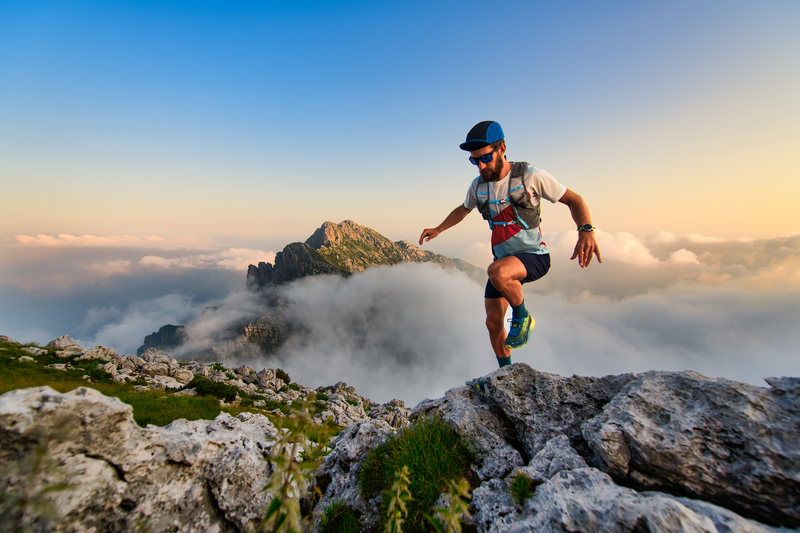
95% of researchers rate our articles as excellent or good
Learn more about the work of our research integrity team to safeguard the quality of each article we publish.
Find out more
ORIGINAL RESEARCH article
Front. Hum. Neurosci. , 25 September 2018
Sec. Motor Neuroscience
Volume 12 - 2018 | https://doi.org/10.3389/fnhum.2018.00373
Dancers and musicians differ in brain structure from untrained individuals. Structural covariance (SC) analysis can provide further insight into training-associated brain plasticity by evaluating interregional relationships in gray matter (GM) structure. The objectives of the present study were to compare SC of cortical thickness (CT) between expert dancers, expert musicians and untrained controls, as well as to examine the relationship between SC and performance on dance- and music-related tasks. A reduced correlation between CT in the left dorsolateral prefrontal cortex (DLPFC) and mean CT across the whole brain was found in the dancers compared to the controls, and a reduced correlation between these two CT measures was associated with higher performance on a dance video game task. This suggests that the left DLPFC is structurally decoupled in dancers and may be more strongly affected by local training-related factors than global factors in this group. This work provides a better understanding of structural brain connectivity and training-induced brain plasticity, as well as their interaction with behavior in dance and music.
Investigating the brains of individuals with specialized training, such as dancers and musicians, provides insight into training-associated brain plasticity as well as brain-behavioral relationships. Dance and music share fundamental similarities, including their reliance on sensorimotor integration as well as the structured and easily quantifiable nature of the training process. However, dance and music training also differ. For example, dance training commonly involves whole-body movements and following sound, while music training generally employs effector-specific movements to produce sound. The comparison of the neural correlates of dance vs. music can further understanding of brain characteristics related to auditory-motor artistic training in general, as well as characteristics that reflect more unique demands of the two types of training. Many studies have investigated the neural correlates of music training (for reviews, see Herholz and Zatorre, 2012; Schlaug, 2015), and there is growing interest to study the neural correlates of dance (e.g., Bläsing et al., 2012; Karpati et al., 2015, 2017; Bar and DeSouza, 2016; Di Nota et al., 2016; Giacosa et al., 2016). The present study aims to build on this literature by applying structural covariance (SC) analysis to investigate interregional gray matter (GM) structural relationships in dancers and musicians relative to untrained controls.
The neural correlates of dance and music have been examined using a wide variety of neuroimaging techniques (e.g., structural MRI, functional MRI, PET, fNIRS) and, within these techniques, a variety of measures and analysis methods (e.g., surface-based morphometry, voxel-based morphometry, diffusion tensor imaging). For example, studies comparing local GM structure between experts and non-experts have found differences in widespread areas including auditory and motor regions (e.g., Amunts et al., 1997; Schlaug, 2001; Schneider et al., 2002; Gaser and Schlaug, 2003; Bermudez et al., 2009; Han et al., 2009; Hänggi et al., 2010; Elmer et al., 2013; Fauvel et al., 2014; James et al., 2014; Nigmatullina et al., 2015; Karpati et al., 2017). Our study directly comparing dancers vs. musicians found that, although both differed from untrained controls, they did not differ from each other in local GM structure (Karpati et al., 2017). GM structure has also been demonstrated to correlate with performance on dance- and music-related tasks (e.g., Foster and Zatorre, 2010b; Karpati et al., 2017).
In contrast, differences between dancers and musicians were found in white matter (WM). Dancers demonstrated reduced fractional anisotropy (FA), suggesting reduced fiber coherence and increased fanning or crossing fibers, in interhemispheric, motor and sensorimotor integration tracts (Giacosa et al., 2016). This is consistent with previous work demonstrating increased FA in musicians relative to nonmusicians (e.g., Han et al., 2009; Halwani et al., 2011; Steele et al., 2013; Rüber et al., 2015), and reduced FA in dancers vs. nondancers (Hänggi et al., 2010). This suggests that the characteristics that differentiate these types of training (e.g., focus on whole-body vs. effector-specific movements) may be associated with different patterns of interregional connections.
Further insight into the interregional relationships of dancers and musicians has been provided by functional neuroimaging studies, which have found enhanced resting functional connectivity in a motor control pathway in dancers (Li et al., 2015), and in auditory, motor and somatosensory areas in musicians compared to their untrained counterparts (Luo et al., 2012; Choi et al., 2015; Klein et al., 2016; Palomar-García et al., 2017). Furthermore, activation in sensorimotor regions has been observed during music- (e.g., Gaab et al., 2003; Meister et al., 2004; Bangert et al., 2006; Bengtsson and Ullén, 2006; Foster and Zatorre, 2010a; Kleber et al., 2010; Lee et al., 2011; Klein and Zatorre, 2015) and dance-related tasks (e.g., Calvo-Merino et al., 2005; Brown et al., 2006; Cross et al., 2006; Pilgramm et al., 2010; Tachibana et al., 2011; Jola et al., 2013; Bar and DeSouza, 2016) which is influenced by short- and long-term training (e.g., Bangert et al., 2006; Cross et al., 2009; Foster and Zatorre, 2010a; Kleber et al., 2010; Pilgramm et al., 2010; Lappe et al., 2011). Taken together, these studies have observed a relationship between both brain structure and interregional connectivity with dance and music. They suggest that structure and interregional relationships may be influenced by training and associated with dance and music-related skills. SC analysis will build on these findings by identifying cortical regions that show unique relationships with overall GM structure, which may indicate regions that are influenced by local training-related factors.
The SC analysis used in the present study is based on the Mapping Anatomical Correlations Across Cerebral Cortex (MACACC) method developed by Lerch et al. (2006). This analysis measures how the GM structure of one brain area correlates with structure of other areas, and such correlations can be compared between groups or correlated with a behavioral measure (Lerch et al., 2006). Interregional correlations as measured by SC analysis show some consistency (35%–40%) with other measures of connectivity, such as WM tractography maps and functional resting state networks. Given this proportion of consistency, SC analysis has been demonstrated to provide unique information regarding interregional relationships (Lerch et al., 2006; Gong et al., 2012; Clos et al., 2014; Hardwick et al., 2015; Reid et al., 2016). Additional factors that may contribute to SC findings include indirect WM connections and indirect functional connectivity (i.e., parallel paths; Evans, 2013; Reid et al., 2016). Of particular importance to the present study, SC findings may also be related to mutual trophic influences on the connected regions (Ferrer et al., 1995; He et al., 2007; Cohen-Cory et al., 2010; Evans, 2013) and such influences may be affected by experience-related brain plasticity (Draganski and May, 2008; Evans, 2013; Lövdén et al., 2013; Kolb and Gibb, 2014). Therefore, SC findings may be related to a combination of WM tracts, synchronous neuronal firing, and mutual trophic influences between the correlated regions.
