- 1Danish Research Centre for Magnetic Resonance (DRCMR), Centre for Functional and Diagnostic Imaging and Research, Copenhagen University Hospital Hvidovre, Hvidovre, Denmark
- 2San Camillo Hospital IRCCS, Venice, Italy
- 3Department of Neurology, Copenhagen University Hospital Bispebjerg, Copenhagen, Denmark
- 4Queensland Brain Institute, The University of Queensland, Brisbane, QLD, Australia
The ability to rapidly adjust our actions to changes in the environment is a key function of human motor control. Previous work implicated the dorsal premotor cortex (dPMC) in the up-dating of action plans based on environmental cues. Here we used electroencephalography (EEG) to identify neural signatures of up-dating cue-action relationships in the dPMC and connected frontoparietal areas. Ten healthy subjects performed a pre-cued alternate choice task. Simple geometric shapes cued button presses with the right or left index finger. The shapes of the pre-cue and go-cue differed in two third of trials. In these incongruent trials, the go-cue prompted a re-evaluation of the pre-cued action plan, slowing response time relative to trials with identical cues. This re-evaluation selectively increased theta band activity without modifying activity in alpha and beta band. Source-based analysis revealed a widespread theta increase in dorsal and mesial frontoparietal areas, including dPMC, supplementary motor area (SMA), primary motor and posterior parietal cortices (PPC). Theta activity scaled positively with response slowing and increased more strongly when the pre-cue was invalid and required subjects to select the alternate response. Together, the results indicate that theta activity in dPMC and connected frontoparietal areas is involved in the re-adjustment of cue-induced action tendencies.
Highlights
- Incongruent go-cues slow down response time in a pre-cued alternate-choice task.
- Response slowing results from the need to re-evaluate the cue and its associated action.
- Re-evaluation is characterized by a selective increase in frontoparietal theta band activity.
- The dynamics of theta activity in left dPMC scales with response slowing.
Introduction
In everyday life, we constantly adjust our actions according to external cues, enabling flexible adjustments to changes in the environment. A sudden change in the environment may prompt us to reconsider our action plans. This re-evaluation may lead to a confirmation of the planned action or to the selection of an alternative action. A bilateral dorsal and mesial frontoparietal network has been implicated in the re-evaluation of pre-planned actions based on external cues, including premotor cortex (PMC), posterior parietal cortex (PPC), supplementary motor area (SMA) and medial prefrontal cortex (Paus, 2001; Monsell, 2003; Nachev et al., 2008; Mars et al., 2009; Buch et al., 2010; Neubert et al., 2010; O’Doherty, 2011; Hartwigsen et al., 2012; Mutha et al., 2014). The dorsal PMC (dPMC) plays a key role in the updating of pre-planned actions and non-routine stimulus-response mapping (Ward et al., 2010; Hartwigsen et al., 2012; Moisa et al., 2012; Hartwigsen and Siebner, 2015). The role of the left dPMC (L dPMC) in cue-based adjustments of action selection has also been studied invasively in behaving animals, exploring preparatory activity of small neuronal populations during trained sensorimotor tasks (Mushiake et al., 1991; Cisek and Kalaska, 2005). The dPMC is also relevant to functional recovery in patients with motor paresis after stroke (Seitz et al., 1998; Johansen-Berg et al., 2002; Fridman et al., 2004; Di Pino et al., 2014). In humans, the involvement of dPMC in re-evaluation of existing action plans has been studied with functional brain mapping techniques, such as functional magnetic resonance imaging (fMRI; Picard and Strick, 2001; Rae et al., 2014) or electroencephalography (EEG; Gratton et al., 1990; Eimer et al., 1995; Verleger et al., 2009). Others have used focal transcranial magnetic stimulation (TMS) to perturb neural processing, trace changes in cortical excitability, or interface TMS with fMRI (Koch et al., 2006; O’Shea et al., 2007; Kroeger et al., 2010; Ward et al., 2010; Duque et al., 2012; Hartwigsen et al., 2012; Bestmann and Duque, 2015).
Although it is widely accepted that brain oscillations make critical contributions to action selection and control (Humphries et al., 2006; Tombini et al., 2009; Deiber et al., 2012; Cheyne, 2013; Brittain and Brown, 2014), it remains to be clarified which oscillatory activity patterns emerge in the dPMC and connected frontoparietal brain regions during cue-induced re-evaluation of pre-planned actions.
In this EEG study, we recorded cortical oscillatory activity during a novel pre-cued alternate-choice task. Simple geometric shapes cued button presses with the right or left index finger. The shapes of the pre-cue and go-cue differed in two third of trials. Partially incongruent go-cues required a re-evaluation of the motor plan, but no change of the previously planned motor output (motor re-evaluation). Incongruent go-cues required a re-evaluation of the go-cue and its associated action (motor reprogramming). We hypothesized that those trials requiring a re-evaluation of cue-action associations would show an increase in oscillatory activity during the response period.
We expected that the increase in activity would be most prominent in the dPMC and interconnected frontoparietal areas and would positively correlate with the slowing in response time.
Although action re-evaluation and motor reprogramming also depend on the activity and cortical regions localized deep near the longitudinal fissure, such as anterior cingulate cortex (ACC), and subcortical structures such as the subtalamic nucleus (STN; Wessel and Aron, 2017), we only focused on the cortical surface to which EEG is sensitive and accurate.
Materials and Methods
Participants and Study Design
Ten healthy volunteers participated in the study. All participants but one were right handed (Oldfield, 1971). None was under medications or had history of neurological or psychiatric disorders. The protocol was approved by the Ethics Committee of the Capital Region of Denmark. All subjects gave written informed consent in accordance with the Declaration of Helsinki. Subjects performed a visually precued, bimanual two-choice reaction time (RT) task (Figure 1). Two shapes (square and circle) were associated to the movement of the left finger, a triangle and a diamond to the movement of the right finger. One of these four cues were randomly presented on the screen (PreCue). Each shape of the PreCue could be followed (GoCue) by the same shape (Fully Congruent, 33%), a different shape triggering the movement of the same side (Partially Incongruent, 33%) and a different shape triggering the movement of the opposite side (Fully Incongruent, 33%). Hence, in 66% of the trials the PreCue validly predicted the movement side (Fully Congruent + Partially Incongruent). In further details, this task allowed to assess the cortical mechanisms related to motor re-evaluation (Partially Incongruent vs. Fully Congruent) and motor reprogramming (Fully Incongruent).
