiley), p. 11–33.
Draganski, B., Gaser, C., Busch, V., Schuierer, G., Bogdahn, U., and May, A. (2004). Neuroplasticity: changes in grey matter induced by training. Nature 427, 311–312. doi: 10.1038/427311a
Draganski, B., and May, A. (2008). Training-induced structural changes in the adult human brain. Behav. Brain Res. 192, 137–142. doi: 10.1016/j.bbr.2008.02.015
Ekerdt, C. E. M., Kühn, C., Anwander, A., Brauer, J., and Friederici, A. D. (2020). Word learning reveals white matter plasticity in preschool children. Brain Struct. Function 225, 607–619. doi: 10.1007/s00429-020-02024-7
Everts, R., Mürner-Lavanchy, I., Schroth, G., and Steinlin, M. (2017). Neural change following different memory training approaches in very preterm born children - A pilot study. Dev. Neurorehabil. 20, 14–24. doi: 10.3109/17518423.2015.1027010
Fanelli, D. (2010). “Positive” results increase down the hierarchy of the sciences. PLoS ONE 5:e10068. doi: 10.1371/journal.pone.0010068
Fenton, A., Meynell, L., and Baylis, F. (2009). Ethical challenges and interpretive difficulties with non-clinical applications of pediatric FMRI. Am. J. Bioethics 9, 3–13. doi: 10.1080/15265160802617829
Fuhrmann, D., Knoll, L. J., and Blakemore, S. J. (2015). Adolescence as a sensitive period of brain development. Trends Cognitve Sci. 19, 558–566, doi: 10.1016/j.tics.2015.07.008
Gabrieli, J. D. E., Ghosh, S. S., and Whitfield-Gabrieli, S. (2015). Prediction as a humanitarian and pragmatic contribution from human cognitive neuroscience. Neuron 85, 11–26. doi: 10.1016/j.neuron.2014.10.047
Gao, W., Alcauter, S., Smith, J. K., Gilmore, J. H., and Lin, W. (2015). Development of human brain cortical network architecture during infancy. Brain Structure Funct. 220, 1173–1186. doi: 10.1007/s00429-014-0710-3
Garrett, A., Cohen, J. A., Zack, S., Carrion, V., Jo, B., Blader, J., et al. (2019). Longitudinal changes in brain function associated with symptom improvement in youth with PTSD. J. Psychiatr. Res. 114, 161–169. doi: 10.1016/j.jpsychires.2019.04.021
Gebauer, D., Fink, A., Filippini, N., Johansen-Berg, H., Reishofer, G., Koschutnig, K., et al. (2012). Differences in integrity of white matter and changes with training in spelling impaired children: a diffusion tensor imaging study. Brain Structure Funct. 217, 747–760. doi: 10.1007/s00429-011-0371-4
Giedd, J. N., Blumenthal, J., Jeffries, N. O., Castellanos, F. X., Liu, H., Zijdenbos, A., et al. (1999). Brain development during childhood and adolescence: a longitudinal MRI study. Nature Neurosci. 2, 861–863. doi: 10.1038/13158
Gogtay, N., Giedd, J. N., Lusk, L., Hayashi, K. M., Greenstein, D., Vaituzis, A. C., et al. (2004). Dynamic mapping of human cortical development during childhood through early adulthood. Proc. Natl. Acad. Sci. U.S.A. 101, 8174–8179. doi: 10.1073/pnas.0402680101
Gómez-Robles, A., Hopkins, W. D., Schapiro, S. J., and Sherwood, C. C. (2015). Relaxed genetic control of cortical organization in human brains compared with chimpanzees. Proc. Natl. Acad. Sci. U.S.A. 112, 14799–14804. doi: 10.1073/pnas.1512646112
Green, C. S., Strobach, T., and Schubert, T. (2014). On methodological standards in training and transfer experiments. Psychol. Res. 78, 756–772. doi: 10.1007/s00426-013-0535-3
Hagmann, P., Cammoun, L., Gigandet, X., Gerhard, S., Grant, P. E., Wedeen, V., et al. (2010). MR connectomics: principles and challenges. J. Neurosci. Methods 194, 34–45. doi: 10.1016/j.jneumeth.2010.01.014
Han, D. H., Kim, S. M., Lee, Y. S., and Renshaw, P. F. (2012). The effect of family therapy on the changes in the severity of on-line game play and brain activity in adolescents with on-line game addiction. Psychiatr. Res. 202, 126–131. doi: 10.1016/j.pscychresns.2012.02.011
