- 1Department of Psychology and Neuroscience, University of North Carolina, Chapel Hill, NC, United States
- 2Bowles Center for Alcohol Studies, University of North Carolina, Chapel Hill, NC, United States
- 3Neuroscience Curriculum, University of North Carolina, Chapel Hill, NC, United States
- 4Biomedical Research Imaging Center, University of North Carolina, Chapel Hill, NC, United States
Evidence suggests that dopamine levels in the prefrontal cortex (PFC) modulate executive functions. A key regulator of PFC dopamine is catechol-O-methyltransferase (COMT). The activity level of the COMT enzyme are influenced by sex and the Val158Met polymorphism (rs4680) of the COMT gene, with male sex and Val alleles both being associated with higher bulk enzyme activity, and presumably lower PFC dopamine. COMT genotype has not only been associated with individual differences in frontal dopamine-mediated behaviors, but also with variations in neuroimaging measures of brain activity and functional connectivity. In this study, we investigated whether COMT genotype predicts individual differences in neural activity and connectivity, and whether such effects are sex-dependent. We tested 93 healthy adults (48 females), genotyped for the Val158Met polymorphism, in a delay discounting task and at rest during fMRI. Delay discounting behavior was predicted by an interaction of COMT genotype and sex, consistent with a U-shaped relationship with enzyme activity. COMT genotype and sex similarly exhibited U-shaped relationships with individual differences in neural activation, particularly among networks that were most engaged by the task, including the default-mode network. Effects of COMT genotype and sex on functional connectivity during rest were also U-shaped. In contrast, flexible reorganization of network connections across task conditions varied linearly with COMT among both sexes. These data provide insight into the potential influences of COMT-regulated variations in catecholamine levels on brain function, which may represent endophenotypes for disorders of impulsivity.
Introduction
The catechol-O-methyltransferase (COMT) enzyme is responsible for degrading the majority of prefrontal cortical dopamine. The gene encoding COMT (COMT) has a common functional polymorphism, a valine (Val) to methionine (Met) substitution (Val158Met, rs4680), which is associated with substantially reduced COMT activity (Lachman et al., 1996). Thus, activity levels of the COMT enzyme vary according to genotype (i.e., Met/Met < Val/Met < Val/Val). Because variations in COMT enzyme activity levels are presumed to produce corresponding alterations of frontal dopamine levels (Gogos et al., 1998), this genotype is hypothesized to influence extracellular dopamine levels in the frontal cortex in an allele dose-dependent manner (i.e., Val/Val < Val/Met < Met/Met).
Consistent with the role of the COMT enzyme in prefrontal catecholamine degradation, numerous studies have linked this COMT functional polymorphism to individual differences in prefrontal-mediated functions. For example, COMT genotype has been associated with a variety of executive behaviors including delay discounting (Boettiger et al., 2007; Paloyelis et al., 2010; Smith and Boettiger, 2012), working memory (Bruder et al., 2005), and set shifting (Malhotra et al., 2002; Tunbridge et al., 2004). Corresponding effects of COMT genotype on behavioral task-related neural activity measured with functional magnetic resonance imaging (fMRI) have been demonstrated (Smolka et al., 2005; Winterer et al., 2006; Boettiger et al., 2007; Congdon et al., 2009; de Frias et al., 2010; Stokes et al., 2011), often with large effect sizes (Mier et al., 2010). COMT genotype has more recently been associated with brain functional connectivity at rest (Tian et al., 2013; Tunbridge et al., 2013; Markett et al., 2016). Thus, this polymorphism has been extensively linked to individual differences in executive behaviors and with associated functional neuroimaging measures, which is often attributed to its influence on prefrontal dopamine.
Intermediate levels of dopamine are deemed optimal for many executive functions. Reflecting its presumed influence on prefrontal dopamine levels (Cools and Robbins, 2004), effects of the COMT Val158Met polymorphism frequently exhibit U-shaped or inverted U-shaped relationships (Vijayraghavan et al., 2007; Smith and Boettiger, 2012). Although the direction of COMT genotype effects is sometimes inconsistent across studies (Mier et al., 2010), perhaps due to differences in the “optimal” dopamine levels for different behaviors (Stein et al., 2006), discrepancies in COMT effects may also occur when individuals are shifted along the U-shaped curve due to state or trait variations in dopamine. For example, effects of this genotype are proposed to depend on other modulators of dopamine including stress (Stein et al., 2006) or task performance (Jacobs and D'Esposito, 2011), which result in apparent shifts of the observed relationships along a U-shaped curve. Interactions of COMT Val158Met genotype with other genetic polymorphisms that regulate dopamine signaling also produce inverted U-shaped effects corresponding with the number of dopamine-promoting alleles (Yacubian et al., 2007; Tian et al., 2013). Furthermore, the relationship between COMT genotype and impulsive decision-making behavior in a delay discounting task are modulated by age, producing a U-shaped relationship with both genotype and putative age-related declines in frontal dopamine levels (Smith and Boettiger, 2012). Similar inverted U-shaped effects across genotype and age have been noted for functional connectivity of the medial frontal cortex at rest (Meyer et al., 2016), indicating that COMT effects persist in the absence of any particular behavior.
Another major moderator of COMT effects is sex. Sex and sex steroids represent significant sources of variation in COMT enzyme activity whereby COMT enzyme activity is reduced in women compared with men (Chen et al., 2004), likely due to suppression by estradiol (Cohn and Axelrod, 1971). In fact, the size of the effect of sex on COMT enzyme activity is comparable to the size of the effect of each Met allele (Cohn and Axelrod, 1971). This sex-related modulation of COMT enzyme activity, coupled with estradiol-induced increases in frontal dopamine signaling (Jacobs and D'Esposito, 2011; Schendzielorz et al., 2011; Smith et al., 2014a), supports the contention that females generally have greater levels of prefrontal dopamine than males (Jacobs and D'Esposito, 2011). Thus, whereas Val/Val males would generally be predicted to have the lowest dopamine levels, Met/Met females of similar age would have the highest levels. However, given the complex effects of sex and sex steroids on both COMT enzyme activity and dopamine levels, the precise order of effects of sex and COMT genotype on dopamine levels may not strictly correspond with those on COMT enzyme activity (Chen et al., 2004). Regardless, the impact of these sex-dependent variations in COMT enzyme activity on measures of brain function and behavior have received little consideration to date.
Although a few studies have reported sex differences in COMT genotype effects on behavior, they are often restricted to reports of significant effects in one sex but not the other (Harrison and Tunbridge, 2008), with only limited evidence of U-shaped effects (Lang et al., 2007; Chen et al., 2011). Nonetheless, like COMT genotype, sex and sex steroids influence prefrontal-mediated behaviors (Bobova et al., 2009; Jacobs and D'Esposito, 2011; Peper et al., 2013; Smith et al., 2014a), and mounting evidence suggests that effects of COMT genotype differ between males and females (Gogos et al., 1998; Rybakowski et al., 2006; Lang et al., 2007; Harrison and Tunbridge, 2008; Chen et al., 2011; Jacobs and D'Esposito, 2011; Laatikainen et al., 2013). For example, sexually-dimorphic effects of COMT genotype have been reported for set-shifting (Rybakowski et al., 2006) and sensation-seeking personality (Lang et al., 2007). However, no study to our knowledge has examined the potential moderating influences of sex on COMT genotype in the context of delay discounting nor in the context of neuroimaging. Such an investigation of sex-dependent influences of COMT may shed light on the neurobiology of the sex differences present in many psychiatric disorders (Harrison and Tunbridge, 2008).
