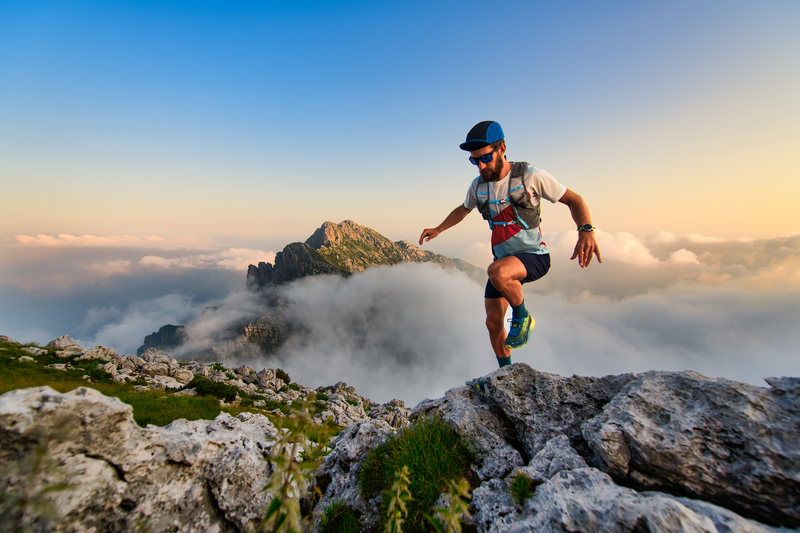
94% of researchers rate our articles as excellent or good
Learn more about the work of our research integrity team to safeguard the quality of each article we publish.
Find out more
GENERAL COMMENTARY article
Front. Hum. Neurosci. , 30 August 2016
Sec. Cognitive Neuroscience
Volume 10 - 2016 | https://doi.org/10.3389/fnhum.2016.00423
This article is a commentary on:
The entropic brain: a theory of conscious states informed by neuroimaging research with psychedelic drugs
A commentary on
The entropic brain: a theory of conscious states informed by neuroimaging research with psychedelic drugs
by Carhart-Harris, R. L., Leech, R., Hellyer, P. J., Shanahan, M., Feilding, A., Tagliazucchi, E., et al. (2014). Front. Hum. Neurosci. 8:20. doi: 10.3389/fnhum.2014.00020
The “entropic brain hypothesis” holds that the quality of conscious states depends on the system's entropy (Carhart-Harris et al., 2014). Brain activity is said to become “more random and so harder to predict in primary states – of which the psychedelic state is an exemplar.” Psychedelic-induced brain activity would be associated with elevated entropy in some of its aspects with respect to normal wakeful consciousness. This would indicate that psychedelic-induced brain activity would exhibit criticality, while normal wakeful consciousness would be subcritical.
But can entropy be a unique indicator of the “quality of consciousness?” Are there reasons to believe that psychedelic-induced activity is not critical?
Complex systems such as the brain are neither completely regular nor completely random. Entropy a measure of randomness, may then seem ill-qualified, per se, to account for brain activity. Indeed to account for the complex blend of regularity and randomness, a wealth of so-called complexity measures have been proposed (Crutchfield, 2012), some specifically for brain activity (Tononi et al., 1994; Tognoli and Kelso, 2014). A single-scale indicator of randomness to describe the consciousness spectrum simply boils down to a complexity measure depurated of its integration or disequilibrium term.
Efforts to characterize brain states, and consciousness in particular, in terms of entropy of the associated dynamics have a 20-year long history. For instance, a significant reduction in EEG entropy (Pezard et al., 1996), and co-variation between entropy and mood improvement were found in depressive patients (Thomasson et al., 2000). Furthermore, entropy monitoring is now a standard method to evaluate the depth of anesthesia (Bein, 2006; Jordan et al., 2008; Olofsen et al., 2008).
A system is metastable when it spends an extended time in a configuration other than the system's least energy state (Kitzbichler et al., 2009; Allegrini et al., 2010; Tognoli and Kelso, 2014). Activity shows a dwell and escape dynamics: Within a metastable state, physical quantities, e.g., energy, fluctuate reversibly around a constant value, while the escape part of the dynamics is associated with shifts from one metastable state to another. Metastability is characterized in terms of dynamical variables, e.g., avalanche size and duration, or inter-burst intervals.
