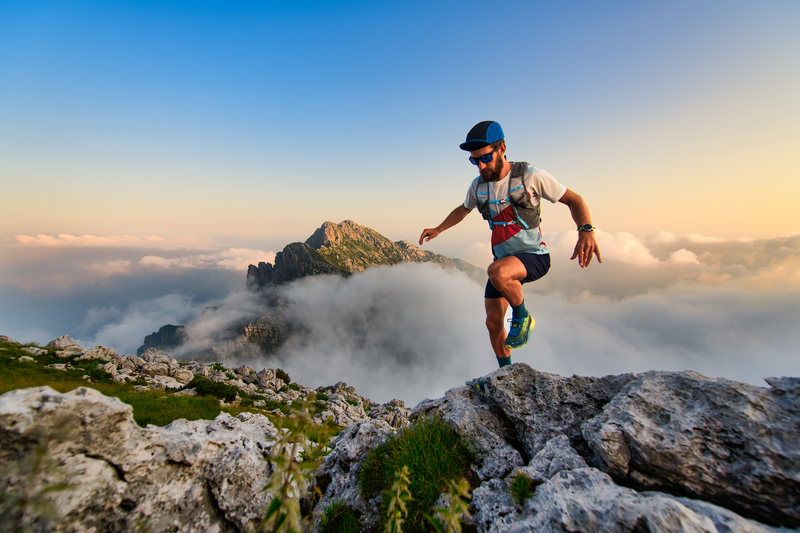
95% of researchers rate our articles as excellent or good
Learn more about the work of our research integrity team to safeguard the quality of each article we publish.
Find out more
ORIGINAL RESEARCH article
Front. Hum. Neurosci. , 03 February 2017
Sec. Brain Health and Clinical Neuroscience
Volume 11 - 2017 | https://doi.org/10.3389/fnhum.2017.00043
This article is part of the Research Topic Can't Get You Out of My Head: Brain-Body Interactions in Perseverative Cognition View all 10 articles
Anxiety impairs both inhibition of distraction and attentional focus. It is unclear whether these impairments are reduced or exacerbated when loading working memory with non-affective information. Cardiac vagal control has been related to top–down regulation of anxiety; therefore, vagal control may reflect load-related inhibition of distraction under anxiety. The present study examined whether: (1) the enhancing and impairing effects of load on inhibition exist together in a non-linear function, (2) there is a similar association between inhibition and concurrent vagal control under anxiety. During anxiogenic threat-of-noise, 116 subjects maintained a digit series of varying lengths (0, 2, 4, and 6 digits) while completing a visual flanker task. The task was broken into four blocks, with a baseline period preceding each. Electrocardiography was acquired throughout to quantify vagal control as high-frequency heart rate variability (HRV). There were significant quadratic relations of working memory load to flanker performance and to HRV, but no associations between HRV and performance. Results indicate that low load was associated with relatively better inhibition and increased HRV. These findings suggest that attentional performance under anxiety depends on the availability of working memory resources, which might be reflected by vagal control. These results have implications for treating anxiety disorders, in which regulation of anxiety can be optimized for attentional focus.
Anxiety disorders are characterized by distractibility and difficulty with focusing on daily activities (Beck et al., 2005). Such features are thought to partially result from the tendency of anxiety to impair inhibition of distractor interference, an executive function that involves overriding the influence of prepotent but irrelevant attentional stimuli (Friedman and Miyake, 2004; Bishop, 2007). Although inhibition can refer to suppression of a variety of responses, this term is used in this paper in specific reference to inhibition of distractor interference. Inhibition is a major requirement for attentional focus, and is typically gaged with Stroop-like tasks that involve conflict (Miyake et al., 2000; Lavie et al., 2004; Petersen and Posner, 2012). There are inconsistent findings about whether working memory (WM) load and its autonomic correlate, cardiac vagal control, improve or impair inhibition during anxiety (e.g., Pu et al., 2010; van Dillen and Derks, 2012; Berggren et al., 2013). The current study addressed this issue by testing for quadratic relations that encapsulate both the enhancing and impairing effects of WM load and cardiac vagal control on inhibition performance during anxiety.
Anxiety and other negative states tend to grab attention and disrupt the ability to inhibit irrelevant stimuli, even when those stimuli have little affective quality (e.g., Pallak et al., 1975; Hart et al., 2010; Dolcos et al., 2011; Choi et al., 2012). This impairment of inhibition might be attributed to cognitive aspects of anxiety (e.g., worry; Mathews, 1990; Borkovec et al., 1998). By consuming limited WM resources, anxiety reduces the capacity for distractor inhibition (Eysenck and Calvo, 1992; Hayes et al., 2008; Verkuil et al., 2009; Lavie, 2010).
Persistent anxiety and its attentional deficits are often treated with interventions that target the enhancement of emotion regulation (ER) skills that rely on WM (e.g., cognitive restructuring; Beck, 1979; Hofmann and Asmundson, 2008; Hofmann et al., 2009). A shared feature of many cognitive ER strategies is WM load, which involves filling up the capacity-limited “blackboard” for conscious thought (i.e., WM) with non-affective material (e.g., a letter string; Baddeley, 1992; Engle, 2002; Ochsner and Gross, 2008; van Dillen et al., 2009; Buhle et al., 2014). Therefore, in the current study, WM load is conceptualized as a core mechanism underlying voluntary, top–down regulation of emotion and anxiety. WM load increases tend to reduce anxiety and other negative emotional states by shifting cognitive resources away from emotion-laden thoughts (e.g., worry; van Dillen and Koole, 2007; van Dillen et al., 2009; Kanske et al., 2011; King and Schaefer, 2011). Through attenuating anxiety in this way, WM load increases also reduce anxiety-related impairments to inhibition and selective attention (Schutz and Davis, 2000; Bradley et al., 2010; van Dillen and Derks, 2012; Vytal et al., 2012; Clarke and Johnstone, 2013).
The notion that WM load enhances concurrent inhibition is consistent with the Neurovisceral Integration Model (Thayer and Lane, 2000, 2002, 2009). In this view, prefrontal cortex (PFC) areas related to WM tonically suppress subcortical areas important for anxiety and worry. Such PFC-mediated suppression is manifested as augmented cardiac vagal control, the vagus nerve’s inhibitory effect on heart rate (HR; Berntson, 1997; Ter Horst and Postema, 1997). Cardiac vagal control is often quantified by high-frequency variability in the HR time series that often occur in phase with oscillations in respiration (HF-HRV; Malliani et al., 1991). HRV will be hereinafter used to refer to vagally mediated HF-HRV. High HRV at rest and during tasks has been speculated to proxy PFC-mediated cognitive regulation (perhaps load-dependent regulation; see below) of negative emotional states, including anxiety (for reviews, see Thayer and Lane, 2002; Appelhans and Luecken, 2006; Friedman, 2007). High cardiac vagal control (i.e., high HRV), through reflecting the degree of cognitive regulation over performance-harming anxiety or “stress,” has been linked to improved inhibition and attentional performance (e.g., Hansen et al., 2003; Johnsen et al., 2003; Thayer et al., 2009; Elliot et al., 2011).
In the present study, we focused on HRV responses that relate to state regulatory efforts, as opposed to resting HRV, which reflects trait processes (Thayer et al., 2012). Within-subject increases in HRV might relate to WM load that regulates anxiety and enhances inhibition (Thayer and Lane, 2009). This possibility is supported by a number of studies. First, within-person increases in HRV tend to co-vary with ER strategies (e.g., reappraisal and expressive suppression) that load WM (Butler et al., 2006; Denson et al., 2011). Second, high HRV has been associated with simultaneously heightened dorsolateral PFC (a WM-related brain area) activity that relates to both reduced emotionality and increased WM load (Lane et al., 2009; Qin et al., 2009).
Contrary to the above-cited research, engagement in WM-dependent ER can impair performance on concurrent or subsequent tasks that require inhibition (Friese et al., 2013; Ortner et al., 2013). These effects may be explained by the Load Theory of Selective Attention and Cognitive Control, in which WM capacity is required for inhibition of distractor interference (de Fockert et al., 2001; Lavie et al., 2004). In this sense, ER’s inherent WM load is thought to reduce WM capacity for maintaining inhibition-related goals. As an indicator of WM-dependent ER, task levels of HRV might relate to ongoing impairments to inhibition driven by usage of WM resources. Partially supporting this notion, subjects with relatively higher resting (but not task) HRV showed a greater likelihood to use WM-dependent ER during a negative emotion picture paradigm, but showed worse performance on a subsequent Stroop task (Pu et al., 2010). In this prior study, it is possible that high HRV was associated with impaired inhibition because individuals with high HRV exhausted WM resources during ER.
