- 1Department of Biomedical Engineering, Oregon Health & Science University, Portland, OR, United States
- 2Department of Neurology, Oregon Health & Science University, Portland, OR, United States
- 3Veterans Adminstration Portland Health Care System, Portland, OR, United States
Postural instability in Parkinson’s disease (PD) is characterized by impaired postural responses to transient perturbations, increased postural sway in stance and difficulty transitioning between tasks. In addition, some studies suggest that loss of dopamine in the basal ganglia due to PD results in difficulty in using proprioceptive information for motor control. Here, we quantify the ability of subjects with PD and age-matched control subjects to use and re-weight sensory information for postural control during steady-state conditions of continuous rotations of the stance surface or visual surround. We measure the postural sway of subjects in response to a pseudorandom, surface-tilt stimulus with eyes closed, and in response to a pseudorandom, visual-tilt stimulus. We use a feedback control model of the postural control system to interpret our results, focusing on sensory weighting as a function of stimulus amplitude. We find that subjects with PD can re-weight their dependence upon sensory information in response to changes in surface- or visual-stimulus amplitude. Specifically, subjects with PD behaved like age-matched control subjects by decreasing proprioceptive contribution to stance control with increasing surface-tilt amplitude and decreasing visual contribution with increasing visual-tilt amplitude. However, subjects with PD do not decrease their reliance on proprioception as much as age-matched controls for small increases in surface-stimulus amplitudes. Levodopa medication did not affect sensory re-weighting behaviors for postural control. The impairment in PD subject’s ability to respond differently to small changes in surface rotation amplitudes is consistent with an increased threshold for perceiving proprioceptive signals, which may result from decreased signal-to-noise in the dopaminergic pathways associated with sensory processing and/or sensory integration.
Introduction
Evidence suggests that the basal ganglia are involved in processing and integrating sensory information (Abbruzzese and Berardelli, 2003; Nagy et al., 2006). There is increasing evidence that basal ganglia-related diseases, such as Parkinson’s disease (PD), are associated with kinesthetic deficits, including reduced tactile discrimination, poor joint kinesthesia, asymmetrical spatial pointing, and over-estimating of reaching and stepping when vision is not available (Maschke et al., 2003; Jacobs and Horak, 2006; Tagliabue et al., 2009; Wright et al., 2010). PD also results in motor signs of postural instability, rigidity, tremor and bradykinesia (Horak et al., 1992; Bloem et al., 2001), due to loss of dopaminergic and other neurons throughout the central nervous system, with the severity of motor symptoms related to the amount of nigral-striatal dopamine (Agid, 1991). Although rigidity, tremor and bradykinesia are improved with dopamine replacement therapy, postural control and risk of falls does not improve and may even worsen with levodopa (Horak et al., 1992, 1996).
People with PD fall five times more than age-matched controls (Fasano et al., 2017). Evidence for abnormal postural control in patients with PD comes from studies of unperturbed, quiet stance and studies where balance was perturbed by various sensory stimuli. For quiet stance studies, the effect of PD on postural sway, as quantified by center of pressure (CoP) or center of mass (CoM) displacement, is controversial and may depend upon the sensory conditions and on how CoP or CoM displacement is quantified (Mancini et al., 2011; Curtze et al., 2015; Ozinga et al., 2017; Cruz et al., 2018). Sway area in subjects with PD, when standing with eyes open or closed, can be similar to sway in age-matched controls (Bronstein et al., 1990; Chong et al., 1999a; Bronte-Stewart et al., 2002), especially during early stages of PD (Frenklach et al., 2009). However, sway velocity and jerk have been shown to be increased, even in early PD without medication (Mancini and Horak, 2010; Mancini et al., 2011). In addition, CoP displacement is increased in patients with PD off medication as compared to controls, especially in the mediolateral direction, and levodopa replacement increases CoP displacement (Rocchi et al., 2002). As PD progresses, postural sway area tends to be correlated with the severity of PD (Frenklach et al., 2009).
Studies using perturbed stance have also shown conflicting results. Subjects with PD can generate appropriate sway, even while experiencing a sinusoidal surface displacement (De Nunzio et al., 2007). However, approximately 50% of subjects with PD sway more than age-matched controls with eyes closed on a sway-referenced surface (Bronte-Stewart et al., 2002). Increased sway in subjects with PD under this condition could indicate vestibular dysfunction, because stance with eyes closed on a sway-referenced surface requires increased reliance upon vestibular information. However, peripheral vestibular function is thought to be normal in subjects with PD (Pastor et al., 1993; Bronstein et al., 1996), and a recent study suggests that subjects with PD rely more on vestibular information than control subjects to control postural sway during stance, irrespective of treatment with medication or stimulation of the subthalamic nucleus (Maurer, 2009).
Alternatively, increased sway during sway-referenced conditions with eyes closed could be related to an impaired ability to quickly reorganize the sensory contributions to balance control. To maintain postural stability under suddenly changing sensory conditions, individuals must quickly alter how much they depend upon vision, proprioception, and vestibular information (Peterka and Loughlin, 2004; Jeka et al., 2008; Assländer and Peterka, 2016). It is well known that subjects with PD have a reduced ability to quickly change postural set when sensory or cognitive conditions suddenly change (Chong et al., 2000). For example, subjects with PD take longer than controls to achieve steady-state postural responses following eyes closed to eyes open transitions during sinusoidal surface displacements (Brown et al., 2006; De Nunzio et al., 2007). Furthermore, subjects with PD do not decrease sway with repeated exposure to lateral displacement of visual stimuli (Bronstein et al., 1990). However, many of the studies that manipulate the availability of orientation cues from different sensory systems use short-duration tests. As a result, the observed behavioral differences between subjects with PD and controls may be due to the reduced ability of subjects with PD to quickly adjust when sensory conditions are altered rather than a fundamental inability of subjects with PD to appropriately use sensory information if they are given enough time to adjust.
Our primary goal was to test the fundamental abilities of subjects with PD to adjust to sensory conditions and to regulate sensory integration for postural control in steady-state conditions. We quantified subjects’ relative reliance on visual, vestibular, and proprioceptive information for postural orientation in response to sensory stimuli. Young, healthy subjects typically rely primarily on proprioceptive cues during eyes closed stance but shift toward decreased reliance on proprioception and increased reliance on vestibular cues when the stance is perturbed by support surface rotations of increasing amplitude (Peterka, 2002). Similarly, when visual cues are perturbed by visual surround rotations of increasing amplitude, subjects decrease their reliance on visual orientation cues (Peterka, 2002).
The quantitative assessment of reliance on a particular sensory modality is made by estimating sensory weighting parameters (Peterka, 2002). The sensory weights are parameters in a linear feedback control system model of the postural control system. Specifically, in our postural control model (Figure 1), the relative reliance on each sensory modality (i.e., vision, proprioceptive, vestibular) is represented as a weighting parameter. Sensory weighting is constrained by Wvis + Wvest + Wprop = 1, where Wvis is the visual weight, Wvest is the vestibular weight, and Wprop is the proprioceptive weight. Therefore, the sensory weight for each of the three weighting parameters can range from 0 to 1. When a subject’s eyes are closed, Wvis = 0 and the sensory weighting constraint is reduced to Wvest + Wprop = 1. Additional parameters of the model include a position- and a velocity-dependent neural control parameter, a neural time delay, and torque feedback implemented as a low-pass filter with a gain and time constant (Figure 1). Previous results showed that young adult subjects with normal sensory function very precisely regulate sensory weights with little variation across subjects and systematically alter these weights as the amplitude of the perturbations change (Peterka, 2002).
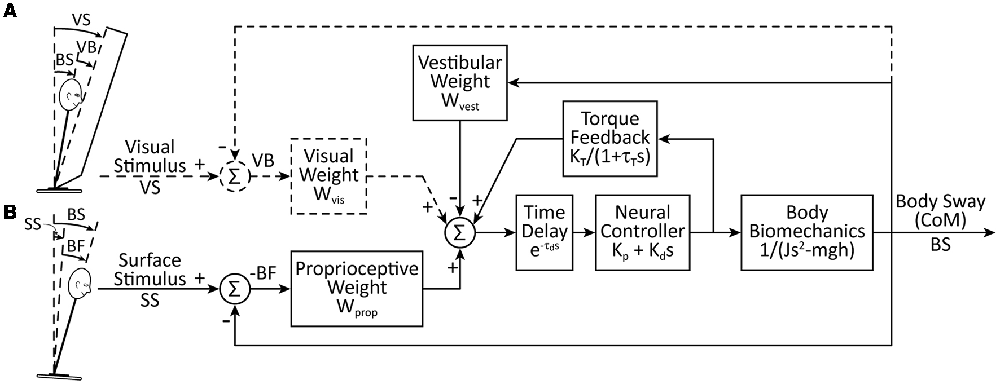
Figure 1. A simplified feedback control model of postural control, including sensory weighting and torque feedback. For the data and analysis we present here, we considered (A) a visual-stimulus with a stationary surface and (B) a surface-stimulus with eyes closed. The model for the surface-stimulus condition (Wvis = 0) is indicated by the solid lines in the schematic. For the visual-stimulus condition, the dashed lines are added to the model and there is no surface stimulus. For the sensory integration component of the model, we constrained Wprop + Wvest + Wvis = 1. The body biomechanics are modeled as an inverted pendulum. The biomechanics, neural controller, torque feedback, and time delay blocks include Laplace transform representations of the differential equations of these model components where s is the Laplace variable.
Our secondary goal was to determine whether levodopa influences sensory integration. Although dopamine replacement with levodopa medication improves many motor symptoms of PD, its effects on postural control are complex. For example, levodopa improves rigidity, bradykinesia, and tremor, but automatic postural responses (Horak et al., 1996) and postural sway during stance (Nardone and Schieppati, 2006; Rocchi et al., 2006a) worsen with levodopa. Previously, we reported that levodopa does not reduce excessive axial postural tone during stance, despite the reduction of limb rigidity (Wright et al., 2007). However, some components of postural control may improve with levodopa, such as the magnitude of anticipatory postural adjustments prior to movement (Burleigh-Jacobs et al., 1997). There is also evidence that dopaminergic medication further impairs kinesthesia (O’Suilleabhain et al., 2001; Mongeon et al., 2009), although studies have not reported how dopamine replacement affects sensory re-weighting for postural control.
Given that subjects with PD are reported to have various limitations and deficits regarding sensory processing, we hypothesized that sensory re-weighting for postural control would be impaired in subjects with PD. To test this hypothesis, we measured the postural sway of PD and age-matched control subjects while standing in response to pseudorandom surface- and visual-rotations and then used our postural control model to estimate postural control parameters. In this study, we focused primarily on the change of sensory weighting parameters when sensory conditions change. We also tested the additional hypothesis that levodopa medication would improve sensory weighting for postural control.
Materials and Methods
Subjects
The Institutional Review Board at Oregon Health and Science University (OHSU) approved the protocol for this experiment, and all subjects gave informed consent prior to participating. Eight subjects with PD (three female) and eight healthy, age-matched controls (two female) were recruited from the Balance Disorders Laboratory database and the Parkinson’s Center of Oregon Clinic at OHSU.
Subjects with PD were selected based on the following inclusion criteria: (1) a diagnosis of idiopathic PD; (2) levodopa responsive, as demonstrated by a lower score on the Unified PD Rating Scale (UPDRS) motor examination when on anti-Parkinsonian medication compared to off medication; and (3) the ability to stand unsupported for 5 min both on and off medication. Subjects with PD were excluded if they had other neurological, sensory, or muscular disorders (e.g., diabetes, peripheral neuropathies, uncorrected visual problems, arthritis, stroke, or seizure).
Control subjects were selected so that no significant differences existed between subjects with PD and controls in age (p = 0.79), height (p = 0.96), or weight (p = 0.71). Additional selection criteria for control subjects were: (1) no known neurological, sensory, or muscular problems; and (2) the ability to stand unsupported for 5 min. Table 1 describes the anthropometric and clinical characteristics of the subjects with PD and the mean anthropometric characteristics of the control subjects. All subjects with PD in the off medication state (PDOff), except Subject 1, either had a Hoehn and Yahr score of three or greater or showed impaired balance control in response to a backwards pull on the shoulders [part of the postural instability and gait (PIGD) sub-score of the UPDRS].