Here we investigate the hypothesis that COMT Val158Met genotype interacts with sex to affect certain measures of behavior and neural function according to a U-shaped relationship. Given the strong prior associations of this COMT polymorphism with prefrontal-mediated behaviors, and particularly for intertemporal decision-making (Paloyelis et al., 2010; Smith and Boettiger, 2012) and its neural correlates (Boettiger et al., 2007), we focused our investigation on data from an fMRI delay-discounting task. Rather than limiting our investigation to a specific brain region or network, we examined COMT genotype effects across multiple large-scale functional neural networks. Using fMRI, we scanned 93 healthy men and women both during a resting state and while performing a delay discounting task in which they made four different categories of decisions. We considered the effects of COMT Val158Met genotype and sex at multiple levels of function, including behavior, neural activation, neural connectivity, and neural flexibility. We report significant interacting effects of sex and genotype, producing U-shaped relationships between putative frontal dopamine and delay discounting behavior, neural activations during delay discounting, and functional connectivity and functional organization during the resting state. In contrast, effects of COMT genotype on a measure of functional connectivity flexibility were not U-shaped. The results demonstrate novel associations of COMT genotype and sex on multiple large-scale neural networks and provide further evidence that neurocognitive effects of this genotype may extend beyond its role in frontal dopamine regulation.
Materials and Methods
Subjects
Healthy adults (n = 93; 48 females) aged 18–40 years (mean = 25.9 years) provided informed consent to participate in this study, which was approved by the University of North Carolina, Chapel Hill (UNC) Office of Human Research Ethics. Right-handedness, English as a native language, and at least a high school education (mean years of education = 16.3 ± 2.5) were required for study inclusion. Study exclusion criteria included psychiatric disorders based a structured clinical interview using DSM-IV criteria (Sheehan et al., 1998), known neurological disorders, or current psychoactive drug use (including medication but excluding moderate alcohol or caffeine intake). A urinalysis drug screen and breathalyzer test for recent alcohol consumption conducted on the day of the scan confirmed sobriety.
Genotyping
All participants provided a saliva sample for genotyping (DNA Genotek, Kanata, Ontario, Canada). Participants were genotyped for the COMT Val158Met polymorphism (rs4680) using TaqMan technology (Applied Biosystems, Foster City, CA), as previously described (Smith and Boettiger, 2012; Kelm and Boettiger, 2013; Smith et al., 2014a,b, 2015; Swift-Scanlan et al., 2014). Participant genotypes comprised 20 Val/Val (12 males, 8 females), 48 Val/Met (20 males, 28 females), and 25 Met/Met (13 males, 12 females). Allele frequencies in this sample did not deviate from Hardy–Weinberg equilibrium (χ2 = 0.12, df = 1, p > 0.05). Participants were tested blind to genotype.
Delay Discounting Task
While in the MRI scanner, participants performed a delay discounting task optimized for fMRI studies. The details of this task, in which participants selected between sets of smaller, immediate hypothetical monetary rewards (“Now”), or larger, delayed rewards (“Later”), have been reported previously (Boettiger et al., 2007, 2009; Altamirano et al., 2011; Smith and Boettiger, 2012; Kelm and Boettiger, 2013; Smith et al., 2014a, 2015; Elton et al., 2017) and are further detailed in the Supplementary Materials. Subjects underwent six consecutive functional MRI scans (~8 min per scan) each of which included 32 Now-vs.-Later decisions, consisting of four decision trial types presented psuedorandomly: “WANT,” “DON'T WANT,” “SOONER,” or “LARGER.”
Behavior
To examine behavioral effects of COMT genotype, an impulsive choice ratio (ICR, Mitchell et al., 2005) was calculated as the proportion of Now choices (i.e., selection of the smaller, immediate reward) relative to all choices made during WANT trials:
Greater discounting of delayed rewards corresponds with higher ICR values.
Magnetic Resonance Imaging (MRI) Data Acquisition
For both resting-state and task fMRI scans, T2*-weighted echo-planar images (EPI) were acquired on a Siemens 3T Tim Trio MRI scanner with a 32-channel head coil using the following parameters: TR = 2,000 ms, TE = 25 ms, flip angle = 50°, 35 slices tilted 30° from the horizontal plane; FoV = 192 × 192 mm; voxel size = 3 × 3 × 4 mm with a 0.5 mm gap, matrix = 64 × 64. The resting-state scan (n = 1) and each task scan (n = 6) included 243 frames (~8 min). For the resting-state scan, participants viewed a white fixation cross on a black screen. The delay discounting task was displayed using E-Prime-2 software (Psychology Software Tools, Pittsburg, PA), which recorded participants' responses on an MRI-compatible button box.
T1-weighted high-resolution magnetization-prepared rapid gradient-echo (MPRAGE) structural images were also acquired for alignment and tissue segmentation purposes using the following parameters: TR = 2,530 ms TE = 2.27 ms, flip angle = 9°, matrix = 176 × 512, 512 slices, final resolution = 1 × 0.5 × 0.5 mm3.
MRI Data Preprocessing
Functional image preprocessing consisted of the following steps completed in Analysis of Functional Neuroimages (AFNI Cox, 1996) software (v.16.0.1): correction for slice acquisition time, reorienting of oblique slices to the axial plane, image realignment for motion correction, despiking of noise time points, warping of functional images to a Montreal Neurological Institute (MNI) template, nuisance variable regression (white matter and cerebral spinal fluid signals as well as six motion covariates), linear detrending, spatial smoothing with an 8 mm full-width half-maximum Gaussian kernel, and scaling to percent signal change. Time points with high levels of artefactual signal were detected from a combination of head motion calculations and global signal intensity measures using The Artifact Detection Tools toolbox (ART; http://www.nitrc.org/projects/artifact_detect), which produced a set of corresponding regressors of no interest for activation and connectivity analyses.
Regions-of-Interest
Regions-of-interest (ROIs) used in all analyses were 6 mm spherical volumes centered on 264 coordinates identified by Power and colleagues as functionally important nodes attributed to 13 large-scale functional networks (Power et al., 2011; Figure 1), including auditory (AU), cerebellar (CE), cingulo-opercular task control (CO), default mode (DM), dorsal attention (DA), fronto-parietal task control (FP), memory retrieval (ME), salience (SA), lateral sensorimotor (SML), medial sensorimotor (SMM), subcortical (SC), ventral attention (VA), and visual (VI) networks. To obtain a representative time series for each ROI, the first principle component time series was extracted from voxels within the ROI using AFNI's 3dmaskSVD after applying a high-pass filter (0.008 Hz) for task scans and both high-pass and low-pass filters for the resting-state scan (0.008–0.1 Hz).