Carhart-Harris and colleagues define metastability as a “measure of the variance in the network's intrinsic synchrony” (p. 10). However such a measure quantifies (the number of) metastable states (Haldeman and Beggs, 2005; Shanahan, 2010), rather than metastability, so that entropy, metastability, and variance turn out to provide essentially the same information. Carhart-Harris' entropy is a configurational entropy, which essentially counts the metastable states of the energy landscape. A genuine configurational entropy establishes a connection between the topography of the energy landscape and the system's dynamics. However, the coarse-graining level must be drastic for one to claim that a few hundred time-points (Carhart-Harris et al., 2012) sufficiently explore the brain's phase space, (how the number of metastable states scales with coarse-graining level is also unknown). Thus, the associated entropy cannot be considered a genuine configurational entropy.
Criticality of the psychedelic state is inferred through the following reasoning: Criticality maximizes the amount of metastable states (Haldeman and Beggs, 2005), and therefore entropy; psychedelic states are associated with more metastable states than normal wakefulness; therefore, the corresponding state is critical, while normal wakefulness is subcritical (Priesemann et al., 2014).
Even from a complexity view-point, some value of the configuration entropy may well correspond to brain activity patterns for which the system is critical. However, there are reasons to doubt that psychedelic-associated activity is critical. (1) Criticality implies (a set of) scaling variables, e.g., avalanche size and duration. Nothing guarantees that the dynamics producing these metastable states in Carhart-Harris et al. (2014) would also generate a dynamics showing scaling as in Haldeman and Beggs (2005). Furthermore, the few hundred timepoints in Carhart-Harris' data (Carhart-Harris et al., 2012) are insufficient to show temporal scaling behavior. (2) While Carhart-Harris' data show that the psychedelic state is associated with more variance than normal wakefulness, other states could in principle be associated with yet more variance than the one associated with the psychedelic state. Furthermore, increasing variance from a subcritical state doesn't guarantee that the system will become critical or that it will have a better power-law fit. (3) Recent evidence shows that, unless parameters are fine-tuned, cortical neural networks dynamics lies in a relatively broad pseudo-critical region, where it is either subcritical or supercritical, a state termed self-organized quasi-criticality (Bonachela and Muñoz, 2010), or extended criticality (Longo et al., 2012), corresponding to Griffiths phases (Moretti and Muñoz, 2013). Accordingly, the “natural world” is presumably not “more critical” than the brain, a notion that would be inconsistent with a recently proposed mechanism of information transfer in complex systems (West et al., 2008; Aquino et al., 2011).
Furthermore, there is an essential contradiction in the suggestion that primary consciousness would be a “psychological atavism” and a “suboptimal mode” and, at the same time, “more critical” than normal states (p. 12). On the one hand, intuitively, the psychedelic state wouldn't appear to be functionally optimal. The hyper-connectivity which characterizes it (Tagliazucchi et al., 2016) is in general associated brain pathology (Whitfield-Gabrieli et al., 2009; Supekar et al., 2013; Hillary et al., 2015). During the psychedelic state the number of forbidden connectivity patterns may decrease, but some of these patterns may be associated with suboptimal (possibly even functionally detrimental) states. On the other hand, however, criticality has been shown to be optimal in terms of transmission and storage of information, computational capabilities, large network stability and sensitivity to sensory stimuli (Shew et al., 2009; Bonachela and Muñoz, 2010). In what sense can the brain be at the same time critical and suboptimal? And if primary consciousness is critical, and therefore in so many ways optimal, what's the evolutionary meaning of secondary states? A possible understanding of criticality and optimality of the psychedelic state is suggested by the results of Zare and Grigolini (2013) suggest. In this study, genuine temporal complexity was found to occur earlier than avalanche-size complexity, in a narrow range where information transfer becomes maximal. Interpreting this information transport enhancement as a signature of criticality, power law avalanches become a manifestation of supercriticality. Accordingly, normal waking and the psychedelic state may respectively lie in the critical and supercritical regimes.
A true configurational entropy requires extensive phase space exploration, otherwise it merely reflects the random side of complexity. Under these conditions, quantifying the number of metastable states is not enough, per se, to assess criticality. Rather than just moving brain activity toward or away from criticality, context-specific demands can modulate the scaling properties of brain fluctuations (Popivanov et al., 2006; Bianco et al., 2007; Buiatti et al., 2007; Bhattacharya, 2009; Zilber et al., 2012), by modifying the scale at which they show scaling or by inducing transitions between different scaling regimes or cross-overs between universality classes (Papo, 2014).
The author confirms being the sole contributor of this work and approved it for publication.
The author declares that the research was conducted in the absence of any commercial or financial relationships that could be construed as a potential conflict of interest.