We also included data from four younger controls (mean age = 37 years.) that were part of a previously published study (Peterka, 2002).
Experimental Apparatus
We used a custom-built, balance-testing device for the experiments (Peterka, 2002). The device is comprised of a motor-driven support surface and a motor-driven visual surround. The subject stood on the support surface and faced the visual surround. The support surface can rotate in a toe-up/toe-down direction about the subject’s ankle joints and uses force sensors (Transducer Techniques, Temecula, CA, USA) to measure the subject’s CoP. The visual surround is a half-cylinder, imprinted with a random, complex checkerboard pattern, which can rotate in the anteroposterior direction about the subject’s ankle joint. The balance-testing device measures anterior-posterior body sway by recording the displacement of two sway rods; each sway rod is comprised of a potentiometer (Midori Precisions Co, LTD—Tokyo, Japan) connected to a light metal rod. One sway rod rests in a hook at the subject’s hip height, and the second sway rod rests in a hook at the subject’s shoulder height (see Peterka et al., 2018 for details).
Experimental Design
Each experiment consisted of six blocks of trials: (1) a calibration trial, (2) surface-stimulus trials, (3) a sway-referenced trial, (4) visual-stimulus trials, (5) a quiet-stance trial, and (6) surface-stimulus trials (repeat). Table 2 lists the blocks of trials and the number of trials comprising each block. The surface-stimulus trials (blocks 2 and 6) and the visual-stimulus trials (block 4) were the focus of the experiment; these blocks tested whether subjects could re-weight sensory information in response to changing sensory stimuli. The sway-referenced trial (block 3) and quiet-stance trial (block 5) tested whether the behavioral characteristics of our subjects with PD were comparable to previously published results.
Control subjects performed the experiment once, and subjects with PD performed the experiment twice: once on and once off medication. For off-medication testing, subjects were tested at least 12 h after their last dose of anti-Parkinsonian medication. Five subjects (PD1, PD2, PD3, PD7, and PD8) were tested off medication the first day and on medication the second day. Two subjects were tested on medication the first day and off medication the second day (PD4 and PD6). One subject (PD5) was tested off and then on medication on the same day.
During all testing, except during calibration, subjects wore headphones and listened to an audio book to minimize conscious control of their posture. To prevent injury in the event of losing balance, subjects wore a harness attached to the ceiling of the room, and a researcher spotted them at all times. Subjects rested between trials to minimize fatigue. If a subject fell on a trial, the trial was repeated. If the second attempt also resulted in a fall, a third trial was attempted. All subjects successfully completed all trial types in three attempts, except for the sway-referenced trial. If a subject did not successfully complete a full sway-referenced trial within three attempts, we proceeded to the next block of trials.
Calibration Trial
The calibration trial (block 1) defined the relationship between the displacement of the sway rods and the displacement of a subject’s CoM (Peterka, 2002; Peterka et al., 2018). During the 120 s trial, subjects were vocally cued to lean slowly forward and backward through a range of hip and/or ankle angles while they stood with eyes opened on a stationary support surface.
Surface-Stimulus Trials
The purpose of the surface-stimulus trials (blocks 2 and 6) was to determine the dynamic characteristics of responses to surface perturbations and to identify how subjects alter their use of proprioceptive orientation cues as a function of stimulus amplitude.
Each surface-stimulus trial was performed with eyes closed. Following 10 s of standing on a stationary surface, the surface rotated according to a stimulus derived from a pseudorandom ternary sequence (PRTS; Davies, 1970). The PRTS was chosen because it has properties similar to white noise (i.e., flat velocity power spectrum over a wide bandwidth), and it appears unpredictable to subjects (see Peterka, 2002 for details). Each trial consisted of four sequential cycles of the PRTS, with each cycle lasting 43.72 s.
In each surface-stimulus block (blocks 2 and 6), subjects performed three surface-stimulus trials with peak-to-peak amplitudes of 2°, 1°, and 4° for the first, second, and third trials, respectively. Subjects rested between trials to prevent fatigue. The surface-stimulus trials of block 6 were a repetition of the surface-stimulus trials of block 2, to determine any learning effect on the subject’s ability to maintain balance.
Visual-Stimulus Trials
The purpose of the visual-stimulus trials (block 4) was to determine the dynamic characteristics of responses to visual perturbations and to identify how subjects alter their use of visual cues for spatial orientation as a function of stimulus amplitude.
On each visual-stimulus trial, subjects stood with eyes opened on a stationary support surface looking forward into the visual surround, but not staring at a single point in the pattern. After 10 s, the visual surround rotated according to a PRTS stimulus while the support surface remained stationary. Each trial consisted of four sequential cycles of the PRTS, with each cycle lasting 60.5 s. Subjects performed three visual-stimulus trials with peak-to-peak amplitudes of 2°, 1°, and 4° on the first, second, and third trials, respectively. The subjects rested between trials to prevent fatigue.
A lower bandwidth PRTS was used for the visual stimulus trials than the surface-stimulus trials, because the motor controlling the visual surround had a lower bandwidth than the motor controlling the support surface. Therefore, the PRTS for the visual-stimulus trials contained lower frequencies than the PRTS for the surface-stimulus trials. Consequently, the length of a single cycle of the PRTS was longer for the visual-stimulus trials (60.5 s) than the surface-stimulus trials (43.72 s).
Quiet-Standing Trial
The purpose of the quiet-standing trial (block 5) was to quantify the magnitude of the subject’s unperturbed body sway. For the quiet-standing trial, the subject stood upright with eyes closed on the stationary support surface for 120 s.
Sway-Referenced Trial With Eyes Closed
The sway-referenced trial (block 3) determined whether subjects could change their reliance on sensory information to maintain their balance when vision was absent and relevant proprioceptive cues were suddenly eliminated. In this trial, subjects were required to rely on vestibular information to maintain balance. During the sway-referenced trial, the subjects stood upright on a stationary support surface with their eyes closed. After 60 s, the angular displacement of the lower body, measured using the hip sway rod, was used to control the angular position of the support surface for 60 s; the surface rotated in direct proportion to the subject’s lower body angle with a proportionality constant of 1 (Peterka and Loughlin, 2004). The trial ended with the subject standing quietly on the stationary support surface for another 60 s. These sway-referenced trials are comparable to condition five of the clinical Sensory Organization Test (Horak, 1987; Black et al., 1988).
Data Analysis
Linear Model for Calibration Trial
Using sway data from each subject’s calibration trial, a linear model was used to relate sway rod displacement to CoM displacement, CoM = A1SS + A2SH + OFF, where SS and SH are body displacements measured using the sway rods at shoulder level and hip level, respectively. The coefficients A1 and A2 are multipliers for the shoulder and hip displacement, respectively, and OFF is an offset. Based on the assumption that, for slow movements, CoP displacement approximates CoM displacement (Brenière, 1996; Winter et al., 1998), the A1, A2, and OFF coefficients were determined by minimizing the mean squared error of measured CoP minus the estimated CoM using the fmincon function in MATLAB R2008b (The MathWorks, Inc., Natick, MA, USA). For subsequent trials, the calculated A1, A2, and OFF coefficients from each subject were used in the linear model, described above, to calculate the CoM displacement from the sway rod measured displacements. A recent publication provides a detailed explanation of this method and includes a Matlab program for the calibration analysis (Supplementary Materials in Peterka et al., 2018).
CoM measurement using this method accounted for the combined motions of the upper and lower body segments and thus provided valid CoM measures whether subjects use ankle or hip strategies. Previous studies have shown that subjects with PD tend to use an inverted pendulum ankle strategy to control standing posture while a mixed hip-ankle strategy is used in control subjects (Baston et al., 2016; Matsuda et al., 2016).
Root Mean Square (RMS)
We calculated the root mean square (RMS) for: (1) the CoM displacement for surface- and visual-stimulus trials; (2) the PRTS stimulus displacement for the surface- and visual-stimulus trials; (3) the CoM displacement for successful sway-referenced trials; and (4) the CoP displacement for quiet-standing trials. The signals were zero-meaned prior to calculating their RMS values.
For surface- and visual-stimulus trials, CoM sway data for each subject were first averaged across all available cycles and then an RMS value of the average waveform was calculated. A comparison of the RMS of CoM sway between blocks 2 and 6 indicated that there was no significant learning between blocks in response to surface-stimuli. Therefore, all six cycles from these two blocks were averaged before calculating the RMS, which increased the signal-to-noise ratio of our data. Three cycles of CoM sway data were used for the RMS calculation of responses to visual stimuli for each subject.
Analysis for PRTS Trials
Analysis of the surface- and visual-stimulus trials in the time and frequency domains has been previously described (Peterka, 2002). In brief, we considered the subject’s response to the first cycle of the PRTS stimulus to be a transitional cycle during which the subject’s response did not yet reach a steady-state. Therefore, only the second, third, and fourth cycles of a given trial were included in the analysis. For all time domain analyses, we calculated a subject’s average response to each stimulus by averaging the response (CoM displacement) across the three steady-state cycles (cycles 2, 3 and 4). For the frequency domain analysis, we used spectral analyses of each cycle of the stimulus and response in a given trial. The various spectra were averaged across cycles, and further averaged across adjacent frequencies, to yield frequency response function (FRF) and coherence function values at frequency points that were approximately linearly spaced on a logarithmic frequency scale for each stimulus type and for each subject (see Peterka, 2002 for details). FRFs are expressed as gain and phase values that represent the amplitude and timing, respectively, of CoM sway relative to the stimulus across frequency. We computed the mean FRF across subjects and calculated the mean gain and phase curves from the mean FRF.
Feedback Control Model
Model Choice and Optimization
We implemented a model-based interpretation of responses to surface- and visual-stimuli by applying a feedback model of the postural control system, which has been described previously (Peterka, 2003; Cenciarini and Peterka, 2006). Briefly, our model included components for sensory weighting (Wprop, Wvis, and Wvest), neural stiffness (Kp), neural damping (Kd), neural time delay (τd), and torque feedback (KT) with a low-pass filter time-constant (τT).
Model parameters were estimated using optimized fits to the FRF data. For both the surface- and visual-stimulus trials, fits were made to the three FRFs from each block simultaneously. For the surface-stimulus trials, optimal fits were performed for each subject individually allowing Wprop and τd to vary across the three stimulus amplitudes, but allowing only single values of Kp, Kd, τT, and KT. These constraints on the optimization fits provided parsimonious descriptions of the experimental FRFs while limiting the total number of free parameters. We also calculated the mean FRF across all subjects and the optimal parameters that fit the mean data.
For the visual-stimulus trials, optimal fits were made only to the mean FRFs for each stimulus amplitude, because the responses to visual-stimulus trials were noisier, and the FRFs fits to individual subjects were not insightful. The visual-stimulus trials were optimized allowing Wvis to vary across the three stimulus amplitudes, but allowing only single values of τd, Kp, Kd, τT, and KT.
Statistical Analyses
For all statistical analyses, we considered: (1) the effect of PD; and/or (2) the effect of anti-Parkinsonian medication on postural control mechanisms. To determine the effect of PD, we quantified the effect of PD on postural control mechanisms by comparing the behavior of control subjects (C) to PDOff. To determine the effect of medication, we quantified the effect of medication on steady-state postural control mechanisms by comparing the behavior of PDOff to subjects with PD on medication (PDOn). The threshold for significance was p < 0.05 for all statistical tests. All statistical analyses were computed in R (The R Project for Statistical Computing; www.r-project.org).
Individual Comparisons
Due to the size of our groups, we could not reasonably test whether our data were normally distributed. Therefore, we used the more conservative non-parametric tests to determine significance of individual comparisons. For individual comparisons, the effect of disease (i.e., C compared to PDOff) was assessed with the two-sample Wilcoxon rank-sum test, and the effect of medication (i.e., PDOff compared to PDOn) was assessed with the paired Wilcoxon signed-rank test. For statistical testing of the clinical measures of the UPDRS, and sub-scores of the UPDRS, we used a single-sided distribution, because we were testing whether there was an improvement in the UPDRS score on vs. off medication. All other individual comparison tests were calculated with a two-sided distribution.