Many studies have employed SC analysis using a seed-based approach, where correlations were examined between structure (e.g., cortical thickness, CT) in a predetermined seed region and structure in other brain regions. These studies have investigated SC in clinical populations (e.g., Raznahan et al., 2010; Bernhardt et al., 2014; Voss and Zatorre, 2015; Zhao et al., 2015; Sharda et al., 2016, 2017) or general healthy groups (e.g., Camilleri et al., 2015; Hardwick et al., 2015). Only one study has applied SC analysis to a trained population. Bermudez et al. (2009) applied a seed-based MACACC analysis to a sample of musicians and non-musicians. They found that, in the non-musicians, the area of the brain where CT was correlated with CT in right frontal seed regions was more expansive than in musicians. This suggests that trained groups show a different CT covariance profile relative to untrained groups, which may be modulated by factors associated with training-related brain plasticity. It supports the idea that SC analysis provides additional information regarding the brain structural characteristics of trained populations that cannot be examined using only group comparisons of regional structure.
Although the use of a seed-based approach allows the investigation of specific brain networks or a priori regions of interest, it is also limited by the choice of specific regions. Another method, designed by Lerch et al. (2006), avoids any limitation or bias arising from seed selection by instead examining the SC of each vertex across the whole brain with each other vertex. This provides a measure of the relative interconnectivity of each vertex. The correlation of structure at each vertex to each other vertex is very computationally expensive, however a proxy measure involving correlating structure at each vertex to the individual’s mean structural measure across the whole brain (e.g., mean CT) has been developed (Lerch et al., 2006) and replicated (Lee et al., 2014a). Although the proxy method is not perfectly identical to the full method as a result of unequal standard deviations of CT at each vertex (Lerch et al., 2006), the two studies listed above directly compared the two methods and found a nearly identical pattern of results, leading to the conclusion that the proxy method is an appropriate substitute for the full method. This seed-free method has been used to investigate the relationship between SC and cognitive task performance in adolescents (Lee et al., 2014a,b), but it has not yet been applied to any trained populations.
In the present study, we apply this method to investigate the relationship of SC with dance and music. The objectives of this study were to: (1) test for differences in SC between expert dancers, expert musicians and untrained controls; and (2) examine the relationship between SC and performance on dance- and music-related tasks. This will provide further understanding of the brain structural characteristics of these groups, as well as training-associated brain plasticity in general, by testing for regions where GM structure may have a unique relationship with overall GM structure. This can indicate regions that may have increased or decreased influence from the same factors as the rest of the cortex. Those regions that are relatively less influenced by such whole-brain factors may be more influenced by local training-related factors.
Based on previous findings of local GM structure, WM and functional connectivity differences among dancers, musicians and untrained controls in sensorimotor regions (e.g., Bermudez et al., 2009; Li et al., 2015; Rüber et al., 2015; Giacosa et al., 2016; Karpati et al., 2017; Palomar-García et al., 2017) group differences in SC were expected in similar sensorimotor regions. A reduced correlation between GM structure in sensorimotor regions and the rest of the cortex was expected in the trained groups as an indication of the influence of local training-associated factors on these regions. Complementary findings were expected in the brain-behavioral analysis, with regions showing SC differences in dancers and musicians also demonstrating a relationship between SC and dance- and music-related tasks, respectively. Since SC provides a unique measure relative to WM or functional connectivity, (e.g., Gong et al., 2012; Alexander-Bloch et al., 2013; Evans, 2013; Reid et al., 2016), additional SC differences were expected in brain areas that have not previously been detected studies comparing such traits between dancers, musicians and controls.
Three groups of participants (aged 18–40 years old) were recruited for this study: expert dancers (N = 20), expert musicians (N = 19) and a control group of non-musicians/non-dancers (N = 20; Table 1). Dancers and musicians were either currently practicing as professionals or were students involved in professional training programs. Their training was assessed via a detailed questionnaire developed in our laboratories (Bailey and Penhune, 2010; Coffey et al., 2011). Dancers and musicians had on average approximately 15 years of experience in their respective disciplines, and controls had on average less than 1 year of experience in dance, music, figure skating and aerobics. All participants were physically active (e.g., biking, running, or other fitness activities). Dancers were currently practicing contemporary dance as their principal style, but had a variety of training backgrounds including ballet, tap, jazz, swing and ballroom. Dancers whose main style was too similar to the dance task used here (i.e., urban, street or hip-hop) were excluded. Musicians had various instrumental backgrounds, including keyboard instruments, strings, woodwinds, brass and percussion. None of the musicians had absolute pitch. Since the dance task was based on a video game, participants were screened for experience with dance video games; 56 out of 59 participants reported that they never or rarely (up to three times per year) played dance video games. The remaining three participants (one dancer and two musicians) reported a maximum 4 months of lifetime experience with dance video games. The groups did not differ in age, sex distribution, body mass index (BMI) or level of education (Table 1). Participants had no past or current learning or developmental disorder, neurological or psychiatric condition, or alcohol or substance abuse. This study was carried out in accordance with the recommendations of the Research Ethics Board at the Montreal Neurological Institute and Hospital with written informed consent from all subjects. All subjects gave written informed consent in accordance with the Declaration of Helsinki. The protocol was approved by the Research Ethics Board at the Montreal Neurological Institute and Hospital.
Participants completed a dance-related task (dance imitation) and a music-related task (melody discrimination). The dance imitation task required participants to imitate seven dance routines of increasing levels of complexity. These routines were selected from the video game Dance Central for Xbox Kinect version 1 (Harmonix1). This task assesses the ability to observe and imitate whole body dance movements in real time with music, and was scored using a measure of percent moves correct provided by the Kinect system.
In the melody discrimination task (Foster and Zatorre, 2010a, b), participants were asked to determine if pairs of melodies were the same or different based on changes in pitch. Participants completed four blocks of 30 trials each. This task measures auditory processing and pitch discrimination, and was scored using a measure of percent trials correct. Additional details about these tasks as well as behavioral analyses on this sample have been reported in an earlier study (Karpati et al., 2016).
T1-weighted brain images were acquired for all participants at the Montreal Neurological Institute (MNI) on a 3T Siemens Trio MR scanner with a 32-channel head coil. MRI scanning parameters were as follows: echo time = 2.98 ms, repetition time = 2,300 ms, voxel size 1 mm × 1 mm × 1 mm. Earplugs and headphones were used to reduce noise perception, and foam pads were used to reduce head motion.
Images were processed using the CIVET pipeline (version 1.1.11, Ad-Dab’bagh et al., 20062). They were registered to the ICBM152 nonlinear model (Collins et al., 1994; Grabner et al., 2006) with 12 degrees of freedom for registration, and corrected for signal intensity nonuniformity (Sled et al., 1998). Images were segmented into GM and WM, cerebrospinal fluid and background (Zijdenbos et al., 1998; Tohka et al., 2004). Deformable models were fitted to the images in order to extract the boundaries between GM and each of WM and cerebrospinal fluid (MacDonald et al., 2000; Kim et al., 2005), resulting in two surfaces with 81,920 polygons each. Following the surface extraction, participants’ cortical mid-surfaces (calculated using the mid-points of the linked inner and outer surfaces) were nonlinearly aligned using the SURFTRACC algorithm and a depth-potential function to a hemisphere-unbiased iterative surface template in order to establish intersubject vertex correspondence (Robbins et al., 2004; Lyttelton et al., 2007; Boucher et al., 2009). Then, a CT map was calculated for each participant, where CT (the distance between the pia mater and GM/WM boundary) was measured at each vertex using the t-link metric (Ad-Dab’bagh et al., 2005; Lerch and Evans, 2005) and then blurred with a 20 mm surface-based blurring kernel (Chung and Taylor, 2004).
In order to measure the overall correlation strength of CT of each vertex with all other vertices, a proxy measure of the correlation between vertex CT and whole-brain mean CT was used (Lerch et al., 2006; Lee et al., 2014a,b). This measure of SC was compared between groups (dancers, musicians and controls) at each vertex across the whole brain, using an F-test for the presence of a group by mean CT interaction (Eq. 1) followed by pairwise comparisons using the same general linear model.
Following the finding of a significant cluster in the above F-test, additional post hoc tests were conducted to investigate several factors that may contribute to this result. This included:
1. An ANOVA for group differences in whole-brain mean CT to investigate whether global factors affecting whole-brain CT may differ between groups.