Allegrini, P., Menicucci, D., Paradisi, P., and Gemignani, A. (2010). Fractal complexity in spontaneous EEG metastable-state transitions: new vistas on integrated neural dynamics. Front. Physiol. 1:128. doi: 10.3389/fphys.2010.00128
Aquino, G., Bologna, M., West, B. J., and Grigolini, P. (2011). Transmission of information between complex systems: 1/f resonance. Phys. Rev. E 83:051130. doi: 10.1103/physreve.83.051130
Bein, B. (2006). Entropy. Best Pract. Res. Clin. Anaesthesiol. 20, 101–109. doi: 10.1016/j.bpa.2005.07.009
Bhattacharya, J. (2009). Increase of universality in human brain during mental imagery from visual perception. PLoS ONE 4:e4121. doi: 10.1371/journal.pone.0004121
Bianco, S., Ignaccolo, M., Rider, M. S., Ross, M. J., Winsor, P., and Grigolini, P. (2007). Brain, music, and non-Poisson renewal processes. Phys. Rev. E 75:061911. doi: 10.1103/physreve.75.061911
Bonachela, J. A., and Muñoz, M. A. (2010). Self-organization without conservation: are neuronal avalanches generically critical? J. Stat. Mech. 2:P02015. doi: 10.1088/1742-5468/2010/02/p02015
Buiatti, M., Papo, D., Baudonnière, P. M., and van Vreeswijk, C. (2007). Feedback modulates the temporal scale-free dynamics of brain electrical activity in a hypothesis testing task. Neuroscience 146, 1400–1412. doi: 10.1016/j.neuroscience.2007.02.048
Carhart-Harris, R. L., Erritzoe, D., Williams, T., Stone, J. M., Reed, L. J., Colasanti, A., et al. (2012). Neural correlates of the psychedelic state as determined by fMRI studies with psilocybin. Proc. Natl. Acad. Sci. U.S.A. 109, 2138–2143. doi: 10.1073/pnas.1119598109
Carhart-Harris, R. L., Leech, R., Hellyer, P. J., Shanahan, M., Feilding, A., Tagliazucchi, E., et al. (2014). The entropic brain: a theory of conscious states informed by neuroimaging research with psychedelic drugs. Front. Hum. Neurosci. 8:20. doi: 10.3389/fnhum.2014.00020
Haldeman, C., and Beggs, J. M. (2005). Critical branching captures activity in living neural networks and maximizes the number of metastable states. Phys. Rev. Lett. 94:058101. doi: 10.1103/PhysRevLett.94.058101
Hillary, F. G., Roman, C. A., Venkatesan, U., Rajtmajer, S. M., Bajo, R., and Castellanos, N. D. (2015). Hyperconnectivity is a fundamental response to neurological disruption. Neuropsychology 29, 59. doi: 10.1037/neu0000110
Jordan, D., Stockmanns, G., Kochs, E. F., Pilge, S., and Schneider, G. (2008). Electroencephalographic order pattern analysis for the separation of consciousness and unconsciousness. An analysis of approximate entropy, permutation entropy, recurrence rate, and phase coupling of order recurrence plots. Anesthesiology 109, 1014–1022. doi: 10.1097/ALN.0b013e31818d6c55
Kitzbichler, M. G., Smith, M. L., Christensen, S. R., and Bullmore, E. (2009). Broadband criticality of human brain network synchronization. PLoS Comput. Biol. 5:e1000314. doi: 10.1371/journal.pcbi.1000314
Longo, G., Montévil, M., and Pocheville, A. (2012). From bottom-up approaches to levels of organization and extended critical transitions. Front. Physiol. 3:232. doi: 10.3389/fphys.2012.00232
Moretti, P., and Muñoz, M. A. (2013). Griffiths phases and the stretching of criticality in brain networks. Nat. Commun. 4, 2521. doi: 10.1038/ncomms3521
Olofsen, E., Sleigh, J. W., and Dahan, E. (2008). Permutation entropy of the electroencephalogram: a measure of anaesthetic drug effect. Br. J. Anaesth. 101, 810–821. doi: 10.1093/bja/aen290
Papo, D. (2014). Functional significance of complex fluctuations in brain activity: from resting state to cognitive neuroscience. Front. Syst. Neurosci. 8:112. doi: 10.3389/fnsys.2014.00112
Pezard, L., Nandrino, J. L., Renault, B., el Massioui, F., Allilaire, J. F., Müller, J., et al. (1996). Depression as a dynamical disease. Biol. Psychiatry 39, 991–999. doi: 10.1016/0006-3223(95)00307-X
Popivanov, D., Stomonyakov, V., Minchev, Z., Jivkova, S., Dojnov, P., Jivkov, S., et al. (2006). Multifractality of decomposed EEG during imaginary and real visual-motor tracking. Biol. Cybern. 94, 149–156. doi: 10.1007/s00422-005-0037-5