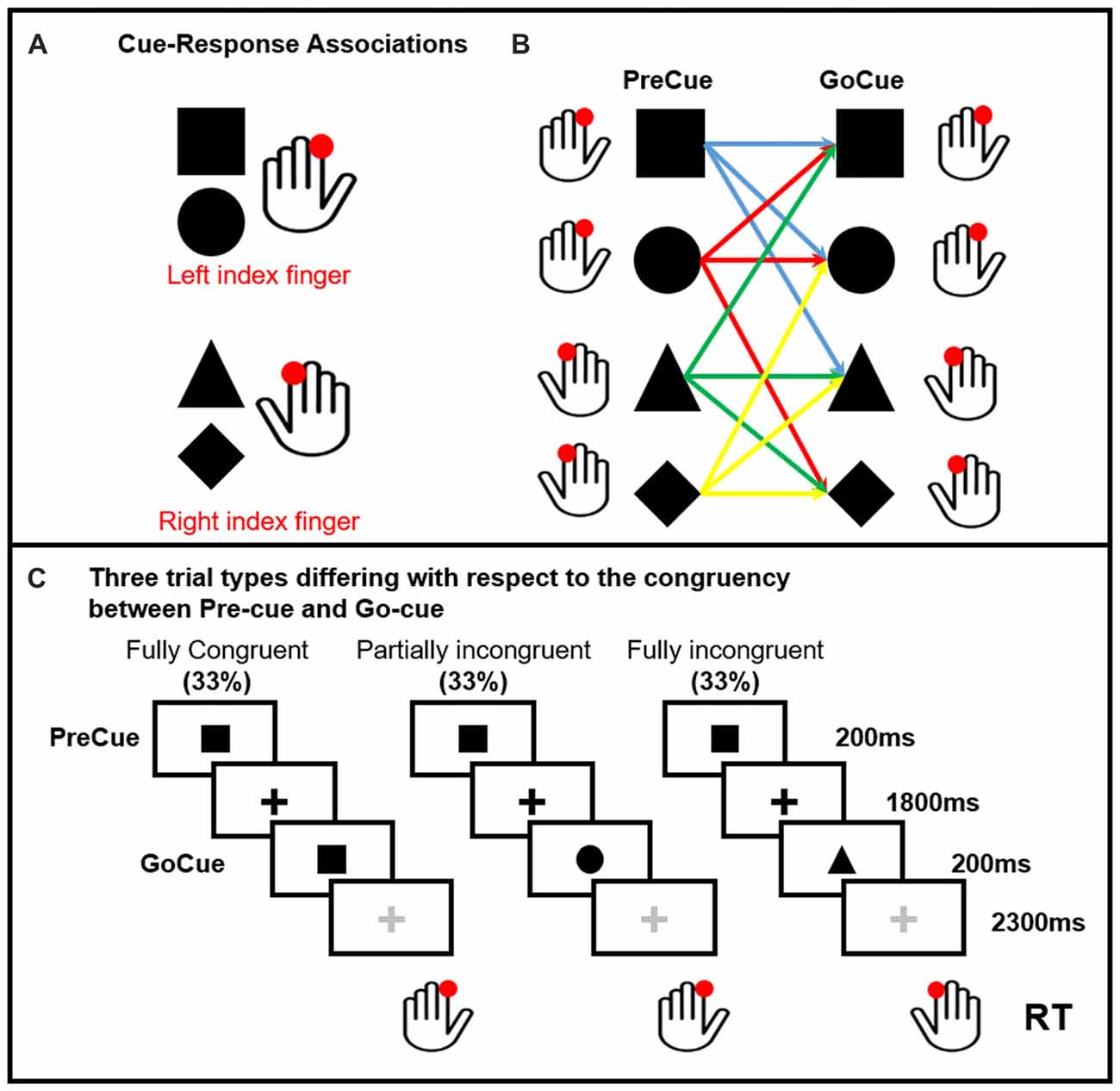
Figure 1. Pre-cued alternate-choice task. Participants were asked to respond as fast as possible to a GoCue with a correct button press. Arbitrary shape-response associations were learned by the participants prior to the experiment. Panel (A) Cue-response associations: the square and circle required a button press with the left index finger. The triangle and diamond required a button press with the right index finger. The cue-response associations were varied across individuals, but kept constant within subjects. Panel (B) shows all the possible PreCue GoCue combinations. Panel (C) Trial structure: a PreCue appeared for 200 ms on the screen and predicted the movement with a probability of 66%. A GoCue appeared 1,800 ms after the PreCue had disappeared and was presented for 200 ms. In one third of the trials, PreCue and GoCue were identical (i.e., fully congruent trials). Hence, the PreCue predicted the GoCue and the action. In 33% of trials the shape of the GoCue differed from the shape of the PrCue, but the GoCue instructed the same movement as the PreCue (i.e., partially incongruent trials). Hence, the altered go-cue required only a re-evaluation of the cue but no change in selection of the pre-cued response. In the last third of the trials, the PreCue and GoCue differed in shape and coded different actions (i.e., fully incongruent trials). In this trial condition, not only the go-cue needed to be re-evaluated, but also the pre-cued response needed to be suppressed and the alternate response had to be selected (i.e., motor reprogramming).
The task consisted of five runs of 120 trials each (600 trials in total, 200 per condition). The PreCue and GoCue appeared for 200 ms each. The PreCue-GoCue inter-stimulus interval was 2 s, while the GoCue-PreCue interval was 2.5 s. A fixation cross was presented all along the experiment. The color of the fixation cross was black in the PreCue-GoCue interval and gray in the GoCue-PreCue period. The shapes and the fixation cross had all the same size. The subjects were instructed to perform a button press with their left or right index finger (“A” and “L” buttons of a computer keyboard respectively) as quickly and accurately as possible. Stimulus presentation and response recordings were obtained using Psychopy software (Peirce, 2007, 2008). EEG data were acquired all along the experiment. As the overall goal of the study was to investigate the mechanisms linked to motor reprogramming, the analysis was focused on data aligned to the GoCue.
EEG Recording and Analysis
EEG was acquired from 64 electrodes mounted on an elastic cap (Easycap). Data were sampled at 5,000 Hz, amplified using a BrainAmp MRI plus system and recorded using the BrainVision Recorder (Brain Products GmbH, Germany). Impedances of all electrodes were kept below 5 kΩ. Pre-processing was performed using EEGLAB (Delorme and Makeig, 2004) and in-house code (MATLAB R2014b, The Mathworks, Natick, MA, USA) and included the following steps: (a) downsampling to 1,000 Hz; (b) band-pass filter (0.3–200 Hz); (c) notch filter (49–51 Hz); (d) visual inspection to remove bad channels; (e) average re-referencing; (f) data epoching (epochs of 2.45 s, from −1.7 s to 0.75 s after the GoCue); (g) visual rejection of bad epochs; (h) independent component analysis (ICA) to remove artifacts, such as eye movements, eye blinks, muscle artifact; and (i) interpolation of bad channels using spherical splines. Preliminary analysis aimed at identifying the frequency band relevant to the task focusing on the time interval from 0 ms to 500 ms after the GoCue and on theta (3–6 Hz), alpha (8–13 Hz), beta (15–30 Hz) and gamma (30–60 Hz) bands. The time-frequency (TF) decomposition was performed at sensor level using FFT and Hanning window tapering (500 ms with 90% overlap), setting time-steps of 100 ms. To avoid border effects and achieve a robust estimation of the low frequency activity, frequency decomposition was applied on a wider time-window, spanning between −1,700 ms and 750 ms, relative to onset of the GoCue. The activity of interest (0–500 ms) was normalized considering a PreCue baseline (−1,000 to 0 ms). For the frequency bands significantly different across conditions a source imaging analysis was performed using Brainstorm (Tadel et al., 2011). This assessment aimed at identifying the cortical regions relevant to motor reprogramming, the relationship between brain activity originating in such regions and RT and the time dynamic of the generation of brain oscillations. The head model for the source imaging was built from an anatomical template (Colin27). Cortical surface was reconstructed via Freesurfer and tessellated into 8,000 vertices (Dale et al., 1999). The forward model was computed applying the OpenMEEG Boundary Element Method (BEM; Gramfort et al., 2010), with three layers and a conductivity of 0.33 S/m for the brain, 0.165 S/m for the skull and 0.33 S/m for the skin (brain-to-skull ratio: 1/20; Pellegrino et al., 2016a,b, 2018; von Ellenrieder et al., 2016; Hedrich et al., 2017). The inverse problem was solved by using a whitened and depth-weighted linear L2-minimum norm estimate, with the dipole orientations constrained to be normal to the cortex. A common imaging Kernel was applied to compute single trial cortical reconstructions. For each trial and each cortical vertex, the TF decomposition was estimated from 0 ms to 500 ms and from 3 Hz to 6 Hz (this was the only frequency band significantly different across conditions at the preliminary sensor-level analysis, see “Results” section). The following regions of interest (ROIs) were manually identified on the cortical surface according to previous literature: Bilateral Medial Prefrontal Cortex (MPFC; motor part; Paus, 2001; Ramnani and Owen, 2004), Left and Right Dorsolateral PreFrontal (DLPF) Cortex (Simons and Spiers, 2003), Bilateral Rostral SMA, Bilateral Ventral SMA (Paus, 2001; Nachev et al., 2008), Left and Right primary motor cortex (M1; Yousry et al., 1997), Left and Right dorsal and ventral PMC (Mayka et al., 2006), Left and Right Posterior Parietal, Left and Right inferior frontal gyrus (IFG; Petrides and Pandya, 2002). All these regions are well-known for being engaged in motor reprogramming and are compatible with the topographical distribution of the effect found on sensor level (see “Results” section). The TF of all vertices belonging to each ROIs was then averaged. As compared to applying TF decomposition to the average time-course of the ROIs, this procedure is more robust and potentially less prone to bias related to EEG cancellation phenomena due to cortical folding (Chowdhury et al., 2018). Although ACC and the STN are two key players in action re-evaluation and motor reprogramming, as well as in conflict solving (Botvinick et al., 2004; Sohn et al., 2007), we did not place a seed in these regions as the accuracy of distributed magnetic source imaging in estimating deep sources is very low and questionable (Koessler et al., 2015; Pellegrino et al., 2018).