2. A region-of-interest test for group differences in CT in the observed cluster using a general linear model to conduct an F-test (Eq. 2). This tested for subtle group differences in local structure in this area, which may indicate training-associated plasticity processes acting in this area that were not identified using the whole-brain analysis of local structure reported previously (Karpati et al., 2017).
3. Levene’s test for group differences in variance of CT in this cluster, since correlations are less likely to occur with a variable that has low variance.
4. An F-test for group differences in the correlation between CT in this cluster and CT in all other vertices, as well as a qualitative examination of correlations with cluster CT across the whole brain in each group. This allowed for a comparison of the location and extent of areas where CT is correlated with cluster CT, thereby supporting findings of overall increased or decreased whole-brain correlation with the cluster.
The relationship between SC and performance on the dance imitation and melody discrimination tasks was also investigated. Across all participants, an interaction between task score and mean CT was tested at each vertex across the whole brain (Eq. 3) for each task separately.
All analysis was conducted using SurfStat software3. Age, sex and a proxy measure of brain volume (pBV, Karama et al., 2011) were included as covariates. Vertex clusters were defined using a forming threshold of p < 0.01, and correction for family-wise error at p < 0.05 was then applied at the cluster level using random field theory (Friston et al., 1994). Effect sizes for significant results were measured as partial eta squared (; Lakens, 2013).
An F-test (df = 2, 50) for the presence of a group (dancer, musician or control) by mean CT interaction yielded a significant cluster in the left middle frontal gyrus (MFG; p = 0.03; Figure 1A; Table 2). This cluster will be referred to as the Group MFG Cluster. Individual group maps of mean CT-regional CT relationship are included as Supplementary Figure S1. Pairwise comparisons (df = 50) show that this MFG cluster result is driven by a reduced correlation between mean CT and regional CT in this area in the dancers compared to the controls (p < 0.01; Table 2). No significant differences in the strength of the mean CT-regional CT correlation were found between musicians and either dancers or controls. The relationship between mean CT and CT in this cluster was r = 0.29 (p = 0.2) for dancers, r = 0.9 (p < 0.0001) for musicians, and r = 0.8 (p < 0.0001) for controls (Figure 1B). Effect size calculations indicated a partial eta squared of 0.30 for the F-test cluster and 0.29 for the pairwise cluster (Table 2), corresponding to a large effect size (Cohen, 1988).
Figure 1. The result of the F-test for the presence of a group by mean cortical thickness (CT) interaction is shown in panel (A) demonstrating a significant cluster in the left middle frontal gyrus (MFG; p = 0.03). In panel (B) a scatterplot shows the relationship between mean CT and CT in this cluster (i.e., mean CT of the cluster, adjusted for covariates) in the dancers, musicians and controls. Dancers have a significantly lower correlation between mean CT and left MFG CT compared to controls.
Following the significant finding above, further testing was conducted to characterize variation in whole brain mean CT and MFG cluster CT separately:
1. An ANOVA for group differences in whole-brain mean CT revealed no significant differences (p > 0.1).
2. A region-of-interest F-test for group differences in CT in the Group MFG Cluster did not show any significant differences (p = 0.4).
3. Levene’s test for group differences in variance in CT in the Group MFG Cluster showed a trend towards a group difference (Levene’s statistic = 2.7, p = 0.07). Pairwise comparisons showed that this trend is driven by reduced variance in the dancers compared to controls (Levene’s statistic = 6.9, p = 0.01). Musicians did not differ significantly from either dancers or controls in variance in CT in this region (Levene’s statistic ≤1.9, p > 0.1). The distribution of CT in this region for each group is shown in Figure 2.
4. An F-test conducted across the whole brain for the presence of a group by Group MFG Cluster CT interaction did not show any significant results. However, examination of brain regions in which Group MFG Cluster CT is correlated in each group separately showed that, in the musician and control groups, CT in this cluster is significantly correlated with CT in a variety of bilateral brain regions (cluster p ≤ 0.05). In the dancer group, CT in this cluster is not correlated with any regions outside of the left frontal lobe. Correlation maps showing regions where CT is correlated with Group MFG Cluster CT for each group is presented in Figure 3.
Figure 2. This box plot shows the distribution of CT in the Group MFG Cluster (i.e., mean CT of the cluster, adjusted for covariates) for each group. Boxes represent means and standard deviations. There is a trend towards a group difference in variance in CT in this region (Levene’s test p = 0.07). Pairwise comparisons showed that this trend is driven by reduced variance in the dancers compared to controls (Levene’s statistic = 6.9, p = 0.01).
Figure 3. Regions where CT is correlated (cluster p ≤ 0.05) with CT in the left MFG cluster (i.e., mean CT of the cluster, adjusted for covariates) are shown in yellow for each group. In both musicians and controls, CT in this cluster is significantly correlated with CT in a variety of bilateral regions. In contrast, in the dancers, CT in this cluster is not correlated with CT in any areas outside of the left frontal lobe.
A general linear model (df = 52) testing for an interaction between dance imitation task score and mean CT showed a negative interaction between these two factors in a cluster in the left MFG (p < 0.01; Table 3; Figure 4A). This indicates that higher dance task scores are associated with a lower correlation between mean CT and left MFG CT, which is illustrated in Figure 4B. A general linear model (df = 52) testing for an interaction between melody discrimination task score and mean CT revealed an interaction between these two factors in a cluster in the right superior temporal gyrus (STG; p = 0.01; Table 3; Figure 5A). This indicates that higher melody task scores are associated with a lower correlation between mean CT and right STG CT, which is shown in Figure 5B. Effect size calculations indicated a partial eta squared of 0.23 for the dance cluster and 0.21 for the melody cluster (Table 3), corresponding to a medium effect size (Cohen, 1988).
Figure 4. A negative interaction between score on the dance imitation task and mean CT was found in a cluster in the left MFG (p = 0.006) as shown in panel (A). In panel (B) this interaction is visualized in a 3-dimensional scatterplot showing dance task score, mean CT and left MFG CT (i.e., mean CT of the cluster, adjusted for covariates) in the dancers (blue), musicians (red) and controls (green). This demonstrates that a higher score on the dance task is associated with a reduced correlation between mean CT and left MFG CT.
Figure 5. A negative interaction between score on the melody discrimination task and mean CT was found in a cluster in the right superior temporal gyrus (STG; p = 0.01) as shown in panel (A). In panel (B), this interaction is visualized in a 3-dimensional scatterplot showing melody task score, mean CT and right STG CT (i.e., mean CT of the cluster, adjusted for covariates) in the dancers (blue), musicians (red) and controls (green). This demonstrates that a higher score on the melody task is associated with a reduced correlation between mean CT and right STG CT.
This study is the first to compare SC (i.e., the relative correlation of structure in each region to overall GM structure) between expert dancers, expert musicians and untrained controls. The relationship between SC and performance on measures of dance- and music-related skills (i.e., dance imitation and melody discrimination tasks) was also examined. Dancers showed a negligible correlation between CT in the left MFG and whole-brain mean CT, in contrast to controls and musicians, whose MFG CT was strongly correlated with mean CT. Across all participants, a reduced correlation between left MFG CT and mean CT was associated with higher scores on the dance imitation task, and a reduced correlation between right STG CT and mean CT was related to better performance on the melody discrimination task. These findings show that SC can provide unique insight into training-associated brain plasticity and brain-behavior relationships. SC analysis allows the identification of regions that may show unique relationships with overall structure in a trained vs. untrained group, thereby indicating regions that may be influenced by local training-related factors.
Dancers showed a difference in SC relative to untrained controls. Specifically, the correlation between left MFG CT and mean CT was reduced in the dancers. In support of this result, post hoc tests showed that left MFG CT was correlated with CT in widespread brain areas in the controls, while it was not correlated with CT in any areas outside of the left frontal lobe in dancers. These findings suggest that this region, localized to the dorsolateral prefrontal cortex (DLPFC; Brodmann areas 9 and 46; Petrides, 2000; Fletcher and Henson, 2001; Krawczyk, 2002; Hoshi, 2006), has a unique relationship with overall GM structure in the dancers. This can be interpreted as a structural decoupling of the DLPFC in dancers (i.e., an independence of GM structure in this area relative to overall GM structure). Consistent with this finding, a reduced correlation between left DLPFC CT and mean CT was associated with higher scores on the dance imitation task. This SC-behavior correlation is likely driven by the difference in the SC of this region between dancers and controls, as described above, as well as enhanced behavioral performance in dancers relative to controls on this task (Karpati et al., 2016). The lack of predicted findings in sensorimotor areas furthers the idea that SC provides complementary but not identical information to local GM comparisons. This suggests that the factors influencing SC may be different from those affecting local GM structure.