Evidence for the deleterious impacts of WM load and of HRV on concurrent inhibition during anxiety is perplexing, in view of work that highlights the performance-enhancing qualities of WM load. Rather than treating these differing results as incompatible, it may be that both negative and positive relations among WM load and inhibition exist together within a larger non-linear function (Marcovitch et al., 2010). A novel theoretical model is presented here that specifies a quadratic relation between inhibition and WM load under high anxiety, with WM load being conceptualized as a core mechanism of anxiety regulation (Hendricks and Buchanan, 2015; Figure 1). In this quadratic function, increased load may help inhibition by reducing anxious cognitions when such increases are in the range of no load to moderate load (i.e., when minimal WM resources are drained from the concurrent inhibition task; Figure 1A). In parallel, WM load increases are also hypothesized to deplete shared resources, which may counteract any performance-enhancing effects, thereby flattening the positive load-inhibition relation from no to moderate load (Figure 1A). Moderate load may represent a critical point past which too much WM capacity is used; additional load impairs the ability to reduce distractor interference and hence causes an increasingly negative load-inhibition association (Lavie, 2005; Berggren et al., 2013; Ortner et al., 2013; Figure 1C). In that task HRV levels have been speculated to represent a bodily manifestation of load-related regulation of anxiety (i.e., WM load), a nearly identical quadratic function between HRV and concurrent inhibition was predicted (see Figure 1; Lane et al., 2009).
FIGURE 1. Hypothesized quadratic association between WM load and inhibition under high state anxiety. Sub-axes with dotted lines reflect association between concurrent anxiety levels and inhibition. (A) Represents no load (0 digits) followed by low load (2 digits). (B) Reflects moderate load (4 digits), and (C) represents high load (6 digits).
Partial support for the theoretical model came from a study that showed a quadratic HRV-performance relation in individuals who frequently use a WM-dependent ER strategy (i.e., expressive suppression; Spangler et al., 2015). Because cognitive resources were not manipulated, these effects may be attributable to other factors than WM, such as moderate HRV reflecting optimal levels of arousal for performance (see Marcovitch et al., 2010 for a similar quadratic function in children).
The primary aim of the current study was to examine quadratic associations between WM load and task HRV to inhibition under high state anxiety, in order to test whether intermediary levels of WM load and vagal control optimize distraction inhibition during anxiety. These aims were approached in an experiment that combined an anticipatory noise blast paradigm (to induce anxious cognition) with a common dual WM-inhibition task adapted from Lavie et al. (2004). While being either safe from or under threat of noise (Patrick and Berthot, 1995; Grillon et al., 2008, 2009), subjects loaded WM capacity and simultaneously completed an Eriksen flanker task, a common measure of inhibition (Lavie et al., 2004).
Under situations of high state anxiety (threat trials), WM load was predicted to show a negative quadratic association with inhibition performance (Hypothesis 1). An exploratory corollary of this hypothesis was that the function’s shape would resemble that depicted in Figure 1, such that moderate load would be associated with relatively optimal inhibition. Due to its theoretical links with WM-dependent regulation of anxiety (Lane et al., 2009), task HRV was predicted to have a positive linear relation with WM load under high state anxiety (threat trials; Hypothesis 2). Insofar that WM load is related to HRV during high anxiety, we predicted that task HRV would also show a negative quadratic association with inhibition performance (Hypothesis 3).
Special focus was given to contrasting relations of WM load and HRV to inhibition between threat and safe trials, in order to investigate unique mechanisms accounting for the “threat” function. Load increases under low anxiety (i.e., safety) should drain WM capacity without performance enhancements via anxiety reduction. Therefore, in testing relations of inhibition to load and HRV under safety, a general absence of curvilinearity was predicted. Hypotheses were tested with a series of multilevel models that were conducted with and without self-reported subjective anxiety as a covariate, in order to assess whether the resultant functions were driven by emotional factors rather than by WM load.
Subjects were 120 (68 female) undergraduates at Virginia Tech (Mage = 19.3 years, SD= 2.8 years), who were recruited both online and with flyers posted on campus. Participation was compensated with extra credit in a psychology course. Exclusionary criteria were made on the basis of self-reported: (1) cigarette smoking or tobacco use, (2) diagnoses of cardiovascular disease, (3) and psychiatric/neurological disorders. Subjects were instructed to abstain from alcohol for 24 h, caffeine for 12 h, food for 2 h, and vigorous exercise for 2 h prior to participation. Four subjects out of 120 enrolled were excluded due to equipment malfunction, yielding 116 subjects retained for analyses (66 female; Mage = 19.1 years, SD = 1.85 years). This study was approved by the Virginia Tech Institutional Review Board, and informed consent was obtained from all subjects.
Subjects were greeted by the experimenter upon arrival at the lab and informed of the nature of the study and the noise blast paradigm. After providing written consent, subjects were attached to physiological recording equipment, and they completed self-report questionnaires. Next, two practice trials of the experimental task were conducted with noise delivery, and subjects were given the opportunity to ask questions about the task.
After the first physiological baseline recording, subjects performed the experimental task, which was comprised of 28 trials, of which there were seven trials for each level of WM load (0, 2, 4, 6; see below). Each trial included a series of flanker responses (to measure inhibition) as well as WM maintenance; a typical trial is described in detail under the Experimental Task section below. Twelve of the 28 trials involved safety from noise blast (three safety trials per level of load), while another 12 trials included threat of noise blast without actual noise delivery (three threat trials per load level). Of importance, an additional four trials included threat of noise blast with the delivery of actual noise (1 blast trial per level of load). This design yielded 24 retained trials (12 trials for safety and 12 trials for threat); the four threat trials with noise blast were excluded from data analysis due to the confounding effects of startle and pain on performance (Kalisch et al., 2006). In threat trials, delivery of noise, or lack thereof, was randomized with the qualification that 25% of threat trials would involve actual noise (see Procedure above; Kalisch et al., 2006). In these trials, the timing of the blasts was randomly determined so that only one noise occurred during the flanker/WM section of the trial. Randomization of trial and stimulus delivery was implemented within the DMDX software (Forster and Forster, 2003).
Each subject completed all 28 trials and thus experienced 4 threat trials and 3 safety trials for every WM level (0, 2, 4, and 6). For the WM manipulation, digit series lengths of 0, 2, 4, and 6 were chosen to correspond to no, low, moderate, and high WM load, respectively (Lavie et al., 2004; van Dillen et al., 2013). To avoid switching costs, the seven trials with the same level of WM load were blocked together, and safety/threat was randomly counterbalanced within each of the WM blocks (Lavie et al., 2004). This created four WM load blocks that were randomly counterbalanced. Each of the four WM blocks was preceded by a 3-min “vanilla” baseline that was composed of a calming nature film (Jennings et al., 1992). Multiple baselines were incorporated for a more accurate representation of Task HRV for each level of WM. The entire run of the experiment lasted about 1 h.
A typical trial of the experimental task is depicted in Figure 2. Trials were scripted and presented on a PC using DMDX software (Forster and Forster, 2003). At the beginning of the trial, subjects heard one of two tones via headphones. A low tone indicated safety (0% chance) from white noise blast, and a high tone indicated threat (i.e., “some possibility”) of noise blast that may be delivered at some point in the upcoming trial. Following tone presentation, a fixation cross appeared on the computer screen for 500 ms. Next, subjects saw a series of digits that were presented for 2 s. When subjects viewed these numbers, they were expected to silently keep in mind the digits for the remainder of the trial, rather than focus on their emotions. They were also expected to correctly answer a recognition probe later in the trial. The length of the presented series (# digits) varied between 0, 2, 4, and 6, depending on the WM block. For each trial, digits in the series were chosen at random from 1 to 9 with the following qualifications: no more than two consecutive digits could appear in the series (e.g., 1, 2, 3, 7), and the same numbers could not be used twice (e.g., 7, 7, 9, 5; Lavie et al., 2004). Once the series disappeared, subjects rehearsed the digit series for the remainder of the trial. Specifications for digit presentation were adapted from Lavie et al. (2004), with the added instructions that subjects should maintain the series rather than focusing on their emotions. A masking array was subsequently presented for 1 s to visually orient subjects for the upcoming flanker task.
While maintaining digits silently, the subject completed a series of 14 flanker sub-trials for each of the 28 trials of the experimental task. Each flanker sub-trial began with a 500 ms fixation cross. In accord with Lavie et al. (2004), a single flanker sub-trial consisted of a target letter in lowercase (the letters x or z) that was presented in the middle of the screen. At the same time, a peripheral distractor letter was presented at a subtended location relative to the target. For each response, subjects were asked to ignore the peripheral letter and to classify the target letter as an x or z by typing 1 or 2, respectively, on a computer keyboard. The visual array of letters lasted 100 ms, and then subjects were given 2 s to classify the letter with a typed response. Length of sub-trials did not vary by subjects’ response times, such that the next flanker sub-trial was always initiated after 2 s. The intertrial interval for flanker sub-trials was 200 ms. The flanker sub-trials differed such that the target was either congruent with the peripheral letter (e.g., target z and peripheral z) or incongruent with the peripheral letter (e.g., target z and peripheral x, or target x and peripheral z). In each overall trial (safe or threat), 7 of the flanker sub-trials were incongruent and the other 7 sub-trials were congruent, with their order being randomized within each of the 28 trials.