Statistics
Behavioral data were analyzed using IBM SPSS Statistics v22. Analysis of RT was carried out with a Repeated Measure ANOVA. The rate of errors was compared across conditions using a Chi-Square test. For the preliminary analysis on sensor level, the frequency content of the three Conditions (Fully Congruent, Partially Incongruent, Fully Incongruent) were compared using the paired parametric approach embedded in EEGLab. The effect of brain oscillations on motor performance was assessed using linear regressions performed with IBM SPSS Statistics v22 on data extracted from Brainstorm source imaging, as further explained in the “Results” section. The significant level was set to p < 0.05.
Results
Motor Performance
We first present the analysis of the motor performance with a focus on two measures: RT and error rate.
When looking at RT, we found that it strongly depended on task difficulty (Figure 2). The repeated measure ANOVA with the factors Condition (Fully Congruent, Partially Congruent, Fully Incongruent) and Side (Dominant and Non-dominant) demonstrated a RT differences across conditions (F(2,18) = 48.840, p < 0.001) but no significant difference between sides (Factor Side and Side by Condition interaction: p > 0.200 consistently). The post hoc analysis confirmed that the RT was higher for Fully Incongruent vs. Partially Incongruent (p = 0.001) and Partially Incongruent vs. Fully Congruent (p < 0.001).
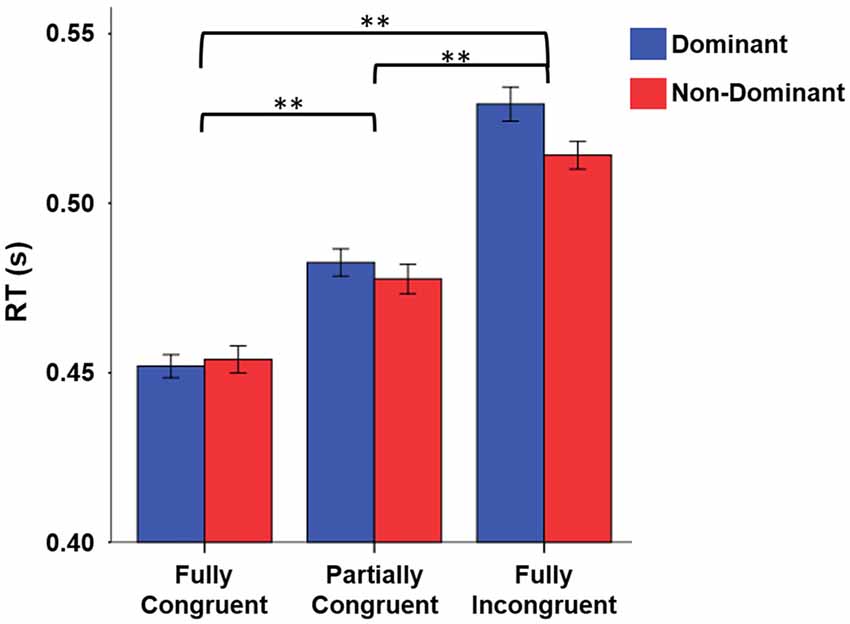
Figure 2. Reaction time (RT) differences by Condition by Side. RT was significantly higher for Fully Incongruent than Partially Congruent than Fully Congruent. No differences were found between Dominant and Non-Dominant side. **p < 0.001.
A similar pattern emerged for error rate. Fully Incongruent had more errors than Partially Incongruent and the latter more than Fully Congruent (Chi-Square 84.763, p < 0.001; Fully Incongruent 137 (6.85%), Partially Incongruent 60 (3.00%), Fully Congruent 36 (1.80%)). Similarly to RT, also in this case no significant difference was found between Sides (p > 0.05). The cumulative number of errors as well as the number of missed responses were overall quite low (224 (3.73%) and 37 (0.6%), respectively).
In summary, both measures confirmed that there was an increasing task difficulty, with Fully Incongruent being more challenging than Partially Incongruent and the latter more challenging than Fully Congruent. On the basis of these behavioral results, we focused our EEG analysis on the correct trials only and pooled together data of dominant and non-dominant hand.
EEG Analysis
The results of the sensor-level analysis are reported in Figure 3. They show that theta activity followed a similar pattern as motor performance. In details, Fully Incongruent displayed a significant higher theta than Partially Incongruent, and the latter had significantly more theta than Fully Congruent. Notably, this feature was very specific for theta, as none of the other frequency bands disclosed any significant difference across conditions.
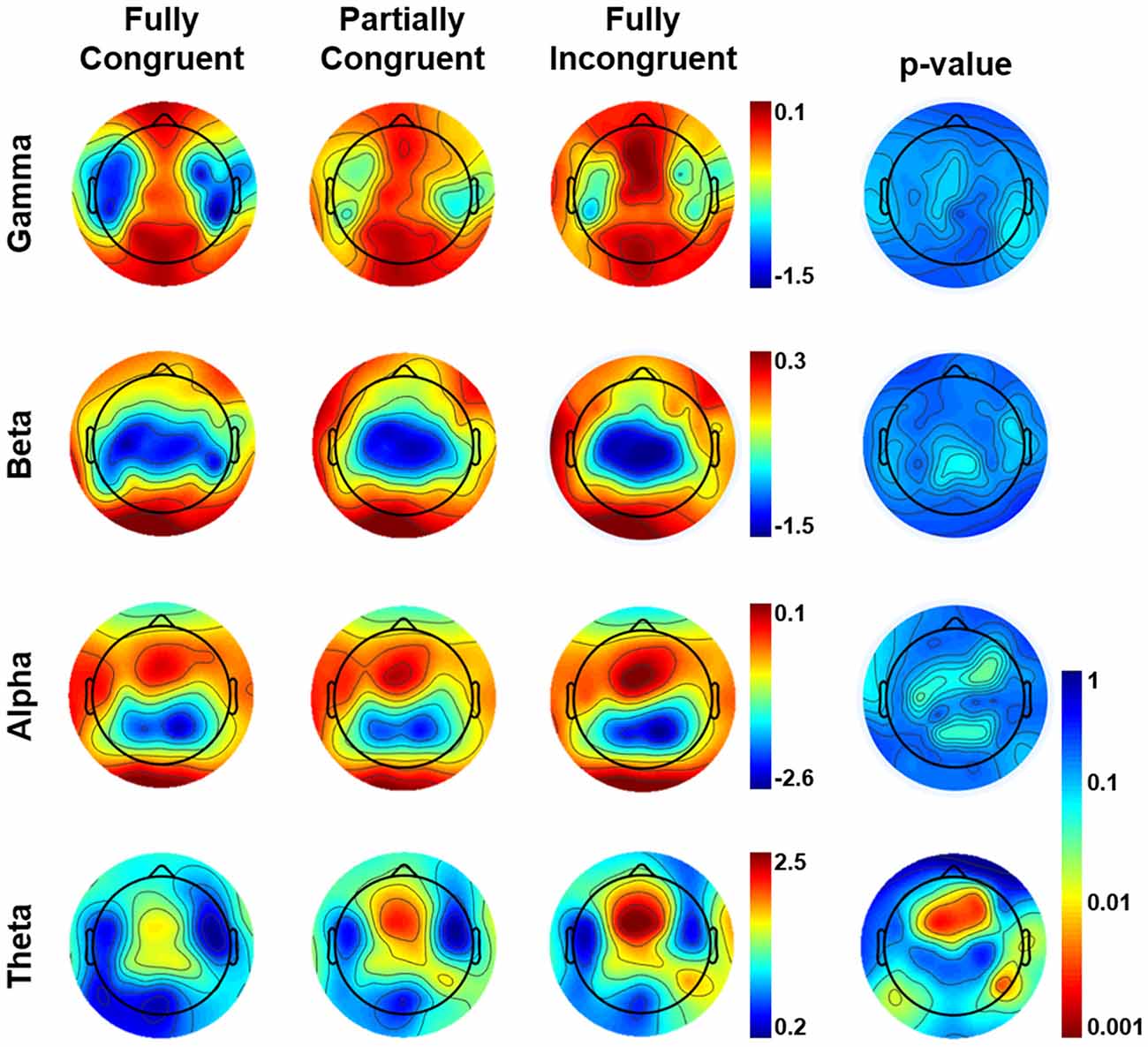
Figure 3. Topographical distribution of frequency power by Condition by frequency band in the (0–500) ms time-window of interest. The most-right column shows the Bonferroni corrected p-value of the comparison across conditions. From Fully Incongruent to Partially Congruent to Fully Congruent there is an increasing amount of theta activity. To be noted that no significant differences were found for other frequencies.