Repeated-Measures ANOVAs
We used repeated-measures ANOVAs (i.e., using aov in R) to test the hypotheses that PD influences: (1) CoM displacement in response to surface-stimuli, (2) Wprop in response to surface-stimuli, and (3) the time-delay parameter in our feedback control model. In the above three ANOVAs, RMS of CoM displacement, Wprop, and τd were the dependent variables, respectively. We included factors for group (i.e., Controls vs. PDOff), stimulus amplitude (i.e., 1°, 2°, and 4°), and an interaction effect (i.e., group × stimulus amplitude). We also included a random factor for subject, accounting for the fact that each subject performed the experiment for all three stimulus amplitudes.
Similarly, we used a repeated-measures ANOVA to test the hypothesis that levodopa influenced Wprop in response to surface-stimuli. This ANOVA included factors for medication conditions (i.e., ON vs. OFF), stimulus amplitude, and an interaction effect. This ANOVA also included a random factor for subject, accounting for the fact that each subject performed the experiment for all three stimulus amplitudes and two medication conditions.
Results
Clinical Balance and Quiet Stance Measures
Quiet stance measures of sway and clinical signs on and off levodopa were similar to those reported in previous studies. During quiet stance with eyes open, RMS of CoP displacement was not significantly different between PDOff and control subjects in the anteroposterior direction (Figure 2A; p = 0.38) or in the mediolateral direction (p = 0.083). Levodopa increased the RMS of CoP displacement from PDOff to PDOn in the mediolateral (p = 0.023), but not in the anteroposterior direction (p = 0.20), as shown previously (Mitchell et al., 1995; Rocchi et al., 2002; Curtze et al., 2015).
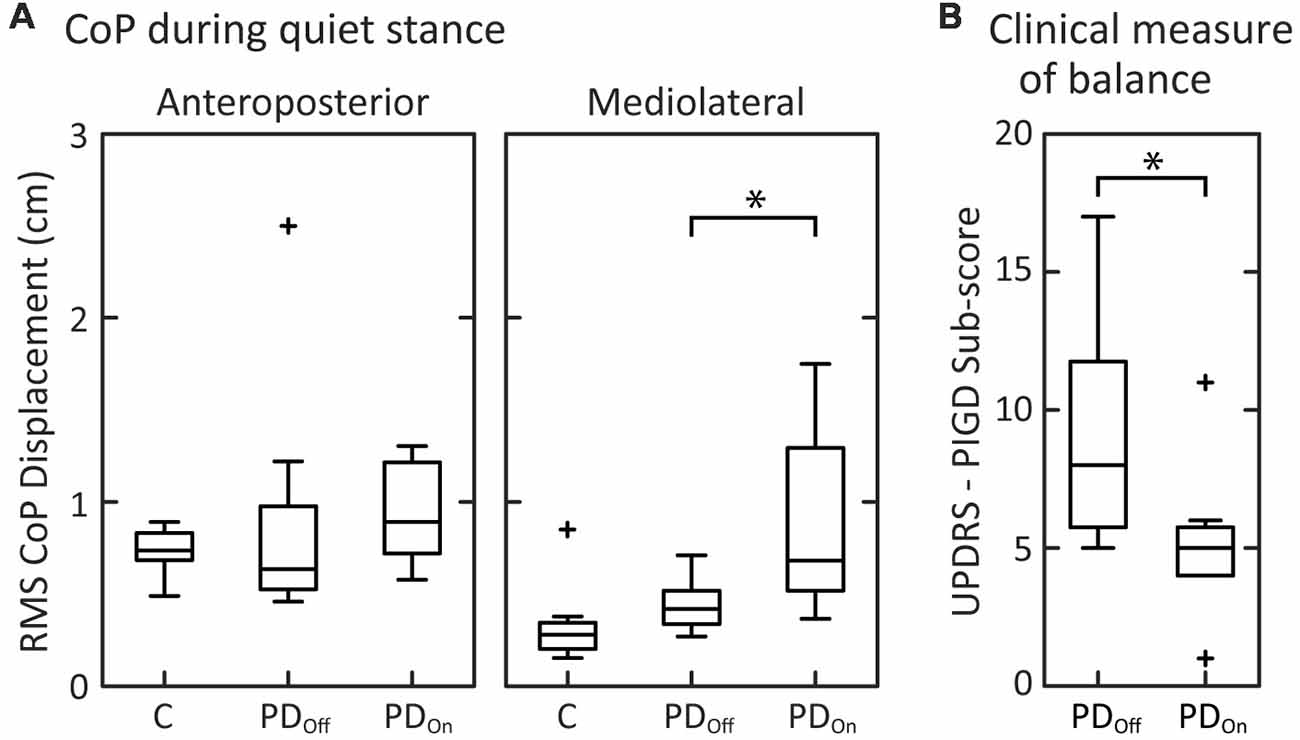
Figure 2. Clinical measures of balance and center of pressure (CoP) measurements. (A) Box and whisker plots of the CoP during quiet stance for the anteroposterior and mediolateral directions. (B) Box and whisker plots of the postural instability and gait disorders components (PIGD) of the Unified Parkinson’s Disease Rating Scale (UPDRS; N = 7; PD1 is not included due to incomplete data for the UPDRS off medication). For each subject group, the center line is the median, the bottom of the box is the 25th percentile (Q1), and the top of the box is the 75th percentile (Q3). The whiskers extend to include all data points that are within the range defined by Q1−1.5(Q3 - Q1) and Q3 + 1.5(Q3 - Q1). Data points that extend beyond the whiskers are defined as outliers and denoted with a “+.” Brackets with “*” indicate significant differences between mean values.
The peak-to-peak CoM sway of the PDOn and elderly control subjects, who did not fall on the sway-referenced trial, were consistent with previously published results (Chong et al., 1999a). Two of the eight controls, three of the eight PDOn subjects, and four of the eight PDOff subjects fell on all three attempts of standing on a sway-referenced surface with eyes closed. This result is consistent with previous results (Bronte-Stewart et al., 2002; Frenklach et al., 2009) showing that a subset of patients with PD fall on all sway-referenced attempts, regardless of disease severity.
As expected, levodopa improved the UPDRS III Motor score (p = 0.011). Levodopa also improved the PIGD sub-score of the UPDRS (Items 26–30; Figure 2B; p = 0.018) as well as rigidity (Item 22; Table 1; p = 0.021), and bradykinesia (Item 31; Table 1; p = 0.027).
Stimulus-Evoked Sway
For all stimulus amplitudes, control subjects and subjects with PD on and off medication tended to orient the angular displacement of their body CoM to either the moving support surface (Figure 3A) or visual surround (Figure 3B). At the lowest stimulus amplitudes, CoM sway was larger than the surface-stimulus amplitude (Figure 3A, row 1). CoM sway increased with increasing surface-stimulus amplitude. However, control subjects did not sway as much as subjects with PD at the largest surface-stimulus amplitude (Figure 3A, row 3). Control subjects swayed less than the surface-stimulus amplitude, and PDs swayed approximately the same as the surface-stimulus amplitude in response to the 4° surface-stimulus. CoM sway was similar between PDOff and PDOn for all surface-stimulus amplitudes (Figure 3A, two rightmost columns). The variability across subjects of the CoM sway was larger in PDOff and PDOn than control subjects (Figure 3A, gray shaded regions).
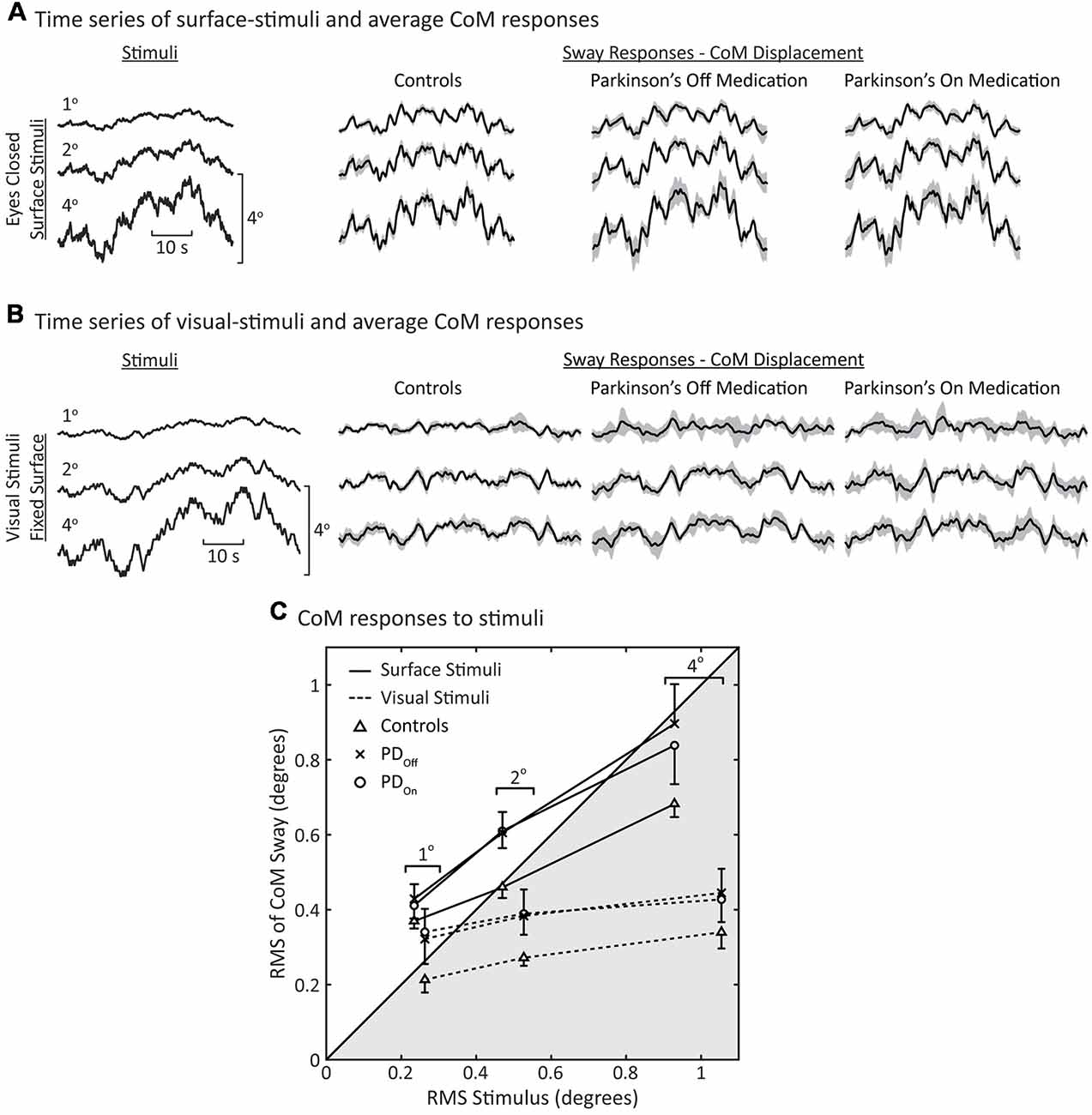
Figure 3. Sway during surface and visual stimuli. Time series of average center of mass (CoM) responses to (A) surface stimuli and (B) visual stimuli. The shaded gray regions represent the 95% confidence intervals of the mean response across subjects. (C) Root mean square (RMS) of the CoM sway vs. RMS of the stimulus. Each data point represents the average behavior across subjects (N = 8 for each group). Data in the unshaded half of the figure represents CoM responses that are larger than the stimulus (ratio >1); the shaded portion of the plot represents responses that are smaller than the stimulus (ratio <1). Note that the surface and visual stimuli were different pseudorandom ternary sequences (PRTSs; see Stimuli columns in (A,B) and see “Materials and Methods” section), consequently the RMS of the stimuli are slightly different for the same peak-to-peak stimulus amplitude. The error bars denote standard errors for the surface-stimulus data. For clarity, only single-sided error bars are shown.