Unlike other studies, 14 sub-trials were combined to create a ∼39.5 s period of dual task performance (flanker responses with concomitant WM maintenance). This was done to satisfy the 30 s length as a potential minimum for reliable HRV recording (G. Berntson, personal communication, September 10, 2014; Task Force of the European Society of Cardiology and the North American Society of Pacing and Electrophysiology, 1996).
After the series of flanker sub-trials with WM maintenance, a recognition probe appeared on the screen for 5 s. The probe consisted of a single letter; the subject indicated whether or not the letter appeared in the preceding series by pressing 1 or 2, respectively. At the end of the trial, subjects were given another 5 s to retrospectively report anxiety experienced during the preceding flanker/WM task. A single trial of the experimental task lasted approximately 55 s, and the task was scripted so that the length of trials did not vary by response time.
Digit series length during the flanker task varied such that load level assumed the following values for each subject: 0, 2, 4, and 6. Although these values were the result of an experimental manipulation (see above), they are conceptualized as a variable that is on a continuous ratio-like scale (Braver et al., 1997).
Response times (RT) to classify target letters amidst distractors were collected. Accuracy in classifying letters was also attained, but RT was of primary interest for hypothesis testing. As in Lavie et al. (2004), only RTs from correct classifications were included in interference calculations and analyses. Interference scores were computed by subtracting RTs of congruent trials from that of incongruent trials, and this difference score served as a measure of inhibition performance (Stins et al., 2004; Dennis and Chen, 2007; Hart et al., 2010; Qi et al., 2014). This method yielded 28 interference scores for each person (one difference score for each of the seven threat and seven safe trials for each level of WM load). To better compare results to that of other studies, interference scores were reverse scored before being entered into data analyses, such that higher levels on this measure indexed relatively better inhibition. Higher inhibition scores reflected smaller differences between congruent and incongruent trials in RTs to classify targets, suggesting relatively better suppression of incongruent stimuli (Lavie et al., 2004).
At the end of each trial (threat, safe), anxious experience was reported on a 7-point Likert Scale to the following question: “How anxious did you feel during the letter task?” Higher numbers indicated greater state anxiety, such that 1 indicated that anxiety was “not at all” present and 7 denoted that anxiety was “very” present (van Dillen et al., 2009).
Information was collected about health issues that could potentially confound the validity of study findings. This questionnaire allowed experimenters to validate whether subjects followed the abstention recommendations outlined above.
Electrocardiography (ECG) was continuously recorded throughout the experimental session. ECG was collected with Ag/AgCl spot electrodes on the subject’s thorax at a modified Lead II configuration in which one electrode at the right collarbone and the other at the bottom left rib. Analog ECG was amplified with the ECG100C (Biopac Systems Inc., Goleta, CA, USA), and then integrated and sampled with an MP150 device (Biopac Systems Inc., Goleta, CA, USA). Digital signals were next routed and saved to a PC in the next room for offline analysis using AcqKnowledge software (Version 4.3). A modified Pan-Tompkins algorithm was conducted on ECG waveforms to identify R-spikes. R-spikes that were missing or misclassified due to motion artifact (which occurred in less than <1% of R-spikes) were manually identified and corrected in the ECG record. The rare cases of unidentifiable and ectopic beats (<1% of R-spikes) were removed from the ECG time series (Lippman et al., 1994). Interbeat intervals (IBI) were then computed from the ECG signal as the distance between consecutive R-spikes in millisecond (ms) units. Using Kubios software (Version 2.2), HRV was derived from the IBI signal using a Fast Fourier Transform function and quantified as spectral power (ms2) in the domain of normal respiration (0.15–0.4 Hz; Task Force of the European Society of Cardiology and the North American Society of Pacing and Electrophysiology, 1996).
Separate HRV estimates were yielded for all four baseline periods. Baseline HRV was derived from the last 2 min of each 3-min IBI time series in order to remove vagal influences related to cardiac stress recovery. To assess task-specific HRV accounting for baseline levels, a series of reactivity difference scores was computed for each trial by subtracting HRV during flanker performance/WM maintenance (duration = 39.5 s) from the preceding baseline HRV value (Llabre et al., 1991). This process yielded 28 different task HRV values per subject. Prior to creating differences scores, HRV values were log transformed to normalize their distribution. Task IBI levels were calculated with reactivity difference scores in the same manner.
The aversive stimulus was a 3-s, 105 dB blast of PC-generated white noise (adapted from Grillon et al., 2008, 2009). Noise level was controlled with an external amplifier, and noise blasts were delivered via headphones.
Variables were inspected for skew and both HRV and self-reported anxiety were log transformed to normalize their distributions. Before entering data into analyses, severe outliers were excluded (>3.5 SD). With this criterion, 18 inhibition scores (out of 2,784 scores across participants; <1%) and 11 Task HRV values (out of 2,784 values; <1%) were excluded. Hypotheses were tested with a series of random intercept models, a type of multilevel model that accounts for nesting of observations (inhibition performance, HRV) within subjects with a random slope (Raudenbush and Bryk, 2002). This method, unlike ordinary least squares regression, prevents violating assumptions of non-independence and allows for more fine-grained estimation of within- (Level-1) and between-subject (Level-2) variation (Kreft and de Leeuw, 1998). Level-1 intercepts were allowed to randomly vary between subjects and all other predictors were estimated as fixed effects, and can be interpreted as the average of within-person relations among inhibition, HRV, and load across subjects (Kreft and de Leeuw, 1998). The structure of primary models are specified below.
Working memory Load and its quadratic effect were treated as continuous variables. Quadratic terms for WM Load were built by first mean centering and then squaring WM Load’s linear term (Cohen et al., 2003). Linear and curvilinear by linear interactions between WM Load and other variables (e.g., Trial and Trait Anxiety) were computed by multiplying terms, and these interactions were probed with simple slope analysis (Aiken and West, 1991; Cohen et al., 2003). In each model, Level-1 continuous variables (e.g., WM Load, Self-reported Anxiety, HRV) were group-mean centered to reduce multicollinearity and to aid interpretation of coefficients (Kreft et al., 1995). For each hypothesis, analyses were conducted to substantiate significant interactions of load or HRV with Trial (Threat, Safe) before testing hypothesized relations in threat and safety contexts separately (Robinson et al., 2013). Trial (Threat, Safe) was coded as a dummy variable, such that 0 and 1 represented threat and safe trials, respectively.
All multilevel models presented in relation to primary hypotheses included self-reported anxiety as a covariate. This was done because, counter to the theoretical model, results indicate that WM load increases were met with increases rather than decreases in anxiety (see below). As such, it became increasingly desirable to examine relatively “pure” effects of WM load and their physiological correlates (Task HRV) apart from the unexpected changes in anxiety.
To test Hypothesis 1, a comprehensive random intercept model was used to assess whether the quadratic association between WM load and performance was moderated by Trial (Safe, Threat).
Level-1: Flanker performance = β0j + β1j (WM Load)ij + β2j (WM Load)2ij + β3j (Trial)ij + β4j (Self-reported anxiety)ij + β5j (WM Load × Trial)ij + β6j (WM Load2× Trial)ij + Rij
Level-2: β = ϒ00 + U0j
If the interaction between WM Load2 and Trial was significant, models containing WM Load, WM Load2, and self-reported anxiety as predictors and flanker performance as the outcome measure were conducted for threat and safe trials separately (Cohen et al., 2003; Robinson et al., 2013).
Hypothesis 2 was tested with random intercept models of the same form as that which was used to examine Hypothesis 1, except flanker performance was replaced by Task HRV as the outcome measure. Quadratic terms for WM Load were modeled for exploratory purposes. To test Hypothesis 3, models were conducted that were identical to those used for Hypothesis 1, except that linear and quadratic terms for WM Load were switched for Task HRV and Task HRV2, respectively. Analyses relating to manipulation checks and basic model tenets were conducted with a series multilevel models and t-tests.
The effectiveness of the anticipatory noise blast paradigm in increasing state anxiety was examined with a random intercept model, in which Trial (threat, safe) was modeled as a fixed effect on trials from the 0 Load condition (i.e., when there were little to no WM demands). This analysis generated a significant effect of Trial (B = -0.690, p < 0.001), which suggests that during no WM load there were higher levels of subjective anxiety during threat than in safe trials. Descriptive statistics for all variables appear in Table 1.