We followed-up on these findings with a source analysis restricted to the theta band. The main results are reported in Figure 4. The evaluation of theta temporal dynamic revealed that theta differences between conditions began before the movement onset (average RT from 450 ms to 520 ms for Fully Congruent and Fully Incongruent, respectively). Motor reprogramming (Fully Incongruent vs. Fully Congruent) was characterized by higher theta in multiple regions, and especially in dPMC, supplementary motor cortex and PPC. Conversely, motor re-evaluation (Partially Incongruent vs. Fully Congruent) was characterized by higher dPMC and supplementary motor theta activity.
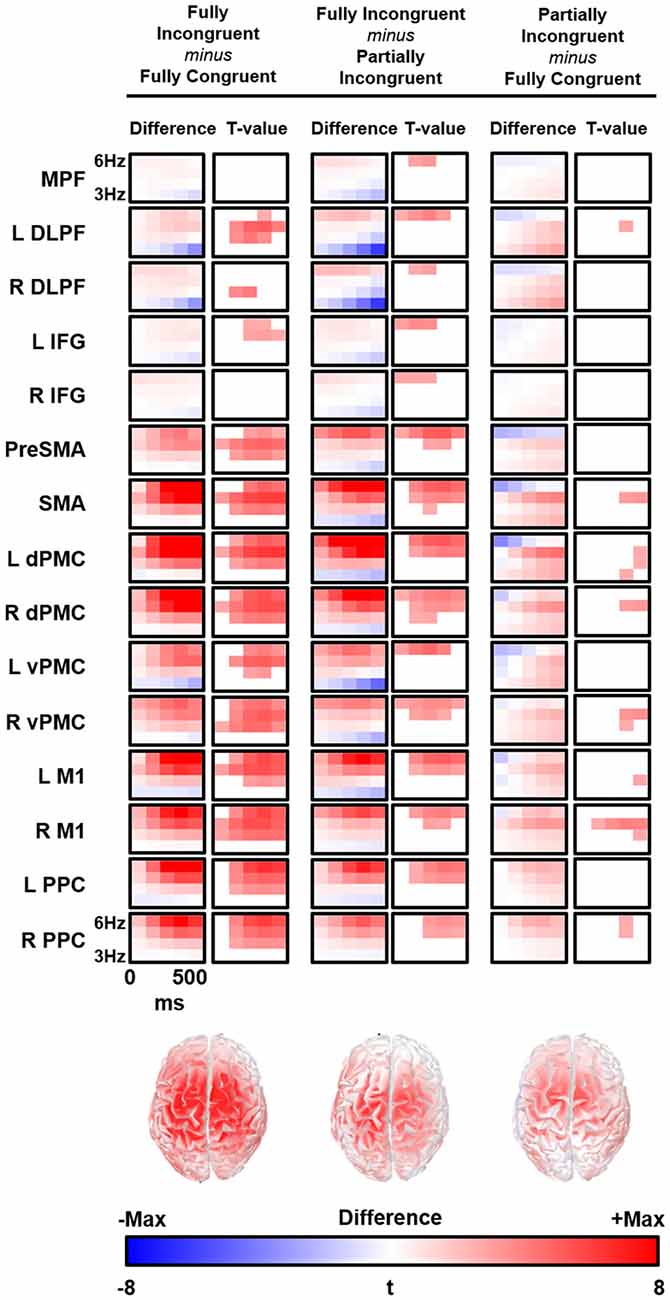
Figure 4. Time-frequency (TF) difference (left column) and the corresponding t-map (right column) is shown for each regions of interest (ROIs). The maps of the t-value are thresholded at Bonferroni corrected p < 0.05. Bottom line shows the cortical distribution of theta activity difference between conditions at 300 ms and 5 Hz. R, Right; L, Left; MPFC, Bilateral Medial PreFrontal Cortex; DLPF, Dorsolateral PreFrontal; IFG, Inferior Frontal Gyrus; PreSMA, Bilteral Pre Supplementary Motor Area; SMA, Bilateral Supplementary Motor Area; dPMC, Dorsal PreMotor Cortex; vPMC, Ventral PreMotor Cortex; M1, Primary Motor Cortex; PPC, Posterior Parietal Cortex.
Effect of Theta Activity on RT
As we had found that more challenging conditions (motor re-evaluation and motor reprogramming) had longer response times and higher theta activity arising from multiple cortical regions, we were interested to find whether this relationship also explained inter-individual variability in RT within a given experimental condition. Therefore, we performed condition-specific analysis to test for a link between differences in motor performance and differences in theta activity for each experimental condition and explored in which cortical areas the condition-specific expression of theta activity scaled with motor performance.
We conducted a multiple linear regression analysis with RT differences between conditions as dependent variable and two predictors: the three experimental conditions CondDiff and t-scores of the theta of the ROIs. CondDiff had three levels: (a) Partially Incongruent minus Fully Congruent; (b) Fully Incongruent minus Partially Incongruent; and (c) Fully Incongruent minus Fully Congruent. Whereas CondDiff was pushed into the model, t-values of all ROIs were added in a stepwise fashion. CondDiff alone explained about 40% of RT variance (F = 10.414, p < 0.001). L dPMC was the only region improving significantly the model (about 60% of variance explained; F = 14.952, p < 0.001; Standardized beta = 0.468, t = 3.742, p = 0.001), thus suggesting that differences in motor performance scale with difference in L dPMC theta. Therefore, this model provided strong evidence that L dPMC, which was the target region of our task and experimental design, was a main driver of motor performance. To be noted, no other regions gave a significant additional contribution to explain differences in RT. In more details, the positive beta coefficient denoted that the higher was the difference in theta, the higher was the difference in performance between conditions (Figure 5). We also fitted an additional model with an interaction term, but no significant differences were found. This additional finding revealed that the relationship between L dPMC and RT was similar across CondDiff levels.
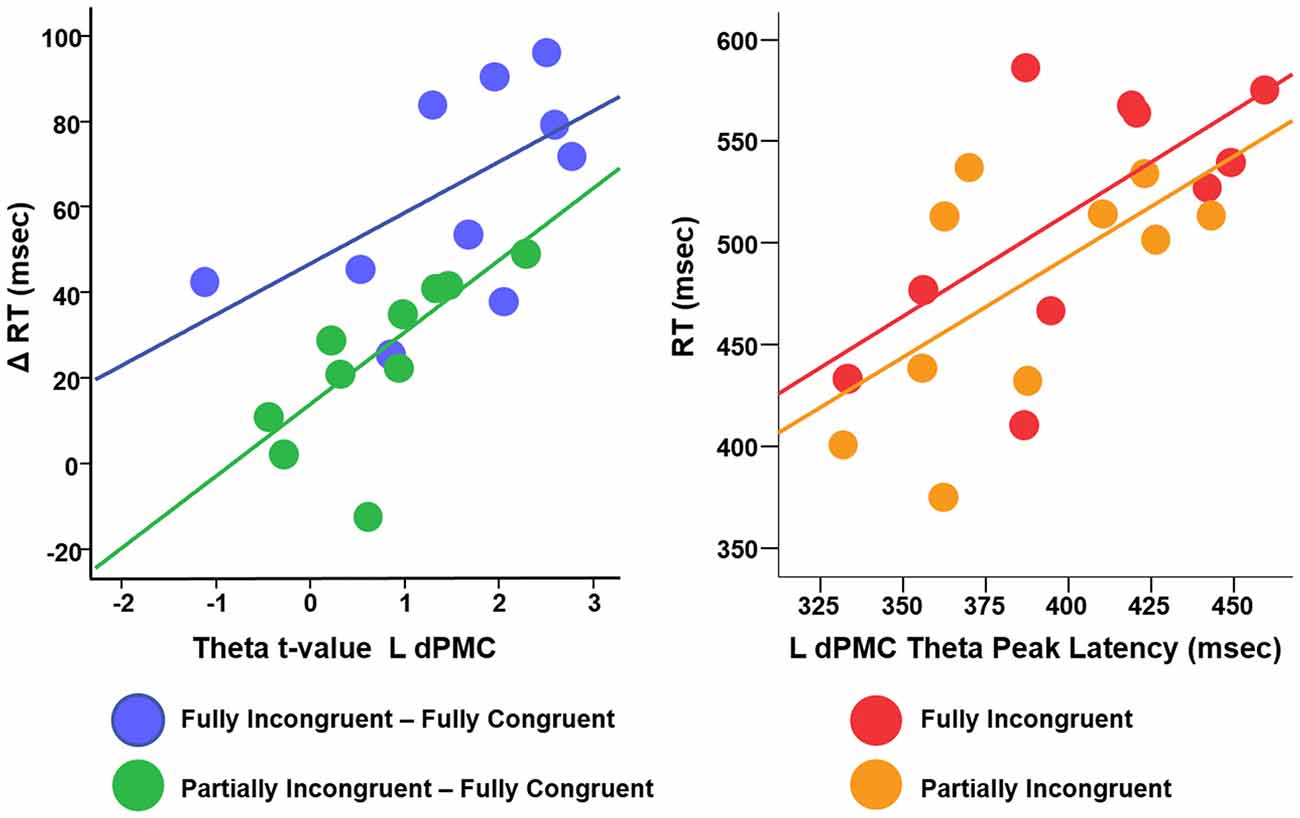
Figure 5. Relationship between difference RT cost (ΔRT) and theta t-values (left panel) and between RT and theta peak latency (right panel).