Hoekzema, E., Carmona, S., Ramos-Quiroga, J. A., Barba, E., Bielsa, A., Tremols, V., et al. (2011). Training-induced neuroanatomical plasticity in ADHD: a tensor-based morphometric study. Human Brain Mapp. 32, 1741–1749. doi: 10.1002/hbm.21143
Hoekzema, E., Carmona, S., Tremols, V., Gispert, J. D., Guitart, M., Fauquet, J., et al. (2010). Enhanced neural activity in frontal and cerebellar circuits after cognitive training in children with attention-deficit/hyperactivity disorder. Human Brain Mapp. 31, 1942–1950. doi: 10.1002/hbm.20988
Honey, C. J., Sporns, O., Cammoun, L., Gigandet, X., Thiran, J. P., Meuli, R., et al. (2009). Predicting human resting-state functional connectivity from structural connectivity. Proc. Natl. Acad. Sci. U.S.A. 106, 2035–2040. doi: 10.1073/pnas.0811168106
Horowitz-Kraus, T., and HollS, K. (2015). Greater functional connectivity between reading and error-detection regions following training with the reading acceleration program in children with reading difficulties. Annals Dyslexia 65, 1–23. doi: 10.1007/s11881-015-0096-9
Huber, E., Donnelly, P. M., Rokem, A., and Yeatman, J. D. (2018). Rapid and widespread white matter plasticity during an intensive reading intervention. Nat. Commun. 9:2260. doi: 10.1038/s41467-018-04627-5
Huttenlocher, P. R. (1990). Morphometric study of human cerebral cortex development. Neuropsychologia 28, 517–527. doi: 10.1016/0028-3932(90)90031-I
Hyun, G. J., Jung, T.-W., Park, J. H., Kang, K. D., Kim, S. M., Son, Y. D., et al. (2016). Changes in gait balance and brain connectivity in response to equine-assisted activity and training in children with attention deficit hyperactivity disorder. J. Alternat. Complementar. Med. 22, 286–293. doi: 10.1089/acm.2015.0299
Insel, T. (2013). IACC Full Committee Meeting—January 29, 2013 | IACC. (n.d.). Available online at: https://iacc.hhs.gov/meetings/iacc-meetings/2013/full-committee-meeting/january29/ (accessed October 13, 2020).
Ismail, F. Y., Fatemi, A., and Johnston, M. V. (2017). Cerebral plasticity: Windows of opportunity in the developing brain. Eur. J. Paediatr. Neurol. 21, 23–48. doi: 10.1016/j.ejpn.2016.07.007
Iuculano, T., Rosenberg-Lee, M., Richardson, J., Tenison, C., Fuchs, L., Supekar, K., et al. (2015). Cognitive tutoring induces widespread neuroplasticity and remediates brain function in children with mathematical learning disabilities. Nat. Commun. 6:8453. doi: 10.1038/ncomms9453
Johnston, M. V. (2009). Plasticity in the developing brain: implications for rehabilitation. Dev. Disabilit. Res. Rev. 15, 94–101. doi: 10.1002/ddrr.64
Jolles, D., and Crone, E. A. (2012). Training the developing brain: a neurocognitive perspective. Front. Human Neurosci. 6:76. doi: 10.3389/fnhum.2012.00076
Jolles, D., Supekar, K., Richardson, J., Tenison, C., Ashkenazi, S., Rosenberg-Lee, M., et al. (2016a). Reconfiguration of parietal circuits with cognitive tutoring in elementary school children. Cortex 83, 231–245. doi: 10.1016/j.cortex.2016.08.004
Jolles, D., Wassermann, D., Chokhani, R., Richardson, J., Tenison, C., Bammer, R., et al. (2016b). Plasticity of left perisylvian white-matter tracts is associated with individual differences in math learning. Brain Structure Funct. 221, 1337–1351. doi: 10.1007/s00429-014-0975-6
Jolles, D. D., van Buchem, M. A., Crone, E. A., and Rombouts, S. A. R. B. (2013). Functional brain connectivity at rest changes after working memory training. Human Brain Mapp. 34, 396–406. doi: 10.1002/hbm.21444
Jolles, D. D., van Buchem, M. A., Rombouts, S. A. R. B., and Crone, E. A. (2012). Practice effects in the developing brain: a pilot study. Dev. Cogn. Neurosci. 2, S180–S191. doi: 10.1016/j.dcn.2011.09.001