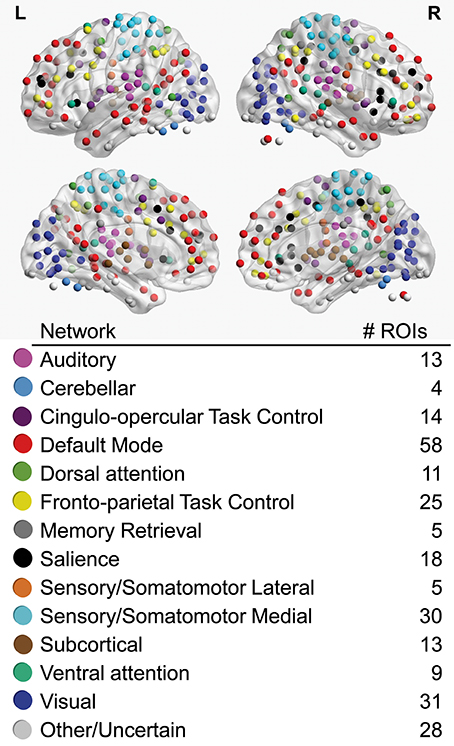
Figure 1. Regions-of-interest (ROIs), color-coded by association with large-scale networks, are visualized on left (L) and right (R) lateral (upper) and medial (lower) cerebral hemispheres. The total number of ROIs associated with each large-scale network is noted.
Task-Related Neural Activations
Task-related activation for each ROI was defined by a contrast isolating subjective decision-making processes: WANT - (0.5 × SOONER + 0.5 × LARGER). Decision type cues were modeled as delta functions and decision-making trials were modeled as short epochs with a duration equal to the participant's response time for a given trial. The task design was convolved with a canonical hemodynamic response function in Statistical Parametric Mapping (SPM8) software. Additionally, we modeled six directions of head motion and covariates for outlier time points (ART toolbox), in addition to zero, first, and second order polynomial regressors for each task run. The model was fit with restricted maximum likelihood estimation in 3dREMLfit in AFNI.
Resting-State Functional Connectivity
For the resting-state data, pairwise Pearson correlations between ROI time series were calculated and Fisher z-transformed to normalize the distribution, producing a 264 × 264 correlation matrix. Seven participants with missing resting-state data were not included in resting-state analyses, leaving 20 Val/Val (12 males, 8 females), 45 Val/Met (18 males, 27 females), and 21 Met/Met (11 males, 10 females).
Several graph theory indices were calculated to measure the functional organization of the brain during the resting-state, including functional connectivity strength, clustering coefficient, betweenness centrality, modularity (Newman's Q), local efficiency, and global efficiency. Functional connectivity strength was defined as the mean of the absolute value of the entire weighted graph. Using the Brain Connectivity Toolbox for Matlab, all other graph indices were calculated on binary, undirected graphs, and were averaged from a series of adjacency matrices of positive connections with densities between 5 and 30% at 1% intervals. Indices calculated at the node level (i.e., clustering coefficient, betweenness centrality, and local efficiency) were averaged across all 264 nodes to provide a single, whole-brain measure.
Functional Connectivity Flexibility
Beta-series analysis enabled the calculation of task-related functional connectivity between ROIs during each of the four decision types (Rissman et al., 2004). Task design matrices were consistent with those described for task activations (above), with the exception that each decision-making trial was modeled as a separate regressor. The model was fit with restricted maximum likelihood estimation in 3dREMLfit in AFNI. Beta estimates for each of the four decision trial types (i.e., WANT, DON'T WANT, SOONER, LATER) were concatenated for each ROI. Next the pairwise Pearson correlations between ROIs were calculated for each decision trial type, producing four correlation matrices, which were Fisher z-transformed.
To calculate the extent to which functional connections reorganize between the five states (resting and four task decision types), functional connectivity flexibility was calculated as the Euclidean distance between each pair of correlation matrices, averaged across states for each ROI. For the 7 participants missing resting-state data, this measure only considered the four task decision states, and a covariate was included to account for this difference in statistical analyses.
Statistical Testing of COMT Effects
Statistical tests of the effects of COMT genotype were estimated in linear models with the GLIMMIX procedure in SAS 9.4 (SAS Institute Inc., Cary, NC, USA). Genotype was coded as the number of Met alleles (Val/Val = 0, Val/Met = 1, Met/Met = 2) and sex was coded as 0 (males) or 1 (females). For network-based analyses of activation and functional connectivity, we estimated multilevel linear mixed models to account for the nested nature of the data, since each network includes multiple ROIs. These models were tested separately for each network (or pairs of networks for functional connectivity analyses) and, to account for variability of effects across network ROIs, included random slopes and intercepts for each ROI (or pairs of ROIs for functional connectivity analyses). In all models, COMT genotype effects covaried for age, socioeconomic status, menstrual cycle phase (categorically-defined to include women in cycle days 1–7, 8–15, and 18–30), and current hormonal birth control usage (binary). Analyses of resting-state functional connectivity also covaried for the average framewise displacement during the scan (Power et al., 2012) to control for apparent connections arising from head motion. Functional connectivity flexibility analyses similarly covaried for average framewise motion across the resting-state and six task scans. Each set of network-level results underwent false discovery rate (FDR) correction for 13 network comparisons.
Results
Behavioral Data
The mean (±SD) ICR value for this sample was 0.63 ± 0.32 (males: 0.67 ± 0.29; females: 0.59 ± 0.35). Due to non-normal distributions of raw ICR values, we performed an arcsine-root transformation prior to parametric testing. There was a significant genotype × sex interaction effect on ICR (t = −2.50, p = 0.014), producing a U-shaped relationship in which genotypes encoding the lowest and highest frontal dopamine levels, represented by Val/Val males and Met/Met females, respectively, demonstrated higher ICR values relative to genotypes encoding intermediate levels of frontal dopamine (Figure 2). These behavioral findings, together with the key neuromodulatory role of dopamine, led us then to examine sex and COMT genotype effects on brain network function, detailed in the following sections.
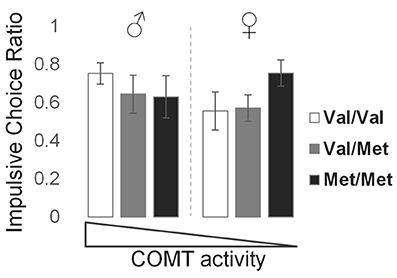
Figure 2. Effects of COMT genotype and sex on impulsive choice during a delay discounting task. Bar graphs represent the mean and standard error of impulsive choice ratio (ICR) values for each group, in order of theoretical frontal dopamine levels.
Task-Related Neural Activations
We detected significant genotype × sex interaction effects on network activation related to intertemporal decision-making for the DM, SC, AU, SMM, CO, DA, and CE networks (Figure 3A). The strength and direction of this relationship depended on the strength and direction of task-related neural activation. To quantify this relationship between task-related activation and COMT genotype × sex interaction effects, beta estimates of the genotype × sex interaction effect on task-related activation were plotted against the corresponding beta estimates of task-related activation for each ROI; a Pearson correlation analysis indicated a significant positive correlation (r = 0.69, p < 0.001; Figure 3B) in which greater positive task-related activation predicted a stronger U-shaped relationship with COMT genotype, whereas more negative task-related activation predicted an inverted U-shaped relationship with COMT genotype.