As with surface stimuli, all subjects tended to orient their CoM sway to the visual stimulus (Figure 3B). Overall, responses to visual stimuli were smaller in magnitude than responses to surface stimuli (Figures 3A,B). There were no obvious differences in the average sway of PDOff, PDOn, and control subjects in response to visual-stimuli. However, the variability across subjects was greater for subjects with PD than controls in response to visual stimuli (Figure 3B, gray shaded regions).
To quantify the degree by which subjects increased body sway in response to increased surface-stimulus amplitudes, we calculated the RMS of the CoM sway and stimulus (Figure 3C). There was an increase in the RMS of CoM sway with increasing surface-stimulus amplitude for PDOff, PDOn, and control subjects. This increase in the RMS of CoM sway was smaller than the increase in the RMS of the stimulus. PDOff, PDOn and control subjects had similar sway in response to 1° surface stimuli, and all subject groups swayed more than the stimulus amplitude in response to the 1° surface stimulus (Figure 3C, 1° data in white region).
For larger surface-stimulus amplitudes, subjects with PD showed greater RMS sway than controls (Figure 3C, solid lines). Statistical analysis based on a repeated-measures ANOVA showed a significant main effect of stimulus amplitude (p < 0.001) on the RMS of CoM sway. The main effect of disease on the RMS of CoM sway was not significant (p = 0.059), nor was the interaction between disease and stimulus amplitude (p = 0.11).
For visual stimuli, the RMS sway was similar for PDOff and PDOn with controls having lower RMS sway than subjects with PD at all stimulus amplitudes (Figure 3C, dashed lines). For subjects with PD and controls, RMS sway levels showed moderate increases with increasing visual stimulus amplitude and these sway levels were smaller than for surface stimuli.
Postural Dynamics
Individual Responses to Surface-Stimuli
We used a frequency domain analysis to characterize each subjects’ postural sway over a range of perturbation frequencies. Figure 4 shows examples of experimental FRFs and model fits for an individual PDOff subject’s response to three surface-stimulus amplitudes. The main feature of the FRF is a decreasing gain with increasing stimulus amplitude (Figure 4, top). The phase is similar across stimulus amplitude for most frequencies, except at higher frequencies where there is a slightly larger phase lag for the 1° than for 2° and 4° stimulus amplitudes (Figure 4, middle). The coherence of the experimental data decreases with increasing frequency (Figure 4, bottom). Figure 4 also shows the model fits to the experimental data (see Figure 1 for model). The model fits replicate the main features of the experimental data, with decreasing dependence on proprioception (smaller Wprop) accounting for the gain decrease as stimulus amplitude increases and decreasing time delay (τd) accounting for phase changes at higher frequencies.
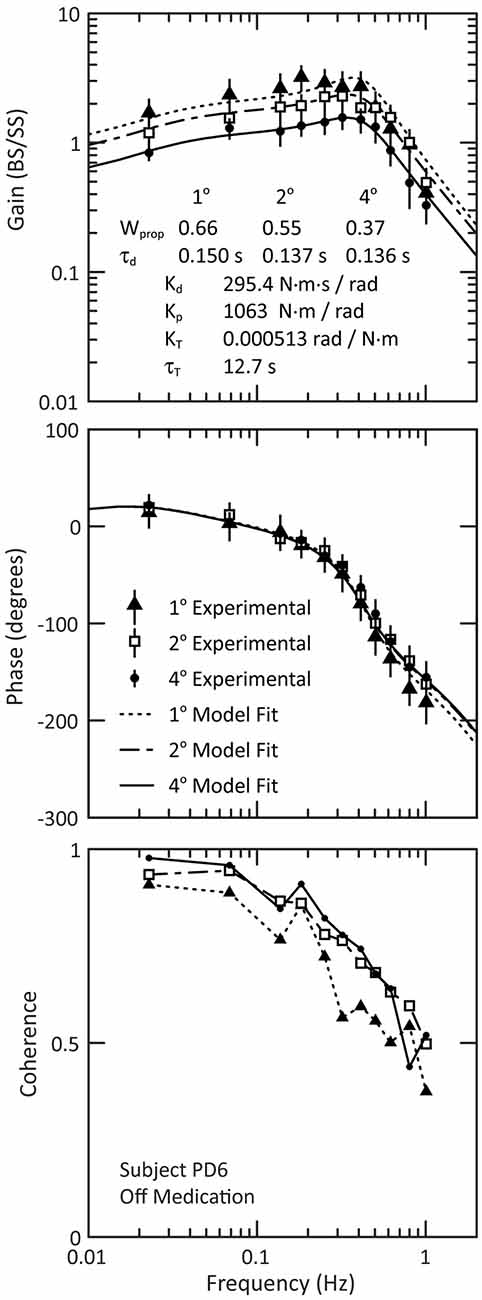
Figure 4. Example of experimental frequency response functions and model fits for PD subject PD6 off medication. The experimental data are shown for the gain (top figure) and phase (middle figure), and the 95% confidence intervals of the mean are indicated by the vertical lines through each data point of the gain and phase curves. The bottom figure shows the coherence of the experimental data. Note that the lines in the coherence plot (bottom figure) are for visualization of the experimental data; these lines are not related to the model fits.
Group Responses to Surface-Stimuli
The results observed for the individual PDOff subject (Figure 4) were representative not only of the mean behavior across all PDOff subjects but also of the mean behavior for the control and PDOn subjects. The mean behavior of each subject group included a decrease in the gain with increasing stimulus amplitude, and small phase changes at higher stimulus frequencies (Figure 5). There were no qualitative differences between PDOff, PDOn, and control subjects in the gain, phase or coherence curves for any stimulus amplitude. Consequently, the parameters of the model fits to the mean group data were similar for PDOff, PDOn, and control subjects.
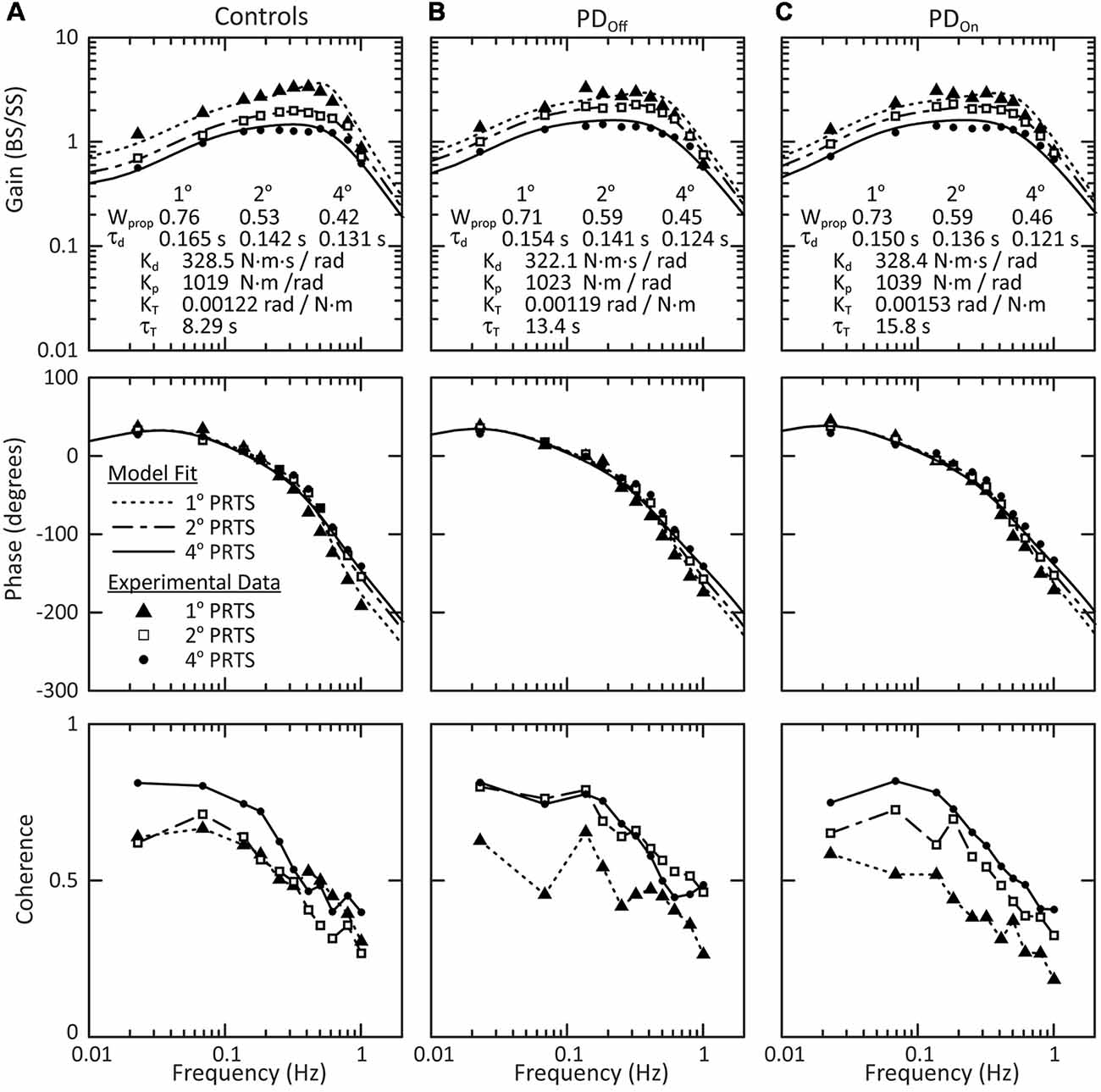
Figure 5. Mean behavior across subjects in response to surface stimuli with eyes closed. Gain, phase and coherence curves for (A) control subjects, (B) subjects with PD off medication, and (C) subjects with PD on medication. The data points denote experimental data for the mean behavior across subjects and the curves on the gain and phase plots are the model fits to this mean behavior. The parameters of the model fits are listed in the gain plots. Data for all three stimulus amplitudes were fit simultaneously, with a Wprop and τd for each stimulus amplitude, and a single Kd, Kp, KT, and τT across all stimulus amplitudes. The lines connecting data points in the coherence plots (bottom row) are for visualization and are not related to model fits.
Model-Based Interpretation for Surface Stimuli
There were no significant effects of disease (controls vs. PDOff) on sensory-to-motor characteristics of posture control: Kp (p = 0.88), Kd (p = 0.80), τd (p = 0.74), KT (p = 0.64), or τT (p = 0.083). Medication (PDOff vs. PDOn) also did not significantly affect Kp (p > 0.99), Kd (p = 0.38), τd (p = 0.82), KT (p = 0.20), or τT (p = 0.25). All subject groups showed a significant decrease in proprioceptive weighting (Wprop) with increasing surface-stimulus amplitude (Figure 6A; p < 0.001). Although the mean Wprop was larger for controls than for subjects with PD at 1° and smaller for controls than for subjects with PD at 2° and 4°, there was no significant main effect of disease (controls vs. PDOff) on Wprop (Figure 6A; p = 0.84), and there was no interaction effect between group and stimulus amplitude (p = 0.28). In addition, there was no main effect of medication on Wprop (p = 0.92) and no interaction between medication and stimulus amplitude (p = 0.82).