In summary, this analysis demonstrated that, although motor re-evaluation and motor-reprogramming are characterized by differences in theta band arising from dPMC and other interconnected regions, only theta activity in L dPMC was linearly related to motor performance. Therefore, the following analyses focus on L dPMC theta activity and further investigates how it relates to motor performance.
While we previously showed that differences in theta activity relate to differences in task performance, here we investigated whether the amount of theta had an impact on the motor performance within our three experimental conditions (Fully Congruent, Partially Incongruent, Fully Incongruent).
A linear regression with RT as dependent variable, Condition and L dPMC theta as predictors showed a negative association between RT and amount of theta oscillations (F = 4.090, p = 0.017; Standardized beta = −0.370, t = −2.29, p = 0.03), similar for the three conditions (interaction term p > 0.200). This analysis demonstrated that faster responses significantly depend on higher theta and that this relationship was similar for Fully Congruent; Partially Incongruent and Fully Incongruent.
We finally investigated the effect of theta temporal dynamic on motor performance. We defined as Peak Latency the average time (per Condition per Subject) when theta reached its peak in amplitude. Although the resolution of TF decomposition was set to 100 ms, Peak Latency could assume any value in the (0–500 ms) time window, being an average over many trials. We computed a linear regression with RT as dependent variable, Condition (three levels: Fully Congruent; Partially Incongruent; Fully Incongruent) and Peak Latency as predictors. This analysis revealed a significant and positive relationship between Peak Latency and RT (F = 8.23, p = 0.001, Adjusted R2 = 43%, Standardized beta = 0.569, t = 3.93, p = 0.001), suggesting that an earlier theta build-up scales with faster responses. Similarly to the previous analysis, also in this case the relationship did not significantly change across Conditions, as the interaction term was not significant (p > 0.200; Figure 5). In other words, for all the three conditions, earlier the theta in L dPMC reached its peak in L dPMC, faster was the response.
Discussion
In this study, using a novel pre-cued RT task, we were able to demonstrate that theta activity generated in the L dPMC is a neural signature of motor re-evaluation and motor re-programming. This finding is in good agreement with the notion of a dorsomedial visuomotor “action pathway,” as recently discussed by Gallivan and Goodale (2018).
With our novel task we wanted to disentangle the effect of motor reprogramming and motor re-evaluation. Action selection was therefore decomposed in three different components: a baseline consisting in releasing a previously prepared motor action (Fully Congruent), a motor re-evaluation where the subject had to reassure that the movement previously prepared could be released (Partially Incongruent) and an entire motor reprogramming when a cue signaled to execute a different movement (Fully Incongruent; Figure 1). We demonstrated that both motor re-evaluation and motor reprogramming have a behavioral cost (longer RT) and, more importantly, that this cost pairs differences in theta activity in L dPMC. Theta increase in motor re-evaluation and motor-reprogramming fully scaled with the RT costs.
Theta activity over the fronto-central cortical regions has been traditionally called midline theta and seemed to be largely generated in the medial prefrontal cortex (Ishii et al., 1999). It often occurs in conjunction with cortical synchronization in other frequency bands (Mizuki et al., 1982; Tombini et al., 2009) and it has been often interpreted as a diffuse and non-specific activation. It increases in a number of tasks with a relevant cognitive load (Nigbur et al., 2011), ranging from internalized attention and positive emotional states (Aftanas and Golocheikine, 2001), to learning (Laukka et al., 1995), memory (Jensen and Tesche, 2002; Tombini et al., 2012; Hsieh and Ranganath, 2014), stimulus response conflicts (Luu et al., 2004; Nigbur et al., 2012) and spatial conflict processing (Cohen and Ridderinkhof, 2013). In the context of online action control, theta activity is expressed when overlearned sensorimotor response mapping is challenged by external cues and preferentially expressed in the dPMC. The relationship of this activity pattern to neural activity more generically implicated in conflict solving needs to be addressed in future studies that involve additional tasks which do not require a motor response.
Our study, however, revealed that, in the field of motor control, theta activity is both task and spatially specific. First, although motor control depends upon the modulation of oscillations at multiple frequencies (Neuper et al., 2009; Tombini et al., 2009; Pellegrino et al., 2012), in our study only theta was different across conditions, but no other frequency bands. Notably, the slope of the relationship between theta and motor performance did not significantly differ for motor re-evaluation and motor re-programming, suggesting that theta (but not other frequency bands) is functionally relevant when the cue-movement associative rule is non-routine (Figure 3).
Second, our source analysis revealed that, although theta is a signature generated over a large network covering multiple fronto-central-parietal regions, the activity mostly relevant to motor performance was generated in L dPMC. Previous studies demonstrated that theta can be generated locally, within the sensori-motor regions during the late stages of motor learning (Perfetti et al., 2011b), in the fronto-parietal cortex for motor planning and on-line motor adjustments (Perfetti et al., 2011a) and as signature of brain plasticity in both physiological and pathological conditions (Kirov et al., 2009; Hung et al., 2013; Assenza et al., 2015; Pellegrino et al., 2016c; Tombini et al., 2012). As our task was explicitly designed to engage L dPMC, which is well known for its role in motor control, we could infer that this specific region generates theta waves to perform both motor re-evaluation and motor reprogramming. In a more general perspective, and in comparison with the view of theta activity being a widespread and non-specific marker of cognitive load, we support the idea that theta oscillations can be generated locally and their functional role is closely dependent on the specific role of their generator. That said, it is not surprising that other cortical areas showed an increase of theta, as L dPMC is a key area in a large dorsal network subserving motor control and motor integration (Ward et al., 2010; Hartwigsen et al., 2012; Moisa et al., 2012; Giambattistelli et al., 2014; Hartwigsen and Siebner, 2015). It is therefore likely that theta activity across these areas improves sensory-motor integration (Tombini et al., 2009; Cohen and Ridderinkhof, 2013; Figure 5). This interpretation is further corroborated by the evaluation of the relationship between the dynamic of L dPMC theta and motor performance within experimental conditions. Indeed, motor performance depended on both the amount and temporal dynamic of theta generation: the higher was theta, the shorter was the RT; the earlier theta reached its peak the quicker was the movement (Figure 5). As the relationship was similar for all three conditions -including the Fully Congruent trials where there was no violation of the associative rule- we might infer on a general positive values of theta oscillations in improving motor performance. In other words, this might suggest that increased theta does not only compensate an increased cognitive load (Laukka et al., 1995; Jensen and Tesche, 2002; Nigbur et al., 2011; Hsieh and Ranganath, 2014), but is instrumental to an accurate and quick motor act.