Priesemann, V., Wibral, M., Valderrama, M., Pröpper, R., Le Van Quyen, M., Geisel, T., et al. (2014). Spike avalanches in vivo suggest a driven, slightly subcritical brain state. Front. Syst. Neurosci. 8:108. doi: 10.3389/fnsys.2014.00108
Shanahan, M. (2010). Metastable chimera states in community-structured oscillator networks. Chaos 20, 013108. doi: 10.1063/1.3305451
Shew, W. L., Yang, H., Petermann, T., Roy, R., and Plenz, D. (2009). Neuronal avalanches imply maximum dynamic range in cortical networks at criticality. J. Neurosci. 29, 15595–15600. doi: 10.1523/JNEUROSCI.3864-09.2009
Supekar, K., Uddin, L. Q., Khouzam, A., Phillips, J., Gaillard, W. D., Kenworthy, L. E., et al. (2013). Brain hyperconnectivity in children with autism and its links to social deficits. Cell Rep. 5, 738–747. doi: 10.1016/j.celrep.2013.10.001
Tagliazucchi, E., Roseman, L., Kaelen, M., Orban, C., Muthukumaraswamy, S. D., Murphy, K., et al. (2016). Increased global functional connectivity correlates with LSD-induced ego dissolution. Cur. Biol. 26, 1043–1050. doi: 10.1016/j.cub.2016.02.010
Thomasson, N., Pezard, L., Allilaire, J. F., Renault, B., and Martinerie, J. (2000). Nonlinear EEG changes associated with clinical improvement in depressed patients. Nonlinear. Dynam. Psychol. Life Sci. 4, 203–218. doi: 10.1023/A:1009580427443
Tognoli, E., and Kelso, J. A. S. (2014). The metastable brain. Neuron 81, 35–48. doi: 10.1016/j.neuron.2013.12.022
Tononi, G., Sporns, O., and Edelman, G. M. (1994). A measure for brain complexity: relating functional segregation and integration in the nervous system. Proc. Natl. Acad. Sci. U.S.A. 91, 5033–5037. doi: 10.1073/pnas.91.11.5033
West, B. J., Geneston, E. L., and Grigolini, P. (2008). Maximizing information exchange between complex networks. Phys. Rep. 468, 1–99. doi: 10.1016/j.physrep.2008.06.003
Whitfield-Gabrieli, S., Thermenos, H. W., Milanovic, S., Tsuang, M. T., Faraone, S. V., McCarley, R. W., et al. (2009). Hyperactivity and hyperconnectivity of the default network in schizophrenia and in first-degree relatives of persons with schizophrenia. Proc. Natl. Acad. Sci. U.S.A. 106, 1279–1284. doi: 10.1073/pnas.0809141106
Zare, M., and Grigolini, P. (2013). Criticality and avalanches in neural networks. Chaos Solit. Fract. 55, 80–94. doi: 10.1016/j.chaos.2013.05.009
Keywords: metastability, scaling, criticality, entropy, brain activity, temporal complexity
Citation: Papo D (2016) Commentary: The entropic brain: a theory of conscious states informed by neuroimaging research with psychedelic drugs. Front. Hum. Neurosci. 10:423. doi: 10.3389/fnhum.2016.00423
Received: 27 April 2016; Accepted: 09 August 2016;
Published: 30 August 2016.
Edited by:
Michael A. Silver, University of California, Berkeley, USAReviewed by:
Olivia Carter, University of Melbourne, AustraliaCopyright © 2016 Papo. This is an open-access article distributed under the terms of the Creative Commons Attribution License (CC BY). The use, distribution or reproduction in other forums is permitted, provided the original author(s) or licensor are credited and that the original publication in this journal is cited, in accordance with accepted academic practice. No use, distribution or reproduction is permitted which does not comply with these terms.
*Correspondence: David Papo, cGFwb2RhdkBnbWFpbC5jb20=
Disclaimer: All claims expressed in this article are solely those of the authors and do not necessarily represent those of their affiliated organizations, or those of the publisher, the editors and the reviewers. Any product that may be evaluated in this article or claim that may be made by its manufacturer is not guaranteed or endorsed by the publisher.
Research integrity at Frontiers
Learn more about the work of our research integrity team to safeguard the quality of each article we publish.