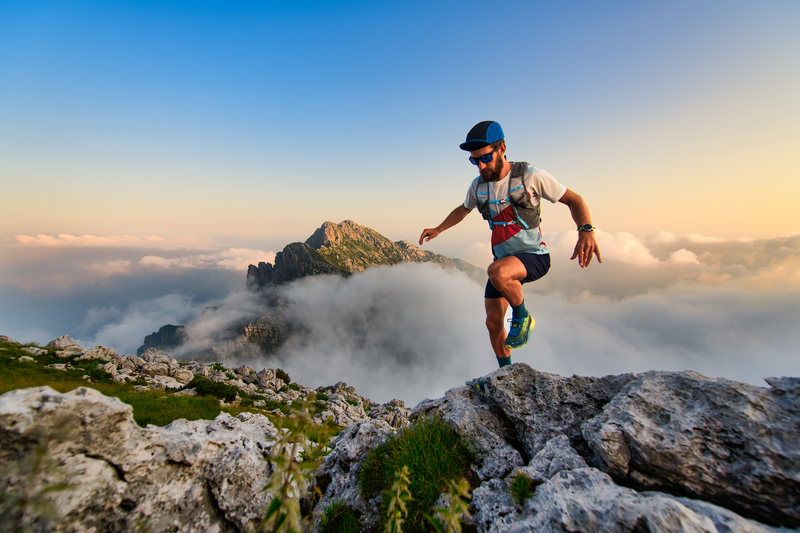
94% of researchers rate our articles as excellent or good
Learn more about the work of our research integrity team to safeguard the quality of each article we publish.
Find out more
ORIGINAL RESEARCH article
Front. Hum. Neurosci. , 02 November 2016
Sec. Brain Imaging and Stimulation
Volume 10 - 2016 | https://doi.org/10.3389/fnhum.2016.00540
Catechol-O-methyltransferase (COMT) and brain-derived neurotrophic factor (BDNF) genes have been found to interactively influence working memory (WM) as well as brain activation during WM tasks. However, whether the two genes have interactive effects on resting-state activities of the brain and whether these spontaneous activations correlate with WM are still unknown. This study included behavioral data from WM tasks and genetic data (COMT rs4680 and BDNF Val66Met) from 417 healthy Chinese adults and resting-state fMRI data from 298 of them. Significant interactive effects of BDNF and COMT were found for WM performance as well as for resting-state regional homogeneity (ReHo) in WM-related brain areas, including the left medial frontal gyrus (lMeFG), left superior frontal gyrus (lSFG), right superior and medial frontal gyrus (rSMFG), right medial orbitofrontal gyrus (rMOFG), right middle frontal gyrus (rMFG), precuneus, bilateral superior temporal gyrus, left superior occipital gyrus, right middle occipital gyrus, and right inferior parietal lobule. Simple effects analyses showed that compared to other genotypes, subjects with COMT-VV/BDNF-VV had higher WM and lower ReHo in all five frontal brain areas. The results supported the hypothesis that COMT and BDNF polymorphisms influence WM performance and spontaneous brain activity (i.e., ReHo).
Working memory (WM) refers to a limited capacity system allowing the temporary storage and manipulation of information (Baddeley and Hitch, 1974) and plays a crucial role in a wide range of cognitive tasks such as reading, problem solving, and learning (Baddeley and Della Sala, 1983; Conway et al., 2003). Researchers have recently examined the genetic underpinnings of WM and identified two candidate genes, Catechol-O-methyltransferase (COMT) and brain-derived neurotrophic factor (BDNF; Egan et al., 2001; Numata et al., 2007; Nagel et al., 2008; Wang et al., 2013).
COMT has been found to be associated with dopamine concentration in the prefrontal cortex (PFC; Lachman et al., 1996b; Akil et al., 2003; Chen et al., 2004), which is in turn associated with WM (Egan et al., 2001). The COMT Met allele has also been found to be associated with attention shifting, which plays an important role in the updating of WM (Nolan et al., 2004) and efficient physiological responses during the two-back WM task (Egan et al., 2001). Interestingly, studies have found ethnic differences in the effects of COMT. Most studies that used Caucasian samples (Bruder et al., 2005; Aguilera et al., 2008) found that compared with the Val allele, the Met allele was correlated with better cognitive abilities, especially WM. On the other hand, in Asian samples, the Met allele was instead associated with higher incidence of major depressive disorder (MDD; Wang et al., 2016), lower IQ (Qian et al., 2009), lower scores on competency tests (Yeh et al., 2009), and lower WM (Wang et al., 2013).
BDNF plays an important role in neuronal survival and differentiation and synaptic plasticity. Even though not all studies are consistent (for exceptions, see Egan et al., 2003; Hansell et al., 2007), there is a growing number of studies showing associations between BDNF (and its product BDNF) and cognitive ability. On the biological level, several animal studies have shown that performance on WM tasks can be affected by both BDNF overexpression (Papaleo et al., 2011) and under-expression in mice (Li et al., 2010). Higher BDNF levels in the frontal cortex have been linked to fewer WM errors in aged rats (Bimonte et al., 2003) and Ts65D mice (Bimonte-Nelson et al., 2003). In human studies, BDNF rs6265 Val/Met polymorphisms have been correlated with WM. A study of healthy Han Chinese found that BDNF rs6265 Val homozygotes performed better in digital WM tasks than did Met homozygotes (Gong et al., 2009). In studies of bipolar patients, the Met variant of BDNF (rs6265) has been associated with altered hippocampus and PFC formation, which are in turn linked to poorer WM performance. Egan et al. (2003) found that a common BDNF (val66met) polymorphism was associated with episodic memory, although not with WM as mentioned earlier.
It should be mentioned that due to genetic pleiotropy, both COMT and BDNF have been found to be associated with several other aspects of cognition beyond WM, including reaction time (Das et al., 2014), cognitive flexibility (Kang et al., 2013), aging-related cognitive degradation (Ghisletta et al., 2014; Voelcker-Rehage et al., 2015), executive function (Leckie et al., 2014; Sapkota et al., 2015), and neurocognitive deficits in people with schizophrenia (Ahmed et al., 2015) or Parkinson's disease (Khalil et al., 2016). Nevertheless, WM has been the main focus of the previous literature in this field.
In terms of specific interaction effects of the two genes on WM, however, there was only one study that found that the effect of COMT on WM was modulated by BDNF in a sample of aging Germans (Nagel et al., 2008). Other studies suggested such an effect indirectly by demonstrating similar interaction effects on the anatomy of the hippocampus (Hajek et al., 2012) and PFC (Gothelf et al., 2005; Ho et al., 2007; Takahashi et al., 2008), brain cortical plasticity and frontostriatal circuit brain activities (Wang et al., 2015), all of which could affect memory performance. Interactions between COMT and BDNF polymorphisms exist for WM likely because both genes are widely expressed in the cortex and white matter (in humans and mice, see The Human Protein Atlas, http://www.proteinatlas.org/, and Allen Brain Atlas, http://www.brainatlas.org) (Su et al., 2004). They both have been found to influence dopamine levels in the midbrain (Meyer-Lindenberg et al., 2005; Berton et al., 2006) and the PFC (Lachman et al., 1996a; Gogos et al., 1998; Malhotra et al., 2002; Chen et al., 2004).
The current study aimed to explore the potential interactions between BDNF Val66Met and COMT Val158Met on resting brain regional homogeneity (ReHo) as well as WM in a sample of Chinese subjects. Resting-state fMRI has increasingly been used to investigate neural basis of cognitive ability including WM (Christoff and Gabrieli, 2000; Stark and Squire, 2001) both in patients and in healthy people. For example, a previous study found that resting-state functional connectivity was positively correlated with WM performance (Hampson et al., 2006). Zou et al. (2013) further found that the amplitude of low-frequency fluctuation (ALFF) of certain brain areas such as the precuneus was correlated with WM performance (Zou et al., 2013). ReHo, calculated by using Kendall's coefficient concordance (KCC) to measure the degree of synchronization between the time-series of a voxel and its nearest voxels, is believed to reflect the regional spontaneous blood oxygenation level dependent (BOLD) fluctuations of the whole brain during rest (Zang et al., 2004). Many studies have found abnormal ReHo associated with various brain diseases, such as depression (Guo et al., 2011; Peng et al., 2011), Parkinson's disease (Wu et al., 2009), autism spectrum disorder (Paakki et al., 2010), and Alzheimer's disease and mild cognitive impairment (Zhang et al., 2012). Compared to resting-state functional connectivity (RSFC), which focuses on the long-distance interregional temporal correlations of BOLD signals, ReHo focuses on the functions within each region, in the case of this study, the frontal lobe. Although, no study has examined the effects of COMT and BDNF on ReHo of resting-state brain activity, the aforementioned literature review led us to hypothesize that COMT and BDNF would have main and interactive effects on both WM and ReHo.