Paired sample t-tests were conducted to examine HRV changes from baseline to threat and from baseline to safety. Compared to baseline, HRV was lower during threat, t(115) = 4.96, p < 0.001, Cohen’s d= 0.201, and safe trials, t(115) = 3.31, p = 0.001, Cohen’s d= 0.127. Baseline-to-task changes in IBI were handled with the same statistical approach. IBI contrasts for threat, t(115) = 1.95, p = 0.054, Cohen’s d= 0.058, and safety, t(115) = 0.328, p = 0.748, Cohen’s d= 0.008, were not significant. For a direct examination of threat-of-noise on HRV, a random intercept model containing Trial as a fixed effect was conducted on HRV during no load (i.e., 0 Load) trials, and there was no significant effect for Trial (B = 0.056, p = 0.255).
The model above that tested effects of Trial (Threat, Safe) on self-reported anxiety was used to investigate WM load’s effect on diminishing anxiety. In addition to the Trial effect (see above), there was a significant positive association between WM Load and self-reported anxiety (B = 0.015, p = 0.005) for threat trials. There was also a significant interaction between Trial and WM Load (B = 0.036, p < 0.001), such that the positive association between WM Load and anxiety was stronger in safe relative to threat trials.
To substantiate that threat of noise blast negatively impacted inhibition performance, a multilevel model was conducted only on trials from 0 Load blocks. In this analysis, performance was the outcome measure and Trial was treated as a fixed effect. Inhibition performance was lower during unregulated threat compared to safe trials, as indicated by a significant effect of Trial (B = 22.39, p = 0.002).
The random intercept model examining Load effects on inhibition between threat and safety yielded significant effects for Trial (B = -13.83, p = 0.031) and WM Load2 (B = -1.31, p = 0.045). The main effect of WM Load2 was qualified by a significant WM Load2× Trial interaction (B = 3.36, p < 0.001). This interaction confirms that the quadratic relation between WM load and inhibition differs between threat and safe trials and justifies follow-up tests of WM Load effects for threat separately. See Table 2 for a summary of random intercept models that tested load-inhibition relations.
For the model that examined threat trials, only the effect of WM Load2 was significant (B = -1.31, p = 0.038), which indicated a negative quadratic function between WM load and inhibition performance under high state anxiety (i.e., threat). The precise shape of this function can be seen in Figure 3.
FIGURE 3. Quadratic association between WM load and inhibition during threat of noise blast. WM load levels: 0 (no load), 2 (low load), 4 (moderate load), and 6 (high load).
This quadratic relation can be explained as follows. Load increases from no to low load (0 to 2 digits) were associated with augmentations in performance, such that there was a positive load-inhibition relation. This positive relation reversed completely at low load (2 digits), whereby load increases from low to moderate load (2–4 digits) were met with decreases in inhibition performance (i.e., a negative relation). The negative relation grew stronger as load increased to 6 digits. The quadratic trend indicates that inhibition performance under anxiety is relatively better during low load (2 digits) compared to both no load and higher load (4 and 6 digits).
The multilevel model examining load effects in safe trials indicated that there was a significant linear relation between WM Load and inhibition (B = -3.26, p = 0.009), but this linear effect was qualified by a significant quadratic association between WM Load and inhibition (B = 2.13, p = 0.001). As is seen in Figure 4, the negative relation appeared to attenuate and flatten across levels of load, until there was a slight reversal of the load-inhibition association from moderate to high load (4–6 digits).
FIGURE 4. Quadratic association between WM Load and inhibition during safety from noise blast. Note. WM load levels: 0 (no load), 2 (low load),4 (moderate load), and 6 (high load).
The random intercept model that examined load effects on HRV between threat and safety revealed no significant interaction between WM Load and Trial (B = 0.007, p = 0.603). In examining threat trials singularly, the linear load-HRV relation was not significant (see model statistics in Table 3). However, for threat trials, there was an unpredicted significant effect for WM Load2 (B = -0.015, p = 0.006), as well as for Self-reported Anxiety (B = -0.096, p = 0.010). There were no significant effects in the model examining safety trials. Further inspection of WM Load’s quadratic effect under threat (see Figure 5) indicates that there was a positive load-HRV association from no to low load, which began to reverse from low to moderate low. The association then becomes increasingly negative, such that further increases in load past moderate levels (4 digits) were met with reductions in Task HRV.
FIGURE 5. Quadratic association between WM Load and Task IIRV during threat of noise blast, Note. WM load levels: 0 (no load), 2 (low load),4 (moderate load), and 6 (high load).
The multilevel model examining effects of HRV and HRV2 on inhibition between threat and safety indicated that there was no significant interaction between HRV2 and Trial (B = 3.91, p = 0.401). In fact, there were no significant main effects or interaction in this model. See Table 3 for a summary of random intercept models examining HRV’s relations to inhibition. In the model examining threat trials only, the quadratic association between Task HRV and inhibition was not significant (B = 3.91, p = 0.401). In general, these data indicate that there were no associations between Task HRV and inhibition performance.
The primary aim of this study was to test for a negative quadratic relation between WM load and inhibition of distractors under high state anxiety, and to examine whether cardiac vagal control reflects WM load that both enhances and impairs inhibition under anxiety. Results partially confirmed hypotheses by showing a negative quadratic function between WM load and inhibition under high state anxiety. A number of unpredicted but potentially fruitful results emerged, which include a quadratic association between WM load and task HRV, in which HRV was highest under low load relative to all other load levels.
Contrary to hypotheses, there were no direct relations of HRV to inhibition. Findings suggest that under high state anxiety, the relation of WM load to distractor inhibition and cardiac vagal control depend on the availability of WM capacity (Lavie et al., 2004; Schmeichel et al., 2008; Thayer and Lane, 2009). As is discussed below, such availability, which might be reflected in task HRV, is the result of opposing effects of load-dependent anxiety reduction and load-dependent consumption of cognitive resources (Pessoa, 2009).
Differences in anxiety ratings between threat and safety trials indicate that the noise blast paradigm was effective in inducing anxious cognition, as has been shown previously (Grillon and Ameli, 1998; Skolnick and Davidson, 2002; Lissek et al., 2005; Grillon et al., 2008). Further supporting the model and prior research, induced anxiety impaired inhibition, as is shown by worse inhibition in threat relative to safe trials during unregulated anxiety (e.g., Bishop et al., 2004; Hart et al., 2010; Choi et al., 2012). Contrary to the model and previous studies (e.g., van Dillen et al., 2009; Vytal et al., 2012; Patel et al., 2015), WM load increases were related to augmentations, and not reductions, in self-reported anxiety. It is possible that load reduced anxiety’s cognitive components (i.e., worry), which are related to WM, while bodily aspects of anxiety persisted (i.e., “anxious arousal) to be reflected in the self-report (Endler and Kocovski, 2001; Vytal et al., 2012; Sharp et al., 2015). Previous studies suggest that anxiety-related interoceptive cues can be detected consciously and thus self-reported; however, these interoceptive functions implicate neural functions that are not directly tied to WM (Nitschke et al., 1999; Critchley et al., 2004).
Hypothesis 1 was partially supported in that there was a negative quadratic relation under high but not low state anxiety. Potential mechanisms that drive the non-linearity in the function can be clarified by focusing on its linear components, as is done below.
As hypothesized, there was a positive relation between load and inhibition in the range of no to low WM load. This positive relation is consistent with other studies in which relatively high load enhanced concurrent performance through attenuating negative emotional processing (Vytal et al., 2012; Clarke and Johnstone, 2013; Patel et al., 2015). High relative to low levels of WM load can speed reaction times to classify a happy target face amidst an angry distractor face, as well as reduce neural processing of the angry distractor face (van Dillen and Derks, 2012). As suggested in previous research, load increases in the present study may have enhanced inhibition by leaving less WM capacity for maintaining performance-harming anxiety (van Dillen and Koole, 2007). Prior studies have shown better performance under high versus low load, but the current study only showed a performance enhancement for low compared to no load, potentially because high load in the current task was especially demanding (three task demands) compared to prior research. Therefore, it is possible that present load effects across the entire function are restricted to a high range of WM load that is well beyond that of prior studies. That is, all of the present study’s load conditions might correspond to “high” load in other studies. Such a possibility is speculative, as a direct comparison of load conditions is difficult due to different tasks being used between studies (e.g., n-back, arithmetic problems, Sternberg WM task). Yet, lack of inhibition enhancements from no to low load under safety supports the notion that enhancements during threat were caused by anxiety reductions, because safety did not likely involve enough anxiety to allow for notable load-dependent anxiety reductions.