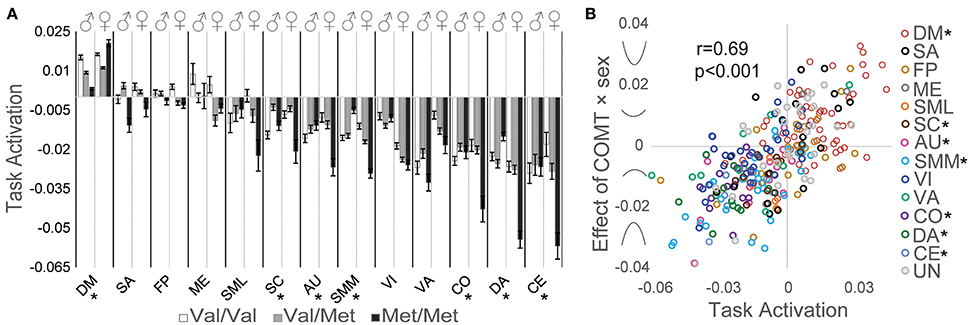
Figure 3. Effects of COMT genotype and sex on network activation during a delay discounting task. (A) Activation of each network is plotted by COMT genotype and sex. For display purposes, beta estimates of task-related activation for all ROIs of a given network are averaged. Error bars reflect standard errors of the mean. (B) Scatter plot of task activation effects plotted against COMT genotype × sex interaction effects on each of the 264 ROIs, color coded by association with large-scale networks. Symbols on the y-axis correspond with the direction of interaction effects, producing u-shaped (positive values) or inverted u-shaped (negative values) relationships. (*) Denotes statistically significant COMT genotype × sex interaction effect measured at the network level. AU, auditory; CE, cerebellar; CO, cingulo-opercular task control; DM, default mode; DA, dorsal attention, FP, fronto-parietal task control; ME, memory retrieval; SA, salience; SML, lateral sensorimotor; SMM, medial sensorimotor; SC, subcortical; VA, ventral attention; VI, visual.
Resting-State Functional Connectivity
We also detected effects of COMT genotype on functional connectivity during rest. Network connectivity by genotype and sex is plotted for the four most positively (Figure 4A) and most negatively (Figure 4B) correlated network pairs during the resting state. As observed for the task-based activation data, we similarly detected a relationship between resting-state functional connectivity and the strength and direction of COMT genotype-by-sex effects (Figure 4C): greater positive functional connectivity associated with stronger COMT genotype × sex U-shaped effects, whereas more negative functional connectivity associated with inverted U-shaped relationships based on Spearman correlation analysis (ρ = 0.63, p < 0.001).
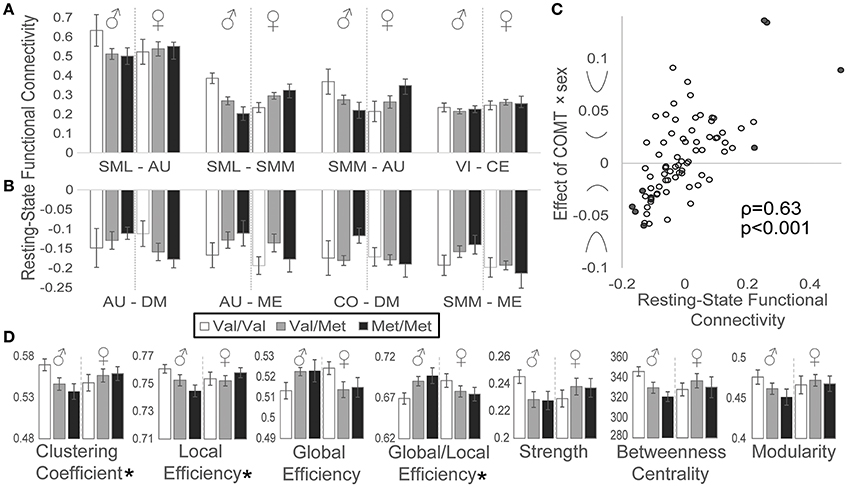
Figure 4. Effects of COMT genotype and sex on functional connectivity during a resting state. Between-network functional connectivity is plotted by COMT genotype and sex for the (A) four most positive and (B) four most negative connections. For display purposes, between-network connectivity was plotted as the averaged Fisher-z-transformed correlation between all ROIs belonging to each pair of networks. Error bars reflect standard errors of the means. (C) Scatter plot of resting-state functional connectivity plotted against COMT genotype × sex interaction effects for each network pair. Symbols on the y-axis correspond with the direction of interaction effects, producing u-shaped (positive values) or inverted u-shaped (negative values) relationships. Filled circles indicate network connections highlighted in panels A and B. (D) Graph theory measures are plotted by COMT genotype and sex. (*) Denotes statistically significant COMT genotype × sex interaction effect measured at the network level. Error bars reflect standard errors of the means. AU, auditory; CE, cerebellar; CO, cingulo-opercular task control; DM, default mode; ME, memory retrieval; SML, lateral sensorimotor; SMM, medial sensorimotor; VI, visual.
In addition to measuring simple correlations between network time series, we also evaluated effects of sex and COMT genotype on several graph theory metrics (Figure 4D). We observed significant genotype × sex interaction effects, reflecting U-shaped relationships, on both the clustering coefficient (t = 2.00, p = 0.049) and local efficiency (t = 2.01, p = 0.048), which both measure aspects of how nodes cluster together. Conversely, COMT effects on global efficiency, the inverse of the average shortest path length between all nodes, demonstrated a trend toward an inverted U-shaped curve, although this effect did not reach statistical significance (t = −1.86, p = 0.067). However, the tendency toward favoring global over local efficiency, represented by the ratio of global efficiency/local efficiency, exhibited a significant inverted U-shaped relationship (t = −2.30, p = 0.024). We failed to detect significant effects of COMT genotype and/or sex on functional connectivity strength, betweenness centrality, or modularity.
Functional Connectivity Flexibility
To quantify how much functional connectivity patterns changed across different states, we next investigated COMT genotype and sex effects on the distance between functional connectivity matrices across resting and task states (Figure 5). For the FP, SML, and SMM networks, we observed a linear effect of COMT that did not significantly differ between the sexes (Figure 5A). However, we found significant genotype × sex interaction effects on multiple networks: AU, CE, CO, DA, DM, ME, SA, SC, VA, and VI. Whereas effects of COMT genotype exhibited linear effects on data from males for all networks, for females, Akaike Information Criteria indicated an improved fit of quadratic vs. linear relationships for the CO, SA, SC, and VI networks. A linear relationship best described the effect of COMT genotype on the FP, SML, SMM, AU, CE, DA, DM, ME, and VA networks among females. In Figure 5, the strength of COMT genotype effects for each of the 264 ROIs is depicted for all subjects (Figure 5B), linear effects in males (Figure 5C), quadratic effects in females (Figure 5D), and linear effects in females (Figure 5E). The observed COMT effects on functional connectivity flexibility did not appear to be driven by functional connectivity changes associated with any one brain state (Supplementary Figure 1).