Participants were Chinese college students recruited from Beijing Normal University. Genetic data (BDNF and COMT) and WM data were available for 428 participants, but 11 participants were excluded from further analysis because they were outliers in their WM performance (outside of 3 standard deviations of the group mean). Of the remaining 417 subjects (57% female, mean age = 21.31 years), resting state fMRI data were available for 298 participants (Table 1). All subjects were Han Chinese and reported no history of psychiatric diseases, head injuries, or stroke/seizure. Moreover, scores on the Beck Depression Inventory (Beck et al., 1996) and Beck Anxiety Inventory (Beck and Steer, 1990) showed that none of the participants met the criteria for either major depression or anxiety disorder. No participants met the criterion for nicotine dependence according to the Fagerström Test for Nicotine Dependence (Heatherton et al., 1991), but four participants in the larger sample reported using a large amount of alcohol based on the Alcohol Use Disorders Identification Test (Saunders et al., 1993). This experiment was approved by the Institutional Review Board (IRB) of the State Key Laboratory of Cognitive Neuroscience and Learning at Beijing Normal University, China. A written consent form was obtained from each participant after a full explanation of the study procedure. Participants were compensated for their participation.
WM performance was assessed by a two-back WM paradigm with three subtasks (semantic, phonological, and morphological judgment). Each task included four blocks of 10 trials each. Participants were asked to make continuous judgments between the word currently presented and the word presented two trials earlier in terms of their semantic (whether they belonged to the same semantic category), phonological (whether they rhymed with each other), or morphological relations (whether they were the same Tibetan character; Xue et al., 2004). For more details on the specific procedure, please see Li et al. (2011). Following the previous studies (Fry and Hale, 1996; Rosenheck et al., 2006; Zhu et al., 2010; Li et al., 2011; Wang and Gathercole, 2013), the average accuracy scores of the three WM subtasks were used in order to obtain a more stable and reliable estimate of WM. We also measured the participants' IQ with the Wechsler Adult Intelligence Scale. IQ was used as a control variable.
The genomic DNA for each participant was extracted from a 4 ml venous blood sample and purified by standard procedures. The COMT val158met was genotyped using PCR (Qian et al., 2003) with the following primers (forward: 5′-TCGTGGACGCCGTGATTCAGG-3′ and reverse: 5′-ACAACGGGTCAGGCATGCA-3′). The PCR mixture consisted of × 1 Taq Buffer (MBI), 0.2 mM dNTP, 0.25 mM primers, 1 mM MgCl2, 1.0 unit Taq polymorphism and 100 ng DNA. PCR was initiated at 95⋅C for 3 min, then at 94⋅C for 30 s, 68⋅C for 60 s, 72⋅C for 90 s for 35 cycles, then extended at 72⋅C for 7 min. The Val158Met variation was differentiated using NlaIII restriction fragment length polymorphism analysis on 4% agarose gel electrophoresis. Two common fragments (81 and 22 bp) and variable fragments of 114 bp (Val158/wild type) and 96 + 18 bp (Met158/mutant) were available. Genotypes were determined from Gel Doc 2000 (Bio-Rad, Hercules, CA). Because the short fragments (22 and 18 bp) diffused in the agarose gel, only three bands (114, 96, and 81 bp) were detectable.
BDNF was genotyped through the polymerase chain reaction-restriction fragment length polymorphism (PCR-RFLP) method. The forward primer was ITS-1: (5′-ACTCTGGAGAGCGTGAAT-3′) and the reverse primer was ITS-2: (5′-ATACTGTCACACACGCTC-3′). The PCR amplification reactions were performed in a 15 μl reaction mixture containing 2 μl template DNA, 1.5 μl MgCl2, 0.3 μl of each primer, 0.3 μl dNTP, 0.12 μl Taq polymerase, 1.5 μl 10 × buffer, 3 μl PCR Optimizer, and water up to a total volume of 15 μl. The thermocycler conditions were as follows: 95⋅C for 5 min, 35 cycles of 95⋅C for 30 s, 62⋅C for 30 s, 72⋅C for 30 s, and a final extension at 72⋅C for 10 min. Amplified products of 308 bp was electrophoresed in a 2% agarose gel and visualized with ethidium bromide. The polymorphism was genotyped via restriction digestion with 5 μl of the PCR product, 2U Eco72I, 0.8 μl buffer, 0.08 μl BSA, and water up to the total volume of 8 μl. The digested products were electrophoresed on a 2% agarose gel and visualized with ethidium bromide staining.
Ambiguous or unidentifiable results were re-amplified and rescored. A subset of the samples (10%) were randomly selected and tested twice for confirmation. The detection rates of COMT and BDNF were 97.9 and 99.5%, respectively, in the original larger sample, but only subjects with good genotype data were later enrolled in the WM and brain imaging study.
MR images were collected using a SIEMENS TRIO 3-Tesla scanner in the Brain Imaging Center of Beijing Normal University. Participants lay supine with their heads snugly fixed by a belt and foam pads to minimize head motion. Each participant underwent an 8 min resting state fMRI (RS-fMRI) scanning session and a 3D anatomic session. During the RS-fMRI session, the participants were instructed to keep their eyes closed, be as still as possible, and not to think about anything in particular. Images were obtained with the following parameters: 33 axial slices, thickness/gap = 3/0.6 mm, matrix = 64*64, repetition time (TR) = 2000 ms, echo time (TE) = 30 ms, flip angle = 90⋅, field of view (FOV) = 200*200 mm2. The 3D T1-weighted magnetization-prepared rapid gradient echo (MPRAGE) image was acquired with the following parameters: 128 sagittal slices, slice thickness/gap = 1.33/0 mm, matrix = 256*256, TR = 2530 ms, TE = 3.39 ms, inversion time (Ti) = 1100 ms, flip angle = 7⋅, FOV = 256*256 mm2.
Data Processing Assistant for Resting-State fMRI (DPARSF, http://rfmri.org/DPARSF; Yan and Zang, 2010) was used to preprocess the RS-fMRI data. Steps included: (1) discarding the first 10 volumes; (2) correcting for within-scan acquisition time differences between slices and head motions; (3) coregistering the T1 image to the mean functional image using a linear transformation; (4) segmenting the coregistered T1 images into gray matter, white matter and cerebrospinal fluid; (5) normalizing the head motion corrected functional images to standard template using the transformation matrix estimated from T1 segmentation and reslicing them to 3 mm isotropic resolution; (6) linear detrending and temporal band-pass filtering (0.01~0.08 Hz) to reduce the effects of low-frequency drift and high-frequency physiological noise; and (7) regressing out the nuisance signals including the six head motion profiles, global mean signal, cerebrospinal fluid signal, and white matter signal. ReHo was calculated from these preprocessed images using Kendall coefficient of concordance (KCC; Kendall and Gibbons, 1990) based on 27 nearest neighboring voxels. Then the ReHo maps were spatially smoothed (FWHM = 6 mm) and standardized by dividing the maps by the mean ReHo of the entire brain (Zang et al., 2004).
ANCOVA was used to examine the effects of COMT and BDNF on WM performance with gender, age, and IQ as covariates. With the larger (N = 417) sample with genetic and WM data, we ran a COMT(3 groups) * BDNF(3 groups) ANCOVA.