In accord with the model, there was a reversal of the positive linear relation between load and inhibition under anxiety such that the relation became negative from low to moderate load. As WM capacity became increasingly scarce, higher load related to relatively worse inhibition under anxiety. The negative load-inhibition relation became even stronger from moderate to high levels of load. These findings may be due to reliance of distractor inhibition on limited WM capacity (Baddeley, 1992; Engle, 2002), and because high load tends to worsen inhibition of irrelevant visual distractors (Lavie, 2005, 2010). The reversal and intensification of the load-inhibition relation suggests that competition between WM load and other cognitive functions (e.g., inhibition) may be stronger when WM capacity limits are reduced and resources are scarce (Cowan, 2001; Pessoa, 2009; Forster, 2013). In effect, as WM was increasingly depleted past low load, performance may have been impaired in proportion to capacity availability, such that load-induced impairments increasingly outweighed concurrent load-dependent performance improvements. As mentioned above, it is possible that load’s impairments to inhibitions require heavy taxation of WM capacity. Previous studies may have missed this section of the function because the present study’s added task demands made the 6-digit condition sufficiently high to impair inhibition (e.g., van Dillen and Derks, 2012; Vytal et al., 2012).
A discrepancy between the yielded function and the model (Figure 1) is that there was neither attenuation nor a plateau in the positive relation at moderate load. In effect, inhibition performance under anxiety was optimal under low rather than moderate load. It is possible that performance would have been even better if three digits were maintained, a condition not included in this study. Another possibility is that low load represents a meaningful level of WM usage past which further load increases drain resources needed for inhibition. If the latter is the case, a logical query arises as to why load increases from no to low load were uniquely associated with inhibition enhancements rather than impairments. A potential explanation might relate to the fact that: (1) emotion-related cognition demands more cognitive resources than low load neutral cognition (Vytal et al., 2012), and (2) the attenuation of anxiety by load is stronger under high relative to low anxiety (van Dillen and Koole, 2007; Stout et al., 2013). By shifting resources away from heavily depleting anxiety, low load likely frees up much more WM capacity than it fills with digit maintenance alone, and this effect may improve concurrent inhibition performance.
Compared to low load neutral information, threatening stimuli strongly consume WM capacity, as measured by neural and behavioral measures (Dolcos and McCarthy, 2006; Stout et al., 2013). In the current study, poor inhibition at no relative to low load may have been caused by unregulated anxiety (0 Load) draining more shared resources than low load maintenance (e.g., Hajcak and Olvet, 2008; Kanske et al., 2011; Dolcos and Denkova, 2014). Second, smaller amounts of WM load might be more effective at clearing anxious cognition from WM capacity when WM resources are increasingly used by these cognitions (van Dillen and Koole, 2007). Thus, from no to low load (when there is increased anxious cognition in WM capacity), minimal task-related increases in load may have dissipated anxious cognition and thus had a net effect of freeing up more WM capacity than was filled by low load manipulation (i.e., 2 digits). As such, this free capacity was available for the concurrent inhibition task. With further increases in task-related load, however, inhibition may have declined because less WM capacity was free for maintaining inhibition goals (Miller and Cohen, 2001; Lavie et al., 2004; Qi et al., 2014). This “capacity availability” account of results is supported by unexpected HRV findings, as is discussed below.
It was hypothesized that task HRV would reflect WM load used to decrease anxious cognition. Rather than supporting a linear relation between HRV and WM-dependent regulation over anxiety, a more complex non-linear association between WM load and HRV was observed. This quadratic association aligns with elements of the Neurovisceral Integration Model and past studies that view vagal control not as an index of degree of cognitive regulation, but as a reflection of PFC resources available for ongoing cognitive-affective demands (Jorna, 1992; Elliot et al., 2011). In fact, Thayer and Lane (2009, p. 85) have suggested that “HRV functions at both the trait and state levels as a resource.”
Heart rate variability changes in response to load followed a similar trend to that of load-induced performance changes. Task HRV was highest at low levels of load (2 digits), when inhibition was optimized; then, the load-HRV association reversed from low to moderate load just as the load-inhibition association did (see Figures 3 and 5). Since inhibition is dependent on WM availability, it is possible that task HRV reflected the degree of “free” WM capacity that resulted from both anxiety reduction and load itself (Engle, 2002; Lavie, 2010). At low load, when inhibition performance was optimized, HRV may have reflected a large amount of WM resources that were salvaged through “deleting” anxious cognition and made available for removal of distractor interference (Dolcos and Denkova, 2014). The decline of HRV after low load may reflect WM capacity being increasingly filled with task-related load, consistent with the parallel load-dependent decreases in performance seen in Figure 3 (Croizet et al., 2004). If HRV is interpreted as an indicator of resource availability, the yielded load-HRV quadratic relation is consistent with studies in which task HRV was negatively related to ongoing task demands and positively related to cognitive performance that requires high levels of available WM capacity (Hansen et al., 2003; Lehrer et al., 2010; Elliot et al., 2011; Allen and Friedman, 2016).
The absence of the predicted quadratic association between HRV and inhibition conflicts with our finding of a quadratic function between HRV and executive function in those who frequently use a WM-dependent ER strategy (Spangler et al., 2015). This quadratic association included resting HRV, which unlike phasic HRV, has been theoretically linked to trait processes whereby ER’s costly effects potentially accrue over time (Butler et al., 2006). Although there has been one report of a quadratic association between task HRV and executive function in children (Marcovitch et al., 2010), it might be that task HRV taps into the state-related availability of resources that can be used for inhibition of distraction.
Since many ER strategies entail WM loading, the present findings qualify theoretical perspectives in which ER is held to assist performance via the use of executive control (Thayer and Lane, 2000; Blair and Ursache, 2011; Cohen et al., 2012). Cognitive regulation of high anxiety may only enhance concurrent inhibition insofar as that regulation does not heavily load WM. Clarification is also given to the view of ER as damaging to attentional focus by suggesting that ER strategies may only hurt performance when they are highly loading, as in the case of expressive suppression (Kalisch et al., 2006; Goldin et al., 2008; Friese et al., 2013; Ortner et al., 2013).
Regarding cardiac vagal control, our findings indicate that deploying WM resources in the service of ER does not cause simple increases in HRV, as might be predicted from previously shown HRV augmentations during ER. The present results instead suggest that on-task HRV levels reflect inter-function competition of WM-related regulation, anxiety, and inhibition. This view is somewhat inconsistent with the Neurovisceral Integration Model, which highlights the anatomical-functional integration of ER and “cold” executive functions, which work together in self-regulation (Thayer and Lane, 2009). However, by virtue of integrated neurocognitive resources in the PFC, there is inherent resource competition between emotion, ER, and executive control, of which HRV might be a reflection (Pessoa, 2008, 2009).
The current study also underscores the potential value of using minimally loading ER strategies for treatment in anxiety disorders, of which a major feature is difficulty in concentration (Beck et al., 2005). Interventions like CBT that involve complex cognitive ER strategies (e.g., reappraisal) may do more harm than good by impairing anxious individuals’ ability to inhibit irrelevant information, and in doing so, worsen anxious symptoms (Olatunji et al., 2007). ER strategies might be better chosen according to their level of load, so that damaging effects on attention and daily functioning are minimized.
The present study has limitations that might be addressed in future research on the relationships among load, inhibition, and HRV under anxiety. First, state anxiety was only measured via self-report, which has been shown to diverge from other aspects of anxiety (Sharp et al., 2015). Future studies might include measures of eyeblink startle to more comprehensively assess anxious states and to better substantiate the left side of the yielded non-linear functions (Grillon, 2008). There was also no direct WM capacity measure in this study. Future research could include neuroimaging to more directly index resource competition at the central nervous system level. Although HRV data from noise blast trials were removed from analyses, it is conceivable that the noise blasts influenced HRV estimates in surrounding trials. This possibility is somewhat unlikely, as cardiac vagal responses to noise blast return to baseline levels within a time period (i.e., three to four heartbeats; <5 s) shorter than the present study’s intervals between HRV measurements (Chen et al., 2014). This study also had a number of strengths that should be noted, including a relatively large sample size and many within-subjects observations. These factors allowed for a powerful test of hypothesized three-way interactions (e.g., quadratic effects varying between safety and threat).
In sum, this study provides evidence that minimal WM load can attenuate the impairing effects of anxiety on distractor inhibition, while more heavily loading tasks may do just as much harm to inhibition as anxiety itself. The current study also underscores cardiac vagal control as a potential correlate of WM resource availability, a factor that relates to attentional performance under threat. Broadly speaking, this study may inform treatments for anxiety disorders, in which regulation of emotion and anxiety can be modified to prevent lapses in attention.
This study was approved by the Institutional Review Board (IRB) at Virginia Tech. All participants were greeted and the experimenter explained each section of the informed consent form, in order to educate participants on the nature of the study procedures and purpose, risks and benefits, as well as their freedom to withdraw at any point time with no penalty. Participants were then given the opportunity to ask questions, after which they signed the informed consent form. No vulnerable populations were used in this study.