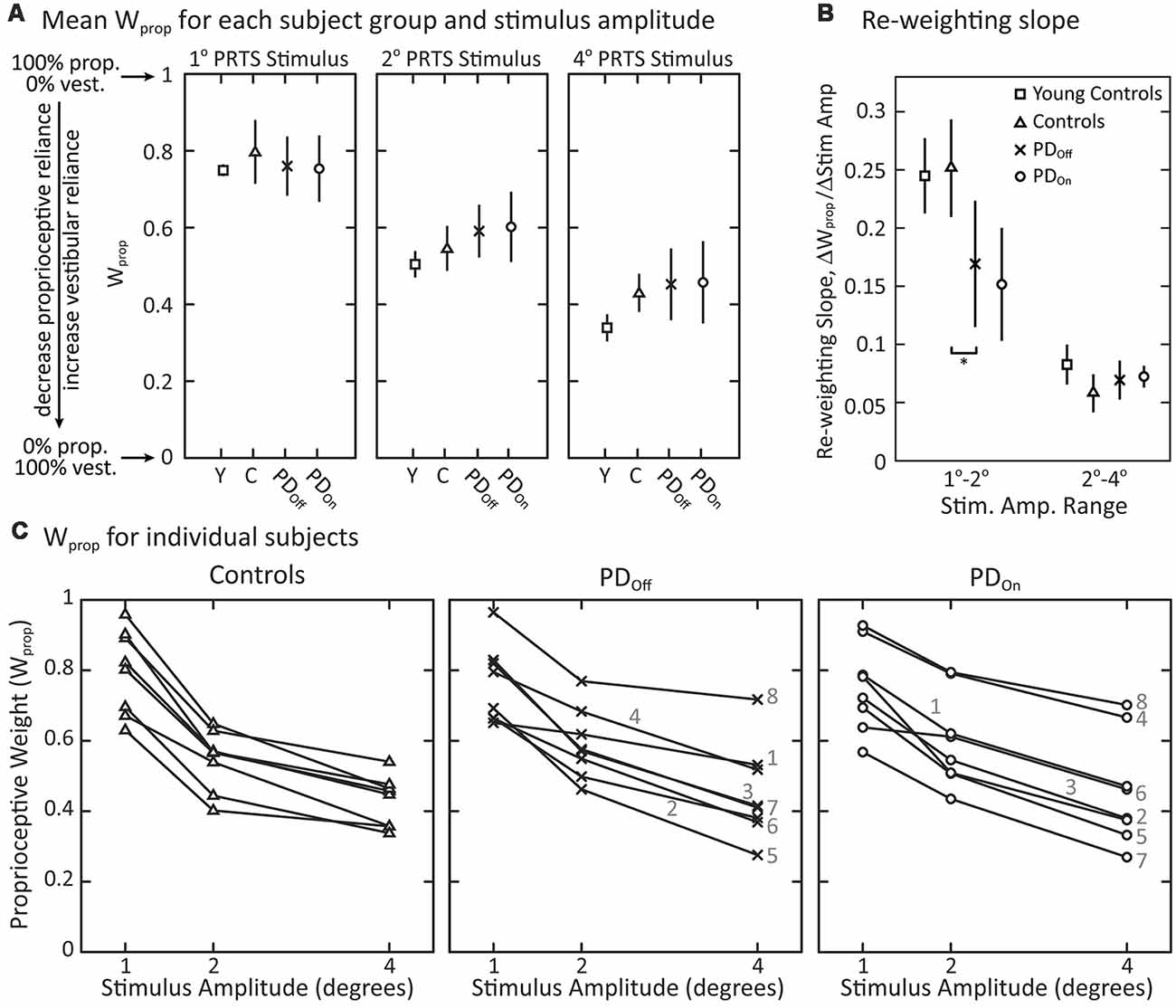
Figure 6. Proprioceptive weighting in response to surface-stimuli with eyes closed. (A) Sensory weighting across subject groups and stimulus amplitudes. Data are shown for young controls (Y: N = 4; age range 28–47 years), older controls (C: N = 8; age range 57–77 years), and subjects with PD off (PDOff: N = 8) and on (PDOn: N = 8) medication. A proprioceptive weight of 1 indicates 100% reliance on proprioceptive information, while a weight of 0 indicates 100% reliance on vestibular information. The symbols represent mean behavior for each group, and the error bars denote the 95% confidence interval of the mean. (B) Slope factor representing the normalized change in proprioceptive weights between 1° and 2° surface-stimuli and between 2° and 4° surface-stimuli for each subject group. All changes in Wprop are positive, indicating a decrease in Wprop for increasing stimulus amplitude. The error bars denote the 95% confidence intervals of the mean and “*” indicates a significant difference between subjects with PD off medication and age-matched controls. Young controls were not included in statistical tests. (C) Sensory weighting in individual subjects in response to surface stimuli for controls, and subjects with PD off medication and on medication. Each data point represents a single subject at the given stimulus amplitude. Lines connect the data points for an individual subject across stimulus amplitudes. For subjects with PD, the numbers 1–8 correspond to the subject identifiers in Table 1 (e.g., PD1 denoted as 1). All subject groups showed significant decreases in Wprop with increasing stimulus amplitude.
Viewing the changing Wprop values of individual subjects across stimulus amplitude revealed some qualitative differences between subjects with PD and age-matched control subjects (Figure 6C). There was more inter-subject variability in Wprop in subjects with PD than in control subjects in response to the 4° surface-stimulus amplitude (Figure 6C). This inter-subject variability in the PD groups was due, in part, to the large proprioceptive weights for a single PDOff subject (PD8) and two PDOn subjects (PD4, PD8) across all stimulus amplitudes. In fact, when off medication, PD8 weighted proprioception more than any control subject for the 2° and 4° surface-stimulus amplitudes. In the on medication condition, PD4 and PD8 had a larger Wprop than any controls for the 2° and 4° surface-stimuli. Medication noticeably changed Wprop in two of the eight subjects with PD (Figure 6C); Wprop increased in PD4 and decreased in PD7 across all stimulus amplitudes when on vs. off medication. Thus, large changes in Wprop occurred with medication in some individual subjects, but the direction of change was not systematic. In addition, three (PD1, PD4, PD8) of the four PDOff subjects who fell on all attempts at sway-referenced trials had larger Wprop in the 2° and 4° surface-stimulus trials than the PDOff subjects that did not fall. One of the three PDOn subjects (PD8) who fell on all attempts at sway-referenced trials also had larger proprioceptive weights for all surface-stimulus trials than PDOn subjects who did not fall.
Additional differences between control and individual subjects with PD were related to how well they changed proprioceptive weighting between surface-stimulus amplitudes (Figure 6C). To quantify this difference in Wprop between control and subjects with PD, we computed the slope of the proprioceptive weights between successive surface-stimulus amplitudes. PDOff subjects’ slope of proprioceptive weighting was less than controls between 1° and 2° surface-stimulus amplitudes (Figure 6B; p = 0.038). However, there was no difference between PDOff and control subjects in the slope of proprioceptive weighting between 2° and 4° surface-stimulus amplitudes (p = 0.51).
Group Response to Visual-Stimuli
In response to visual-stimuli, both PD and age-matched control subjects had decreasing FRF gains with increasing stimulus amplitude (Figure 7, top row). For a given stimulus amplitude, the gain in response to a visual stimulus was lower than the gain in response to a surface stimulus (compare Figures 5, Figure 7). In contrast to responses to surface stimuli, the phase curves in response to visual stimuli were similar across stimulus amplitude for all frequencies (Figure 7, middle row). Individual subject’s responses to visual stimuli were small and variable. Consistent with this low gain and high variability, the coherence of the experimental data was consistently low for individual subjects (not shown) and the mean coherence across subjects (Figure 7, bottom row). Therefore, we did not consider statistics for individual subject responses to visual stimuli, and conclusions were based on a qualitative assessment of the average response across subjects.
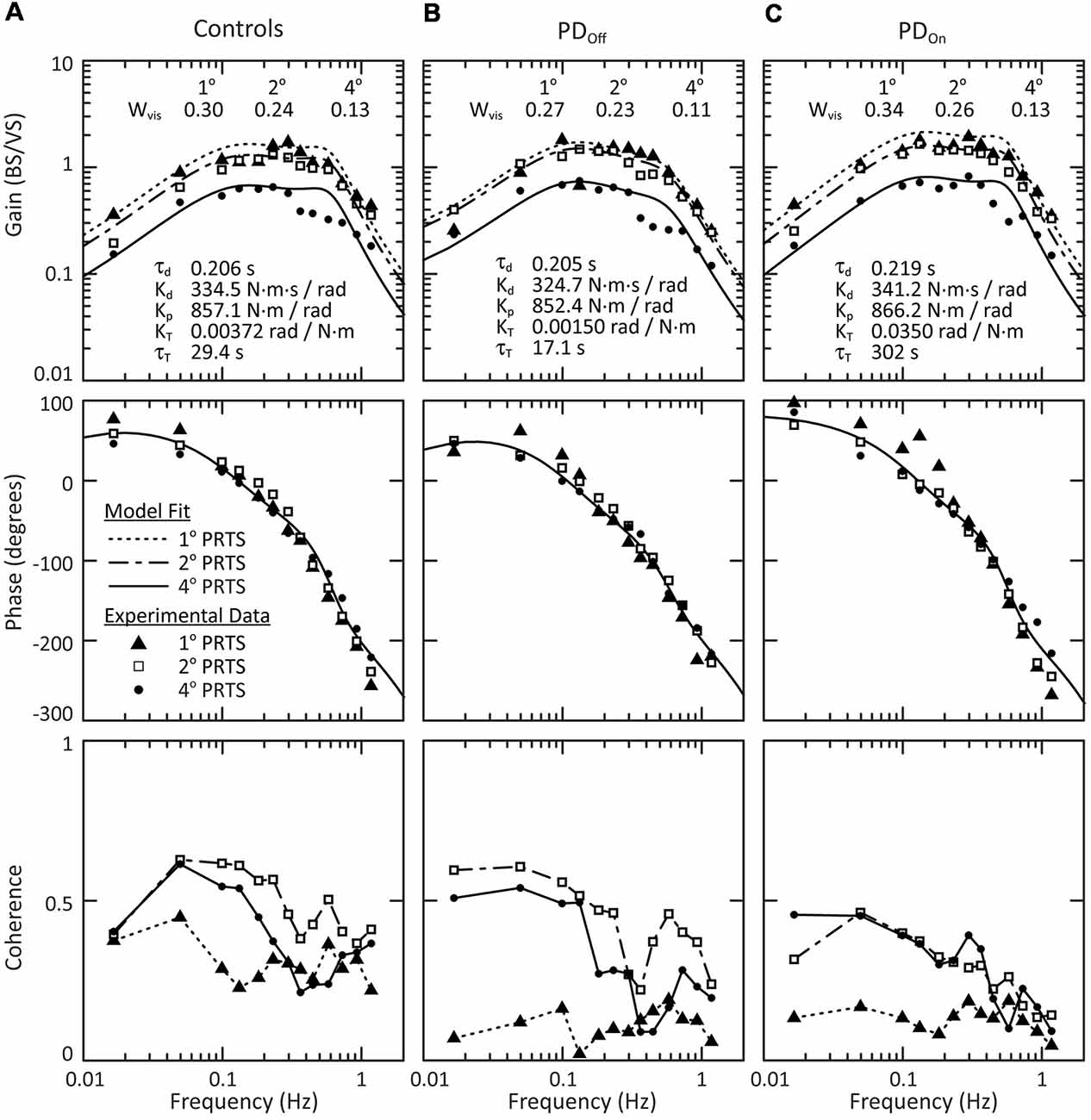
Figure 7. Mean behavior across subjects in response to visual stimuli. Gain, phase and coherence plots for (A) control subjects, (B) subjects with PD off medication, and (C) subjects with PD on medication. The data points denote experimental data for the mean behavior across subject and the curves on the gain and phase plots are the model fits to this mean behavior. The parameters of the model fits are listed on the gain plots. Data for all three stimulus amplitudes were fit simultaneously, with a Wvis for each stimulus amplitude and a single τd, Kd, Kp, KT, and τT across all stimulus amplitudes. The lines connecting data points on the coherence plots (bottom row) are for visualization and are not related to the model fits.
All groups showed a mean tendency to decrease reliance on visual information with increasing visual-stimulus amplitude, as indicated by a monotonic decrease in Wvis with increasing visual-stimulus amplitude (Figure 8). Neither PD nor levodopa influenced visual weighting in response to visual stimuli (Figure 8).