Genetic effects on resting brain state were analyzed using SPM8 (http://www.fil.ion.ucl.ac.uk/spm) implemented in Matlab 7.8 (The Mathworks, Massachusetts, US) for Windows. Considering the smaller sample size with imaging data, we followed previous research (Li et al.'s, 2016) and combined M/V with M/M to form the Met-carrier group. Subjects were divided into four groups, ordered from high to low in presumed dopamine signaling (see Li et al.'s, 2016): COMT-M+/BDNF-VV, COMT-M+/BDNF-M+, COMT-VV/BDNF-VV, and COMT-VV/BDNF-M+. The design matrix was specified with four regressors defined as “1” (subject in the group) or “0” (subject not in the group). A random effects general linear model was fitted onto ReHo maps with age, gender, and IQ as covariates. Genetic main and interaction effects maps were obtained by setting up contrasts, and Region of Interest (ROI) analysis was further conducted to explore interactions by defining the significant clusters located in the frontal lobe as ROIs and averaging the ReHo in each ROI for each subject. We focused on the frontal lobe for two main reasons. First, the frontal lobe has been consistently found to be a vital brain area correlated with WM in lesion patients and healthy populations (Owen et al., 1990; Curtis and D'Esposito, 2003; Barbey et al., 2013). Most neuroimaging studies have targeted the frontal cortex to investigate the neural basis of N-back WM (Brunoni and Vanderhasselt, 2014). Second, despite the widely distributed expression of COMT and BDNF gene in the brain, studies have found that WM was associated with dopamine concentration in the PFC (Lachman et al., 1996b; Akil et al., 2003; Chen et al., 2004) and was affected by COMT and BDNF levels in the frontal cortex (Bimonte et al., 2003). Then WM was correlated with the averaged ReHo in each ROI. We also regressed WM to whole-brain ReHo to confirm the relationship between ReHo and WM. Furthermore, as the global brain signal regression (GSReg) may lead to artificial results (Gotts et al., 2013; Saad et al., 2013), we reanalyzed our data without GSReg to confirm the stability of the findings.
For this study, the significance threshold was set at 0.05 for the omnibus F-test in ANCOVA. When the omnibus F-value was significant, it was followed by LDS post-hoc test, p < 0.05. The statistical results of the whole-brain analysis of fMRI data were corrected for multiple comparisons using the “AlphaSim” implemented in REST (http://www.restfmri.net; Song et al., 2011). This function is based on the Monte Carlo simulation in AFNI (https://afni.nimh.nih.gov/pub/dist/doc/manual/AlphaSim.pdf). Voxels with p < 0.01 and cluster Size > 459 mm3 (17 voxels) were considered significant, which corresponds to corrected p < 0.05.
The mean IQ of the sample was 125.92 for the 417 sample and 125.94 for the 298 sample.
For the COMT gene, 237 subjects were V/V, 157 were V/M, and 23 were M/M. The sample was in Hardy–Weinberg Equilibrium (HWE, χ2 = 0.11, p > 0.05). The Met minor allele frequency in our sample was 0.24, which is comparable to the HapMap data for Han Chinese (0.29 for Han Chinese in Beijing and 0.26 for Chinese in Metropolitan, Denver, Colorado, according to the HapMap Genome Browser release #28).
For the BDNF gene, the genotype frequencies (113 V/V, 201 V/M, and 103 M/M) were also in HWE (χ2 = 0.31, p > 0.05). The frequency of the BDNF Met allele (0.49) in our sample is similar to other Asian data, 0.41 in a Japanese sample (Shimizu et al., 2004), 0.47 in a Chinese Han population (Yi et al., 2011), and 0.47 in a Korean sample (Kim et al., 2007).
The distribution of genotypes did not vary significantly by gender, age, and IQ for either the total 417 sample or the 298 subsample (Table 1). They were nevertheless used as covariates in the main analyses.
For the whole sample (N = 417), the mean accuracy on the WM task was 0.86 (SD = 0.06). Males and females had comparable mean accuracy, 0.85 (SD = 0.06) and 0.86 (SD = 0.06), respectively, F(1, 415) = 1.27, p = 0.26.
A 3 × 3 genotypes ANCOVA demonstrated that both genes had significant main effects on WM [COMT: F(2, 405) = 5.92, p = 0.003; BDNF: F(2, 405) = 3.09, p = 0.046], and the interaction effect also was significant [F(4, 405) = 2.62, p = 0.034] in the whole sample, N = 417 (see Figure 1A). Post-hoc analysis of the COMT main effect showed that Val homozygotes had higher WM (M = 0.867) than the heterozygotes (M = 0.851), p = 0.033, but neither group differed from the Met homozygotes (M = 0.845) (see Figure 1B), partly due to the latter group's small sample size. Post-hoc analyses of the BDNF main effect did not reveal significant contrasts (see Figure 1C). Finally, the interaction effect of the two genes was due to significant simple effects of COMT for the two groups of individuals who were homozygous for BDNF (i.e., V/V and M/M, p = 0.029 and 0.016, respectively), but not for the BDNF-VM individuals. After combining the VM and MM, the COMT-VV was still higher than COMT-M+ for BDNF-VV [F(1, 410) = 8.42, p = 0.004; see Figure S1].
Figure 1. COMT and BDNF gene effect on WM performance in the 417 sample. (A) The interaction effect of COMT and BDNF (post-hoc LSD test showed that COMT-VV was higher than COMT-MM for both BDNF-VV and BDNF-MM groups, p < 0.05); (B) The main effect of COMT (post-hoc LSD test showed that COMT-VV was higher than COMT-VM, p < 0.05); (C) The main effect of BDNF (post-hoc LSD test showed no significant contrasts).
The main effects of the COMT gene on ReHo were significant in several regions. COMT Val homozygotes had significant higher ReHo than the COMT Met carriers in the bilateral cerebellum anterior lobe, left cerebellum posterior lobe, left putamen, right hippocampus, fusiform gyrus, and middle occipital gyrus, but lower ReHo in right superior and inferior frontal gyrus, left middle frontal gyrus, bilateral superior temporal gyrus, right postcentral gyrus, bilateral precentral gyrus (Figures 2A,C and Table S1). In terms of the main effects of the BDNF gene, Val homozygotes had significant higher ReHo than the BDNF Met carriers in the bilateral lingual gyrus, left limbic lobe, right precentral and postcentral gyrus, right precuneus, right superior frontal gyrus, but lower ReHo in left middle and inferior temporal gyrus, right superior and medial frontal gyrus and left inferior frontal gyrus, bilateral parahippocampal gyrus, left paracentral lobule, and bilateral cerebellum posterior lobe (Figures 2B,D and Table S2).
Figure 2. Main effects of COMT (A,C) and BDNF (B,D) on ReHo (N = 298). The coordinates of the cross in (A) and (B) are [−36, 45, −3] and [−12, −66, −3], respectively. Positive t-values mean that the Val homozygotes showed higher ReHo than the Met carriers and negative t values mean that the Val homozygotes showed lower ReHo than the Met carriers.
Significant interactions between COMT and BDNF were found in left medial frontal gyrus (lMeFG), left superior frontal gyrus (lSFG), right superior and medial frontal gyrus (rSMFG), right medial orbitofrontal gyrus (rMOFG), right middle frontal gyrus (rMFG), precuneus, bilateral superior temporal gyrus, left superior occipital gyrus, right middle occipital gyrus, and right inferior parietal lobule (Tables 2, 3 and Figure 3).
Table 2. Brain areas showing significant COMT and BDNF gene interaction effects on ReHo with age, gender, and IQ as covariates, with AlphaSim correction (p < 0.01; N = 298).
Table 3. Main and interactive effects of COMT and BDNF on mean ReHo in five significant ROIs located in frontal lobe (N = 298).
Figure 3. Interaction effects of COMT and BDNF on ReHo of the whole brain (N = 298). The coordinates of the cross are [6, 48, −7]. Circles indicate the five regions of interest (ROIs) located in the frontal cortex: ROI-1, right medial frontal gyrus (rMeFG); ROI-2, left superior frontal gyrus (lSFG); ROI-3, right superior and medial frontal gyrus (rSMFG); ROI-4, right medial orbitofrontal gyrus (rMOFG); and ROI-5, right middle frontal gyrus (rMFG).
To plot the interaction effects, we focused on the five clusters located in the frontal lobe. The mean ReHo for each ROI was extracted for each subject. Simple effects analyses showed that all five ROIs consistently showed a U-shaped pattern across the four groups arranged along the presumed strength of dopamine signaling. COMT-M+/BDNF-VV and COMT-VV/BDNF-M+ had significantly higher ReHo than COMT-M+/BDNF-M+ and COMT-VV/BDNF-VV; Figure 4).