SC findings may be driven by a variety of factors, including direct WM connections, synchronous neuronal firing, and training-associated mutual trophic influences (Ferrer et al., 1995; Lerch et al., 2006; He et al., 2007; Cohen-Cory et al., 2010; Gong et al., 2012; Evans, 2013; Clos et al., 2014; Hardwick et al., 2015; Reid et al., 2016). The potential contributions of each of these factors to the current results, including support or lack thereof for each, will be discussed.
Our laboratory has investigated WM in this sample. We found reductions in FA along with increased radial diffusivity in widespread left frontal tracts, including the superior longitudinal fasciculus and corona radiata, in the dancers relative to musicians (Giacosa et al., 2016). This is consistent with observations by Hänggi et al. (2010) of reduced FA in the left MFG in dancers relative to non-dancers. The findings from both these studies are in the area of the observed DLPFC cluster. These findings have been interpreted as increased heterogeneity of fiber orientation or increased fanning/crossing fibers in the dancers (Giacosa et al., 2016). These broad connections have likely developed to meet the demands of whole-body dance training. Although these observations indicate differences in interregional connectivity in left frontal regions in dancers, which supports the present findings, it is not clear exactly how such WM findings relate to SC differences. This is because a relationship between SC and FA or other diffusivity measures has not yet been investigated, and negative SC relationships have shown poor location correspondence with WM tracts in previous work (Gong et al., 2012; Reid et al., 2016). It is possible that WM connectivity is a factor underlying the present result, however further studies comparing SC with WM diffusivity measures are needed to clarify the contribution of WM to such SC findings.
Synchronous neuronal firing between regions has been suggested to play a role in positive SC relationships (Alexander-Bloch et al., 2013; Evans, 2013), therefore a negative SC relationship as observed in the present result may be related to independent firing patterns. In the case of the present results, this hypothesis would imply that the DLPFC activates independently of the rest of the cortex in dancers. This is not supported by the only study that has investigated resting state functional connectivity in dancers vs. non-dancers (Li et al., 2015) as they did not find group differences in this area. Given the research conducted to date, there is no evidence to suggest that functional asynchronicity is a contributor to the current findings. However, further work perhaps using a region of interest approach focused on the DLPFC as well as a combination of resting state and task-based analyses, will increase understanding of the structure-function relationship in this area in dancers.
A third factor that may contribute to SC findings is common trophic influences acting on correlated regions (Ferrer et al., 1995; He et al., 2007; Cohen-Cory et al., 2010; Evans, 2013). Regions which receive mutual influences are hypothesized to demonstrate positive SC relationships, therefore a negative SC relationship as found in the present result would indicate different influences between regions. In the present finding, this would suggest that DLPFC structure is influenced by unique factors relative to the rest of the cortex in dancers. This idea is supported by findings of the post hoc tests, which demonstrated that dancers (compared to controls) had reduced variance in CT in this area. This suggests that there may be an optimal range of CT in this region for dance performance, and unique factors may work to ensure this regional CT is achieved regardless of CT across the rest of the brain. These unique factors that may be acting on the DLPFC in dancers are likely due to their training, which is consistent with previous reports of effects of experience- and training-related brain plasticity on brain structural trophic factors (Zatorre et al., 2012; Lövdén et al., 2013; Kolb and Gibb, 2014). Neuroplasticity is associated with a chain of interacting events beginning with changes in gene expression, which in turn may lead to alterations in the expression of growth factors such as brain-derived neurotrophic factor (BDNF; Zatorre et al., 2012; Lövdén et al., 2013; Kolb and Gibb, 2014). Findings of a relationship between BDNF expression and CT (Yang et al., 2012; Legge et al., 2015; Song et al., 2015; Na et al., 2016) support the idea that training-associated brain plasticity may play a role in the structural decoupling of CT in the DLPFC in dancers. Although it is possible that there are several factors contributing to the current result, the idea of local training-related factors acting on this region in the dancers appears to be the most plausible and justified by previous work.
The DLPFC is known as a region involved in executive processing functions applicable to many domains (e.g., Rowe et al., 2000; Krawczyk, 2002; Petrides, 2005; Champod and Petrides, 2007). Activity in the DLPFC has been observed in many movement-related tasks, including action observation with intent to imitate (Decety et al., 1997; Buccino et al., 2004; Krüger et al., 2014), action planning and selection (Buccino et al., 2004; Hoshi, 2006; Ubaldi et al., 2015), action prediction (Cross et al., 2013) and motor imagery (Gerardin et al., 2000; Grèzes and Decety, 2001; Malouin et al., 2003; Mizuguchi et al., 2013; Sauvage et al., 2013; Wriessnegger et al., 2016). Although these are functions that everyone executes on a daily basis, dancers likely execute them more accurately (e.g., Washburn et al., 2014), frequently and consciously than untrained controls. For example, motor imagery is often integrated into dancers’ training (Overby, 1990) and they spend large amounts of time learning movements through imitation (Harbonnier-Torpin and Barbier, 2012). This may lead to a need for a particular structure of this area in dancers in order to accommodate their unique needs for these types of functions. Additional support for the importance of the DLPFC in dance comes from the finding that internal representations of movements are generated in the DLPFC (Arnsten and Jin, 2014) and have been found to be different in dancers vs. non-dancers (Bläsing et al., 2009). Further work comparing activity in the DLPFC between dancers and untrained controls across a battery of motor, perceptual and cognitive tasks, and how this relates to structure, will help to clarify the role of this region in dance.
In contrast to the difference in SC observed between dancers and controls, the SC of musicians was not found to be significantly different from either dancers or controls. The only previous study to investigate SC in musicians found a difference is SC between musicians and non-musicians using a seed-based approach focused on right frontal regions (Bermudez et al., 2009). The present study employed an unbiased, seed-free whole-brain approach, which may reveal different aspects of SC. The finding of a significant difference in SC between dancers and controls without a difference between musicians and controls is consistent with previous findings from our laboratory of differences in interregional connections in WM between dancers and musicians (Giacosa et al., 2016). Taken together, these findings suggest that musicians may have unique interregional brain structural relationships relative to dancers and controls, and their SC of the DLPFC as measured in the present study are intermediate between those of dancers and controls. Future work comparing functional connectivity in these groups is necessary to further distinguish their interregional relationships.
Although no group differences in SC were found between musicians and either dancers or controls, a relationship between SC in the right STG and performance on a music-related task (i.e., melody discrimination) was found. A reduced correlation between CT in the right STG and mean CT was associated with better scores on the melody discrimination task. This is consistent with previous findings of, and may be driven by, increased CT in the right STG in musicians vs. non-musicians (Bermudez et al., 2009; Karpati et al., 2017), better performance on this melody task in musicians vs. non-musicians (Foster and Zatorre, 2010a; Karpati et al., 2016), and a positive correlation between right STG CT and melody task performance (Karpati et al., 2017).
This study is the first to employ a seed-free method of SC analysis in trained populations, and to examine the relationship between SC and dance. SC was compared between expert dancers, expert musicians and untrained controls; and correlated with performance on dance- and music-related tasks. The left DLPFC was found to be structurally decoupled in dancers, and SC in this area was related to performance on the dance imitation task. These findings suggest that the left DLPFC may be more influenced by local training-related factors, relative to global factors that affect overall brain structure, in dancers. They also indicate that training-associated brain plasticity may extend beyond regional group differences in brain structure and into the structural relationship between regions.
This work provides a better understanding of the neural correlates of music and dance, training-related structural brain plasticity, and brain-behavior relationships. Future longitudinal studies to differentiate pre-existing brain structural characteristics vs. those caused by training will provide further insight into these concepts. This work can be applied to the development of arts-based therapies for clinical populations. Understanding how dance and music training may influence brain structure, and the regions influenced, would allow for the design of specific therapies in order to target regions and brain processes that would be most beneficial for particular populations.