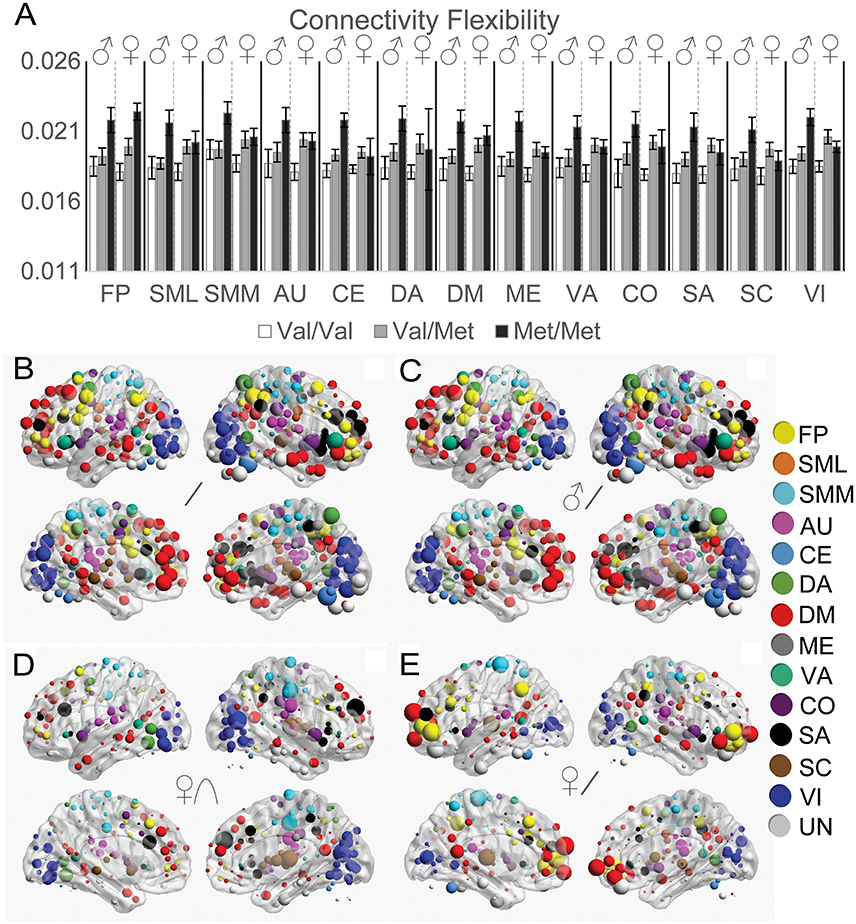
Figure 5. Effects of COMT genotype and sex on functional connectivity flexibility between resting and task states. (A) Functional connectivity flexibility is plotted by COMT genotype and sex for each large-scale network. For display purposes, connectivity flexibility was averaged across all ROIs in a given network. Error bars reflect standard errors of the means. The strength of COMT genotype effects for each of the 264 ROIs is displayed on left and right cerebral hemispheres according to statistical tests of (B) linear effects across all subjects, controlling for sex, (C) linear effects in males, (D) quadratic effects in females, and (E) linear effects in females. The size of the spheres correspond with the size of the COMT genotype effects, scaled independently for each of the image sets in (B–E). AU, auditory; CE, cerebellar; CO, cingulo-opercular task control; DM, default mode; DA, dorsal attention, FP, fronto-parietal task control; ME, memory retrieval; SA, salience; SML, lateral sensorimotor; SMM, medial sensorimotor; SC, subcortical; VA, ventral attention; VI, visual.
Discussion
We investigated the role of the Val158Met polymorphism of the COMT gene on several properties of neural function. Measures of task-evoked activity and resting-state functional connections demonstrated significant genotype × sex interactions, resulting in U-shaped or inverted U-shaped relationships with COMT enzyme activity; the direction and extent of these genotype effects depended on the direction and extent of the activity or connectivity. The findings are largely consistent with the extensive literature demonstrating U-shaped effects of COMT genotype and theoretical frontal dopamine levels on executive function. A model describing the theoretical relationship between sex, genotype, and executive functions based on the data relationships observed in the current study is presented in Figure 6.
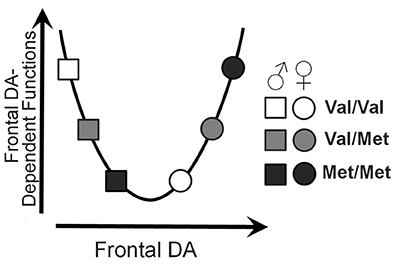
Figure 6. U-shaped model of frontal dopamine function. Theoretical influences of both sex and COMT genotype on frontal dopamine levels by which sex and COMT genotype may affect frontal dopamine-dependent functions.
The neural effects of COMT genotype observed in this study were evident in all networks that we tested and appear to promote brain-wide alterations in neural activity and connectivity. Although, COMT activity is thought to affect dopamine signaling principally in the frontal cortex through alterations in synaptic clearance rates of dopamine, evidence from rodent studies also supports an association of COMT activity with dopamine release in the striatum (Simpson et al., 2014) and hippocampus (Laatikainen et al., 2013), and with dopamine metabolism throughout the brain (Laatikainen et al., 2013). Although some effects may be mediated through frontal dopamine signaling in functionally-connected brain regions, a previous task-activation study notably identified effects of COMT Val158Met genotype only for posterior default mode deactivation (Malhotra et al., 2002).
In fact, our findings demonstrate that the effects of COMT genotype on fMRI blood oxygen level-dependent (BOLD) signal are most pronounced in regions that are modulated most during a delay discounting task and, additionally, among those connections that are strongest during the resting state. A possible explanation for these findings come from studies in rats showing that a COMT inhibitor did not affect basal dopamine levels but instead enhanced extracellular dopamine levels in response to elicited dopamine release (Tunbridge et al., 2004). Thus, an inference from the current findings is that the observed COMT genotype-by-sex effects may be driven by task-evoked dopamine release in the associated regions/networks. Although a role for norepinephrine cannot be excluded, most evidence to-date has emphasized a dominant role for the COMT enzyme in preferentially modulating dopamine activity in the brain (Gogos et al., 1998; Tunbridge et al., 2004; Laatikainen et al., 2013).
The effects of COMT genotype on the resting state extended to influences on brain organization as indexed by graph theoretical measures. In particular, there were significant genotype × sex interactions on the clustering coefficient and local efficiency. The clustering coefficient indexes the extent to which a node's neighbors (i.e., the other nodes to which it is connected) are also connected to each other. A related measure, local efficiency is the inverse of the average shortest path length connecting a node's neighbors. Interestingly, the observed effects of COMT genotype on these measures suggest that very low or very high dopamine enhances the clustering and local efficiency throughout the brain. Combining these findings with the observation that the ratio of global-to-local efficiency had an inverted U-shaped relationship to putative COMT activity suggest that intermediate levels of COMT activity—and perhaps, therefore, intermediate levels of dopamine—promote greater global efficiency at the expense of local efficiency, whereas potentially suboptimal levels of dopamine (either very high or very low) favor localized clustering and local efficiency.
In this study, we introduce a novel metric of functional connectivity flexibility, calculated as the average Euclidean distance between functional connectivity matrices across multiple brain states, which indicates the extent to which connections between regions are reorganized in response to state changes. For many regions, both males and females exhibited a positive linear relationship with COMT genotype (i.e., Val/Val < Val/Met < Met/Met), while a subset of regions/networks exhibit a quadratic relationship among females. These findings are seemingly in contrast with behavioral evidence that the Val/Val genotype is associated with higher cognitive flexibility in a task-switching paradigm (Colzato et al., 2010). One interpretation is that Met/Met individuals must undergo more extensive neural reconfiguration to produce behavioral changes, leading to decreased efficiency. Another possibility is that an increased signal-to-noise ratio in fMRI data associated with the Met/Met genotype (Winterer et al., 2006) enhanced the detection of functional connections in both the positive and negative directions, producing larger flexibility scores.