Figure 4. Effects of COMT and BDNF on mean ReHo of each ROI (N = 298). (A) right medial frontal gyrus (rMeFG); (B) left superior frontal gyrus (lSFG); (C) right superior and medial frontal gyrus (rSMFG); (D) right medial orbitofrontal gyrus (rMOFG); (E) right middle frontal gyrus (rMFG). For all five regions of interest (ROIs), each of the middle two groups (i.e., with presumed medium level of dopamine signaling) showed significantly lower ReHo than each of the other two groups (i.e., low or high level of dopamine signaling; p < 0.05). Details on additional significant contrasts are available from the authors.
Finally, we correlated the average ReHo of each of the five ROIs with WM performance. Results showed that ReHo of rSMFG had a modest negative correlation with WM performance (r = −0.122, p = 0.036). Moreover, the regression analysis with whole-brain images showed that the ReHo of rSMFG showed a negative correlation with WM performance (see Figure 5 and Table 4). The results of the reanalysis without GSReg showed that, although the number of clusters showing significant COMT and BDNF gene effect on ReHo decreased from 26 to 19, the rSMFG was still included (Table S3) and showed the same pattern of results as with GSReg (Figure S2). Furthermore, we regressed WM to whole-brain ReHo and confirmed the negative relationship between ReHo and WM (Table S4).
Figure 5. Brain areas showing positive (red) or negative (blue) correlations with WM, based on whole brain ReHo analysis, with AlphaSim correction (p < 0.01; N = 298). The coordinates of the cross are [22, 50, 7].
Table 4. Brain areas showing significant correlations between WM and ReHo throughout the whole brain, with AlphaSim correction (p < 0.01; N = 298).
The present study was designed to examine COMT and BDNF gene interactions on WM and resting state fMRI (i.e., ReHo). As hypothesized, the COMT and BDNF had main and interaction effects on WM. The positive effect of the COMT Val allele on cognitive abilities is consistent with previous research with Asian samples (Qian et al., 2009; Yeh et al., 2009; Wang et al., 2016), but opposite of previous findings from Western samples that COMT-Met carriers showed better cognitive performance in both patients (Bilder et al., 2002; Joober et al., 2002) and healthy individuals (Caldú et al., 2007; Sheldrick et al., 2008). The main effect of BDNF on WM was modest, yielding no significant post hoc contrasts. Indeed, previous research has reported mixed findings, with some studies linking the Val allele to better performance on learning (Soliman et al., 2010), episodic memory (Egan et al., 2003), and WM tasks (Echeverria et al., 2005; Rybakowski et al., 2006), whereas others showed conflicting findings (Hansell et al., 2007; Schofield et al., 2009). Some of these conflicting results may well have been due to potential interactions between BDNF and COMT as found in our study on WM and ReHo, as well as in previous studies on paired-associates learning, stimulation-induced cortical plasticity (Witte et al., 2012), gray matter volumes (Mortby et al., 2014), and functional connectivity (Wang et al., 2015; Li et al.'s, 2016).
Most relevant to our work, Li et al.'s (2016) found that COMT-M+/BDNF-M+ and COMT-VV/BDNF-VV groups showed higher global functional connectivity density than the other two groups (COMT-VV/BDNF-M+ and COMT-M+/BDNF-VV). These results have been explained by interactive effects of BDNF and COMT on dopamine levels. Both COMT and BDNF influence the cytoarchitecture and connectivity among the subregions. Specifically, BDNF can affect the axonal and dendritic plasticity of neurons and their natural death (Ghosh et al., 1994), whereas COMT modulates the level of dopamine in the PFC (Okazawa et al., 1992; Nishio et al., 1998; Venero et al., 2000). In terms of their interaction, BDNF can stimulate dopamine activity (Blöchl and Sirrenberg, 1996; Murer et al., 2001). Previous biochemical research (Mannisto and Kaakkola, 1999; Winterer and Weinberger, 2004; Savitz et al., 2006; Peciña et al., 2014) showed that the COMT Met allele and the BDNF Val allele were linked to higher dopamine levels than their respective counterparts. Consequently the combination of COMT-M+ and BDNF-VV is expected to lead to a high level of dopamine, and the combination of COMT-VV and BDNF-M+ a low level of dopamine. In contrast, the other two combinations (COMT-VV and BDNF-VV; COMT-M+ and BDNF-M+) are expected to show moderate levels of dopamine. Li et al. (2016) finding that the latter two groups (with presumed medium levels of dopamine signaling) showed higher global functional connectivity density than the other two groups (either low or high levels of dopamine signaling) fits the commonly accepted inverted U-shaped association between dopamine level and outcomes (see Wang et al., 2015; Li et al.'s, 2016 for detailed discussions).
Mapping onto Li et al. (2016) findings, we found consistent U-shaped associations between presumed dopamine level and ReHo of five regions in the frontal lobe. These associations were statistically significant in terms of both interactions and post-hoc contrasts. As an index of a specific voxel's synchronization with its neighbors, ReHo reflects functional specificity of a brain region (Zang et al., 2004). Altered ReHo has been widely considered as an early indicator of brain dysfunction in patients (Wu et al., 2009, 2011; Chen et al., 2012; Cui et al., 2016). For example, a few studies have documented increased ReHo in the right dorsolateral PFC of patients with schizophrenia (Cui et al., 2016) and in the right medial frontal gyrus of patients with Parkinson's disease (Wu et al., 2009). Wu et al. (2009) further showed that when patients with Parkinson's disease were treated with levodopa, their right frontal gyrus showed decreased ReHo. Moreover, lower ReHo in a given region may indicate that it is more likely to be modulated by other regions (Yu et al., 2014), which may enhance the updating function in the WM process (Cools and Robbins, 2004).
Moreover, for the first time, the current study found the COMT and BDNF had interaction effect on ReHo. Moreover, ReHo in one of the regions (rSMFG) had a modest negative correlation with WM, which was confirmed by whole-brain analysis. The rSMFG has been consistently related to WM in previous lesion studies (Jacobsen and Nissen, 1937; Milner, 1963), electrophysiological studies (Fuster and Alexander, 1971; Funahashi et al., 1989, 1993), rTMS studies (Mottaghy et al., 2002), and fMRI studies (D'Esposito et al., 2000; Burgess and Braver, 2010; Marklund and Persson, 2012).
Consistent with the imaging data showing the importance of a moderate level of dopamine, individuals with the combination of COMT-VV and BDNF-VV (a combination expected to produce a medium amount of dopamine) showed top performance on the WM task. Surprisingly, however, the other combination (COMT-MM and BDNF-MM) that is also expected to produce a medium amount of dopamine showed low scores on the WM task. It should be noted, of course, that this group of minor allele homozygotes had the smallest sample size. A subsequent reanalysis of the data with Met carriers showed that COMT-M+/BDNF-M+ did not differ from COMT-M+/BDNF-VV and COMT-VV/BDNF-M+ (see Figure S1). Future research needs to include a larger sample in order to understand the group of COMT-MM and BDNF-MM.
Finally, although our results were generally consistent with other studies of the interaction effects of COMT and BDNF on other brain functions, our results need to be interpreted with caution due to several limitations of the present study. First, as mentioned earlier, our sample included too few COMT minor allele homozygotes to allow for a detailed analysis of the subjects. Second, the correlation between ReHo of rSMFG and WM was modest. It would not have survived a correction for multiple comparisons. Third, we did not collect any WM task-related fMRI data. Fourth, we did not administer a post-scan questionnaire to assess whether subjects followed the instructions not to fall asleep and not to think of anything in particular during scanning. Finally, given the ethnic differences in the effects of COMT on WM, it is imperative to directly compare these effects between Asian and non-Asian populations.
Conceived and designed the experiments: Chuansheng C, QH, GX, WW, QD. Performed the experiments: GX, WW, YH. Analyzed the data: WC, MX. Wrote the paper: WC, Chunhui C, KW, and Chuansheng C.
This work was supported by the National Natural Science Foundation of China (31571150), the 863 program (2015AA020912), the Fundamental Research Funds for the Central Universities (2012LYB05), the 111 Project (B07008) of the Ministry of Education of China. We thank all graduate research assistants who helped with data collection.
The authors declare that the research was conducted in the absence of any commercial or financial relationships that could be construed as a potential conflict of interest.
The reviewer KR and handling Editor declared their shared affiliation, and the handling Editor states that the process nevertheless met the standards of a fair and objective review.