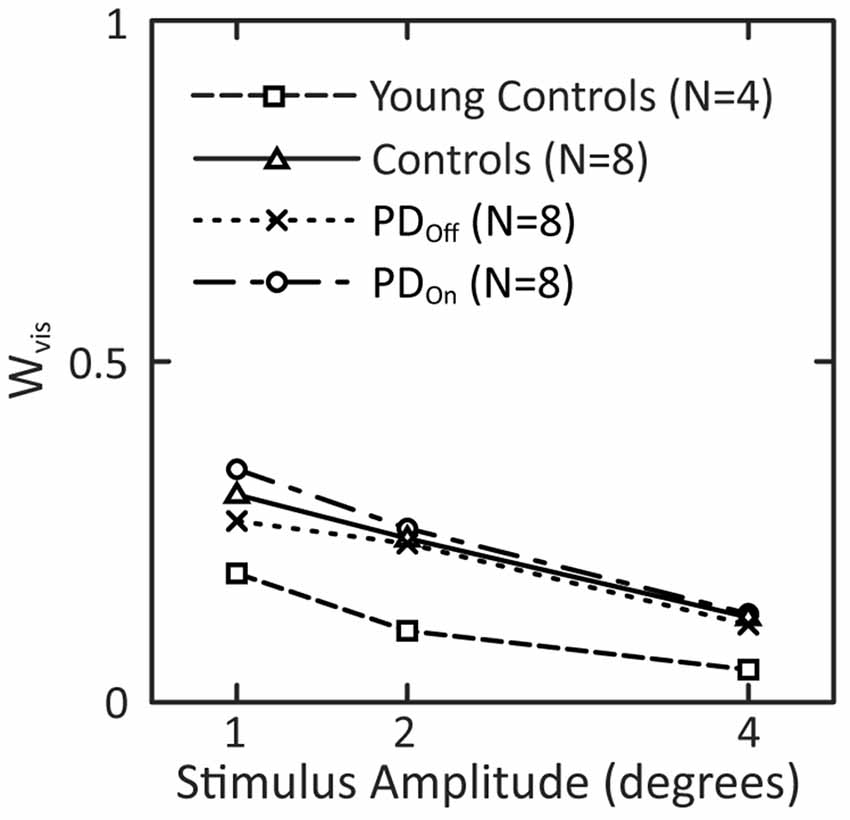
Figure 8. Visual weights in response to visual-stimuli of different stimulus amplitudes. Data for subjects with PD and age-matched controls are taken from the model fits to the mean FRF data in Figure 7. Visual weights from fits to mean FRF data from four young controls are added to this plot.
Effect of Aging on Sensory Weighting
There was a tendency for our age-matched control subjects to have larger mean proprioceptive weights than younger controls (data from Peterka, 2002) in response to each surface stimulus (Figure 6A). On average across surface-stimulus amplitudes, Wprop for older controls was 1.1 times the Wprop for younger controls. The difference in the mean Wprop between older and younger control subjects was greatest for the 4° surface-stimulus amplitude. In addition, subjects with PD had larger proprioceptive weights than younger subjects for the 2° and 4° stimulus amplitudes (Figure 6A). The difference in proprioceptive weighting between 1° and 2° surface stimuli were similar for age-matched and younger control subjects, and both control groups had noticeably larger slopes than subjects with PD (Figure 6B).
The mean visual weight, Wvis, at each individual amplitude was smaller for young control subjects than either PD or age-matched control subjects (Figure 8). Wvis for older controls was on average 2.2 times larger than the Wvis for the younger controls. In addition, Wvis for PDOn and PDOff subjects was on average 2.0 and 2.3 times, respectively, larger than Wvis for younger controls.
Discussion
Despite profound balance and motor control deficits, our results demonstrate that subjects with PD can re-weight proprioceptive, visual, and vestibular information for postural control when sensory conditions change. Each PD and age-matched control subject decreased reliance on proprioceptive information as the surface-stimulus amplitude increased (Figures 6A,C). However, subjects with PD did not change proprioceptive weights as much as control subjects between the smallest surface-stimulus amplitudes (Figure 6B).
To appreciate the functional significance of the relatively small differences in sensory weighting between subjects with PD and controls, comparisons can be made to sensory weight changes associated with other neurological deficits. Specifically, sensory weights have been measured in subjects with bilateral (Figure 10 in Peterka, 2002) and unilateral vestibular loss (Figure 4 in Peterka et al., 2011) using similar methods. Bilateral vestibular loss subjects are 100% reliant on proprioception (Wprop = 1) and are unable to change weight when amplitudes of surface stimuli change on tests performed with eyes closed. Bilateral vestibular loss subjects were also unable to change visual weights with changing visual stimulus amplitude. Unilateral vestibular loss subjects were able to decrease Wprop with increasing surface amplitude, but their Wprop values were larger than age-matched controls by an average value of 0.28. Additionally, there was very little overlap in the distributons of Wprop values from unilateral loss and control subjects. These results in vestibular deficient subjects are in contrast to the substantial overlap of Wprop values in subjects with PD and controls at all stimulus amplitudes (Figure 6C) and emphasize the minimal effect of PD on sensory weighting and re-weighting.
The subjects in our study would be considered at increased risk for falls compared to age-matched controls given that they had moderate to severe PD (UPDRS Motor score 20–63), were diagnosed from 3 to 36 years ago, were dependent upon levodopa, and seven out of eight showed clinically apparent balance and gait problems. The moderately-to-severely affected patients in our study showed larger spontaneous sway in the mediolateral (ML) direction, especially when taking their levodopa medication, consistent with previous studies (Rocchi et al., 2002) and possibly related to dyskinesia (Chung et al., 2010; Curtze et al., 2015). In addition, fewer of our subjects with PD than age-matched controls were able to stand unsupported on a sway-referenced surface with eyes closed, especially when off levodopa, which is similar to other studies (Bronte-Stewart et al., 2002).
Other studies have shown that subjects with PD as severe as those in our study, have significant impairments in automatic postural responses, postural instability during gait, and in anticipatory postural adjustments (Horak et al., 1996; Rocchi et al., 2006b; Tagliabue et al., 2009). Despite including subjects with PD with a range of disease severity, we did not see any significant correlations between disease severity and impairments in sensory weighting. However, there was some indication that disease severity may be related to sensory weighting in that the two subjects with PD (PD4 and PD8) who had the disease the longest and had among the worst clinical PIGD scores in the UPDRS (Table 1) also relied more on proprioception than the other subjects with PD when on or off medication (Figure 6C). Future studies with larger sample sizes would be necessary to investigate this possible relatationship of severity and sensory weighting with other relevant outcome measures such as incidence of falls.
Sensory Integration and Sensory Transitions
Our results demonstrate that subjects with PD are capable of re-weighting sensory information for postural control. This is consistent with a previous study concluding that subjects with PD can integrate sensory information to successfully perform a turning task before and after walking on a circular treadmill (Earhart et al., 2007). However, previous findings from our laboratory show that subjects with PD take more trials than control subjects to switch postural synergies when sensory conditions change (Horak et al., 1992; Chong et al., 1999b,c, 2000). For example, subjects with PD do not immediately inhibit ankle muscles when holding a handle or sitting on a stool during surface perturbations (Schieppati and Nardone, 1991; Horak et al., 1992, 1996). In addition, on the first trial of each of the sensory organization tests, subjects with PD fall more often than controls (Chong et al., 1999a). However, subjects with PD improve with repeated exposure to each sensory condition, such that by the third trial of a particular sensory condition, subjects with PD reach near control levels (Chong et al., 1999a). The third trial of each sensory condition is most similar to the steady-state conditions of our experiment. Therefore, this previous result is consistent with the mostly appropriate steady-state performance of our subjects with PD when compared to age-matched controls.
In response to changing surface-stimulus amplitudes in the absence of vision, our subjects with PD demonstrated an ability to re-weight proprioceptive information in a similar manner as age-matched controls. This result indicates that vision is not required for subjects with PD to generate appropriate steady-state postural responses. On the contrary, previous studies suggest that subjects with PD are more visually-dependent than age-matched controls, especially when visual information is misleading (Bronstein et al., 1990). For example, subjects with PD consistently undershoot voluntary arm movements and involuntary postural stepping responses when they cannot see their limbs (Jacobs and Horak, 2006; Tagliabue et al., 2009). It has also been shown that body sway is more driven by sinusoidally moving visual surrounds in subjects with PD than age-matched controls (Maurer et al., 2003). Although our subjects with PD showed slightly greater sway than our age-matched controls (Figure 3C), both groups showed sway increases with increasing visual-stimulus amplitude. These results differ qualitatively from the Maurer study in that their control subjects showed essentially no increase in sway with increasing visual-stimulus amplitude and showed significantly less sway than their subjects with PD. The difference between our results and the Maurer study may be due to the unusually young ages of their subjects with PD and hence the ages of their age-matched controls (48 years mean age of both groups). Consistent with age accounting for increased sensitivity to visual stimuli are the results in Figure 8 showing that Wvis measures for young controls are about half the value of Wvis for our age-matched controls. That is, normal aging may result in a shift toward increased reliance on vision for balance; early onset PD may accelerate the shift, but older subjects show a similar reliance on vision independent of disease state.
Furthermore, some of our subjects with PD performed poorly on sway-referenced surface trials with eyes closed. In these trials, subjects must rely on vestibular information, as vision is absent and the sway-referenced surface minimizes proprioceptive cues. However, vestibular function has been shown to be normal in PD (Pastor et al., 1993). In fact, it has been shown that subjects with PD with deep brain stimulation weight proprioceptive information less and over-weight vestibular sense for postural control compared to age-matched controls (Maurer, 2009). Thus, it is unlikely that either vestibular dysfunction or an inability to use vestibular information for postural control account for the poor performance in subjects with PD on eyes closed sway-referenced trials. Consistent with our sensory re-weighting results, we suggest that most subjects with PD have normal vestibular function and are able to utilize it if given enough time to adjust to altered sensory conditions. The cause of poor performance on surface sway-referencing is likely a reduced ability of subjects with PD to quickly re-weight toward increased reliance on vestibular cues at the start of each test. Poor performance on eyes-closed sway-referenced trials is also observed in older subjects without PD and with normal vestibular function (Peterka and Black, 1990).
Re-weighting Sensitivity
Although subjects with PD were able to re-weight away from proprioception as surface-stimulus amplitude increased (Figures 6A,C), the difference in proprioceptive weights between the two smallest amplitudes of surface-stimuli was smaller in subjects with PD than age-matched controls but was the same for the two largest amplitudes (Figure 6B). This result is consistent with previous studies showing that subjects with PD have a higher threshold for perceiving the amplitude of proprioceptive stimuli (i.e., kinesthesia) than age-matched controls (Konczak et al., 2009), including an impaired ability to consciously perceive limb position (Maschke et al., 2003) or axial position (Wright et al., 2010). In addition, previous studies have demonstrated an increased neural synchrony in the basal ganglia circuitry with PD (Levy et al., 2000; Raz et al., 2001; Goldberg et al., 2004), suggesting a decrease in the signal-to-noise ratio of neural activity in the basal ganglia as dopamine levels decrease (Bergman et al., 1998; Bevan et al., 2002; Bar-Gad et al., 2003; Hammond et al., 2007). The higher threshold for detecting changes between the smallest proprioceptive stimuli that we observed in our study could be related to a decreased signal-to-noise ratio in the processing of sensory signals by dopaminergic circuitry.
Stiffness in PD
The parameter Kp, identified in model curve fits to FRF data (Figures 5, 7), characterizes the amount of corrective torque generated per unit of body sway and, thus, can be considered to quantify the stiffness of the postural control system (Latash and Zatsiorsky, 1993). That is, if one were to apply a perturbing torque of a given amplitude to subjects of equal body dimensions, a subject with a larger Kp would have a smaller sway amplitude than a subject with a smaller Kp. Because rigidity is considered to be a hallmark of PD it may appear unexpected that Kp did not differ between subjects with PD and controls, and medication had no effect on Kp in subjects with PD.