Juenger, H., Kuhnke, N., Braun, C., Ummenhofer, F., Wilke, M., Walther, M., et al. (2013). Two types of exercise-induced neuroplasticity in congenital hemiparesis: a transcranial magnetic stimulation, functional MRI, and magnetoencephalography study. Dev. Med. Child Neurol. 55, 941–951. doi: 10.1111/dmcn.12209
Kadosh, K. C., Linden, D. E. J., and Lau, J. Y. F. (2013). Plasticity during childhood and adolescence: innovative approaches to investigating neurocognitive development. Dev. Sci. 16, 574–583. doi: 10.1111/desc.12054
Karoly, H. C., Schacht, J. P., Jacobus, J., Meredith, L. R., Taylor, C. T., Tapert, S. F., et al. (2019). Preliminary evidence that computerized approach avoidance training is not associated with changes in fMRI cannabis cue reactivity in non-treatment-seeking adolescent cannabis users. Drug Alcohol Dependence 200, 145–152. doi: 10.1016/j.drugalcdep.2019.04.007
Keller, T. A., and Just, M. A. (2009). Altering cortical connectivity: remediation-induced changes in the white matter of poor readers. Neuron 64, 624–631. doi: 10.1016/j.neuron.2009.10.018
Kelly, A. M. C., and Garavan, H. (2005). Human functional neuroimaging of brain changes associated with practice. Cereb. Cortex 15, 1089–1102. doi: 10.1093/cercor/bhi005
Kelly, C., and Castellanos, F. X. (2014). Strengthening connections: functional connectivity and brain plasticity. Neuropsychol. Rev. 24, 63–76. doi: 10.1007/s11065-014-9252-y
Kesler, S. R., Sheau, K., Koovakkattu, D., and Reiss, A. L. (2011). Changes in frontal-parietal activation and math skills performance following adaptive number sense training: preliminary results from a pilot study. Neuropsychol. Rehabil. 21, 433–454. doi: 10.1080/09602011.2011.578446
Kievit, R. A., Brandmaier, A. M., Ziegler, G., van Harmelen, A. L., de Mooij, S., Moutoussis, M., et al. (2018). Developmental cognitive neuroscience using latent change score models: a tutorial and applications. Dev. Cognit. Neurosci. 33, 99–117. doi: 10.1016/j.dcn.2017.11.007
Kim, J. I., Kang, Y.-H., Lee, J.-M., Cha, J., Park, Y.-H., Kweon, K.-J., et al. (2018). Resting-state functional magnetic resonance imaging investigation of the neural correlates of cognitive-behavioral therapy for externalizing behavior problems in adolescent bullies. Progr. Neuro-Psychopharmacol. Biol. Psychiatr. 86:193–202. doi: 10.1016/j.pnpbp.2018.05.024
Kleibeuker, S. W., Stevenson, C. E., van der Aar, L., Overgaauw, S., van Duijvenvoorde, A. C., and Crone, E. A. (2017). Training in the adolescent brain: an fMRI training study on divergent thinking. Dev. Psychol. 53, 353–365. doi: 10.1037/dev0000239
Kleim, J. A., and Jones, T. A. (2008). Principles of experience-dependent neural plasticity: implications for rehabilitation after brain damage. J. Speech Language Hearing Res. 51, S225–S239. doi: 10.1044/1092-4388(2008/018)
Kwon, J.-Y., Chang, W. H., Chang, H. J., Yi, S.-H., Kim, M.-Y., Kim, E.-H., et al. (2014). Changes in diffusion tensor tractographic findings associated with constraint-induced movement therapy in young children with cerebral palsy. Clin. Neurophysiol. 125, 2397–2403. doi: 10.1016/j.clinph.2014.02.025
Laird, A. R., Fox, P. M., Eickhoff, S. B., Turner, J. A., Ray, K. L., McKay, D. R., et al. (2011). Behavioral interpretations of intrinsic connectivity networks. J. Cogn. Neurosci. 23, 4022–4037. doi: 10.1162/jocn_a_00077
Laube, C., van den Bos, W., and Fandakova, Y. (2020). The relationship between pubertal hormones and brain plasticity: implications for cognitive training in adolescence. Dev. Cogn. Neurosci. 42:100753. doi: 10.1016/j.dcn.2020.100753