The Supplementary Material for this article can be found online at: http://journal.frontiersin.org/article/10.3389/fnhum.2016.00540/full#supplementary-material
Aguilera, M., Barrantes-Vidal, N., Arias, B., Moya, J., Villa, H., Ibáñez, M. I., et al. (2008). Putative role of the COMT gene polymorphism (Val158Met) on verbal working memory functioning in a healthy population. Am. J. Med. Genet. B Neuropsychiatr. Genet. 147B, 898–902. doi: 10.1002/ajmg.b.30705
Ahmed, A. O., Mantini, A. M., Fridberg, D. J., and Buckley, P. F. (2015). Brain-derived neurotrophic factor (BDNF) and neurocognitive deficits in people with schizophrenia: a meta-analysis. Psychiatry Res. 226, 1–13. doi: 10.1016/j.psychres.2014.12.069
Akil, M., Kolachana, B. S., Rothmond, D. A., Hyde, T. M., Weinberger, D. R., and Kleinman, J. E. (2003). Catechol-O-methyltransferase genotype and dopamine regulation in the human brain. J. Neurosci. 23, 2008–2013.
Baddeley, A. D., and Della Sala, S. (1983). Working memory. Philos. Trans. R. Soc. Lond. B. Biol. Sci. 302, 311–324. doi: 10.1098/rstb.1983.0057
Baddeley, A. D., and Hitch, G. (1974). “Working memory,” in The Psychology of Learning and Motivation, ed G. A. Bower (Cambridge, MA: Academic Press), 47–89.
Barbey, A. K., Koenigs, M., and Grafman, J. (2013). Dorsolateral prefrontal contributions to human working memory. Cortex 49, 1195–1205. doi: 10.1016/j.cortex.2012.05.022
Beck, A. T., and Steer, R. A. (1990). Manual for the Beck Anxiety Inventory. San Antonio, TX: The Psychological Corporation.
Beck, A. T., Steer, R. A., and Brown, G. K. (1996). Beck Depression Inventory. San Antonio, TX: The Psychological Corporation.
Berton, O., McClung, C. A., Dileone, R. J., Krishnan, V., Renthal, W., Russo, S. J., et al. (2006). Essential role of BDNF in the mesolimbic dopamine pathway in social defeat stress. Science 311, 864–868. doi: 10.1126/science.1120972
Bilder, R. M., Volavka, J., Czobor, P., Malhotra, A. K., Kennedy, J. L., Ni, X., et al. (2002). Neurocognitive correlates of the COMT Val(158)Met polymorphism in chronic schizophrenia. Biol. Psychiatry 52, 701–707. doi: 10.1016/S0006-3223(02)01416-6
Bimonte, H. A., Nelson, M. E., and Granholm, A. C. (2003). Age-related deficits as working memory load increases: relationships with growth factors. Neurobiol. Aging 24, 37–48. doi: 10.1016/S0197-4580(02)00015-5
Bimonte-Nelson, H. A., Hunter, C. L., Nelson, M. E., and Granholm, A. C. (2003). Frontal cortex BDNF levels correlate with working memory in an animal model of Down syndrome. Behav. Brain Res. 139, 47–57. doi: 10.1016/S0166-4328(02)00082-7
Blöchl, A., and Sirrenberg, C. (1996). Neurotrophins stimulate the release of dopamine from rat mesencephalic neurons via Trk and p75Lntr receptors. J. Biol. Chem. 271, 21100–21107. doi: 10.1074/jbc.271.35.21100
Bruder, G. E., Keilp, J. G., Xu, H., Shikhman, M., Schori, E., Gorman, J. M., et al. (2005). Catechol-O-methyltransferase (COMT) genotypes and working memory: associations with differing cognitive operations. Biol. Psychiatry 58, 901–907. doi: 10.1016/j.biopsych.2005.05.010
Brunoni, A. R., and Vanderhasselt, M. A. (2014). Working memory improvement with non-invasive brain stimulation of the dorsolateral prefrontal cortex: a systematic review and meta-analysis. Brain Cogn. 86, 1–9. doi: 10.1016/j.bandc.2014.01.008
Burgess, G. C., and Braver, T. S. (2010). Neural mechanisms of interference control in working memory: effects of interference expectancy and fluid intelligence. PLoS ONE 5:e12861. doi: 10.1371/journal.pone.0012861
Caldú, X., Vendrell, P., Bartrés-Faz, D., Clemente, I., Bargalló, N., Jurado, M. A., et al. (2007). Impact of the COMT Val108/158 Met and DAT genotypes on prefrontal function in healthy subjects. Neuroimage 37, 1437–1444. doi: 10.1016/j.neuroimage.2007.06.021
Chen, H. J., Zhu, X. Q., Yang, M., Liu, B., Zhang, Y., Wang, Y., et al. (2012). Changes in the regional homogeneity of resting-state brain activity in minimal hepatic encephalopathy. Neurosci. Lett. 507, 5–9. doi: 10.1016/j.neulet.2011.11.033
Chen, J., Lipska, B. K., Halim, N., Ma, Q. D., Matsumoto, M., Melhem, S., et al. (2004). Functional analysis of genetic variation in catechol-O-methyltransferase (COMT): effects on mRNA, protein, and enzyme activity in postmortem human brain. Am. J. Hum. Genet. 75, 807–821. doi: 10.1086/425589
Christoff, K., and Gabrieli, J. D. E. (2000). The frontopolar cortex and human cognition:evidence for a rostrocaudal hierarchical organization within the human prefrontal cortex. Psychobiology 28, 168–186. doi: 10.3758/BF03331976
Conway, A. R., Kane, M. J., and Engle, R. W. (2003). Working memory capacity and its relation to general intelligence. Trends Cogn. Sci. 7, 547–552. doi: 10.1016/j.tics.2003.10.005
Cools, R., and Robbins, T. W. (2004). Chemistry of the adaptive mind. Philos. Trans. A Math. Phys. Eng. Sci. 362, 2871–2888. doi: 10.1098/rsta.2004.1468
Cui, L. B., Liu, K., Li, C., Wang, L. X., Guo, F., Tian, P., et al. (2016). Putamen-related regional and network functional deficits in first-episode schizophrenia with auditory verbal hallucinations. Schizophr. Res. 173, 13–22. doi: 10.1016/j.schres.2016.02.039
Curtis, C. E., and D'Esposito, M. (2003). Persistent activity in the prefrontal cortex during working memory. Trends Cogn. Sci. 7, 415–423. doi: 10.1016/S1364-6613(03)00197-9
Das, D., Tan, X. Y., Bielak, A. A. M., Cherbuin, N., Easteal, S., and Anstey, K. J. (2014). Cognitive ability, intraindividual variability, and common genetic variants of catechol-O-methyltransferase and brain-derived neurotrophic factor: a longitudinal study in a population-based sample of older adults. Psychol. Aging 29, 393–403. doi: 10.1037/a0035702
D'Esposito, M., Postle, B. R., and Rypma, B. (2000). Prefrontal cortical contributions to working memory: evidence from event-related fMRI studies. Exp. Brain Res. 133, 3–11. doi: 10.1007/s002210000395
Echeverria, D., Woods, J. S., Heyer, N. J., Rohlman, D. S., Farin, F. M., Bittner, A. C. Jr., et al. (2005). Chronic low-level mercury exposure, BDNF polymorphism, and associations with cognitive and motor function. Neurotoxicol. Teratol. 27, 781–796. doi: 10.1016/j.ntt.2005.08.001
Egan, M. F., Goldberg, T. E., Kolachana, B. S., Callicott, J. H., Mazzanti, C. M., Straub, R. E., et al. (2001). Effect of COMT Val108/158 Met genotype on frontal lobe function and risk for schizophrenia. Proc. Natl. Acad. Sci. U.S.A. 98, 6917–6922. doi: 10.1073/pnas.111134598
Egan, M. F., Kojima, M., Callicott, J. H., Goldberg, T. E., Kolachana, B. S., Bertolino, A., et al. (2003). The BDNF val66met polymorphism affects activity-dependent secretion of BDNF and human memory and hippocampal function. Cell 112, 257–269. doi: 10.1016/S0092-8674(03)00035-7
Fry, A. F., and Hale, S. (1996). Processing speed, working memory, and fluid intelligence: evidence for a developmental cascade. Psychol. Sci. 7, 237–241. doi: 10.1111/j.1467-9280.1996.tb00366.x
Funahashi, S., Bruce, C. J., and Goldman-Rakic, P. S. (1989). Mnemonic coding of visual space in the monkey's dorsolateral prefrontal cortex. J. Neurophysiol. 61, 331–349.