The studies performed by the group of Rushworth in healthy subjects as well as patients affected by stroke, had already demonstrated that L dPMC is part of a network involved in the so called “motor attention.” This network comprises two key nodes: the L dPMC, which is mainly devoted to selection of movements, and the left anterior inferior parietal lobule and the posterior superior parietal lobule which would be more involved in the preparation and the redirection of movements and movement intentions. For instance, stroke patients with left hemispheric lesions often show higher BOLD activation in the healthy PMC and the strongest motor impairment when this region is temporarily inactivated via TMS (Rushworth et al., 2003; Rushworth and Taylor, 2006).
Some limitations of this study should be underlined. First, a partial limitation is that EEG source analysis was not based (seeded) on individual MRI imaging. The tomographic reconstruction of EEG activity revealed, however, very broad maps, in keeping with the knowledge that motor system is organized over large cortical regions and networks (Raffin et al., 2015). Second, we cannot rule out that some theta activity localized over cortical surface was in fact generated in deeper regions, such as ACC and STN. We did not directly explore these regions as they are too deep to be properly sampled by our source imaging approach. Third, the analysis of theta activity time-dynamic was eventually restricted to the region most relevant to the task. However, future investigations should pay devote more attention to the time-dynamic of topographical maps.
In conclusion, our data confirm the notion that PMC is implicated in action re-evaluation and reprogramming and its signature in terms of brain oscillations is in theta band. This theta activity might be a good target for brain stimulation or other interventions to improve action selection in a damaged brain.
Author Contributions
GP: experimental design, data acquisition, data analysis, data interpretation and manuscript preparation. LT: data analysis and manuscript revision. DH: experimental design, data interpretation and manuscript revision. KL: data acquisition. HS: experimental design, data acquisition, data interpretation, manuscript preparation and revision.
Funding
GP was funded by a Ministry of Health (Ministero della Salute) Operating Grant to the San Camillo IRCCS Venice. HS was supported by a synergy grant from the Novo Nordisk Foundation (Interdisciplinary Synergy Program 2014; grant number NNF14OC0011413) and a grant of excellence from the Lundbeck Foundation (Grant of Excellence “Mapping, Modulation and Modelling the Control of Actions”; grant number R59-A5399).
Conflict of Interest Statement
The authors declare that the research was conducted in the absence of any commercial or financial relationships that could be construed as a potential conflict of interest.
Acknowledgments
We acknowledge the generous contribution of the participants and the valuable support of Prof. Axel Thielscher and Dr. Norbert Brüggemann (experimental design) and Dr. Konrad Stanek (experimental set-up).
References
Aftanas, L., and Golocheikine, S. (2001). Human anterior and frontal midline theta and lower alpha reflect emotionally positive state and internalized attention: high-resolution EEG investigation of meditation. Neurosci. Lett. 310, 57–60. doi: 10.1016/s0304-3940(01)02094-8
Assenza, G., Pellegrino, G., Tombini, M., Di Pino, G., and Di Lazzaro, V. (2015). Wakefulness delta waves increase after cortical plasticity induction. Clin. Neurophysiol. 126, 1221–1227. doi: 10.1016/j.clinph.2014.09.029
Bestmann, S., and Duque, J. (2015). Transcranial magnetic stimulation decomposing the processes underlying action preparation. Neuroscientist 22, 392–405. doi: 10.1177/1073858415592594
Botvinick, M. M., Cohen, J. D., and Carter, C. S. (2004). Conflict monitoring and anterior cingulate cortex: an update. Trends Cogn. Sci. 8, 539–546. doi: 10.1016/j.tics.2004.10.003
Brittain, J.-S., and Brown, P. (2014). Oscillations and the basal ganglia: motor control and beyond. Neuroimage 85, 637–647. doi: 10.1016/j.neuroimage.2013.05.084
Buch, E. R., Mars, R. B., Boorman, E. D., and Rushworth, M. F. (2010). A network centered on ventral premotor cortex exerts both facilitatory and inhibitory control over primary motor cortex during action reprogramming. J. Neurosci. 30, 1395–1401. doi: 10.1523/JNEUROSCI.4882-09.2010
Cheyne, D. O. (2013). MEG studies of sensorimotor rhythms: a review. Exp. Neurol. 245, 27–39. doi: 10.1016/j.expneurol.2012.08.030
Chowdhury, R. A., Pellegrino, G., Aydin, Ü., Lina, J. M., Dubeau, F., Kobayashi, E., et al. (2018). Reproducibility of EEG-MEG fusion source analysis of interictal spikes: relevance in presurgical evaluation of epilepsy. Hum. Brain Mapp. 39, 880–901. doi: 10.1002/hbm.23889
Cisek, P., and Kalaska, J. F. (2005). Neural correlates of reaching decisions in dorsal premotor cortex: specification of multiple direction choices and final selection of action. Neuron 45, 801–814. doi: 10.1016/j.neuron.2005.01.027
Cohen, M. X., and Ridderinkhof, K. R. (2013). EEG source reconstruction reveals frontal-parietal dynamics of spatial conflict processing. PLoS One 8:e57293. doi: 10.1371/journal.pone.0057293
Dale, A. M., Fischl, B., and Sereno, M. I. (1999). Cortical surface-based analysis: I. Segmentation and surface reconstruction. Neuroimage 9, 179–194. doi: 10.1006/nimg.1998.0395
Deiber, M.-P., Sallard, E., Ludwig, C., Ghezzi, C., Barral, J., and Ibañez, V. (2012). EEG alpha activity reflects motor preparation rather than the mode of action selection. Front. Integr. Neurosci. 6:59. doi: 10.3389/fnint.2012.00059
Delorme, A., and Makeig, S. (2004). EEGLAB: an open source toolbox for analysis of single-trial EEG dynamics including independent component analysis. J. Neurosci. Methods 134, 9–21. doi: 10.1016/j.jneumeth.2003.10.009
Di Pino, G., Pellegrino, G., Assenza, G., Capone, F., Ferreri, F., Formica, D., et al. (2014). Modulation of brain plasticity in stroke: a novel model for neurorehabilitation. Nat. Rev. Neurol. 10, 597–608. doi: 10.1038/nrneurol.2014.162
Duque, J., Labruna, L., Verset, S., Olivier, E., and Ivry, R. B. (2012). Dissociating the role of prefrontal and premotor cortices in controlling inhibitory mechanisms during motor preparation. J. Neurosci. 32, 806–816. doi: 10.1523/JNEUROSCI.4299-12.2012
Eimer, M., Hommel, B., and Prinz, W. (1995). SR compatibility and response selection. Acta Psychol. 90, 301–313. doi: 10.1016/0001-6918(95)00022-M
Fridman, E. A., Hanakawa, T., Chung, M., Hummel, F., Leiguarda, R. C., and Cohen, L. G. (2004). Reorganization of the human ipsilesional premotor cortex after stroke. Brain 127, 747–758. doi: 10.1093/brain/awh082
Gallivan, J. P., and Goodale, M. A. (2018). The dorsal “action” pathway. Handb. Clin. Neurol. 151, 449–466. doi: 10.1016/B978-0-444-63622-5.00023-1
Giambattistelli, F., Tomasevic, L., Pellegrino, G., Porcaro, C., Melgari, J. M., Rossini, P. M., et al. (2014). The spontaneous fluctuation of the excitability of a single node modulates the internodes connectivity: a TMS-EEG study. Hum. Brain Mapp. 35, 1740–1749. doi: 10.1002/hbm.22288