Lebel, C., and Beaulieu, C. (2011). Longitudinal development of human brain wiring continues from childhood into adulthood. J. Neurosci. 31, 10937–10947. doi: 10.1523/JNEUROSCI.5302-10.2011
Lee, D. R., Kim, Y. H., Kim, D. A., Lee, J. A., Hwang, P. W., Lee, M. J., et al. (2014). Innovative strength training-induced neuroplasticity and increased muscle size and strength in children with spastic cerebral palsy: an experimenter-blind case study–three-month follow-up. NeuroRehabilitation 35, 131–136. doi: 10.3233/NRE-131036
Lee, N., Park, S., and Kim, J. (2017). Hippotherapy and neurofeedback training effect on the brain function and serum brain-derived neurotrophic factor level changes in children with attention-deficit or/and hyperactivity disorder. J. Exercise Nutrition Biochem. 21, 35–42. doi: 10.20463/jenb.2017.0018
Lerch, J. P., Yiu, A. P., Martinez-Canabal, A., Pekar, T., Bohbot, V. D., et al. (2011). Maze training in mice induces MRI-detectable brain shape changes specific to the type of learning. NeuroImage 54, 2086–2095. doi: 10.1016/j.neuroimage.2010.09.086
Lévesque, J., Beauregard, M., and Mensour, B. (2006). Effect of neurofeedback training on the neural substrates of selective attention in children with attention-deficit/hyperactivity disorder: a functional magnetic resonance imaging study. Neurosci. Lett. 394, 216–221. doi: 10.1016/j.neulet.2005.10.100
Logothetis, N. K., Pauls, J., Augath, M., Trinath, T., and Oeltermann, A. (2001). Neurophysiological investigation of the basis of the fMRI signal. Nature 412, 150–157. doi: 10.1038/35084005
Lövdén, M., Schaefer, S., Noack, H., Bodammer, N. C., Kühn, S., Heinze, H.-J., et al. (2012). Spatial navigation training protects the hippocampus against age-related changes during early and late adulthood. Neurobiol. Aging 33, 620.e9–620.e22. doi: 10.1016/j.neurobiolaging.2011.02.013
Maguire, E. A., Gadian, D. G., Johnsrude, I. S., Good, C. D., Ashburner, J., Frackowiak, R. S. J., et al. (2000). Navigation-related structural change in the hippocampi of taxi drivers. Proc. Natl. Acad. Sci. U.S.A. 97, 4398–4403. doi: 10.1073/pnas.070039597
Maslowsky, J., Mogg, K., Bradley, B. P., McClure-Tone, E., Ernst, M., and Monk, C. S. (2010). A preliminary investigation of neural correlates of treatment in adolescents with generalized anxiety disorder. J. Child Adolescent Psychopharmacol. 20, 105–111. doi: 10.1089/cap.2009.0049
Mata, F., Verdejo-Roman, J., Soriano-Mas, C., Yücel, M., and Verdejo-Garcia, A. (2016). Changes in choice evoked brain activations after a weight loss intervention in adolescents. Appetite 103, 113–117. doi: 10.1016/j.appet.2016.04.002
Maximo, J. O., Murdaugh, D. L., O'Kelley, S., and Kana, R. K. (2017). Changes in intrinsic local connectivity after reading intervention in children with autism. Brain Language 175, 11–17. doi: 10.1016/j.bandl.2017.08.008
McCrory, E. J., Gerin, M. I., and Viding, E. (2017). Annual research review: childhood maltreatment, latent vulnerability and the shift to preventative psychiatry – the contribution of functional brain imaging. J. Child Psychol. Psychiatr. 58, 338–357. doi: 10.1111/jcpp.12713
Miyawaki, T., Matsui, K., and Takashima, S. (1998). Developmental characteristics of vessel density in the human fetal and infant brains. Early Human Dev. 53, 65–72. doi: 10.1016/S0378-3782(98)00043-7
Moher D. Liberati A. Tetzlaff J. Altman D. G. The PRISMA Group (2009). Preferred reporting items for systematic reviews and meta-analyses: the PRISMA statement. PLoS Med 6:e1000097. doi: 10.1371/journal.pmed.1000097
Morland, C., Andersson, K. A., Haugen, Ø. P., Hadzic, A., Kleppa, L., Gille, A., et al. (2017). Exercise induces cerebral VEGF and angiogenesis via the lactate receptor HCAR1. Nat. Commun. 8:15557. doi: 10.1038/ncomms15557