Notably, there was not an apparent U-shaped relationship between the flexibility of functional connectivity and theoretical dopamine levels. One possible explanation is that very high levels of dopamine do not impair functional connectivity flexibility, producing a mostly linear relationship. This interpretation would receive support if females, with higher dopamine levels, demonstrated increased flexibility relative to males, which was not the case. Another possibility is that these effects are primarily driven by factors other than dopamine. One such possible factor is blood pressure, since COMT genotype has been linked to variations in blood pressure among men (Annerbrink et al., 2008), likely due to differences in peripheral catecholamine metabolism, and blood pressure influences the BOLD signal (Kalisch et al., 2001).
Although the current consensus is that the COMT Val158Met polymorphism does not play a dominant role in most mental health disorders (Hosak, 2007; Klein et al., 2016), the pleiotropic influence of this gene on intermediate phenotypes of several psychiatric disorders has drawn considerable interest. For example, a prominent effect of COMT genotype was found for delay discounting-related activation of the default-mode network, known to support future-oriented thinking (Spreng et al., 2009), highlighting a potential neural mechanism by which COMT may modulate this intermediate phenotype of addiction. Additionally, modulation of COMT activity by sex may contribute to sex differences in many psychiatric disorders (Harrison and Tunbridge, 2008). In fact, the current data suggest that the original “worrier-vs.-warrior” conceptualization (Stein et al., 2006) in which Val alleles confer protection from anxiety (“warriors”) whereas Met alleles offer advantages for attention (“worriers”) may need to be refined. Rather, males (particularly Val/Val) may be more likely to exhibit a “warrior” phenotype, and females (particularly Met/Met) may be more likely to characterize the “worrier” phenotype; as such, male and female sex may generally be protective against the “worrier” phenotype and “warrior” phenotype, respectively. This distinction is consistent with the fact that males are overrepresented within “externalizing disorders” like ADHD and antisocial personality, whereas females are more prone to “internalizing disorders” such as anxiety and depression.
Limitations
Although COMT genotype is closely related to COMT activity and therefore to frontal dopamine levels, there is not a perfect correspondence between these measures. Future studies that evaluate effects of more direct manipulation of dopamine levels are warranted. Studies of COMT methylation could also account for variance in COMT activity related to epigenetic modifications. Furthermore, we did not measure blood pressure or other physiological measures, so we could not account for potential peripheral effects of COMT genotype. Moreover, despite the seemingly robust effects of this polymorphism, some cell sizes were relatively small (e.g., 8 Val/Val females), limiting confidence in the stability of the findings. Finally, the generalizability of these findings to other tasks remains to be tested.
Conclusion
We report linear and U-shaped relationships associated with a COMT polymorphism and sex with fMRI measures of neural function. Our results indicate brain-wide effects of COMT genotype, indicating potentially broader effects of COMT genotype beyond localized effects on frontal dopamine dynamics.
Ethics Statement
This study was carried out in accordance with the recommendations of the Declaration of Helsinki of the World Medical Association (WMA) with written informed consent from all subjects. All subjects gave written informed consent in accordance with the Declaration of Helsinki. The protocol was approved by the University of North Carolina, Chapel Hill (UNC) Office of Human Research Ethics.
Author Contributions
CB and CS were responsible for the study concept and design. CS and MP contributed to the acquisition of data and assisted with behavioral data analysis. AE assisted with behavioral analysis and performed the fMRI data analysis. AE and CB drafted the manuscript. All authors critically reviewed content and approved final version for publication.
Funding
This work was supported by Award Numbers UL1TR001111, KL2TR001109, P60AA011605, and the Alcohol Research Foundation (ABMRF; CB), T32DA007244 and F31AA020132 (CS), T32AA007573 (AE), and the Howard Hughes Medical Institute (MP). CB has consulted for BlackThorn Therapeutics and received compensation.
Conflict of Interest Statement
The authors declare that the research was conducted in the absence of any commercial or financial relationships that could be construed as a potential conflict of interest.
Acknowledgments
We thank Melisa Menceloglu, Grace Guo, and S. Hunter Oppler for assistance with behavioral data entry.
Supplementary Material
Supplementary materialThe Supplementary Material for this article can be found online at: https://www.frontiersin.org/articles/10.3389/fnhum.2017.00578/full#supplementary-material
References
Altamirano, L. J., Fields, H. L., D'Esposito, M., and Boettiger, C. A. (2011). Interaction between family history of alcoholism and locus of control in the opioid regulation of impulsive responding under the influence of alcohol. Alcohol. Clin. Exp. Res. 35, 1905–1914. doi: 10.1111/j.1530-0277.2011.01535.x
Annerbrink, K., Westberg, L., Nilsson, S., Rosmond, R., Holm, G., and Eriksson, E. (2008). Catechol O-methyltransferase val158-met polymorphism is associated with abdominal obesity and blood pressure in men. Metab. Clin. Exp. 57, 708–711 doi: 10.1016/j.metabol.2008.01.012
Bobova, L., Finn, P. R., Rickert, M. E., and Lucas, J. (2009). Disinhibitory psychopathology and delay discounting in alcohol dependence: personality and cognitive correlates. Exp. Clin. Psychopharmacol. 17, 51–61. doi: 10.1037/a0014503
Boettiger, C. A., Kelley, E. A., Mitchell, J. M., D'Esposito, M., and Fields, H. L. (2009). Now or Later? An fMRI study of the effects of endogenous opioid blockade on a decision-making network. Pharmacol. Biochem. Behav. 93, 291–299. doi: 10.1016/j.pbb.2009.02.008
Boettiger, C. A., Mitchell, J. M., Tavares, V. C., Robertson, M., Joslyn, G., and Fields, H. L. (2007). Immediate reward bias in humans: fronto-parietal networks and a role for the catechol-O-methyltransferase 158(Val/Val) genotype. J. Neurosci. 27, 14383–14391. doi: 10.1523/JNEUROSCI.2551-07.2007
Bruder, G. E., Keilp, J. G., Xu, H., Shikhman, M., Schori, E., and Gilliam, T. C. (2005). Catechol-O-methyltransferase (COMT) genotypes and working memory: associations with differing cognitive operations. Biol. Psychiatry 58, 901–907. doi: 10.1016/j.biopsych.2005.05.010
Chen, C., Moyzis, R., Dong, Q., He, Q., Zhu, B., Li, J., et al. (2011). Sex modulates the associations between the COMT gene and personality traits. Neuropsychopharmacology 36, 1593–1598. doi: 10.1038/npp.2011.39
Chen, J., Lipska, B. K., Halim, N., Ma, Q. D., Matsumoto, M., and Weinberger, D. R. (2004). Functional analysis of genetic variation in catechol-O-methyltransferase (COMT): effects on mrna, protein, and enzyme activity in postmortem human brain. am. J. Hum. Genet. 75, 807–821. doi: 10.1086/425589