DS wrote this under the mentorship and direction of BF. DS developed the research question and conducted this study as a part of his dissertation, with BF providing invaluable feedback and edits on the project’s implementation and on the submitted manuscript
The authors declare that the research was conducted in the absence of any commercial or financial relationships that could be construed as a potential conflict of interest.
Special thanks to Xiao Yang, Lilian Hummer, and Laura Braunstein for their assistance with this study.
Aiken, L. S., and West, S. G. (1991). Multiple regression: Testing and Interpreting Interactions. Thousand Oaks, CA: Sage.
Allen, B., and Friedman, B. H. (2016). Threatening the heart and mind of gender stereotypes: can imagined contact influence the physiology of stereotype threat? Psychophysiology 53, 105–112. doi: 10.1111/psyp.12580
Appelhans, B. M., and Luecken, L. J. (2006). Heart rate variability as an index of regulated emotional responding. Rev. Gen. Psychol. 10:229. doi: 10.1037/1089-2680.10.3.229
Beck, A. T., Emery, G., and Greenberg, R. L. (2005). Anxiety Disorders and Phobias: A Cognitive Perspective. New York, NY: Basic Books.
Berggren, N., Richards, A., Taylor, J., and Derakshan, N. (2013). Affective attention under cognitive load: reduced emotional biases but emergent anxiety-related costs to inhibitory control. Front. Hum. Neurosci. 7:188. doi: 10.3389/fnhum.2013.00188
Berntson, G. G. (1997). Heart rate variability: origins, methods, and interpretive caveats. Psychophysiology 34, 623–648. doi: 10.1111/j.1469-8986.1997.tb02140.x
Bishop, S., Duncan, J., Brett, M., and Lawrence, A. D. (2004). Prefrontal cortical function and anxiety: controlling attention to threat-related stimuli. Nat. Neurosci. 7, 184–188. doi: 10.1038/nn1173
Bishop, S. J. (2007). Neurocognitive mechanisms of anxiety: an integrative account. Trends Cogn. Sci. 11, 307–316. doi: 10.1016/j.tics.2007.05.008
Blair, C., and Ursache, A. (2011). “A bidirectional model of executive functions and self-regulation,” in Handbook of Self-Regulation, 2nd Edn, eds R. F. Baumeister and K. D. Vohs (New York, NY: Guilford Press), 300–320.
Borkovec, T. D., Ray, W. J., and Stober, J. (1998). Worry: a cognitive phenomenon intimately linked to affective, physiological, and interpersonal behavioral processes. Cognit. Ther. Res. 22, 561–576. doi: 10.1023/A:1018790003416
Bradley, R. T., McCraty, R., Atkinson, M., Tomasino, D., Daugherty, A., and Arguelles, L. (2010). Emotion self-regulation, psychophysiological coherence, and test anxiety: results from an experiment using electrophysiological measures. Appl. Psychophysiol. Biofeedback 35, 261–283. doi: 10.1007/s10484-010-9134-x
Braver, T. S., Cohen, J. D., Nystrom, L. E., Jonides, J., Smith, E. E., and Noll, D. C. (1997). A parametric study of prefrontal cortex involvement in human working memory. Neuroimage 5, 49–62. doi: 10.1006/nimg.1996.0247
Buhle, J. T., Silvers, J. A., Wager, T. D., Lopez, R., Onyemekwu, C., Kober, H., et al. (2014). Cognitive reappraisal of emotion: a meta-analysis of human neuroimaging studies. Cereb. Cortex 24, 2981–2990. doi: 10.1093/cercor/bht154
Butler, E. A., Wilhelm, F. H., and Gross, J. J. (2006). Respiratory sinus arrhythmia, emotion, and emotion regulation during social interaction. Psychophysiology 43, 612–622. doi: 10.1111/j.1469-8986.2006.00467.x
Chen, K. H., Aksan, N., Anderson, S. W., Grafft, A., and Chapleau, M. W. (2014). Habituation of parasympathetic-mediated heart rate responses to recurring acoustic startle. Front. Psychol. 5:1288. doi: 10.3389/fpsyg.2014.01288
Choi, J. M., Padmala, S., and Pessoa, L. (2012). Impact of state anxiety on the interaction between threat monitoring and cognition. Neuroimage 59, 1912–1923. doi: 10.1016/j.neuroimage.2011.08.102
Clarke, R., and Johnstone, T. (2013). Prefrontal inhibition of threat processing reduces working memory interference. Front. Hum. Neurosci. 7:228. doi: 10.3389/fnhum.2013.00228
Cohen, J., Cohen, P., West, S. G., and Aiken, L. S. (2003). Applied Multiple Regression/Correlation Analysis for the Behavioral Sciences. Mahwah, NJ: Erlbaum.
Cohen, N., Henik, A., and Moyal, N. (2012). Executive control attenuates emotional effects–for high reappraisers only? Emotion 12:970. doi: 10.1037/a0026890
Cowan, N. (2001). Metatheory of storage capacity limits. Behav. Brain Sci. 24, 154–176. doi: 10.1017/S0140525X0161392X
Critchley, H. D., Wiens, S., Rotshtein, P., Öhman, A., and Dolan, R. J. (2004). Neural systems supporting interoceptive awareness. Nat. Neurosci. 7, 189–195. doi: 10.1038/nn1176
Croizet, J. C., Després, G., Gauzins, M. E., Huguet, P., Leyens, J. P., and Méot, A. (2004). Stereotype threat undermines intellectual performance by triggering a disruptive mental load. Pers. Soc. Psychol. Bull. 30, 721–731. doi: 10.1177/0146167204263961
de Fockert, J. W., Rees, G., Frith, C. D., and Lavie, N. (2001). The role of working memory in visual selective attention. Science 291, 1803–1806. doi: 10.1126/science.1056496
Dennis, T. A., and Chen, C. C. (2007). Neurophysiological mechanisms in the emotional modulation of attention: the interplay between threat sensitivity and attentional control. Biol. Psychol. 76, 1–10. doi: 10.1016/j.biopsycho.2007.05.001
Denson, T. F., Grisham, J. R., and Moulds, M. L. (2011). Cognitive reappraisal increases heart rate variability in response to an anger provocation. Motiv. Emot. 35, 14–22. doi: 10.1007/s11031-011-9201-5
Dolcos, F., and Denkova, E. (2014). Current emotion research in cognitive neuroscience: linking enhancing and impairing effects of emotion on cognition. Emot. Rev. 6, 362–375. doi: 10.1177/1754073914536449
Dolcos, F., Iordan, A. D., and Dolcos, S. (2011). Neural correlates of emotion–cognition interactions: a review of evidence from brain imaging investigations. J. Cogn. Psychol. 23, 669–694. doi: 10.1080/20445911.2011.594433
Dolcos, F., and McCarthy, G. (2006). Brain systems mediating cognitive interference by emotional distraction. J. Neurosci. 26, 2072–2079. doi: 10.1523/JNEUROSCI.5042-05.2006
Elliot, A. J., Payen, V., Brisswalter, J., Cury, F., and Thayer, J. F. (2011). A subtle threat cue, heart rate variability, and cognitive performance. Psychophysiology 48, 1340–1345. doi: 10.1111/j.1469-8986.2011.01216.x
Endler, N. S., and Kocovski, N. L. (2001). State and trait anxiety revisited. J. Anxiety Disord. 15, 231–245. doi: 10.1016/S0887-6185(01)00060-3
Engle, R. W. (2002). Working memory capacity as executive attention. Curr. Dir. Psychol. Sci. 11, 19–23. doi: 10.1111/1467-8721.00160
Eysenck, M. W., and Calvo, M. G. (1992). Anxiety and performance: the processing efficiency theory. Cognit. Emot. 6, 409–434. doi: 10.1080/02699939208409696
Forster, K. I., and Forster, J. C. (2003). DMDX: a Windows display program with millisecond accuracy. Behav. Res. Methods Instrum. Comput. 35, 116–124. doi: 10.3758/BF03195503
Forster, S. (2013). Distraction and mind-wandering under load. Front. Psychol. 4:283. doi: 10.3389/fpsyg.2013.00283
Friedman, B. H. (2007). An autonomic flexibility–neurovisceral integration model of anxiety and cardiac vagal tone. Biol. Psychol. 74, 185–199.