Mukherjee, P., Berman, J. I., Chung, S. W., Hess, C. P., and Henry, R. G. (2008). Diffusion tensor MR imaging and fiber tractography: theoretic underpinnings. AJNR 29, 632–641. doi: 10.3174/ajnr.A1051
Murdaugh, D. L., Deshpande, H. D., and Kana, R. K. (2016). The impact of reading intervention on brain responses underlying language in children with autism. Autism Res. 9, 141–154. doi: 10.1002/aur.1503
Murdaugh, D. L., Maximo, J. O., and Kana, R. K. (2015). Changes in intrinsic connectivity of the brain's reading network following intervention in children with autism. Human Brain Mapp. 36, 2965–2979. doi: 10.1002/hbm.22821
Nash, K., Stevens, S., Clairman, H., and Rovet, J. (2017). Preliminary findings that a targeted intervention leads to altered brain function in children with fetal alcohol spectrum disorder. Brain Sci. 8:7. doi: 10.3390/brainsci8010007
Newman, S. D., Hansen, M. T., and Gutierrez, A. (2016). An fMRI study of the impact of block building and board games on spatial ability. Front. Psychol. 7:1278. doi: 10.3389/fpsyg.2016.01278
O'Neill, J., Piacentini, J. C., Chang, S., Levitt, J. G., Rozenman, M., Bergman, L., et al. (2012). MRSI correlates of cognitive–behavioral therapy in pediatric obsessive–compulsive disorder. Progress in Neuro-Psychopharmacology and Biol. Psychiatr. 36, 161–168. doi: 10.1016/j.pnpbp.2011.09.007
Park, S., Lee, J.-M., Baik, Y., Kim, K., Yun, H. J., Kwon, H., et al. (2015). A preliminary study of the effects of an arts education program on executive function, behavior, and brain structure in a sample of nonclinical school-aged children. J. Child Neurol. 30, 1757–1766. doi: 10.1177/0883073815579710
Patsenko, E. G., Adluru, N., Birn, R. M., Stodola, D. E., Kral, T. R. A., Farajian, R., et al. (2019). Mindfulness video game improves connectivity of the fronto-parietal attentional network in adolescents: a multi-modal imaging study. Sci. Rep. 9:18667. doi: 10.1038/s41598-019-53393-x
Phillips, J. P., Sullivan, K. J., Burtner, P. A., Caprihan, A., Provost, B., and Bernitsky-Beddingfield, A. (2007). Ankle dorsiflexion fMRI in children with cerebral palsy undergoing intensive body-weight-supported treadmill training: a pilot study. Dev. Med. Child Neurol. 49, 39–44. doi: 10.1017/S0012162207000102.x
Piekarski, D. J., Johnson, C. M., Boivin, J. R., Thomas, A. W., Lin, W. C., Delevich, K., et al. (2017). Does puberty mark a transition in sensitive periods for plasticity in the associative neocortex? Brain Res. 1654, 123–144, doi: 10.1016/j.brainres.2016.08.042
Pierpaoli, C., Marenco, S., Rohde, G. K., Jones, D. K., and Barnett, A. (2003). Analyzing the contribution of cardiac pulsation to the variability of quantities derived from the diffusion tensor. Proc. Int. Soc. Magn. Reson. Med. 11:70. Available online at: https://www.ncbi.nlm.nih.gov/pmc/articles/PMC3539838/
Poldrack, R. A. (2000). Imaging brain plasticity: conceptual and methodological issues–a theoretical review. NeuroImage 12, 1–13. doi: 10.1006/nimg.2000.0596
Poldrack, R. A., Baker, C. I., Durnez, J., Gorgolewski, K. J., Matthews, P. M., Munaf,ò, M. R., et al. (2017). Scanning the horizon: towards transparent and reproducible neuroimaging research. Nat. Rev. Neurosci. 18, 115–126. doi: 10.1038/nrn.2016.167
Poldrack, R. A., and Mumford, J. A. (2009). Independence in ROI analysis: where is the voodoo? Soc. Cognit. Affect. Neurosci. 4, 208–213. doi: 10.1093/scan/nsp011
Popay, P., Roberts, H., Sowden, A., Petticrew, M., Arai, L., and Rodgers, M. (2006). Guidance on the Conduct of Narrative Synthesis in Systematic Reviews. A product from the ESRC Methods Programme. Lancaster: Institute of Health Research.