Funahashi, S., Bruce, C. J., and Goldman-Rakic, P. S. (1993). Dorsolateral prefrontal lesions and oculomotor delayed-response performance: evidence for mnemonic “scotomas”. J. Neurosci. 13, 1479–1497.
Fuster, J. M., and Alexander, G. E. (1971). Neuron activity related to short-term memory. Science 173, 652–654. doi: 10.1126/science.173.3997.652
Ghisletta, P., Bäckman, L., Bertram, L., Brandmaier, A. M., Gerstorf, D., Liu, T., et al. (2014). The Val/Met polymorphism of the brain-derived neurotrophic factor (BDNF) gene predicts decline in perceptual speed in older adults. Psychol. Aging 29, 384–392. doi: 10.1037/a0035201
Ghosh, A., Carnahan, J., and Greenberg, M. E. (1994). Requirement for BDNF in activity-dependent survival of cortical neurons. Science 263, 1618–1623. doi: 10.1126/science.7907431
Gogos, J. A., Morgan, M., Luine, V., Santha, M., Ogawa, S., Pfaff, D., et al. (1998). Catechol-O-methyltransferase-deficient mice exhibit sexually dimorphic changes in catecholamine levels and behavior. Proc. Natl. Acad. Sci. U.S.A. 95, 9991–9996. doi: 10.1073/pnas.95.17.9991
Gong, P., Zheng, A., Chen, D., Ge, W., Lv, C., Zhang, K., et al. (2009). Effect of BDNF Val66Met polymorphism on digital working memory and spatial localization in a healthy Chinese Han population. J. Mol. Neurosci. 38, 250–256. doi: 10.1007/s12031-009-9205-8
Gothelf, D., Eliez, S., Thompson, T., Hinard, C., Penniman, L., Feinstein, C., et al. (2005). COMT genotype predicts longitudinal cognitive decline and psychosis in 22q11.2 deletion syndrome. Nat. Neurosci. 8, 1500–1502. doi: 10.1038/nn1572
Gotts, S. J., Saad, Z. S., Jo, H. J., Wallace, G. L., Cox, R. W., and Martin, A. (2013). The perils of global signal regression for group comparisons: a case study of Autism Spectrum Disorders. Front. Hum. Neurosci. 7:356. doi: 10.3389/fnhum.2013.00356
Guo, W. B., Sun, X. L., Liu, L., Xu, Q., Wu, R. R., Liu, Z. N., et al. (2011). Disrupted regional homogeneity in treatment-resistant depression: a resting-state fMRI study. Prog. Neuropsychopharmacol. Biol. Psychiatry 35, 1297–1302. doi: 10.1016/j.pnpbp.2011.02.006
Hajek, T., Kopecek, M., and Höschl, C. (2012). Reduced hippocampal volumes in healthy carriers of brain-derived neurotrophic factor Val66Met polymorphism: meta-analysis. World J. Biol. Psychiatry 13, 178–187. doi: 10.3109/15622975.2011.580005
Hampson, M., Driesen, N. R., Skudlarski, P., Gore, J. C., and Constable, R. T. (2006). Brain connectivity related to working memory performance. J. Neurosci. 26, 13338–13343. doi: 10.1523/JNEUROSCI.3408-06.2006
Hansell, N. K., James, M. R., Duffy, D. L., Birley, A. J., Luciano, M., Geffen, G. M., et al. (2007). Effect of the BDNF V166M polymorphism on working memory in healthy adolescents. Genes Brain Behav. 6, 260–268. doi: 10.1111/j.1601-183X.2006.00254.x
Heatherton, T. F., Kozlowski, L. T., Frecker, R. C., and Fagerström, K. O. (1991). The Fagerstrom Test for nicotine dependence - a revision of the fagerstrom tolerance questionnaire. Br. J. Addict. 86, 1119–1127. doi: 10.1111/j.1360-0443.1991.tb01879.x
Ho, B. C., Andreasen, N. C., Dawson, J. D., and Wassink, T. H. (2007). Association between brain-derived neurotrophic factor Val66Met gene polymorphism and progressive brain volume changes in schizophrenia. Am. J. Psychiatry 164, 1890–1899. doi: 10.1176/appi.ajp.2007.05111903
Jacobsen, C. F., and Nissen, H. W. (1937). Studies of cerebral function in primates. IV. The effects of frontal lobe lesions on the delayed alternation habit in monkeys. J. Comp. Psychol. 23, 101–112. doi: 10.1037/h0056632
Joober, R., Gauthier, J., Lal, S., Bloom, D., Lalonde, P., Rouleau, G., et al. (2002). Catechol-O-methyltransferase Val-108/158-Met gene variants associated with performance on the Wisconsin Card Sorting Test. Arch. Gen. Psychiatry 59, 662–663. doi: 10.1001/archpsyc.59.7.662
Kang, J. I., Kim, S. J., Song, Y. Y., Namkoong, K., and An, S. K. (2013). Genetic influence of COMT and BDNF gene polymorphisms on resilience in healthy college students. Neuropsychobiology 68, 174–180. doi: 10.1159/000353257
Khalil, H., Alomari, M. A., Khabour, O. F., Al-Hieshan, A., and Bajwa, J. A. (2016). Relationship of circulatory BDNF with cognitive deficits in people with Parkinson's disease. J. Neurol. Sci. 362, 217–220. doi: 10.1016/j.jns.2016.01.032
Kim, J. M., Stewart, R., Kim, S. W., Yang, S. J., Shin, I. S., Kim, Y. H., et al. (2007). Interactions between life stressors and susceptibility genes (5-HTTLPR and BDNF) on depression in Korean elders. Biol. Psychiatry 62, 423–428. doi: 10.1016/j.biopsych.2006.11.020
Lachman, H. M., Morrow, B., Shprintzen, R., Veit, S., Parsia, S. S., Faedda, G., et al. (1996a). Association of codon 108/158 catechol-O-methyltransferase gene polymorphism with the psychiatric manifestations of velo-cardio-facial syndrome. Am. J. Med. Genet. 67, 468–472.
Lachman, H. M., Papolos, D. F., Saito, T., Yu, Y. M., Szumlanski, C. L., and Weinshilboum, R. M. (1996b). Human catechol-O-methyltransferase pharmacogenetics: description of a functional polymorphism and its potential application to neuropsychiatric disorders. Pharmacogenetics 6, 243–250. doi: 10.1097/00008571-199606000-00007
Leckie, R. L., Oberlin, L. E., Voss, M. W., Prakash, R. S., Szabo-Reed, A., Chaddock-Heyman, L., et al. (2014). BDNF mediates improvements in executive function following a 1-year exercise intervention. Front. Hum. Neurosci. 8:985. doi: 10.3389/fnhum.2014.00985
Li, B., Arime, Y., Hall, F. S., Uhl, G. R., and Sora, I. (2010). Impaired spatial working memory and decreased frontal cortex BDNF protein level in dopamine transporter knockout mice. Eur. J. Pharmacol. 628, 104–107. doi: 10.1016/j.ejphar.2009.11.036
Li, J., Chen, C., He, Q., Li, H., Li, J., Moyzis, R. K., et al. (2011). Neurotensin receptor 1 gene (NTSR1) polymorphism is associated with working memory. PLoS ONE 6:e17365. doi: 10.1371/journal.pone.0017365
Li, W., Liu, B., Xu, J., Jiang, T., and Yu, C. (2016). Interaction of COMT rs4680 and BDNF rs6265 polymorphisms on functional connectivity density of the left frontal eye field in healthy young adults. Hum. Brain Mapp. 37, 2468–2478. doi: 10.1002/hbm.23187
Malhotra, A. K., Kestler, L. J., Mazzanti, C., Bates, J. A., Goldberg, T., and Goldman, D. (2002). A functional polymorphism in the COMT gene and performance on a test of prefrontal cognition. Am. J. Psychiatry 159, 652–654. doi: 10.1176/appi.ajp.159.4.652
Männistö, P. T., and Kaakkola, S. (1999). Catechol-O-methyltransferase (COMT): biochemistry, molecular biology, pharmacology, and clinical efficacy of the new selective COMT inhibitors. Pharmacol. Rev. 51, 593–628.