Gramfort, A., Papadopoulo, T., Olivi, E., and Clerc, M. (2010). OpenMEEG: opensource software for quasistatic bioelectromagnetics. Biomed. Eng. Online 9:45. doi: 10.1186/1475-925x-9-45
Gratton, G., Bosco, C. M., Kramer, A. F., Coles, M. G., Wickens, C. D., and Donchin, E. (1990). Event-related brain potentials as indices of information extraction and response priming. Electroencephalogr. Clin. Neurophysiol. 75, 419–432. doi: 10.1016/0013-4694(90)90087-z
Hartwigsen, G., Bestmann, S., Ward, N. S., Woerbel, S., Mastroeni, C., Granert, O., et al. (2012). Left dorsal premotor cortex and supramarginal gyrus complement each other during rapid action reprogramming. J. Neurosci. 32, 16162–16171. doi: 10.1523/JNEUROSCI.1010-12.2012
Hartwigsen, G., and Siebner, H. R. (2015). Joint contribution of left dorsal premotor cortex and supramarginal gyrus to rapid action reprogramming. Brain Stimul. 8, 945–952. doi: 10.1016/j.brs.2015.04.011
Hedrich, T., Pellegrino, G., Kobayashi, E., Lina, J., and Grova, C. (2017). Comparison of the spatial resolution of source imaging techniques in high-density EEG and MEG. Neuroimage 157, 531–544. doi: 10.1016/j.neuroimage.2017.06.022
Hsieh, L. T., and Ranganath, C. (2014). Frontal midline theta oscillations during working memory maintenance and episodic encoding and retrieval. Neuroimage 85, 721–729. doi: 10.1016/j.neuroimage.2013.08.003
Humphries, M. D., Stewart, R. D., and Gurney, K. N. (2006). A physiologically plausible model of action selection and oscillatory activity in the basal ganglia. J. Neurosci. 26, 12921–12942. doi: 10.1523/JNEUROSCI.3486-06.2006
Hung, C.-S., Sarasso, S., Ferrarelli, F., Riedner, B., Ghilardi, M. F., Cirelli, C., et al. (2013). Local experience-dependent changes in the wake EEG after prolonged wakefulness. Sleep 36, 59–72. doi: 10.5665/sleep.2302
Ishii, R., Shinosaki, K., Ukai, S., Inouye, T., Ishihara, T., Yoshimine, T., et al. (1999). Medial prefrontal cortex generates frontal midline theta rhythm. Neuroreport 10, 675–679. doi: 10.1097/00001756-199903170-00003
Jensen, O., and Tesche, C. D. (2002). Frontal theta activity in humans increases with memory load in a working memory task. Eur. J. Neurosci. 15, 1395–1399. doi: 10.1046/j.1460-9568.2002.01975.x
Johansen-Berg, H., Rushworth, M. F., Bogdanovic, M. D., Kischka, U., Wimalaratna, S., and Matthews, P. M. (2002). The role of ipsilateral premotor cortex in hand movement after stroke. Proc. Natl. Acad. Sci. U S A 99, 14518–14523. doi: 10.1073/pnas.222536799
Kirov, R., Weiss, C., Siebner, H. R., Born, J., and Marshall, L. (2009). Slow oscillation electrical brain stimulation during waking promotes EEG theta activity and memory encoding. Proc. Natl. Acad. Sci. U S A 106, 15460–15465. doi: 10.1073/pnas.0904438106
Koch, G., Franca, M., Del Olmo, M. F., Cheeran, B., Milton, R., Sauco, M. A., et al. (2006). Time course of functional connectivity between dorsal premotor and contralateral motor cortex during movement selection. J. Neurosci. 26, 7452–7459. doi: 10.1523/JNEUROSCI.1158-06.2006
Koessler, L., Cecchin, T., Colnat-Coulbois, S., Vignal, J.-P., Jonas, J., Vespignani, H., et al. (2015). Catching the invisible: mesial temporal source contribution to simultaneous EEG and SEEG recordings. Brain Topogr. 28, 5–20. doi: 10.1007/s10548-014-0417-z
Kroeger, J., Bäumer, T., Jonas, M., Rothwell, J. C., Siebner, H. R., and Munchau, A. (2010). Charting the excitability of premotor to motor connections while withholding or initiating a selected movement. Eur. J. Neurosci. 32, 1771–1779. doi: 10.1111/j.1460-9568.2010.07442.x
Laukka, S. J., Järvilehto, T., Alexandrov, Y. I., and Lindqvist, J. (1995). Frontal midline theta related to learning in a simulated driving task. Biol. Psychol. 40, 313–320. doi: 10.1016/0301-0511(95)05122-q
Luu, P., Tucker, D. M., and Makeig, S. (2004). Frontal midline theta and the error-related negativity: neurophysiological mechanisms of action regulation. Clin. Neurophysiol. 115, 1821–1835. doi: 10.1016/j.clinph.2004.03.031
Mars, R. B., Klein, M. C., Neubert, F.-X., Olivier, E., Buch, E. R., Boorman, E. D., et al. (2009). Short-latency influence of medial frontal cortex on primary motor cortex during action selection under conflict. J. Neurosci. 29, 6926–6931. doi: 10.1523/JNEUROSCI.1396-09.2009
Mayka, M. A., Corcos, D. M., Leurgans, S. E., and Vaillancourt, D. E. (2006). Three-dimensional locations and boundaries of motor and premotor cortices as defined by functional brain imaging: a meta-analysis. Neuroimage 31, 1453–1474. doi: 10.1016/j.neuroimage.2006.02.004
Mizuki, Y., Takii, O., Tanaka, T., Tanaka, M., and Inanaga, K. (1982). Periodic appearance of frontal midline theta activity during performance of a sensory-motor task. Folia Psychiatr. Neurol. Jpn. 36, 375–382. doi: 10.1111/j.1440-1819.1982.tb03109.x
Moisa, M., Siebner, H. R., Pohmann, R., and Thielscher, A. (2012). Uncovering a context-specific connectional fingerprint of human dorsal premotor cortex. J. Neurosci. 32, 7244–7252. doi: 10.1523/JNEUROSCI.2757-11.2012
Monsell, S. (2003). Task switching. Trends Cogn. Sci. 7, 134–140. doi: 10.1016/S1364-6613(03)00028-7
Mushiake, H., Inase, M., and Tanji, J. (1991). Neuronal activity in the primate premotor, supplementary and precentral motor cortex during visually guided and internally determined sequential movements. J. Neurophysiol. 66, 705–718. doi: 10.1152/jn.1991.66.3.705
Mutha, P. K., Stapp, L. H., Sainburg, R. L., and Haaland, K. Y. (2014). Frontal and parietal cortex contributions to action modification. Cortex 57, 38–50. doi: 10.1016/j.cortex.2014.03.005
Nachev, P., Kennard, C., and Husain, M. (2008). Functional role of the supplementary and pre-supplementary motor areas. Nat. Rev. Neurosci. 9, 856–869. doi: 10.1038/nrn2478
Neubert, F.-X., Mars, R. B., Buch, E. R., Olivier, E., and Rushworth, M. F. (2010). Cortical and subcortical interactions during action reprogramming and their related white matter pathways. Proc. Natl. Acad. Sci. U S A 107, 13240–13245. doi: 10.1073/pnas.1000674107
Neuper, C., Scherer, R., Wriessnegger, S., and Pfurtscheller, G. (2009). Motor imagery and action observation: modulation of sensorimotor brain rhythms during mental control of a brain-computer interface. Clin. Neurophysiol. 120, 239–247. doi: 10.1016/j.clinph.2008.11.015
Nigbur, R., Cohen, M. X., Ridderinkhof, K. R., and Stürmer, B. (2012). Theta dynamics reveal domain-specific control over stimulus and response conflict. J. Cogn. Neurosci. 24, 1264–1274. doi: 10.1162/jocn_a_00128
Nigbur, R., Ivanova, G., and Stürmer, B. (2011). Theta power as a marker for cognitive interference. Clin. Neurophysiol. 122, 2185–2194. doi: 10.1016/j.clinph.2011.03.030
O’Doherty, J. P. (2011). Contributions of the ventromedial prefrontal cortex to goal-directed action selection. Ann. N Y Acad. Sci. 1239, 118–129. doi: 10.1111/j.1749-6632.2011.06290.x
O’Shea, J., Johansen-Berg, H., Trief, D., Gobel, S., and Rushworth, M. F. (2007). Functionally specific reorganization in human premotor cortex. Neuron 54, 479–490. doi: 10.1016/j.neuron.2007.04.021
Oldfield, R. C. (1971). The assessment and analysis of handedness: the Edinburgh inventory. Neuropsychologia 9, 97–113. doi: 10.1016/0028-3932(71)90067-4
Paus, T. (2001). Primate anterior cingulate cortex: where motor control, drive and cognition interface. Nat. Rev. Neurosci. 2, 417–424. doi: 10.1038/35077500