Rapoport, J. L., and Gogtay, N. (2008). Brain neuroplasticity in healthy, hyperactive and psychotic children: insights from neuroimaging. Neuropsychopharmacology 33, 181–197. doi: 10.1038/sj.npp.1301553
Reid, L. B., Pagnozzi, A. M., Fiori, S., Boyd, R. N., Dowson, N., and Rose, S. E. (2017). Measuring neuroplasticity associated with cerebral palsy rehabilitation: An MRI based power analysis. Int. J. Dev. Neurosci. 58, 17–25. doi: 10.1016/j.ijdevneu.2017.01.010
Riggs, L., Piscione, J., Laughlin, S., Cunningham, T., Timmons, B. W., Courneya, K. S., et al. (2017). Exercise training for neural recovery in a restricted sample of pediatric brain tumor survivors: a controlled clinical trial with crossover of training versus no training. Neuro-Oncology 19, 440–450. doi: 10.1093/neuonc/now177
Robillard, K. N., Lee, K. M., Chiu, K. B., and MacLean, A. G. (2016). Glial Cell Morphological and Density Changes Through the Lifespan of Rhesus Macaques. Brain Behavior Immunity 55, 60–69. doi: 10.1016/j.bbi.2016.01.006
Rocca, M. A., Fumagalli, S., Pagani, E., Gatti, R., Riccitelli, G. C., Preziosa, P., et al. (2017). Action observation training modifies brain gray matter structure in healthy adult individuals. Brain Imaging Behav. 11, 1343–1352. doi: 10.1007/s11682-016-9625-3
Romeo, R. R., Christodoulou, J. A., Halverson, K. K., Murtagh, J., Cyr, A. B., Schimmel, C., et al. (2018). Socioeconomic status and reading disability: neuroanatomy and plasticity in response to intervention. Cereb. Cortex 28, 2297–2312. doi: 10.1093/cercor/bhx131
Rosenberg-Lee, M., Iuculano, T., Bae, S. R., Richardson, J., Qin, S., Jolles, D., et al. (2018). Short-term cognitive training recapitulates hippocampal functional changes associated with one year of longitudinal skill development. Trends Neurosci. Educat. 10, 19–29. doi: 10.1016/j.tine.2017.12.001
Sampaio-Baptista, C., Filippini, N., Stagg, C. J., Near, J., Scholz, J., and Johansen-Berg, H. (2015). Changes in functional connectivity and GABA levels with long-term motor learning. NeuroImage 106, 15–20. doi: 10.1016/j.neuroimage.2014.11.032
Satterthwaite, T. D., Wolf, D. H., Loughead, J., Ruparel, K., Elliott, M. A., Hakonarson, H., et al. (2012). Impact of in-scanner head motion on multiple measures of functional connectivity: relevance for studies of neurodevelopment in youth. NeuroImage 60, 623–632. doi: 10.1016/j.neuroimage.2011.12.063
Scheau, C., Preda, E., Popa, G., Ghergus, A., Capsa, R., and Lupescu, I. (2012). Magnetic resonance spectroscopy – a non-invasive method in evaluating focal and diffuse central nervous system disease. J. Med. Life 5, 423–427. Available online at: http://citeseerx.ist.psu.edu/viewdoc/download?doi=10.1.1.192.4688&rep=rep1&type=pdf
Schnack, H. G., van Haren, N. E. M., Brouwer, R. M., Evans, A., Durston, S., Boomsma, D. I., et al. (2015). Changes in thickness and surface area of the human cortex and their relationship with intelligence. Cereb. Cortex 25, 1608–1617. doi: 10.1093/cercor/bht357
Simmons, J. P., Nelson, L. D., and Simonsohn, U. (2011). False-positive psychology: undisclosed flexibility in data collection and analysis allows presenting anything as significant. Psychol. Sci. 22, 1359–1366. doi: 10.1177/0956797611417632
Simonsohn, U., Nelson, L. D., and Simmons, J. P. (2014). P-curve: a key to the file-drawer. J. Exp. Psychol. 143, 534–547. doi: 10.1037/a0033242
Singh, M. K., Nimarko, A. F., Garrett, A. S., Gorelik, A. J., Roybal, D. J., Walshaw, P. D., et al. (2020). Changes in intrinsic brain connectivity in family-focused therapy versus standard psychoeducation among youth at high risk for bipolar disorder. J. Am. 31:S0890-8567(20)31334-4. doi: 10.1016/j.jaac.2020.07.892