Marklund, P., and Persson, J. (2012). Context-dependent switching between proactive and reactive working memory control mechanisms in the right inferior frontal gyrus. Neuroimage 63, 1552–1560. doi: 10.1016/j.neuroimage.2012.08.016
Meyer-Lindenberg, A., Kohn, P. D., Kolachana, B., Kippenhan, S., McInerney-Leo, A., Nussbaum, R., et al. (2005). Midbrain dopamine and prefrontal function in humans: interaction and modulation by COMT genotype. Nat. Neurosci. 8, 594–596. doi: 10.1038/nn1438
Milner, B. (1963). Effects of different brain lesions on card sorting: the role of the frontal lobes. Arch Neurol. 9, 100–110. doi: 10.1001/archneur.1963.00460070100010
Mortby, M. E., Das, D., Anstey, K. J., Janke, A., Easteal, S. E., Sachdev, P. S., et al. (2014). “COMT and BDNF gene interactions predict brain structure in ageing,” in Paper Presented at the Alzheimer's Association International Conference, Vol. 10 (Copenhagen: Elsevier Inc.), 95.
Mottaghy, F. M., Döring, T., Müller-Gartner, H. W., Töpper, R., and Krause, B. J. (2002). Bilateral parieto-frontal network for verbal working memory: an interference approach using repetitive transcranial magnetic stimulation (rTMS). Eur. J. Neurosci. 16, 1627–1632. doi: 10.1046/j.1460-9568.2002.02209.x
Murer, M. G., Yan, Q., and Raisman-Vozari, R. (2001). Brain-derived neurotrophic factor in the control human brain, and in Alzheimer's disease and Parkinson's disease. Prog. Neurobiol. 63, 71–124. doi: 10.1016/S0301-0082(00)00014-9
Nagel, I. E., Chicherio, C., Li, S. C., von Oertzen, T., Sander, T., Villringer, A., et al. (2008). Human aging magnifies genetic effects on executive functioning and working memory. Front. Hum. Neurosci. 2:1. doi: 10.3389/neuro.09.001.2008
Nishio, T., Furukawa, S., Akiguchi, I., and Sunohara, N. (1998). Medial nigral dopamine neurons have rich neurotrophin support in humans. Neuroreport 9, 2847–2851. doi: 10.1097/00001756-199808240-00030
Nolan, K. A., Bilder, R. M., Lachman, H. M., and Volavka, J. (2004). Catechol O-methyltransferase Val158Met polymorphism in schizophrenia: differential effects of Val and Met alleles on cognitive stability and flexibility. Am. J. Psychiatry 161, 359–361. doi: 10.1176/appi.ajp.161.2.359
Numata, S., Ueno, S., Iga, J., Yamauchi, K., Hongwei, S., Kinouchi, S., et al. (2007). Interaction between catechol-O-methyltransferase (COMT) Val108/158Met and brain-derived neurotrophic factor (BDNF) Val66Met polymorphisms in age at onset and clinical symptoms in schizophrenia. J. Neural Transm. 114, 255–259. doi: 10.1007/s00702-006-0543-1
Okazawa, H., Murata, M., Watanabe, M., Kamei, M., and Kanazawa, I. (1992). Dopaminergic stimulation up-regulates the in vivo expression of brain-derived neurotrophic factor (BDNF) in the striatum. FEBS Lett. 313, 138–142. doi: 10.1016/0014-5793(92)81430-T
Owen, A. M., Downes, J. J., Sahakian, B. J., Polkey, C. E., and Robbins, T. W. (1990). Planning and spatial working memory following frontal lobe lesions in man. Neuropsychologia 28, 1021–1034. doi: 10.1016/0028-3932(90)90137-D
Paakki, J. J., Rahko, J., Long, X. Y., Moilanen, I., Tervonen, O., Nikkinen, J., et al. (2010). Alterations in regional homogeneity of resting-state brain activity in autism spectrum disorders. Brain Res. 1321, 169–179. doi: 10.1016/j.brainres.2009.12.081
Papaleo, F., Silverman, J. L., Aney, J., Tian, Q., Barkan, C. L., Chadman, K. K., et al. (2011). Working memory deficits, increased anxiety-like traits, and seizure susceptibility in BDNF overexpressing mice. Learn. Mem. 18, 534–544. doi: 10.1101/lm.2213711
Peciña, M., Martínez-Jauand, M., Love, T., Heffernan, J., Montoya, P., Hodgkinson, C., et al. (2014). Valence-specific effects of BDNF Val66Met polymorphism on dopaminergic stress and reward processing in humans. J. Neurosci. 34, 5874–5881. doi: 10.1523/JNEUROSCI.2152-13.2014
Peng, D. H., Jiang, K. D., Fang, Y. R., Xu, Y. F., Shen, T., Long, X. Y., et al. (2011). Decreased regional homogeneity in major depression as revealed by resting-state functional magnetic resonance imaging. Chin. Med. J. 124, 369–373. doi: 10.3760/cma.j.issn.0366-6999.2011.03.009
Qian, Q. J., Wang, Y. F., Yang, L., Li, J., Guan, L. L., Chen, Y., et al. (2009). [Association study of intelligence of attention deficit hyperactivity disorder children in China]. Beijing Da Xue Xue Bao 41, 285–290.
Qian, Q., Wang, Y., Zhou, R., Li, J., Wang, B., Glatt, S., et al. (2003). Family-based and case-control association studies of catechol-O-methyltransferase in attention deficit hyperactivity disorder suggest genetic sexual dimorphism. Am. J. Med. Genet. B. Neuropsychiatr. Genet. 118B, 103–109. doi: 10.1002/ajmg.b.10064
Rosenheck, R., Leslie, D., Keefe, R., McEvoy, J., Swartz, M., Perkins, D., et al. (2006). Barriers to employment for people with schizophrenia. Am. J. Psychiatry 163, 411–417. doi: 10.1176/appi.ajp.163.3.411
Rybakowski, J. K., Borkowska, A., Skibinska, M., and Hauser, J. (2006). Illness-specific association of val66met BDNF polymorphism with performance on Wisconsin Card Sorting Test in bipolar mood disorder. Mol. Psychiatry 11, 122–124. doi: 10.1038/sj.mp.4001765
Saad, Z. S., Reynolds, R. C., Jo, H. J., Gotts, S. J., Chen, G., Martin, A., et al. (2013). Correcting brain-wide correlation differences in resting-state FMRI. Brain Connect. 3, 339–352. doi: 10.1089/brain.2013.0156
Sapkota, S., Vergote, D., Westaway, D., Jhamandas, J., and Dixon, R. A. (2015). Synergistic associations of catechol-O-methyltransferase and brain-derived neurotrophic factor with executive function in aging are selective and modified by apolipoprotein E. Neurobiol. Aging 36, 249–256. doi: 10.1016/j.neurobiolaging.2014.06.020
Saunders, J. B., Aasland, O. G., Babor, T. F., de la fuente, J. R., and Grant, M. (1993). Development of the alcohol-use disorders identification test (Audit) - WHO collaborative project on early detection of persons with harmful alcohol-consumption–II. Addiction 88, 791–804. doi: 10.1111/j.1360-0443.1993.tb02093.x
Savitz, J., Solms, M., and Ramesar, R. (2006). The molecular genetics of cognition: dopamine, COMT and BDNF. Genes Brain Behav. 5, 311–328. doi: 10.1111/j.1601-183X.2005.00163.x
Schofield, P. R., Williams, L. M., Paul, R. H., Gatt, J. M., Brown, K., Luty, A., et al. (2009). Disturbances in selective information processing associated with the BDNF Val66Met polymorphism: evidence from cognition, the P300 and fronto-hippocampal systems. Biol. Psychol. 80, 176–188. doi: 10.1016/j.biopsycho.2008.09.001
Sheldrick, A. J., Krug, A., Markov, V., Leube, D., Michel, T. M., Zerres, K., et al. (2008). Effect of COMT val158met genotype on cognition and personality. Eur. Psychiatry 23, 385–389. doi: 10.1016/j.eurpsy.2008.05.002
Shimizu, E., Hashimoto, K., and Iyo, M. (2004). Ethnic difference of the BDNF 196G/A (val66met) polymorphism frequencies: the possibility to explain ethnic mental traits. Am. J. Med. Genet. B Neuropsychiatr. Genet. 126B, 122–123. doi: 10.1002/ajmg.b.20118