Conception and experimental design of this study was conducted collaboratively by FK, CG, NF, VP and KH. FK and CG recruited participants, and collected the behavioral and neuroimaging data. FK analyzed the data with guidance from NF. FK led the result interpretation, with input from CG, NF, VP and KH. FK wrote the manuscript draft. FK, CG, NF, VP and KH contributed to manuscript revision.
This work was funded by a grant from the Natural Sciences and Engineering Research Council of Canada (NSERC) to KH. This work was included in FK’s doctoral thesis (Karpati, 2017).
The authors declare that the research was conducted in the absence of any commercial or financial relationships that could be construed as a potential conflict of interest.
We would like to thank our participants for their time, as well as Alessia Di Cesare, Veronica Yuk and Dana Boebinger for their assistance in the data collection process.
The Supplementary Material for this article can be found online at: https://www.frontiersin.org/articles/10.3389/fnhum.2018.00373/full#supplementary-material
Ad-Dab’bagh, Y., Einarson, D., Lyttelton, O., Muehlboeck, J. S., Mok, K., Ivanov, O., et al. (2006). “The CIVET image-processing environment: a fully automated comprehensive pipeline for anatomical neuroimaging research,” in Proceedings of the 12th Annual Meeting of the Organization for Human Brain Mapping, ed. M. Corbetta (Florence: Elsevier).
Ad-Dab’bagh, Y., Singh, V., Robbins, S., Lerch, J., Lyttelton, O., Fombonne, E., et al. (2005). “Native space cortical thickness measurement and the absence of correlation to cerebral volume,” in Proceedings of the 11th Annual Meeting of the Organization for Human Brain Mapping, ed. K. Zilles (Toronto, ON: Elsevier).
Alexander-Bloch, A., Giedd, J. N., and Bullmore, E. (2013). Imaging structural co-variance between human brain regions. Nat. Rev. Neurosci. 14, 322–336. doi: 10.1038/nrn3465
Amunts, K., Schlaug, G., Jäncke, L., Steinmetz, H., Schleicher, A., Dabringhaus, A., et al. (1997). Motor cortex and hand motor skills: structural compliance in the human brain. Hum. Brain Mapp. 5, 206–215. doi: 10.1002/(sici)1097-0193(1997)5:3<206::aid-hbm5>3.0.co;2-7
Arnsten, A. F. T., and Jin, L. E. (2014). Molecular influences on working memory circuits in dorsolateral prefrontal cortex. Prog. Mol. Biol. Transl. Sci. 122, 211–231. doi: 10.1016/b978-0-12-420170-5.00008-8
Bailey, J. A., and Penhune, V. B. (2010). Rhythm synchronization performance and auditory working memory in early- and late-trained musicians. Exp. Brain Res. 204, 91–101. doi: 10.1007/s00221-010-2299-y
Bangert, M., Peschel, T., Schlaug, G., Rotte, M., Drescher, D., Hinrichs, H., et al. (2006). Shared networks for auditory and motor processing in professional pianists: evidence from fMRI conjunction. Neuroimage 30, 917–926. doi: 10.1016/j.neuroimage.2005.10.044
Bar, R. J., and DeSouza, J. F. X. (2016). Tracking plasticity: effects of long-term rehearsal in expert dancers encoding music to movement. PLoS One 11:e0147731. doi: 10.1371/journal.pone.0147731
Bengtsson, S. L., and Ullén, F. (2006). Dissociation between melodic and rhythmic processing during piano performance from musical scores. Neuroimage 30, 272–284. doi: 10.1016/j.neuroimage.2005.09.019
Bermudez, P., Lerch, J. P., Evans, A. C., and Zatorre, R. J. (2009). Neuroanatomical correlates of musicianship as revealed by cortical thickness and voxel-based morphometry. Cereb. Cortex 19, 1583–1596. doi: 10.1093/cercor/bhn196
Bernhardt, B. C., Valk, S. L., Silani, G., Bird, G., Frith, U., and Singer, T. (2014). Selective disruption of sociocognitive structural brain networks in autism and alexithymia. Cereb. Cortex 24, 3258–3267. doi: 10.1093/cercor/bht182
Bläsing, B., Calvo-Merino, B., Cross, E. S., Jola, C., Honisch, J., and Stevens, C. J. (2012). Neurocognitive control in dance perception and performance. Acta Psychol. 139, 300–308. doi: 10.1016/j.actpsy.2011.12.005
Bläsing, B., Tenenbaum, G., and Schack, T. (2009). The cognitive structure of movements in classical dance. Psychol. Sport Exerc. 10, 350–360. doi: 10.1016/j.psychsport.2008.10.001
Boucher, M., Whitesides, S., and Evans, A. (2009). Depth potential function for folding pattern representation, registration and analysis. Med. Image Anal. 13, 203–214. doi: 10.1016/j.media.2008.09.001
Brown, S., Martinez, M. J., and Parsons, L. M. (2006). The neural basis of human dance. Cereb. Cortex 16, 1157–1167. doi: 10.1093/cercor/bhj057
Buccino, G., Vogt, S., Ritzl, A., Fink, G. R., Zilles, K., Freund, H.-J., et al. (2004). Neural circuits underlying imitation learning of hand actions: an event-related fMRI study. Neuron 42, 323–334. doi: 10.1016/s0896-6273(04)00181-3
Calvo-Merino, B., Glaser, D. E., Grèzes, J., Passingham, R. E., and Haggard, P. (2005). Action observation and acquired motor skills: an fMRI study with expert dancers. Cereb. Cortex 15, 1243–1249. doi: 10.1093/cercor/bhi007
Camilleri, J. A., Reid, A. T., Müller, V. I., Grefkes, C., Amunts, K., and Eickhoff, S. B. (2015). Multi-modal imaging of neural correlates of motor speed performance in the trail making test. Front. Neurol. 6:219. doi: 10.3389/fneur.2015.00219
Champod, A. S., and Petrides, M. (2007). Dissociable roles of the posterior parietal and the prefrontal cortex in manipulation and monitoring processes. Proc. Natl. Acad. Sci. U S A 104, 14837–14842. doi: 10.1073/pnas.0607101104
Choi, U.-S., Sung, Y.-W., Hong, S., Chung, J.-Y., and Ogawa, S. (2015). Structural and functional plasticity specific to musical training with wind instruments. Front. Hum. Neurosci. 9:597. doi: 10.3389/fnhum.2015.00597
Chung, M. K., and Taylor, J. (2004). “Diffusion smoothing on brain surface via finite element method,” in Proceedings of the IEEE International Symposium (Arlington, VA: IEEE), 432–435.
Clos, M., Rottschy, C., Laird, A. R., Fox, P. T., and Eickhoff, S. B. (2014). Comparison of structural covariance with functional connectivity approaches exemplified by an investigation of the left anterior insula. Neuroimage 99, 269–280. doi: 10.1016/j.neuroimage.2014.05.030
Coffey, E. B. J., Herholz, S. C., Scala, S., and Zatorre, R. J. (2011). “Montreal music history questionnaire: a tool for the assessment of music-related experience in music cognition research,” in The Neurosciences and Music IV: Learning and Memory, eds K. Overy, I. Peretz, R. J. Zatorre, L. Lopez and M. Manjo (Edinburgh: John Wiley & Sons), 350.
Cohen, J. (1988). Statistical Power Analysis for the Behavioral Sciences. 2nd Edn. Hillsdale, NJ: Lawrence Erlbaum Associates.