Peirce, J. W. (2007). PsychoPy—psychophysics software in Python. J. Neurosci. Methods 162, 8–13. doi: 10.1016/j.jneumeth.2006.11.017
Peirce, J. W. (2008). Generating stimuli for neuroscience using PsychoPy. Front. Neuroinform. 2:10. doi: 10.3389/neuro.11.010.2008
Pellegrino, G., Hedrich, T., Chowdhury, R., Hall, J. A., Lina, J. M., Dubeau, F., et al. (2016a). Source localization of the seizure onset zone from ictal EEG/MEG data. Hum. Brain Mapp. 37, 2528–2546. doi: 10.1002/hbm.23191
Pellegrino, G., Machado, A., von Ellenrieder, N., Watanabe, S., Hall, J. A., Lina, J.-M., et al. (2016b). Hemodynamic response to interictal epileptiform discharges addressed by personalized EEG-fNIRS recordings. Front. Neurosci. 10:102. doi: 10.3389/fnins.2016.00102
Pellegrino, G., Tombini, M., Curcio, G., Campana, C., Di Pino, G., Assenza, G., et al. (2016c). Slow activity in focal epilepsy during sleep and wakefulness. Clin. EEG Neurosci. 48, 200–208. doi: 10.1177/1550059416652055
Pellegrino, G., Hedrich, T., Chowdhury, R. A., Hall, J. A., Dubeau, F., Lina, J. M., et al. (2018). Clinical yield of magnetoencephalography distributed source imaging in epilepsy: a comparison with equivalent current dipole method. Hum. Brain Mapp. 39, 218–231. doi: 10.1002/hbm.23837
Pellegrino, G., Tomasevic, L., Tombini, M., Assenza, G., Bravi, M., Sterzi, S., et al. (2012). Inter-hemispheric coupling changes associate with motor improvements after robotic stroke rehabilitation. Restor. Neurol. Neurosci. 30, 497–510. doi: 10.3233/RNN-2012-120227
Perfetti, B., Moisello, C., Landsness, E. C., Kvint, S., Lanzafame, S., Onofrj, M., et al. (2011a). Modulation of gamma and theta spectral amplitude and phase synchronization is associated with the development of visuo-motor learning. J. Neurosci. 31, 14810–14819. doi: 10.1523/JNEUROSCI.1319-11.2011
Perfetti, B., Moisello, C., Landsness, E. C., Kvint, S., Pruski, A., Onofrj, M., et al. (2011b). Temporal evolution of oscillatory activity predicts performance in a choice-reaction time reaching task. J. Neurophysiol. 105, 18–27. doi: 10.1152/jn.00778.2010
Petrides, M., and Pandya, D. (2002). Comparative cytoarchitectonic analysis of the human and the macaque ventrolateral prefrontal cortex and corticocortical connection patterns in the monkey. Eur. J. Neurosci. 16, 291–310. doi: 10.1046/j.1460-9568.2001.02090.x
Picard, N., and Strick, P. L. (2001). Imaging the premotor areas. Curr. Opin. Neurobiol. 11, 663–672. doi: 10.1016/s0959-4388(01)00266-5
Rae, C. L., Hughes, L. E., Weaver, C., Anderson, M. C., and Rowe, J. B. (2014). Selection and stopping in voluntary action: a meta-analysis and combined fMRI study. Neuroimage 86, 381–391. doi: 10.1016/j.neuroimage.2013.10.012
Raffin, E., Pellegrino, G., Di Lazzaro, V., Thielscher, A., and Siebner, H. R. (2015). Bringing transcranial mapping into shape: sulcus-aligned mapping captures motor somatotopy in human primary motor hand area. Neuroimage 120, 164–175. doi: 10.1016/j.neuroimage.2015.07.024
Ramnani, N., and Owen, A. M. (2004). Anterior prefrontal cortex: insights into function from anatomy and neuroimaging. Nat. Rev. Neurosci. 5, 184–194. doi: 10.1038/nrn1343
Rushworth, M., Johansen-Berg, H., Göbel, S. M., and Devlin, J. (2003). The left parietal and premotor cortices: motor attention and selection. Neuroimage 20, S89–S100. doi: 10.1016/j.neuroimage.2003.09.011
Rushworth, M., and Taylor, P. (2006). TMS in the parietal cortex: updating representations for attention and action. Neuropsychologia 44, 2700–2716. doi: 10.1016/j.neuropsychologia.2005.12.007
Seitz, R. J., Höflich, P., Binkofski, F., Tellmann, L., Herzog, H., and Freund, H.-J. (1998). Role of the premotor cortex in recovery from middle cerebral artery infarction. Arch. Neurol. 55, 1081–1088. doi: 10.1001/archneur.55.8.1081
Simons, J. S., and Spiers, H. J. (2003). Prefrontal and medial temporal lobe interactions in long-term memory. Nat. Rev. Neurosci. 4, 637–648. doi: 10.1038/nrn1178
Sohn, M.-H., Albert, M. V., Jung, K., Carter, C. S., and Anderson, J. R. (2007). Anticipation of conflict monitoring in the anterior cingulate cortex and the prefrontal cortex. Proc. Natl. Acad. Sci. U S A 104, 10330–10334. doi: 10.1073/pnas.0703225104
Tadel, F., Baillet, S., Mosher, J. C., Pantazis, D., and Leahy, R. M. (2011). Brainstorm: a user-friendly application for MEG/EEG analysis. Comput. Intell. Neurosci. 2011:879716. doi: 10.1155/2011/879716
Tombini, M., Pellegrino, G., Zappasodi, F., Quattrocchi, C., Assenza, G., Melgari, J., et al. (2012). Complex visual hallucinations after occipital extrastriate ischemic stroke. Cortex 48, 774–777. doi: 10.1016/j.cortex.2011.04.027
Tombini, M., Zappasodi, F., Zollo, L., Pellegrino, G., Cavallo, G., Tecchio, F., et al. (2009). Brain activity preceding a 2D manual catching task. Neuroimage 47, 1735–1746. doi: 10.1016/j.neuroimage.2009.04.046
Verleger, R., Kuniecki, M., Moller, F., Fritzmannova, M., and Siebner, H. R. (2009). On how the motor cortices resolve an inter-hemispheric response conflict: an event-related EEG potential-guided TMS study of the flankers task. Eur. J. Neurosci. 30, 318–326. doi: 10.1111/j.1460-9568.2009.06817.x
von Ellenrieder, N., Pellegrino, G., Hedrich, T., Gotman, J., Lina, J.-M., Grova, C., et al. (2016). Detection and magnetic source imaging of fast oscillations (40–160 Hz) recorded with magnetoencephalography in focal epilepsy patients. Brain Topogr. 29, 218–231. doi: 10.1007/s10548-016-0471-9
Ward, N. S., Bestmann, S., Hartwigsen, G., Weiss, M. M., Christensen, L. O., Frackowiak, R. S., et al. (2010). Low-frequency transcranial magnetic stimulation over left dorsal premotor cortex improves the dynamic control of visuospatially cued actions. J. Neurosci. 30, 9216–9223. doi: 10.1523/JNEUROSCI.4499-09.2010
Wessel, J. R., and Aron, A. R. (2017). On the globality of motor suppression: unexpected events and their influence on behavior and cognition. Neuron 93, 259–280. doi: 10.1016/j.neuron.2016.12.013
Keywords: action selection, motor reprogramming, theta, EEG, dorsal premotor cortex, motor, performance, reaction time
Citation: Pellegrino G, Tomasevic L, Herz DM, Larsen KM and Siebner HR (2018) Theta Activity in the Left Dorsal Premotor Cortex During Action Re-Evaluation and Motor Reprogramming. Front. Hum. Neurosci. 12:364. doi: 10.3389/fnhum.2018.00364
Received: 07 April 2018; Accepted: 23 August 2018;
Published: 21 September 2018.
Edited by:
Felix Blankenburg, Freie Universität Berlin, GermanyReviewed by:
Aldo Ragazzoni, Fondazione PAS, ItalyMatt J. N. Brown, California State University, Sacramento, United States
Bernadette Van Wijk, University of Amsterdam, Netherlands
Copyright © 2018 Pellegrino, Tomasevic, Herz, Larsen and Siebner. This is an open-access article distributed under the terms of the Creative Commons Attribution License (CC BY). The use, distribution or reproduction in other forums is permitted, provided the original author(s) and the copyright owner(s) are credited and that the original publication in this journal is cited, in accordance with accepted academic practice. No use, distribution or reproduction is permitted which does not comply with these terms.
*Correspondence: Giovanni Pellegrino, giovannipellegrino@gmail.com