Siniatchkin, M., Glatthaar, N., Müller, G. G., von, Prehn-Kristensen, A., Wolff, S., Knöchel, S., et al. (2012). Behavioural treatment increases activity in the cognitive neuronal networks in children with attention deficit/hyperactivity disorder. Brain Topography, 25, 332–344. doi: 10.1007/s10548-012-0221-6
Smith, S. M., Fox, P. T., Miller, K. L., Glahn, D. C., Fox, P. M., Mackay, C. E., et al. (2009). Correspondence of the brain's functional architecture during activation and rest. Proc. Natl. Acad. Sci. U.S.A. 106, 13040–13045. doi: 10.1073/pnas.0905267106
Soh, D. W., Skocic, J., Nash, K., Stevens, S., Turner, G. R., and Rovet, J. (2015). Self-regulation therapy increases frontal gray matter in children with fetal alcohol spectrum disorder: evaluation by voxel-based morphometry. Front. Human Neurosci. 9:108. doi: 10.3389/fnhum.2015.00108
Sonuga-Barke, E. J. S. (2017). Commentary: Extraordinary environments, extreme neuroplasticity and mental disorder - reflections on pathways from adversity to mental disorder prompted by McCrory, Gerin, and Viding (2017). J. Child Psychol. Psychiatr. Allied Disciplines 58, 358–360. doi: 10.1111/jcpp.12726
Sorrells, S. F., Paredes, M. F., Cebrian-Silla, A., Sandoval, K., Qi, D., Kelley, K. W., et al. (2018). Human hippocampal neurogenesis drops sharply in children to undetectable levels in adults. Nature 555, 377–381. doi: 10.1038/nature25975
Sotnikova, A., Steinmann, E., Wendisch, V., Müller, G. G., Stephani, U., Petermann, F., et al. (2012). Long-term effects of a multimodal behavioural ADHD training: a fMRI study. Zeitschrift Für Neuropsychol. 23, 205–213. doi: 10.1024/1016-264X/a000075
Sterling, C., Taub, E., Davis, D., Rickards, T., Gauthier, L. V., Griffin, A., et al. (2013). Structural neuroplastic change after constraint-induced movement therapy in children with cerebral palsy. Pediatrics 131, e1664–e1669. doi: 10.1542/peds.2012-2051
Stevens, M. C., Gaynor, A., Bessette, K. L., and Pearlson, G. D. (2016). A preliminary study of the effects of working memory training on brain function. Brain Imaging Behav. 10, 387–407. doi: 10.1007/s11682-015-9416-2
Stoddard, J., Sharif-Askary, B., Harkins, E. A., Frank, H. R., Brotman, M. A., Penton-Voak, I. S., et al. (2016). An open pilot study of training hostile interpretation bias to treat disruptive mood dysregulation disorder. J. Child Adolescent Psychopharmacol. 26, 49–57. doi: 10.1089/cap.2015.0100
Straub, J., Plener, P. L., Sproeber, N., Sprenger, L., Koelch, M. G., Groen, G., et al. (2015). Neural correlates of successful psychotherapy of depression in adolescents. J. Affect. Disord. 183, 239–246. doi: 10.1016/j.jad.2015.05.020
Strawn, J. R., Cotton, S., Luberto, C. M., Patino, L. R., Stahl, L. A., Weber, W. A., et al. (2016). Neural function before and after mindfulness-based cognitive therapy in anxious adolescents at risk for developing bipolar disorder. J. Child Adolescent Psychopharmacol. 26, 372–379. doi: 10.1089/cap.2015.0054
Supekar, K., Iuculano, T., Chen, L., and Menon, V. (2015). Remediation of childhood math anxiety and associated neural circuits through cognitive tutoring. J. Neurosci. 35, 12574–12583. doi: 10.1523/JNEUROSCI.0786-15.2015
Szulc-Lerch, K. U., Timmons, B. W., Bouffet, E., Laughlin, S., de Medeiros, C. B., Skocic, J., et al. (2018). Repairing the brain with physical exercise: cortical thickness and brain volume increases in long-term pediatric brain tumor survivors in response to a structured exercise intervention. NeuroImage 18, 972–985. doi: 10.1016/j.nicl.2018.02.021