Cohen-Cory, S., Kidane, A. H., Shirkey, N. J., and Marshak, S. (2010). Brain-derived neurotrophic factor and the development of structural neuronal connectivity. Dev. Neurobiol. 70, 271–288. doi: 10.1002/dneu.20774
Collins, D. L., Neelin, P., Peters, T. M., and Evans, A. C. (1994). Automatic 3D inter-subject registration of MR volumetric data in standardized Talairach space. J. Comput. Assist. Tomogr. 18, 192–205. doi: 10.1097/00004728-199403000-00005
Cross, E. S., Hamilton, A. F., and Grafton, S. T. (2006). Building a motor simulation de novo: observation of dance by dancers. Neuroimage 31, 1257–1267. doi: 10.1016/j.neuroimage.2006.01.033
Cross, E. S., Kraemer, D. J. M., Hamilton, A. F. de C., Kelley, W. M., and Grafton, S. T. (2009). Sensitivity of the action observation network to physical and observational learning. Cereb. Cortex 19, 315–326. doi: 10.1093/cercor/bhn083
Cross, E. S., Stadler, W., Parkinson, J., Schütz-Bosbach, S., and Prinz, W. (2013). The influence of visual training on predicting complex action sequences. Hum. Brain Mapp. 34, 467–486. doi: 10.1002/hbm.21450
Decety, J., Grèzes, J., Costes, N., Perani, D., Jeannerod, M., Procyk, E., et al. (1997). Brain activity during observation of actions. Influence of action content and subject’s strategy. Brain 120, 1763–1777. doi: 10.1093/brain/120.10.1763
Di Nota, P., Levkov, G., Bar, R., and DeSouza, J. F. X. (2016). Lateral occipitotemporal cortex (LOTC) activity is greatest while viewing dance compared to visualization and movement: learning and expertise effects. Exp. Brain Res. 234, 2007–2023. doi: 10.1007/s00221-016-4607-7
Draganski, B., and May, A. (2008). Training-induced structural changes in the adult human brain. Behav. Brain Res. 192, 137–142. doi: 10.1016/j.bbr.2008.02.015
Elmer, S., Hänggi, J., Meyer, M., and Jäncke, L. (2013). Increased cortical surface area of the left planum temporale in musicians facilitates the categorization of phonetic and temporal speech sounds. Cortex 49, 2812–2821. doi: 10.1016/j.cortex.2013.03.007
Evans, A. C. (2013). Networks of anatomical covariance. Neuroimage 80, 489–504. doi: 10.1016/j.neuroimage.2013.05.054
Fauvel, B., Groussard, M., Chételat, G., Fouquet, M., Landeau, B., Eustache, F., et al. (2014). Morphological brain plasticity induced by musical expertise is accompanied by modulation of functional connectivity at rest. Neuroimage 90, 179–188. doi: 10.1016/j.neuroimage.2013.12.065
Ferrer, I., Blanco, R., Carulla, M., Condom, M., Alcántara, S., Olivé, M., et al. (1995). Transforming growth factor-α immunoreactivity in the developing and adult brain. Neuroscience 66, 189–199. doi: 10.1016/0306-4522(94)00584-r
Fletcher, P. C., and Henson, R. N. A. (2001). Frontal lobes and human memory: insights from functional neuroimaging. Brain 124, 849–881. doi: 10.1093/brain/124.5.849
Foster, N. E. V., and Zatorre, R. J. (2010a). A role for the intraparietal sulcus in transforming musical pitch information. Cereb. Cortex 20, 1350–1359. doi: 10.1093/cercor/bhp199
Foster, N. E. V., and Zatorre, R. J. (2010b). Cortical structure predicts success in performing musical transformation judgments. Neuroimage 53, 26–36. doi: 10.1016/j.neuroimage.2010.06.042
Friston, K. J., Worsley, K. J., Frackowiak, R. S., Mazziotta, J. C., and Evans, A. C. (1994). Assessing the significance of focal activations using their spatial extent. Hum. Brain Mapp. 1, 210–220. doi: 10.1002/hbm.460010306
Gaab, N., Gaser, C., Zaehle, T., Jancke, L., and Schlaug, G. (2003). Functional anatomy of pitch memory—an fMRI study with sparse temporal sampling. Neuroimage 19, 1417–1426. doi: 10.1016/s1053-8119(03)00224-6
Gaser, C., and Schlaug, G. (2003). Brain structures differ between musicians and non-musicians. J. Neurosci. 23, 9240–9245. doi: 10.1523/jneurosci.23-27-09240.2003
Gerardin, E., Sirigu, A., Lehéricy, S., Poline, J. B., Gaymard, B., Marsault, C., et al. (2000). Partially overlapping neural networks for real and imagined hand movements. Cereb. Cortex 10, 1093–1104. doi: 10.1093/cercor/10.11.1093
Giacosa, C., Karpati, F. J., Foster, N. E. V., Penhune, V. B., and Hyde, K. L. (2016). Dance and music training have different effects on white matter diffusivity in sensorimotor pathways. Neuroimage 135, 273–286. doi: 10.1016/j.neuroimage.2016.04.048
Gong, G., He, Y., Chen, Z. J., and Evans, A. C. (2012). Convergence and divergence of thickness correlations with diffusion connections across the human cerebral cortex. Neuroimage 59, 1239–1248. doi: 10.1016/j.neuroimage.2011.08.017
Grabner, G., Janke, A. L., Budge, M. M., Smith, D., Pruessner, J., and Collins, D. L. (2006). Symmetric atlasing and model based segmentation: an application to the hippocampus in older adults. Med. Image Comput. Comput. Assist. Interv. 9, 58–66. doi: 10.1007/11866763_8
Grèzes, J., and Decety, J. (2001). Functional anatomy of execution, mental simulation, observation and verb generation of actions: a meta-analysis. Hum. Brain Mapp. 12, 1–19. doi: 10.1002/1097-0193(200101)12:1<1::aid-hbm10>3.0.co;2-v
Halwani, G. F., Loui, P., Rüber, T., and Schlaug, G. (2011). Effects of practice and experience on the arcuate fasciculus: comparing singers, instrumentalists and non-musicians. Front. Psychol. 2:156. doi: 10.3389/fpsyg.2011.00156
Han, Y., Yang, H., Lv, Y.-T., Zhu, C.-Z., He, Y., Tang, H.-H., et al. (2009). Gray matter density and white matter integrity in pianists’ brain: a combined structural and diffusion tensor MRI study. Neurosci. Lett. 459, 3–6. doi: 10.1016/j.neulet.2008.07.056
Hänggi, J., Koeneke, S., Bezzola, L., and Jäncke, L. (2010). Structural neuroplasticity in the sensorimotor network of professional female ballet dancers. Hum. Brain Mapp. 31, 1196–1206. doi: 10.1002/hbm.20928
Harbonnier-Torpin, N., and Barbier, J.-M. (2012). “How seeing helps doing and doing allows to see more”: the process of imitation in the dance class. Res. Dance Educ. 13, 301–325. doi: 10.1080/14647893.2012.677423
Hardwick, R. M., Lesage, E., Eickhoff, C. R., Clos, M., Fox, P., and Eickhoff, S. B. (2015). Multimodal connectivity of motor learning-related dorsal premotor cortex. Neuroimage 123, 114–128. doi: 10.1016/j.neuroimage.2015.08.024
He, Y., Chen, Z. J., and Evans, A. C. (2007). Small-world anatomical networks in the human brain revealed by cortical thickness from MRI. Cereb. Cortex 17, 2407–2419. doi: 10.1093/cercor/bhl149
Herholz, S. C., and Zatorre, R. J. (2012). Musical training as a framework for brain plasticity: behavior, function and structure. Neuron 76, 486–502. doi: 10.1016/j.neuron.2012.10.011
Hoshi, E. (2006). Functional specialization within the dorsolateral prefrontal cortex: a review of anatomical and physiological studies of non-human primates. Neurosci. Res. 54, 73–84. doi: 10.1016/j.neures.2005.10.013
James, C. E., Oechslin, M. S., Van De Ville, D., Hauert, C.-A., Descloux, C., and Lazeyras, F. (2014). Musical training intensity yields opposite effects on grey matter density in cognitive versus sensorimotor networks. Brain Struct. Funct. 219, 353–366. doi: 10.1007/s00429-013-0504-z
Jola, C., McAleer, P., Grosbras, M.-H., Love, S. A., Morison, G., and Pollick, F. E. (2013). Uni- and multisensory brain areas are synchronised across spectators when watching unedited dance recordings. Iperception 4, 265–284. doi: 10.1068/i0536
Karama, S., Colom, R., Johnson, W., Deary, I. J., Haier, R., Waber, D. P., et al. (2011). Cortical thickness correlates of specific cognitive performance accounted for by the general factor of intelligence in healthy children aged 6 to 18. Neuroimage 55, 1443–1453. doi: 10.1016/j.neuroimage.2011.01.016
Karpati, F. (2017). The Brain Structural and Behavioural Correlates of Specialized Training: Dance Versus Music [Doctoral Thesis]. Montreal, QC: McGill University.