Soliman, F., Glatt, C. E., Bath, K. G., Levita, L., Jones, R. M., Pattwell, S. S., et al. (2010). A genetic variant BDNF Polymorphism alters extinction learning in both mouse and human. Science 327, 863–866. doi: 10.1126/science.1181886
Song, X. W., Dong, Z. Y., Long, X. Y., Li, S. F., Zuo, X. N., Zhu, C. Z., et al. (2011). REST: a toolkit for resting-state functional magnetic resonance imaging data processing. PLoS ONE 6:e25031. doi: 10.1371/journal.pone.0025031
Stark, C. E., and Squire, L. R. (2001). When zero is not zero: the problem of ambiguous baseline conditions in fMRI. Proc. Natl. Acad. Sci. U.S.A. 98, 12760–12766. doi: 10.1073/pnas.221462998
Su, A. I., Wiltshire, T., Batalov, S., Lapp, H., Ching, K. A., Block, D., et al. (2004). A gene atlas of the mouse and human protein-encoding transcriptomes. Proc. Natl. Acad. Sci. U.S.A. 101, 6062–6067. doi: 10.1073/pnas.0400782101
Takahashi, T., Suzuki, M., Tsunoda, M., Kawamura, Y., Takahashi, N., Tsuneki, H., et al. (2008). Association between the brain-derived neurotrophic factor Val66Met polymorphism and brain morphology in a Japanese sample of schizophrenia and healthy comparisons. Neurosci. Lett. 435, 34–39. doi: 10.1016/j.neulet.2008.02.004
Venero, J. L., Vizuete, M. L., Revuelta, M., Vargas, C., Cano, J., and Machado, A. (2000). Upregulation of BDNF mRNA and trkB mRNA in the nigrostriatal system and in the lesion site following unilateral transection of the medial forebrain bundle. Exp. Neurol. 161, 38–48. doi: 10.1006/exnr.1999.7243
Voelcker-Rehage, C., Jeltsch, A., Godde, B., Becker, S., and Staudinger, U. M. (2015). COMT gene polymorphisms, cognitive performance, and physical fitness in older adults. Psychol. Sport Exerc. 20, 20–28. doi: 10.1016/j.psychsport.2015.04.001
Wang, C., Liu, B., Long, H., Fan, L., Li, J., Zhang, X., et al. (2015). Epistatic interaction of BDNF and COMT on the Frontostriatal System. Neuroscience 298, 380–388. doi: 10.1016/j.neuroscience.2015.04.014
Wang, M., Ma, Y., Yuan, W., Su, K., and Li, M. D. (2016). Meta-analysis of the COMT Val158Met polymorphism in major depressive disorder: effect of ethnicity. J. Neuroimmune Pharmacol. 11, 434–445. doi: 10.1007/s11481-016-9651-3
Wang, S., and Gathercole, S. E. (2013). Working memory deficits in children with reading difficulties: memory span and dual task coordination. J. Exp. Child Psychol. 115, 188–197. doi: 10.1016/j.jecp.2012.11.015
Wang, Y., Li, J., Chen, C., Zhu, B., Moysis, R. K., Lei, X., et al. (2013). COMT rs4680 Met is not always the ‘smart allele’: val allele is associated with better working memory and larger hippocampal volume in healthy Chinese. Genes Brain Behav. 12, 323–329. doi: 10.1111/gbb.12022
Winterer, G., and Weinberger, D. R. (2004). Genes, dopamine and cortical signal-to-noise ratio in schizophrenia. Trends Neurosci. 27, 683–690. doi: 10.1016/j.tins.2004.08.002
Witte, A. V., Kürten, J., Jansen, S., Schirmacher, A., Brand, E., Sommer, J., et al. (2012). Interaction of BDNF and COMT polymorphisms on paired-associative stimulation-induced cortical plasticity. J. Neurosci. 32, 4553–4561. doi: 10.1523/JNEUROSCI.6010-11.2012
Wu, Q. Z., Li, D. M., Kuang, W. H., Zhang, T. J., Lui, S., Huang, X. Q., et al. (2011). Abnormal regional spontaneous neural activity in treatment-refractory depression revealed by resting-state fMRI. Hum. Brain Mapp. 32, 1290–1299. doi: 10.1002/hbm.21108
Wu, T., Long, X., Zang, Y., Wang, L., Hallett, M., Li, K., et al. (2009). Regional homogeneity changes in patients with Parkinson's disease. Hum. Brain Mapp. 30, 1502–1510. doi: 10.1002/hbm.20622
Xue, G., Dong, Q., Jin, Z., and Chen, C. (2004). Mapping of verbal working memory in nonfluent Chinese-English bilinguals with functional MRI. Neuroimage 22, 1–10. doi: 10.1016/j.neuroimage.2004.01.013
Yan, C.-G., and Zang, Y.-F. (2010). DPARSF: a MATLAB toolbox for “pipeline” data analysis of resting-state fMRI. Front. Syst. Neurosci. 4:13. doi: 10.3389/fnsys.2010.00013
Yeh, T. K., Chang, C. Y., Hu, C. Y., Yeh, T. C., and Lin, M. Y. (2009). Association of catechol-O-methyltransferase (COMT) polymorphism and academic achievement in a Chinese cohort. Brain Cogn. 71, 300–305. doi: 10.1016/j.bandc.2009.07.011
Yi, Z., Zhang, C., Wu, Z. G., Hong, W., Li, Z. Z., Fang, Y. R., et al. (2011). Lack of effect of brain derived neurotrophic factor (BDNF) Val66Met polymorphism on early onset schizophrenia in Chinese Han population. Brain Res. 1417, 146–150. doi: 10.1016/j.brainres.2011.08.037
Yu, R., Gollub, R. L., Vangel, M., Kaptchuk, T., Smoller, J. W., and Kong, J. (2014). Placebo Analgesia and reward processing: integrating genetics, personality, and intrinsic brain activity. Hum. Brain Mapp. 35, 4583–4593. doi: 10.1002/hbm.22496
Zang, Y., Jiang, T., Lu, Y., He, Y., and Tian, L. (2004). Regional homogeneity approach to fMRI data analysis. Neuroimage 22, 394–400. doi: 10.1016/j.neuroimage.2003.12.030
Zhang, Z., Liu, Y., Jiang, T., Zhou, B., An, N., Dai, H., et al. (2012). Altered spontaneous activity in Alzheimer's disease and mild cognitive impairment revealed by Regional Homogeneity. Neuroimage 59, 1429–1440. doi: 10.1016/j.neuroimage.2011.08.049
Zhu, B., Chen, C., Loftus, E. F., Lin, C., He, Q., Chen, C., et al. (2010). Individual differences in false memory from misinformation: cognitive factors. Memory 18, 543–555. doi: 10.1080/09658211.2010.487051
Keywords: COMT, BDNF, regional homogeneity, interaction effect, working memory
Citation: Chen W, Chen C, Xia M, Wu K, Chen C, He Q, Xue G, Wang W, He Y and Dong Q (2016) Interaction Effects of BDNF and COMT Genes on Resting-State Brain Activity and Working Memory. Front. Hum. Neurosci. 10:540. doi: 10.3389/fnhum.2016.00540
Received: 08 June 2016; Accepted: 11 October 2016;
Published: 02 November 2016.
Edited by:
Srikantan S. Nagarajan, University of California, San Francisco, USAReviewed by:
Arun Bokde, Trinity College Dublin, IrelandCopyright © 2016 Chen, Chen, Xia, Wu, Chen, He, Xue, Wang, He and Dong. This is an open-access article distributed under the terms of the Creative Commons Attribution License (CC BY). The use, distribution or reproduction in other forums is permitted, provided the original author(s) or licensor are credited and that the original publication in this journal is cited, in accordance with accepted academic practice. No use, distribution or reproduction is permitted which does not comply with these terms.
*Correspondence: Qi Dong, ZG9uZ3FpQGJudS5lZHUuY24=
†These authors have contributed equally to this work.
Disclaimer: All claims expressed in this article are solely those of the authors and do not necessarily represent those of their affiliated organizations, or those of the publisher, the editors and the reviewers. Any product that may be evaluated in this article or claim that may be made by its manufacturer is not guaranteed or endorsed by the publisher.
Research integrity at Frontiers
Learn more about the work of our research integrity team to safeguard the quality of each article we publish.