However, in interpreting the meaning of stiffness and rigidity it is important to consider the context of the task that the subject is asked to perform, because the motor actions required for some tasks are highly constrained while motor actions for other tasks are not constrained. For example, when arm rigidity is tested, as in a UPDRS measurement of rigidity, control subjects allow free movement of their arms and low rigidity is observed, while subjects with PD resist movement of their arm, and high rigidity is observed. Similarly, tests show that subjects with PD off levodopa medication have increased axial rigidity when compared to controls, and levodopa does not significantly reduce axial rigidity (Wright et al., 2007). In both arm movement and trunk twisting tasks there are no functionally detrimental consequences if subjects allow arm or axial trunk movements in response to a perturbing force. That is, these tasks are not fundamentally constrained. Control subjects are apparently able to recognize the context of these situations and naturally allow free movement whereas subjects with PD are unable to adjust to the context.
In contrast, the task of maintaining upright stance places constraints on motor control. Both subjects with PD and controls must maintain a minimal Kp in order to resist the destabilizing torque due to gravity. Therefore, control subjects do not have an option of choosing a postural stiffness that is much less than that of subjects with PD. The upper limit of Kp is also constrained because large Kp values also produce instability (Masani et al., 2008). Therefore, only limited differences in Kp between subjects with PD and controls are possible. Furthermore, the very close correspondence between Kp values in subjects with PD and controls suggests that subjects with PD were able to regulate stiffness under steady-state conditions as well as control subjects to achieve dynamic control of upright stance (Peterka and Loughlin, 2004).
Aging and Sensory Weighting
In response to a surface and visual stimuli, subjects with PD and age-matched controls show greater use of proprioceptive (Figure 6A) and visual (Figure 8) information (corresponding to larger Wprop and Wvis measures, respectively) compared to younger control subjects from a previous study (Peterka, 2002). For surface stimuli, greater sensitivity has been confirmed in more recent studies comparing younger and older adults with normal balance function (Cenciarini et al., 2010; Wiesmeier et al., 2015). These results indicate that subject age, not PD, determined the extent to which subjects utilized visual and proprioceptive information for postural control.
Effects of Dopamine Replacement
Levodopa improved clinical indicators of balance and gait, as well as rigidity, bradykinesia and tremor, as measured by the UPDRS. However, consistent with the literature, we demonstrated that levodopa increases CoP displacement in the mediolateral direction during quiet stance, consistent with increased risk for falling (Mitchell et al., 1995; Rocchi et al., 2002). Previously, we showed that automatic postural responses to transient perturbations were further reduced by levodopa medication (Horak et al., 1996). Our current results indicate that levodopa neither improves nor impairs sensory weighting in PD patients in conditions where sufficient time is allotted to achieve steady-state behavior.
Clinical Implications
Despite larger than normal postural sway during quiet stance and during larger sensory stimuli, our results show that subjects with PD do have the ability to change reliance on sensory information for postural control, given enough time to switch between tasks. This result does not conflict with our previous results showing that subjects with PD have difficulty switching quickly between different task demands (Horak et al., 1992; Chong et al., 1999b,c, 2000). Rather, it may be necessary for subjects with PD to transition slowly between tasks to avoid falls in the transition periods. In other words, postural instability in subjects with PD may be specific to the transition period. Consistent with this notion, we recently demonstrated that postural instability during walking in subjects with PD was specific to the transition period during heel-strike (Fino et al., 2018). If subjects with PD can ease through transition periods, they may be able to participate in activities that appear to challenge their postural stability (e.g., walking on a sandy beach). Conversely, if subjects with PD are required to produce a postural response during their transition period, they will likely demonstrate impaired postural responses that potentially increase the risk of falling. In addition, tasks requiring central processing of relatively small changes in sensory signals, such as walking from a firm to a more compliant surface, may be more affected by the loss of dopamine neurons, due to a decreased signal-to-noise ratio in the neural processing of sensory information and/or sensory integration signals. Consequently, patients may have trouble with tasks involving smaller changes in sensory conditions, but be successful at tasks in which there are larger changes in sensory conditions.
Ethics Statement
The Institutional Review Board at Oregon Health and Science University (OHSU) approved the protocol for this experiment, and all subjects gave written informed consent prior to participating.
Author Contributions
KF contributed to the analysis, interpretation of the results, and preparation of the manuscript. RP and FH both contributed to the research funding and resources, conception, experimental design, data collection, analysis and interpretation of the results, and editing of the manuscript.
Funding
This research was supported by NIH grants R37-AG006457 and T32-NS045553.
Conflict of Interest Statement
The authors declare that the research was conducted in the absence of any commercial or financial relationships that could be construed as a potential conflict of interest.
Acknowledgments
We thank the Parkinson’s Center of Oregon for referring subjects to participate in this study. We also thank K. Statler and T. Nagel for collecting data, P. Carlson-Kuhta and J. Jacobs for administering the UPDRS, and S. Oster for editorial assistance.
Abbreviations
CoM, center of mass; CoP, center of pressure; FRF, frequency response function; J, body moment of inertia about ankle joints; Kd, neural control damping constant; Kp, neural control stiffness constant; KT, torque feedback gain; mgh, body mass times gravity constant times height of CoM above ankle joint; PD, Parkinson’s disease; PDOff, Parkinson’s disease subjects off medication; PDOn, Parkinson’s disease subjects on medication; PIGD, postural instability and gait disorders; RMS, root-mean-square; τd, neural control time delay; τT, torque feedback time-constant; UPDRS, Unified Parkinson’s Disease Rating Scale; Wprop, proprioceptive weight; Wvest, vestibular weight; Wvis, visual weight.
References
Abbruzzese, G., and Berardelli, A. (2003). Sensorimotor integration in movement disorders. Mov. Disord. 18, 231–240. doi: 10.1002/mds.10327
Agid, Y. (1991). Parkinson’s disease: pathophysiology. Lancet 337, 1321–1324. doi: 10.1016/0140-6736(91)92989-F
Assländer, L., and Peterka, R. J. (2016). Sensory reweighting dynamics following removal and addition of visual and proprioceptive cues. J. Neurophysiol. 116, 272–285. doi: 10.1152/jn.01145.2015
Bar-Gad, I., Morris, G., and Bergman, H. (2003). Information processing, dimensionality reduction and reinforcement learning in the basal ganglia. Prog. Neurobiol. 71, 439–473. doi: 10.1016/j.pneurobio.2003.12.001
Baston, C., Mancini, M., Rocchi, L., and Horak, F. (2016). Effects of levodopa on postural strategies in Parkinson’s disease. Gait Posture 46, 26–29. doi: 10.1016/j.gaitpost.2016.02.009
Bergman, H., Feingold, A., Nini, A., Raz, A., Slovin, H., Abeles, M., et al. (1998). Physiological aspects of information processing in the basal ganglia of normal and parkinsonian primates. Trends Neurosci. 21, 32–38. doi: 10.1016/s0166-2236(97)01151-x
Bevan, M. D., Magill, P. J., Terman, D., Bolam, J. P., and Wilson, C. J. (2002). Move to the rhythm: oscillations in the subthalamic nucleus-external globus pallidus network. Trends Neurosci. 25, 525–531. doi: 10.1016/s0166-2236(02)02235-x
Black, F. O., Shupert, C. L., Horak, F. B., and Nashner, L. M. (1988). Abnormal postural control associated with peripheral vestibular disorders. Prog. Brain Res. 76, 263–275. doi: 10.1016/s0079-6123(08)64513-6
Bloem, B. R., van Vugt, J. P., and Beckley, D. J. (2001). Postural instability and falls in Parkinson’s disease. Adv. Neurol. 87, 209–223.
Brenière, Y. (1996). Why we walk the way we do. J. Mot. Behav. 28, 291–298. doi: 10.1080/00222895.1996.10544598
Bronstein, A. M., Hood, J. D., Gresty, M. A., and Panagi, C. (1990). Visual control of balance in cerebellar and parkinsonian syndromes. Brain 113, 767–779. doi: 10.1093/brain/113.3.767
Bronstein, A. M., Yardley, L., Moore, A. P., and Cleeves, L. (1996). Visually and posturally mediated tilt illusion in Parkinson’s disease and in labyrinthine defective subjects. Neurology 47, 651–656. doi: 10.1212/wnl.47.3.651
Bronte-Stewart, H. M., Minn, A. Y., Rodrigues, K., Buckley, E. L., and Nashner, L. M. (2002). Postural instability in idiopathic Parkinson’s disease: the role of medication and unilateral pallidotomy. Brain 125, 2100–2114. doi: 10.1093/brain/awf207
Brown, L. A., Cooper, S. A., Doan, J. B., Dickin, D. C., Whishaw, I. Q., Pellis, S. M., et al. (2006). Parkinsonian deficits in sensory integration for postural control: temporal response to changes in visual input. Parkinsonism Relat. Disord. 12, 376–381. doi: 10.1016/j.parkreldis.2006.03.004
Burleigh-Jacobs, A., Horak, F. B., Nutt, J. G., and Obeso, J. A. (1997). Step initiation in Parkinson’s disease: influence of levodopa and external sensory triggers. Mov. Disord. 12, 206–215. doi: 10.1002/mds.870120211
Cenciarini, M., Loughlin, P. J., Sparto, P. J., and Redfern, M. S. (2010). Stiffness and damping in postural control increase with age. IEEE Trans. Biomed. Eng. 57, 267–275. doi: 10.1109/tbme.2009.2031874
Cenciarini, M., and Peterka, R. J. (2006). Stimulus-dependent changes in the vestibular contribution to human postural control. J. Neurophysiol. 95, 2733–2750. doi: 10.1152/jn.00856.2004
Chong, R. K., Horak, F. B., Frank, J., and Kaye, J. (1999a). Sensory organization for balance: specific deficits in Alzheimer’s but not in Parkinson’s disease. J. Gerontol. A Biol. Sci. Med. Sci. 54, M122–M128. doi: 10.1093/gerona/54.3.m122
Chong, R. K., Horak, F. B., and Woollacott, M. H. (1999b). Time-dependent influence of sensorimotor set on automatic responses in perturbed stance. Exp. Brain Res. 124, 513–519. doi: 10.1007/s002210050647
Chong, R. K., Jones, C. L., and Horak, F. B. (1999c). Postural set for balance control is normal in Alzheimer’s but not in Parkinson’s disease. J. Gerontol. A Biol. Sci. Med. Sci. 54, M129–M135. doi: 10.1093/gerona/54.3.m129
Chong, R. K., Horak, F. B., and Woollacott, M. H. (2000). Parkinson’s disease impairs the ability to change set quickly. J. Neurol. Sci. 175, 57–70. doi: 10.1016/s0022-510x(00)00277-x
Chung, K. A., Lobb, B. M., Nutt, J. G., McNames, J., and Horak, F. (2010). Objective measurement of dyskinesia in Parkinson’s disease using a force plate. Mov. Disord. 25, 602–608. doi: 10.1002/mds.22856
Cruz, C. F., Peionte, M. E. P., Okai-Nobrega, L. A., Okamoto, E., Fortaleza, A. C. S., Mancini, M., et al. (2018). Parkinson’s disease does not alter automatic visual-motor coupling in postural control. Neurosci. Lett. 686, 47–52. doi: 10.1016/j.neulet.2018.08.050
Curtze, C., Nutt, J. G., Carlson-Kuhta, P., Mancini, M., and Horak, F. B. (2015). Levodopa is a double-edged sword for balance and gain in people with Parkinson’s disease. Mov. Disord. 30, 1361–1370. doi: 10.1002/mds.26269
Davies, W. D. T. (1970). System Identification for Self-Adaptive Control. London, UK: Wiley-Interscience.