Friedman, N. P., and Miyake, A. (2004). The relations among inhibition and interference control functions: a latent-variable analysis. J. Exp. Psychol. 133:101. doi: 10.1037/0096-3445.133.1.101
Friese, M., Binder, J., Luechinger, R., Boesiger, P., and Rasch, B. (2013). Suppressing emotions impairs subsequent stroop performance and reduces prefrontal brain activation. PLoS ONE 8:e60385. doi: 10.1371/journal.pone.0060385
Goldin, P. R., McRae, K., Ramel, W., and Gross, J. J. (2008). The neural bases of emotion regulation: reappraisal and suppression of negative emotion. Biol. Psychiatry 63, 577–586. doi: 10.1016/j.biopsych.2007.05.031
Grillon, C. (2008). Models and mechanisms of anxiety: evidence from startle studies. Psychopharmacology 199, 421–437. doi: 10.1007/s00213-007-1019-1
Grillon, C., and Ameli, R. (1998). Effects of threat of shock, shock electrode placement and darkness on startle. Int. J. Psychophysiol. 28, 223–231. doi: 10.1016/S0167-8760(97)00072-X
Grillon, C., Lissek, S., Rabin, S., McDowell, D., Dvir, S., and Pine, D. S. (2008). Increased anxiety during anticipation of unpredictable but not predictable aversive stimuli as a psychophysiologic marker of panic disorder. Am. J. Psychiatry 165, 898–904. doi: 10.1176/appi.ajp.2007.07101581
Grillon, C., Pine, D. S., Lissek, S., Rabin, S., Bonne, O., and Vythilingam, M. (2009). Increased anxiety during anticipation of unpredictable aversive stimuli in posttraumatic stress disorder but not in generalized anxiety disorder. Biol. Psychiatry 66, 47–53. doi: 10.1016/j.biopsych.2008.12.028
Hajcak, G., and Olvet, D. M. (2008). The persistence of attention to emotion: brain potentials during and after picture presentation. Emotion 8:250. doi: 10.1037/1528-3542.8.2.250
Hansen, A. L., Johnsen, B. H., and Thayer, J. F. (2003). Vagal influence on working memory and attention. Int. J. Psychophysiol. 48, 263–274. doi: 10.1016/S0167-8760(03)00073-4
Hart, S. J., Green, S. R., Casp, M., and Belger, A. (2010). Emotional priming effects during Stroop task performance. Neuroimage 49, 2662–2670. doi: 10.1016/j.neuroimage.2009.10.076
Hayes, S., Hirsch, C., and Mathews, A. (2008). Restriction of working memory capacity during worry. J. Abnorm. Psychol. 117:712. doi: 10.1037/a0012908
Hendricks, M. A., and Buchanan, T. W. (2015). Individual differences in cognitive control processes and their relationship to emotion regulation. Cogn. Emot. 30, 912–924. doi: 10.1080/02699931.2015.1032893
Hofmann, S. G., and Asmundson, G. J. (2008). Acceptance and mindfulness-based therapy: new wave or old hat? Clin. Psychol. Rev. 28, 1–16. doi: 10.1016/j.cpr.2007.09.003
Hofmann, S. G., Heering, S., Sawyer, A. T., and Asnaani, A. (2009). How to handle anxiety: the effects of reappraisal, acceptance, and suppression strategies on anxious arousal. Behav. Res. Ther. 47, 389–394. doi: 10.1016/j.brat.2009.02.010
Jennings, J. R., Kamarck, T., Stewart, C., Eddy, M., and Johnson, P. (1992). Alternate cardiovascular baseline assessment techniques: vanilla or resting baseline. Psychophysiology 29, 742–750. doi: 10.1111/j.1469-8986.1992.tb02052.x
Johnsen, B. H., Thayer, J. F., Laberg, J. C., Wormnes, B., Raadal, M., Skaret, E., et al. (2003). Attentional and physiological characteristics of patients with dental anxiety. J. Anxiety Disord. 17, 75–87. doi: 10.1016/S0887-6185(02)00178-0
Jorna, P. G. (1992). Spectral analysis of heart rate and psychological state: a review of its validity as a workload index. Biol. Psychol. 34, 237–257. doi: 10.1016/0301-0511(92)90017-O
Kalisch, R., Wiech, K., Herrmann, K., and Dolan, R. J. (2006). Neural correlates of self-distraction from anxiety and a process model of cognitive emotion regulation. J. Cogn. Neurosci. 18, 1266–1276. doi: 10.1162/jocn.2006.18.8.1266
Kanske, P., Heissler, J., Schönfelder, S., Bongers, A., and Wessa, M. (2011). How to regulate emotion? Neural networks for reappraisal and distraction. Cereb. Cortex 21, 1379–1388. doi: 10.1093/cercor/bhq216
King, R., and Schaefer, A. (2011). The emotional startle effect is disrupted by a concurrent working memory task. Psychophysiology 48, 269–272. doi: 10.1111/j.1469-8986.2010.01062.x
Kreft, I. G., De Leeuw, J., and Aiken, L. S. (1995). The effect of different forms of centering in hierarchical linear models. Multivariate Behav. Res. 30, 1–21. doi: 10.1207/s15327906mbr3001_1
Lane, R. D., McRae, K., Reiman, E. M., Chen, K., Ahern, G. L., and Thayer, J. F. (2009). Neural correlates of heart rate variability during emotion. Neuroimage 44, 213–222. doi: 10.1016/j.neuroimage.2008.07.056
Lavie, N. (2005). Distracted and confused?: selective attention under load. Trends Cogn. Sci. 9, 75–82. doi: 10.1016/j.tics.2004.12.004
Lavie, N. (2010). Attention, distraction, and cognitive control under load. Curr. Dir. Psychol. Sci. 19, 143–148. doi: 10.1177/0963721410370295
Lavie, N., Hirst, A., de Fockert, J. W., and Viding, E. (2004). Load theory of selective attention and cognitive control. J. Exp. Psychol. 133:339. doi: 10.1037/0096-3445.133.3.339
Lehrer, P., Karavidas, M., Lu, S. E., Vaschillo, E., Vaschillo, B., and Cheng, A. (2010). Cardiac data increase association between self-report and both expert ratings of task load and task performance in flight simulator tasks: an exploratory study. Int. J. Psychophysiol. 76, 80–87. doi: 10.1016/j.ijpsycho.2010.02.006
Lippman, N. E. A. L., Stein, K. M., and Lerman, B. B. (1994). Comparison of methods for removal of ectopy in measurement of heart rate variability. Am. J. Physiol. Heart Circ. Physiol. 267, H411–H418.
Lissek, S., Baas, J. M., Pine, D. S., Orme, K., Dvir, S., Nugent, M., et al. (2005). Airpuff startle probes: an efficacious and less aversive alternative to white-noise. Biol. Psychol. 68, 283–297. doi: 10.1016/j.biopsycho.2004.07.007
Llabre, M. M., Spitzer, S. B., Saab, P. G., Ironson, G. H., and Schneiderman, N. (1991). The reliability and specificity of delta versus residualized change as measures of cardiovascular reactivity to behavioral challenges. Psychophysiology 28, 701–711. doi: 10.1111/j.1469-8986.1991.tb01017.x
Malliani, A., Pagani, M., Lombardi, F., and Cerutti, S. (1991). Cardiovascular neural regulation explored in the frequency domain. Circulation 84, 482–492. doi: 10.1161/01.CIR.84.2.482
Marcovitch, S., Leigh, J., Calkins, S. D., Leerks, E. M., O’Brien, M., and Blankson, A. N. (2010). Moderate vagal withdrawal in 3.5-year-old-children is associated with optimal performance on executive function tasks. Dev. Psychobiol. 52, 603–608. doi: 10.1002/dev.20462
Mathews, A. (1990). Why worry? The cognitive function of anxiety. Behav. Res. Ther. 28, 455–468. doi: 10.1016/0005-7967(90)90132-3
Miller, E. K., and Cohen, J. D. (2001). An integrative theory of prefrontal cortex function. Annu. Rev. Neurosci. 24, 167–202. doi: 10.1146/annurev.neuro.24.1.167
Miyake, A., Friedman, N. P., Emerson, M. J., Witzki, A. H., Howerter, A., and Wager, T. D. (2000). The unity and diversity of executive functions and their contributions to complex “frontal lobe” tasks: a latent variable analysis. Cognit. Psychol. 41, 49–100. doi: 10.1006/cogp.1999.0734
Nitschke, J. B., Heller, W., Palmieri, P. A., and Miller, G. A. (1999). Contrasting patterns of brain activity in anxious apprehension and anxious arousal. Psychophysiology 36, 628–637. doi: 10.1111/1469-8986.3650628
Ochsner, K. N., and Gross, J. J. (2008). Cognitive emotion regulation insights from social cognitive and affective neuroscience. Curr. Dir. Psychol. Sci. 17, 153–158. doi: 10.1111/j.1467-8721.2008.00566.x
Olatunji, B. O., Cisler, J. M., and Tolin, D. F. (2007). Quality of life in the anxiety disorders: a meta-analytic review. Clin. Psychol. Rev. 27, 572–581. doi: 10.1016/j.cpr.2007.01.015
Ortner, C. N., Zelazo, P. D., and Anderson, A. K. (2013). Effects of emotion regulation on concurrent attentional performance. Motiv. Emot. 37, 346–354. doi: 10.1007/s11031-012-9310-9
Pallak, M. S., Pittman, T. S., Heller, J. F., and Munson, P. (1975). The effect of arousal on Stroop color-word task performance. Bull. Psychon. Soc. 6, 248–250. doi: 10.3758/BF03336652
Patel, N., Vytal, K., Pavletic, N., Stoodley, C., Pine, D. S., Grillon, C., et al. (2015). Interaction of threat and verbal working memory in adolescents. Psychophysiology 53, 518–526. doi: 10.1111/psyp.12582
Patrick, C. J., and Berthot, B. D. (1995). Startle potentiation during anticipation of a noxious stimulus: active versus passive response sets. Psychophysiology 32, 72–80. doi: 10.1111/j.1469-8986.1995.tb03408.x
Pessoa, L. (2008). On the relationship between emotion and cognition. Nat. Rev. Neurosci. 9, 148–158. doi: 10.1038/nrn2317
Pessoa, L. (2009). How do emotion and motivation direct executive control? Trends Cogn. Sci. 13, 160–166. doi: 10.1016/j.tics.2009.01.006
Petersen, S. E., and Posner, M. I. (2012). The attention system of the human brain: 20 years after. Annu. Rev. Neurosci. 35:73. doi: 10.1146/annurev-neuro-062111-150525
Pu, J., Schmeichel, B. J., and Demaree, H. A. (2010). Cardiac vagal control predicts spontaneous regulation of negative emotional expression and subsequent cognitive performance. Biol. Psychol. 84, 531–540. doi: 10.1016/j.biopsycho.2009.07.006
Qi, S., Zeng, Q., Luo, Y., Duan, H., Ding, C., Hu, W., et al. (2014). Impact of working memory load on cognitive control in trait anxiety: an ERP study. PLoS ONE 9:e111791. doi: 10.1371/journal.pone.0111791
Qin, S., Hermans, E. J., van Marle, H. J., Luo, J., and Fernández, G. (2009). Acute psychological stress reduces working memory-related activity in the dorsolateral prefrontal cortex. Biol. Psychiatry 66, 25–32. doi: 10.1016/j.biopsych.2009.03.006
Raudenbush, S. W., and Bryk, A. S. (2002). Hierarchical Linear Models: Applications and Data Analysis Methods. Thousand Oaks, CA: Sage.