Cohn, C. K., and Axelrod, J. (1971). The effect of estradiol on catechol-O-methyltransferase activity in rat liver. Life Sci. I. 10, 1351–1354. doi: 10.1016/0024-3205(71)90335-3
Colzato, L. S., Waszak, F., Nieuwenhuis, S., Posthuma, D., and Hommel, B. (2010). The flexible mind is associated with the catechol-O-methyltransferase (COMT) Val158Met polymorphism: evidence for a role of dopamine in the control of task-switching. Neuropsychologia 48, 2764–2768. doi: 10.1016/j.neuropsychologia.2010.04.023
Congdon, E., Constable, R. T., Lesch, K. P., and Canli, T. (2009). Influence of SLC6A3 and COMT variation on neural activation during response inhibition. Biol. Psychol. 81, 144–52. doi: 10.1016/j.biopsycho.2009.03.005
Cools, R., and Robbins, T., W. (2004). Chemistry of the adaptive mind. Philos. Transact. A. Math. Phys. Eng. Sci. 362, 2871–2888. doi: 10.1098/rsta.2004.1468
Cox, R. W. (1996). AFNI: software for analysis and visualization of functional magnetic resonance neuroimages. Comput. Biomed. Res. 29, 162–173. doi: 10.1006/cbmr.1996.0014
de Frias, C. M., Marklund, P., Eriksson, E., Larsson, A., Oman, L., Annerbrink, K., and Nyberg, L. (2010). Influence of COMT gene polymorphism on fMRI-assessed sustained and transient activity during a working memory task. J. Cogn. Neurosci. 22, 1614–1622. doi: 10.1162/jocn.2009.21318
Elton, A., Smith, C. T., Parrish, M. H., and Boettiger, C. A. (2017). Neural systems underlying individual differences in intertemporal decision-making. J. Cogn. Neurosci. 29, 467–479. doi: 10.1162/jocn_a_01069
Gogos, J. A., Morgan, M., Luine, V., Santha, M., Ogawa, S., and Karayiorgou, M. (1998). Catechol-O-methyltransferase-deficient mice exhibit sexually dimorphic changes in catecholamine levels and behavior. Proc. Natl. Acad. Sci. U.S.A. 95, 9991–9996. doi: 10.1073/pnas.95.17.9991
Harrison, P. J., and Tunbridge, E. M. (2008). Catechol-O-methyltransferase (COMT): a gene contributing to sex differences in brain function, and to sexual dimorphism in the predisposition to psychiatric disorders. Neuropsychopharmacology, 33, 3037–3045. doi: 10.1038/sj.npp.1301543
Hosak, L. (2007). Role of the COMT gene Val158Met polymorphism in mental disorders: a review. Eur. Psychiatry 22, 276–278. doi: 10.1016/j.eurpsy.2007.02.002
Jacobs, E., and D'Esposito, M. (2011). Estrogen shapes dopamine-dependent cognitive processes: implications for women's health. J. Neurosci. 31, 5286–5293. doi: 10.1523/JNEUROSCI.6394-10.2011
Kalisch, R., Elbel, G. K., Gossl, C., Czisch, M., and Auer, D. P. (2001). Blood pressure changes induced by arterial blood withdrawal influence bold signal in anesthesized rats at 7 Tesla: implications for pharmacologic mri. Neuroimage 14, 891–898. doi: 10.1006/nimg.2001.0890
Kelm, M. K., and Boettiger, C. A. (2013). Effects of acute dopamine precusor depletion on immediate reward selection bias and working memory depend on catechol-O-methyltransferase genotype. J. Cogn. Neurosci. 25, 2061–2071. doi: 10.1162/jocn_a_00464
Klein, M., Schmoeger, M., Kasper, S., and Schosser, A. (2016). Meta-analysis of the COMT Val158Met polymorphism in major depressive disorder: the role of gender. World J. Biol. Psychiatry 17, 147–158. doi: 10.3109/15622975.2015.1083615
Laatikainen, L. M., Sharp, T., Harrison, P. J., and Tunbridge, E. M. (2013). Sexually dimorphic effects of catechol-O-methyltransferase (COMT) inhibition on dopamine metabolism in multiple brain regions. PLoS ONE 8:e61839. doi: 10.1371/journal.pone.0061839
Lachman, H. M., Papolos, D. F., Saito, T., Yu, Y. M., Szumlanski, C. L., and Weinshilboum, R. M. (1996). Human catechol-O-methyltransferase pharmacogenetics: description of a functional polymorphism and its potential application to neuropsychiatric disorders. Pharmacogenetics 6, 243–250. doi: 10.1097/00008571-199606000-00007
Lang, U. E., Bajbouj, M., Sander, T., and Gallinat, J. (2007). Gender-dependent association of the functional catechol-O-methyltransferase Val158Met genotype with sensation seeking personality trait. Neuropsychopharmacology 32, 1950–1955. doi: 10.1038/sj.npp.1301335
Malhotra, A. K., Kestler, L. J., Mazzanti, C., Bates, J. A., Goldberg, T., and Goldman, D. (2002). A functional polymorphism in the COMT gene and performance on a test of prefrontal cognition. Am. J. Psychiatry 159, 652–654. doi: 10.1176/appi.ajp.159.4.652
Markett, S., Montag, C., Heeren, B., Saryiska, R., Lachmann, B., Weber, B., et al. (2016). Voxelwise eigenvector centrality mapping of the human functional connectome reveals an influence of the catechol-O-methyltransferase val158met polymorphism on the default mode and somatomotor network. Brain Struct. Funct. 221, 2755–2765. doi: 10.1007/s00429-015-1069-9
Meyer, B. M., Huemer, J., Rabl, U., Boubela, R. N., Kalcher, K., and Pezawas, L. (2016). Oppositional COMT Val158Met effects on resting state functional connectivity in adolescents and adults. Brain Struct. Funct. 221, 103–114. doi: 10.1007/s00429-014-0895-5
Mier, D., Kirsch, P., and Meyer-Lindenberg, A. (2010). Neural substrates of pleiotropic action of genetic variation in COMT: a meta-analysis. Mol. Psychiatry 15, 918–927. doi: 10.1038/mp.2009.36
Mitchell, J. M., Fields, H. L., D'Esposito, M., and Boettiger, C. A. (2005). Impulsive responding in alcoholics. Alcohol. Clin. Exp. Res. 29, 2158–2169. doi: 10.1097/01.alc.0000191755.63639.4a
Paloyelis, Y., Asherson, P., Mehta, M. A., Faraone, S. V., and Kuntsi, J. (2010). DAT1 and COMT effects on delay discounting and trait impulsivity in male adolescents with attention deficit/hyperactivity disorder and healthy controls. Neuropsychopharmacology 35, 2414–2426. doi: 10.1038/npp.2010.124
Peper, J. S., Mandl, R. C., Braams, B. R., de Water, E., Heijboer, A. C., Koolschijn, P. C., et al. (2013). Delay discounting and frontostriatal fiber tracts: a combined, D. T. I., and MTR study on impulsive choices in healthy young adults. Cereb. Cortex 23, 1695–1702. doi: 10.1093/cercor/bhs163
Power, J. D., Alexander Cohen, L., Steven Nelson, M., Gagan Wig, S., Kelly Barnes, A., Jessica Church, A., et al. (2011). Functional network organization of the human brain. Neuron 72, 665–678. doi: 10.1016/j.neuron.2011.09.006
Power, J. D., Barnes, K. A., Snyder, A. Z., Schlaggar, B. L., and Petersen, S. E. (2012). Spurious but systematic correlations in functional connectivity MRI networks arise from subject motion. Neuroimage 59, 2142–2154. doi: 10.1016/j.neuroimage.2011.10.018
Rissman, J., Gazzaley, A., and D'Esposito, M. (2004). Measuring functional connectivity during distinct stages of a cognitive task. Neuroimage 23, 752–763. doi: 10.1016/j.neuroimage.2004.06.035
Rybakowski, J. K., Borkowska, A., Czerski, P. M., Dmitrzak-Weglarz, M., Skibinska, M., Kapelski, P., et al. (2006). Performance on the Wisconsin Card Sorting Test in schizophrenia and genes of dopaminergic inactivation (COMT, DAT, NET). Psychiatry Res. 143, 13–19. doi: 10.1016/j.psychres.2005.10.008
Schendzielorz, N., Rysa, A., Reenila, I., Raasmaja, A., and Mannisto, P., T. (2011). Complex estrogenic regulation of catechol-O-methyltransferase (COMT) in rats. J. Physiol. Pharmacol. 62, 483–490.