Theurel, A., and Gentaz, E. (2018). The regulation of emotions in adolescents: age differences and emotion-specific patterns. PLoS ONE, 13:195501. doi: 10.1371/journal.pone.0195501
Thomas, C., and Baker, C. I. (2013). Teaching an adult brain new tricks: a critical review of evidence for training-dependent structural plasticity in humans. NeuroImage 73, 225–236. doi: 10.1016/j.neuroimage.2012.03.069
Vander Stappen, C., Dricot, L., and Van Reybroeck, M. (2020). RAN training in dyslexia: Behavioral and brain correlates. Neuropsychologia 146:107566. doi: 10.1016/j.neuropsychologia.2020.107566
Wang, C., Weng, J., Yao, Y., Dong, S., Liu, Y., and Chen, F. (2017). Effect of abacus training on executive function development and underlying neural correlates in Chinese children. Human Brain Mapp. 38, 5234–5249. doi: 10.1002/hbm.23728
Weinstein, M., Myers, V., Green, D., Schertz, M., Shiran, S. I., Geva, R., et al. (2015). Brain plasticity following intensive bimanual therapy in children with hemiparesis: preliminary evidence. Neural Plasticity 2015:798481. doi: 10.1155/2015/798481
Wenger, E., Brozzoli, C., Lindenberger, U., and Lövdén, M. (2017). Expansion and renormalization of human brain structure during skill acquisition. Trends Cognit. Sci. 21, 930–939. doi: 10.1016/j.tics.2017.09.008
Whitwell, J. L. (2009). Voxel-based morphometry: an automated technique for assessing structural changes in the brain. J. Neurosci. 29, 9661–9664. doi: 10.1523/JNEUROSCI.2160-09.2009
Yuan, J. P., Connolly, C. G., Henje Blom, E., Sugrue, L. P., Yang, T. T., Xu, D., et al. (2020). Grey matter changes in adolescents participating in a meditation training. Front Hum. Neurosci. 14:319. doi: 10.3389/fnhum.2020.00319
Yuan, W., Treble-Barna, A., Sohlberg, M. M., Harn, B., and Wade, S. L. (2017). Changes in structural connectivity following a cognitive intervention in children with traumatic brain injury. Neurorehabil. Neural Repair 31, 190–201. doi: 10.1177/1545968316675430
Zatorre, R. J., Fields, R. D., and Johansen-Berg, H. (2012). Plasticity in gray and white: neuroimaging changes in brain structure during learning. Nat. Neurosci. 15, 528–536. doi: 10.1038/nn.3045
Zou, P., Li, Y., Conklin, H. M., Mulhern, R. K., Butler, R. W., and Ogg, R. J. (2012). Evidence of change in brain activity among childhood cancer survivors participating in a cognitive remediation program. Arch. Clin. Neuropsychol. 27, 915–929. doi: 10.1093/arclin/acs095
Keywords: brain, plasticity, training, adolescent, child, development, neuroimaging, MRI
Citation: Tymofiyeva O and Gaschler R (2021) Training-Induced Neural Plasticity in Youth: A Systematic Review of Structural and Functional MRI Studies. Front. Hum. Neurosci. 14:497245. doi: 10.3389/fnhum.2020.497245
Received: 08 February 2020; Accepted: 01 December 2020;
Published: 18 January 2021.
Edited by:
Megan Herting, University of Southern California, United StatesReviewed by:
Corinna Laube, Fresenius University of Applied Sciences, GermanyTibor Auer, University of Surrey, United Kingdom
Copyright © 2021 Tymofiyeva and Gaschler. This is an open-access article distributed under the terms of the Creative Commons Attribution License (CC BY). The use, distribution or reproduction in other forums is permitted, provided the original author(s) and the copyright owner(s) are credited and that the original publication in this journal is cited, in accordance with accepted academic practice. No use, distribution or reproduction is permitted which does not comply with these terms.
*Correspondence: Olga Tymofiyeva, Olga.Tymofiyeva@ucsf.edu