De Nunzio, A. M., Nardone, A., and Schieppati, M. (2007). The control of equilibrium in Parkinson’s disease patients: delayed adaptation of balancing strategy to shifts in sensory set during a dynamic task. Brain Res. Bull. 74, 258–270. doi: 10.1016/j.brainresbull.2007.06.020
Earhart, G. M., Stevens, E. S., Perlmutter, J. S., and Hong, M. (2007). Perception of active and passive turning in Parkinson disease. Neurorehabil. Neural Repair 21, 116–122. doi: 10.1177/1545968306290674
Fasano, A., Canning, C. G., Hausdorff, J. M., Lord, S., and Rochester, L. (2017). Falls in Parkinson’s disease: a complex and evolving picture. Mov. Disord. 32, 1524–1536. doi: 10.1002/mds.27195
Fino, P. C., Mancini, M., Curtze, C., Nutt, J. G., and Horak, F. B. (2018). Gait stability has phase-dependent dual-task costs in Parkinson’s disease. Front. Neurol. 9:373. doi: 10.3389/fneur.2018.00373
Frenklach, A., Louie, S., Koop, M. M., and Bronte-Stewart, H. (2009). Excessive postural sway and the risk of falls at different stages of Parkinson’s disease. Mov. Disord. 24, 377–385. doi: 10.1002/mds.22358
Goldberg, J. A., Rokni, U., Boraud, T., Vaadia, E., and Bergman, H. (2004). Spike synchronization in the cortex/basal-ganglia networks of Parkinsonian primates reflects global dynamics of the local field potentials. J. Neurosci. 24, 6003–6010. doi: 10.1523/JNEUROSCI.4848-03.2004
Hammond, C., Bergman, H., and Brown, P. (2007). Pathological synchronization in Parkinson’s disease: networks, models and treatments. Trends Neurosci. 30, 357–364. doi: 10.1016/j.tins.2007.05.004
Horak, F. B. (1987). Clinical measurement of postural control in adults. Phys. Ther. 67, 1881–1885. doi: 10.1093/ptj/67.12.1881
Horak, F. B., Frank, J., and Nutt, J. (1996). Effects of dopamine on postural control in parkinsonian subjects: scaling, set, and tone. J. Neurophysiol. 75, 2380–2396. doi: 10.1152/jn.1996.75.6.2380
Horak, F. B., Nutt, J. G., and Nashner, L. M. (1992). Postural inflexibility in parkinsonian subjects. J. Neurol. Sci. 111, 46–58. doi: 10.1016/0022-510x(92)90111-w
Jacobs, J. V., and Horak, F. B. (2006). Abnormal proprioceptive-motor integration contributes to hypometric postural responses of subjects with Parkinson’s disease. Neuroscience 141, 999–1009. doi: 10.1016/j.neuroscience.2006.04.014
Jeka, J. J., Oie, K. S., and Kiemel, T. (2008). Asymmetric adaptation with functional advantage in human sensorimotor control. Exp. Brain Res. 191, 453–463. doi: 10.1007/s00221-008-1539-x
Konczak, J., Corcos, D. M., Horak, F., Poizner, H., Shapiro, M., Tuite, P., et al. (2009). Proprioception and motor control in Parkinson’s disease. J. Mot. Behav. 41, 543–552. doi: 10.3200/35-09-002
Latash, M. L., and Zatsiorsky, V. M. (1993). Joint stiffness: myth or reality? Hum. Mov. Sci. 12, 653–692. doi: 10.1016/0167-9457(93)90010-m
Levy, R., Hutchison, W. D., Lozano, A. M., and Dostrovsky, J. O. (2000). High-frequency synchronization of neuronal activity in the subthalamic nucleus of parkinsonian patients with limb tremor. J. Neurosci. 20, 7766–7775. doi: 10.1523/jneurosci.20-20-07766.2000
Mancini, M., and Horak, F. B. (2010). The relevance of clinical balance assessment tools to differentiate balance deficits. Eur. J. Phys. Rehabil. Med. 46, 239–248.
Mancini, M., Horak, F. B., Zampieri, C., Carlson-Kuhta, P., Nutt, J., and Chiari, L. (2011). Trunk accelerometry reveals postural instability in untreated Parkinson’s disease. Parkinsonism Relat. Disord. 17, 557–562. doi: 10.1016/j.parkreldis.2011.05.010
Masani, K., Vette, A. H., Kawashima, N., and Popovic, M. R. (2008). Neuromusculoskeletal torque-generation process has a large destabilizing effect on the control mechanism of quiet standing. J. Neurophysiol. 100, 1465–1475. doi: 10.1152/jn.00801.2007
Maschke, M., Gomez, C. M., Tuite, P. J., and Konczak, J. (2003). Dysfunction of the basal ganglia, but not the cerebellum, impairs kinaesthesia. Brain 126, 2312–2322. doi: 10.1093/brain/awg230
Matsuda, K., Suzuki, Y., Yoshikawa, N., Yamamoto, T., Kiyono, K., Tanahashi, T., et al. (2016). Postural flexibility during quiet standing in healthy elderly and patients with Parkinson’s disease. Conf. Proc. IEEE Eng. Med. Biol. Soc. 2016, 29–32. doi: 10.1109/embc.2016.7590632
Maurer, C. (2009). Postural deficits in Parkinson’s disease are caused by insufficient feedback motor error correction and by deficits in sensory reweighting. Clin. Neurophysiol. 120:e82. doi: 10.1016/j.clinph.2008.07.198
Maurer, C., Mergner, T., Xie, J., Faist, M., Pollak, P., and Lücking, C. H. (2003). Effect of chronic bilateral subthalamic nucleus (STN) stimulation on postural control in Parkinson’s disease. Brain 126, 1146–1163. doi: 10.1093/brain/awg100
Mitchell, S. L., Collins, J. J., DeLuca, C. J., Burrows, A., and Lipsitz, L. A. (1995). Open-loop and closed-loop postural control mechanisms in Parkinson’s disease: increased mediolateral activity during quiet standing. Neuosci. Lett. 197, 133–136. doi: 10.1016/0304-3940(95)11924-l
Mongeon, D., Blanchet, P., and Messier, J. (2009). Impact of Parkinson’s disease and dopaminergic medication on proprioceptive processing. Neuroscience 158, 426–440. doi: 10.1016/j.neuroscience.2008.10.013
Nagy, A., Eördegh, G., Parüczy, Z., Márkus, Z., and Benedek, G. (2006). Multisensory integration in the basal ganglia. Eur. J. Neurosci. 24, 917–924. doi: 10.1111/j.1460-9568.2006.04942.x
Nardone, A., and Schieppati, M. (2006). Balance in Parkinson’s disease under static and dynamic conditions. Mov. Disord. 21, 1515–1520. doi: 10.1002/mds.21015
Nutt, J. G., Burchiel, K. J., Comella, C. L., Jankovic, J., Lang, A. E., Laws, E. R., et al. (2003). Randomized, double-blind trial of glial cell line-derived neurotrophic factor (GDNF) in PD. Neurology 60, 69–73. doi: 10.1212/wnl.60.1.69
O’Suilleabhain, P., Bullard, J., and Dewey, R. B. (2001). Proprioception in Parkinson’s disease is acutely depressed by dopaminergic medications. J. Neurol. Neurosurg. Psychiatry 71, 607–610. doi: 10.1136/jnnp.71.5.607
Ozinga, S. J., Koop, M. M., Linder, S. M., Machado, A. G., Dey, T., and Alberts, J. L. (2017). Three-dimensional evaluation of postural stability in Parkinson’s disease with mobile technology. NeuroRehabilitation 41, 211–218. doi: 10.3233/nre-171473
Pastor, M. A., Day, B. L., and Marsden, C. D. (1993). Vestibular induced postural responses in Parkinson’s disease. Brain 116, 1177–1190. doi: 10.1093/brain/116.5.1177
Peterka, R. J. (2002). Sensorimotor integration in human postural control. J. Neurophysiol. 88, 1097–1118. doi: 10.1152/jn.2002.88.3.1097
Peterka, R. J. (2003). Simplifying the complexities of maintaining balance. IEEE Eng. Med. Biol. Mag. 22, 63–68. doi: 10.1109/memb.2003.1195698
Peterka, R. J., and Black, F. O. (1990). Age-related changes in human postural control: sensory organization tests. J. Vestib. Res. 1, 73–85.
Peterka, R. J., and Loughlin, P. J. (2004). Dynamic regulation of sensorimotor integration in human postural control. J. Neurophysiol. 91, 410–423. doi: 10.1152/jn.00516.2003
Peterka, R. J., Murchison, C. F., Parrington, L., Fino, P. C., and King, L. A. (2018). Implementation of a central sensorimotor integration test for characterizaion of human balance control during stance. Front. Neurol. 9:1045. doi: 10.3389/fneur.2018.01045
Peterka, R. J., Statler, K. D., Wrisley, D. M., and Horak, F. B. (2011). Postural compensation for unilateral vestibular loss. Front. Neurol. 2:57. doi: 10.3389/fneur.2011.00057
Raz, A., Frechter-Mazar, V., Feingold, A., Abeles, M., Vaadia, E., and Bergman, H. (2001). Activity of pallidal and striatal tonically active neurons is correlated in mptp-treated monkeys but not in normal monkeys. J. Neurosci. 21:RC128. doi: 10.1523/JNEUROSCI.21-03-j0006.2001
Rocchi, L., Chiari, L., Cappello, A., and Horak, F. B. (2006a). Identification of distinct characteristics of postural sway in Parkinson’s disease: a feature selection procedure based on principal component analysis. Neurosci. Lett. 394, 140–145. doi: 10.1016/j.neulet.2005.10.020
Rocchi, L., Chiari, L., Mancini, M., Carlson-Kuhta, P., Gross, A., and Horak, F. B. (2006b). Step initiation in Parkinson’s disease: influence of initial stance conditions. Neurosci. Lett. 406, 128–132. doi: 10.1016/j.neulet.2006.07.027
Rocchi, L., Chiari, L., and Horak, F. B. (2002). Effects of deep brain stimulation and levodopa on postural sway in Parkinson’s disease. J. Neurol. Neurosurg. Psychiatry 73, 267–274. doi: 10.1136/jnnp.73.3.267
Schieppati, M., and Nardone, A. (1991). Free and supported stance in Parkinson’s disease. The effect of posture and ‘postural set’ on leg muscle responses to perturbation, and its relation to the severity of the disease. Brain 114, 1227–1244. doi: 10.1093/brain/114.3.1227
Tagliabue, M., Ferrigno, G., and Horak, F. (2009). Effects of Parkinson’s disease on proprioceptive control of posture and reaching while standing. Neuroscience 158, 1206–1214. doi: 10.1016/j.neuroscience.2008.12.007
Wiesmeier, I. K., Dalin, D., and Maurer, C. (2015). Elderly use proprioception rather than visual or vestibular cues for postural motor control. Front. Aging Neurosci. 7:97. doi: 10.3389/fnagi.2015.00097
Winter, D. A., Patla, A. E., Prince, F., Ishac, M., and Gielo-Perczak, K. (1998). Stiffness control of balance in quiet standing. J. Neurophysiol. 80, 1211–1221. doi: 10.1152/jn.1998.80.3.1211
Wright, W. G., Gurfinkel, V. S., King, L. A., Nutt, J. G., Cordo, P. J., and Horak, F. B. (2010). Axial kinesthesia is impaired in Parkinson’s disease: effects of levodopa. Exp. Neurol. 225, 202–209. doi: 10.1016/j.expneurol.2010.06.016
Keywords: basal ganglia, sensory integration, feedback, computational model, balance, Parkinson’s disease
Citation: Feller KJ, Peterka RJ and Horak FB (2019) Sensory Re-weighting for Postural Control in Parkinson’s Disease. Front. Hum. Neurosci. 13:126. doi: 10.3389/fnhum.2019.00126
Received: 18 November 2018; Accepted: 27 March 2019;
Published: 17 April 2019.
Edited by:
Matt J. N. Brown, California State University, Sacramento, United StatesReviewed by:
Rahul Goel, Baylor College of Medicine, United StatesArata Horii, Niigata University, Japan
Copyright © 2019 Feller, Peterka and Horak. This is an open-access article distributed under the terms of the Creative Commons Attribution License (CC BY). The use, distribution or reproduction in other forums is permitted, provided the original author(s) and the copyright owner(s) are credited and that the original publication in this journal is cited, in accordance with accepted academic practice. No use, distribution or reproduction is permitted which does not comply with these terms.
*Correspondence: Fay B. Horak, aG9yYWtmQG9oc3UuZWR1