Karpati, F. J., Giacosa, C., Foster, N. E. V., Penhune, V. B., and Hyde, K. L. (2015). Dance and the brain: a review. Ann. N Y Acad. Sci. 1337, 140–146. doi: 10.1111/nyas.12632
Karpati, F. J., Giacosa, C., Foster, N. E. V., Penhune, V. B., and Hyde, K. L. (2016). Sensorimotor integration is enhanced in dancers and musicians. Exp. Brain Res. 234, 893–903. doi: 10.1007/s00221-015-4524-1
Karpati, F. J., Giacosa, C., Foster, N. E. V., Penhune, V. B., and Hyde, K. L. (2017). Dance and music share gray matter structural correlates. Brain Res. 1657, 62–73. doi: 10.1016/j.brainres.2016.11.029
Kim, J. S., Singh, V., Lee, J. K., Lerch, J., Ad-Dab’bagh, Y., MacDonald, D., et al. (2005). Automated 3-D extraction and evaluation of the inner and outer cortical surfaces using a Laplacian map and partial volume effect classification. Neuroimage 27, 210–221. doi: 10.1016/j.neuroimage.2005.03.036
Kleber, B., Veit, R., Birbaumer, N., Gruzelier, J., and Lotze, M. (2010). The brain of opera singers: experience-dependent changes in functional activation. Cereb. Cortex 20, 1144–1152. doi: 10.1093/cercor/bhp177
Klein, C., Liem, F., Hänggi, J., Elmer, S., and Jäncke, L. (2016). The “silent” imprint of musical training. Hum. Brain Mapp. 37, 536–546. doi: 10.1002/hbm.23045
Klein, M. E., and Zatorre, R. J. (2015). Representations of invariant musical categories are decodable by pattern analysis of locally distributed BOLD responses in superior temporal and intraparietal sulci. Cereb. Cortex 25, 1947–1957. doi: 10.1093/cercor/bhu003
Kolb, B., and Gibb, R. (2014). Searching for the principles of brain plasticity and behavior. Cortex 58, 251–260. doi: 10.1016/j.cortex.2013.11.012
Krawczyk, D. C. (2002). Contributions of the prefrontal cortex to the neural basis of human decision making. Neurosci. Biobehav. Rev. 26, 631–664. doi: 10.1016/s0149-7634(02)00021-0
Krüger, B., Bischoff, M., Blecker, C., Langhanns, C., Kindermann, S., Sauerbier, I., et al. (2014). Parietal and premotor cortices: activation reflects imitation accuracy during observation, delayed imitation and concurrent imitation. Neuroimage 100, 39–50. doi: 10.1016/j.neuroimage.2014.05.074
Lakens, D. (2013). Calculating and reporting effect sizes to facilitate cumulative science: a practical primer for t-tests and ANOVAs. Front. Psychol. 4:863. doi: 10.3389/fpsyg.2013.00863
Lappe, C., Trainor, L. J., Herholz, S. C., and Pantev, C. (2011). Cortical plasticity induced by short-term multimodal musical rhythm training. PLoS One 6:e21493. doi: 10.1371/journal.pone.0021493
Lee, Y.-S., Janata, P., Frost, C., Hanke, M., and Granger, R. (2011). Investigation of melodic contour processing in the brain using multivariate pattern-based fMRI. Neuroimage 57, 293–300. doi: 10.1016/j.neuroimage.2011.02.006
Lee, N. R., Raznahan, A., Wallace, G. L., Alexander-Bloch, A., Clasen, L. S., Lerch, J. P., et al. (2014a). Anatomical coupling among distributed cortical regions in youth varies as a function of individual differences in vocabulary abilities. Hum. Brain Mapp. 35, 1885–1895. doi: 10.1002/hbm.22299
Lee, N. R., Wallace, G. L., Raznahan, A., Clasen, L. S., and Giedd, J. N. (2014b). Trail making test performance in youth varies as a function of anatomical coupling between the prefrontal cortex and distributed cortical regions. Front. Psychol. 5:496. doi: 10.3389/fpsyg.2014.00496
Legge, R. M., Sendi, S., Cole, J. H., Cohen-Woods, S., Costafreda, S. G., Simmons, A., et al. (2015). Modulatory effects of brain-derived neurotrophic factor Val66Met polymorphism on prefrontal regions in major depressive disorder. Br. J. Psychiatry 206, 379–384. doi: 10.1192/bjp.bp.113.143529
Lerch, J. P., and Evans, A. C. (2005). Cortical thickness analysis examined through power analysis and a population simulation. Neuroimage 24, 163–173. doi: 10.1016/j.neuroimage.2004.07.045
Lerch, J. P., Worsley, K., Shaw, W. P., Greenstein, D. K., Lenroot, R. K., Giedd, J., et al. (2006). Mapping anatomical correlations across cerebral cortex (MACACC) using cortical thickness from MRI. Neuroimage 31, 993–1003. doi: 10.1016/j.neuroimage.2006.01.042
Li, G., He, H., Huang, M., Zhang, X., Lu, J., Lai, Y., et al. (2015). Identifying enhanced cortico-basal ganglia loops associated with prolonged dance training. Sci. Rep. 5:10271. doi: 10.1038/srep10271
Lövdén, M., Wenger, E., Mårtensson, J., Lindenberger, U., and Bäckman, L. (2013). Structural brain plasticity in adult learning and development. Neurosci. Biobehav. Rev. 37, 2296–2310. doi: 10.1016/j.neubiorev.2013.02.014
Luo, C., Guo, Z., Lai, Y., Liao, W., Liu, Q., Kendrick, K., et al. (2012). Musical training induces functional plasticity in perceptual and motor networks: insights from resting-state fMRI. PLoS One 7:e36568. doi: 10.1371/journal.pone.0036568
Lyttelton, O., Boucher, M., Robbins, S., and Evans, A. (2007). An unbiased iterative group registration template for cortical surface analysis. Neuroimage 34, 1535–1544. doi: 10.1016/j.neuroimage.2006.10.041
MacDonald, D., Kabani, N., Avis, D., and Evans, A. C. (2000). Automated 3-D extraction of inner and outer surfaces of cerebral cortex from MRI. Neuroimage 12, 340–356. doi: 10.1006/nimg.1999.0534
Malouin, F., Richards, C. L., Jackson, P. L., Dumas, F., and Doyon, J. (2003). Brain activations during motor imagery of locomotor-related tasks: a PET study. Hum. Brain Mapp. 19, 47–62. doi: 10.1002/hbm.10103
Meister, I., Krings, T., Foltys, H., Boroojerdi, B., Müller, M., Töpper, R., et al. (2004). Playing piano in the mind—an fMRI study on music imagery and performance in pianists. Cogn. Brain Res. 19, 219–228. doi: 10.1016/j.cogbrainres.2003.12.005
Mizuguchi, N., Nakata, H., Hayashi, T., Sakamoto, M., Muraoka, T., Uchida, Y., et al. (2013). Brain activity during motor imagery of an action with an object: a functional magnetic resonance imaging study. Neurosci. Res. 76, 150–155. doi: 10.1016/j.neures.2013.03.012
Na, K.-S., Won, E., Kang, J., Chang, H. S., Yoon, H.-K., Tae, W. S., et al. (2016). Brain-derived neurotrophic factor promoter methylation and cortical thickness in recurrent major depressive disorder. Sci. Rep. 6:21089. doi: 10.1038/srep21089
Nigmatullina, Y., Hellyer, P. J., Nachev, P., Sharp, D. J., and Seemungal, B. M. (2015). The neuroanatomical correlates of training-related perceptuo-reflex uncoupling in dancers. Cereb. Cortex 25, 554–562. doi: 10.1093/cercor/bht266
Overby, L. (1990). Use of imagery by dance teachers: development and implementation of two research instruments. J. Phys. Educ. Recreat. Dance 61, 24–27. doi: 10.1080/07303084.1990.10606437
Palomar-García, M-Á., Zatorre, R. J., Ventura-Campos, N., Bueichekú, E., and Ávila, C. (2017). Modulation of functional connectivity in auditory-motor networks in musicians compared with nonmusicians. Cereb. Cortex 27, 2768–2778. doi: 10.1093/cercor/bhw120
Petrides, M. (2000). The role of the mid-dorsolateral prefrontal cortex in working memory. Exp. Brain Res. 133, 44–54. doi: 10.1007/s002210000399