Sheehan, D. V., Lecrubier, Y., Sheehan, K. H., Amorim, P., Janavs, J., Weiller, E., et al. (1998). The Mini-International Neuropsychiatric Interview (M.I.N.I.): the development and validation of a structured diagnostic psychiatric interview for DSM-IV and ICD-10. J. Clin. Psychiatry 59(Suppl. 1), 22–33;quiz 34–57.
Simpson, E. H., Morud, J., Winiger, V., Biezonski, D., Zhu, J. P., Bach, M. E., et al. (2014). Genetic variation in COMT activity impacts learning and dopamine release capacity in the striatum. Learn. Mem. 21, 205–201. doi: 10.1101/lm.032094.113
Smith, C. T., and Boettiger, C. A. (2012). Age modulates the effect of COMT genotype on delay discounting behavior. Psychopharmacology 222, 609–617. doi: 10.1007/s00213-012-2653-9
Smith, C. T., Sierra, Y., Oppler, S. H., and Boettiger, C. A. (2014a). Ovarian cycle effects on immediate reward selection bias in humans: a role for estradiol. J. Neurosci. 34, 5468–5476. doi: 10.1523/JNEUROSCI.0014-14.2014
Smith, C. T., Steel, E. A., Parrish, M. H., Kelm, M. K., and Boettiger, C. A. (2015). Intertemporal choice behavior in emerging adults and adults: effects of age interact with alcohol use and family history status. Front. Hum. Neurosci. 9:627. doi: 10.3389/fnhum.2015.00627
Smith, C. T., Swift-Scanlan, T., and Boettiger, C. A. (2014b). Genetic polymorphisms regulating dopamine signaling in the frontal cortex interact to affect target detection under high working memory load. J. Cogn. Neurosci. 26, 395–407. doi: 10.1162/jocn_a_00501
Smolka, M. N., Schumann, G., Wrase, J., Grusser, S. M., Flor, H., Mann, K., et al. (2005). Catechol-O-methyltransferase val158met genotype affects processing of emotional stimuli in the amygdala and prefrontal cortex. J. Neurosci. 25, 836–842. doi: 10.1523/JNEUROSCI.1792-04.2005
Spreng, R. N., Mar, R. A., and Kim, A. S. (2009). The common neural basis of autobiographical memory, prospection, navigation, theory of mind, and the default mode: a quantitative meta-analysis. J. Cogn. Neurosci. 21, 489–510. doi: 10.1162/jocn.2008.21029
Stein, D. J., Newman, T. K., Savitz, J., and Ramesar, R. (2006). Warriors versus worriers: the role of COMT gene variants. CNS Spectr. 11, 745–748. doi: 10.1017/S1092852900014863
Stokes, P. R. A., Rhodes, R. A., Grasby, P. M., and Mehta, M. A. (2011). The effects of the COMT val108/158met polymorphism on BOLD activation during working memory, planning, and response inhibition: a role for the posterior cingulate cortex? Neuropsychopharmacology 36, 763–771. doi: 10.1038/npp.2010.210
Swift-Scanlan, T., Smith, C. T., Bardowell, S. A., and Boettiger, C. A. (2014). Comprehensive interrogation of CpG island methylation in the gene encoding COMT, a key estrogen and catecholamine regulator. BMC Med. Genomics. 7:5. doi: 10.1186/1755-8794-7-5
Tian, T., Qin, W., Liu, B., Jiang, T., and Yu, C. (2013). Functional connectivity in healthy subjects is nonlinearly modulated by the COMT and DRD2 polymorphisms in a functional system-dependent manner. J. Neurosci. 33, 17519–17526. doi: 10.1523/JNEUROSCI.2163-13.2013
Tunbridge, E. M., Bannerman, D. M., Sharp, T., and Harrison, P. J. (2004). Catechol-o-methyltransferase inhibition improves set-shifting performance and elevates stimulated dopamine release in the rat prefrontal cortex. J. Neurosci. 24, 5331–5335. doi: 10.1523/JNEUROSCI.1124-04.2004
Tunbridge, E. M., Farrell, S. M., Harrison, P. J., and Mackay, C. E. (2013). Catechol-O-methyltransferase (COMT) influences the connectivity of the prefrontal cortex at rest. Neuroimage 68, 49–54. doi: 10.1016/j.neuroimage.2012.11.059
Vijayraghavan, S., Wang, M., Birnbaum, S. G., Williams, G. V., and Arnsten, A. F. (2007). Inverted-U dopamine D1 receptor actions on prefrontal neurons engaged in working memory. Nat. Neurosci. 10, 376–384. doi: 10.1038/nn1846
Winterer, G., Musso, F., Vucurevic, G., Stoeter, P., Konrad, A., Seker, B., et al. (2006). COMT genotype predicts BOLD signal and noise characteristics in prefrontal circuits. Neuroimage 32, 1722–1732. doi: 10.1016/j.neuroimage.2006.05.058
Keywords: fMRI, dopamine, COMT, delay discounting, sex differences
Citation: Elton A, Smith CT, Parrish MH and Boettiger CA (2017) COMT Val158Met Polymorphism Exerts Sex-Dependent Effects on fMRI Measures of Brain Function. Front. Hum. Neurosci. 11:578. doi: 10.3389/fnhum.2017.00578
Received: 03 October 2017; Accepted: 16 November 2017;
Published: 06 December 2017.
Edited by:
Jessica A. Turner, Georgia State University, United StatesReviewed by:
Yvette Nicole Lamb, University of Auckland, New ZealandWissam El-Hage, François Rabelais University, France
Copyright © 2017 Elton, Smith, Parrish and Boettiger. This is an open-access article distributed under the terms of the Creative Commons Attribution License (CC BY). The use, distribution or reproduction in other forums is permitted, provided the original author(s) or licensor are credited and that the original publication in this journal is cited, in accordance with accepted academic practice. No use, distribution or reproduction is permitted which does not comply with these terms.
*Correspondence: Charlotte A. Boettiger, Y2FiQHVuYy5lZHU=