Robinson, C. D., Tomek, S., and Schumacker, R. E. (2013). Tests of moderation effects: difference in simple slopes versus the interaction term. Mult. Linear Regression Viewp. 39, 16–25.
Schmeichel, B. J., Volokhov, R. N., and Demaree, H. A. (2008). Working memory capacity and the self-regulation of emotional expression and experience. J. Pers. Soc. Psychol. 95:1526. doi: 10.1037/a0013345
Schutz, P. A., and Davis, H. A. (2000). Emotions and self-regulation during test taking. Educ. Psychol. 35, 243–256. doi: 10.1207/S15326985EP3504_03
Sharp, P. B., Miller, G. A., and Heller, W. (2015). Transdiagnostic dimensions of anxiety: neural mechanisms, executive functions, and new directions. Int. J. Psychophysiol. 98, 365–377. doi: 10.1016/j.ijpsycho.2015.07.001
Skolnick, A. J., and Davidson, R. J. (2002). Affective modulation of eyeblink startle with reward and threat. Psychophysiology 39, 835–850. doi: 10.1111/1469-8986.3960835
Spangler, D. P., Bell, M. A., and Deater-Deckard, K. (2015). Emotion suppression moderates the quadratic association between RSA and executive function. Psychophysiology 52, 1175–1185. doi: 10.1111/psyp.12451
Stins, J. F., Van Baal, G. C. M., Polderman, T. J., Verhulst, F. C., and Boomsma, D. I. (2004). Heritability of Stroop and flanker performance in 12-year old children. BMC Neurosci. 5:49. doi: 10.1186/1471-2202-5-49
Stout, D. M., Shackman, A. J., and Larson, C. L. (2013). Failure to filter: anxious individuals show inefficient gating of threat from working memory. Front. Hum. Neurosci. 7:58. doi: 10.3389/fnhum.2013.00058
Task Force of the European Society of Cardiology and the North American Society of Pacing and Electrophysiology (1996). Heart rate variability: standards of measurement, physiological interpretation and clinical use. Circulation 93, 1043–1065. doi: 10.1161/01.CIR.93.5.1043
Ter Horst, G. J., and Postema, F. (1997). Forebrain parasympathetic control of heart activity: retrograde transneuronal viral labeling in rats. Am. J. Physiol. Heart Circ. Physiol. 273, H2926–H2930.
Thayer, J. F., Åhs, F., Fredrikson, M., Sollers, J. J., and Wager, T. D. (2012). A meta-analysis of heart rate variability and neuroimaging studies: implications for heart rate variability as a marker of stress and health. Neurosci. Biobehav. Rev. 36, 747–756. doi: 10.1016/j.neubiorev.2011.11.009
Thayer, J. F., Hansen, A. L., Saus-Rose, E., and Johnsen, B. H. (2009). Heart rate variability, prefrontal neural function, and cognitive performance: the neurovisceral integration perspective on self-regulation, adaptation, and health. Ann. Behav. Med. 37, 141–153. doi: 10.1007/s12160-009-9101-z
Thayer, J. F., and Lane, R. D. (2000). A model of neurovisceral integration in emotion regulation and dysregulation. J. Affect. Disord. 61, 201–216. doi: 10.1016/S0165-0327(00)00338-4
Thayer, J. F., and Lane, R. D. (2002). Perseverative thinking and health: neurovisceral concomitants. Psychol. Health 17, 685–695. doi: 10.1080/08870440290025867
Thayer, J. F., and Lane, R. D. (2009). Claude Bernard and the heart–brain connection: further elaboration of a model of neurovisceral integration. Neurosci. Biobehav. Rev. 33, 81–88. doi: 10.1016/j.neubiorev.2008.08.004
van Dillen, L. F., and Derks, B. (2012). Working memory load reduces facilitated processing of threatening faces: an ERP study. Emotion 12:1340. doi: 10.1037/a0028624
van Dillen, L. F., Heslenfeld, D. J., and Koole, S. L. (2009). Tuning down the emotional brain: an fMRI study of the effects of cognitive load on the processing of affective images. Neuroimage 45, 1212–1219. doi: 10.1016/j.neuroimage.2009.01.016
van Dillen, L. F., and Koole, S. L. (2007). Clearing the mind: a working memory model of distraction from negative mood. Emotion 7, 715. doi: 10.1037/1528-3542.7.4.715
van Dillen, L. F., Papies, E. K., and Hofmann, W. (2013). Turning a blind eye to temptation: how cognitive load can facilitate self-regulation. J. Pers. Soc. Psychol. 104:427. doi: 10.1037/a0031262
Verkuil, B., Brosschot, J. F., Borkovec, T. D., and Thayer, J. F. (2009). Acute autonomic effects of experimental worry and cognitive problem solving: why worry about worry? Int. J. Clin. Health Psychol. 9, 439–453.
Keywords: anxiety, heart rate variability (HRV), distractor interference, working memory, inhibition
Citation: Spangler DP and Friedman BH (2017) A Little Goes a Long Way: Low Working Memory Load Is Associated with Optimal Distractor Inhibition and Increased Vagal Control under Anxiety. Front. Hum. Neurosci. 11:43. doi: 10.3389/fnhum.2017.00043
Received: 29 July 2016; Accepted: 19 January 2017;
Published: 03 February 2017.
Edited by:
Cristina Ottaviani, Santa Lucia Foundation, ItalyReviewed by:
Michael Gaebler, Max Planck Institute for Human Cognitive and Brain Sciences and University of Leipzig, GermanyCopyright © 2017 Spangler and Friedman. This is an open-access article distributed under the terms of the Creative Commons Attribution License (CC BY). The use, distribution or reproduction in other forums is permitted, provided the original author(s) or licensor are credited and that the original publication in this journal is cited, in accordance with accepted academic practice. No use, distribution or reproduction is permitted which does not comply with these terms.
*Correspondence: Derek P. Spangler, ZHBzcGFuZ0BnbWFpbC5jb20=
†Present address: Derek P. Spangler, Department of Psychology, The Ohio State University, Columbus, OH, USA
Disclaimer: All claims expressed in this article are solely those of the authors and do not necessarily represent those of their affiliated organizations, or those of the publisher, the editors and the reviewers. Any product that may be evaluated in this article or claim that may be made by its manufacturer is not guaranteed or endorsed by the publisher.
Research integrity at Frontiers
Learn more about the work of our research integrity team to safeguard the quality of each article we publish.