Petrides, M. (2005). Lateral prefrontal cortex: architectonic and functional organization. Philos. Trans. R. Soc. Lond. B Biol. Sci. 360, 781–795. doi: 10.1098/rstb.2005.1631
Pilgramm, S., Lorey, B., Stark, R., Munzert, J., Vaitl, D., and Zentgraf, K. (2010). Differential activation of the lateral premotor cortex during action observation. BMC Neurosci. 11:89. doi: 10.1186/1471-2202-11-89
Raznahan, A., Cutter, W., Lalonde, F., Robertson, D., Daly, E., Conway, G. S., et al. (2010). Cortical anatomy in human X monosomy. Neuroimage 49, 2915–2923. doi: 10.1016/j.neuroimage.2009.11.057
Reid, A. T., Lewis, J., Bezgin, G., Khundrakpam, B., Eickhoff, S. B., Mcintosh, A. R., et al. (2016). A cross-modal, cross-species comparison of connectivity measures in the primate brain. Neuroimage 125, 311–331. doi: 10.1016/j.neuroimage.2015.10.057
Robbins, S., Evans, A. C., Collins, D. L., and Whitesides, S. (2004). Tuning and comparing spatial normalization methods. Med. Image. Anal. 8, 311–323. doi: 10.1016/j.media.2004.06.009
Rowe, J., Toni, I., Josephs, O., Frackowiak, R. S. J., and Passingham, R. E. (2000). The prefrontal cortex: response selection or maintenance within working memory? Science 288, 1656–1660. doi: 10.1126/science.288.5471.1656
Rüber, T., Lindenberg, R., and Schlaug, G. (2015). Differential adaptation of descending motor tracts in musicians. Cereb. Cortex 25, 1490–1498. doi: 10.1093/cercor/bht331
Sauvage, C., Jissendi, P., Seignan, S., Manto, M., and Habas, C. (2013). Brain areas involved in the control of speed during a motor sequence of the foot: real movement versus mental imagery. J. Neuroradiol. 40, 267–280. doi: 10.1016/j.neurad.2012.10.001
Schlaug, G. (2001). The brain of musicians: a model for functional and structural adaptation. Ann. N Y Acad. Sci. 930, 281–299. doi: 10.1111/j.1749-6632.2001.tb05739.x
Schlaug, G. (2015). Musicians and music making as a model for the study of brain plasticity. Prog. Brain Res. 217, 37–55. doi: 10.1016/bs.pbr.2014.11.020
Schneider, P., Scherg, M., Dosch, H. G., Specht, H. J., Gutschalk, A., and Rupp, A. (2002). Morphology of Heschl’s gyrus reflects enhanced activation in the auditory cortex of musicians. Nat. Neurosci. 5, 688–694. doi: 10.1007/978-3-662-04874-0
Sharda, M., Foster, N. E. V., Tryfon, A., Doyle-Thomas, K. A. R., Ouimet, T., Anagnostou, E., et al. (2017). Language ability predicts cortical structure and covariance in boys with autism spectrum disorder. Cereb. Cortex 27, 1849–1862. doi: 10.1093/cercor/bhw024
Sharda, M., Khundrakpam, B. S., Evans, A. C., and Singh, N. C. (2016). Disruption of structural covariance networks for language in autism is modulated by verbal ability. Brain Struct. Funct. 221, 1017–1032. doi: 10.1007/s00429-014-0953-z
Sled, J. G., Zijdenbos, A. P., and Evans, A. C. (1998). A non-parametric method for automatic correction of intensity non-uniformity in MRI data. IEEE Trans. Med. Imaging 17, 87–97. doi: 10.1109/42.668698
Song, X., Quan, M., Lv, L., Li, X., Pang, L., Kennedy, D., et al. (2015). Decreased cortical thickness in drug naïve first episode schizophrenia: in relation to serum levels of BDNF. J. Psychiatr. Res. 60, 22–28. doi: 10.1016/j.jpsychires.2014.09.009
Steele, C. J., Bailey, J. A., Zatorre, R. J., and Penhune, V. B. (2013). Early musical training and white-matter plasticity in the corpus callosum: evidence for a sensitive period. J. Neurosci. 33, 1282–1290. doi: 10.1523/JNEUROSCI.3578-12.2013
Tachibana, A., Noah, J. A., Bronner, S., Ono, Y., and Onozuka, M. (2011). Parietal and temporal activity during a multimodal dance video game: an fNIRS study. Neurosci. Lett. 503, 125–130. doi: 10.1016/j.neulet.2011.08.023
Tohka, J., Zijdenbos, A., and Evans, A. (2004). Fast and robust parameter estimation for statistical partial volume models in brain MRI. Neuroimage 23, 84–97. doi: 10.1016/j.neuroimage.2004.05.007
Ubaldi, S., Barchiesi, G., and Cattaneo, L. (2015). Bottom-up and top-down visuomotor responses to action observation. Cereb. Cortex 25, 1032–1041. doi: 10.1093/cercor/bht295
Voss, P., and Zatorre, R. J. (2015). Early visual deprivation changes cortical anatomical covariance in dorsal-stream structures. Neuroimage 108, 194–202. doi: 10.1016/j.neuroimage.2014.12.063
Washburn, A., DeMarco, M., de Vries, S., Ariyabuddhiphongs, K., Schmidt, R. C., Richardson, M. J., et al. (2014). Dancers entrain more effectively than non-dancers to another actor’s movements. Front. Hum. Neurosci. 8:800. doi: 10.3389/fnhum.2014.00800
Wriessnegger, S. C., Steyrl, D., Koschutnig, K., and Müller-Putz, G. R. (2016). Cooperation in mind: motor imagery of joint and single actions is represented in different brain areas. Brain Cogn. 109, 19–25. doi: 10.1016/j.bandc.2016.08.008
Yang, X., Liu, P., Sun, J., Wang, G., Zeng, F., Yuan, K., et al. (2012). Impact of brain-derived neurotrophic factor Val66Met polymorphism on cortical thickness and voxel-based morphometry in healthy Chinese young adults. PLoS One 7:e37777. doi: 10.1371/journal.pone.0037777
Zatorre, R. J., Fields, R. D., and Johansen-Berg, H. (2012). Plasticity in gray and white: neuroimaging changes in brain structure during learning. Nat. Neurosci. 15, 528–536. doi: 10.1038/nn.3045
Zhao, L., Wang, E., Zhang, X., Karama, S., Khundrakpam, B., Zhang, H., et al. (2015). Cortical structural connectivity alterations in primary insomnia: insights from MRI-based morphometric correlation analysis. Biomed Res. Int. 2015:817595. doi: 10.1155/2015/817595
Keywords: dance, music, gray matter, structural covariance, dorsolateral prefrontal cortex
Citation: Karpati FJ, Giacosa C, Foster NEV, Penhune VB and Hyde KL (2018) Structural Covariance Analysis Reveals Differences Between Dancers and Untrained Controls. Front. Hum. Neurosci. 12:373. doi: 10.3389/fnhum.2018.00373
Received: 06 December 2017; Accepted: 30 August 2018;
Published: 25 September 2018.
Edited by:
Jinhui Wang, South China Normal University, ChinaReviewed by:
Yuanchao Zhang, University of Science and Technology of China, ChinaCopyright © 2018 Karpati, Giacosa, Foster, Penhune and Hyde. This is an open-access article distributed under the terms of the Creative Commons Attribution License (CC BY). The use, distribution or reproduction in other forums is permitted, provided the original author(s) and the copyright owner(s) are credited and that the original publication in this journal is cited, in accordance with accepted academic practice. No use, distribution or reproduction is permitted which does not comply with these terms.
*Correspondence: Krista L. Hyde, a3Jpc3RhLmh5ZGVAdW1vbnRyZWFsLmNh
Disclaimer: All claims expressed in this article are solely those of the authors and do not necessarily represent those of their affiliated organizations, or those of the publisher, the editors and the reviewers. Any product that may be evaluated in this article or claim that may be made by its manufacturer is not guaranteed or endorsed by the publisher.
Research integrity at Frontiers
Learn more about the work of our research integrity team to safeguard the quality of each article we publish.